Keywords
Computer Science and Digital Science
- A2.3.1. Embedded systems
- A3.4.1. Supervised learning
- A3.4.2. Unsupervised learning
- A3.4.3. Reinforcement learning
- A3.4.5. Bayesian methods
- A3.4.6. Neural networks
- A3.4.7. Kernel methods
- A3.4.8. Deep learning
- A5.4.1. Object recognition
- A5.4.5. Object tracking and motion analysis
- A5.4.6. Object localization
- A5.9.2. Estimation, modeling
- A5.9.4. Signal processing over graphs
- A5.9.6. Optimization tools
- A9.3. Signal analysis
Other Research Topics and Application Domains
- B3.3.1. Earth and subsoil
- B3.3.2. Water: sea & ocean, lake & river
- B3.3.3. Nearshore
- B3.4. Risks
- B3.5. Agronomy
- B3.6. Ecology
- B8.3. Urbanism and urban planning
- B8.4. Security and personal assistance
1 Team members, visitors, external collaborators
Research Scientist
- Josiane Zerubia [Team leader, Inria, Senior Researcher, HDR]
Post-Doctoral Fellows
- Bahram Jafrasteh [Côte d'Azur University, until Aug 2020]
- Bilel Kanoun [Côte d'Azur University, from Sept 2020]
PhD Students
- Jules Mabon [Inria, from Oct 2020]
- Martina Pastorino [Inria and UGenoa (DITEN), from Nov 2020]
Interns and Apprentices
- Martina Pastorino [Inria and UGenoa (DITEN), Intern, from Apr 2020 until Oct 2020]
- Erik Takács [Inria and Szeged University (RGVC), Intern, from June 2020]
Administrative Assistant
- Nathalie Bellesso [Inria]
External Collaborators
- Mary Comer, [Purdue University, ECE, Associate Professor]
- Ihsen Hedhli [Laval University, IID, Research scientist]
- Zoltan Kato [Szeged University, RGVC, Professor]
- Tianyu Li [Purdue University, ECE, PhD Student ]
- Isabelle Manighetti [OCA, Géoazur, SEISMES, Professor ]
- Gabriele Moser [Genoa University, DITEN, Professor]
- Sebastiano Serpico [Genoa University, DITEN, Professor]
2 Overall objectives
The AYANA AEx is an interdisciplinary project using knowledge in stochastic modeling, image processing, artificial intelligence, remote sensing and embedded electronics/computing. The aerospace sector is expanding and changing ("New Space"). It is currently undergoing a great many changes both from the point of view of the sensors at the spectral level (uncooled IRT, far ultraviolet, etc.) and at the material level (the arrival of nano-technologies or the new generation of "Systems on chips" (SoCs) for example), that from the point of view of the carriers of these sensors: high resolution geostationary satellites; Leo-type low-orbiting satellites; or mini-satellites and industrial cube-sats in constellation. AYANA will work on a large number of data, consisting of very large images, having very varied resolutions and spectral components, and forming time series at frequencies of 1 to 60 Hz. For the embedded electronics/computing part, AYANA will work in close collaboration with specialists in the field located in Europe, working at space agencies and/or for industrial contractors.
3 Research program
3.1 FAULTS R GEMS: Properties of faults, a key to realistic generic earthquake modeling and hazard simulation
Decades of research on earthquakes have yielded meager prospects for earthquake predictability: we cannot predict the time, location and magnitude of a forthcoming earthquake with sufficient accuracy for immediate societal value. Therefore, the best we can do is to mitigate their impact by anticipating the most “destructive properties” of the largest earthquakes to come: longest extent of rupture zones, largest magnitudes, amplitudes of displacements, accelerations of the ground. This topic has motivated many studies in last decades. Yet, despite these efforts, major discrepancies still remain between available model outputs and natural earthquake behaviors. An important source of discrepancy is related to the incomplete integration of actual geometrical and mechanical properties of earthquake causative faults in existing rupture models. We first aim to document the compliance of rocks in natural permanent damage zones. These data –key to earthquake modeling– are presently lacking. A second objective is to introduce the observed macroscopic fault properties –compliant permanent damage, segmentation, maturity– into 3D dynamic earthquake models. A third objective is to conduct a pilot study aiming at examining the gain of prior fault property and rupture scenario knowledge for Earthquake Early Warning (EEW). This research project is partially funded by the ANR Fault R Gems, whose PI is Prof. I. Manighetti from Geoazur. Two sucessive postdocs (Barham Jafrasteh and Bilel Kanoun) have worked on this research topic funded by UCA-Jedi.
3.2 Probabilistic models on graphs and machine learning in remote sensing applied to natural disaster response
We currently develop novel probabilistic graphical models combined with machine learning in order to manage natural disasters such as earthquakes and flooding. The first model will introduce a semantic component to the graph at the current scale of the hierarchical graph, and will necessitate a new graph probabilistic model. The quad-tree proposed by Ihsen Hedhli in AYIN team in 2016 is no longer fit to resolve this issue. Applications from urban reconstruction or reforestation after natural disasters will be achieved on images from Pleiades optical satellites (provided by the French Space Agency, CNES) and CosmoSKyMed radar satellites (provided by the Italian Space Agency, ASI). This project is conducted in partnership with the University of Genoa (Prof. G.Moser and Prof. S. Serpico) via the co-supervision of a PhD student, financed by the Italian government. The PhD student, Martina Pastorino, has worked with Josiane Zerubia and Gabriele Moser in 2020 during her double Master degree at both University Genoa and IMT Atlantique. She started her PhD in co-supervision between University of Genoa DITEN (Prof. Moser) and Inria (Prof. Zerubia) in November 2020.
