Keywords
Computer Science and Digital Science
- A5. Interaction, multimedia and robotics
- A5.1. Human-Computer Interaction
- A5.10. Robotics
- A5.10.1. Design
- A5.10.2. Perception
- A5.10.3. Planning
- A5.10.4. Robot control
- A5.10.5. Robot interaction (with the environment, humans, other robots)
- A5.10.6. Swarm robotics
- A5.10.7. Learning
- A5.10.8. Cognitive robotics and systems
- A5.11. Smart spaces
- A5.11.1. Human activity analysis and recognition
- A8.2. Optimization
- A8.2.2. Evolutionary algorithms
- A9.2. Machine learning
- A9.5. Robotics
- A9.7. AI algorithmics
- A9.9. Distributed AI, Multi-agent
Other Research Topics and Application Domains
- B2.1. Well being
- B2.5.3. Assistance for elderly
- B5.1. Factory of the future
- B5.6. Robotic systems
- B7.2.1. Smart vehicles
- B9.6. Humanities
- B9.6.1. Psychology
1 Team members, visitors, external collaborators
Research Scientists
- François Charpillet [Team leader, Inria, Senior Researcher, HDR]
- Olivier Buffet [Inria, Researcher, HDR]
- Francis Colas [Inria, Researcher, HDR]
- Serena Ivaldi [Inria, Researcher]
- Pauline Maurice [CNRS, Researcher]
- Jean-Baptiste Mouret [Inria, Senior Researcher, HDR]
Faculty Members
- Amine Boumaza [Univ de Lorraine, Associate Professor]
- Alexis Scheuer [Univ de Lorraine, Associate Professor]
- Vincent Thomas [Univ de Lorraine, Associate Professor]
Post-Doctoral Fellows
- Mihai Andries [Inria, until Apr 2020]
- Glenn Maguire [Inria, until Jun 2020]
PhD Students
- Lina Achaji [PSA Stellantis, From 1st march]
- Timothee Anne [Univ de Lorraine, from Sep 2020]
- Waldez Azevedo Gomes Junior [Inria]
- Abir Bouaouda [Univ de Lorraine, from Oct 2020]
- Raphael Bousigues [Inria, from Dec 2020]
- Jessica Colombel [Inria]
- Yassine El Khadiri [Diatelic, CIFRE]
- Yoann Fleytoux [Inria]
- Adam Gaier [Hochschule Bonn-Rhein-Sieg, until Aug 2020]
- Nicolas Gauville [Groupe SAFRAN, CIFRE]
- Melanie Jouaiti [Univ de Lorraine, from Jun 2020 until Sep 2020]
- Rituraj Kaushik [Inria, until Jul 2020]
- Adrien Malaise [Inria, until Aug 2020]
- Nima Mehdi [Inria, from Jun 2020]
- Luigi Penco [Inria]
- Vladislav Tempez [Univ de Lorraine]
- Julien Uzzan [Groupe PSA, CIFRE]
- Lorenzo Vianello [Univ de Lorraine]
- Yang You [Inria]
- Eloise Zehnder [Univ de Lorraine]
- Jacques Zhong [CEA, from Oct 2020]
Technical Staff
- Ivan Bergonzani [Inria, Engineer, from Nov 2020]
- Brice Clement [INSERM, Engineer]
- Eloise Dalin [Inria, Engineer]
- Pierre Desreumaux [Inria, Engineer, until Aug 2020]
- Edoardo Ghini [Inria, Engineer, from Oct 2020]
- Raphael Lartot [Inria, Engineer, from Dec 2020]
- Glenn Maguire [Inria, Engineer, from Jul 2020]
- Lucien Renaud [Inria, Engineer]
Interns and Apprentices
- Ivan Bergonzani [Inria, until Mar 2020]
- Giorgio Boccarella [Inria, from Apr 2020 until Sep 2020]
- Raphael Bousigues [Inria, from Mar 2020 until Sep 2020]
- Aurelien Delage [INSA Lyon, from Mar 2020 until Aug 2020]
- Sylvain Geiser [Univ de Lorraine, until Sep 2020]
- Anuyan Ithayakumar [Univ de Lorraine, from Oct 2020]
- Pierre Laclau [Inria, until Feb 2020]
- Andrea Macri [Inria, until Feb 2020]
- Celian Muller-Machi [Univ de Lorraine, until Jun 2020]
- Diana Pop [Univ de Lorraine, from Apr 2020 until May 2020]
- Lucas Schwab [Univ de Lorraine, from Mar 2020 until Aug 2020]
Administrative Assistants
- Véronique Constant [Inria]
- Antoinette Courrier [CNRS]
Visiting Scientist
- Anji Ma [Beijing Institute of Technology]
2 Overall objectives
The goal of the Larsen team is to move robots beyond the research laboratories and manufacturing industries: current robots are far from being the fully autonomous, reliable, and interactive robots that could co-exist with us in our society and run for days, weeks, or months. While there is undoubtedly progress to be made on the hardware side, robotics platforms are quickly maturing and we believe the main challenges to achieve our goal are now on the software side. We want our software to be able to run on low-cost mobile robots that are therefore not equipped with high-performance sensors or actuators, so that our techniques can realistically be deployed and evaluated in real settings, such as in service and assistive robotic applications. We envision that these robots will be able to cooperate with each other but also with intelligent spaces or apartments which can also be seen as robots spread in the environments. Like robots, intelligent spaces are equipped with sensors that make them sensitive to human needs, habits, gestures, etc., and actuators to be adaptive and responsive to environment changes and human needs. These intelligent spaces can give robots improved skills, with less expensive sensors and actuators enlarging their field of view of human activities, making them able to behave more intelligently and with better awareness of people evolving in their environment. As robots and intelligent spaces share common characteristics, we will use, for the sake of simplicity, the term robot for both mobile robots and intelligent spaces.
Among the particular issues we want to address, we aim at designing robots having the ability to:
- handle dynamic environment and unforeseen situations;
- cope with physical damage;
- interact physically and socially with humans;
- collaborate with each other;
- exploit the multitude of sensor measurements from their surroundings;
- enhance their acceptability and usability by end-users without robotics background.
All these abilities can be summarized by the following two objectives:
- life-long autonomy: continuously perform tasks while adapting to sudden or gradual changes in both the environment and the morphology of the robot;
- natural interaction with robotics systems: interact with both other robots and humans for long periods of time, taking into account that people and robots learn from each other when they live together.
3 Research program
3.1 Lifelong autonomy
Scientific context
So far, only a few autonomous robots have been deployed for a long time (weeks, months, or years) outside of factories and laboratories. They are mostly mobile robots that simply “move around” (e.g., vacuum cleaners or museum “guides”) and data collecting robots (e.g., boats or underwater “gliders” that collect data about the water of the ocean).
A large part of the long-term autonomy community is focused on simultaneous localization and mapping (SLAM), with a recent emphasis on changing and outdoor environments 38, 50. A more recent theme is life-long learning: during long-term deployment, we cannot hope to equip robots with everything they need to know, therefore some things will have to be learned along the way. Most of the work on this topic leverages machine learning and/or evolutionary algorithms to improve the ability of robots to react to unforeseen changes 38, 46.
Main challenges
The first major challenge is to endow robots with a stable situation awareness in open and dynamic environments. This covers both the state estimation of the robot by itself as well as the perception/representation of the environment. Both problems have been claimed to be solved but it is only the case for static environments 45.
In the Larsen team, we aim at deployment in environments shared with humans which imply dynamic objects that degrade both the mapping and localization of a robot, especially in cluttered spaces. Moreover, when robots stay longer in the environment than for the acquisition of a snapshot map, they have to face structural changes, such as the displacement of a piece of furniture or the opening or closing of a door. The current approach is to simply update an implicitly static map with all observations with no attempt at distinguishing the suitable changes. For localization in not-too-cluttered or not-too-empty environments, this is generally sufficient as a significant fraction of the environment should remain stable. But for life-long autonomy, and in particular navigation, the quality of the map, and especially the knowledge of the stable parts, is primordial.
A second major obstacle to moving robots outside of labs and factories is their fragility: Current robots often break in a few hours, if not a few minutes. This fragility mainly stems from the overall complexity of robotic systems, which involve many actuators, many sensors, and complex decisions, and from the diversity of situations that robots can encounter. Low-cost robots exacerbate this issue because they can be broken in many ways (high-quality material is expensive), because they have low self-sensing abilities (sensors are expensive and increase the overall complexity), and because they are typically targeted towards non-controlled environments (e.g., houses rather than factories, in which robots are protected from most unexpected events). More generally, this fragility is a symptom of the lack of adaptive abilities in current robots.
Angle of attack
To solve the state estimation problem, our approach is to combine classical estimation filters (Extended Kalman Filters, Unscented Kalman Filters, or particle filters) with a Bayesian reasoning model in order to internally simulate various configurations of the robot in its environment. This should allow for adaptive estimation that can be used as one aspect of long-term adaptation. To handle dynamic and structural changes in an environment, we aim at assessing, for each piece of observation, whether it is static or not.
We also plan to address active sensing to improve the situation awareness of robots. Literally, active sensing is the ability of an interacting agent to act so as to control what it senses from its environment with the typical objective of acquiring information about this environment. A formalism for representing and solving active sensing problems has already been proposed by members of the team 37 and we aim to use this to formalize decision making problems of improving situation awareness.
Situation awareness of robots can also be tackled by cooperation, whether it be between robots or between robots and sensors in the environment (led out intelligent spaces) or between robots and humans. This is in rupture with classical robotics, in which robots are conceived as self-contained. But, in order to cope with as diverse environments as possible, these classical robots use precise, expensive, and specialized sensors, whose cost prohibits their use in large-scale deployments for service or assistance applications. Furthermore, when all sensors are on the robot, they share the same point of view on the environment, which is a limit for perception. Therefore, we propose to complement a cheaper robot with sensors distributed in a target environment. This is an emerging research direction that shares some of the problematics of multi-robot operation and we are therefore collaborating with other teams at Inria that address the issue of communication and interoperability.
To address the fragility problem, the traditional approach is to first diagnose the situation, then use a planning algorithm to create/select a contingency plan. But, again, this calls for both expensive sensors on the robot for the diagnosis and extensive work to predict and plan for all the possible faults that, in an open and dynamic environment, are almost infinite. An alternative approach is then to skip the diagnosis and let the robot discover by trial and error a behavior that works in spite of the damage with a reinforcement learning algorithm 56, 46. However, current reinforcement learning algorithms require hundreds of trials/episodes to learn a single, often simplified, task 46, which makes them impossible to use for real robots and more ambitious tasks. We therefore need to design new trial-and-error algorithms that will allow robots to learn with a much smaller number of trials (typically, a dozen). We think the key idea is to guide online learning on the physical robot with dynamic simulations. For instance, in our recent work, we successfully mixed evolutionary search in simulation, physical tests on the robot, and machine learning to allow a robot to recover from physical damage 47, 1.
A final approach to address fragility is to deploy several robots or a swarm of robots or to make robots evolve in an active environment. We will consider several paradigms such as (1) those inspired from collective natural phenomena in which the environment plays an active role for coordinating the activity of a huge number of biological entities such as ants and (2) those based on online learning 44. We envision to transfer our knowledge of such phenomenon to engineer new artificial devices such as an intelligent floor (which is in fact a spatially distributed network in which each node can sense, compute and communicate with contiguous nodes and can interact with moving entities on top of it) in order to assist people and robots (see the principle in 54, 44, 36).
