Keywords
Computer Science and Digital Science
- A6.1.5. Multiphysics modeling
- A6.2.1. Numerical analysis of PDE and ODE
- A6.2.4. Statistical methods
- A6.2.5. Numerical Linear Algebra
- A6.2.6. Optimization
- A6.3.1. Inverse problems
- A6.3.3. Data processing
- A6.3.4. Model reduction
- A6.3.5. Uncertainty Quantification
- A6.4.3. Observability and Controlability
Other Research Topics and Application Domains
- B3.1. Sustainable development
- B3.2. Climate and meteorology
- B3.3.1. Earth and subsoil
- B4.3.2. Hydro-energy
- B4.3.3. Wind energy
- B4.3.4. Solar Energy
- B5.1. Factory of the future
- B5.2. Design and manufacturing
- B5.9. Industrial maintenance
- B6.5. Information systems
- B7.2.2. Smart road
- B8.1. Smart building/home
- B8.1.1. Energy for smart buildings
- B8.1.2. Sensor networks for smart buildings
- B8.2. Connected city
1 Team members, visitors, external collaborators
Research Scientists
- Laurent Mevel [Team leader, Inria, Senior Researcher, HDR]
- Xavier Chapeleau [Université Gustave Eiffel, Researcher]
- Enora Denimal [Inria, Researcher, from Oct 2020]
- Michael Doehler [Inria, Researcher]
- Jean Dumoulin [Université Gustave Eiffel, Researcher]
- Qinghua Zhang [Inria, Senior Researcher, HDR]
Post-Doctoral Fellows
- Swarup Mahato [Université Gustave Eiffel, from Feb 2020]
- Boualem Merainani [Inria, from May 2020]
PhD Students
- Ambroise Cadoret [IFPEN, from Oct 2020]
- Alexander Mendler [Université de la Colombie-Britannique - Canada]
- David Pallier [Université Gustave Eiffel , IETR]
- Quentin Sourisseau [Université Gustave Eiffel]
- Eva Viefhues [BAM]
- Bian Xiong [Inria]
Technical Staff
- Szymon Gres [Inria, Engineer, until Mar 2020]
- Ivan Guéguen [Université Gustave Eiffel, Engineer]
- Vincent Le Cam [Université Gustave Eiffel, Engineer]
- Thibaud Toullier [Université Gustave Eiffel, Engineer]
Administrative Assistants
- Hélène de La Ruée [Inria, until Oct 2020]
- Hélène de La Ruée [Univ de Rennes I, from Oct 2020]
Visiting Scientist
- Wentao Tang [Institut de technologie de Harbin - Chine, until May 2020]
2 Overall objectives
2.1 In Summary
The objective of this team is the development of Structural Health Monitoring techniques by intrinsic coupling of statistics and thermo-aeroelastic mixing modeling for the development of robust and autonomous structural health monitoring solutions of mechanical structures. The emphasis of the team is the handling of very large systems such as the recent wind energy converters currently being installed in Europe, building on the expertise acquired by the team on bridges as an example of civil engineering structure, and for aircrafts and helicopters in the context of aero elastic instability monitoring. The necessity of system identification and damage detection systems robust to environmental variations and being designed to handle a very large model dimension motivates us. As examples, the explosion in the installed number of sensors and the robustness to temperature variation will be the main focus of the team. This implies new statistical and numerical technologies as well as improvements on the modeling of the underlying physical models. Many techniques and methods originate from the mechanical community and thus exhibit a very deep understanding of the underlying physics and mechanical behavior of the structure. On the other side, system identification techniques developed within the control community are more related to data modeling and take into account the underlying random nature of measurement noise. Bringing these two communities together is the objective of this joint team between Inria and IFSTTAR. It will results hopefully in methods numerically robust, statistically efficient and also mixing modeling of both the uncertainties related to the data and the associated complex physical models related to the laws of physics and finite element models.
Damage detection in civil structures has been a main focus over the last decade. Still, those techniques need to be matured to be operable and installed on structures in operation, and thus be robust to environmental nuisances. Then, damage localization, quantification and prognosis should be in that order addressed by the team. To be precise and efficient, it requires correct mixing between signal processing, statistical analysis, Finite Elements Models (FEM) updating and a yet to be available precise modeling of the environmental effects such as temperature through 3D field reconstruction.
Theoretical and practical questions are more and more complex. For example, in civil engineering, from handling hundreds of sensors automatically during some long period of time to localize and quantify damage with or without numerical models. Very large heavily instrumented structures are yet to come and they will ask for a paradigm in how we treat them from a renewed point of view. As the structures become large and complex, also the thermal and aeroelastic (among others) models become complex. Bridges and aircrafts are the main focus of our research. Opening our expertise on new applications topics such as helicopters and wind energy converters is also part of our priorities.
2.2 Objectives
The main objectives of the team are first to pursue current algorithmic research activities, in order to accommodate still-to-be-developed complex physical models. More precisely, we want successively
- To develop statistical algorithms robust to noise and variation in the environment
- To handle transient and highly varying systems under operational conditions
- To consider the impact of uncertainties on the currentl available identification algorithms and develop efficient, robust and fast implementation of such quantities
- To consider relevant non trivial thermal models for usage in rejection based structural health monitoring and more generally to mix numerical models, physical modeling and data
- To develop theoretical and software tools for monitoring and localization of damages on civil structures or instability for aircrafts
- To explore new paradigms for handling very large and complex structures heavily instrumented (distributed computing)
- To study the characteristics of the monitored mechanic structures in terms of electromagnetic propagation, in order to develop monitoring methods based on electrical instrumentations.
- To consider society concerns (damage quantification and remaining life prognosis)
2.3 Introduction to physics driven dynamical models in the context of civil engineering elastic structures
The design and maintenance of flexible structures subject to noise and vibrations is an important topic in civil and mechanical engineering. It is an important component of comfort (cars and buildings) and contributes significantly to the safety related aspects of design and maintenance (aircrafts, aerospace vehicles and payloads, long-span bridges, high-rise towers... ). Requirements from these application areas are numerous and demanding.
Detailed physical models derived from first principles are developed as part of system design. These models involve the dynamics of vibrations, sometimes complemented by other physical aspects (fluid-structure interaction, aerodynamics, thermodynamics).
Laboratory and in-operation tests are performed on mock-up or real structures, in order to get so-called modal models, ie to extract the modes and damping factors (these correspond to system poles), the mode shapes (corresponding eigenvectors), and loads. These results are used for updating the design model for a better fit to data, and sometimes for certification purposes (e.g. in flight domain opening for new aircrafts, reception for large bridges).
The monitoring of structures is an important activity for the system maintenance and health assessment. This is particularly important for civil structures. Damaged structures would typically exhibit often very small changes in their stiffness due to the occurrence of cracks, loss of prestressing or post tensioning, chemical reactions, evolution of the bearing behavior and most importantly scour. A key difficulty is that such system characteristics are also sensitive to environmental conditions, such as temperature effects (for civil structures), or external loads (for aircrafts). In fact these environmental effects usually dominate the effect of damage. This is why, for very critical structures such as aircrafts, detailed active inspection of the structures is performed as part of the maintenance. Of course, whenever modal information is used to localize a damage, the localization of a damage should be expressed in terms of the physical model, not in terms of the modal model used in system identification. Consequently, the following elements are encountered and must be jointly dealt with when addressing these applications: design models from the system physics, modal models used in structural identification, and, of course, data from sensors. Corresponding characteristics are given now: Design models are Finite Element models, sometimes with tens or hundreds of thousands elements, depending on professional habits which may vary from one sector to another. These models are linear if only small vibrations are considered; still, these models can be large if medium-frequency spectrum of the load is significant. In addition, nonlinearities enter as soon as large vibrations or other physical effects (aerodynamics, thermodynamics, ..) are considered. Moreover stress-strain paths and therefore the response (and load) history comes into play.
Sensors can range from a handful of accelerometers or strain gauges, to thousands of them, if NEMS ( Nano Electro Mechanical Structures), MEMS (Microelectromechanical systems) or optical fiber sensors are used. Moreover, the sensor output can be a two-dimensional matrix if electro magnet (IR (infrared), SAR, shearography ...) or other imaging technologies are used.
2.4 Multi-fold thermal effects
The temperature constitutes an often dominant load because it can generate a deflection as important as that due to the self-weight of a bridge. In addition, it sometimes provokes abrupt slips of bridge spans on their bearing devices, which can generate significant transient stresses as well as a permanent deformation, thus contributing to fatigue.
But it is also well-known that the dynamic behavior of structures under monitoring can vary under the influence of several factors, including the temperature variations, because they modify the stiffness and thus the modes of vibration. As a matter of fact, depending on the boundary conditions of the structure, possibly uniform thermal variations can cause very important variations of the spectrum of the structure, up to
2.5 Toward a multidisciplinary approach
Unlike previously mentioned blind approaches, successful endeavours to eliminate the temperature from subspace-based damage detection algorithms prove the relevance of relying on predictive thermo-mechanical models yielding the prestress state and associated strains due to temperature variations. As part of the CONSTRUCTIF project supported by the Action Concertée Incitative Sécurité Informatique of the French Ministry for Education and Research, very encouraging results in this direction were obtained and published. They were substantiated by laboratory experiments of academic type on a simple beam subjected to a known uniform temperature. Considering the international pressure toward reliable methods for thermal elimination, these preliminary results pave the ground to a new SHM paradigm. Moreover, for one-dimensional problems, it was shown that real time temperature identification based on optimal control theory is possible provided the norm of the reconstructed heat flux is properly chosen. Finally, thermo-mechanical models of vibrating thin structures subject to thermal prestress, prestrain, geometric imperfection and damping have been extensively revisited. This project led by Inria involved IFSTTAR where the experiments were carried out. The project was over in July 2006. Note that thermo-mechanics of bridge piles combined with an ad hoc estimation of thermal gradients becomes of interest to practicing engineers. Thus, I4S's approach should suit advanced professional practice. Finite element analysis is also used to predict stresses and displacements of large bridges in Hong-Kong bay .
