Keywords
Computer Science and Digital Science
- A3.4. Machine learning and statistics
- A3.4.1. Supervised learning
- A3.4.2. Unsupervised learning
- A3.4.4. Optimization and learning
- A3.4.6. Neural networks
- A3.4.7. Kernel methods
- A3.4.8. Deep learning
- A5.3. Image processing and analysis
- A5.3.2. Sparse modeling and image representation
- A5.3.3. Pattern recognition
- A5.3.4. Registration
- A5.4.3. Content retrieval
- A5.4.4. 3D and spatio-temporal reconstruction
- A5.4.5. Object tracking and motion analysis
- A5.4.6. Object localization
- A5.9. Signal processing
- A5.9.3. Reconstruction, enhancement
- A5.9.5. Sparsity-aware processing
- A5.9.6. Optimization tools
- A6.1. Methods in mathematical modeling
- A6.1.1. Continuous Modeling (PDE, ODE)
- A6.1.2. Stochastic Modeling
- A6.3.1. Inverse problems
Other Research Topics and Application Domains
- B1.1. Biology
- B1.1.3. Developmental biology
- B2.6. Biological and medical imaging
1 Team members, visitors, external collaborators
Research Scientists
- Xavier Descombes [Team leader, Inria, Senior Researcher, HDR]
- Laure Blanc-Féraud [CNRS, Senior Researcher, HDR]
- Luca Calatroni [CNRS, Researcher]
- Eric Debreuve [CNRS, Researcher, HDR]
- Grégoire Malandain [Inria, Senior Researcher, HDR]
- Caroline Medioni [CNRS, Researcher]
Faculty Members
- Florence Besse [CNRS, Associate Professor]
- Fabienne De Graeve [Univ Côte d'Azur, Associate Professor, until Aug 2020]
PhD Students
- Arne Henrik Bechensteen [Univ Côte d'Azur, until Nov 2020]
- Asma Chalabi [Univ Côte d'Azur, From 01/10/2020, Co-supervision (Main supervision: Jérémie Roux, IRCAN, Nice); Localisation: IRCAN, Nice]
- Cedric Dubois [Univ Côte d'Azur, From 01/10/2019]
- Anca-Ioana Grapa [Univ Côte d'Azur, until Aug 2020]
- Sarah Laroui [Univ Côte d'Azur, CIFRE, From 01/08/2018, Industrial partner: Bayer CropScience, Lyon]
- Bastien Laville [Univ Côte d'Azur, from Oct 2020]
- Rim Rahali [National Engineering School of Gabes, Gabes University, Tunisia (visiting Morpheme during 3 months), from Mar 2020 until Jun 2020]
- Clara Sanchez [Univ Côte d'Azur, From 01/10/2019, Co-supervision (Main supervision: Carole Rovère, IPMC, Sophia Antipolis); Localisation: IPMC, Sophia Antipolis]
- Vasiliki Stergiopoulou [CNRS]
- Rudan Xiao [Univ Côte d'Azur]
Technical Staff
- Somia Rahmoun [Inria, Engineer, until Sep 2020]
Interns and Apprentices
- Fatima Zahra El Barche [Inria, from Feb 2020 until Aug 2020]
- Jerome Emonet [Univ Côte d'Azur, from Feb 2020 until Jul 2020]
- Bastien Laville [Univ Côte d'Azur, from Apr 2020 until Sep 2020]
- Marta Lazzaretti [Univ Côte d'Azur, from Mar 2020 until Jul 2020]
- Angie Moullet [Inria, until May 2020]
- Morgane Nadal [Ecole normale supérieure Paris-Saclay, from Feb 2020 until Jul 2020]
- Gabriele Scrivanti [Univ Côte d'Azur, from Jun 2020 until Jul 2020]
- Abed Zaoui [CNRS, from Apr 2020 until Sep 2020]
Administrative Assistant
- Isabelle Strobant [Inria]
Visiting Scientists
- Alin Achim [Bristol University, UK, until Jun 2020]
- Pasquale Cascarano [Università degli studi di Bologna, from Mar 2020 until May 2020 (planned), left in March 2020 due to COVID19 pande, PhD ]
External Collaborators
- Gilles Aubert [Univ Côte d'Azur, HDR]
- Sébastien Schaub [CNRS]
2 Overall objectives
Morpheme is a joint project between INRIA, CNRS and the University of Nice-Sophia Antipolis, involving the Computer Science, Signals and Systems Laboratory (I3S) (UMR 6070) and the Institute for Biology of Valrose (iBV) (CNRS/INSERM).
The scientific objectives of MORPHEME are to characterize and model the development and the morphological properties of biological structures from the cell to the supra-cellular scale. Being at the interface between computational science and biology, we plan to understand the morphological changes that occur during development combining in vivo imaging, image processing and computational modeling.
The morphology and topology of mesoscopic structures, indeed, do have a key influence on the functional behavior of organs. Our goal is to characterize different populations or development conditions based on the shape of cellular and supra-cellular structures, including micro-vascular networks and dendrite/axon networks. Using microscopy or tomography images, we plan to extract quantitative parameters to characterize morphometry over time and in different samples. We will then statistically analyze shapes and complex structures to identify relevant markers and define classification tools. Finally, we will propose models explaining the temporal evolution of the observed samples. With this, we hope to better understand the development of normal tissues, but also characterize at the supra-cellular level different pathologies such as the Fragile X Syndrome, Alzheimer or diabetes.
3 Research program
3.1 Research program
The recent advent of an increasing number of new microscopy techniques giving access to high throughput screenings and micro or nano-metric resolutions provides a means for quantitative imaging of biological structures and phenomena. To conduct quantitative biological studies based on these new data, it is necessary to develop non-standard specific tools. This requires using a multi-disciplinary approach. We need biologists to define experiment protocols and interpret the results, but also physicists to model the sensors, computer scientists to develop algorithms and mathematicians to model the resulting information. These different expertises are combined within the Morpheme team. This generates a fecund frame for exchanging expertise, knowledge, leading to an optimal framework for the different tasks (imaging, image analysis, classification, modeling). We thus aim at providing adapted and robust tools required to describe, explain and model fundamental phenomena underlying the morphogenesis of cellular and supra-cellular biological structures. Combining experimental manipulations, in vivo imaging, image processing and computational modeling, we plan to provide methods for the quantitative analysis of the morphological changes that occur during development. This is of key importance as the morphology and topology of mesoscopic structures govern organ and cell function. Alterations in the genetic programs underlying cellular morphogenesis have been linked to a range of pathologies.
Biological questions we will focus on include:
- what are the parameters and the factors controlling the establishment of ramified structures? (Are they really organize to ensure maximal coverage? How are genetic and physical constraints limiting their morphology?),
- how are newly generated cells incorporated into reorganizing tissues during development? (is the relative position of cells governed by the lineage they belong to?)
Our goal is to characterize different populations or development conditions
based on the shape of cellular and supra-cellular structures, e.g. micro-vascular
networks, dendrite/axon networks, tissues from 2D, 2D+t, 3D or 3D+t images (obtained with confocal microscopy, video-microscopy, photon-microscopy or micro-tomography). We plan to extract shapes or quantitative parameters to characterize the morphometric properties of different samples. On the one hand, we will
propose numerical and biological models explaining the temporal evolution of the
sample, and on the other hand, we will statistically analyze shapes and complex
structures to identify relevant markers for classification purposes. This should
contribute to a better understanding of the development of normal tissues but
also to a characterization at the supra-cellular scale of different pathologies such
as Alzheimer, cancer, diabetes, or the Fragile X Syndrome.
In this multidisciplinary context, several challenges have to be faced. The
expertise of biologists concerning sample generation, as well as optimization of
experimental protocols and imaging conditions, is of course crucial. However,
the imaging protocols optimized for a qualitative analysis may be sub-optimal
for quantitative biology. Second, sample imaging is only a first step, as we need
to extract quantitative information. Achieving quantitative imaging remains an
open issue in biology, and requires close interactions between biologists, computer
scientists and applied mathematicians. On the one hand, experimental and imaging protocols should integrate constraints from the downstream computer-assisted
analysis, yielding to a trade-off between qualitative optimized and quantitative optimized protocols. On the other hand, computer analysis should integrate constraints specific to the biological problem, from acquisition to quantitative information extraction. There is therefore a need of specificity for embedding precise
biological information for a given task. Besides, a level of generality is also desirable for addressing data from different teams acquired with different protocols
and/or sensors.
The mathematical modeling of the physics of the acquisition system will yield
higher performance reconstruction/restoration algorithms in terms of accuracy.
Therefore, physicists and computer scientists have to work together. Quantitative
information extraction also has to deal with both the complexity of the structures of interest (e.g., very dense network, small structure detection in a volume,
multiscale behavior,
-
Imaging: this includes i) definition of the studied populations (experimental
conditions) and preparation of samples, ii) definition of relevant quantitative
characteristics and optimized acquisition protocol (staining, imaging,
) for the specific biological question, and iii) reconstruction/restoration of native data to improve the image readability and interpretation. - Feature extraction: this consists in detecting and delineating the biological structures of interest from images. Embedding biological properties in the algorithms and models is a key issue. Two main challenges are the variability, both in shape and scale, of biological structures and the huge size of data sets. Following features along time will allow to address morphogenesis and structure development.
- Classification/Interpretation: considering a database of images containing different populations, we can infer the parameters associated with a given model on each dataset from which the biological structure under study has been extracted. We plan to define classification schemes for characterizing the different populations based either on the model parameters, or on some specific metric between the extracted structures.
- Modeling: two aspects will be considered. This first one consists in modeling biological phenomena such as axon growing or network topology in different contexts. One main advantage of our team is the possibility to use the image information for calibrating and/or validating the biological models. Calibration induces parameter inference as a main challenge. The second aspect consists in using a prior based on biological properties for extracting relevant information from images. Here again, combining biology and computer science expertise is a key point.
4 Application domains
Among the applications addressed by Morpheme team we can cite:
- Kidney cancer classification from histological images
- IMP-RNA granules detection and classification from confocal image
- Extra-cellular matrix detection and characterization from confocal images
- Axon growth modeling
- Glial cell detection and characterization for the study of high-fat diets
- Death time detection and type classification of cells in microscopy time-lapses
- Morphogenesis and embryogenesis
- Numerical super-resolution techniques
- Interpretation of contrast- and orientation-based visual illusions
- Digital restoration of artworks (illuminated manuscripts)
5 Highlights of the year
5.1 Awards
- Luca Calatroni was awarded the Prix d'Excellence 2020 from Univ. Côte d'Azur for the H2020 RISE NoMADS project.
5.2 Publication
6 New software and platforms
6.1 New software
6.1.1 Obj.MPP
- Keywords: Object detection, Marked Point Process, Parametric model
- Functional Description: Obj.MPP implements the detection of parametric objects using a Marked Point Process (MPP). A parametric object is an n-dimensional piece of signal defined by a finite set of parameters. Detecting an object in a signal amounts to finding a position at which the signal can be described well enough by a specific set of parameters (unknowns of the detection problem). The detection task amounts to finding all such objects. Typically, the signal is a 2-dimensional grayscale image and the parametric objects are bright disks on a dark background. In this case, each object is defined by a single parameter: the disk radius. Note however that the core function of Obj.MPP is not tied to a particular context (2-dimensional imaging is just an example).
-
URL:
https://
team. inria. fr/ morpheme/ obj-mpp-object-detection-using-a-marked-point-process/ - Publications: hal-00793677, hal-01383165, hal-00801448, hal-00616371
- Author: Eric Debreuve
- Contact: Eric Debreuve
6.1.2 ATOLS
- Name: Adaptative Threshold Operator based on Level Sets
- Keywords: Object detection, Level Set
- Functional Description: Atols is a Python script allowing to detect features on images using a contrast scoring. Thus, it's possible to detect features at different levels of intensity unlike a simple threshold which would only keep features above its value.
-
URL:
https://
team. inria. fr/ morpheme/ software/ - Authors: Kevin Giulietti, Guillaume Lavisse
- Contact: Xavier Descombes
6.1.3 Small particle detection
- Keywords: Image processing, Image segmentation, Object detection, Computational biology, Fluorescence microscopy, Biomedical imaging
-
Functional Description:
An algorithm primarily design to detect objects whose sizes aren’t larger a few pixels (particles) on fluorescence microscopy images.
It is an simplified version of marked point process.
-
URL:
https://
gitlab. inria. fr/ ncedilni/ spade - Publications: hal-01867816, hal-01867805
- Contacts: Nicolas Cedilnik, Eric Debreuve, Xavier Descombes
7 New results
7.1 A continuous relaxation of the problem
Participants: Gilles Aubert, Arne Henrik Bechensteen, Laure Blanc-Féraud.
This work focuses on the minimization of the least square loss function under a
This work is published in Journal of Mathematical Imaging and Vision 10.