3.3 Marked point process models for object detection and tracking in temporal series of high resolution images
The model proposed by Paula Craciun's PhD thesis in 2015 in AYIN team, supposed the speed of tracked objects of interest in a series of satellite images to be quasi-constant between two frames. However this hypothesis is very limiting, particularly when objects of interest are subject to strong and sudden acceleration or deceleration. The model we proposed within AYIN team is then no longer viable. Two solutions will be considered within AYANA team : either a generalist model of marked point processes (MPP), supposing an unknown and variable velocity, or a multi-model of MPPs, simpler with regard to the velocity that can have a very limited amount of values (ie, quasi-constant velocity for each MPP). The whole model will have to be redesigned, and is to be tested with data from a constellation of small satellites, where the objects of interest can be for instance "speed-boats" or "go-fast cars". Some comparaisons with deep learning based methods belonging to Airbus Defense and Space (Airbus DS) will be done. Then this new model should be brought to diverses platforms (ground based or on-board). The modeling and ground-based application part will be studied within a PhD thesis (Jules Mabon), and the on-board version will be developed as part of a postdoctoral project (Camilo Aguilar-Hererra), both being conducted in partnership with Airbus DS, and the company Erems for the onboard hardware. This project is financed by BPI France within the LiChIE contract. This research project has started in 2020 for a duration of 4 years.
4 Application domains
Our research is applied within all Earth observation domains such as: urban planning, precision farming, natural disaster management, geological features detection, geospatial mapping, and security management.
5 Highlights of the year
5.1 Awards
Josiane Zerubia received the following awards in 2020 :
- IAPR Fellow in July (she is also EURASIP fellow (from 2019) and IEEE Fellow (from 2002))
- Doctor Honoris Causa from University of Szeged in Hungary in November
- Prize of Excellence of Université Côte d'Azur in December for the third time (2016, 2019, 2020)
6 New results
6.1 Multisensor and multiresolution remote sensing image classification through a causal hierarchical markov framework and decision tree ensembles
Participants: Martina Pastorino, Josiane Zerubia.
External collaborators: Gabriele Moser, Sebastiano Serpico, Ihsen Hedhli.
Keywords: image processing, stochastic models, optimization.
This work consisted in the development of a hierarchical probabilistic graphical model to tackle joint classification of multiresolution and multisensor remote sensing images of the same scene. This problem is crucial in the study of satellite imagery and jointly involves multiresolution and multisensor image fusion. Due to the heterogeneity of high or very high resolution (HR/VHR) remote sensing imagery, including optical (e.g., Pléiades, WorldView-3, SPOT-6/7) and synthetic aperture radar (SAR; e.g., COSMO-SkyMed Second Generation, TerraSAR-X, RADARSAT Constellation) data, a challenging problem is the development of image classifiers that can exploit information from multimodal input observations. The proposed framework consisted of a hierarchical Markov model with a quadtree structure to model information contained in different spatial scales, a planar Markov model to account for contextual spatial information at each resolution, and decision tree ensembles for pixelwise modeling. This probabilistic graphical model and its topology are especially fit for application to VHR image data. The theoretical properties of the proposed model were analyzed: the causality of the whole framework has been mathematically proved, granting the use of time-efficient inference algorithms based on the Marginal Posterior Mode (MPM) criterion, which are non-iterative when applied to quadtree structures. This is mostly advantageous for classification methods linked to multiresolution tasks formulated on hierarchical Markov models. Within the proposed framework, two multimodal classification algorithms were developed, that incorporate Markov mesh and spatial Markov chain concepts. The results obtained in the experimental validation conducted with two datasets containing VHR multispectral, panchromatic, and radar satellite images, verifies the effectiveness of the proposed framework (Figure 1). The proposed approach 3 was also compared to previous methods that are based on alternate strategies for multimodal fusion 1213 and a journal paper was submitted to Remote Sensing in December.
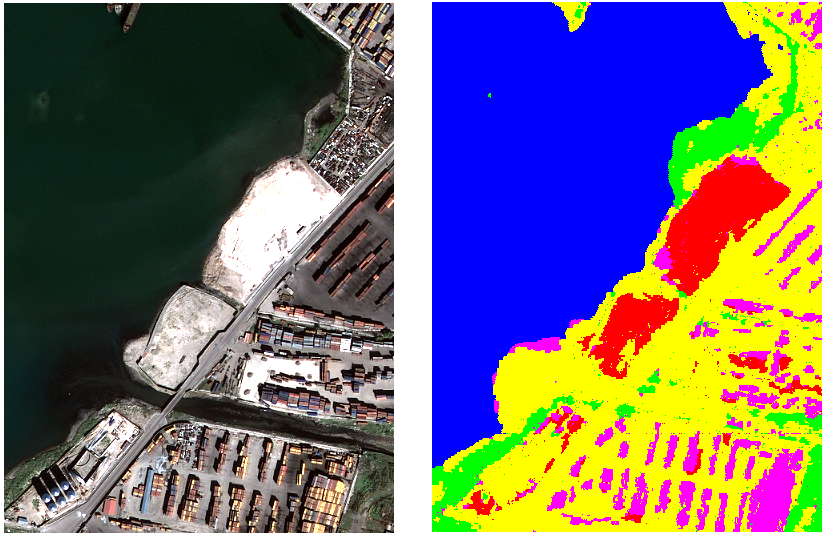
6.2 Semantic segmentation of remote sensing images combining hierarchical probabilistic graphical models and deep convolutional neural networks
Participants: Martina Pastorino, Josiane Zerubia.
External collaborators: Gabriele Moser, Sebastiano Serpico.
Keywords: image processing, stochastic models, deep learning, fully convolutional networks.