Temperature rejection is the primary focus and challenge for I4S's SHM projects in civil engineering, like SIMS project in Canada, ISMS in Danemark or SIPRIS in France.
A recent collaboration between Inria and IFSTTAR has demonstrated the efficiency of reflectometry-based methods for health monitoring of some civil engineering structures, notably external post-tensioned cables. Based on a mathematical model of electromagnetic propagation in mechanical structures, the measurement of reflected and transmitted electromagnetic waves by the monitored structures allows to detect structural failures. The interaction of such methods with those based on mechanical and thermal measurements will reinforce the multidisciplinary approach developed in our team.
2.6 Models for monitoring under environmental changes - scientific background
We will be interested in studying linear stochastic systems, more precisely, assume at hand a sequence of observations
where
1/ identify and characterize the structure of interest. It may be possible by matching a parametric model to the observed time series
2/ decide if the measured data describe a system in a so called "reference" state (the term "reference" is used in the context of fault detection, where the reference is considered to be safe) and monitor its deviations with respect f its nominal reference state.
Both problems should be addressed differently if
1/ we consider that the allocated time to measurement is large enough, resulting in a sequence of
2/ we are interested in systems, whose dynamic is fast with respect to the sampling rate, most often asking for reaction in terms of seconds. It is, for example, the case for mission critical applications such as in-flight control or real-time security and safety assessment. Both aeronautics and transport or utilities infrastructures are concerned. In this case, fast algorithms with sample-by-sample reaction are necessary.
The monitoring of mechanical structures can not be addressed without taking into account the close environment of the considered system and their interactions. Typically, monitored structures of interest do not reside in laboratory but are considered in operational conditions, undergoing temperature, wind and humidity variations, as well as traffic, water flows and other natural or man-made loads. Those variations do imply a variation of the eigenproperties of the monitored structure, variations to be separated from the damage/instability induced variations.
For example, in civil engineering, an essential problem for in-operation health monitoring of civil structures is the variation of the environment itself. Unlike laboratory experiments, civil structure modal properties change during time as temperature and humidity vary. Traffic and comparable transient events also influence the structures. Thus, structural modal properties are modified by slow low variations, as well as fast transient non stationarities. From a damage detection point of view, the former has to be detected, whereas the latter has to be neglected and should not perturb the detection. Of course, from a structural health monitoring point of view the knowledge of the true load is itself of paramount importance.
In this context, the considered perturbations will be of two kinds, either
1/ the influence of the temperature on civil structures, such as bridges or wind energy converters : as we will notice, those induced variations can be modeled by a additive component on the system stiffness matrix depending on the current temperature, as
We will then have to monitor the variations in
2/ the influence of the aeroelastic forces on aeronautical structures such as aircrafts or rockets and on flexible civil structures such as long-span bridges : we will see as well that this influence implies a modification of the classical mechanical equation (2)
where
Most of the research at Inria for a decade has been devoted to the study of subspace methods and how they handle the problems described above.
Model (2) is characterized by the following property (we formulate it for the single sensor case, to simplify notations): Let
There are numerous ways to implement those methods. This approach has seen a wide acceptance in the industry and benefits from a large background in the automatic control literature. Up to now, there was a discrepancy between the a priori efficiency of the method and some not so efficient implementations of this algorithm. In practice, for the last ten years, stabilization diagrams have been used to handle the instability and the weakness with respect to noise, as well as the poor capability of those methods to determine model orders from data. Those methods implied some engineering expertise and heavy post processing to discriminate between models and noise. This complexity has led the mechanical community to adopt preferably frequency domain methods such as Polyreference LSCF. Our focus has been on improving the numerical stability of the subspace algorithms by studying how to compute the least square solution step in this algorithm. This yields to a very efficient noise free algorithm, which has provided a renewed acceptance in the mechanical engineering community for the subspace algorithms. Now we focus on improving speed and robustness of those algorithms.
Subspace methods can also be used to test whether a given data set conforms a model: just check whether this property holds, for a given pair {data, model}. Since equality holds only asymptotically, equality must be tested against some threshold
In oder to discriminate between damage and temperature variations, we need to monitor the variations in
This approach has been used also for flutter monitoring in Rafik Zouari's PhD thesis for handling the aeroelastic effect.
3 Research program
3.1 Vibration analysis
In this section, the main features for the key monitoring issues, namely identification, detection, and diagnostics, are provided, and a particular instantiation relevant for vibration monitoring is described.
It should be stressed that the foundations for identification, detection, and diagnostics, are fairly general, if not generic. Handling high order linear dynamical systems, in connection with finite elements models, which call for using subspace-based methods, is specific to vibration-based SHM. Actually, one particular feature of model-based sensor information data processing as exercised in I4S, is the combined use of black-box or semi-physical models together with physical ones. Black-box and semi-physical models are, for example, eigenstructure parameterizations of linear MIMO systems, of interest for modal analysis and vibration-based SHM. Such models are intended to be identifiable. However, due to the large model orders that need to be considered, the issue of model order selection is really a challenge. Traditional advanced techniques from statistics such as the various forms of Akaike criteria (AIC, BIC, MDL, ...) do not work at all. This gives rise to new research activities specific to handling high order models.
Our approach to monitoring assumes that a model of the monitored system is available.
This is a reasonable assumption, especially within the SHM areas.
The main feature of our monitoring method is its intrinsic ability
to the early warning of small deviations of a system with respect
to a reference (safe) behavior under usual operating
conditions, namely without any artificial excitation or other external action.
Such a normal behavior is summarized in a reference parameter vector
3.2 Identification
The behavior of the monitored continuous system is assumed to be described by
a parametric model
For reasons closely related to the vibrations monitoring applications,
we have been investigating subspace-based methods, for both the identification
and the monitoring of the eigenstructure
namely the
The (canonical) parameter vector in that case is :
where
Subspace-based methods is the generic name for linear systems identification algorithms based on either time domain measurements or output covariance matrices, in which different subspaces of Gaussian random vectors play a key role 49.
Let
be the output covariance and Hankel matrices, respectively; and:
where:
are the observability and controllability matrices, respectively.
The observation matrix
Since the actual model order is generally not known, this procedure is run with increasing model orders.
3.3 Detection
Our approach to on-board detection is based on the so-called asymptotic statistical local approach. It is worth noticing that these investigations of ours have been initially motivated by a vibration monitoring application example. It should also be stressed that, as opposite to many monitoring approaches, our method does not require repeated identification for each newly collected data sample.
For achieving the early detection of small deviations with respect to the normal behavior,
our approach generates, on the basis of the reference parameter vector
- The early detection of a slight mismatch between the model and the data;
- A preliminary diagnostics and localization of the deviation(s);
- The tradeoff between the magnitude of the detected changes and the uncertainty resulting from the estimation error in the reference model and the measurement noise level.
These indicators are computationally cheap, and thus can be embedded. This is of particular interest in some applications, such as flutter monitoring.
Choosing the eigenvectors of matrix
where
This property can be checked as follows. From the nominal
Matrix
Residual associated with subspace identification.
Assume now that a reference
and to define the residual vector:
Let
As in most fault detection approaches, the key issue is to design a residual, which is ideally close to zero under normal operation, and has low sensitivity to noises and other nuisance perturbations, but high sensitivity to small deviations, before they develop into events to be avoided (damages, faults, ...). The originality of our approach is to :
- Design the residual basically as a parameter estimating function,
- Evaluate the residual thanks to a kind of central limit theorem, stating that the residual is asymptotically Gaussian and reflects the presence of a deviation in the parameter vector through a change in its own mean vector, which switches from zero in the reference situation to a non-zero value.
The central limit theorem shows 43 that the residual is asymptotically Gaussian :
where the asymptotic covariance matrix
where
3.4 Diagnostics
A further monitoring step, often called fault isolation,
consists in determining which (subsets of) components
of the parameter vector
The question: which (subsets of) components of
In most SHM applications, a complex physical system, characterized by a generally
non identifiable parameter vector
The isolation methods sketched above are possible solutions to the former. Our approach to the latter diagnosis problem is basically a detection approach again, and not a (generally ill-posed) inverse problem estimation approach.
The basic idea is to note that the physical sensitivity matrix writes
It should be clear that the selection of a particular parameterization
3.5 Infrared thermography and heat transfer
This section introduces the infrared radiation and its link with the temperature, in the next part different measurement methods based on that principle are presented.