Keywords: inverse problems,
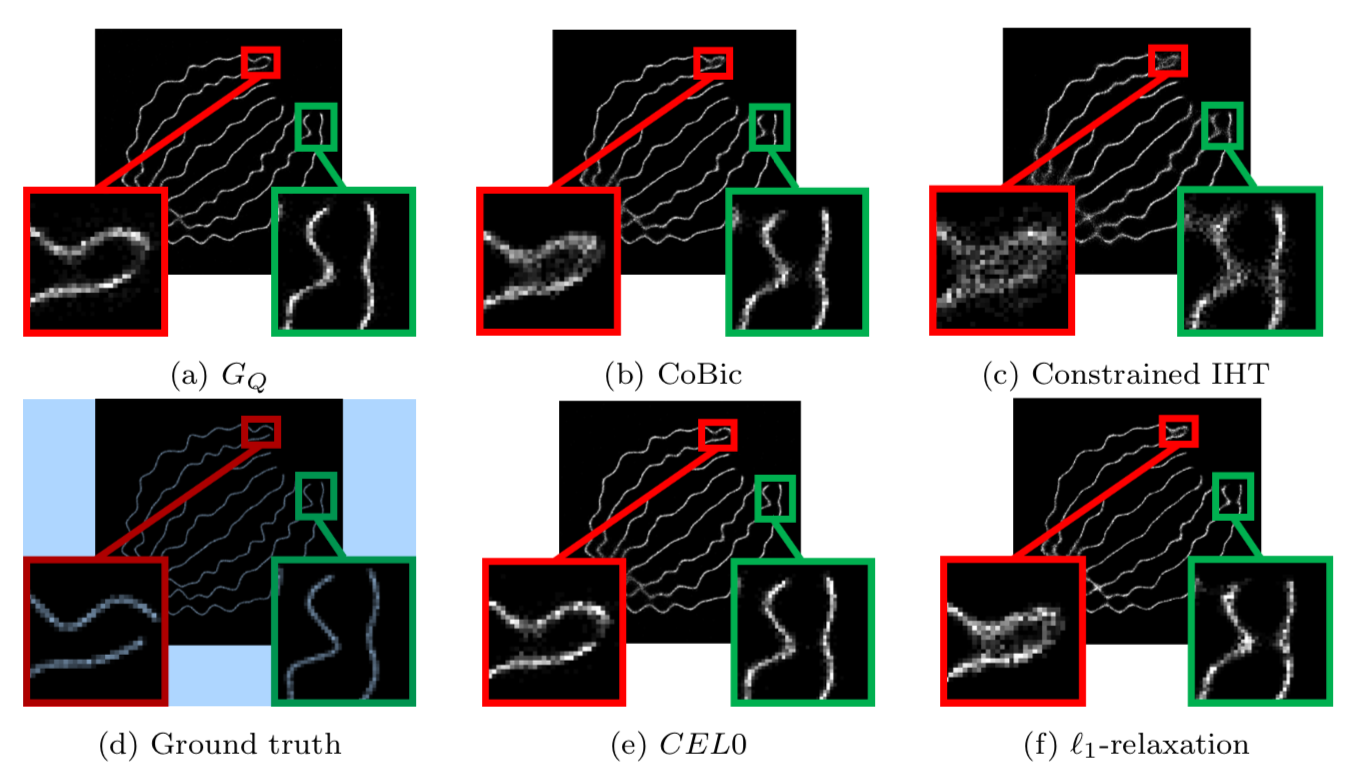
7.2 Molecule localisation in super-resolution microscopy with Poisson data
Participants: Luca Calatroni, Marta Lazzaretti.
This work has been carried out in collaboration with C. Estatico (DIMA, University of Genova).
We propose a continuous non-convex variational model for Single Molecule Localisation Microscopy (SMLM) super-resolution in order to overcome light diffraction barriers. Namely, inspired by previous work 32, 26 we consider a variation of the Continuous Exact
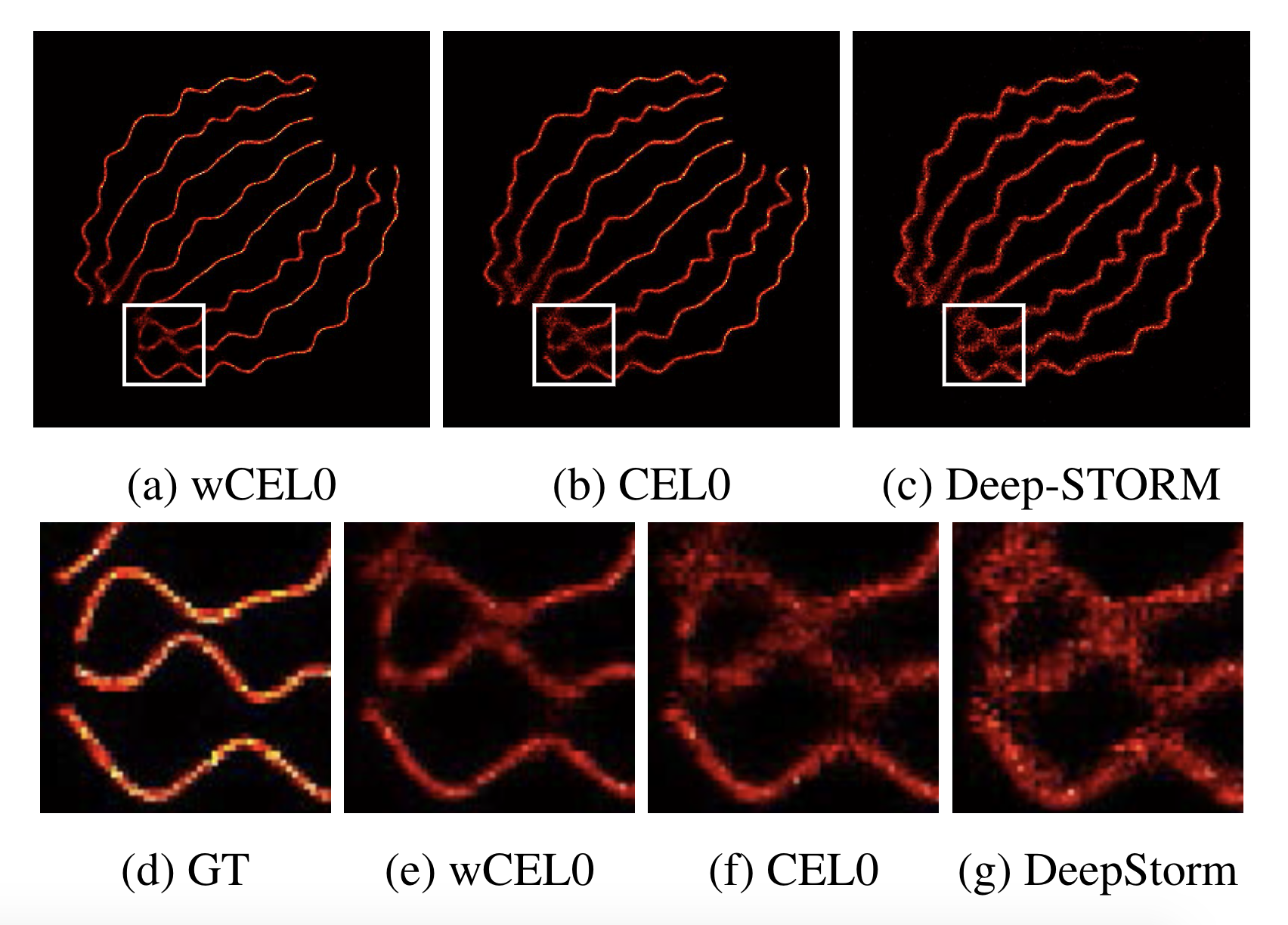
This will appear in the conference proceedings of the IEEE ISBI 2021 conference.
7.3 Covariance-based super-Resolution Microscopy with intensity Estimation
Participants: Luca Calatroni, Laure Blanc-Féraud, Vasiliki Stergiopoulou.
We exploit molecules fluctuations for the design of a super-resolution live-cell imaging approach relying on common microscopes equipment and conventional fluorescent dyes. We call the proposed method COL0RME for COvariance-based
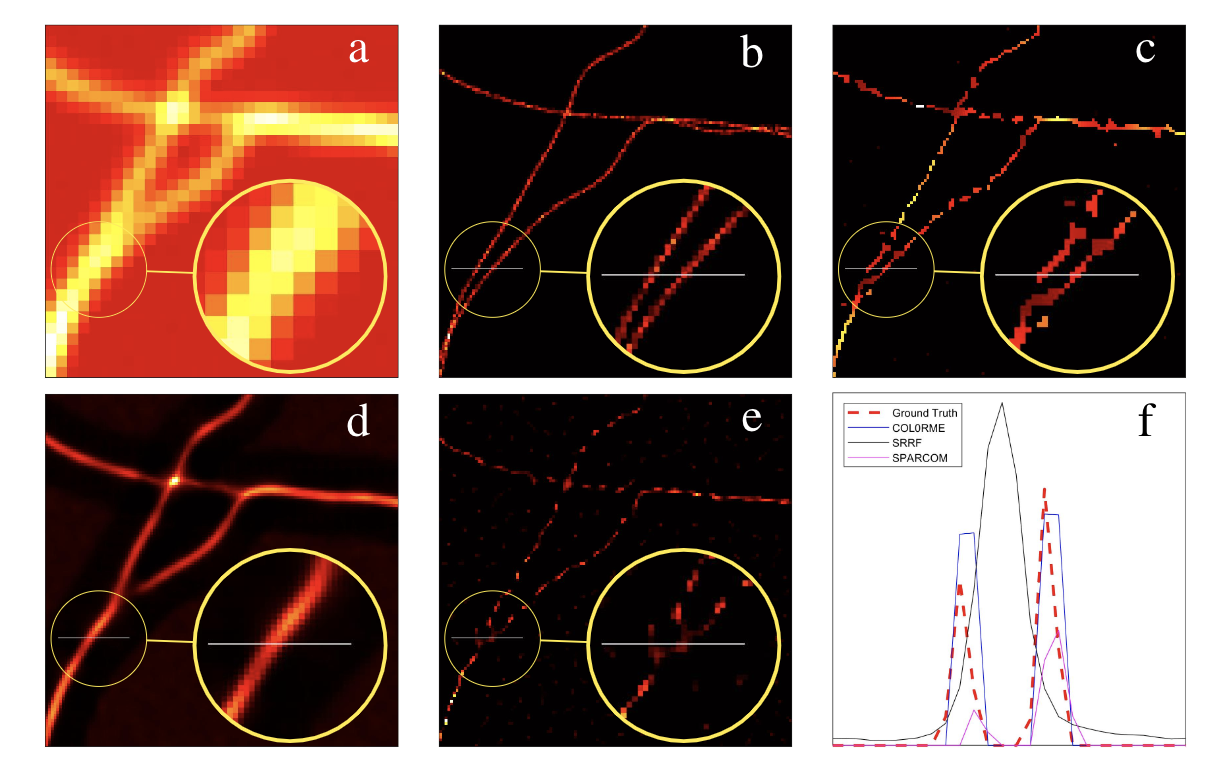
This will appear in the proceedings of the IEEE ISBI 2021 conference.
Vasiliki Stergoipoulou's work is supported by the French Government through the 3IA Côte d’Azur Investments in the Future project managed by the National Research Agency (ANR) with the reference number ANR-19-P3IA-0002.
7.4 Statistical framework for the parametric study of Fibronectin Variants in healthy and disease state
Participants: Anca-Ioana Grapa, Laure Blanc-Féraud, Xavier Descombes.
This work is done in collaboration with Ellen Van Obberghen-Schilling and Georgios Efthymiou (iBV).
We were interested in the numerical analysis and modeling of the Fibronectin (FN) networks, a major extracellular matrix (ECM) molecule, expressed in pathological states (fibrosis, cancer, etc). Since the functions of these variants are not well defined in the context of their role within the tumor microenvironment, we hope that an analytic framework for the study of FN parametric maps can differentiate between the tumoral and the normal-like state for one specific FN variant, and statistically characterize the differences, with respect to fibrillar features.
Fiber networks representation
In previous work, we derived a graph-based representation of FN fibers, built on top of Gabor features (fiber scale, orientation, etc). More specifically, Gabor filters were used to enhance the fibrillar structures, followed by a morphological skeletonization of the maximum response of the Gabor filter set. We then derived the corresponding fiber graph networks.
Statistical analysis of deformation maps
FN graph representation enabled the study of parametric deformation maps (e.g. fiber length deformation maps) in a statistical framework for the comparison of "normal" and "tumour-like" state FN variants. Inspired from the methodologies found in the statistical mapping of the functional images, we performed this analysis relying on two different approaches, the first one based on the theory of Gaussian Random Fields (GRF), and the second one, relying on the computation of empirical distributions, as described in the following paragraphs.
The normal FN deformation maps were first modelled as realizations of the GRF, and thus represented the learning set for deriving the model parameters. We were then able to determine a set of probabilities that characterize a degree of belonging to the Gaussian field, of certain regions from the tumoral deformation maps taken at various thresholds. In other words, the current statistical analysis can identify the foreign regions with respect to the GRF within both types of maps under the null hypothesis, with a view to compare their properties (see Figure 4).
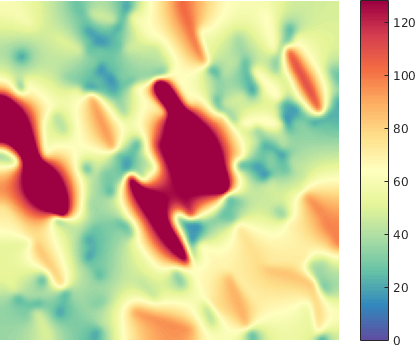
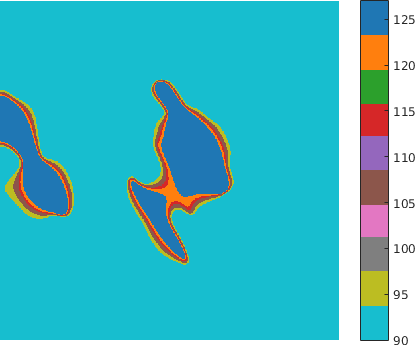
Additionally, we considered deformation maps between pairs of registered (i.e. matched) FN networks, normal vs tumor, (see Figure 5 for a example of a deformation map in terms of fiber length - which can be regarded as a local stretching of the fibers that should be applied to first graph in order to obtain the second one), and applied a similar statistical analysis. The matching (alignment) between the images and the relative analysis are important to mitigate the effect of non-stationarity in different tissues (variability within the tissues).
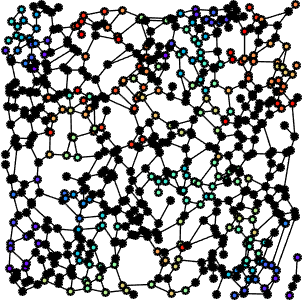
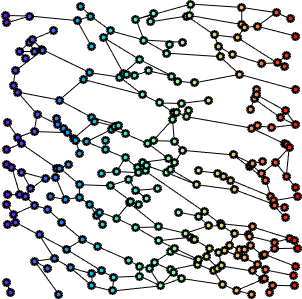
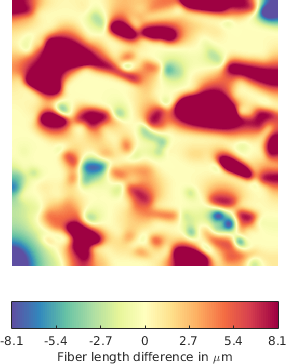
The methodology described above was applied for the quantitative and qualitative comparison of differences with respect to the fiber length for one variant FN B-A+ in both normal and tumoral-like state. Here we illustrate one example of the application under the following conditions (P value p = 0.05):
- Learning dataset : 50 parametric maps (Normal)
- Test set: 70 parametric maps (Tumoral) and 20 parametric maps (Normal)
- Thresholds of intensity T = [95 105 115 120 127]
The results in Table 1 illustrate for every threshold, the average number of identified foreign clusters per image as well as the average cluster area. By comparison, within the normal parametric maps of the test dataset, the method does not identify any foreign cluster when
Thresholds | 95 | 105 | 115 | 120 | 127 |
Max Intensity - Avg nb/im | 0.31 | 0.31 | 0.36 | 0.37 | 0.40 |
Max Intensity - Avg area/im | 6913.33 | 5761.59 | 2777.69 | 2330.17 | 1723.45 |
Surface - Avg nb/im | 0.04 | 0.09 | 0.13 | 0.23 | 0.37 |
Surface - Avg area/im | 4922.07 | 4935.79 | 3019.36 | 2529.45 | 2075.28 |
The statistical analysis shows that the differences with respect to the fiber length, between "normal" and "tumoral" variants are statistically significant.