The work carried out consisted in the development of a novel method to deal with the semantic labelling of very high resolution (VHR) remote sensing data. Recent advances in deep learning, especially deep convolutional neural networks and fully convolutional networks (FCNs), have shown outstanding performances in this task. However, as for other methods, the map accuracy depends on the quantity and quality of ground truth (GT) used to train them. Having densely annotated data (i.e., a detailed, pixel-level GT) allows obtaining effective models, but requires high efforts in annotation. At the same time, probabilistic graphical models (PGMs) have sparked even more interest in the past few years, because of the ever-growing availability of VHR data and the correspondingly increasing need for structured predictions. The objective of this research work has been to combine different ideas from these approaches (deep learning and stochastic models) to develop novel methods for remote sensing image classification, specifically focusing on how deep fully convolutional networks can be exploited to deal with multiscale data through the integration with hierarchical Markov models. This approach made use of a quadtree to model the interactions of the pixels, inter-layer and intra-layer. The marginal posterior mode (MPM) criterion for inference was used in the proposed framework. In order to develop a refined pipeline mixing deep learning and PGMs for meeting the rising need for accurate semantic mapping in remote sensing images, this work studied two well-renowned deep learning architectures, i.e., U-Net and SegNet. Experimental validation was conducted with the ISPRS 2D Semantic Labelling Challenge Vaihingen dataset, in some cases with some modifications, applied to approximate sparse ground truths that are common in real remote sensing applications, and to assess the improvements that the proposed method could bring to the accuracy of the semantic segmentation. The results (Figure 2) are significant, as the proposed methodology has a higher producer accuracy (the map accuracy from the point of view of the map maker) compared to the standard FCNs considered, especially when the input training data are scarce and approach the real-world GTs available for land-cover mapping applications. Two conference papers were submitted to IGARSS`21 and EUSIPCO`21.
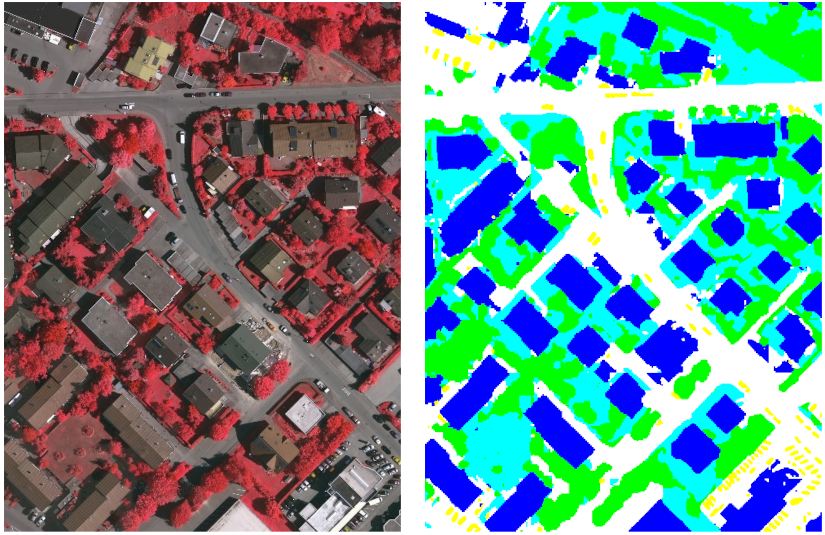
6.3 Change detection in multi-temporal remote sensing imagery using statistical and deep learning approaches
Participants: Erik Takács, Josiane Zerubia.
External collaborators: Zoltan Kato.
Keywords: change detection, image segmentation, deep learning, remote sensing.
This project aimed at designing a deep neural network for change detection. As a premise, we considered the problem as a segmentation task; computing the difference of two remote sensing images which have different domains and using a threshold method to get a change map. However this would have risen some false positives, such as illumination changes and other natural changes that are not of interest to us. In order to solve this problem we used deep neural networks to get a change map which only includes the relevant changes. Our implementation consisted of two encoders with the two images as input, a feature difference layer which takes as input the outputs of the two encoders and outputs a deep feature difference and lastly a decoder which constructed the change map. In order to segment the changed and unchanged areas we used the binary cross-entropy as loss function since the change detection task can be considered as a pixel wise binary classification between two classes: changed or unchanged. As we needed a large dataset for the segmentation, we used the public Change Detection Dataset (CDD) which consists of 16000 image pairs (256x256 pixels) and their associated ground truth . We trained our model on 5000 image pairs as a training set and 1500 image pairs as a validation set because of tight memory capacity. We evaluated different deep learning models 8, 10, 11 on public datasets. Among the tested models (Figure 3) the Code-Aligned Autoencoders (CAA) reached the best results on every dataset.
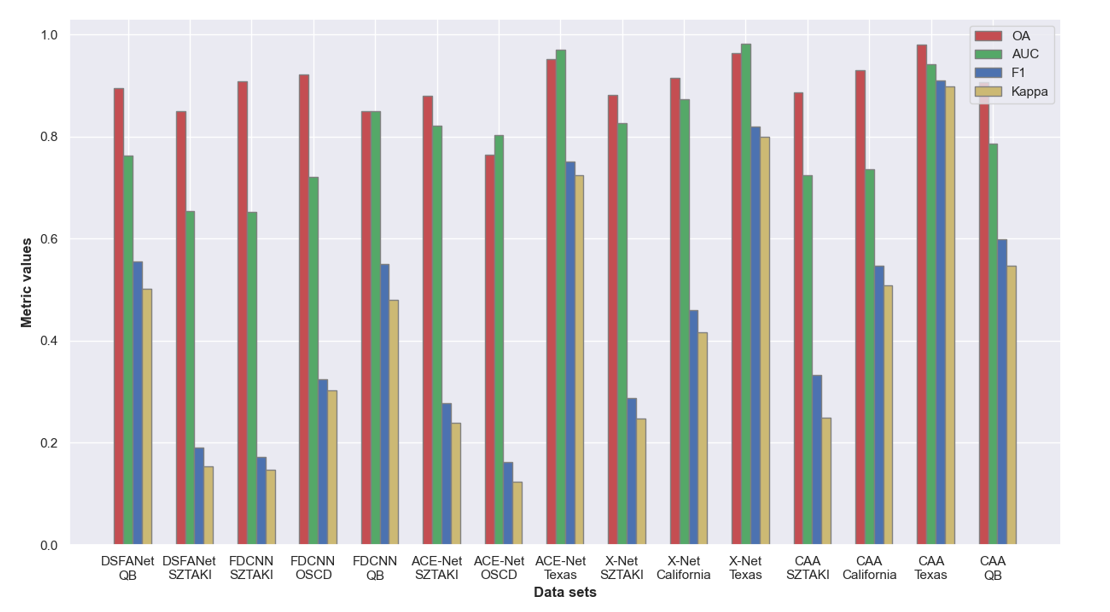
6.4 GAN and U-net abilities to automate tectonic fault mapping in remote sensing optical images
Participants: Bahram Jafrasteh, Josiane Zerubia.