3.5.1 Infrared radiation
Infrared is an electromagnetic radiation having a wavelength between
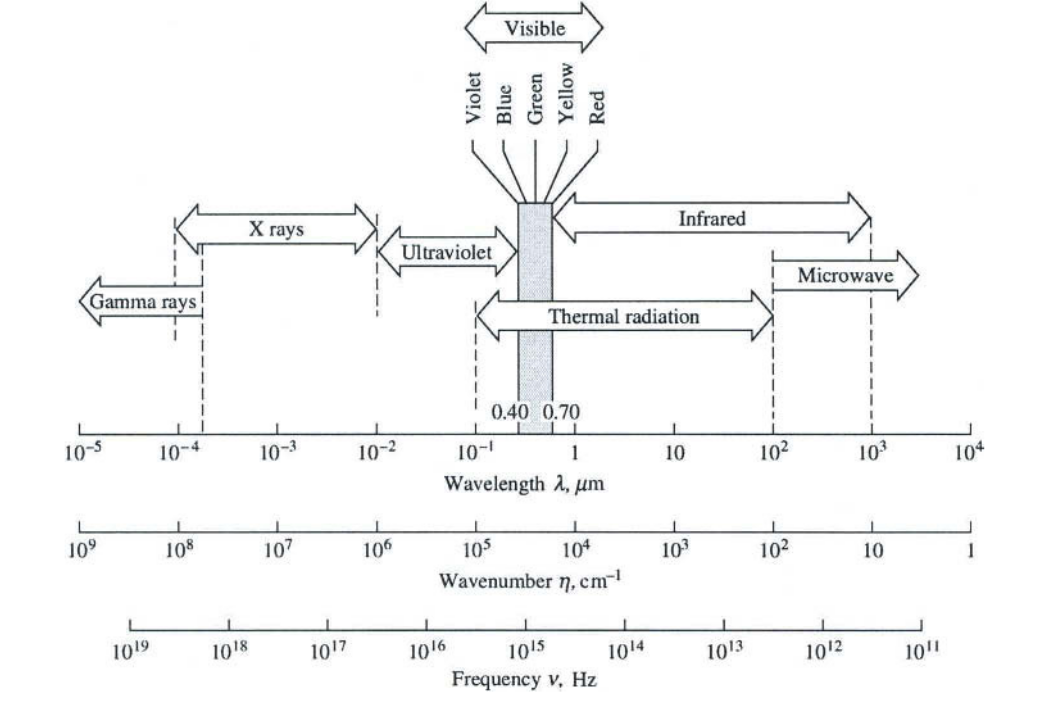
For scientific purposes, infrared can be divided in three ranges of wavelength in which the application varies, see Table 1.
Band name | wavelength |
Uses |
Near infrared (PIR, IR-A, NIR) |
|
Reflected solar heat flux |
Mid infrared (MIR, IR-B) |
|
Thermal infrared |
Far infrared (LIR, IR-C, FIR) |
|
Astronomy |
Our work is concentrated in the mid infrared spectral band. Keep in mind that Table 1 represents the ISO 20473 division scheme, in the literature boundaries between bands can move slightly.
The Plank's law, proposed by Max Planck in 1901, allows to compute the black body emission spectrum for various temperatures (and only temperatures), see Figure 2 left. The black body is a theoretical construction, it represents perfect energy emitter at a given temperature, cf. Equation (20).
With
with
-
, the electromagnetic wave speed (in vacuum is the light speed in m.s ). -
J.K The Boltzmann (Entropy definition from Ludwig Boltzmann 1873). It can be seen as a proportionality factor between the temperature and the energy of a system. -
J.s The Plank constant. It is the link between the photons energy and their frequency.
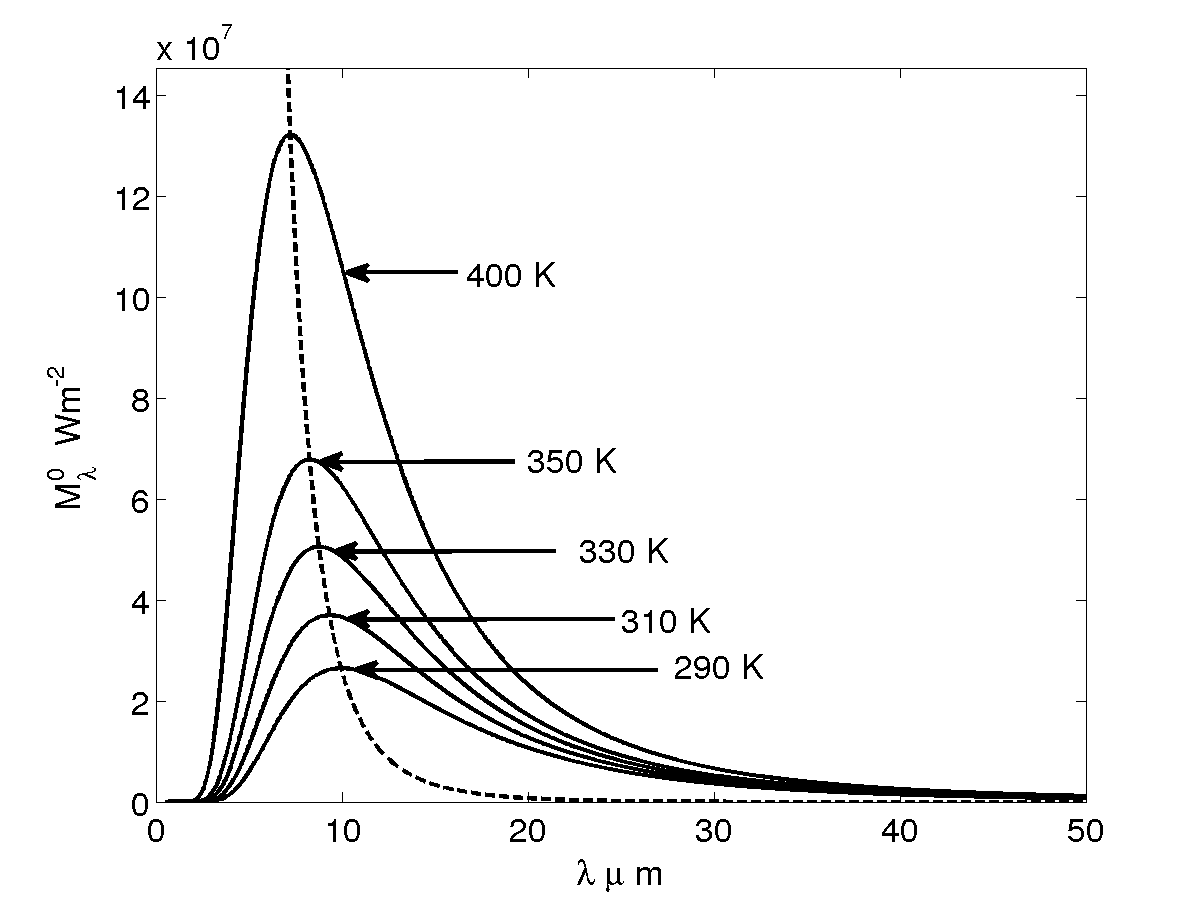
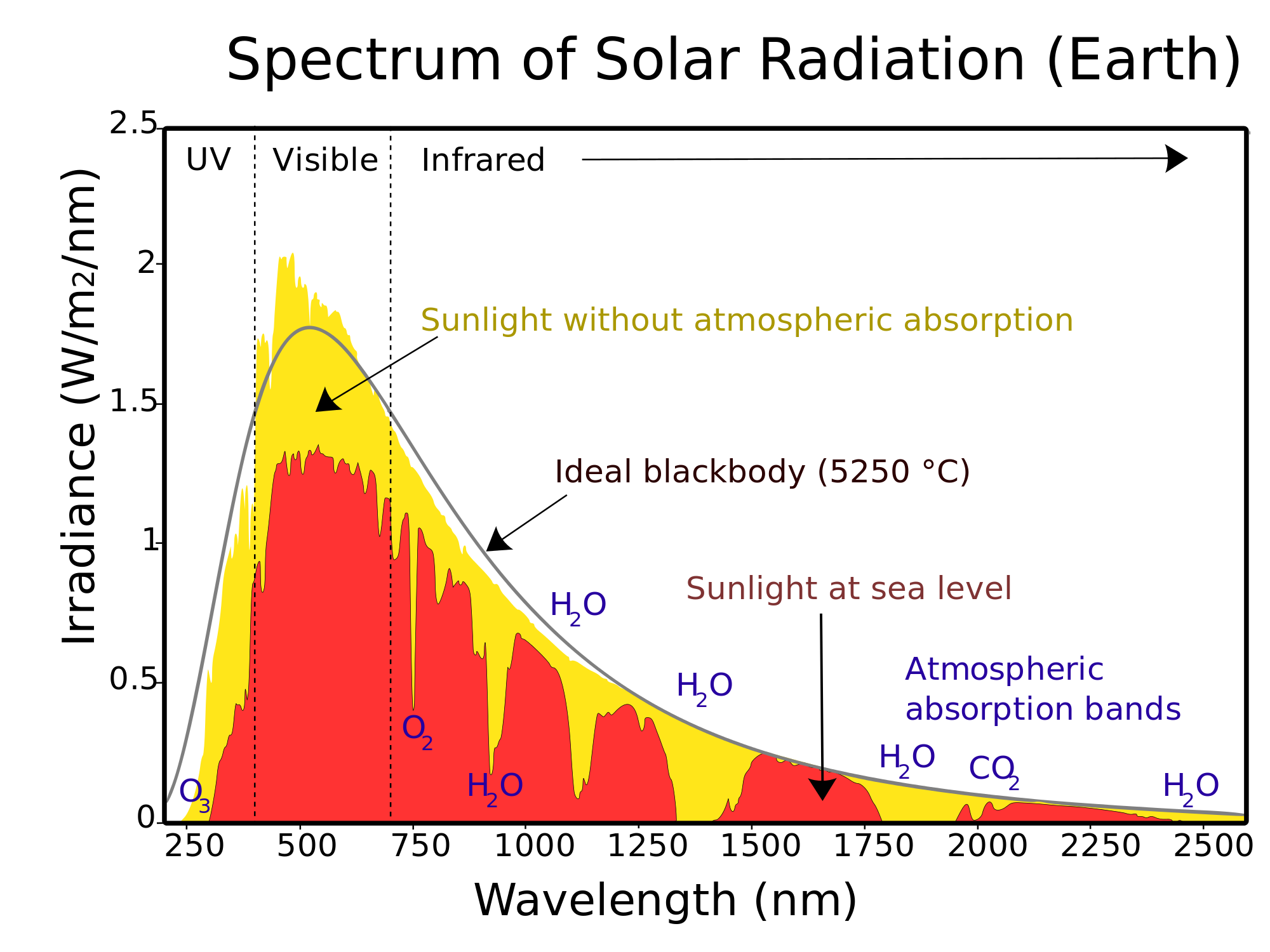
By generalizing the Plank's law with the Stefan Boltzmann law (proposed first in 1879 and then in 1884 by Joseph Stefan and Ludwig Boltzmann), it is possible to address mathematically the energy spectrum of real body at each wavelength depending on the temperature, the optical condition and the real body properties, which is the base of the infrared thermography.