Future perspectives
The results of the statistical analysis of parametric maps (fiber length) were encouraging with respect to both qualitative and quantitative differences among FN variants in normal and tumor-like cases. A more comprehensive analysis can include the study of larger samples, and the analysis of various fiber parameters.
Anca Grapa's work is supported by the French Government (National Research Agency, ANR) through the ”Investments for the Future” LABEX SIGNALIFE: program reference ANR-11-LABX-0028-01.
7.5 Estimation of the total bio-volume of copepods from 2-dimensional views
Participants: Cédric Dubois, Eric Debreuve.
This work was made in collaboration with Jean-Olivier Irisson (LOV, Villefranche-sur-mer, France).
We proposed a method to correct the total bio-mass of copepods estimated with the Equivalent Sphere Diameter method (ESD) or the Ellipse method (ELL) for individual volume inference from two-dimensional images (see Figure 6). The copepod body is modeled with an ellipsoid, which allows to mathematically derive the projected silhouette resulting from the 3D-to-2D acquisition process (typical of UVPs (Underwater Vision Profiler) or the ZooScan for example). This framework is further developed to generate realistic samples of copepods bodies with random orientations, compute their projected silhouette, and estimate their volume with ESD or ELL. We can then compute a correction factor for the total volume. This factor can be subsequently applied to correct total volume estimations obtained with ESD or ELL on real data. The proposed method was validated on a large database (+
![]() |
![]() |
Top or bottom view of a copepod with the antennas visible | Side view of a copepod with the antennas not visible |
7.6 Particle detection using ObjMPP
Participants: Somia Rahmoun, Eric Debreuve, Xavier Descombes.
This work is made in collaboration with Tamas Matuzek and Pascal Thérond, iBV, within the ANR project HMOVE
We consider confocal microscopy 3D stacks of drosophila wing disks on which we aim to detect Hedgehog granules. We employ the marked point process approach thanks to our software Objmpp. The image intensity is severely heterogeneous and can be approximately split in two areas (see Figure 7). Due to the differences in the resolutions in Z and in the X-Y plane, the granules appear as "smarties shaped" flat objects . We have two channels (GFP and Antibody) corresponding to markers of different cell compartments. The goal is to compare the granule populations in both conditions. In this study we have compared different setting of Objmpp (2D vs 3D, mask or no mask) and applied several pre-processing steps on the central part of the tissue. As a conclusion, the best results were obtained by applying a spatial normalisation and a top hat filter to the initial data. Then the image is split in two parts on which Objmpp is run with two different values of the threshold parameters. The next step will consists in analyzing the robustness of this setting along the whole tissue.
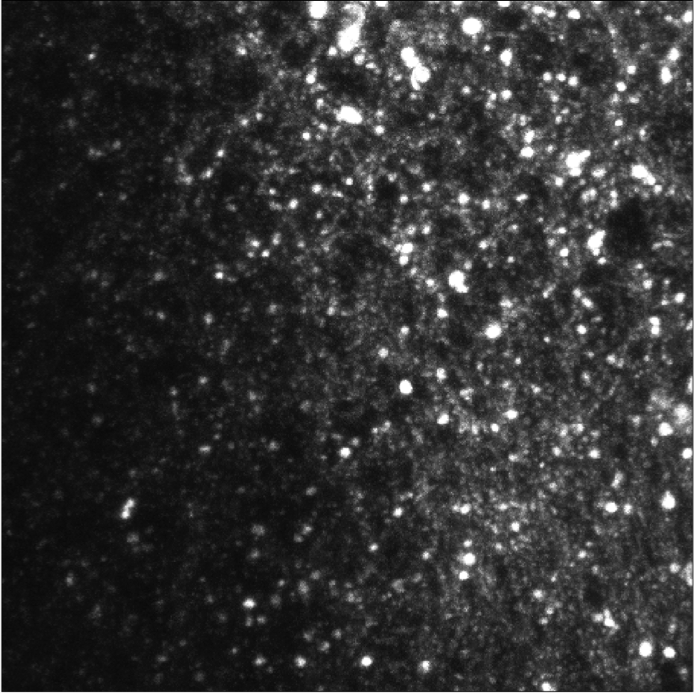
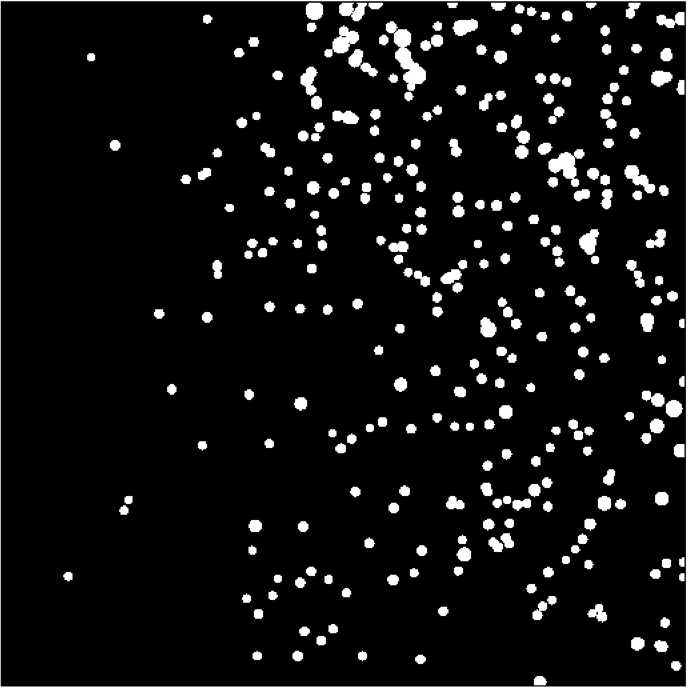
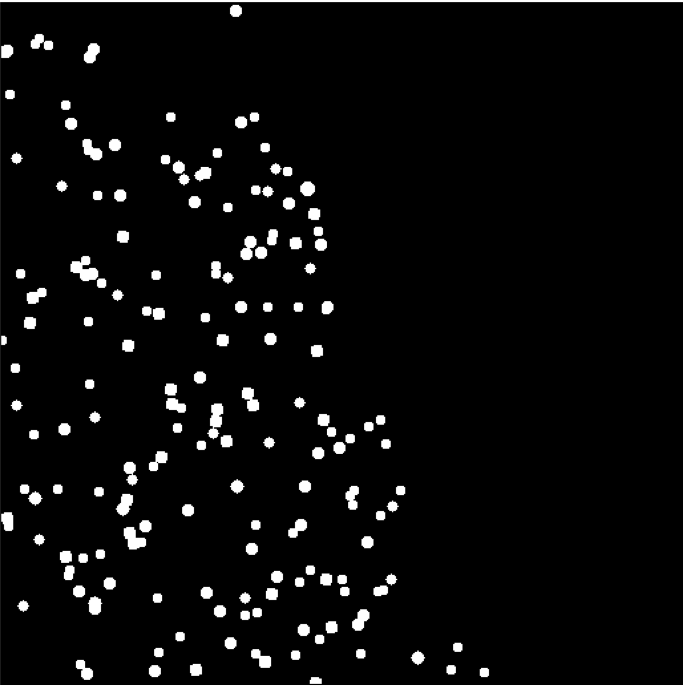
7.7 Organoids segmentation and classification
Participants: Jérôme Emonet, Xavier Descombes.
This work is made in collaboration with Stephan Clavel and Victor Tiroille (C3M)
In this project we consider confocal microscopy images of prostate organoids. Prostate cancer is one of the most prevalent cancer in men. The C3M team has the technology to produce organoid from cells. This allows molecules testing in vitro on biological material issued from a given patient. Organoids are therefore a promising technology for reaching a personalised medicine. We have proposed an algorithm to automatically segment organoids from images. In a first step we estimate a texture parameter defined by the conditional variance associated to a Gaussian Markov random field. We then estimate a geometrical approximation of the objects by fitting ellipse using a marked point process model. The segmentation is then adjusted using a marker controlled watershed (see Figure 8). From the segmented object we computed several geometric parameters such as the size and the circularity as well as an average intensity profile from the center to the object border. Using a k-means algorithm we were able to classify the different objects as debris, compact or cystic organoids. In the next steps we will enrich the phenotypic description of organoids to study the influence of endocrinian disruptor on the organoid growth.
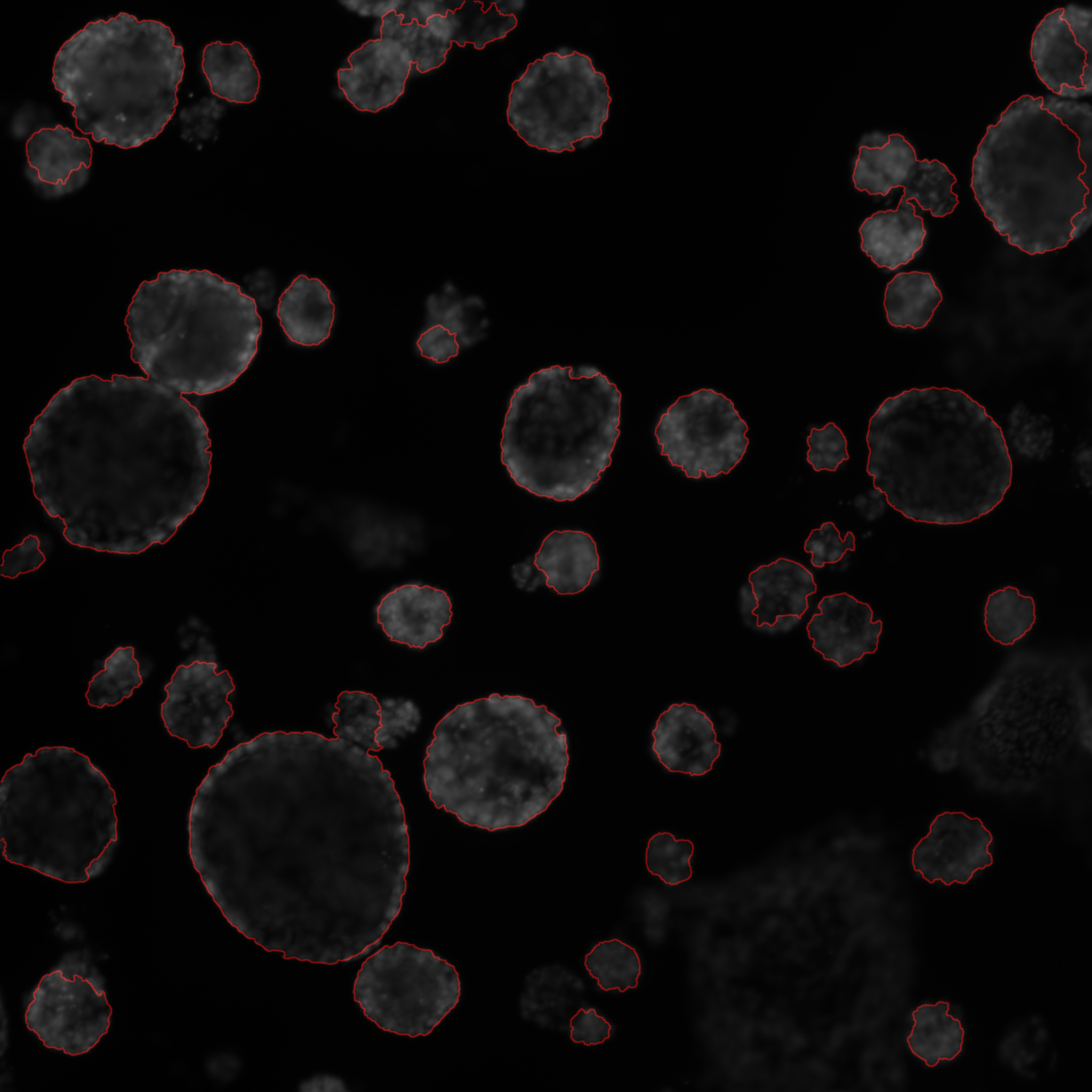
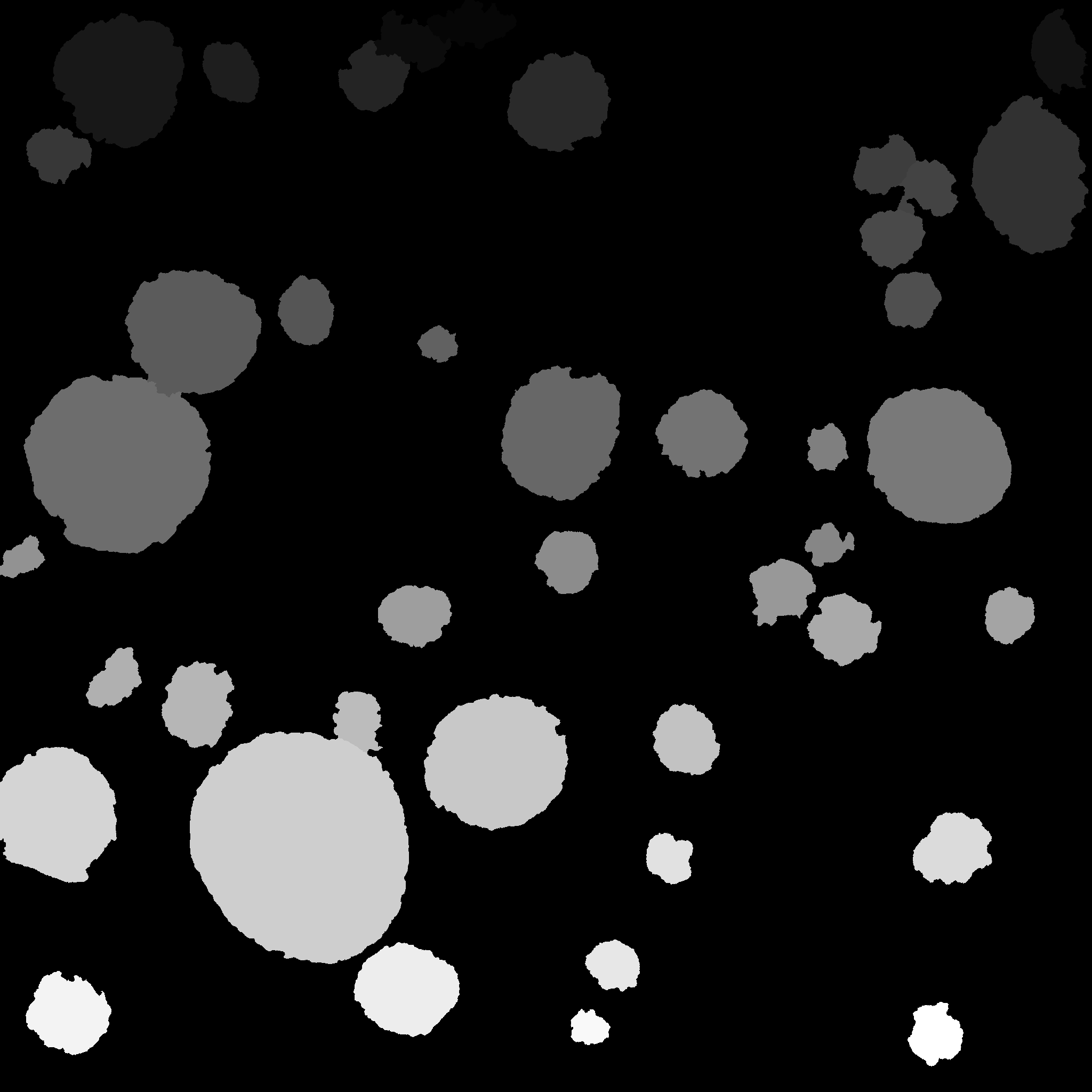
7.8 Uveitis detection from OCT using deep learning
Participants: Fatima Zahra El Barche, Alin Achim, Xavier Descombes.