External collaborators: Isabelle Manighetti.
Keywords: curvilinear feature extraction, machine learning, VHR optical imagery, remote sensing.
Tectonic faults are the source of earthquakes. They commonly form dense multi-scale networks including a master fault and multiple secondary faults and fractures. Documenting the organization and hierarchy of fault networks is among key information to understand the earthquake process. Generally, faults are identified through the traces they form at the ground surface. So far, these traces have been mapped manually, in the field or in remote sensing images. Yet this manual mapping requires tremendous time and relies on expert knowledge, which may not be available. Here, we exploreed U-net and Generative Adversarial Network (GAN) abilities to automate fracture and fault identification and mapping in remote sensing very high resolution (VHR) optical images. We used expert annotations to teach the models the faults appearance in the images, and hence conduct supervised learning. U-net is made of different convolutional layers that extract key features from the images and learn from the expert mapping how to identify the faults. GAN consists of two networks, a Generator and a Discriminator. The Generator has the U-net architecture. It recognizes the fault patterns in the images and generates synthetic faults maps. The Discriminator, whose architecture is that of half of the U-net, discriminates the expert and the synthetic maps, and sends its feedback to the Generator. This allows the Generator to progressively minimize the difference between the synthetic and the ground truth maps. To identify and map faults at the highest resolution, we developed a new loss function based on a combination of Mean Squared Error and Recall. We used two criteria to measure and compare the generalization performance of the GAN and U-net architectures. The qualitative and quantitative comparison of the two methods demonstrates the superiority of U-net for main faults and fractures mapping in remote optical images. However GAN appeared to better detect the fine faults (Figure 4). Machine learning with both U-net and GAN, has thus the potential to greatly assist geophysicists in documenting seismogenic faults. Two papers have been published 1, 4.
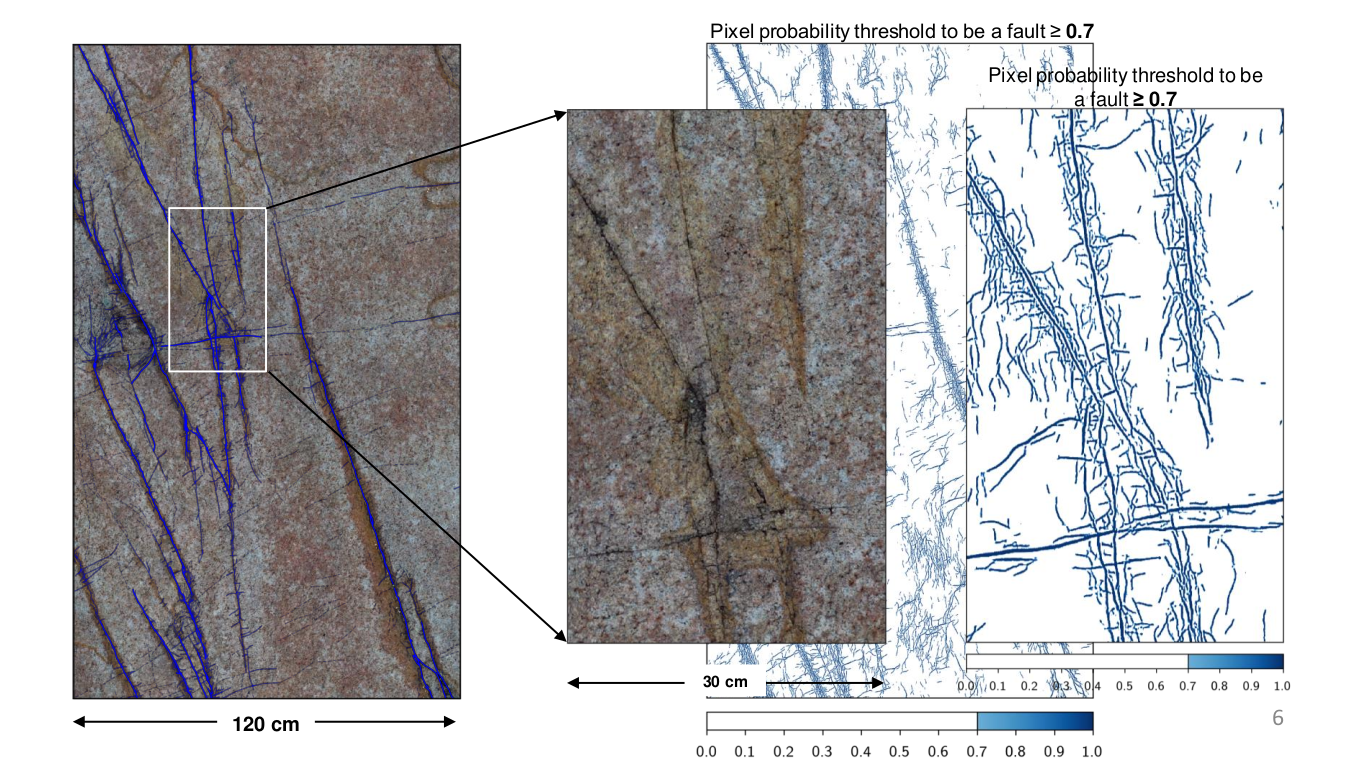
6.5 Extraction of curvilinear structure networks in image data using an innovative deep learning approach
Participants: Bilel Kanoun, Josiane Zerubia.
External collaborators: Isabelle Manighetti.
Keywords: curvilinear feature extraction, machine learning, VHR optical imagery, remote sensing.
Taking into account the results of the above mentioned research work 4, we decided to deeper investigate the U-net approach for faults and fractures mapping on very high resolution (VHR) optical images. For this purpose, we developed an upgraded variant of the classical U-Net neural network 14. The proposed method contains two sub-networks: The former is a cascade down-sampling network for extracting feature maps of fractures and faults from the VHR optical image, and the latter is an up-sampling network for reconstructing those extracted feature maps back to the same size of the input data. We trained our model on a specific given optical dataset together with its manual faults and fractures mapping provided by an expert. The results (Figure 5) are promising and confirm the effectiveness of using such architecture for detecting the fracture and fault traces on the basis of visual assessment.