For example, Figure 2 right presents the energy spectrum of the atmosphere at various levels, it can be seen that the various properties of the atmosphere affect the spectrum at various wavelengths. Other important point is that the infrared solar heat flux can be approximated by a black body at 5523,15 K.
3.5.2 Infrared Thermography
The infrared thermography is a way to measure the thermal radiation received from a medium. With that information about the electromagnetic flux, it is possible to estimate the surface temperature of the body, see section 3.5.1. Various types of detector can assure the measure of the electromagnetic radiation.
Those different detectors can take various forms and/or manufacturing process. For our research purposes, we use uncooled infrared camera using a matrix of microbolometers detectors. A microbolometer, as a lot of transducers, converts a radiation in electric current used to represent the physical quantity (here the heat flux).
This field of activity includes the use and the improvement of vision system, like in 7.
3.6 Heat transfer theory
Once the acquisition process is done, it is useful to model the heat conduction inside the cartesian domain
Where
An energy balance with respect to the first principle yields to the expression of the heat conduction in all point of the domain
With
To solve the system (3.6), it is necessary to express the boundaries conditions of the system. With the developments presented in section 3.5.1 and the Fourier's law, it is possible, for example, to express the thermal radiation and the convection phenomenon which can occur at
Equation (3.6) is the so called Robin condition on the boundary
The systems presented in the different sections above (3.5 to 3.6) are useful to build physical models in order to represents the measured quantity. To estimate key parameters, as the conductivity, model inversion is used, the next section will introduce that principle.
3.7 Inverse model for parameter estimation
Lets take any model
With
Here we want to find the solution
To do that it is important to respect the well posed condition established by Jacques Hadamard in 1902
- A solution exists.
- The solution is unique.
- The solution's behavior changes continuously with the initial conditions.
Unfortunately those condition are rarely respected in our field of study. That is why we dont solve directly the system (25) but we minimise the quadratic coast function (26) which represents the Legendre-Gauss least square algorithm for linear problems.
Where
In some cases the problem is still ill-posed and need to be regularized for example using the Tikhonov regularization. An elegant way to minimize the cost function
Where
Until now the inverse method proposed is valid only when the model
Equation (28) is solved iteratively at each loop
3.8 Reflectometry-based methods for electrical engineering and for civil engineering
The fast development of electronic devices in modern engineering systems involves more and more connections through cables, and consequently, with an increasing number of connection failures. Wires and connectors are subject to ageing and degradation, sometimes under severe environmental conditions. In many applications, the reliability of electrical connexions is related to the quality of production or service, whereas in critical applications reliability becomes also a safety issue. It is thus important to design smart diagnosis systems able to detect connection defects in real time. This fact has motivated research projects on methods for fault diagnosis in this field. Some of these projects are based on techniques of reflectometry, which consist in injecting waves into a cable or a network and in analyzing the reflections. Depending on the injected waveforms and on the methods of analysis, various techniques of reflectometry are available. They all have the common advantage of being non destructive.
At Inria the research activities on reflectometry started within the SISYPHE EPI several years ago and now continue in the I4S EPI. Our most notable contribution in this area is a method based on the inverse scattering theory for the computation of distributed characteristic impedance along a cable from reflectometry measurements 14, 11, 48. It provides an efficient solution for the diagnosis of soft faults in electrical cables, like in the example illustrated in Figure 3. While most reflectometry methods for fault diagnosis are based on the detection and localization of impedance discontinuity, our method yielding the spatial profile of the characteristic impedance is particularly suitable for the diagnosis of soft faults with no or weak impedance discontinuities.
Fault diagnosis for wired networks have also been studied in Inria 50, 46. The main results concern, on the one hand, simple star-shaped networks from measurements made at a single node, on the other hand, complex networks of arbitrary topological structure with complete node observations.
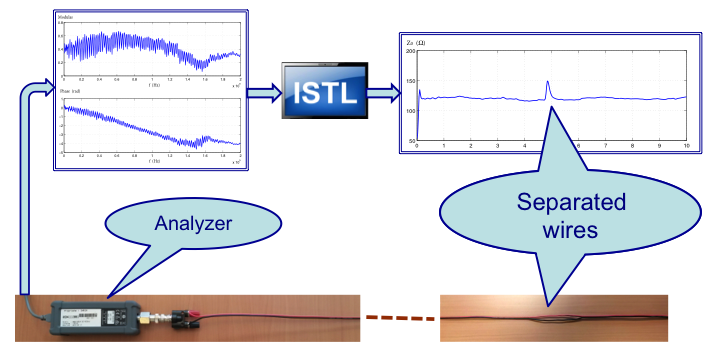
Though initially our studies on reflectometry were aiming at applications in electrical engineering, since the creation of the I4S team, we are also investigating applications in the field of civil engineering, by using electrical cables as sensors for monitoring changes in mechanical structures.
What follows is about some basic elements on mathematical equations of electric cables and networks, the main approach we follow in our study, and our future research directions.
3.8.1 Mathematical model of electric cables and networks
A cable excited by a signal generator can be characterized by the telegrapher's equations 47
where
At the right end of the cable (corresponding to
One way for deriving the above model is to spatially discretize the cable and to characterize each small segment with 4 basic lumped parameter elements for the
A wired network is a set of cables connected at some nodes, where loads and sources can also be connected. Within each cable the current and voltage satisfy the telegrapher's equations, whereas at each node the current and voltage satisfy the Kirchhoff's laws, unless in case of connector failures.
3.8.2 uid76The inverse scattering theory applied to cables
The inverse scattering transform was developed during the 1970s-1980s for the analysis of some nonlinear partial differential equations 45. The visionary idea of applying this theory to solving the cable inverse problem goes also back to the 1980s 44. After having completed some theoretic results directly linked to practice 14, 48, we started to successfully apply the inverse scattering theory to cable soft fault diagnosis, in collaboration with GEEPS-SUPELEC 11.
To link electric cables to the inverse scattering theory, the telegrapher's equations
are transformed in a few steps to fit into a particular form studied in the inverse scattering theory. The Fourier transform is first applied to obtain a frequency domain model,
the spatial coordinate
and the frequency domain variables
with
These transformations lead to the Zakharov-Shabat equations
with
These equations have been well studied in the inverse scattering theory, for the purpose of determining partly the “potential functions”
4 Application domains
Civil engineering:
- Vibration-based damage diagnosis
- Thermal monitoring for non-destructive evaluation
- Energy assessment of buildings
- Railway monitoring
Aeronautics:
- In-flight monitoring - flutter detection
- Ground resonance detection for helicopters
Electrical cables and networks:
- Incipient fault detection
5 Highlights of the year
The article “Detection and Measurement of Matrix Discontinuities in UHPFRC by Means of Distributed Fiber Optics Sensing“, co-authored by our PhD student Antoine Bassil and his supervisor Xavier Chapeleau has made it to the cover of the journal Sensors in the July 2020 issue. The research work has been performed during his secondment at EPFL in Lausanne, Switzerland, within the H2020 project INFRASTAR.
6 New results
6.1 System identification
6.1.1 Set-membership estimation for linear time-varying descriptor systems
Participants: Qinghua Zhang.
This work considers the problem of set-membership estimation for discrete-time linear time-varying descriptor systems subject to unknown but bounded disturbance and noise. We propose a set-membership estimation method based on a descriptor system observer and a zonotopic estimator of the observer error bounds. The observer parameters are optimized in order to minimize the sizes of the zonotopes enclosing all admissible state trajectories. Finally, simulation examples are provided to demonstrate the effectiveness of the proposed method. This work has been done in collaboration with a group headed by Zhenhua Wang in Harbin Institute of Technology. The results have been published in 24.
6.1.2 Transforming Time-Delay System Observers to Adaptive Observers
Participants: Qinghua Zhang.
For joint estimation of states and parameters in timevarying time-delay systems (TDS) involving both distributed and lumped time delays, a general approach is proposed in this work to transforming existing (non adaptive) observers to adaptive observers. In addition to the convergence conditions of the considered existing observers, a persistent excitation condition is introduced in order to ensure the convergence of parameter estimation. In contrast to implicitly formulated convergence conditions, which are usually assumed jointly for both state and parameter estimations in most TDS adaptive observers, the persistent excitation condition in the proposed approach is explicitly formulated and decoupled from the conditions initially assumed for state estimation. This work has been done in collaboration with Tarek Ahmed-Ali and Fouad Giri from Normandie University, and with Xingwen Liu from Southwest Minzu University. The results have been presented at 26.
6.1.3 Ellipsoid bundle and its application to set-membership estimation
Participants: Qinghua Zhang.