3.2 Natural interaction with robotic systems
Scientific context
Interaction with the environment is a primordial requirement for an autonomous robot. When the environment is sensorized, the interaction can include localizing, tracking, and recognizing the behavior of robots and humans. One specific issue lies in the lack of predictive models for human behavior and a critical constraint arises from the incomplete knowledge of the environment and the other agents.
On the other hand, when working in the proximity of or directly with humans, robots must be capable of safely interacting with them, which calls upon a mixture of physical and social skills. Currently, robot operators are usually trained and specialized but potential end-users of robots for service or personal assistance are not skilled robotics experts, which means that the robot needs to be accepted as reliable, trustworthy and efficient 61. Most Human-Robot Interaction (HRI) studies focus on verbal communication 55 but applications such as assistance robotics require a deeper knowledge of the intertwined exchange of social and physical signals to provide suitable robot controllers.
Main challenges
We are here interested in building the bricks for a situated Human-Robot Interaction (HRI) addressing both the physical and social dimension of the close interaction, and the cognitive aspects related to the analysis and interpretation of human movement and activity.
The combination of physical and social signals into robot control is a crucial investigation for assistance robots 57 and robotic co-workers 53. A major obstacle is the control of physical interaction (precisely, the control of contact forces) between the robot and the human while both partners are moving. In mobile robots, this problem is usually addressed by planning the robot movement taking into account the human as an obstacle or as a target, then delegating the execution of this “high-level” motion to whole-body controllers, where a mixture of weighted tasks is used to account for the robot balance, constraints, and desired end-effector trajectories 35.
The first challenge is to make these controllers easier to deploy in real robotics systems, as currently they require a lot of tuning and can become very complex to handle the interaction with unknown dynamical systems such as humans. Here, the key is to combine machine learning techniques with such controllers.
The second challenge is to make the robot react and adapt online to the human feedback, exploiting the whole set of measurable verbal and non-verbal signals that humans naturally produce during a physical or social interaction. Technically, this means finding the optimal policy that adapts the robot controllers online, taking into account feedback from the human. Here, we need to carefully identify the significant feedback signals or some metrics of human feedback. In real-world conditions (i.e., outside the research laboratory environment) the set of signals is technologically limited by the robot's and environmental sensors and the onboard processing capabilities.
The third challenge is for a robot to be able to identify and track people on board. The motivation is to be able to estimate online either the position, the posture, or even moods and intentions of persons surrounding the robot. The main challenge is to be able to do that online, in real-time and in cluttered environments.
Angle of attack
Our key idea is to exploit the physical and social signals produced by the human during the interaction with the robot and the environment in controlled conditions, to learn simple models of human behavior and consequently to use these models to optimize the robot movements and actions. In a first phase, we will exploit human physical signals (e.g., posture and force measurements) to identify the elementary posture tasks during balance and physical interaction. The identified model will be used to optimize the robot whole-body control as prior knowledge to improve both the robot balance and the control of the interaction forces. Technically, we will combine weighted and prioritized controllers with stochastic optimization techniques. To adapt online the control of physical interaction and make it possible with human partners that are not robotics experts, we will exploit verbal and non-verbal signals (e.g., gaze, touch, prosody). The idea here is to estimate online from these signals the human intent along with some inter-individual factors that the robot can exploit to adapt its behavior, maximizing the engagement and acceptability during the interaction.
Another promising approach already investigated in the Larsen team is the capability for a robot and/or an intelligent space to localize humans in its surrounding environment and to understand their activities. This is an important issue to handle both for safe and efficient human-robot interaction.
Simultaneous Tracking and Activity Recognition (STAR) 60 is an approach we want to develop. The activity of a person is highly correlated with his position, and this approach aims at combining tracking and activity recognition to make one benefit from the other. By tracking the individual, the system may help infer its possible activity, while by estimating the activity of the individual, the system may make a better prediction of his/her possible future positions (especially in the case of occlusions). This direction has been tested with simulator and particle filters 43, and one promising direction would be to couple STAR with decision making formalisms like partially observable Markov decision processes (POMDPs). This would allow us to formalize problems such as deciding which action to take given an estimate of the human location and activity. This could also formalize other problems linked to the active sensing direction of the team: how the robotic system should choose its actions in order to better estimate the human location and activity (for instance by moving in the environment or by changing the orientation of its cameras)?
Another issue we want to address is robotic human body pose estimation. Human body pose estimation consists of tracking body parts by analyzing a sequence of input images from single or multiple cameras.
Human posture analysis is of high value for human robot interaction and activity recognition. However, even if the arrival of new sensors like RGB-D cameras has simplified the problem, it still poses a great challenge, especially if we want to do it online, on a robot and in realistic world conditions (cluttered environment). This is even more difficult for a robot to bring together different capabilities both at the perception and navigation level 42. This will be tackled through different techniques, going from Bayesian state estimation (particle filtering), to learning, active and distributed sensing.
4 Application domains
4.1 Personal assistance
During the last fifty years, many medical advances as well as the improvement of the quality of life have resulted in a longer life expectancy in industrial societies. The increase in the number of elderly people is a matter of public health because although elderly people can age in good health, old age also causes embrittlement, in particular on the physical plan which can result in a loss of autonomy. That will force us to re-think the current model regarding the care of elderly people.1 Capacity limits in specialized institutes, along with the preference of elderly people to stay at home as long as possible, explain a growing need for specific services at home.
Ambient intelligence technologies and robotics could contribute to this societal challenge. The spectrum of possible actions in the field of elderly assistance is very large, going from activity monitoring services, mobility or daily activity aids, medical rehabilitation, and social interactions. This will be based on the experimental infrastructure we have built in Nancy (Smart apartment platform) as well as the deep collaboration we have with OHS 2 and the company Pharmagest and it's subsidiary Diatelic, an SAS created in 2002 by a member of the teams and others.
At the same time, these technologies can be beneficial to address the increasing development of musculo-skeletal disorders and diseases that is caused by the non-ergonomic postures of the workers, subject to physically stressful tasks. Wearable technologies, sensors and robotics, can be used to monitor the worker's activity, its impact on their health, and anticipate risky movements. Two domains of applications have been particualry addressed in the last years: industry, and more specifically manufacturing, and healthcare.
4.2 Civil robotics
Many applications for robotics technology exist within the services provided by national and local government. Typical applications include civil infrastructure services 3 such as: urban maintenance and cleaning; civil security services; emergency services involved in disaster management including search and rescue; environmental services such as surveillance of rivers, air quality, and pollution. These applications may be carried out by a wide variety of robot and operating modalities, ranging from single robots to small fleets of homogeneous or heterogeneous robots. Often robot teams will need to cooperate to span a large workspace, for example in urban rubbish collection, and operate in potentially hostile environments, for example in disaster management. These systems are also likely to have extensive interaction with people and their environments.
The skills required for civil robots match those developed in the Larsen project: operating for a long time in potentially hostile environment, potentially with small fleets of robots, and potentially in interaction with people.
5 Social and environmental responsibility
5.1 Footprint of research activities
The carbon footprint of the team has been significantly reduced this year. All meetings, conferences and workshops were conducted in a virtual mode, which reduced the number of travels.
5.2 Impact of research results
Hospitals- The research of the ExoTurn project led to the deployment of a total of four exoskeletons (Laevo) in the Intensive Care Unit Department of the Hospital of Nancy (CHRU). They have been used by the medical staff since April 2020 to perform Prone Positioning on COVID-19 patients with severe ARDS. To the best of our knowledge, other hospitals (in Belgium, Holland and Switzerland) are following on our footsteps and purchased Laevo exoskeletons for the same use. At the same time, the positive feedback from the CHRU of Nancy has motivated us to continue investigating if exoskeletons could be beneficial for the medical staff involved in other type of healthcare activities. A new study will start in February 2021 in the department of vascular surgery.
Ageing and health- This research line has the objective to propose technological solutions to the difficulties of elderly people in an ageing population (due to the increase of life expectancy). The placement of older people in a nursing home (EHPAD) is often only a choice of reason and can be rather poorly experienced by people. One answer to this societal problem is the development of smart home technologies that assist the elderly to stay in their homes longer than they can do today. With this objective we have a long term cooperation with Pharmagest which is currently supported through a PhD thesis (Cifre) which started in june 2017. The objective is to enhance the CareLib solution developed by Diatelic (a subsidiary of the Wellcoop-Pharmagest group) and Larsen team through a previous collaboration (Satelor project). The Carelib offer is a solution consisting of (1) a connected box (with touch screen), (2) a 3D sensor that is able (i) to measure characteristics of the gait such as the speed and step length, (ii) to identify activities of daily life and (iii) to detect emergency situation such as a fall, and (3) universal sensors (motion, ...) installed in each part of the housing. A software licence has been grant by Inria to Pharmagest.
Environment- The new project TELEMOVTOP aims at automatizing the processes of disposal of metal sheets contaminated with asbestos from roofs. This procedure has a high environmental impact and is also a risk for the health of the workers. Robotics can be a major technology innovation in this field. With this project, the team aims at both helping to reduce the workers' risk of exposure to asbestos, and accelerating the disposal project to reduce environmental pollution.
6 New software and platforms
6.1 New software
6.1.1 RobotDART
- Name: RobotDART
- Keywords: Physical simulation, Robotics, Robot Panda
-
Functional Description:
RobotDart combines:
- high-level functions around DART to simulate robots,
- a 3D engine based on the Magnum library (for visualisation),
- a library of common research robots (Franka, IIWA, TALOS, iCub),
- abstractions for sensors (including cameras).
-
News of the Year:
- new 3D engine
- sensor abstraction
- new scheduler
- URDF library
- support of Talos, iCub, and Franka-Emika Panda
-
URL:
https://
github. com/ resibots/ robot_dart - Contact: Jean-Baptiste Mouret
- Partner: University of Patras
6.1.2 inria_wbc
- Name: Inria whole body controller
- Keyword: Robotics
- Functional Description: This controller exploits the TSID library (QP-based inverse dynamics) to implement a position controller for the Talos humanoid robot. It includes: - flexible configuration files - links with the RobotDART library for easy simulations - stabilizer and torque safety checks
- Release Contributions: First version
-
URL:
https://
github. com/ resibots/ inria_wbc - Contact: Jean-Baptiste Mouret
7 New results
7.1 Lifelong autonomy
7.1.1 Quality diversity algorithms
Participants: Jean-Baptiste Mouret, Glenn Maguire, Adam Gaier.
Quality Diversity algorithms (QD) are building blocks of many of our damage recovery and lifelong learning algorithms. They make also a novel family of optimization algorithms, which aim at finding a large set of high-performing solutions that are all behaving differently. We improved our MAP-Elites algorithm 48, which is the “reference” QD algorithm in two directions:
- in Multi-task MAP-Elites 18, we extended the range of quality functions that can be used to be able to solve thousands of tasks of the same family at the same time;
- in the Data-Driven Encoding 15, we learn a low-dimensional representation of high-performing solutions with a variational auto-encoder, which makes it possible to solve problems which are higher-dimensional (1000 dimensions versus 10 to 100 dimensions with the vanilla MAP-Elites).
We also published a review of the field, witth an evolutionary biology perspective 9
7.1.2 Adaptation to damage
Participants: Jean-Baptiste Mouret, Rituraj Kaushik, Timothée Anne.
Our objective is to allow robots (in particular, legged robots) to adapt to damage, in as few trials as possible (if possible, in less than 1 or 2 minutes) using trial-and-error algorithms (reinforcement learning) and models of the intact robot.- With D. Bossens and D. Tarapore (Univ. Southampton), we extended our IT&E algorithm 1 to search automatically for the best combination of behavior descriptors for future adaptations, which is in effect an algorithm of “meta Quality Diversity” 13.