Uveitis is an auto-immune disease that induces retina inflammation. Early prognostic is important to avoid long term visual deficiency. In this project we consider OCT (Optical Coherence Tomography) images to test the ability of convolutional neural networks (CNN) to detect uveitis from such images, Bristol University has collected a database of mouse eyes images. The database contains wild type retina (WT) and retina affected by Uveitis respectively 2, 7 and 14 days after the disease injection. Each dataset is a 3D volume where only part of the slices may be affected by the disease. We consider the classification on 2D slices after removing those that are not concerned by the disease (see Figure 9). After testing several CNN we have retained the VGC16 architecture to perform a binary classification between wild type and affected mice. We obtained an accuracy of
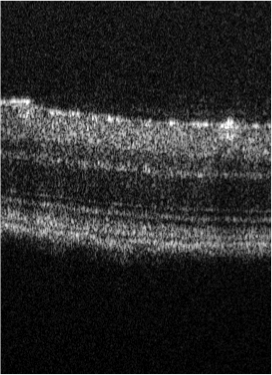
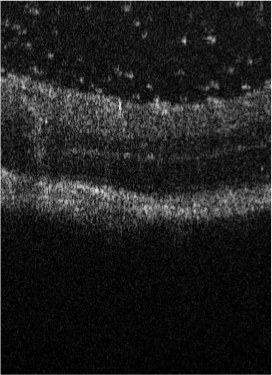
7.9 Non-convex Super-resolution of OCT images via sparse representation
Participants: Luca Calatroni, Gabriele Scrivanti, Alin Achim.
This project has been done in collaboration with S. Morigi (Università di Bologna).
We propose a non-convex variational model for the super-resolution of Optical Coherence Tomography (OCT) images of the murine eye, by enforcing sparsity with respect to suitable dictionaries learned from high-resolution OCT data. The statistical characteristics of OCT images motivate the use of
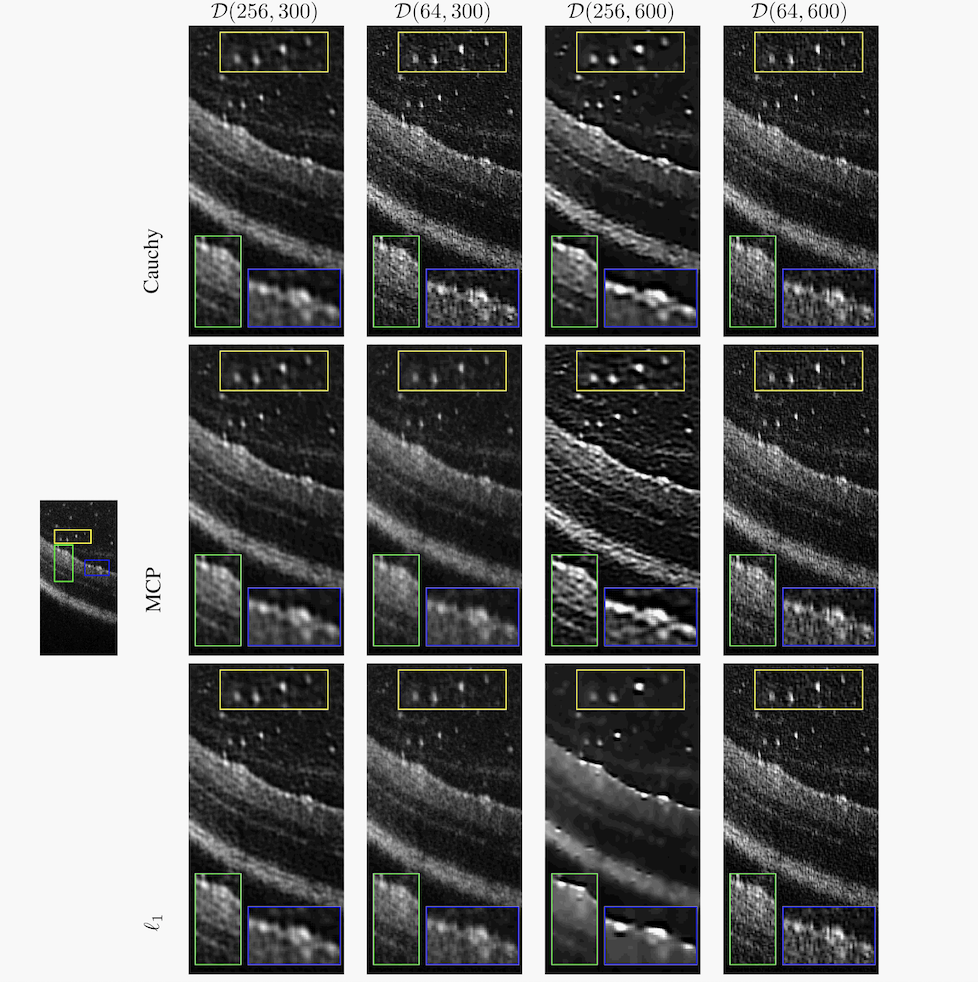
This will appear in the conference proceedings of the IEEE ISBI 2021 conference.
7.10 Estimation of a rejection class in machine learning algorithms
Participants: Sarah Laroui, Eric Debreuve, Xavier Descombes.
This work is made in collaboration with Aurelia Vernay (Bayer) as part of a contract with Bayer
We propose a learning strategy for supervised classification that is able, by design, to reject a sample as not belonging to any of the known classes 18. It follows a general strategy defined by three main steps: model learning independently for each class, threshold learning based on class interactions, and prediction procedure.
Each class model (PDF) consists of a GMM (Gaussian Mixture Model). We propose to learn a threshold on the associated PDF by analyzing how it responds to “its own” sample set, but also to the sample sets of the other classes (see Figure 11). We consider two approaches. In the 1-vs-all approach, the threshold is directly learned from the class samples and the samples from the other classes. In the 1-vs-1 approach we learn a threshold for each of the other classes by considering all pairs. The final threshold corresponds to the maximum of these values between. To estimate this threshold, we consider two methods. Considering the logistic regression, the derived sigmoid moves from the zero-tag position up to the one-tag one and the threshold is defined as the inverse image of the fictitious tag value 0.5, whereas for the misclassification method, the threshold corresponds to the value leading to the same number of false negatives and false positives.
In order to recognize known and new fungi phenotypes (see Figure 12), images are acquired by transmitted light microscopy. We take advantage of the feature learning capacity of Convolutional Neural Networks (CNN). Once MobileNet trained on the four known phenotypes, each image is represented by the feature vector produced by the CNN from its rescaled version. We proposed two options for feature vector definition. The output of the last layer before the classification part of the network (called Bottlenecks features) and the vector of the output layer (Output features). Using those two type of feature vectors, the proposed method provided very good classification accuracies for both the rejection class and the known classes on our data.
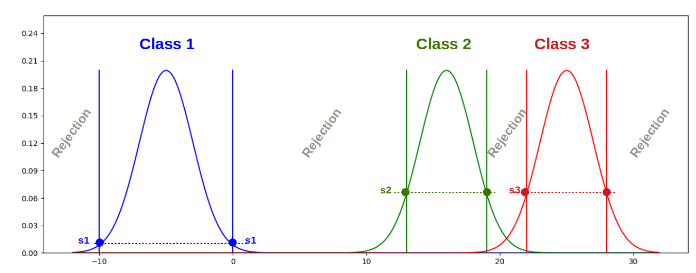
Threshold definition for the rejection class.
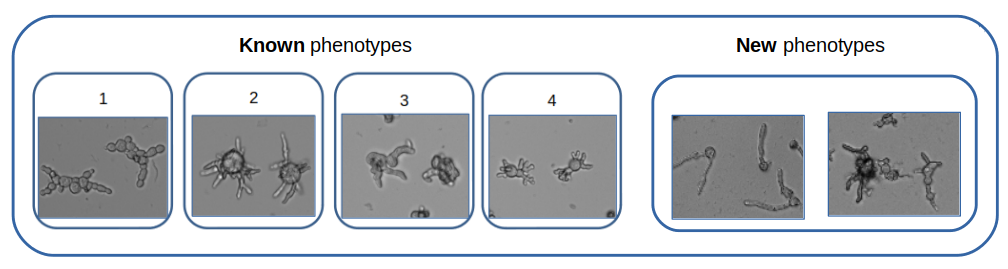
Characteristic phenotypic signatures for known (left) and new (right) phenotypes (transmitted light microscopy, ImageXpress microscope, 10x lens).
7.11 A classification scheme based on optimal transport
Participants: Sarah Laroui, Eric Debreuve, Xavier Descombes.
This work is made in collaboration with Aurelia Vernay (Bayer) as part of a contract with Bayer
Optimal transport provides a distance between two distributions defined as the minimum cost for transforming one distribution into another. It is a powerful tool for the comparison of distributions (continuous or discrete), or directly of point clouds.
As an alternative to GMM which seeks to "stick to the complexity" of the distribution of the data, we have proposed to transform the data so that they follow a simple model (in practice, Gaussian), the complexity of the data then being "hidden" in the transformation. This method is based on the calculation of an optimal transport plan between the data and samples generated according to a Gaussian law of the same mean and co-variance matrix as the data. The PDF of each class is therefore estimated via a transport to the Gaussian distribution chosen in the target space. Once in this space, the classification method with rejection class is the same as for the Gaussian mixture model. On the other hand, the observations whose class is to be predicted must be transported to the target space before being classified.
In this work in progress we have obtained promising results on synthetic data in 2D (see figure 13).
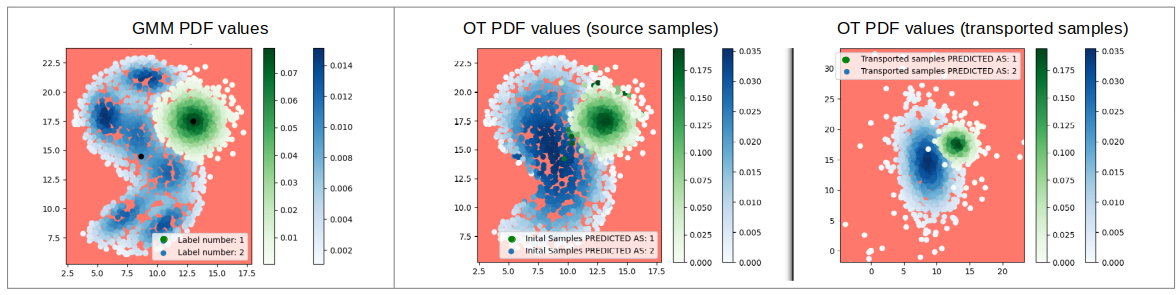
PDF values of the learning samples from a Gaussian mixture estimation (left) and optimal transport estimation (in the initial space (middle) and transported space (right).
7.12 RCC Classification by Vascular Morphology and A new RCC Histopathological Dataset
Participants: Rudan Xiao, Xavier Descombes.
This work is made in collaboration with Damien Ambrosetti, Nice CHU
RCC is a highly malignant tumor in the urinary system,
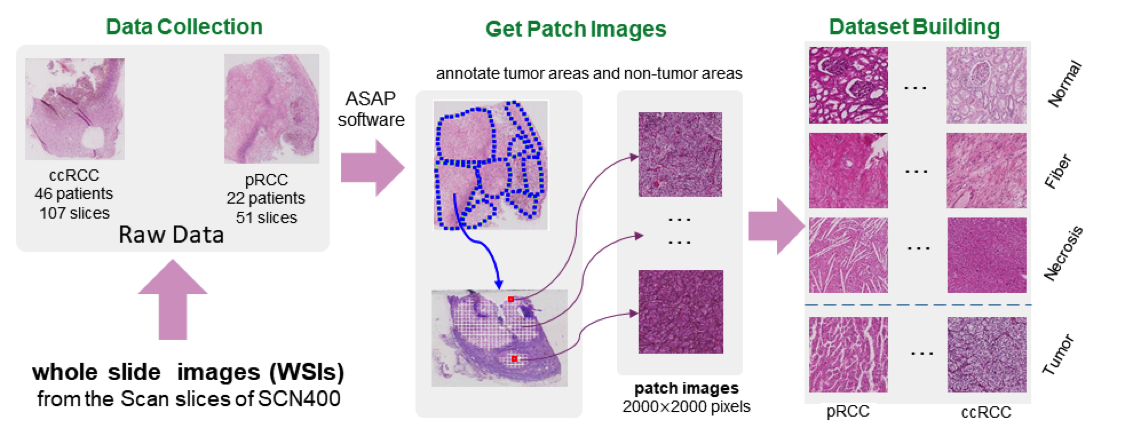
Pipeline of BigRCC dataset building.