This work studies set-membership estimation for discrete linear time-varying systems subject to unknown disturbance and noise, which are bounded by ellipsoids. To improve the existing ellipsoid-based set-membership estimation methods, we propose a new set representation tool, called ellipsoid bundle, which combines the advantages of ellipsoids and zonotopes for uncertainty set representation and computation. Then, ellipsoidal bundles are used to design a new set-membership estimation method. This work has been done in collaboration with a group headed by Zhenhua Wang in Harbin Institute of Technology. The results have been presented at 30.
6.1.4 Fast interval estimation for discrete-time systems based on fixed-time convergence
Participants: Qinghua Zhang.
This work studies interval estimation for discrete-time linear systems with unknown but bounded disturbance and measurement noise. Inspired by the well-known parity space approach in the field of fault diagnosis, we propose a fast interval estimation method with fixed-time convergence property. A singular value decomposition-based parameter optimization algorithm is used to attenuate the effect of uncertainties on the estimation error. A comparison study illustrates the superiority of the proposed method over existing techniques. This work has been done in collaboration with a group headed by Zhenhua Wang in Harbin Institute of Technology and with Tarek Raïssi from Conservatoire National des Arts et Métiers. The results have been presented at 38.
6.1.5 Boundedness of the Kitanidis Filter for Optimal Robust State Estimation
Participants: Qinghua Zhang.
The Kitanidis filter is a natural extension of the Kalman filter to systems subject to arbitrary disturbances or unknown inputs. Though the optimality of the Kitanidis filter was founded for general time varying systems more than 30 years ago, its stability analysis is still limited to time invariant systems, to the author's knowledge. In the framework of general time varying systems, this work establishes upper bounds for the error covariance of the Kitanidis filter and for all the auxiliary variables involved in the filter. These results have been presented at 35.
6.1.6 Variance computation for system matrices and transfer function from input/output subspace system identification
Participants: Szymon Gres, Michael Doehler, Laurent Mevel.
The transfer function of a linear system is defined in terms of the quadruplet of matrices (A, B, C, D) that can be identified from input and output measurements. Similarly these matrices determine the state space evolution for the considered dynamical system. Estimation of the quadruplet has been well studied in the literature from both theoretical and practical points of view. Nonetheless, the uncertainty quantification of their estimation errors has been mainly discussed from a theoretical viewpoint. For several output-only and input/output subspace methods, the variance of the (A, C) matrices can be effectively obtained with recently developed first-order perturbation-based schemes. This paper addresses the estimation of the (B, D) matrices, and the remaining problem of the effective variance computation of their estimates and the resulting transfer function. The proposed schemes are validated on a simulation of a mechanical system. These results have been presented in 27.
6.2 Damage detection and localization
6.2.1 Determination of structural and damage detection system influencing parameters on the value of information
Participants: Michael Doehler.
A method to determine the influencing parameters of a structural and Damage Detection System (DDS) is proposed based on the Value of Information (VoI) analysis. The VoI analysis utilizes the Bayesian pre-posterior decision theory to quantify the value of DDS for the structural integrity management during service life. With the developed approach, a case study on a statically determinate Pratt truss bridge girder is carried out to validate the method. The method has been published in 19.
6.2.2 Minimum Detectable Damage as an Optimization Criterion for Performance-based Sensor Placement
Participants: Michael Doehler, Laurent Mevel, Alexander Mendler.
This paper 29 introduces an optimization criterion that ensures that a minimum damage extent can be detected in each structural component while maintaining a probability of detection that meets the national design standards. The presented strategy helps to find an acceptable number of sensors as well as their optimal layout. Further advantages are that the optimization can be done based on the simulated vibration data from the healthy structure and that the criterion can be tuned so the resulting sensor layout becomes more sensitive to damage hotpots.
6.2.3 Fault detection for linear parameter varying systems under changes in the process noise covariance
Participants: Michael Doehler, Laurent Mevel, Eva Viefhues.
In this paper 33, linear output-only systems depending on a varying external physical parameter are considered. These systems are driven by process noise, whose covariance may also vary between measurements. To deal with the varying parameter, an interpolation approach is pursued, where a limited number of reference models are interpolated to approximate an adequate reference model for the current parameter. The resulting approach is capable of change detection when both the external physical parameter and the process noise conditions are varying.
6.2.4 Clustering of Redundant Parameters for Fault Isolation with Gaussian Residuals
Participants: Michael Doehler, Laurent Mevel, Alexander Mendler.
The effectiveness of the fault diagnosis by Gaussian residuals depends on the model quality, but an increasing number of model parameters also leads to redundancies which, in turn, can distort the fault isolation. This paper 28 proposes a framework to find the optimal parameter clusters for such problems. It explains how the optimal solution is a compromise, because with an increasing number of clusters, the fault isolation resolution increases, but the detectability in each cluster decreases, and the number of false alarms changes. To assess these factors during the clustering process, criteria for the minimum detectable change and the false-alarm susceptibility are introduced and evaluated in an optimization scheme.
6.3 Infrared Thermography
6.3.1 Long term Thermal Mpnitoring by Standard Passive Infrared thermography
Participants: Thibaud Toullier, Jean Dumoulin, Laurent Mevel.
The study in 37 addresses the development of a software for the simulation of radiative exchanges in in-situ 3D scenes. By using the progressive radiosity method, implemented on a graphic card to improve the computation speed, a direct solution to the radiative transfer equation is proposed. The software integrates the SMARTS2 atmospheric model to take into account the solar irradiance. Moreover, a camera model has been implemented to simulate the acquisition chain from the camera’s irradiance to the digital signal. To validate the software, a comparison between simulated data and actual measurements is proposed. Finally, prospects for extending the software are presented.
6.3.2 Simultaneous estimation of temperature and emissivity in Infra-Red imaging
Participants: Thibaud Toullier, Jean Dumoulin, Laurent Mevel.
Temperature estimation through infrared thermography is facing the lack of knowledge of the observed material’s emissivity. The derivation of the physical equations lead to an ill-posed problem. A new Kriged Interacting Particle Kalman Filter is proposed. A state space model relates the measurements to the temperature and the Kalman filter equations yield a filter tracking the temperature over time. Moreover, a particle filter associated to Kriging prediction is interacting with a bank of Kalman filters to estimate the time-varying parameters of the system. The efficiency of the algorithm is tested on a simulated sequence of infrared thermal images. The work has been presented in 32 and 31
6.4 Sensor and hardware based research
6.4.1 Concrete Crack Monitoring Using a Novel Strain Transfer Model for Distributed Fiber Optics Sensors
Participants: Xavier Chapeleau, Antoine Bassil.
In this paper, we study the strain transfer mechanism between a host material and an optical fiber. A new analytical model handling imperfect bonding between layers is proposed. A general expression of the crack-induced strain transfer from fractured concrete material to optical fiber is established in the case of a multilayer system. This new strain transfer model is examined through performing wedge splitting tests on concrete specimens instrumented with embedded and surface-mounted fiber optic cables. The experimental results showed the validity of the crack-induced strain expression fitted to the distributed strains measured using an Optical Backscattering Reflectometry (OBR) system. This work has been published in 16.
6.4.2 Detection and Measurement of Matrix Discontinuities in UHPFRC by Means of Distributed Fiber Optics Sensing
Participants: Xavier Chapeleau, Antoine Bassil.
Following the significant improvement in their properties during the last decade, Distributed Fiber Optics sensing (DFOs) techniques are nowadays implemented for industrial use in the context of Structural Health Monitoring (SHM). In this study, a full scale UHPFRC beam was instrumented with DFOs, Digital Image Correlation (DIC) and extensometers. The performances of these three measurement techniques in terms of strain measurement as well as crack detection and localization are compared. A method for the measurement of opening and closing of localized fictitious cracks in UHPFRC using the Optical Backscattering Reflectometry (OBR) technique is verified. Moreover, the use of correct combination of DFO sensors allows precise detection of microcracks as well as monitoring of fictitious cracks’ opening. This work has been published in 23.
6.4.3 Detection of Multiple Cracks in Four-Point Bending Tests Using the Coda Wave Interferometry Method
Participants: Xavier Chapeleau, Antoine Bassil.
In 25, the assessment of the CWI method applied for multiple cracks opening detection on two specimens based on four-point bending test is presented. Both beams were monitored using a limited number of embedded Ultrasonic (US) transducers as well as other transducers and techniques (e.g., Digital Image Correlation (DIC), LVDT sensors, strain gauges, and Fiber Optics Sensor (FOS)). Results show that strain change and crack formation are successfully and efficiently detected by CWI method even earlier than by the other techniques.
6.4.4 Energy-efficient GPS synchronization for wireless nodes
Participants: David Pallier, Vincent Le Cam.
Synchronization is a challenging problem for wireless nodes, especially for applications demanding good synchronization accuracy over wide areas. In that case, the GPS is a valuable solution as the nodes can independently synchronize to UTC. However, the energy consumption of a GPS receiver (over 100 mW when switched on) is not sustainable on a wireless node. Therefore, in this work, we developed a synchronization scheme based on periodic extinctions of the GPS receiver. The goal is to study the GPS power switching effect on the synchronization accuracy. This work has been published in 21.
7 Bilateral contracts and grants with industry
7.1 Bilateral contracts with industry
Collaboration with SNCF on Road circuits
Participants: Vincent Le Cam, Arthur Bouché.