- We extended our RTE algorithm 41 to be able to use multiple repertoires and use the most appropriate one given the experience of the robot 6.
- We published the result of our collaboration with S. Whiteson (University of Oxford) to improve the robustness to rare events of policies learned with Bayesian optimization and repertoires based on MAP-Elites 10.
- Exploring a different direction for data-efficiency, we introduced a new meta-learning algorithm that allows robots to update their model with very little data, then use it for control 6. Our algorithm relaxes the assumption that there exists a single starting point in the weight space for all future adaptations (which is an implicit assumption of algorithms like MAML).
External collaborators: David Bossens (Univ. Southampton), Danesh Tarapore (Univ. Southampton), Shimon Whiteson (University of Oxford)
7.1.3 Addressing active sensing problems through Monte-Carlo tree search (MCTS)
Participants: Vincent Thomas, Sylvain Geiser, Olivier Buffet.
The problem of active sensing is of paramount interest for building self awareness in robotic systems. It consists in planning actions in a view to gather information (e.g., measured through the entropy over certain state variables) in an optimal way. In the past, we have proposed an original formalism, -POMDPs, and new algorithms for representing and solving such active sensing problems 37 by using point-based algorithms, assuming either convex or Lipschitz-continuous criteria. More recently, we have developed new approaches based on Monte-Carlo Tree Search (MCTS), and in particular Partially Observable Monte-Carlo Planning (POMCP), which provably converges only assuming the continuity of the criterion. Based on this, we have proposed algorithms more suitable to certain robotic tasks by allowing for continuous state and observation spaces.7.1.4 Heuristic search for (partially observable) stochastic games
Participants: Olivier Buffet, Aurélien Delage, Vincent Thomas.
Collaboration with Jilles Dibangoye (INSA-Lyon, INRIA team CHROMA) and Abdallah Saffidine (University of New South Wales (UNSW), Sydney, Australia).
Many robotic scenarios involve multiple interacting agents, robots or humans, e.g., security robots in public areas.
We have mainly worked in the past on the collaborative setting, all agents sharing one objective, in particular through solving Dec-POMDPs by (i) turning them into occupancy MDPs and (ii) using heuristic search techniques and value function approximation 2. A key idea is to take the point of view of a central planner and reason on a sufficient statistic called occupancy state. This year, we have proposed solutions in this framework to two specific questions: (1) How to solve 2-agent collaboration problems where one of the agents knows what the other agent knows ? (2) How to solve Dec-POMDPs through mixed integer linear programming in a planning or learning setting?
We are now also working on applying similar approaches in the important 2-player zero-sum setting, i.e., with two competing agents. We have first proposed and evaluated an algorithm for (fully observable) stochastic games (SGs). Then we have proposed an algorithm for partially observable SGs (POSGs), here turning the problem into an occupancy Markov game, and using Lipschitz-continuity properties to derive bounding approximators. We are now working on an improved approach building on convexity and concavity properties.
[This line of research is pursued through Jilles Dibangoye's ANR JCJC PLASMA.]
7.1.5 Interpretable action policies
Participants: Olivier Buffet.
Collaboration with Iadine Chadès and Jonathan Ferrer Mestres (CSIRO, Brisbane, Australia), and Thomas G. Dietterich (Oregon State University, USA).
Computer-aided task planning requires providing user-friendly plans, in particular plans that make sense to the user. In probabilistic planning (in the MDP formalism), such interpretable plans can be derived by constraining action policies (if happens, do ) to depend on a reduced subset of (abstract) states or state variables. As a first step, we have proposed 3 solution algorithms to find a set of at most abstract states (forming a partition of the original state space) such that any optimal policy of the induced abstract MDP is as close as possible to optimal policies of the original MDP. We have then looked at mixed observability MDPs (MOMDPs), proposing solutions to (i) abstract visible state variables as described above, and (ii) simultaneously limit the number of hyperplanes used to represent the solution value function.
Publication: 14
7.1.6 PAAVUP - models of visual attention
Participants: Olivier Buffet, Vincent Thomas.
Collaboration with Sophie Lemonnier (Associate professor in psychology, Université de Lorraine).
Understanding human decisions and resulting behaviors is of paramount importance to design interactive and collaborative systems. This project focuses on visual attention, a central process of human-system interaction. The aim of the PAAVUP project is to develop and test a predictive model of visual attention that would be adapted to task-solving situations, and thus would depend on the task to solve and the knowledge of the participant, and not only visual characteristics of the environment as it is usually done.
By taking inspiration from Wickens et al.'s theoretical model based on the expectancy and value of information 59, we plan to model visual attention as a decision-making problem under partial observability and to compare the obtained strategies to the one observed in experimental situations. This project is an interdisciplinary project involving the cognitive psychology laboratory. We hope that gathering the skills from our scientific fields will help us to have a better understanding of the theoretical foundations of existing models (computer science and cognitive psychology) and to propose biologically plausible predictive computational models.
7.1.7 Visual prediction of robot's actions
Participants: Anji Ma, Serena Ivaldi.
This work is part of the CHIST-ERA project HEAP, that is focused on benchmarking methods for learning to grasp irregular objects in a heap. We are interested in the question of how to plan for a sequence of actions that enable the robot to bring the heap in a desired state where a grasp can be successful. The robot could push or grasp objects to change the state of the heap, to pick the objects one by one in a certain way. Our working hypothesis is that the robot only has a top camera to observe the heap, and it does not know in advance the objects, nor the heap state, nor that it knows the 3D models of the objects. One way to solve this problem is visual Model Predictive Control(MPC), that was recently proposed to plan actions to bring a robot setup in a certain state. Visual MPC needs a visual prediction model, or the so called visual foresight. In this work, we want to learn visual foresight models of our robot Franka performing several actions on a heap. Differently from previous studies, we are more interested in grasping and manipulations that have a high chance of a variable outcome, so we are designing a visual prediction model that includes a stochastic model to cope with uncertainty. We compare our model with the state of the art models associated to the RoboNet dataset, and we also compare their performance on a smaller dataset collected in our setup.
7.1.8 Multi-robot autonomous exploration of an unknown environment
Participants: Nicolas Gauville, Dominique Malthese (Safran), Christophe Guettier (Safran), François Charpillet.
This work is funded by Safran through a Cifre PHD Grant and it has the objective to study exploration problems where a set of robots has to completely observe an unknown environment such that it can then, for example, build an exaustive map from the recorded informations. We envision this problem in this project as an iterative decision process in which the robots use the available information gathered by their sensors from the beginning of the mission in order to decide the next places to visit with associated paths to reach this places. Accordingly the robots determine and execute then the appropriate manoeuvre to follow those paths until the next decision steps. The resulting exploration has to guarantee that the fleet of robots perceived the whole environment by the end of the mission, and that it can be decided when the mission is finished. In this work we consider the exploration problem with a homogeneous team of robots, each equipped with a LiDAR (laser detection and ranging). The multi-robot exploration problem faces some difficult issues : the robots need to coordinate themself such that they explore different areas. This entails the communication issue: they need to manage communication loss, and also determine the informations to sent other robots.Last year a first publication has been presented at the JFSMA conference and it received the best paper award. This year an improvement of this work has been proposed adressing better (1) the communication bootleneck of the approach and (2) the complexity of the decision process which does not depend on the number of robots anymore. A patent concerning this work has been registered.
7.1.9 Reinforcement learning for autonomous vehicle
Participants: Julien Uzzan, François Aioun (PSA), Thomas Hannagan (PSA), François Charpillet.
This work is funded by PSA Stellantis through a Cifre PhD Grant and it has the objective to study how reinforcement learning technics can participate to the design of autonomous cars. Two application domains have been chosen : longitudinal control and "merge and split". The longitudinal control problem, although it has already been solved in many ways, is still an interesting problem. The description is quite simple. Two vehicles are on a road : the one in front is called the “leader” and the other is the “follower”. We suppose that the road only has one lane, so there is no way for the follower to pass the leader. The objective is to learn a controller for the follower to drive as fast as possible while avoiding crashes with the leader. The algorithm used to train the agent is the Deep Deterministic Policy Gradient algorithm (DDPG). The idea of adding noise to perception during training to give our agent interesting properties such as a more cautious behavior as well as better performances overall has been proposed and a patent has been registered about this approach.
The merge and split problem is much more appealing and it is a problem which completely justify the approach knowing that to our knowledge no classical control methods can solve the problem. It refers to a road driving situation in which two roads of two lanes each merge and then split again, giving rise to an ephemeral common road of four lanes. The vehicles come from both roads and have different destinations. Thus, some will have to fit in, while others will only have to maintain their way. In this road situation the decision process governing the ego vehicle is quite complex as the decision includes not only the ego vehicle state but also the dynamic states of surrounding vehicles.
7.1.10 Automatic tracking of free-flying insects using a cable-driven robot
Participants: Abir Bouaouda, Dominique Martinez (LORIA), Mohamed Boutayeb (CRAN), Rémi Pannequin (CRAN), François Charpillet.
This work has been funded by the ANR IA program and started in October 2020. The aim of this work is to evaluate the contribution of AI techniques such as reinforcement learning to the problem of controlling a cable robot. Reinforcement learning for a cable driven robot doing a tracking task is quite appealing as it can take into account many more parameters than a model based approach. This project follows a series of work carried out in recent years by Dominique Martinez and Mohamed Boutayeb, culminating in a publication in science robotics. The task of the cable robot is to follow a flying insect in order to study their behavior. The end effector moved by the cables is equipped with two cameras.
This year, we have redesigned the robot. In the new configuration only three degrees of freedom are allowed, avoiding any rotation.
7.2 Natural interaction with robotics systems
7.2.1 Whole-body controller for the Talos robot
Participants: Jean-Baptiste Mouret, Serena Ivaldi, Eloïse Dalin, Ivan Bergonzani, Pierre Desreumaux.
We received the Talos robot, our full-size humanoid robot, in December 2019. In 2020, we spent a substantial amount of time and effort to write a state-of-the-art whole-body controller that implements:
- task-space inverse dynamics based on quadratic programming 51, 39, which means that a trajectory for some parts of the robot (e.g., the hands) is specified and the torques for the 32 joints are computed to follow the trajectory and the constraints (balance, limits, etc.). The computed torques are integrated to obtain joint positions.
- a Zero Moment Point (ZMP) admittance stabilizer based on the force-torque sensors of the ankles 40.
- a torque safety loop: at every timestep, the torque measured at each joint is compared (after filtering) to the expected torque (according to the model), and the robot stops if the mismatch is too high. This prevents the robot from breaking itself and from injuring nearby humans.
- a self-collision task based on repulsive spheres, which prevents the hands from colliding with the rest of the body.