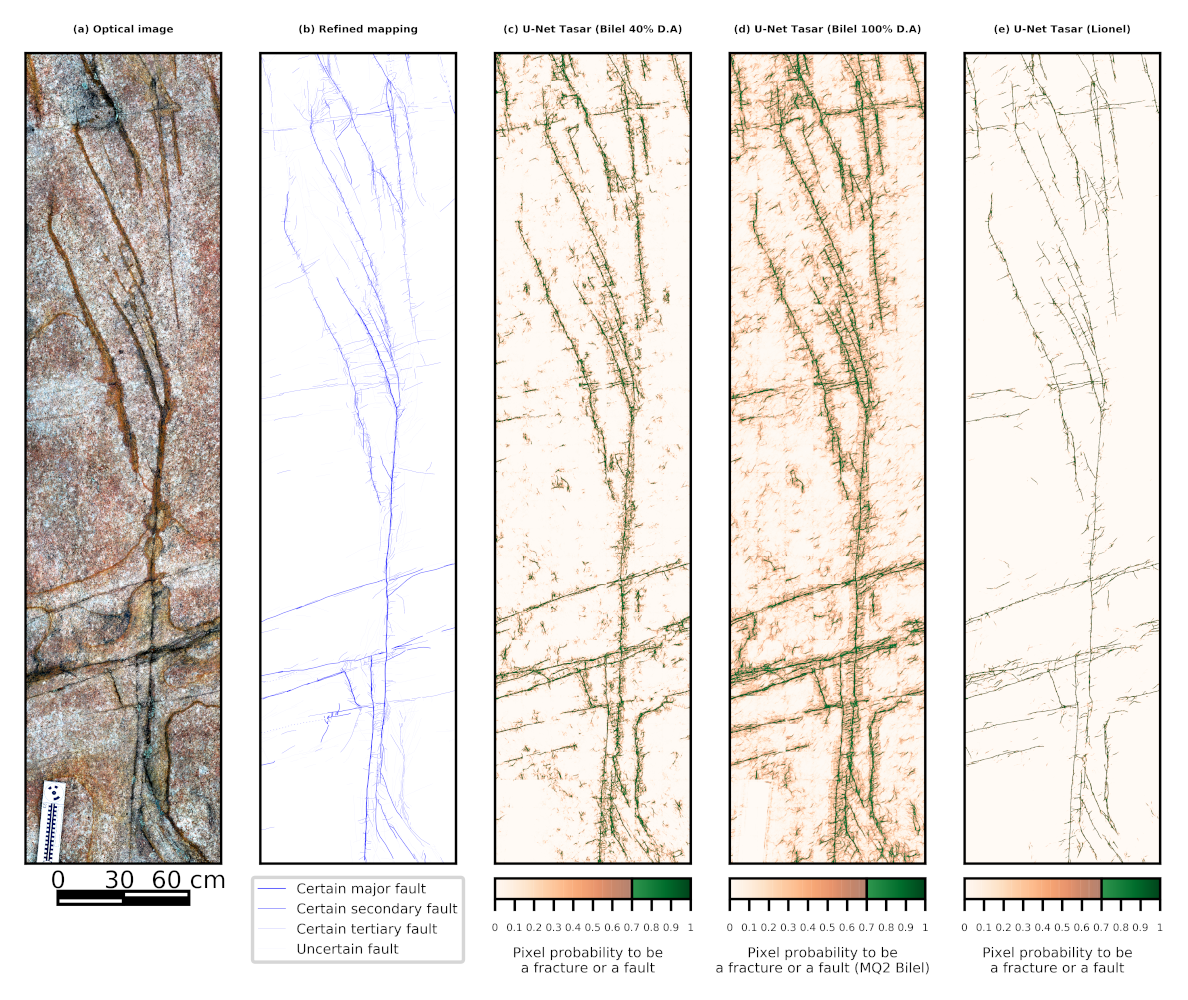
6.6 Feature extraction and tracking of CNN segmentations for improved road detection from satellite imagery
Participants: Josiane Zerubia.
External collaborators: Tianyu Li, Marie Comer.
Keywords: marked point process, deep learning, curvilinear structure detection, road detection, remote sensing.
Road detection from high-resolution satellite images is challenging for contrast-based detection methods due to the complexity of road networks, perturbations such as noise, and occlusions from cars, trees, etc. In this work, we proposed a novel road extraction and tracking method based on road segmentation results from a convolutional network (U-net), providing improved road detection. The proposed method incorporates our previously proposed connected-tube marked point process (MPP) model and a post-tracking algorithm. We present experimental results on the Massachusetts roads dataset (Figures 6 and 7) to show the performance of our method on road detection in remotely-sensed images 9. An international journal paper is in progress.