The 2 objectives of the Circuit de Voie project aimed to detect the phenomenon of deshuntage. With SNCF Innovation Research, we have developed criteria and models to detect, in real time, the appearance of the phenomenon. We have implemented on several PEGASE boxes these models and comparison indicators. As planned, in 2020, the team has concentrated its effort in analyzing the default signals issued from Road Circuit box. We have proposed some new criteria to SNCF based on a statistical model to detect in real time a Road Circuit dysfunctionning. A special delivrable with a huge analysis has been sent to SNCF and is actually under their own analyze. A specific database of good and defect signals has also been provided to SNCF.
Collaboration with SNCF Reseau
Participants: Vincent Le Cam, Arthur Bouché.
SNCF has commissioned 5 new DETECTEAU water level sensors adapted to the conditions of nozzles and waterways in the rail network. From a technological point of view the sensor is of small size and very weak consumption. DETECTEAU communicates according to the LORA network. As planned with SNCF RESEAU : new Railways sites have been instrumented by the detecteau SENSORS and are actually operational to monitor critical level of water under Railways.
Collaboration with SNCF : Hot boxes detection
Participants: Jean Dumoulin, Thibaud Toullier.
The main strategic issue is the maintenance in operational condition of the Hot Box Detectors (DBC). The removal of the DBC from the track is part of Tech4Rail's ambition: reducing equipment to the track. The innovation aimed at in this project is to study and develop a measurement solution to be deployed at the edge of a lane out of danger zone and independent of track equipment. Among the scientific obstacles identified are the following three:
- the behavior of the measurement system in deteriorated meteorological conditions in a real site
- the design and implementation of an automated prototype for in-situ deployment (connection to an existing announcement system, hardware packaging of the system, study and design of a scalable software solution allowing pre-processing data).
- the development of automatic processing tools for the analysis of massive data generated by in-situ measurement systems
7.1.1 Contract with SIEMENS : Poof of Concept monitoring coupled with prediction model for de-icing metro lane surface
Participants: Jean Dumoulin, Nicolas Le Touz, Thibaud Toullier.
This proof of concept aims at combining real site monitoring solutions with adjoint state FE thermal model approach to predict optimal heating required to preserve surface from icing in winter conditions. Furthermore, we introduced in our prediction model connection with in-line weather forecast provided by Meteo France Geoservice at different time horizons and spatial scales.
7.1.2 Contract with Bureau Veritas : FPSO structures monitoring
Participants: Xavier Chapeleau, Quentin Sourisseau.
The maintenance of steel structure installed in harsh offshore environment (like tropical areas), is a great challenge. Vessels and mobile offshore units can be maintained and repaired onshore in shipyards but, fixed units, such as platforms or FPSO (Floating Production, Storage and Offloading) structures, do not come back in dry dock and shall be maintained at sea. On FPSO units/structures, the corrosion is a permanent threat due to high temperature and high humidity conditions. The development of new techniques to repair those decks are actually in development, more particularly bonded repair solution which presents several advantages (short down-time and non-intrusively process). For this reason, the JIP StrengthBond Offshore project initiated by Bureau Veritas Marine and Offshore (BV) has been launched in March 2019 with the partners Total, Petrobras, Naval Group, Siemens, Infra-core and Coldpad. The project aims to achieve the following main objectives:
- Enable a better evaluation of the margin between the actual strength of a repair and the design load,
- Validate the characterization procedure for strength prediction of bonded assembly,
- Define a robust strength prediction method,
- Standardize the qualification process for offshore composite bonded repairs.
In this project involving the laboratories UGE/MAST/SMC and UGE/COSYS/SII-I4S, the PhD student (Quentin Sourisseau) studies different characterization strategies for composite reinforcements bonded to metal structures focusing on fracture mechanics methods. On the basis of experimental and modelling works, it should enable to improve current methodologies. During the first year of the PhD student, innovative methods of monitoring based on DIC (Digital Image Correlation) and DFOS (Distributed Fiber Optic Sensors) were used to estimate the crack length during the tests.
8 Partnerships and cooperations
8.1 International initiatives
8.1.1 Collaboration with University of British Columbia, Canada
Participants: Laurent Mevel, Michael Doehler, Alexander Mendler.
Alexander Mendler's PhD thesis started in September 2018 co-supervised by M. Doehler and C. Ventura. A. Mendler thesis was defended in December 2020.
8.1.2 Collaboration with BAM, Germany
Participants: Laurent Mevel, Michael Doehler, Eva Viefhues.
Eva Viefhues is currently PhD student of Laurent Mevel and Michel Doehler in Berlin, financed by BAM. M. Doehler is also associate researcher at BAM since 2016.
8.1.3 Participation in other international programs
The joint lab ASTI between Inria, University Gustave Eiffel and CNR has been approved and the letters of intent have been signed by all partners.
8.2 European initiatives
DESDEMONA(DEtection of Steel Defects by Enhanced MONitoring and Automated procedure for self-inspection and maintenance)
Participants: Jean Dumoulin, Laurent Mevel, Michael Doehler, Xavier Chapeleau, Qinghua Zhang, Boualem Merainani, Thibaud Toullier, Bian Xiong.
- Call: H2020 -Call: RFCS-2017 (Call of the research programme of the Research Fund for Coal and Steel - 2017)
- Type of Action: RFCS-RPJ (Research project)
- Objective: DESDEMONA objective is the development of novel design methods, systems, procedure and technical solution, to integrate sensing and automation technologies for the purpose of self-inspection and self-monitoring of steel structures.
- Duration: 36 months since 2018 June 1st
- Coordinator: Pr. Vincenzo Gatulli (La Sapienza University of Rome)
- Academic and industrial Partners: Sapienza Università di Roma (Italy), Universidad de Castilla – La Mancha, (Spain), Universidade do Porto (Portugal), Università di Pisa (Italy), UGE (France), Aiviewgroup srl (Italy), Sixense systems (France), Ecisa compania general de construcciones sa (Spain), Università di Cassino e del Lazio Meridionale (Italy), Universidad de Alicante (Spain), Inria (France).
- Inria contact: J. Dumoulin and L. Mevel
- Abstract: DESDEMONA objective is the development of novel design methods, systems, procedure and technical solution, to integrate sensing and automation technologies for the purpose of self-inspection and self-monitoring of steel structures. The approach will lead to an increment of the service life of existing and new steel civil and industrial infrastructure and to a decrease in the cost associated to inspections, improving human activities performed in difficult conditions, safety and workers’ potential by the use of advanced tools. The research aims to expand beyond the current state-of-the-art new high-quality standard and practices for steel structure inspection and maintenance through the interrelated development of the following actions: i) steel structure geometry and condition virtualization through data fusion of image processing, thermography and vibration measurements; ii) developing a procedure for steel defect detection by robotic and automatic systems such as Unmanned Aerial Vehicles (UAV) and ground mobile robots iii) embedding sensor systems to revalorize and transform steel elements and structures into self-diagnostic (smart) elements and materials even through nanotechnologies, iv) realizing an experimental lab-based apparatus and a series of case studies inspected by intelligent and robotic systems. The project outcome will have an impact on the reduction of the cost of steel structures inspection and maintenance and on the increase of user safety and comfort in industrial and civil environment. The proposal with a multidisciplinary approach fulfils the objectives of the Strategic Research Agenda of the European Steel Technology Platform.
8.3 National initiatives
CASC Projet : Acoustic Wave for Wirebreak in cables Monitoring
Participants: Vincent Le Cam, David Betaille.
I4S Teams has won a Governmental call for project (MTES DGITM direction) that consists in modernizing and trying new algorithms in the CASC platform : this solution aims at detecting and localizing Wire breaks in cables of suspended bridges by means of acoustic waves time difference of arrival (TDOA). In paralell of the updating work, new algorithms are actually compared to provide a better "time of arrival" time-stamping (by means of the maximmum of likelihood for instance). A third objective consist in implementing on wireless sensors the success of some delivrebrable of the PhD SENTAUR that target to keep a good time-synchronization in wireless sensors while keeping the GPS-energy lower as possible.
CEA List : Acoustic High Frequency synchronous and wireless
Participants: Vincent Le Cam.
In the area of infrastructure, strengthening links with CEA-LIST and Alstom-Rail will focus on non-destructive ultrasonic testing methods for rails. In 2020, the team have performed pratical developments on a specific daughter board of PEGASE3 dedicated to emission/reception of ultrasonic waves in view of Rail Monitoring. Complementary tests and algorithms have been performed to get in higher TRL of the system : from electro magnetic tests (CEM) to "in phase sampling" algorithms. That global and constructive phase between I4S and CEA-LIST has motivate CEA to sign a new collaboration named ONDULA2. The goal of that project, in continuity with the previous one, is to several complete rail-sensors applied in real railway application test bench ; another apsect consist in transfering the common knowledge to the final customer : Asltom.
ANR Scanning
Participants: Vincent LeCam.
- Type: ANR
- Objectif: embedded sensors for performance evaluation
- Duration:
- Coordinator:
- Partners : UGE
- Inria contact: Vincent Lecam
- Abstract: The SCaNING project therefore proposes to equip the structures with embedded sensors which will provide information similar to that used in NDE while allowing to continuously evaluate performance indicators (compressive strength and Young’s modulus) and the concrete conditions (porosity and water content) to improve indicator reliability and optimize diagnosis. This solution is the only way to ensure predictive maintenance by guaranteeing managers permanently available, pertinent information. In addition, the possibility of using communicating sensors through fully autonomous, low-power networks makes it possible to consider systems with low installation and operation costs. ANR Scanning project is lead by MAST LAMES laboratory of UGE inside wich the full "instrumentation" brick will be ensured by I4S common team.
ANR Resbati
Participants: Ludovic Gaverina, Jean Dumoulin.