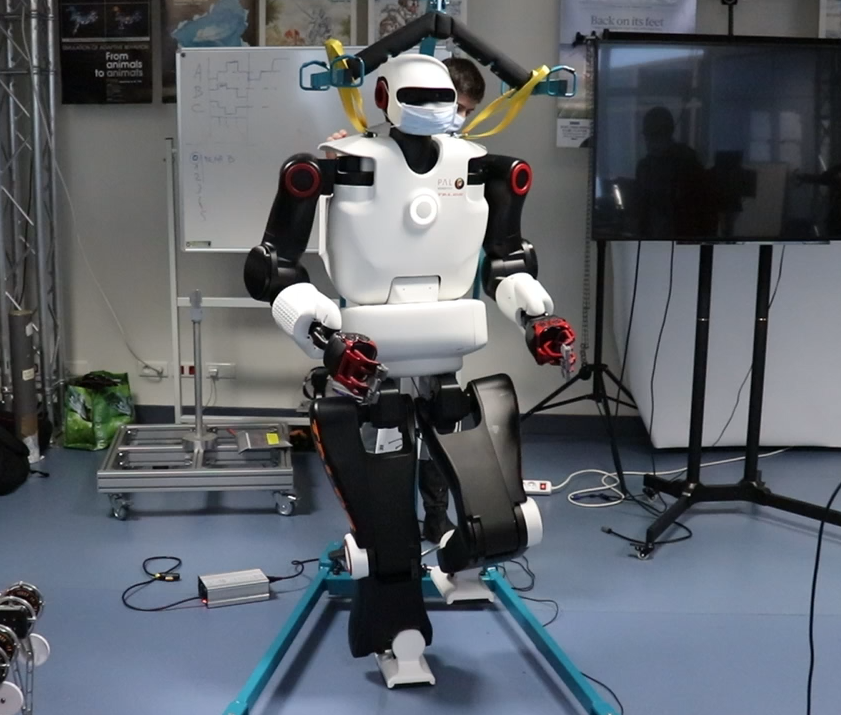
Talos on one foot, running our whole-body controller. At 1000 Hz, the robot uses a quadratic programming solver to compute the position of the 32 joints so that the feet and the hands are at the right position while (1) ensuring that the center of mass stays on top of the center of the foot, (2) there is no self-collision, (3) all the joint limits, velocity limits, and acceleration limits are respected. The ZMP (Zero Moment Point) stabilizer uses the force-torque sensor of the ankle to reject external perturbations.
The controller runs at 1000Hz on the embedded computer (it runs the quadratic optimization at each timestep). It is fully thread-safe and without any memory leak, two features that are required to run large-scale simulations for machine learning.
The source code is available online: https://
We successfully deployed this controller on the real robot (Fig. 1).
To develop this controller, we upgraded our RobotDart simulator https://
We then implemented whole-body retargeting, based on our previous work with iCub 49. Retargeting makes it possible to control the robot using the Xsens motion capture suit worn by an operator. We previously ran experiments like this with iCub, but with a very different controller 49. Unfortunately, the Talos experiments have not yet been validated on the real robot because of the different lockdowns and because the robot broke (2 boards in the ankle) at the end of 2020.
We are also capable of optimizing the parameters of the controller using the nondominated sorting genetic algorithm (NSGA) and our simulator (see below for similar work on the iCub humanoid robot 11).
This controller is the basis of the thesis of Timothée Anne and of all the future work of the team in humanoid robotics.
7.2.2 Optimizing multi-task whole-body controllers for the teleoperation of humanoids
Participants: Luigi Penco, Jean-Baptiste Mouret, Serena Ivaldi.
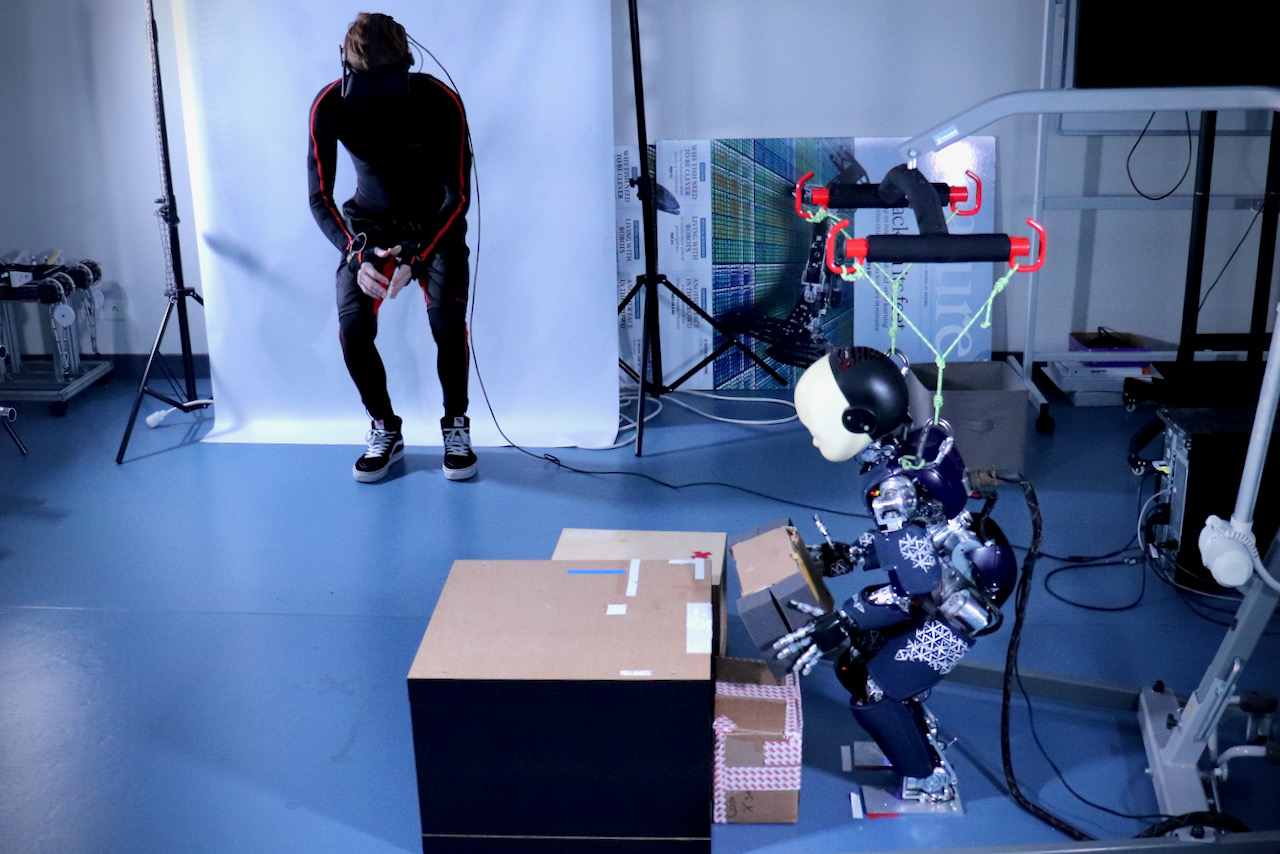
Teleoperation of the iCub robot. The operator wears a motion capture suit and a virtual reality helmet. We optimize the parameters and the structure of the controller with a multi-objective optimization algorithm.
We generate the movement of our humanoid robots using whole-body multi-task controllers based on quadratic programming, in which a multitude of tasks is organized according to strict or soft priorities. Choosing the right task priorities and gains is usually time-consuming and requires a substantial expertise. In this work, we automatically learned the controller configuration (soft and strict task priorities and convergence gains), to search for solutions that track the trajectory accurately while preserving the balance of the robot. We used a multi-objective optimization algorithm (NSGA-II) to find Pareto-optimal solutions that represent a trade-off between performance and robustness. We experimentally validated our method by learning a control configuration for the iCub humanoid, to perform different whole-body tasks in teleoperation (Fig. 2), such as picking up objects, reaching, and opening doors.
Publications: 11
7.2.3 Adaptive control of collaborative robots for preventing musculoskeletal disorders
Participants: Anuyan Ithayakumar, Vincent Thomas, Pauline Maurice.
The use of collaborative robots in a space shared with humans opens up new research directions. Those robots constitute a possible answer to musculoskeletal disorders: not only can they relieve the worker from heavy loads, but they could also guide him/her towards more ergonomic postures. In this context, the objective of the Ergobot project is to build adaptive robot strategies that are optimal regarding productivity but also the long-term health and comfort of the human worker.
To do so, we are developing tools to plan a robot policy taking into account the long-term consequences of the biomechanical demands on the human worker's joints (joint loading) and to distribute the efforts among the different joints during the execution of a repetitive task. The proposed platform must merge within the same framework several works conducted in the LARSEN team, namely virtual human modelling, fatigue estimate and decision making in the face of uncertainties.
A first platform is under development based on simple models. Once ready, this platform will allow us to refine those simple models and to investigate several scientific questions. For intance, fitting human reactions to experimental data, or investigating more complex reward functions to represent compromises between the reduction of the worker physical demand, the efficacy of the task execution and the required cognitive load.
7.2.4 Task-planning for human robot collaboration
Participants: Yang You, François Charpillet, Francis Colas, Olivier Buffet, Vincent Thomas.
Collaboration with Rachid Alami (CNRS Senior Scientist, LAAS Toulouse).
This work is part of the ANR project Flying Co-Worker (FCW) and focuses on high-level decision making for collaborative robotics. When a robot has to assist a human worker, it has no direct access to his current intention or his preferences but has to adapt its behavior to help the human complete his task.
This collaboration problem can be represented as a multi-agent problem and formalized in the decentralized-POMDP framework, where a common reward is shared among the agents. However, the cost of solving this multi-agent problem is prohibitive and, even if the optimal joint policy could be built, it may be too complex to be realistically executed by a human worker. To address the collaboration issue, we propose to consider a mono-agent problem by taking the robot's perspective and assuming the human is part of the environment and follows a known policy. In this context, building the robot consists in computing its best response given the human policy and can be formalized as a Partially Observable Markov Decision Process (POMDP).
This makes the problem computationally simpler, but the difficulty lies in how to choose a relevant human policy for which the robot's best response could be built. We are currently investigating this direction of research trying to automatically build (i) a human policy from the multi-agent model of the collaborative situation and (ii) the corresponding robot best response.
7.2.5 Sensor fusion for human pose estimation
Participants: Nima Mehdi, Francis Colas, Serena Ivaldi, Vincent Thomas.
This work is part of the ANR project Flying Co-Worker (FCW) and focuses on the perception of a human collaborator by a mobile robot for physical interaction. Physical interaction, for instance, object hand-over, requires the precise estimation of the pose of the human and, in particular her hands, with respect to the robot.
On the one hand, the human worker can wear a sensor suit with inertial sensors able to reconstruct the pose with good precision. However, such sensors cannot observe the absolute position and bearing of the human. All positions and orientations are therefore estimated by the suit with respect to an initial frame and this estimation is subject to drift (at a rate of a few cm per minute). On the other hand, the mobile robot can be equipped with cameras for which human pose estimation solutions are available such as OpenPose. The estimation is less precise but the error on the relative positioning is bounded.
This on-going work is tackling this issue with a dynamic Bayesian model combining the fusion of both sensor modalities and estimation through time of the human poses. The estimated state includes a 20-degrees-of-freedom skeletal model of the human as well as origin reference frames for both the wearable and camera sensors. This will allow the robot to jointly estimate the drift of the inertial sensors and the pose of the human.
At the present time, the current implementation uses a monolithic particle-filter approach and is not able to process enough particles to maintain a good enough representation of the probability distribution. Therefore future work will focus on structuring the state space so as to maintain several particle filters for various parts of the state.
7.2.6 Human motor variability in collaborative robotics
Participants: Raphaël Bousigues, Pauline Maurice.
Collaboration with David Daney (INRIA Bordeaux), Vincent Padois (INRIA Bordeaux) and Jonatha Savin (INRS)
Occupational ergonomics studies suggest that motor variability (i.e. using one's kinematic redundancy to perform the same task using different body motions and postures) might be beneficial to reduce the risk of developing musculoskeletal disorders. The positive exploitation of motor variability is, ideally, the natural result achieved by an expert. Such optimum is, however, not always reached, or requires a very long practice time. Methodologies to help the acquisition of motor habits exploiting at best the overall variability are therefore of interest. In this respect, collaborative robots are a possible tool that could enable an individualized acquisition of such good practices at the motor level by guiding the operator.