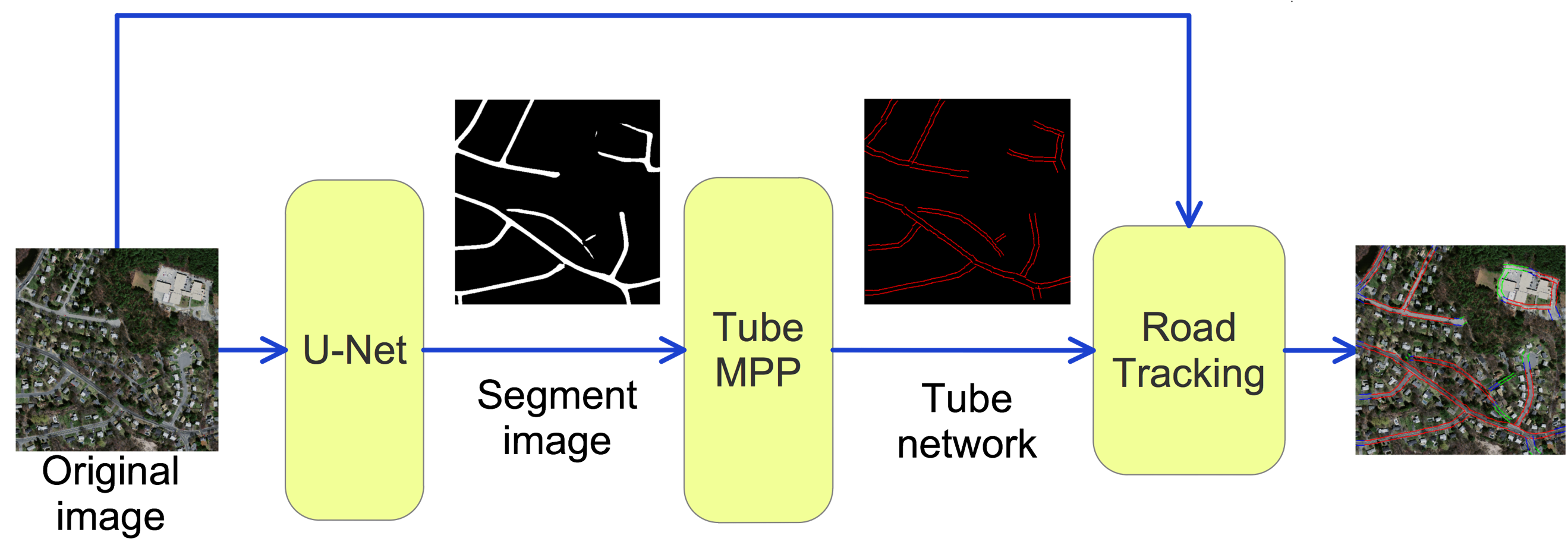
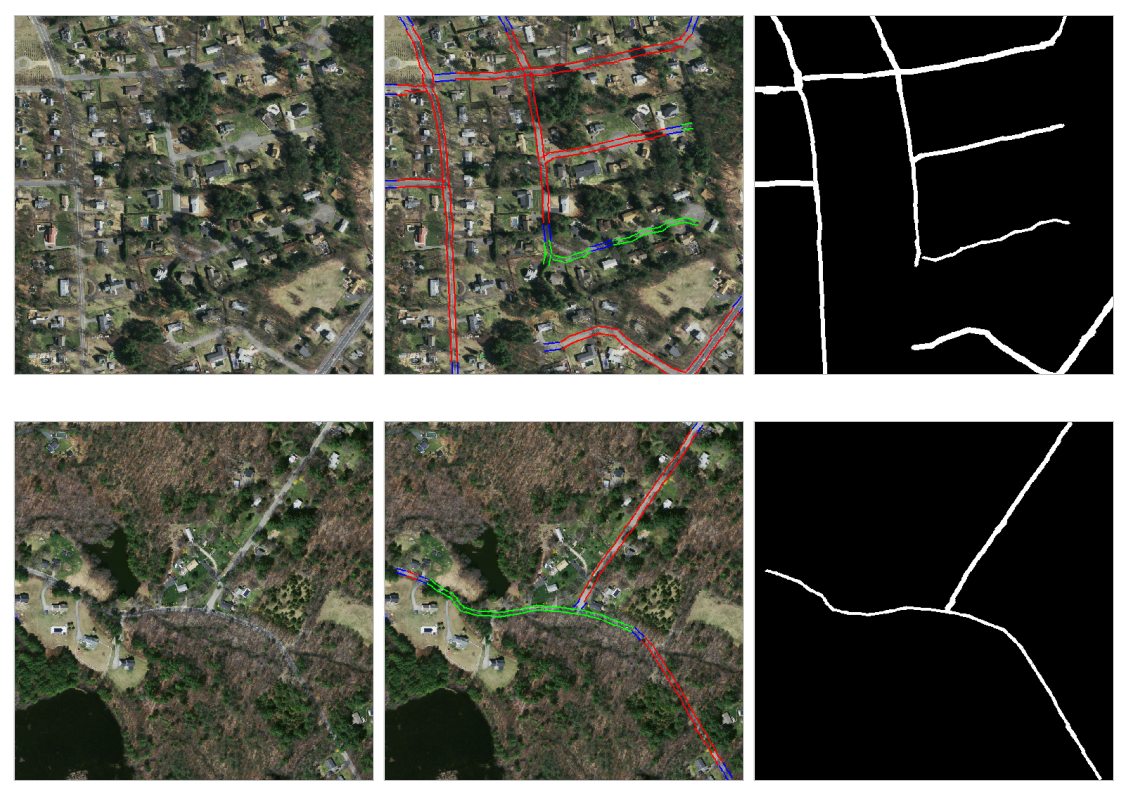
6.7 A tube marked point process model for curvilinear structure detection in images
Participants: Josiane Zerubia.
External collaborators: Tianyu Li, Marie Comer.
Keywords: marked point process, deep learning, curvilinear structure detection, retinal vessel extraction.
Curvilinear structure detection in images has been investigated for decades. In general, the detection of curvilinear structures includes two aspects, binary segmentation of the image and inference of the graph representation of the curvilinear network. In recent years, deep learning has been successfully applied to image segmentation and object detection. However, these supervised methods require accurate hand-labeled training data, which may not be available. In our work, we proposed a connected-tube model based on a marked point process (MPP) for addressing the two issues in an unsupervised way. The proposed method was applied to retinal vessel extraction and segmentation. The vessel network was extracted by the connected-tube MPP model first. Then a new tube-based segmentation method was applied to the extracted tubes 2. We tested this method on STARE and DRIVE databases (Figures 8 and 9).