7.13 Hierarchical classification using Convolutional Neural Networks (CNNs)
Participants: Abed Zaoui, Cédric Dubois, Eric Debreuve.
This work was made in collaboration with Jean-Olivier Irisson, LOV, Villefranche-sur-mer, France
CNN-based models have been used successfully in many computer vision tasks, and particularly for image classification. The Branch CNN model (B-CNN) has a specific architecture allowing to exploit the hierarchical relationships between the classes of a problem within the natural hierarchical structure of CNNs (see Figure 15). By constraining the optimization of the early layers so that they can provide appropriate features to classify the inputs into high-level classes, it is expected to get a higher accuracy on the finer classes at the network output. We tailored a network following this strategy in order to improve the classification of images of zooplankton individuals by using the zooplankton taxonomy established by marine ecologists. Rather than a higher accuracy, we obtained results on par with an equivalent, non-hierarchical CNN, but with a faster learning phase. Although this is encouraging, more work on the network architecture and the learning procedure is needed to indeed reach better performances.
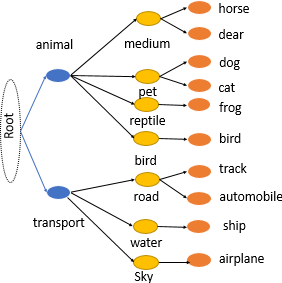
7.14 Computational detection, characterization, and clustering of mouse glial cells to unravel the implication of hypothalamic inflammatory response in obesity
Participants: Morgane Nadal, Eric Debreuve.
This work was made in collaboration with Clara Sanchez and Carole Rovère, IPMC, Sophia Antipolis, France
Overweight and obesity are major public health issues affecting respectively 39% and 13% of the world population (From World Health Organization, 2016). They constitute prominent risks for numerous chronic diseases, including diabetes, cardiovascular diseases, and cancer. Studies in animal models and humans reveal that excess fat diets promote both a peripheral chronic inflammation and a hypothalamic neuroinflammation, which possibly leads to feeding behavior deregulation. Ascertaining whether the inhibition of early activation of two major brain cells involved in feeding behavior (glial cells, more specifically astrocytes and microglia) in the hypothalamus could prevent obesity would offer new prospects for therapeutic treatments. To understand the mechanisms pertaining to obesity-related neuroinflammatory response, we developed a fully automated algorithm, NutriMorph (see Figure 16). Although some algorithms were developed in the past decade to detect and segment neural cells, they are highly specific, not fully automatic, and do not provide the desired morphological analysis. Our algorithm cope with these issues and performs the analysis of cells images (here, microglia of the hypothalamic arcuate nucleus), and the morphological clustering of these cells through statistical analysis and machine learning. Using the k-Means algorithm, it clusters the microglia of the control condition (healthy mice) and the different states of neuroinflammation induced by high-fat diets (obese mice) into subpopulations. Here we show that early hypothalamic inflammation could be already set on within a few hours through modification of microglia subpopulation proportions, instead of a couple of months as previously hypothesized and that the activated microglia show specific morphological characteristics.
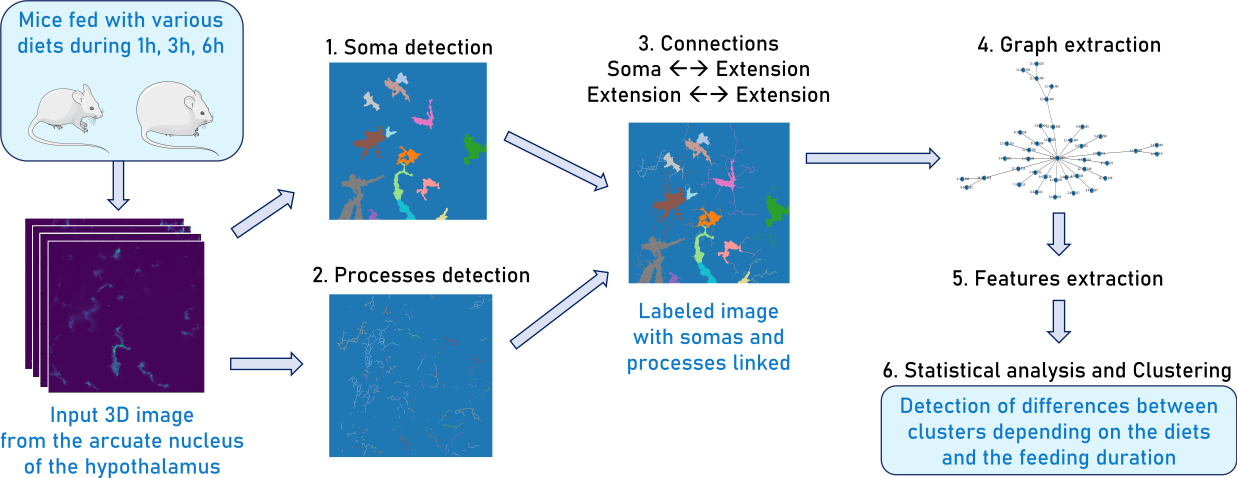
7.15 Cortical-inspired Wilson-Cowan models for vision. Applications to visual illusions
Participants: Luca Calatroni.
This work has been supported by the iCODE institute in Paris and has been carried out in collaboration with Dario Prandi (L2S, Paris), Valentina Franceschi (Univàrsita di Padova, Italy), Alexander Gomez-Villa and Marcelo Bertalmìo (UPF, Spain).
In this project, we reproduced suprathreshold perception phenomena, specifically visual illusions, by Wilson–Cowan (WC)-type models of neuronal dynamics. Our findings show that the ability to replicate the illusions considered is related to how well the neural activity equations comply with the efficient representation principle. Our first contribution consists in showing that the WC equations can reproduce a number of brightness and orientation-dependent illusions. Then we formally prove that there cannot be an energy functional that the WC dynamics are minimizing. This leads us to consider an alternative, variational modeling, which has been previously employed for local histogram equalization (LHE) tasks. To adapt our model to the architecture of V1, we perform an extension that has an explicit dependence on local image orientation. Finally, we report several numerical experiments showing that LHE provides a better reproduction of visual illusions than the original WC formulation, and that its cortical extension is capable also to reproduce complex orientation-dependent illusions, such as grating induction and a modified version of the Poggendorff illusion (see Figure 17). For this latter example, we empirically show the existence of a set of threshold parameters differentiating from inpainting to perception-type reconstructions and describing long-range connectivity between different hypercolumns in V1.
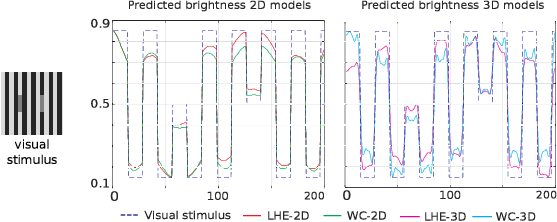
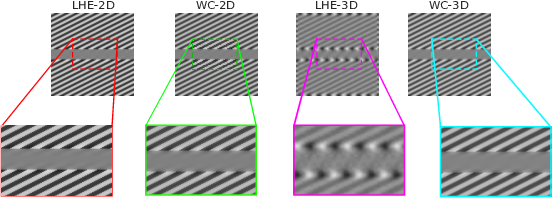
This work has been published in 13, 12
The software is available at the webpage https://
7.16 Variational osmosis for cultural heritage digital restoration
Participants: Luca Calatroni.
This project has been done in collaboration with Simone Parisotto (Fitzwilliam Musem, Cambridge), Nicolas Papadakis (IMB, Bordeaux) Aurelie Bougeau (LABRI, Bordeaux), Carola-Bibiane Schönlieb (DAMTP, Cambridge).
In this project, we proposed a new variational model for non-linear image fusion. Our approach is based on the use of an osmosis energy term related to the one studied in Vogel et al. and Weickert et al. The minimization of the proposed non-convex energy realizes visually plausible image data fusion, invariant to multiplicative brightness changes. On the practical side, it requires minimal supervision and parameter tuning and can encode prior information on the structure of the images to be fused. For the numerical solution of the proposed model, we develop a primal-dual algorithm and we apply the resulting minimization scheme to solve multi-modal face fusion, color transfer and cultural heritage conservation problems (see Figure 18). Visual and quantitative comparisons to state-of-the-art approaches prove the out-performance and the flexibility of our method.
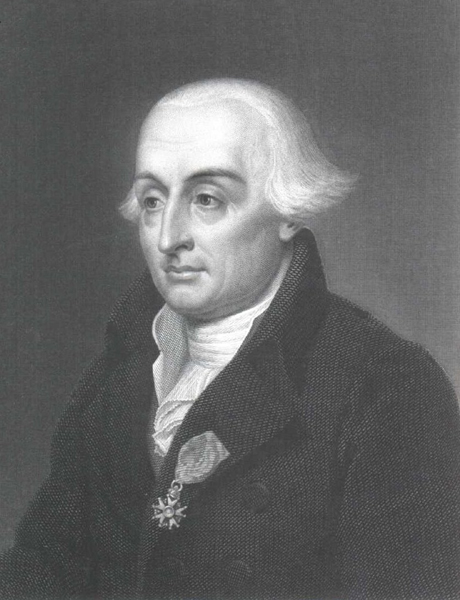
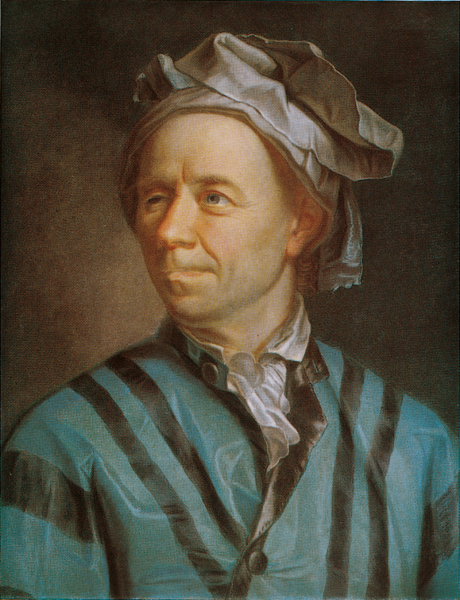
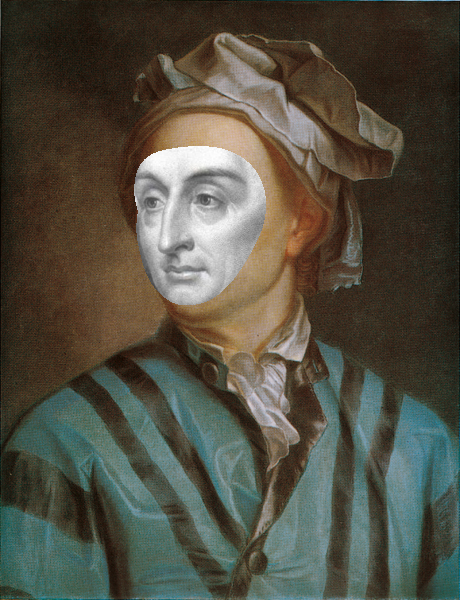
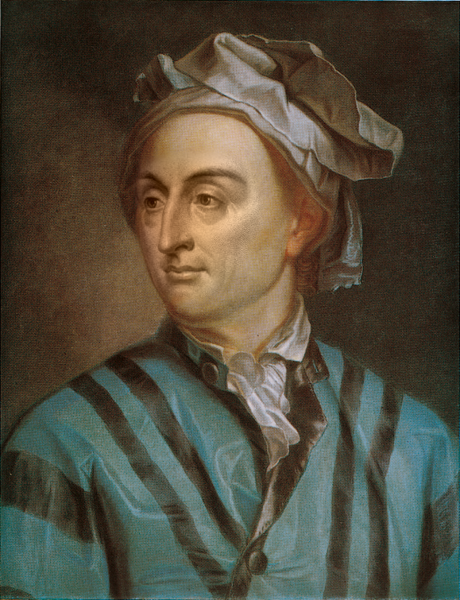
These results are described in the paper 15.
The code is available at the webpage https://
7.17 Fast optimisation via inertial dual diagonal descent
Participants: Luca Calatroni.
This work is in collaboration with L. Rosasco, S. Villa (MaLGA, Genova) and G. Garrigos (LPSM, Paris).
We propose and analyze an accelerated iterative dual diagonal descent algorithm for the solution of linear inverse problems with general regularization and data-fit functions. In particular, we develop an inertial approach of which we analyze both convergence and stability. Using tools from inexact proximal calculus, we prove early stopping results with optimal convergence rates for additive data-fit terms as well as more general cases, such as the Kullback-Leibler divergence, for which different type of proximal point approximations hold.
7.18 Direction-of-Arrival Estimation through Exact Continuous -Norm Relaxation problem
Participants: Laure Blanc-Féraud.
This work is made in collaboration with Emmanuel Soubies (IRIT, Toulouse), Adilson Chinatto and Joã Romano (University of Campinas, Brasil) and Pascal Larzabal (University Paris-Saclay).
Direction of Arrival (DOA) estimation is of fundamental importance in array signal processing. It refers to the process of retrieving the incident angles of signals reaching an antenna array. On-grid methods make the assumption that the incident angles belong to a prescribed grid. DOA estimation is then transformed into a challenging linear
group-sparse optimization problem involving the
This work has been published in IEEE Signal Processing Letters 17.