The overreaching goal of this project is to develop control laws for collaborative robots that encourage motor variability. In order to do so, it is necessary to develop models of motor variability in the context of human-robot collaboration (e.g., how do different constraints or iterventions introduced by the robot affect the motor variability of a novice/expert). In a first step of the project, we aim at developing tools to quantify motor variability, and to apply them to experimental data collected during human-robot collaborative tasks. We are currently investigating interval analysis and dynamic simulation of a human arm model with Quadratic Programming control to evaluate task-specific theoretical motor variability.
7.2.7 Using exoskeletons to assist medical staff manipulating Covid patients in ICU
Participants: Waldez Gomes, Lorenzo Vianello, Pauline Maurice, Serena Ivaldi.
Collaboration with CHRU Nancy and INRS
This work is part of the ExoTurn project. We conducted a pilot study to evaluate the potential and feasibility of back-support exoskeletons to help the caregivers in the Intensive Care Unit of the University Hospital of Nancy (France) executing Prone Positioning (PP) maneuvers on patients suffering from severe COVID-19-related Acute Respiratory Distress Syndrome (ARDS). After comparing four commercial exoskeletons, we selected and used the Laevo passive exoskeleton in the ICU in April 2020, and it has been since used to physically assist during PP following the recrudescence of COVID-19.
Each PP procedure is performed by a team of 5 trained physicians, who remain with their torso bent forward for several minutes, thus causing load, and potential injuries, in the low back. These types of injuries are well-known in industry, where back-support systems are used to alleviate the problem. Because of the dramatic increase of PP performed during the COVID-19 outbreak (from 0.3 to 11-15 per day), the medical staff was exposed to extraordinary physical stress. Exoskeletons, never used before in an ICU to physically assist the caregivers, proved to be helpful and readily feasible to use, even in critical sanitary conditions. Our pilot study supports the adoption of exoskeletons to physically assist the medical staff in the current practice.
The study was conducted initially in a simulated environment, to probe into the helpfulness of an exoskeleton during PP. Four existing commercial passive and active back-support systems (Corfor, Laevo, BackX and CrayX) were evaluated by 5 experienced PP physicians on a patient simulator at the Hospital Simulation Center of the University of Lorraine. We recorded the PP gesture with an Xsens MVN suit and subjective evaluations about the perceived assistance with a questionnaire. Then, two volunteer physicians used the selected exoskeleton (Laevo) in the ICU, evaluating the perceived effort after each PP on a Borg10 scale. Physiological measurements (surface EMG and ECG recordings, measured with Delsys Trigno system) comparing the movements with/ without the exoskeleton with a baseline control (rest) and DHM simulation confirmed the positive assistance of Laevo. After the first trial in the ICU, the use of the exoskeleton by the physicians of the ICU service (more than a dozen) has been evaluated with post-PP questionnaires investigating mainly the perceived effort on a Borg10 scale.
In comparison with the non-assisted situation, all participants perceived a reduction of physical effort for all exoskeletons except the CORFOR. However, CrayX was too cumbersome to wear in an ICU, while BackX was hindering several arm movements of the PP. Participants were satisfied with Laevo in terms of perceived assistance, safety, comfort, easiness of equipment, and freedom of movements. The first volunteers using the Laevo in the ICU reported very positive feedback and reduction of effort; EMG and ECG analysis confirmed the help. Laevo has been used in the ICU of the Hospital of Nancy since April 2020, with an overall positive feedback. Though the number of PP per day in the second wave was lower than in the first, the exoskeleton is still perceived as useful tool to physically assist the physicians.
7.2.8 Activity recognition for the control of a semi-active exoskeleton for overhead work
Participants: Pauline Maurice, Serena Ivaldi.
Collaboration with Claudia Latella and Daniele Pucci (Istituto Italiano di Tecnologia, Genova, Italy), with Jan Babic (Jozef Stefan Institute, Ljubljana, Slovenia), with Benjamin Schirrmeister, Jonas Bornmann and Jose Gonzalez (Ottobock SE & Co. KGaA, Duderstadt, Germany), and with Francesco Nori (Google DeepMind)
This work is part of the H2020 project AnDy. The AnDy project investigates robot control for human-robot collaboration, with a specific focus on improving ergonomics at work through physical robotic assistance. Earlier on in the project, we performed a thorough assessment of the objective and subjective effects of using the Paexo passive exoskeleton designed by Ottobock (partner in the AnDy project) to assist overhead work 8. While the results were promising, the assistive torque provided by the passive exoskeleton cannot adapt online. Such adjustment might however be useful to avoid unnecessary support (e.g. when lowering the arm), or to adjust to the weight of a manipulated object.
In collaboration with AnDy partners (IIT, JSI, Ottobock) we are currently coupling the activity recognition module developed by the Larsen team in the AnDy project 30 with the Paexo mechanical structure to turn it into a semi-active exoskeleton with adaptive support. The output of the activity recognition, in addition to other data, will serve to estimate the required level of support. In parallel, we are preparing the experimental protocol to evaluate the biomechanical, physiological and subjective benefits provided by this semi-active Paexo compared to the passive version. Experiments are planned for the summer 2021 (but might be moved depending on the sanitary situation).
7.2.9 Prediction of human movement to control an active exoskeleton
Participants: Raphaël Lartot, Pauline Maurice, Serena Ivaldi, François Charpillet.
This work is part of the DGA-RAPID project ASMOA. The project is about intention prediction for exoskeleton control. The challenge is to detect the intention of movement of the user of an exoskeleton, based on data from sensors embedded on the exoskeleton (e.g. inertial measurement units (IMUs), strain gauges). The predicted movement serves to optimize the exoskeleton control in order to provide an adapted support in an intuitive manner.
We are currently investigating the use of Probabilistic Movement Primitives (ProMPs) to predict the future limb trajectory of a human, based on the observation of the first samples of the trajectory. ProMP were previously used in the Larsen team: this work builds on code available in the team, and aims at evaluating the technique for exoskeleton applications, in which the tight interaction between the human and the exoskeleton raises many questions.
7.2.10 Learning the user preference for grasping objects from few demonstrations
Participants: Yoann Fleytoux, Jean-Baptiste Mouret, Serena Ivaldi.
This work is part of the CHIST-ERA project HEAP, that is focused on benchmarking methods for learning to grasp irregular objects in a heap. We are focusing on learning from human experts and with humans-in-the-loop. In this work, we are interested in the question of how to learn the preference of a grasp from a human expert. We hypothesize that the expert can simply provide a binary feedback (yes/no) about suggested candidate grasps, which are shown to the user as virtual grasps projected onto the camera images before any robot grasp execution. Our target is to learn from as few "labels" as possible, to reduce the amount of interaction with the expert. To do so, we are proposing to use a binary classifier (based on Gaussian Processes) applied on a reduced latent representation of grasp candidates, represented by image patches on the camera image. We are comparing our method with existing grasp candidate selection algorithms (e.g., DexNet), on both the Cornell dataset of labeled grasps and a novel dataset of heap images that we acquired with our setup (Franka with Intel cameras).
7.2.11 Human posture prediction during human-robot physical interaction
Participants: Lorenzo Vianello, Eloise Dalin, Jean-Baptiste Mouret, Serena Ivaldi.
Collaboration with Alexis Aubry (CRAN)
This work is part of the LUE project C-Shift, that is focused on collaborative robotics. When a human is interacting physically with a robot to accomplish a task, his/her posture is inevitably influenced by the robot movement. Since the human is not controllable, an active robot imposing a collaborative trajectory should predict the most likely human posture. This prediction should consider individual differences and preferences of movement execution, and it is necessary to evaluate the impact of the robot’s action from the point of view of ergonomics. In this work, we propose a method to predict, in probabilistic terms, the human postures of an individual for a given robot trajectory executed in a collaborative scenario. We formalize the problem as the prediction of the human joints velocity given the current posture and robot end-effector velocity. Previous approaches to solve this problem relied on the inverse kinematics, but did not consider the human body redundancy or the kinematic constraints imposed by the physical collaboration, nor any prior observations of the human movement execution. We propose a data-driven approach that addresses these limits. The key idea of our algorithm is to learn the distribution of the null space of the Jacobian and the weights of the weighted pseudo-inverse from demonstrated human movements: both carry information about human postural preferences, to leverage redundancy and ensure that the predicted posture will be coherent with the end-effector position. We show in a simulated toy problem and on real human-robot interaction data that our method outperforms model-based inverse kinematics prediction, sample-based prediction and regression methods that do not consider geometric constraints. Our method is validated on a collaboration scenario with a human interacting physically with the Franka robot.
Publications: 24
7.2.12 Human motion analysis in ecological environment
Participants: Jessica Colombel, David Daney (Auctus Bordeaux), François Charpillet.
The estimation of human motion from sensors that can be used in an ecological environment is an important issue, be it for home assistance for frail people or for human/robot interaction in industrial contexts. We are continuing our work on data fusion from RGB-D sensors using extended Kalman filters. The original approach uses a biomechanical model of the person to obtain anthropomorphically constrained joint angles to make their estimation physically coherent. In addition, we propose a method for the optimal adjustment of the covariance matrices of the extended Kalman filter. The proposed approach was tested with six healthy subjects performing 4 rehabilitation tasks. The accuracy of the joint estimates was evaluated with a reference stereophotogrammetric system. Our results show that an affordable RGB-D sensor can be used for simple home rehabilitation when using a constrained biomechanical model. This work has led to an accepted article in MDPI Sensors 5.
In a second step, we compared the joint centre estimates obtained with the new Kinect 3 (Azure Kinect) sensor, the Kinect 2 (Kinect for Windows) and a reference stereophotogrammetric system. Regardless of the system used, we have shown that our algorithm improves the body tracker data. This study also shows the importance of defining good heuristics to merge the data according to the body tracking operation. This study has been presented by Jessica Colombel at the International Conference on Activity and Behavior Computing, and will be published as a book chapter 25.
7.2.13 Human motion decomposition
Participants: Jessica Colombel, David Daney (Auctus Bordeaux), François Charpillet.
The aim of the work is to find ways of representing human movement in order to extract meaningful physical and cognitive information. After the realization of a state of the art on human movement, several methods are compared: principal component analysis (PCA), Fourier series decomposition and inverse optimal control. These methods are used on a signal comprising all the angles of a walking human being. PCA makes it possible to understand the correlations between the different angles during the trajectory. Fourier series decomposition methods are used for a harmonic analysis of the signal. Finally, inverse optimal control sets up a modeling of the motor control to highlight qualitative properties characteristic of the whole motion. These three methods are tested, combined and compared on data from the EWalk database http://7.3 Pedestrian behavior prediction
Participants: Lina Achaji, François Aioun (PSA), Julien Moreau (PSA), François Charpillet.
This report summarizes the work done during the Ph.D. thesis that started on the 1st of March 2020. This thesis is conducted in the context of the OpenLab collaboration between Inria Nancy and the Groupe PSA. It’s concerned with the behavior, intention, and trajectory prediction of road users in front of autonomous cars using deep learning (DL) techniques.
According to the work plan of the thesis, we spent nearly six months working on the literature and the state of the art to solve this behavior prediction problem. During this time, we produced a state of the art report that contains a study on the definition of prediction, the definition of autonomous Vehicles (AV), and a detailed explanation of the AI methods that we will use during the rest of the thesis.
As for the AI methods that we explained in the report, we first talked about the recurrent neural network methods, which help us build a sequential model for each user of the autonomous vehicle’s road. These methods have multiple variations, advantages, and limits. Thus, we studied each of them in detail. Then, we are also interested in the techniques concerned with the perceiving of the environment. Therefore, we have a large study about the different computer vision methods used in AI, including object detection, segmentation, etc., and their way of usage in our case. Besides, we also studied the AI methods that focus on the interaction between agents in the environments. These methods are called ’geometric deep learning’ or graph neural network methods. We investigated the ways that we can use this type of approach in the context of behavior prediction.