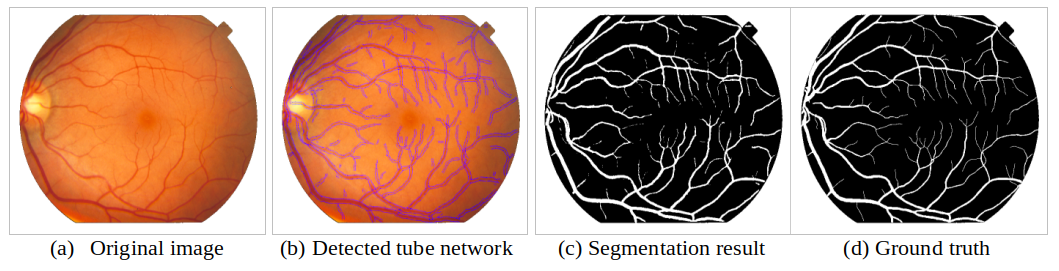
6.8 Combining Stochastic geometry and deep learning for small objects detection in remote sensing datasets
Participants: Jules Mabon, Josiane Zerubia.
External collaborators: Mathias Ortner.
Keywords: stochastic models, deep learning, fully convolutional networks, detection and tracking, remote sensing.
Unmanned aerial vehicles and low-orbit satellites, including cubesats, are increasingly used for wide area surveillance, which results in large amounts of multi-source data that have to be processed and analyzed. These sensor platforms capture vast ground areas at frequencies varying from 1 to 60 Hz depending on the sensor. The number of moving objects in such data is typically very high, accounting for up to thousands of objects. On one hand, stochastic geometry has proven to be extremely powerful for capturing object positions within images using a prior model on their relative positions. For instance, it is possible to introduce a regularizing term that accounts for relative positions of objects (see previous research results in both AYIN and ARIANA teams), in order to represent specific patterns. One major drawback of this approach is that the amount of marks that can be handled has to be limited in order to avoid an explosion of the problem dimensionality. Moreover, parameter optimization is more complex when objects have complex shapes. On the other hand, deep learning approaches have largely proven over the recent years to be extremely efficient in building representations that matches object signatures. In this research project, we aim at improving on the previous point process models for multiple objects tracking developed in AYIN team by Paula Craciun 5, 6, 7. We propose to merge both approaches and build a stochastic point process model in a state space that is an intermediary representation inferred by a convolutional neural network. We recently started by focusing on the detection of vehicles in static satellite images. Airbus DS has been providing the data as part their partnership with AYANA team within the LiChIE contract funded by BPI France. See Figure 10 as an example of the data.
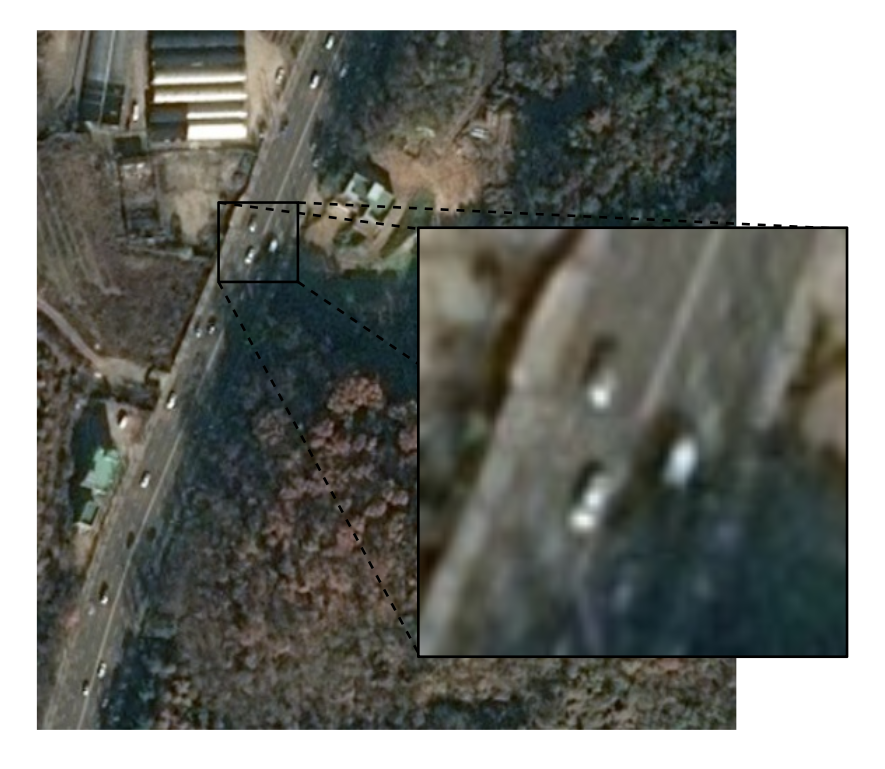
7 Bilateral contracts and grants with industry
7.1 Bilateral contracts with industry
7.1.1 LiChIE contract with Airbus Defense and Space funded by BPI France
Participants: Jules Mabon, Josiane Zerubia.
External collaborators: Mathias Ortner.
Automatic object detection and tracking on sequences of images taken from various constellations of satellites.
8 Partnerships and cooperations
8.1 International initiatives
8.1.1 Inria international partners
Declared Inria international partners
- Genoa University, Italy: Started more than 20 years ago. We currenlty have a co-tutelle PhD thesis agreement between UCA/Inria and UGenoa on semantic segmentation of remote sensing images combining hierarchical probabilistic graphical models and deep convolutional neural networks (funded by the Italian government).
- Szeged University, Hungary: Started more than 25 years ago. We currently have a co-tutelle Master intership on the topic of change detection in multi-temporal remote sensing imagery using statistical and deep learning approaches (funded by the Hungarian government).
Informal international partners
- Purdue University, USA: started in 2017. We currently work together on linear curvature extraction problems using both tube marked point processes and deep learning (funded by Purdue University).
8.2 International research visitors
8.2.1 Visits of international scientists
The two weeks visit of Dr. Igor Rizaev (Bristol University, Dept. EEE, UK) was planned in AYANA team at the end of March 2020 as well as a seminar, but was postponed to the end of 2021 due to the Covid-19 pandemic.
8.3 National initiatives
AYANA team is part of the ANR project FAULTS R GEMS (2017-2021, PI Geoazur) dedicated to realistic generic earthquake modeling and hazard simulation.