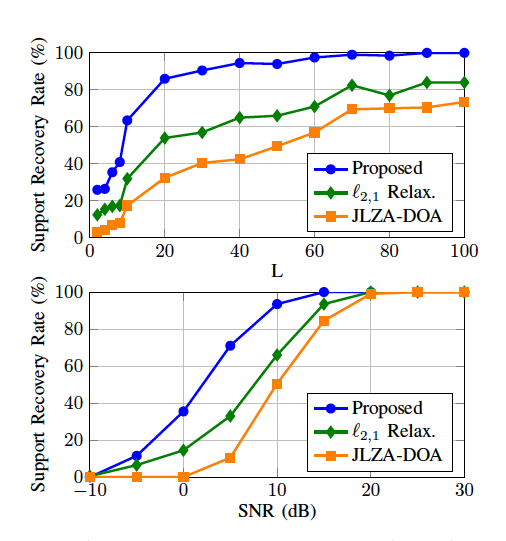
7.19 New Insights on the Optimality Conditions of the Minimization Problem
Participants: Laure Blanc-Féraud, Gilles Aubert.
This work is made in collaboration with Emmanuel Soubies (IRIT, Toulouse).
This work is devoted to the analysis of necessary (not sufficient) optimality conditions for the
Indeed, as global optimality is, in general, intractable, these algorithms only ensure the convergence to suboptimal points that verify some necessary optimality conditions. The degree of restrictiveness of these conditions is thus directly related to the performance of the algorithms. Within this context, our first goal is to provide a comprehensive review of commonly used necessary optimality conditions as well as known relationships between them. Then, we complete this hierarchy of conditions by proving new inclusion properties between the sets of candidate solutions associated to them. Moreover, we go one step further by providing a quantitative analysis of these sets. Finally, we report the results of a numerical experiment dedicated to the comparison of several algorithms with different optimality guaranties. In particular, this illustrates the fact that the performance of an algorithm is related to the restrictiveness of the optimality condition verified by the point it converges to. The results can be synthesized in Fig. 20.
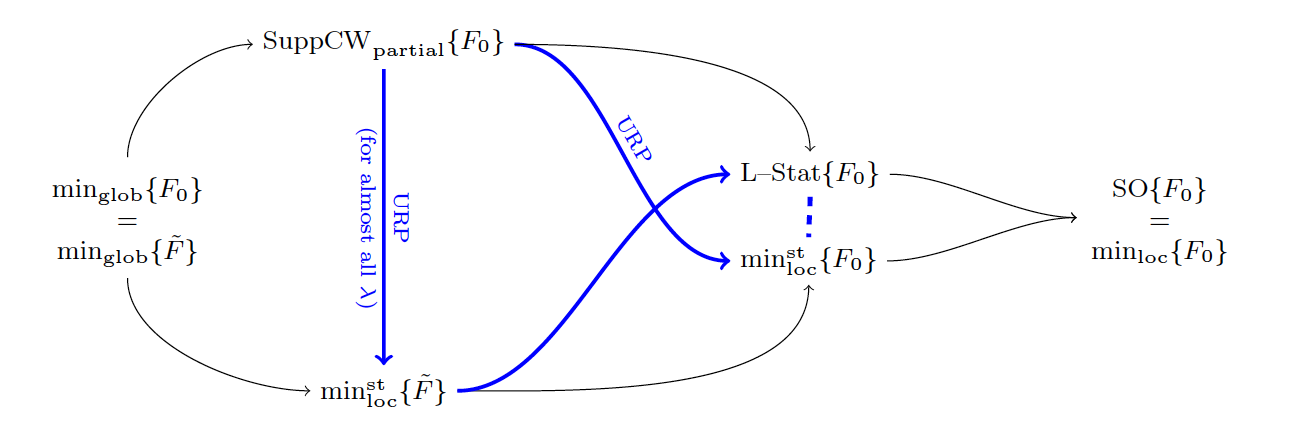
This work has been published in Journal of mathematical Imaging 16.
7.20 Quantification of confidence for SMLM localization via optimal transport
Participants: Bastien Laville, Laure Blanc-Féraud, Gilles Aubert.
Species of the fungal genus Candida are etiological agents responsible for life threatening infections, particularly in immunocompromised patients. For instance, candida albicans is carried by 70
7.21 Cell lineage calculation
Participants: Manuel Petit, Christophe Godin, Grégoire Malandain.
This work is made within the IPL Naviscope.
In recent years, techniques to image the development of biological organisms have made spectacular progresses. Researchers are now able to observe the trajectories corresponding to the development of 3D-plant tissues or animal embryo with cellular resolution. However, such observations yield a large amount of data, which, in turn, require fast and robust analysis tools to extract information while minimizing user interaction. The goal of M. Petit is first to propose new lineage extraction schemes, and then analysis tools over a population of lineages.
7.22 Morphogenesis of the sea urchin embryo
Participants: Angie Moullet, Grégoire Malandain.
This work is made in collaboration with Barthélémy Delorme and Matteo Rauzi (iBV, Nice).
The goal of the project is to understand how biophysical forces are generated and how they work to produce exquisitely precise and controlled tissue shape changes in embryo development. Tissue morphogenesis is a process by which the embryo is reshaped into the final form of a developed animal. Tissues are constituted by cells that are interconnected one another: local changes of cell mechanical properties and shape drive consequent tissue shape change. Nevertheless, the knowledge per se of the mechanisms and mechanics at the cell level which drive cell shape changes is insufficient to explain how tissues change their shape. Emerging properties arise at higher scales resulting from the interaction of cells within tissues and of tissues coordinating and interacting with one another.
To study the embryo evolution at a cellular scale, temporal series have been acquired by a multi-view light-sheet microscope. We will use the Mediterranean sea urchin embryo species Paracentrotus lividus as a model system and focus on the process of tissue folding.
The Astec software toolbox, originally developed for 14, has been adapted to urchins, and enriched with measurements of new morphological parameters (e.g. urchin embryos have a blastocoel that ascidian embryos do not have)(see Figure 21). A. Moullet succeeded in measuring and studying the archenteron length evolution over a few sea urchin embryos.
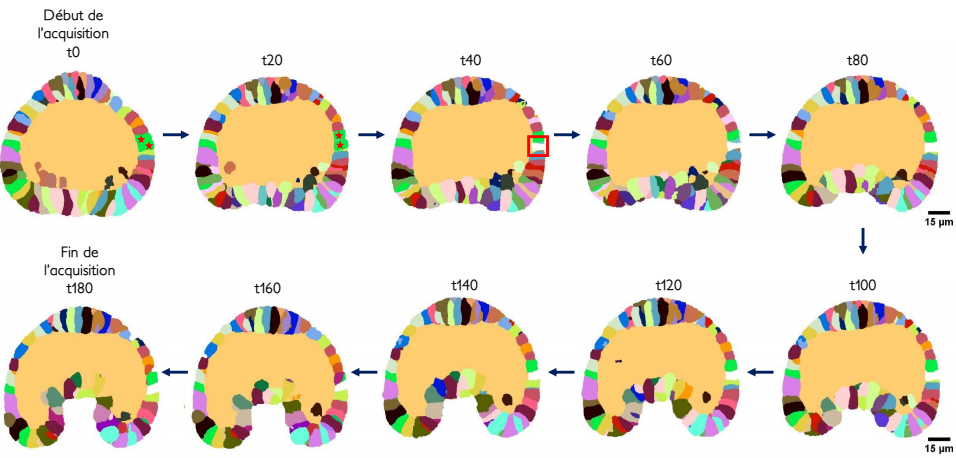
7.23 Image processing pipeline and statistical analysis for the ANR project RNAGRIMP
Participants: Florence Besse, Eric Debreuve, Xavier Descombes, Fabienne de Graeve, Somia Rahmoun, Xuchun Zhang.
From a methodological point of view, the ANR project RNAGRIMP deals with the detection of particles (RNP-IMP granules) inside cell cytoplasms, and their analysis in terms of number, properties, and spatial repartition. Two series of images have been acquired using fluorescence microscopy: one where the cell cytoplasm has been stained with GFP (Green Fluorescent Protein), the second where the nuclei have been stained with DAPI (4',6-diamidino-2-phenylindole). The experimental conditions were varied in order to target different genes potentially implied in the regulation of the RNP-IMP granules. In total, this screening generated 3 millions of images. In the first step of the image processing pipeline, the nuclei are detected on the DAPI images and a classifier into living or dead cell is learned based on morphological and radiometric nuclei properties (average intensity, area, granularity, circularity...). Then, the living cell cytoplasms are segmented on the GFP images. This task is particularly difficult due to the heterogeneity of the cytoplasmic intensity, both within a cell and across cells. Besides, cells regularly form clusters inside which there is no clear separation between adjacent cells. Next, small particles are detected inside the segmented cytoplasms. Finally, a database of particles is fed with the characteristics of the detected particles: corresponding cell and experimental condition, shape properties, radiometric properties, and indicators of position within the cell cytoplasm.
In order to identify which genes are indeed implied in the regulation of the RNP-IMP granules and how, specific statistical tools are being developed to analyze the particle database. The average number of particles per cell, or the cytoplasmic particle density if all cells are roughly the same size, is the primary factor to study. Preliminary results already allow to ensure that candidate regulatory genes can be identified using the proposed image processing pipeline and database analysis (see Fig. 22).
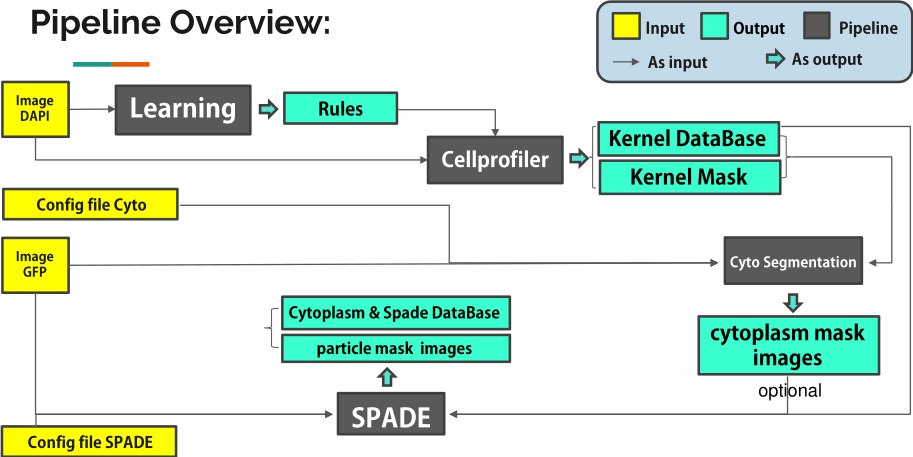
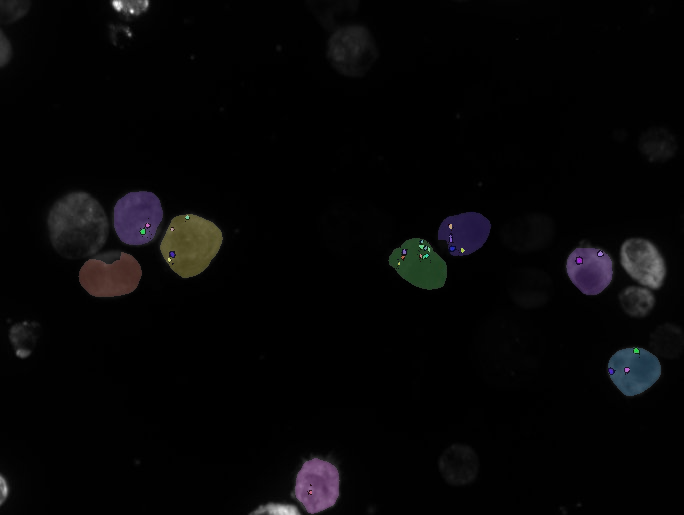
8 Bilateral contracts and grants with industry
8.1 Bilateral contracts with industry
- Bayer CropScience, Lyon: 39-month companion contract (Aug 2018 – Oct 2021) for the Cifre PhD thesis of Sarah Laroui.
9 Partnerships and cooperations
9.1 Informal international partners
- Xavier Descombes is collaborating with Franscesco Ponzio, PhD student at Politecnico di Torino, on deep learning approaches for analyzing histopathological images. This work is part of a collaboration with Nice Hospital on histopathology for kidney cancer.
- Luca Calatroni is collaborating with Prof. Fiorella Sgallari, Prof. Alessandro Lanza, Dr. Monica Pragliola, Prof. Elena Loli Piccolomini, Pasquale Cascarano and Prof. Serena Morigi (University of Bologna, Italy) on variational modesl for image reconstruction.
- Luca Calatroni is collaborating with Dr. Simone Rebegoldi (University of Firenze) on optimisation approaches for imaging.
- Luca Calatroni has collaborated with Dr. Emre Baspinar (MathNeuro team) on neural-field models for vision.
- Luca Calatroni has collaborated with Dr. Guillaume Garrigos (LPSM, Paris), Prof. Lorenzo Rosasco and Prof. Silvia Villa (MaLGA, University of Genova) on inertial optimisation models for imaging and machine learning.
- Laure Blanc-Féraud has collaborated with A Chinato and J. Romano from university of Campinas Brasil on sparse optimization for the DOA (Direction of Arrival) problem in antennas processing.
9.2 International research visitors
9.2.1 Visits of international scientists
- Alin Achim, professor at Bristol University, UK, was in Morpheme within a Leverhlume grant.
- Pasquale Cascarano, PhD student at the University of Bologna, IT, was supposed to visit Morpheme for two months (March-April 2020), but due to the COVID-19 pandemic, the visit was postponed to further date.
9.3 European initiatives
9.3.1 FP7 & H2020 Projects
- Luca Calatroni, involved in the H2020 RISE NoMADS project, has worked to add UCA to the consortium. The project will last till February 2022, and it will be probably extended due to the COVID-19 pandemic. He leads the WP4.
9.4 National initiatives
9.4.1 Labex SIGNALIFE
The Morpheme team belongs to the Labex (Laboratory of Excellence) Signalife. This Labex embeds four biology institutes in Nice, Sophia Antipolis and two Inria teams.
9.4.2 UCA Arch-AI-story
Participants: Luca Calatroni, Laure Blanc-Féraud.
The project involves a large network of research scientists and is led by I. Thery-Parisot (CEPAM).
It focuses on the analysis of high-resolution imaging data (2D, 3D, Tomography) for the characterisation of objects at different scales such as frescoes, manuscripts, biological archives (wood, bones) and geological findings (pottery). It focuses in particular on the exploitation of the potential of applied mathematiques and artificial intelligence to Archeology and Art history. The use of tools coming from Artificial Intelligence and, in particular, of advanced imaging techniques is expected to improve the interpretation of historical and archaeological data. One specific aspect is also the make available the large databases to the AI communities.