For the second part of the work plan, which started in September 2020, we started applying the developed theoretical work to our problem. As a first step, we defined the different ways of handling the prediction problem, and we agreed to start working on the intention prediction of pedestrians to cross the road in front of the AV. Then, we chose a dataset to work on in our experiments, which was the newly released PIE dataset ( https://
7.4 Changepoint Detection and Activity Recognition for Understanding Activities of Daily Living (ADL) @home
Participants: Yassine El Khadiri, Cedric Rose( Diatelic), François Charpillet.
This work is partly funded by Diatelic, a subsidiary of Pharmagest through a Cifre PhD grant. This collaboration is motivated by a program partly funded by region Lorraine (36 mois de plus). The “36 mois de plus” project has the objective to propose technological solutions to the difficulties of elderly people in an ageing population (due to the increase of life expectancy). The placement of older people in a nursing home (EPHAD) is often only a choice of reason and can be rather poorly experienced by people.One answer to this societal problem is the development of smart home technologies that facilitate elderly to stay in their homes longer than they can do today. The objective is to enhance the CareLib solution developed by Diatelic and Larsen Team (Inria Loria) through a previous collaboration (Satelor project). The objective of the ongoing PhD Cifre program is to provide personalized follow-up by learning life habits, the main objective being to track the Activities of Daily Life (ADL) and detect emergency situations needing external interventions (e.g. fall detection). For example an algorithm capable to detect sleep-wake cycles using only motion sensors has been developed. The approach is based on artificial intelligence techniques (Bayesian inference). The algorithms have been evaluated using a publicly available dataset and Diatelic’s own dataset.
7.5 Social robots and loneliness: Acceptability and trust
Participants: Eloise Zehnder, Jérome Dinet (2LPN), François Charpillet.
This PhD work is done between Larsen team and 2LPN the psychology laboratory of University of Lorraine. The main objective of the PhD program is to study how social robots or avatars can fight loneliness and how this is related to acceptability and trust.
During this year several experimental setups have been conducted :
- one in collaboration with Mélanie Jouaiti in which the acceptability of robots has been studied in the context of the use of a social robot (Pepper) with children with high-level autism (interrupted by the sanitary situation). The acceptability has been studied both for children and the accompanying parents.
- A textual analysis has also been done of reviews coming from users of the chatbot companion Replika (paper recently submitted to AHFE2021).
- At the end of the year, another experiment has been conducted with students which compared the effect of 4 different videos with two different variables : appearance and voice (virtual vs human teacher; text-to-speech vs human voice).
8 Bilateral contracts and grants with industry
8.1 Bilateral grants with industry
PhD grant (Cifre) with Diatelic Pharmagest
Participants: François Charpillet, Yassine El Khadiri.
We have a long term collaboration with Diatelic compagny which is a start-up created by François Charpillet and colleagues in 2002. Currently we have a collaboration through a Cifre PhD whose objective is to work on daily activity recognition for monitoring elderly people at home. The work will be included in a product that will be launched next year (CareLib solution).
Two PhD grants with PSA
Participants: François Charpillet, Julien Uzzan, Lina Achaji.
Groupe PSA and Inria annonced on July 5th 2018 the creation of an OpenLab dedicated to artificial intelligence. The studied areas will include autonomous and intelligent vehicles, mobility services, manufacturing, design development tools, the design itself and digital marketing as well as quality and finance. A dozen topics will be covered over a 4 years period. Two PhD programs have been launched with the Larsen team in this context.PhD grant with SAFRAN
Participants: François Charpillet, Nicolas Gauville, Christophe Guettier.
The thesis began on May 6, 2019 after a "prethesis" of 6 month and is related to the Furious Project. The objective is to propose new coordination mechanisms for a group of autonomous robots evolving in an unknown environment for search and rescue (Robot Search and Rescue). The thesis is a continuation of a previous work made during the Cartomatic project which won in 2012 the French robotics contest Defi CAROTTE organized by the General Delegation for Armaments (DGA) and French National Research Agency (ANR).PhD work co-advised with CEA-LIST
Participants: Jacques Zhong, Francis Colas, Pauline Maurice.
Collaboration with Vincent Weistroffer (CEA-LIST) and Claude Andriot (CEA-LIST)
This PhD work started in October, 2020. The objective is to develop a software tool that allows to take into account the diversity of workers' morphology when designing an industrial workstation. The developed tool will enable to test the feasibility and ergonomics of a task for any morphology of workers, based on a few demonstrations of the task in virtual reality by one single worker. The two underlying scientific questions are i) the automatic identification of the task features from a few VR demonstrations, and ii) the transfer of the identified task to digital human models of various morphologies.
9 Partnerships and cooperations
9.1 European initiatives
9.1.1 FP7 & H2020 Projects
An.Dy
- Title: Advancing Anticipatory Behaviors in Dyadic Human-Robot Collaboration
- Duration: 2017/01 - 2021/06
- Coordinator: Francesco Nori (IIT & Google Deepmind)
-
Partners:
- AnyBody Technology AS (Denmark)
- Deutsches Zentrum für Luft- und Raumfahrt EV (Germany)
- Fondaziona Istituto Italiano di Tecnologia (Italy)
- Institut Jozef Stefan (Slovenia)
- Istituto Nazionale Assicurazione Infortuni sul Lavoro Inail (Italy)
- Ottobock SE & Co. KGAA (Germany)
- Xsens Technologies B.V. (Netherlands)
- Inria (France)
- Inria contact: Serena Ivaldi
- Summary: Recent technological progress permits robots to actively and safely share a common workspace with humans. Europe currently leads the robotic market for safety-certified robots, by enabling robots to react to unintentional contacts. AnDy leverages these technologies and strengthens European leadership by endowing robots with the ability to control physical collaboration through intentional interaction. In the first validation scenario, the robot is a collaborative robot (i.e. robot=cobot) and provides physical collaboration for improved health, productivity and flexibility. This scenario falls within the scope of human- robot collaboration (e.g. a manipulator helping an assembly-line-worker in heavy load transportation and installation). In the second validation scenario, the robot is an exoskeleton (i.e. robot=exoskeleton) and provides physical collaboration for improved health, productivity and flexibility. It falls within human-exoskeleton physical interaction (e.g. an actuated exoskeleton assisting human walking). In the third validation scenario, the robot is a humanoid (i.e. robot=humanoid). This scenario falls within the scope of human-humanoid physical collaboration (e.g. whole-body collaboration for assisted walking and assembly).
ResiBots
- Title: Robots with animal-like resilience
- Program: H2020
- Type: ERC
- Duration: May 2015 - October 2020
- Coordinator: Inria
- Inria contact: Jean Baptiste Mouret
- Summary: Despite over 50 years of research in robotics, most existing robots are far from being as resilient as the simplest animals: they are fragile machines that easily stop functioning in difficult conditions. The goal of this proposal is to radically change this situation by providing the algorithmic foundations for low-cost robots that can autonomously recover from unforeseen damages in a few minutes. It is here contended that trial-and-error learning algorithms provide an alternate approach that does not require diagnostic, nor pre-defined contingency plans. In this project, we developed and studied a novel family of such learning algorithms that make it possible for autonomous robots to quickly discover compensatory behaviors.
9.1.2 Collaborations in European programs, except FP7 and H2020
HEAP
- Title: Human-Guided Learning and Benchmarking of Robotic Heap Sorting
- Duration: 2019/04 - 2022/04
- Funding: CHIST-ERA (Era-Net cofund)
- Coordinator: Gerhard Neumann (Karlsruhe Institute of Technolgy & Bosch, formerly Univ. Lincoln)
-
Partners:
- University of Lincoln (UK)
- Technische Universität Wien (Austria)
- Fondazione Istituto Italiano di Tecnologia (Italy)
- Idiap Research Institute (Switzerland)
- Inria (France)
- Inria contact: Serena Ivaldi
- Summary: This project will provide scientific advancements for benchmarking, object recognition, manipulation and human-robot interaction. We focus on sorting a complex, unstructured heap of unknown objects –resembling nuclear waste consisting of a set of broken deformed bodies– as an instance of an extremely complex manipulation task. The consortium aims at building an end-to-end benchmarking framework, which includes rigorous scientific methodology and experimental tools for application in realistic scenarios. Benchmark scenarios will be developed with off-the-shelf manipulators and grippers, allowing to create an affordable setup that can be easily reproduced both physically and in simulation. We will develop benchmark scenarios with varying complexities, i.e., grasping and pushing irregular objects, grasping selected objects from the heap, identifying all object instances and sorting the objects by placing them into corresponding bins. We will provide scanned CAD models of the objects that can be used for 3D printing in order to recreate our benchmark scenarios. Benchmarks with existing grasp planners and manipulation algorithms will be implemented as baseline controllers that are easily exchangeable using ROS. The ability of robots to fully autonomously handle dense clutters or a heap of unknown objects has been very limited due to challenges in scene understanding, grasping, and decision making. Instead, we will rely on semi-autonomous approaches where a human operator can interact with the system (e.g. using tele-operation but not only) and give high-level commands to complement the autonomous skill execution. The amount of autonomy of our system will be adapted to the complexity of the situation. We will also benchmark our semi-autonomous task execution with different human operators and quantify the gap to the current SOTA in autonomous manipulation. Building on our semi-autonomous control framework, we will develop a manipulation skill learning system that learns from demonstrations and corrections of the human operator and can therefore learn complex manipulations in a data-efficient manner. To improve object recognition and segmentation in cluttered heaps, we will develop new perception algorithms and investigate interactive perception in order to improve the robot’s understanding of the scene in terms of object instances, categories and properties.
9.2 National initiatives
9.2.1 ANR : The Flying Co-Worker
- Program: ANR
- Project title: Flying Co-Worker
- Duration: October 2019 – October 2023
- Coordinator: Daniel Sidobre (Laas Toulouse)
- PI for Inria: François Charpillet
- Abstract: Bringing together recent progress in physical and decisional interaction between humans and robots with the control of aerial manipulators, this project addresses the flying coworker, an aerial manipulator robot that act as a teammate of a human worker to transport a long bar or to realise complex tasks. Safety and human-aware robot abilities are at the core of the proposed research to progressively build robots capable to do cooperative handling and to assist a worker by notably delivering objects directly in a safe, efficient, pertinent and acceptable manner. The methodologies developed for ground manipulators cannot be directly used for aerial manipulator systems because of the floating base, of a limited payload, and of strong actuation and energy constraints. From the perception and the interpretation of the human activity, the objective of the project is to build an aerial manipulator capable to plan and control human aware motions to achieve collaborative tasks.
9.2.2 ANR : PLASMA
- Program: ANR
- Project acronym: PLASMA
- Project title: Planification et Apprentissage pour Agir dans des Systèmes Multi-Agents
- Duration: February 2020 – October 2023
- Coordinator: Jilles Dibangoye (INSA-Lyon)
- PI for Inria: Olivier Buffet
- Abstract: The main research goal is to develop a general theory and algorithms with provable guarantees to treat planning and (deep) Reinforcement Learning problems arising from the study of multi-agent sequential decision-making, which may be described as Partially Observable Stochastic Games (POSGs).