- Type: ANR
- Objectif: In-situ measurements of thermal wall resistance
- Duration: 10/2016 to 10/2020 extented to 03/2021
- Coordinator: Laurent Ibos
- Partners : UGE, CERTES, CEREMA, CSTB, LNE, THEMACS, AFNOR
- Inria contact: Jean Dumoulin
- Abstract: RESBATI is an applied research project whose objective is to develop a field measurement device that meets precise specifications to systematically measure the level of thermal insulation of building walls. The preferred metrological tool is infrared thermography. A smart logger and a protype have been developed and presented. A full autonomous system has been studied and developed for in-situ measurement on existing building envelope. In parallel, thermal resistance estimation method was studied. First experiments were carried out with a first generation prototype in 2019. For this purpose different instrumented building walls were built and qualified at CSTB before carrying out in-situ evaluations of the prototype.
8.4 Regional initiatives
8.4.1 Collaboration with IRT Jules Verne and LAUM
Participants: Vincent LeCam.
- Type: IRT
- Objectif: develop more knowledge in default detection and localization applied on big plate of composite structures by means of Lamb waves
- Duration: 12/2020 to 12/2023
- Partners : LAUM, IRT Jules Verne
- Inria contact: Vincent LeCam
- Abstract: Vincent LE CAM is co-supervisor of the PERFORM PhD Project funded by IRT Jules Verne. The PhD Direction is lead by LAUM Laboratory. I4S role is to contribute to implement actual and future algorithms onto Embeded Sensors such as PEGASE (as its synchnonization up to some microseconds makes it possible to send/receive acoustic wave in phase)
8.4.2 RFI Wise Musiwind
Participants: Xavier Chapeleau, Laurent Mevel, Michael Doehler, Swarup Mahato.
- Type: RFI
- Objectif: qualify a very high precision sensor for vibratory monitoring of wind turbines, develop monitoring algorithms using SSI methods and validation indicators
- Duration: 1/2020 to 1/2021
- Coordinator: Xavier Chapeleau
- Partners : VALOREM/VALEMO, Sercel
- Inria contact: Xavier Chapeleau
-
Abstract: Structural health monitoring of wind turbines is becoming a real economic issue for the managers of these structures. Indeed, they are more and more demanding of new structural health control techniques that enable the implementation of an automated and planned monitoring strategy to ensure the structural integrity of their wind turbines throughout their lifetime. Through a multi-sensor approach, the project integrates in particular the newQuietSeisTM low-noise accelerometer (developed by SERCEL) with a generic data acquisition card Pegase 3 on which is embedded innovative signal processing (data analysis).
During the year 2020, the monitoring system has been developped and tested in laboratory. It is composed of three modules. Each module is formed by the association of a QS3 card (containing the QuietSeis accelerometer) with a raspberry Pi used as pilot card. To ensure accurate synchronization and timestamping, the card Pegase3 is used as NTP server in combination with a PPS signal delivered natively by this card.
8.4.3 Collaboration with IETR
Participants: Vincent Le Cam, David Pallier.
The thesis is directed by Sébastien Pillement at IETR. It is funded by RFI WISE Electronique Professionnelle within the SENTAUR project. The objective is to correct the time drift of the quartz in wireless sensor networks. Quartz modelizations, test platforms under real GPS conditions have been built. This has been published in 21.
8.4.4 Vibration analysis by video image processing for civil engineering structure monitoring
Participants: Bian Xiong, Qinghua Zhang.
- Type: ARED (Allocations de Recherche Doctorale)
- Objective: to develop video-based methods for civil engineering structure monitoring.
- Duration: 2018 - 2021
- Coordinator : Inria
- Partners: University Gustave Eiffel
- Inria contact: Qinghua Zhang
- Abstract: The I4S team develops real-time vibration analysis methods for the monitoring of civil engineering structures (bridges, buildings, etc.), usually based on mechanical sensors integrated into the monitored structures. In parallel, the team works also on image processing techniques for non-destructive testing of civil engineering construction materials. This PhD project, co-supervised with Vincent Baltazart (UGE researcher), aims to combine the two approaches in order to develop a method of vibration analysis based on image processing. Given a sequence of images of the structure to be monitored, the motion signal of the structure is derived from video image analysis, then methods of vibration analysis are applied to this motion signal. Such a solution will have the advantage of avoiding the integration of mechanical sensors into monitored structures and simplifying the maintenance of the monitoring system.
9 Dissemination
9.1 Promoting scientific activities
9.1.1 Scientific events: selection
Chair of conference program committees
- V. Le Cam is head and general secretary of the EWSHM scientific committee
Member of the conference program committees
- J.Dumoulin is member of the scientific committee of the GI Division (Geosciences Instrumentation and Data Systems) of EGU (European Geosciences Union) for infrastructure instrumentation and monitoring since 2013 (http://
www. )egu. eu/ gi/ structure/ - J.Dumoulin is member of the scientific committee of QIRT (quantitative Infrared Thermography) since 2014 (http://
www. )qirt. org/
- Q. Zhang is member of IFAC Technical Committee on Modelling, Identification and Signal Processing (TC 1.1)
- Q. Zhang is member of IFAC Technical Committee on Adaptive and Learning Systems (TC 1.2)
- Q. Zhang is member of IFAC Technical Committee on Fault Detection, Supervision and Safety of Technical Processes (TC 6.4)
- Q. Zhang is member of the international program commitee (technical associate editor) of the 21st IFAC World Congress in Berlin, Germany, July 12-17, 2020
- L. Mevel is member of the EWSHM scientific committee
- L. Mevel is member of the SHM-10 scientif committee
- L. Mevel was chair and co-chair of 2 sessions at IFAC World Congress 2020
- V. Le Cam is member of the IWSHM scientific committee
- V. Le Cam is member of the Asian Pacific Workshop
- M. Doehler is member of IFAC Technical Committee on Modelling, Identification, and Signal Processing (TC 1.1)
- M. Doehler is member of the IOMAC scientific committee
Reviewer
M. Doehler was reviewer for IFAC World Congress 2020, SYSID 2021.
J. Dumoulin was reviewer for QIRT 2020 and SFT 2020.
Q. Zhang was reviewer for CDC 2019, IFAC Word Congress 2020.
L. Mevel was reviewer for IFAC World Congress 2020, SYSID 2021.
9.1.2 Journal
Member of the editorial boards
L. Mevel is member of the editorial board of the journal of Mechanical Systems and Signal Processing, the journal Mathematical Problems in Engineering, and of the journal Shock and Vibration.
Q. Zhang is member of the editorial board of the journal of Intelligent Industrial Systems.
J. Dumoulin is member of the editorial board of the journal Quantitative Infrared Thermography, and Executive Editor for the journal Geoscientific Instrumentation and Data Systems.
Reviewer - reviewing activities
L. Mevel was reviewer for f Mechanical Systems and Signal Processing, Engineering Structures, Structural Control and Health Monitoring.
M. Doehler was reviewer for Mechanical Systems and Signal Processing, Journal of Sound and Vibration, IEEE Access, Engineering Structures, Journal of Engineering Mechanics Philosophical Transactions A Mathematical, Physical and Engineering Sciences, Computers and Structures, Structural Health Monitoring.
J. Dumoulin was reviewer for Quantitative Infrared Thermography Journal, GI Journal (EGU), SFT conference, Engineering Geology, International Journal of Pavement Research and Technology, Remote sensing of environment, Structural Health Monitoring, Composites Structures.
9.1.3 Leadership within the scientific community
V. Le Cam organized the 3rd SHM France meeting.
9.1.4 Research administration
V. Le Cam is member of the scientific council of WEN (West Electronic Network) since 2014, which is a cluster of about 200 companies, academics and research laboratories active in electronics.
9.2 Teaching - Supervision - Juries
9.2.1 Teaching
J. Dumoulin
- Master 2 ITII, BTP, module Maintenance et réhabilitation des ouvrages, Transferts thermiques dans les Structures : Des principes physiques à l'application sur site réel, 12 h, Ecole Centrale de Nantes(ECN), France.
V. Le Cam
- Master 2 Civil engineering, Structural Monitoring, 4h, Université de Nantes, France
- Licence 3 Professional SEICOM, 3h of theoretical lessons and 20H of practical lessons on Embedded and Smart Systems, Université de Nantes, France
- ESEO, 32h, practical lessons on embedded and smart systems under Linux, France
- Master 2 Electrical Engineering (GEII), 3h on electronic systems and Structural Monitoring, Université Bretagne Sud, France
- Polytech la Roche sur Yon, 3h embeded wireless algorithms
M. Doehler
- Cycle préparatoire intégré, STPI, mathématiques, 48h TD, INSA Rennes, France
X. Chapeleau
- Licence Pro Mesures physiques, Mesures optiques, 15h, IUT de St Nazaire, Université de Nantes, France
- "Monitoring and auscultation : Optical fiber sensors", 3h, 2nd Infrastar Training School, online, 27-30 October 2020
9.2.2 Supervision
- PhD : Bian Xong, Vibration analysis by video image processing for civil engineering structure monitoring, Q. Zhang, V. Balthazar. Ecole doctorale MathsTIC, Université de Rennes 1, since October 2018.
- PhD : Eva Viefhues, Statistical damage localization for civil structures, L. Mevel and M. Doehler. Ecole doctorale MathSTIC, Université de Rennes 1, since September 2016.
- PhD : David Pallier, Sensor Enhancement to Augmented Usage and Reliability, S. Pillement, IETR, V. Le Cam, Ecole doctorale MathSTIC
- PhD : Alexander Mendler, Vibration-based structural health monitoring of road bridges, C. Ventura and M. Doehler. University of British Columbia, Vancouver, Canada, defended in December 2020.