The project will last for the year 2021.
9.4.3 ANR JCJC RUBIN-VASE
Participants: Luca Calatroni.
This project is in collaboration with Dario Prandi (L2S)[PI], Valentina Franceschi (UniPD), Laurent Perrinet (INT) and Giuseppina Turco (LLF, Paris).
In this project we will design novel variational models defined in suitable functional spaces to translate the neurobiological principle of sensory efficient representation into mathematical terms. This will be done by addressing the following three different problems:
- The modelling of neuronal processes in V1, especially through the study of hallucinatory patterns;
- The design of a neuro-inspired framework for sound processing and speech reconstruction;
- The comparison between neurobiological models and (deep) data-driven ones, notably relying on ideas borrowed from the fields of sparse sensing and predictive coding.
Our goal is to formulate new effective reference models adapted to describe both visual and auditory perceptual phenomena, overcoming the limitations associated to WC equations and L+NL models.
This project will be carried out by coupling in parallel the development of rigorous analytical theories with their numerical and experimental validation. It features a focused team of young researchers with a synergy of combined expertise in variational methods, signal processing, optimisation, control theory, sparse coding, computational neurosciences, and speech analysis. It will start in March/April 2021 and last for three years.
9.4.4 ANR RNAGRIMP
Participants: Florence Besse, Fabienne De Graeve, Xavier Descombes, Eric Debreuve.
Here, we propose to study the molecular bases underlying the assembly and regulation of RNA granules, using the highly conserved IMP-containing granules as a paradigm. Specifically, we propose to perform an unbiased genome-wide RNAi screen on Drosophila cultured cells to identify mutant conditions in which the organization and/or distribution of IMP-containing granules is altered. To quantitatively and statistically analyze mutant conditions, and to define precise and coherent classes of mutants, we will combine high throughput microscopy with the development of a computational pipeline optimized for automatic analysis and classification of images. The function of positive hits isolated in the screen will then be validated in vivo in Drosophila neurons using fly genetics and imaging techniques, and characterized at the molecular and cellular levels using biochemical assays, in vitro phase transition experiments and live-imaging. Finally, the functional conservation of identified regulators will be tested in zebrafish embryos combining gene inactivation and live-imaging techniques. This integrative study will provide the first comprehensive analysis of the functional network that regulates the properties of the conserved IMP RNA granules. Our characterization of the identified regulators in vivo in neuronal cells will be of particular significance in the light of recent evidence linking the progression of several degenerative human diseases to the accumulation of non-functional RNA/protein aggregates.
This 4-years project started january, 2016 and is leaded by F. Besse (iBV, Nice). Participants are iBV, institut de biologie Paris Seine (IBPS, Paris), and Morpheme.
9.4.5 ANR HMOVE
Participants: Xavier Descombes, Eric Debreuve, Somia Rahmoun.
Among the signaling molecules involved in animal morphogenesis are the Hedgehog (Hh) family proteins which act at distance to direct cell fate decisions in invertebrate and vertebrate tissues. To study the underlying process we will develop accurate tracking algorithm to compare trajectories of different Hh pools transportation in live animals. This will allow us to analyze the contribution of the different carriers in the establishment of the Hh gradient. Moreover, we will develop new methods to modify the spatio-temporal and dynamical properties of the extra-cellular Hh gradient and separate the contribution of the apical versus basal Hh pools. We will complete this study with a genome-wide screen to identify genes and related cellular processes responsible for Hh release. The particular interest of this collaboration lies in the combination of development of tracking algorithm to analyze Hh distribution and trajectories with extremely powerful genetics, ease of in vivo manipulation and lack of genetic redundancy of Drosophila.
This 4-years project started january, 2016 and is leaded by P. Thérond (iBV, Nice). Participants are iBV and Morpheme.
9.4.6 ANR Cell Whisper
Participants: Grégoire Malandain.
Successful embryogenesis requires the differentiation of the correct cell types, in defined numbers and in appropriate positions. In most cases, decisions taken by individual cells are instructed by signals emitted by their neighbours. A surprisingly small set of signalling pathways is used for this purpose. The FGF/Ras/ERK pathway is one of these and mutations in some of its individual components cause a class of human developmental syndromes, the RASopathies. Our current knowledge of this pathway is, however, mostly static. We lack an integrated understanding of its spatio-temporal dynamics and we can imperfectly explain its highly non-linear response to a graded increase in input stimulus.
This systems biology project combines advanced quantitative live imaging, pharmacological/optogenetics perturbations and computational modelling to address 3 major unanswered questions, each corresponding to a specific aim:
- Aim 1: What is the spatio-temporal dynamic of intracellular signal transduction in response to FGF?
- Aim 2: What is the molecular basis of the switch-like response to graded extracellular signals?
- Aim 3: Can the results be integrated into a predictive computational model of the pathway?
Through this approach, in a simplified model system, we hope to gain an integrated view of the P. Lemaire (CRBM, Montpellier). Participants are CRBM (Montpellier), LIRMM (Montpellier), MOSAIC (INRIA Grenoble) and Morpheme.
9.4.7 Inria Large-scale initiative Naviscope
Participants: Grégoire Malandain.
This action gathers the expertise of seven Inria research teams (Aviz, Beagle, Hybrid, Morpheme, Parietal, Serpico and Mosaic) and other groups (MaIAGE, INRAE, Jouy-en-Josas and UMR 144, Institut Curie Paris) and aimed at developing original and cutting-edge visualization and navigation methods to assist scientists, enabling semi-automatic analysis, manipulation, and investigation of temporal series of multi-valued volumetric images, with a strong focus on live cell imaging and microscopy application domains. More precisely, the three following challenges will be addressed:
- Novel machine learning methods able to detect the main regions of interest, and automatic quantification of sparse sets of molecular interactions and cell processes during navigation to save memory and computational resources.
- Novel visualization methods able to encode 3D motion/deformation vectors and dynamics features with color/texture-based and non-sub-resolved representations, abstractions, and discretization, as used to show 2D motion and deformation vectors and patterns.
- Effective machine learning-driven navigation and interaction techniques for complex functional 3D+Time data enabling the analysis of sparse sets of localized intra-cellular events and cell processes (migration, division, etc.).
9.4.8 3IA Côte d'Azur
Participants: Laure Blanc-Féraud.
Laure Blanc-Féraud has a chair of 3IA Côte d'Azur. First objective within this chair is to develop a new 3D+t super-resolution fluorescent microscopes. The objective is to co-construct a system (instrument and numerical algorithms) providing fully super-resolved 3D+t fluorescent images, using tools as sparse optimization, time-series analysis, tensors and automatic learning. One application is to provide better 3D localization of membrane proteins (e.g. ion channel subunits, receptors), for studies of their maturation and trafficking. A second project within this chair concerns imaging of extracellular matrix (ECM) components unregulated in cancer, and involves the development of computational tools for modeling and analysis of ECM networks in tumor tissue. Work in progress uses graph modeling and graph matching or optimal transport to define distances capturing the evolution of geometric and topological structures of fiber networks. This project falls within a digital pathology initiative (coll. E. Van Obberghen-Schilling, iBV) involving the use of a combined scanner multispectral imaging system that produces high-resolution images at high throughput. Our goal is to extract biologically and clinically relevant results from these data.
9.5 Regional initiatives
9.5.1 UCA project LeftRightReliable
Participants: Xavier Descombes.
This project, in collaboration with M. Fürthaur (iBV), is supported by the IDEX JEDI, academy 4. We are studying the left-right organizer of the zebrafish to explain the organ laterality. Within the Morpheme team we will develop tools to track both endogenous and exogeneous particles in the Kupffer vesicule. Besides, we will propose a stochastic model of the particle trajectories to estimated the hitting position of the particles onto the membrane.9.5.2 UCA project SuperdynamicFun
Participants: Laure Blanc-Féraud.
This project, in collaboration with R. Arkowitz (iBV), is supported by the IDEX JEDI, academy 4. The aim of this project is the development of a system of spatial and temporal super-resolution in fluctuating molecule microscopy capable of capturing the dynamics of organelles inside filamentous hyphal cells of Albicans fungi in their pathogenic form.10 Dissemination
10.1 Promoting scientific activities
10.1.1 Scientific events: organisation
Member of the organizing committees
- Luca Calatroni organised the one-day tutorial Optimisation for machine learning and the two-day conference Imaging & machine learning which took place on January 29-31 2020. Website.
- Luca Calatroni is co-organiser of the SIAM Activity Group on Imaging Sciences webinars on Imagine and Inverse Problems (IMAGINE). Website.
- Laure Blanc-Féraud co-organized a one day and a half "Nouvelle imagerie pour la santé" (New imagery in health) for the Institute of CNRS INS2I in the framework of the thematic year on health and in memory of Chritian Barillot, 16-17 December.
- Laure Blanc-Féraud organized a half-day on AI & Biology in the framework of the 3IA Côte d'Azur Institute, 9 December.
10.1.2 Scientific events: selection
- Florence Besse gave seminars a the workshop on Molecular and developmental biology of Drosophila (22-26 June, virtual meeting) and the FENS meeting – Symposia on local translational control in neuronal circuit development, synaptic plasticity and memory (11-15th July, virtual meeting)
Member of the conference program committees
- Laure Blanc-Féraud was Associated Editor for the IEEE ISBI conference.
Reviewer
- Laure Blanc-Féraud was reviewer for IEEE ICASSP conference.
- Grégoire Malandain was reviewer for the ISBI and the ICPR conferences.
- Eric Debreuve was reviewer for the ICIP conference.
- Xavier Descombes was reviewer for the ICIP, ICASSP and ISBI conferences.
- Luca Calatroni was reviewer for the IEEE MLSP 2020 conference.
10.1.3 Journals
Member of the editorial boards
- Xavier Descombes is Associate Editor of Digital Signal Processing (DSP).
- Luca Calatroni served as Guest Editor for the Journal of Mathematical Neurosciences for the Special Issue Color representation and cortical-inspired image processing and Topics Board Editor of the Journal of Imaging.
- Laure Blanc-Féraud is part of the editorial board of the Encyclopaedia SCIENCES ISTE/WILEY in charge of the Image domain. The publisher ISTE, in partnership with WILEY for an English language publication, has launched an ambitious programme to produce an encyclopaedia called SCIENCE (see https://
www. ), which covers all fields of science, for a master/engineer level. Laure Blanc-Féraud defined 7 different themes for the Image field, found coordinators for these themes and defined with them about fifteen books to be written.istegroup. com/ fr/ sciences/
Reviewer - reviewing activities
- Xavier Descombes has been reviewer for Neuroimage.
- Luca Calatroni has been reviewer for: SIAM Journal of Imaging Sciences, Journal of Mathematical Imaging and Vision, Inverse Problems and Imaging, Applied Matahematical Modeling, IEEE Signal Processing Letters, IEEE Transactions on Signal Processing, Medical Image Analysis.
10.1.4 Invited talks
- Luca Calatroni was invited to present his work at the ALGORITMY 2020 conference and the Virtual Inverse Days 2020 workshop (University of Helsinki). He was also invited to present his work at the SIAM IS 2020 conference, the IFIP 2020 conference and the SIGMA 2020 conference, but due to the COVID-19 pandemic these conferences were either postponed or the mini-symposia were cancelled.
- Vasiliki Stergiopoulou was invited to present her work at the GdR MIA Thematic day on Non-convex Sparse Optimization on October 9th 2020 and to the 3IA Scientific Day of Axe 3 "AI for computational biology and bio-inspired AI" on December 9th 2020.
- Laure Blanc-Féraud was invited to give a keynote talk at NEUBIAS Conference, Network of BioImage Analysts, Bordeaux, 4-6 March; an invited talk at the Super-resolution school in Nice Sophia Antipolis 25-26 February; an invited seminar at the One World IMAGINE seminar, 27 May, (see https://
www. ).youtube. com/ watch?v=TEWDHjOMrVQ
10.1.5 Leadership within the scientific community
- Xavier Descombes is member of the scientific council of Labex Signalife and of IEEE Biomedical Image and Signal Processing Technical Committee.
- Luca Calatroni has been elected Director of the Group on Mathematical Imaging and Vision (MIVA) sponsored by the Italian Mathematical Union (UMI).
- Laure Blanc-Féraud is member of the IEEE Biomedical Image and Signal Processing Technical Committee.
- Grégoire Malandain is member of the IEEE Biomedical Imaging and Image Processing Technical Committee. He is member of the Scientific Committee of the NUMM department of INRAE.
10.1.6 Scientific expertise
- Xavier Descombes is expert for the research ministry to evaluate CIR proposal.
- Xavier Descombes is member of the evalaution ANR committee CE-45.
- Luca Calatroni has served as reviewer of one CIFRE PhD thesis proposal and one German Research Association (DFG) research grant proposal.
- Eric Debreuve has served as a reviewer of an ANR proposal for the evaluation committee CE45.
- Grégoire Malandain was member of a CNRS research engineer jury.
10.1.7 Research administration
- Luca Calatroni is member of the Directory Board of the I3S laboratory as co-responsible of the SIS research group.
- Laure Blanc-Féraud has lead the visiting committee HCERES of the LIRIS laboratory in Lyon in 9-12 March; she is member of CAP (commission administrative paritaire ) of INS2I Institue of CNRS; she is member of the scientific council of 3IA Côte d'Azur insitute, incharge of the IA & Biology axis; she is member of the scientific board of Idex academy 1 and COSP of EUR DS4H.
10.2 Teaching - Supervision - Juries
10.2.1 Teaching
- Licence : Arne Bechensteen, Outils pour la physique, 42h, L1, Polytech Nice Sophia - Univ Côte d'Azur , France
- Licence : Arne Bechensteen, Programmation impérative PeiP1, 13h30, L1, Polytech Nice Sophia - Univ Côte d'Azur , France
- Master : Arne Bechensteen, Traitement Numérique des Images, 8h, M2, Polytech Nice Sophia - Univ Côte d'Azur , France
- Master : Arne Bechensteen, Compression, analyse et visualisation de contenus multimédia, 2h, M2, Polytech Nice Sophia - Univ Côte d'Azur, France
- Licence: Florence Besse, Subcellular RNA targeting, 4h, L3, ENS Ulm, France
- Master: Florence Besse, Neurobiologie cellulaire et moléculaire, 6h, M1/2, Université Côte d'Azur, France
- Master: Florence Besse, Outils génétiques pour l’étude des circuits neuronaux, 4h, M1/2, Université Côte d'Azur, France
- Master: Florence Besse, Transport axonal, 2h, M1/2, Université Côte d'Azur, France
- Master: Laure Blanc-Féraud, Traitement avancé des images, master SD, 15h Eq. TD, Niveau M2, Université Côte d’Azur, France.