9.2.3 ANR : Proxilearn
- Program: ANR-DGA ASTRID
- Project acronym: Proxilearn
- Project title: Learning for Proximity Flying
- Duration: January 2020 – December 2023
- Coordinator: Inria Nancy – Grand Est
- PI for Inria: Jean-Baptiste Mouret
-
Partner:
- Institut des sciences du Mouvement, CNRS/Aix-Marseille Université
- Summary: The Proxilearn project leverages artificial intelligence techniques to make it possible for micro-UAV (10-20 cm / 50-80g) to fly in very confined spaces (diameter between 40cm and 1.5m): air ducts, tunnels, natural caves, quarries, ... It is focused on two challenges: (1) stabilizing a UAV in spite of the turbulences created by the interaction between the rotors and the environment, and (2) the flight in autonomy with very little light.
9.2.4 Inria-DGA Resilient Humanoid
- Program: Convention Inria-DGA about Artificial Intelligence
- Project title: Resilient humanoid
- Duration: October 2019 – October 2022
- PI for Inria: Jean-Baptiste Mouret
- Summary: This project aims at designing adaptive controllers for humanoid robots: a tele-operated humanoid should be able to adapt to unforeseen conditions, including damage and obstacles. To do so, this project bridges the gap between task-space whole-body controllers based on quadratic programming and data-efficient reinforcement learning. [funding for 1 research engineer]
9.2.5 Inria-DGA U-Drone
- Program: Convention Inria-DGA about Artificial Intelligence
- Project acronym: U-Drone
- Project title: Underground Drone
- Duration: October 2019 – October 2022
- PI for Inria: Jean-Baptiste Mouret
- Summary: This project aims at making progress in the design of flying vehicles for highly confined spaces like pipes, caves, quarries, etc. It complements the Proxilearn ANR project. [funding for 1 research engineer]
9.2.6 DGA-RAPID ASMOA
- Program: DGA-RAPID
- Project acronym: ASMOA
- Project title: Prédiction des intentions d'un utilisateur d'un exosquelette
- Duration: October 2019 – October 2022
- Coordinator: Kompaï Robotics
- PI for Inria: François Charpillet
-
Partners:
- Inria
- Safran Electronics & Defense
- Summary: The ASMOA project is about intention prediction for exoskeleton control. The challenge is to detect the intention of movement of a user of exoskeletons for load handling, to optimize the exoskeleton control following the user’s intended movement, and to evaluate with an experimental campaign the effect of the intelligent controller on the user’s movement and performance. INRIA will develop the prediction and control method based on machine learning techniques for an upper-body active exoskeleton.
9.3 Regional initiatives
TELEMOVTOP
- Title: TELEMOVTOP
- Funding: AMI Numerique Grand Est
- Duration: 2020/10–2022/06
- Coordinator: ISOTOP Etancheite
- Partner: Inria
- Inria contact: Serena Ivaldi
- Summary: The purpose of the project is to create a new intelligent and interactive robot to automate the process of disposal of metal sheets on roofs contaminated by asbestos. The goal is to reduce the risks of contamination for workers exposed to asbestos, the risks of pollution, and at the same time introducing a novel robot in a market that needs to be innovated. The robot will have to be teleoperated by workers that are on remote sites; an interactive autonomy mode will leverage the robot's intelligent and autonomous behaviors while enabling the workers to supervise the job. Inria is reponsible for the high-level control, teleoperaton and HMI of the system.
EXOTURN
- Title: EXOTURN
- Funding: Inria-COVID19 projects and internal funding
- Duration: 2020/03–2022/03
- Coordinator: Serena Ivaldi (Inria) & Dr. Nicla Settembre (CHRU Nancy)
-
Partner:
- INRS
- CHRU Nancy
- Inria (with CNRS and University of Lorraine)
- Inria contact: Serena Ivaldi
- Summary: ExoTurn is an interdisciplinary project that was rapidly started in March 2020 to help the Hospital of Nancy (CHRU) in the fight against the COVID-19. The project is coordinated jointly by Serena Ivaldi (Inria) and Dr. Nicla Settembre (Hospital of Nancy) with the participation of INRS, CNRS and University of Lorraine. The goal of the project is to investigate the feasibility and help of back-support exoskeletons to assist the medical staff during the exhausting procedures of Prone Positioning (PP) maneuvers in the Intensive Care Unit. Prone Positioning is a complex procedure that is necessary during treatment of acute respiratory distress syndrome (ARDS) to optimise oxygenation.
10 Dissemination
10.1 Scientific events: selection
General chair, scientific chair
- Olivier Buffet: Co-conference chair of the 30th international conference on automated planning and scheduling (ICAPS 2020), held virtually (while originally set in Nancy), 19–30 October 2020. 26
Member of the organizing committees
- Pauline Maurice and Serena Ivaldi: Co-organizers of a workshop at the International Conference on Robotics and Automation (IEEE/RAS ICRA 2020) ("Human movement science for physical human-robot collaboration").
- Serena Ivaldi: co-organizer of IEEE/RAS ICRA 2020 Workshop on "Shared Autonomy: Learning and Control" and IEEE ROMAN 2020 Workshop on "Cognitive Robotics for Interaction".
Member of the conference program committees
- Jean-Baptiste Mouret: Senior Program committee of the Artificial Life conference (ALIFE), Program Committee Member of the Genetic and Evolutionary Computation Conference (GECCO 2020)
- François Charpillet : Senior Program Committee Member of the 24th European Conference on Artificial Intelligence (ECAI 2020), Program Committee Member of the 34th AAAI conference on Artificial Intelligence (AAAI 2020), Program Committee Member of the 29th Joint Conference on Artificial Intelligence (IJCAI 2020), Journées Francophones sur les Systèmes Multi-Agents (JFSMA 2020), Activity and Behavior Computing (ABC 2020).
- Francis Colas: Program Committee Member of the 24th European Conference on Artificial Intelligence (ECAI 2020)
- Vincent Thomas : Program Committee Member of the 24th European Conference on Artificial Intelligence (ECAI 2020), Journées Francophones sur la Planification, la Décision et l’Apprentissage pour la conduite de systèmes (JFPDA 2020).
- Pauline Maurice: Associate Editor for IEEE/RAS International Conference on Robotics and Automation (ICRA 2021)
- Olivier Buffet: Program Committee Member of the 29th Joint Conference on Artificial Intelligence (IJCAI 2020), Journées Francophones sur la Planification, la Décision et l’Apprentissage pour la conduite de systèmes (JFPDA 2020).
- Amine Boumaza: Program Committee Member the Genetic and Evolutionary Computation Conference (GECCO 2020) and the Congress on Evolutionary Computation(CEC 2020)
Reviewer
- Joint IEEE 10th International Conference on Development and Learning and Epigenetic Robotics (ICDL-EpiRob) [Olivier Buffet, Serena Ivaldi]
- IEEE/RSJ International Conference on Intelligent Robots and Systems (IROS 2020) [Francis Colas, Jean-Baptiste Mouret]
- IEEE International Conference on Robotics and Automation (ICRA 2021) [Francis Colas, Pauline Maurice, Jean-Baptiste Mouret]
- 42nd Annual Conference of the European Association for Computer Graphics (Eurographics'21) [Francis Colas]
- Robotics: Science and Systems (RSS) 2020 [Serena Ivaldi, Jean-Baptiste Mouret]
- Conference on Robot Learning (CORL) 2020 [Serena Ivaldi]
- The 21st Triennial Congress of the International Ergonomics Association (IEA) 2021 [Serena Ivaldi, Pauline Maurice]
10.1.1 Journal
Member of the editorial boards
- IEEE Robotics and Automation Letters [Serena Ivaldi - associate editor]
- ACM Transactions on Evolutionary Learning and Optimization [Jean-Baptiste Mouret - associate editor]
Reviewer - reviewing activities
- IEEE Robotics and Automation Letters [Francis Colas, Serena Ivaldi, Pauline Maurice, Jean-Baptiste Mouret]
- ACM Transactions on Evolutionary Learning and Optimization [Jean-Baptiste Mouret]
- Nature [Serena Ivaldi]
- Nature Machine Intelligence [Jean-Baptiste Mouret]
- PlosOne [Pauline Maurice]
- PLOS Computation Biology [Jean-Baptiste Mouret]
- Artificial Life [Jean-Baptiste Mouret]
- IEEE Transactions on Medical Robotics and Bionics [Pauline Maurice]
- Wearable Technologies [Pauline Maurice]
- IEEE Robotics and Automation Magazine (RAM) [Serena Ivaldi]
- IEEE Access [Serena Ivaldi]
- Frontiers Robotics and AI [Amine Boumaza]
10.1.2 Invited talks
- Invited talk at the workshop on "Socio-Physical Interaction Skills for Cooperative Human-Robot Systems in Agile Production" at the I-RIM conference [Pauline Maurice].
- Invited talk at the "Robotics and AI" seminar organized by the French Association for Artificial Intelligence [Pauline Maurice].
- Invited talk at RSS 2020 Workshop on "Closing the academia to real-world gap in service robotics" [Serena Ivaldi].
- Invited talk at IROS 2020 Workshop on "Ergonomic Physical Human-Robot Collaboration" [Serena Ivaldi].
- Invited talk at GDR Robotique "Journée GT5 Perception et interaction homme-robot 2020" [Serena Ivaldi].
10.1.3 Leadership within the scientific community
- Serena Ivaldi joined the steering committee of Robotics for Infectious Diseases (http://
roboticsforinfectiousdiseases. org). - François Charpillet is member of the scientific commitee of GDR on Robotics.
10.1.4 Scientific expertise
- François Charpillet : review of a project submitted for a research grant from the Villum Foundation in Denmark, and a review of a project submitted for a research grant from HES-SO (Haute école spécialisée de Suisse occidentale - https://
www. hes-so. ch).
10.2 Teaching - Supervision - Juries
10.2.1 Teaching
- Master: Alexis Scheuer, “Introduction à la robotique autonome”, 30h eq. TD, M1 informatique, Univ. Lorraine, France.
- Master: Alexis Scheuer, “Vie artificielle”, 22,5h eq. TD, 3ème année, CentraleSupélec, France.
- Master: Francis Colas, “Planification de trajectoires”, 12h eq. TD, M2 “Apprentissage, Vision, Robotique”, Univ. Lorraine, France.
- Master: Alexis Scheuer, “Modélisation et commande en robotique”, 16h eq. TD, M2 “Apprentissage, Vision, Robotique”, Univ. Lorraine, France.
- Master: Francis Colas, “Autonomous Robotics”, 39.5h eq. TD, M1, CentraleSupélec, France.
- Master: Pauline Maurice, “Analyse Comportementale”, 10h CM/TP, M2 “Sciences Cognitives”, Univ. Lorraine, France.
- Master: Serena Ivaldi, “Analyse Comportementale”, 16h CM/TD, M2 “Sciences Cognitives”, Univ. Lorraine, France.
- Master: Amine Boumaza, “Méta-heuristiques et recherche locale stochastique”, 30h eq. TD, M1 informatique, Univ. Lorraine, France.
- Master: Vincent Thomas, “Apprentissage et raisonnement dans l'incertain”, 15h eq. TD, M2 M2 “Apprentissage, Vision, Robotique”, Univ. Lorraine, France.
- Master: Vincent Thomas, “Game Design”, 30h eq. TD, M1 Sciences Cognitives, Univ. Lorraine, France.
- Master: Vincent Thomas, “Agent intelligent”, 30h eq. TD, M1 Sciences Cognitives, Univ. Lorraine, France.
- Tutorial, Jean-Baptiste Mouret, “Quality Diversity Optimization”, 3h, ACM GECCO 2020 (with A. Cully, Imperial College, and S. Doncieux, Sorbonne Université)
10.2.2 Supervision
- PhD: Adrien Malaisé, “Capteurs portés dans la robotique collaborative : de l'apprentissage du mouvement à l'acceptabilité de cette technologie”, started in January 2017 and defended on 2020-07-07, Francis Colas (advisor), Serena Ivaldi (co-advisor).