8.4 Regional initiatives
Isabelle Manighetti (OCA, Geoazur), and Josiane Zerubia (Inria-SAM) are co-PI of a UCA-AI grant (2020-2021) of UCA-Jedi Idex to fund a postdoc, complementary to the ANR FAULTS R GEMS project.
9 Dissemination
9.1 Promoting scientific activities
9.1.1 Scientific events: organisation
General chair, scientific chair
- Josiane Zerubia is Technical Chair of the conference EUSIPCO`21 in Dublin, Ireland, with Prof. Sabrina Greco and Prof. Stephen McLaughlin.
Member of the organizing committees
- Josiane Zerubia is part of the organizing committee and member of the National Scientific Committee of the ISPRS congress 2020 and 2021, in Nice, France (virtual).
9.1.2 Scientific events: selection
Member of the conference program committees
- Josiane Zerubia was part of the conference program committee of SPIE Remote Sensing’20 (Edinburgh, UK, virtual).
- Josiane Zerubia was part of the conference program comittee of ICDIP'20 (Osaka, Japan, virtual).
Reviewer
- Josiane Zerubia was a reviewer for the conferences IEEE CVPR’20, IEEE ICASSP’20, IEEE EMBC’20, SPIE Remote Sensing’20 and ISPRS Congress 2020.
9.1.3 Journal
Member of the editorial boards
- Josiane Zerubia is a member of the editorial boards of RFPT (1998-2020), IEEE SPMag (2018- ), and Fondation and Trend in Signal Processing (2007- ).
Reviewer - reviewing activities
- Josiane Zerubia was a reviewer for IEEE SPMag.
9.1.4 Invited talks
- As IEEE SPS Distinguished Lecturer, Josiane Zerubia gave in December 2020 a virtual talk in the Silicon Valley invited by Intel Research, HP Labs and IEEE SPS Santa Clara Chapter.
9.1.5 Leadership within the scientific community
- Josiane Zerubia is IEEE (2002- ), EURASIP (2019- ) and IAPR Fellow (2020- )
- Josiane Zerubia is member of the Teaching Board of the Doctoral School STIET at University of Genoa, Italy (2018- )
- Josiane Zerubia is member of the Scientific Council of UCA-JEDI Academy of Excellence 3, dedicated to "Space, Environment, Risks and Resilience" (2015- )
9.1.6 Scientific expertise
- Josiane Zerubia was a reviewer for the Natural Sciences and Engineering Research Council of Canada, NSERC, in 2020.
- Josiane Zerubia was a member of the recruitement committee to hire an Ass. Professor (tenure track) in Computer Vision at University of Luxembourg from February to May 2020.
- Josiane Zerubia is member of the IEEE SPS Awards Board (2020-2022)
9.1.7 Research administration
9.2 Teaching - Supervision - Juries
9.2.1 Teaching
- Masters: Josiane Zerubia , Image Processing Advanced Techniques, 22.5h eq. TD (15h of lectures, no writen exam due to Covid-19 pandemic), ISAE/SUPAERO, France. Josiane Zerubia is also director of this course (total: 30h of lectures). This course was given to third-year students at ISAE/SUPAERO.
- Masters: Josiane Zerubia , Remote Sensing, 13.5h eq. TD (9h of lectures), Master RISKS, Université Côte d'Azur, France. This course was given to Masters students.
9.2.2 Supervision
- Josiane Zerubia is supervising two postdocs, two PhD students and two Master students within the AYANA team, and co-advises an external PhD student at Purdue University, USA
9.2.3 Juries
- Josiane Zerubia was a reviewer of a PhD thesis at Heriot Watt University in Edinburgh, UK, in September 2020
9.3 Popularization
9.3.1 Articles and contents
- Josiane Zerubia was part of a video produced by the communication service of UCA (prize of excellence) in 2020
9.3.2 Education
- Josiane Zerubia participated to (virtual) activities of both Femmes et Sciences and Terra Numerica in 2020.
9.3.3 Interventions
- Josiane Zerubia intervention on "stereotypes in STEM" planned at the Lycée Dumont d'Urville in Toulon at end of March 2020 has been postponed to October 2021, due to Covid-19 pandemic.
10 Scientific production
10.1 Publications of the year
International peer-reviewed conferences
Reports & preprints
10.2 Cited publications
- 5 inproceedings Integrating RJMCMC and Kalman filters for multiple object tracking GRETSI -- Traitement du Signal et des Images Lyon, France September 2015
- 6 inproceedings Joint detection and tracking of moving objects using spatio-temporal marked point processes IEEE Winter Conference on Applications of Computer Vision Hawaii, United States January 2015
- 7 phdthesis Stochastic geometry for automatic multiple object detection and tracking in remotely sensed high resolution image sequences Université Nice Sophia Antipolis November 2015
- 8 articleUnsupervised Deep Slow Feature Analysis for Change Detection in Multi-Temporal Remote Sensing ImagesIEEE Transactions on Geoscience and Remote Sensing57122019, 9976-9992
- 9 inproceedings Feature extraction and tracking of CNN segmentations for improved road detection from satellite imagery ICIP 2019 - IEEE International Conference on Image Processing Taipei, Taiwan IEEE September 2019
- 10 article Code-Aligned Autoencoders for Unsupervised Change Detection in Multimodal Remote Sensing Images arXiv preprint arXiv:2004.07011 2020
- 11 article Deep Image Translation with an Affinity-Based Change Prior for Unsupervised Multimodal Change Detection arXiv preprint arXiv:2001.04271 2020
- 12 inproceedings Causal Markov Mesh Hierarchical Modeling for the Contextual Classification of Multiresolution Satellite Images ICIP 2019 - IEEE International Conference on Image Processing Taipei, Taiwan September 2019
- 13 inproceedings Joint Classification of Multiresolution and Multisensor Data Using a Multiscale Markov Mesh Model IGARSS 2019 - IEEE International Geoscience and Remote Sensing Symposium Yokohama, Japan July 2019
- 14 phdthesis From Satellite Images to Vector Maps INRIA Sophia Antipolis - Méditerranée ; Universite cote d'Azur September 2020