- Master: Eric Debreuve, Scientific image processing and Machine learning, Master SVS, 15h EqTD, M1 + M2, Université Côte d'Azur, France
- Licence 1ère année : Fabienne De Graeve, Médecine PASS option SV ECUE Génétique Formelle (5 TD de 2h X 3 groupes)
- Licence 3 ième année : Fabienne De Graeve, Sciences de la Vie : ECUE Acteurs Moléculaires de l'Information Cellulaire (CM de 10h, 4 TD de 2h X 3 groupes), ECUE Approches Pratiques en Biologie Moléculaire et Biologie Cellulaire (1TP de 35 h)
- Licence Professionnelle : Fabienne De Graeve, Génie Biologique Histologie Qualité : UE Biologie Cellulaire : CM de 4h, UE Histologie : 1TP de 23h X2 groupes, UE Outils pour la Biologie : 1TP de 8h
- Master : Fabienne De Graeve, Sciences de la Vie : UE Initiation au Traitement d'Images Biologiques (5 TP de 2h), UE Traitement Avancé d'Images, Biologiques (4 TP de 2h), UE Signalisation Cellulaire (CM de 2h et 1 TD de 2h)
- Master: Xavier Descombes, Traitement d’images, Analyse de données, Techniques avancées de traitement d’images, 10h Eq. TD, Niveau M2, ISAE, France.
- Master: Xavier Descombes, Traitement d’images, master, 9h Eq. TD, Niveau M2, Université Côte d’Azur, France.
- Master: Xavier Descombes, Bio-imagerie, master IRIV, 6h Eq. TD, Niveau M2, Université de Strasbourg, France
- Master: Xavier Descombes, Analyse d’images, master GBM, 9h Eq. TD, Niveau M2, Université Côte d’Azur, France.
- Master: Xavier Descombes, Traitement d'images scientifiques, master SVS, 15h Eq. TD Niveau M2, Université Côte d'Azur.
- Licence: Henrique Goulart, Automatique, 30h, niveau L3, Polytech Nice Sophia - Univ Côte d'Azur , France
- Licence: Henrique Goulart, Traitement Numérique du Signal, 48h, niveau L3, Polytech Nice Sophia - Univ Côte d'Azur, France
- Master/Ingénieur: Sarah Laroui, Data Science, M2/Ingénieur 5, Niveau 5eme année ingénieur, 7.5h Eq. TD, Polytech Nice Sophia - Univ Côte d'Azur , France.
- IUT 1ere année: Sarah Laroui, Acquisition et codage de l'information, niveau 1ère Année DUT RT, 13.5h Eq. TD, Institut Universitaire de Technologie (IUT) Sophia, France.
- DUT: Somia Rahmoun, Acquisition et Codage de l'Information, 8h Eq.TD, Bac+1, IUT Réseaux et Télécommunications de Nice Côte d'Azur, France
- Master: ECUE histoire de l'histoire de l'art: Luca Calatroni, Where arts & mathematics meet, niveau M1, 6h, Université Côte d'Azur, France.
- DUT : Cédric Dubois, Programmation, 26h TD, IUT QLIO, Qualité, Logistique Industrielle et Organisation, 2ième année (alternants), Université Côte d'Azur, France. Dans le cadre d'un DCME (64h Eq. TD annuelles).
- DUT : Cédric Dubois, Chaîne de transmission numérique 38h Eq. TD, IUT R
T, Réseaux et Télécommunications, 1ère année, Université Côte d'Azur, France. Dans le cadre d'un DCME (64h Eq. TD annuelles). - DUT : Vasiliki Stergiopoulou, Initiation à la measure du signal, 30h Eq. TD, IUT Réseaux et Télécommunications, UCA, TDRM117.
- DUT : Vasiliki Stergiopoulou, Acquisition et codage de l'information, 24h Eq. TD, IUT Réseaux et Télécommunications, UCA, TDRM118.
- DUT : Vasiliki Stergiopoulou, Initiations à la mesure du signal, 6h Eq. TD, IUT Réseaux et Télécommunications Malaisiens, UCA, TDRN117.
10.2.2 Supervision
- PhD: Anca-Ioana Grapa, Characterization of the organization of the Extracellular Matrix (ECM) by Image Processing, defended, Laure Blanc-Féraud, Xavier Descombes, E. van Obberghen, (iBV). PhD defense on June 11th, 2020.
- PhD: Arne Bechensteen, TIRF-MA and super-resolution by sparse estimation method, defended, Laure Blanc-Féraud, Gilles Aubert, Sébastien Schaub. PhD defense on 25 November.
- PhD in progress: Cédric Dubois, Classification du plancton conjointe en espèce et traits morphologiques et fonctionnels avec contrainte de relations espèces-traits et de hiérarchie des espèces en taxonomie génétique, october 1st, 2019, Eric Debreuve, Jean-Olivier Irisson (LOV, Villefranche-sur-mer).
- PhD in progress: Sarah Laroui, Classification and modelling of botrytis cinerea fungi growth from microscope images: toward the establishment of links between phenotypes and antifongic molecules, 1st August 2018, Eric Debreuve, Xavier Descombes
- PhD in progress: Manuel Petit, Quantifying and modeling trajectories of living form development, 1st November 2019, Grégoire Malandain, Christophe Godin (EPI Mosaic, INRIA, Lyon).
- PhD in progress: Clara Sanchez, Lipides nutritionnels et neuroinflammation : dévelopement d'outil de morphométrie cellulaire, 1st october 2019, Carole Rovère (IPMC), Eric Debreuve.
- PhD in progress: Asma Chalabi, Development of an algorithmic framework of tumor cells dynamic analyses, integrating machine learning and signal processing, 1st october 2020, Jérémie Roux (IRCAN), Eric Debreuve.
- PhD in progress: Vasiliki Stergiopoulou, Learning and optimization for 3D+T super‐resolution in fluorescent microscopy, January 1st 2020, Laure Blanc-Féraud, Luca Calatroni, Sébastien Schaub.
- PhD in progress: Rudan Xiao, Analysis and classification of cellular and vascular markers in histological images: application to kidney cancer, 1st october 2019, Xavier Descombes, Eric Debreuve.
- PhD in progress: Marta Lazzaretti, Duality and continuous relaxation of sparse optimisation problems in Banach spaces, November 1st 2020, Luca Calatroni, Claudio Estatico (University of Genova). PhD funded by Italian PhD grant at the University of Genova, Italy. Application for double PhD degree (UCA+University of Genova) and co-direction in progress.
- PhD in progress: Bastien Laville, Off-the-grid optimization in a time-dependent Radon measures space for super-resolution in microscopy, PhD funded by EUR DS4H grant, 1st October 2020, Laure Blanc-Féraud, Gilles Aubert.
10.2.3 Post-doctorates
- Post-doc: Vanna Lisa Coli, Image processing for ancient pottery, co-supervised by Laure Blanc-Féraud and Juliette Leblond EPI Factas.
- Post-doc: Henrique Goulart, Superresolution microscopy by blinking molecules, supervised by Laure Blanc-Féraud, Eric Debreuve and Sébastien Schaub.
10.2.4 Internships
- Abed Zaoui, Classification hiérarchique d’images de plancton par Deep learning, Eric Debreuve
- Morgane Nadal, Unravelling the implication of neuroinflammation in obesity by computational detection, characterization and clustering of mouse hypothalamic glial cells, Eric Debreuve
- Jérôme Emonet, Detection and classification of organoids from confocal images, Xavier Descombes
- Pat Vatiwutipong, Master of mathematics, "Properties of the d-Radon transform and application to imaging issues in archeology , co-supervised by Juliette Leblond EPI FACTAS and Laure Blanc-Féraud.
- Fatima Zahra El Barche, Deep learing for classifying uveitis in OCT images, Xavier Descombes, Alin Achim
- Gabriele Scrivanti, Super-resolution of OCT images via sparse representation, Luca Calatroni, Alin Achim and Serena Morigi (University of Bologna).
- Marta Lazzaretti, Continuous relaxation of weighted and sparse optimisation models for super-resolution microscopy with Poisson data, Luca Calatroni, Claudio Estatico (University of Genova).
- Bastien Laville, SMLM localization and confidence quantification using optimal transport, Laure Blanc-Féraud, Gilles Aubert.
- Angie Moullet, Semi-automatic image processing pipeline for quantitative analysis of cells dynamic in sea urchin embryo. P. Lividus, Grégoire Malandain, Matteo Rauzi (Sorbonne university).
10.2.5 Juries
- Florence Besse participated to one PhD defense committee (univ. Evry-Paris Saclay) as a reviewer.
- Laure Blanc-Féraud was part of four PhD defense committees (K. Mavreas Inria SAM, L. Donati EPFL as reviewer, A. Grapa as co-supervisor and B. Pascal as presidente). She was part of one "comité de suivi de thèse".
- Xavier Descombes participated as co-supervisor to the PhD thesis committee of Anca-Ioana Grapa (Univ. Côte d'Azur).
- Grégoire Malandain participated as reviewer to the PhD thesis committee of Antoine Legouhy (université de Rennes I).
10.3 Popularization
10.3.1 Articles and contents
The Science article 14 co-authored by Grégoire Malandain was mentionned in
- the journal The New York Times
- the journal Le Monde
- the journal Pour la science
- the journal CNRS Le journal
- the radio program La méthode scientifique
11 Scientific production
11.1 Major publications
- 1 articleVisual illusions via neural dynamics: Wilson-Cowan-type models and the efficient representation principleJournal of Neurophysiology1235May 2020, 1606-1618
- 2 article Detecting and quantifying stress granules in tissues of multicellular organisms with the Obj.MPP analysis tool Traffic July 2019
- 3 article Multiple objects detection in biological images using a marked point process framework Methods 2016
- 4 articleImaging plant growth in 4D : robust tissue reconstruction and lineaging at cell resolution.Nature Methods72010, 547-553
- 5 article Contact area-dependent cell communication and the morphological invariance of ascidian embryogenesis Science July 2020
- 6 articleFrom Curves to Trees: A Tree-like Shapes Distance Using the Elastic Shape Analysis FrameworkNeuroinformatics132April 2015, 175-191
- 7 articleA stochastic framework to model axon interactions within growing neuronal populationsPLoS Computational Biology1412December 2018, e1006627
- 8 articleA Continuous Exact l0 penalty (CEL0) for least squares regularized problemSIAM Journal on Imaging Sciences83July 2015, pp. 1607--1639 (33 pages)
- 9 article Nanometric axial resolution of fibronectin assembly units achieved with an efficient reconstruction approach for multi-angle-TIRF microscopy Scientific Reports 9 1 December 2019
11.2 Publications of the year
International journals
-
10
article
A continuous relaxation of the constrained
problem' Journal of Mathematical Imaging and Vision January 2021 -
11
articleNew
− algorithm for single-molecule localization microscopy'Biomedical optics express112January 2020, 1153 - 12 articleVisual illusions via neural dynamics: Wilson-Cowan-type models and the efficient representation principleJournal of Neurophysiology1235May 2020, 1606-1618
- 13 article Cortical-inspired Wilson-Cowan-type equations for orientation-dependent contrast perception modelling Journal of Mathematical Imaging and Vision June 2020
- 14 article Contact area-dependent cell communication and the morphological invariance of ascidian embryogenesis Science July 2020
- 15 articleVariational Osmosis for Non-linear Image FusionIEEE Transactions on Image Processing292020, 5507-5516
- 16 articleNew Insights on the Optimality Conditions of the l2-l0 Minimization ProblemJournal of Mathematical Imaging and Vision62July 2020, 808–824
- 17 articleDirection-of-Arrival Estimation through Exact Continuous l20-Norm RelaxationIEEE Signal Processing Letters282021, 16-20
International peer-reviewed conferences
- 18 inproceedings How to define a rejection class based on model learning? ICPR2020 - International Conference on Pattern Recognition Milan, Italy January 2021
Conferences without proceedings
- 19 inproceedingsAdaptive parameter selection for weighted-TV image reconstruction problems9th International Conference on New Computational Methods for Inverse Problems, NCMIP 2019 24 May 20191476Cachan, FranceMarch 2020, 012003
Doctoral dissertations and habilitation theses
- 20 thesis Characterization of fibronectin networks using graph-based representations of the fibers from 2D confocal images Université Côte d'Azur June 2020
Reports & preprints
- 21 misc On the inverse Potts functional for single-image super-resolution problems October 2020
- 22 misc Weighted-CEL0 sparse regularisation for molecule localisation in super-resolution microscopy with Poisson data October 2020
- 23 misc Scaled, inexact and adaptive generalized FISTA for strongly convex optimization January 2021
- 24 misc Non-convex Super-resolution of OCT images via sparse representation October 2020
-
25
misc
COL0RME: COvariance-based
super-Resolution Microscopy with intensity Estimation' October 2020
11.3 Cited publications
- 26 inproceedingsHigh density molecule localization for super-resolution microscopy using CEL0 based sparse approximationBiomedical Imaging (ISBI 2017), 2017 IEEE 14th International Symposium onIEEE2017, 28--31
- 27 articleFast live-cell conventional fluorophore nanoscopy with ImageJ through super-resolution radial fluctuationsNature communications, 7(1)2016, 12471-12471
- 28 article2004 WHO classification of the renal tumors of the adultsEuropean urology492006, 798-805
- 29 articleRenal-cell carcinomaNew England Journal of Medicine3351996, 865-875
- 30 articleQuantitative evaluation of software packages for single-molecule localization microscopyNature methods1282015, 717--24
- 31 articleSPARCOM: Sparsity Based Super-resolution Correlation MicroscopySIAM Journal on Imaging Sciences, 12 (1)2019, 392-419
-
32
articleA Unified View of Exact Continuous Penalties for
Minimization'SIAM Journal on Optimization2732017, 2034--2060