- Szymon Greś, research engineer with L. Mevel and M. Doehler, October 2019 - March 2020.
- Thibaud Toullier research engineer with J. Dumoulin, December 2019 - December 2020.
- Boualem Merainani, postdoc in H2020 Desdemona, May 2020 - December 2020.
- Swarup Mahato postdoc in RFI WISE Musiwind, February 2020 - December 2020.
9.2.3 Juries
J. Dumoulin participated in the following PhD defense committees:
- Domenico Vizzari at Centrale Nantes, October 22, 2020.
- Thanh Tung Ha at Université Paris-Est Créteil Val de Marne, December 10, 2020.
9.3 Popularization
9.3.1 Internal or external Inria responsibilities
L. Mevel is member of CLHSCT committee in Rennes.
L. Mevel is member of Comité de centre committee in Rennes.
L. Mevel is deputy head of science of Inria Rennes.
V. Le Cam is head of SII lab at Université Gustave Eiffel in Nantes.
J. Dumoulin is deputy head of SII lab at Université Gustave Eiffel in Nantes.
9.3.2 Education
Showroom demonstration: A damage localization mockup has been developed and installed in the showroom of Inria Rennes. It has been the support for outreach activities like "J'peux pas, j'ai informatique" and others for school classes, showing that computer science also can be related to physics and statistics. An ADT Carnot (2019-2020) has been funded for maturation of the localization mockup.
10 Scientific production
10.1 Major publications
- 1 inproceedings Diagnostic de structures de Génie Civil : Identification des propriétés spatiales et de la surface d’un défaut SFT 2014 Société Française de Thermique Lyon, France May 2014
- 2 articleInverse model for defect characterisation of externally glued CFRP on reinforced concrete structures: comparative study of square pulsed and pulsed thermographyQuantitative InfraRed Thermography Journal111March 2014, 84-114
- 3 articleEfficient Multi-Order Uncertainty Computation for Stochastic Subspace IdentificationMechanical Systems and Signal Processing382June 2013, 346--366
- 4 articleFast Multi-Order Computation of System Matrices in Subspace-Based System IdentificationControl Engineering Practice209September 2012, 882--894
- 5 articleModular Subspace-Based System Identification from Multi-Setup MeasurementsIEEE Transactions on Automatic Control5711November 2012, 2951--2956
- 6 articleSubspace-based fault detection robust to changes in the noise covariancesAutomatica499September 2013, 2734--2743
- 7 articleInfrared thermography system for transport infrastructures survey with inline local atmospheric parameter measurements and offline model for radiation attenuation evaluationsJournal of Applied Remote Sensing812014, 084978--084978
- 8 articleThe detection and thermal characterization of the inner structure of the ‘Musmeci’ bridge deck by infrared thermography monitoringJournal of Geophysics and Engineering106December 2013, 17
- 9 articleA new SSI algorithm for LPTV systems: application to a hinged-bladed helicopterMechanical Systems and Signal Processing421January 2014, 152--166
- 10 articleInverse method for flux characterization using infrared thermography in die forgingNumerical Heat Transfer, Part A Applications3331998, 267--277
- 11 articleExperimental validation of the inverse scattering method for distributed characteristic impedance estimationIEEE Transactions on Antennas and Propagation6362015, 7
- 12 article Robust statistical damage localization with stochastic load vectors Structural Control and Health Monitoring 22 3 March 2015
- 13 articleModal parameter estimation using interacting Kalman filterMechanical Systems and Signal Processing471August 2014, 139--150
- 14 articleInverse Scattering for Soft Fault Diagnosis in Electric Transmission LinesIEEE Transactions on Antennas and Propagation5912011, 141 - 148
10.2 Publications of the year
International journals
- 15 articleInvestigation into the use of thermoelectric modules as an alternative to conventional fluxmeters: application to convective and radiative heat flux in buildingsInternational Journal of Thermal Sciences1602021, 106653
- 16 articleConcrete Crack Monitoring Using a Novel Strain Transfer Model for Distributed Fiber Optics SensorsSensors820January 2020, 1-17
- 17 article Topological Optimization of Under-Platform Dampers with Moving Morphable Components and Global Optimization Algorithm for Nonlinear Frequency Response Journal of Engineering for Gas Turbines and Power January 2021
- 18 articleStudy of an optimal heating command law for structures with non-negligible thermal inertia in varying outdoor conditionsSmart Structures and Systems2722021, 379-386
- 19 article Determination of structural and damage detection system influencing parameters on the value of information Structural Health Monitoring January 2020
- 20 article Shape optimization of a disc-pad system under squeal noise criteria SN Applied Sciences 2 4 April 2020
- 21 articleEnergy-efficient GPS synchronization for wireless nodesIEEE Sensors Journal2142021, 5221 - 5229
- 22 articleRobust optimization: a kriging-based multi-objective optimization approachReliability Engineering and System Safety200August 2020, 30
- 23 articleDetection and Measurement of Matrix Discontinuities in UHPFRC by Means of Distributed Fiber Optics SensingSensors2014January 2020, 1-20
- 24 article Set-membership estimation for linear time-varying descriptor systems Automatica May 2020
- 25 articleDetection of Multiple Cracks in Four-Point Bending Tests Using the Coda Wave Interferometry MethodSensors207January 2020, 17p
International peer-reviewed conferences
- 26 inproceedingsTransforming Time-Delay System Observers to Adaptive ObserversIFAC 2020 - IFAC World CongressBerlin, GermanyJuly 2020, 1-5
- 27 inproceedingsVariance computation for system matrices and transfer function from input/output subspace system identificationIFAC 2020 - 21st IFAC World CongressBerlin, GermanyJuly 2020, 1-6
- 28 inproceedingsClustering of Redundant Parameters for Fault Isolation with Gaussian ResidualsIFAC 2020 - 21st IFAC World CongressBerlin, GermanyJuly 2020, 1-6
- 29 inproceedings The Minimum Detectable Damage as an Optimization Criterion for Performance-based Sensor Placement IMAC 2020 - 38th International Modal Analysis Conference Houston, United States February 2020
- 30 inproceedingsEllipsoid bundle and its application to set-membership estimation ⋆IFAC 2020 - IFAC World CongressBerlin, GermanyJuly 2020, 1-6
- 31 inproceedings A Kriging-based Interacting Particle Kalman Filter for the simultaneous estimation of temperature and emissivity in Infra-Red imaging IFAC 2020 – 21st IFAC World Congress Berlin, Germany July 2020
- 32 inproceedingsComparative study of four statistical methods for the simultaneous estimation of the emissivity and the temperature through multispectral infrared thermographySFT 2020 - Congrès annuel de la Société Française de ThermiqueBelfort, FranceJune 2020, 1-9
- 33 inproceedingsFault detection for linear parameter varying systems under changes in the process noise covarianceIFAC 2020 - 21st IFAC World CongressBerlin, GermanyJuly 2020, 1-6
- 34 inproceedings Analysis of Quadratic Surface Fitting for Subpixel Motion Extraction from Video Images EUSIPCO 2020 - 28th European Signal Processing Conference Amsterdam / Virtual, Netherlands January 2021
- 35 inproceedingsBoundedness of the Kitanidis Filter for Optimal Robust State EstimationIFAC 2020 - 21st IFAC World CongressBerlin, GermanyJuly 2020, 1-5
Conferences without proceedings
- 36 inproceedings Comparative study of steel corrosion characterization by visible and THz imaging techniques EGU 2020 - European Geoscience Union Virtual, Austria May 2020
- 37 inproceedings Study and development of an image synthesis software for the simulation of in-situ 3D scenes monitored by infrared cameras QIRT 2020 - 15th Quantitative InfraRed Thermography conference Virtual, Portugal September 2020
- 38 inproceedingsFast interval estimation for discrete-time systems based on fixed-time convergenceIFAC 2020 - 21st IFAC World CongressBerlin, GermanyJuly 2020, 1-5
Doctoral dissertations and habilitation theses
- 39 thesis Minimum Diagnosable Damage and Optimal Sensor Placement for Structural Health Monitoring University of British Columbia (Vancouver, Canada) December 2020
Reports & preprints
- 40 misc On the Optimality of the Kitanidis Filter December 2020
- 41 misc State and parameter estimation in linear systems with delays April 2020
10.3 Cited publications
- 42 articleFault isolation for diagnosis~: nuisance rejection and multiple hypotheses testingAnnual Reviews in Control262December 2002, 189--202URL: http://dx.doi.org/10.1016/S1367-5788(02)00029-9
- 43 techreportOn the relationship between identification and local tests1104IRISAMay 1997, URL: ftp://ftp.irisa.fr/techreports/1997/PI-1104.ps.gz
- 44 articleThe inverse scattering problem for LCRG transmission linesJournal of Mathematical Physics2312December 1982, 2286-2290
- 45 book Elements of Soliton Theory New York John Wiley & Sons 1980
- 46 phdthesis Fault diagnosis of wired electric networks by reflectometry Université Paris Sud - Paris XI May 2014
- 47 book Analysis of multiconductor transmission lines New York Wiley 2008
- 48 articleAn Inverse Scattering Approach to Soft Fault Diagnosis in Lossy Electric Transmission LinesIEEE Trans. on Antennas and Propagation59102011, 3730-3737URL: http://dx.doi.org/10.1109/TAP.2011.2163772
- 49 book Subspace Identification for Linear Systems Boston Kluwer Academic Publishers 1996
- 50 phdthesis Some inverse scattering problems on star-shaped graphs: application to fault detection on electrical transmission line networks Université de Versailles-Saint Quentin en Yvelines December 2011