- PhD: Melanie Jouaiti, “Améliorer la coordination motrice dans les interactions Homme-Robot avec des contrôleurs bio-inspirés”, started in October 2017 and defended on 2020-06-17, François Charpillet (advisor).
- PhD: Rituraj Kaushik, “Data-Efficient Robot Learning using Priors from Simulators”, started in October 2016 and defended on 2020-07-23, Jean-Baptiste Mouret (advisor)
- PhD: Adam Gaier, “Accelerating Evolutionary Design Exploration with Predictive and Generative Models”, started in October 2016 and defended on 2020-07-17, Jean-Baptiste Mouret (Advisor)
- PhD in progress: Yang You, “Modèles probabilistes pour la collaboration humain-robot”, started in October 2019, Olivier Buffet (advisor), Vincent Thomas (co-advisor)
- PhD in progress: Timothée Anne “Meta-learning for adaptive whole-body control”, started in September 2020, Jean-Baptiste Mouret (advisor)
- PhD in progress: Vladislav Tempez, “Learning to fly a micro-UAVs in highly confined environments”, started in October 2018, Jean-Baptiste Mouret (advisor), Franck Ruffier (co-advisor, CNRS/Aix-Marseille Université)
- PhD in progress: Waldez Azevedo Gomes Junior, “Intelligent Human-Robot collaboration”, started in October 2018, Jean-Baptiste Mouret (advisor), Serena Ivaldi (co-advisor)
- PhD in progress: Yoann Fleytoux, “Human-guided manipulation learning of irregular objects”, started in April 2019, Jean-Baptiste Mouret (advisor), Serena Ivaldi (co-advisor)
- PhD in progress: Luigi Penco, “Intelligent whole-body tele-operation of humanoid robots”, started in April 2019, Jean-Baptiste Mouret (advisor), Serena Ivaldi (co-advisor)
- PhD in progress: Lorenzo Vianello, “Adaptation in human-robot collaboration”, started in December 2019, Alexis Aubry (advisor, CRAN, Univ. Lorraine), Serena Ivaldi (co-advisor)
- PhD in progress : Yassine EL Khadiri, Cifre with Diatelic, “Machine learning for Ambient Assisted Living (AAL)”, started in June 2017, François Charpillet adviser.
- PhD in progress : Eloise Zehnder, “Elderly-technology interaction: accessibility and acceptability of assistive technology (at) at home”, started October 2018, François Charpillet (advisor), Jérome Dinet (advisor, 2LPN).
- PhD in progress : Julien Uzzan, Cifre with PSA, “Navigation of an autonomous vehicle in a complex environment using unsupervised or semi-supervised learning”, started in January 2019, François Charpillet (advisor), François Aioun (co-advisor, PSA).
- PhD in progress : Jessica Colombel, “Analysis of human movement for assistance”, started in Feburary 2019, François Charpillet (advisor) David Daney(advisor, Auctus team, Bordeaux)
- PhD in progress : Nicolas Gauville, Cifre with SAFRAN, “Coordination of autonomous robots evolving in unstructured and unknown environment for Search and Rescue”, started in May 2019, François Charpillet (advisor), Christophe Guettier (co-adviser, Safran).
- PhD in progress : Lina Achaji, Cifre with PSA, “Modélisation de systeèmes dynamiques par des réseaux de neurones à mémoire courte et longue : application pour la prédiction de l'état d'environnement routier”, started in March 2020, François Charpillet (advisor), François Aioun (Co-advisor, PSA).
- PhD in progress: Nima Mehdi, “Perception et interprétation de l’activité humaine”, started in May 2020, Francis Colas (advisor), Serena Ivaldi (co-advisor).
- PhD in progress: Jacques Zhong, “Prise en compte de la variabilité de morphologie de l’opérateur dans des tâches de montage simulées en réalité virtuelle”, started in October 2020, Francis Colas (advisor), Pauline Maurice (co-advisor), Vincent Weistroffer (co-advisor, CEA-LIST).
- PhD in progress: Raphaël Bousigues, “Collaborative robots as a tool for optimizing skill acquisition through the appropriate use of human motor variability”, started in December 2020, Pauline Maurice (co-advisor), Vincent Padois (advisor, INRIA Bordeaux), David Daney (co-advisor, INRIA Bordeaux).
10.2.3 Juries
- François Charpillet was:
- a reviewer for the PhD of Guillaume Bono Univ. Lyon - INSA équipe Chroma
- a reviewer for the PhD of Charles Cocquet Univ. Bretagne - Lab-Sticc
- a reviewer for the PhD of François Suro Univ. Montpellier - LIRMM
- a reviewer for the PhD of Ludovic Minevelle, Univ. Paris Saclay - ENS
- Jury Chair for the PhD of Guillaume Carbajal Univ. Lorraine - Loria
- Olivier Buffet was:
- a reviewer for the PhD of Erwann Lecarpentier (Institut Supérieur de l'Aéronautique et de l'Espace)
- a reviewer for the PhD of Guillaume Matheron (Sorbonne Université)
- Jean-Baptiste Mouret was:
- a reviewer for the PhD of Noémie Jacquier (École Polytechnique Fédérale de Lausanne, Switzerland)
- Serena Ivaldi was:
- an examiner for the PhD of Aliaa Moualla (Université de Cergy-Pontoise)
- an examiner for the PhD of Anthony Voilque (Université Clermont Auvergne)
- an examiner for the PhD Sebastien Mick (Université de Bordeaux)
- a reviewer for the PhD of Amel Cherif (Italian Institute of Technology & University of Genoa, Italy)
10.3 Popularization
10.3.1 Internal or external Inria responsibilities
- Serena Ivaldi talked about robotics at the Inria web event for Fête de la Science: "Cobots, ces précieux robots collaborateurs – Inria fête la science 2020". Video sur Youtube: https://
youtu. be/ irT_uPqQ9Hk. - Alexis Scheuer proposed online activities using a Thymio simulator for Fête de la Science: https://
members. loria. fr/ AScheuer/ comm/ fete-science-2020.
10.3.2 Articles and contents
- Vincent Thomas was a reviewer for a popularization article on information-oriented planning https://
papiermachesciences. org/. - Serena Ivaldi and Pauline Maurice were featured in several articles and TV reportage about the ExoTurn project:
- TV: France 5 (Le Magazine de la santé), France 3 Lorraine
- Radio: Europe 1, Magnum la Radio, France Bleu
- Articles: Global-Industrie, Travail & Securité, L'Est Républicain, Le Journal CNRS
10.3.3 Interventions
- Pauline Maurice participated in “Journée Parité” organized by Loria for high school students.
11 Scientific production
11.1 Major publications
- 1 articleRobots that can adapt like animalsNature5217553May 2015, 503-507
- 2 articleOptimally Solving Dec-POMDPs as Continuous-State MDPsJournal of Artificial Intelligence Research55February 2016, 443-497
11.2 Publications of the year
International journals
International peer-reviewed conferences
National peer-reviewed Conferences
Conferences without proceedings
Scientific book chapters
Edition (books, proceedings, special issue of a journal)
Doctoral dissertations and habilitation theses
Reports & preprints
11.3 Cited publications
- 35 article Prioritized Motion-Force Control of Constrained Fully-Actuated Robots: "Task Space Inverse Dynamics" Robotics and Autonomous Systems 2014, URL: http://dx.doi.org/10.1016/j.robot.2014.08.016
- 36 inproceedings Multi-robot taboo-list exploration of unknown structured environments 2015 IEEE/RSJ International Conference on Intelligent Robots and Systems (IROS 2015) Hamburg, Germany September 2015
- 37 inproceedings A POMDP Extension with Belief-dependent Rewards Advances in Neural Information Processing Systems (NIPS) Vancouver, Canada MIT Press December 2010
- 38 articleSpecial Issue on Long-Term AutonomyThe International Journal of Robotics Research32142013, 1609--1610
- 39 articleQuadratic programming for multirobot and task-space force controlIEEE Transactions on Robotics3512018, 64--77
- 40 inproceedingsStair climbing stabilization of the HRP-4 humanoid robot using whole-body admittance control2019 International Conference on Robotics and Automation (ICRA)IEEE2019, 277--283
- 41 articleReset-free trial-and-error learning for robot damage recoveryRobotics and Autonomous Systems1002018, 236--250
- 42 inproceedingsPose Estimation For A Partially Observable Human Body From RGB-D CamerasIEEE/RJS International Conference on Intelligent Robots and Systems (IROS)Hamburg, GermanySeptember 2015, 8
- 43 inproceedings Simultaneous Tracking and Activity Recognition (STAR) using Advanced Agent-Based Behavioral Simulations ECAI - Proceedings of the Twenty-first European Conference on Artificial Intelligence Pragues, Czech Republic August 2014
- 44 inproceedings Comparison of Selection Methods in On-line Distributed Evolutionary Robotics ALIFE 14: The fourteenth international conference on the synthesis and simulation of living systems Artificial Life 14 New York, United States July 2014
- 45 articleInterview: Is SLAM Solved?KI - Künstliche Intelligenz2432010, 255-257URL: http://dx.doi.org/10.1007/s13218-010-0047-x
- 46 article Reinforcement Learning in Robotics: A Survey The International Journal of Robotics Research August 2013
- 47 articleFast damage recovery in robotics with the t-resilience algorithmThe International Journal of Robotics Research32142013, 1700--1723
- 48 article Illuminating search spaces by mapping elites arXiv preprint arXiv:1504.04909 2015
- 49 inproceedingsRobust real-time whole-body motion retargeting from human to humanoid2018 IEEE-RAS 18th International Conference on Humanoid Robots (Humanoids)IEEE2018, 425--432
- 50 inproceedingsLong-term 3D map maintenance in dynamic environmentsRobotics and Automation (ICRA), 2014 IEEE International Conference onIEEE2014, 3712--3719
- 51 articleImplementing Torque Control with High-Ratio Gear Boxes and without Joint-Torque SensorsInternational Journal of Humanoid Robotics2016, 1550044URL: https://hal.archives-ouvertes.fr/hal-01136936/document
- 52 techreportRobotics 2020 Multi-Annual Roadmap2014, URL: http://www.eu-robotics.net/ppp/objectives-of-our-topic-groups/
- 53 inproceedingsImproved human-robot team performance using Chaski, A human-inspired plan execution system ACM/IEEE International Conference on Human-Robot Interaction (HRI)2011, 29-36
- 54 inproceedings Interactive Surface for Bio-inspired Robotics, Re-examining Foraging Models 23rd IEEE International Conference on Tools with Artificial Intelligence (ICTAI) Boca Raton, United States IEEE November 2011
- 55 inproceedingsRole determination in human-human interaction3rd Joint EuroHaptics Conf. and World Haptics2009, 51-56
- 56 book Introduction to Reinforcement Learning MIT Press 1998
- 57 articleThe grand challenges in Socially Assistive RoboticsIEEE Robotics and Automation Magazine - Special Issue on Grand challenges in Robotics1412007, 1-7
- 58 inproceedings Planification Monte Carlo orientée information JFPDA 2019 - Journées Francophones sur la Planification, la Décision et l'Apprentissage pour la conduite de systèmes Toulouse, France July 2019
- 59 articleAttentional Models of Multitask Pilot Performance Using Advanced Display TechnologyHuman Factors4532003, 360-380
- 60 articleSimultaneous Tracking and Activity Recognition (STAR) Using Many Anonymous, Binary Sensors34682005, 62-79URL: http://dx.doi.org/10.1007/11428572_5
- 61 articleSocial Robots: Views of Staff of a Disability Service OrganizationInternational Journal of Social Robotics632014, 457-468