Keywords
Computer Science and Digital Science
- A3.3.2. Data mining
- A3.3.3. Big data analysis
- A3.4.1. Supervised learning
- A3.4.2. Unsupervised learning
- A3.4.6. Neural networks
- A3.4.8. Deep learning
- A5.3.3. Pattern recognition
- A5.4.1. Object recognition
- A5.4.3. Content retrieval
- A5.7. Audio modeling and processing
- A5.7.1. Sound
- A5.7.3. Speech
- A5.8. Natural language processing
- A9.2. Machine learning
- A9.3. Signal analysis
- A9.4. Natural language processing
Other Research Topics and Application Domains
- B9. Society and Knowledge
- B9.3. Medias
- B9.6.10. Digital humanities
- B9.10. Privacy
1 Team members, visitors, external collaborators
Research Scientists
- Laurent Amsaleg [Team leader, CNRS, Senior Researcher, HDR]
- Ioannis Avrithis [Inria, Advanced Research Position, HDR]
- Vincent Claveau [CNRS, Researcher, HDR]
- Teddy Furon [Inria, Researcher, HDR]
- Guillaume Gravier [CNRS, Senior Researcher, HDR]
Faculty Members
- Ewa Kijak [Univ de Rennes I, Associate Professor]
- Simon Malinowski [Univ de Rennes I, Associate Professor]
- Pascale Sébillot [INSA Rennes, Professor, HDR]
Post-Doctoral Fellow
- Suresh Kirthi Kumaraswamy [CNRS, from Mar 2020 until May 2020]
PhD Students
- Benoit Bonnet [Inria]
- Antoine Chaffin [Imatag, from Nov 2020]
- Cheikh Brahim El Vaigh [Inria, until Sep 2020]
- Deniz Engin [InterDigital, from Sep 2020]
- Marzieh Gheisari Khorasgani [Inria]
- Yann Lifchitz [Groupe SAFRAN]
- Thibault Maho [Inria, from Sep 2020]
- Cyrielle Mallart [Ouest-France Quotidien]
- Duc Hau Nguyen [CNRS, from Sep 2020]
- Raquel Pereira De Almeida [Université pontificale catholique du Minas Gerais Brésil, until Feb 2020]
- Samuel Tap [Zama SAS, from Dec 2020]
- Karim Tit [Thales, from Dec 2020]
- Francois Torregrossa [Pages Jaunes]
- Shashanka Venkataramanan [Inria, from Dec 2020]
- Hanwei Zhang [China Scholarship Council]
Technical Staff
- Mateusz Budnik [Inria, Engineer]
- Guillaume Le Noe-Bienvenu [CNRS, Engineer]
- Florent Michel [Inria, Engineer, until Apr 2020]
Interns and Apprentices
- Antoine Chaffin [Univ de Rennes I, from Feb 2020 until Jul 2020]
- Jade Garcia Bourrée [CNRS, from Jun 2020 until Jul 2020]
- Yoann Lemesle [CNRS, from Jun 2020 until Jul 2020]
- Timothee Neitthoffer [Inria, from Mar 2020 until Aug 2020]
- Vasileios Psomas [Inria, from Feb 2020 until May 2020]
Administrative Assistant
- Aurélie Patier [Univ de Rennes I]
Visiting Scientists
- Amaia Abanda Elustondo [Basque Center for Applied Mathematics, from Sep 2020]
- Filippos Bellos [National and Kapodistrian University of Athens, from Oct 2020]
- Josu Ircio Fernandez [Center for Technological Research Spain, from Oct 2020]
- Michalis Lazarou [Imperial College London, from Sep 2020]
External Collaborator
- Suresh Kirthi Kumaraswamy [Le Mans Université, until Mar 2020]
2 Overall objectives
2.1 Context
Linkmedia is concerned with the processing of extremely large collections of multimedia material. The material we refer to are collections of documents that are created by humans and intended for humans. It is material that is typically created by media players such as TV channels, radios, newspapers, archivists (BBC, INA, ...), as well as the multimedia material that goes through social-networks. It also includes material that includes images, videos and pathology reports for e-health applications, or that is in relation with e-learning which typically includes a fair amount of texts, graphics, images and videos associating in new ways teachers and students. It also includes material in relation with humanities that study societies through the multimedia material that has been produced across the centuries, from early books and paintings to the latest digitally native multimedia artifacts. Some other multimedia material are out of the scope of Linkmedia, such as the ones created by cameras or sensors in the broad areas of video-surveillance or satellite images.
Multimedia collections are rich in contents and potential, that richness being in part within the documents themselves, in part within the relationships between the documents, in part within what humans can discover and understand from the collections before materializing its potential into new applications, new services, new societal discoveries, ... That richness, however, remains today hardly accessible due to the conjunction of several factors originating from the inherent nature of the collections, the complexity of bridging the semantic gap or the current practices and the (limited) technology:
- Multimodal: multimedia collections are composed of very diverse material (images, texts, videos, audio, ...), which require sophisticated approaches at analysis time. Scientific contributions from past decades mostly focused on analyzing each media in isolation one from the other, using modality-specific algorithms. However, revealing the full richness of collections calls for jointly taking into account these multiple modalities, as they are obviously semantically connected. Furthermore, involving resources that are external to collections, such as knowledge bases, can only improve gaining insight into the collections. Knowledge bases form, in a way, another type of modality with specific characteristics that also need to be part of the analysis of media collections. Note that determining what a document is about possibly mobilizes a lot of resources, and this is especially costly and time consuming for audio and video. Multimodality is a great source of richness, but causes major difficulties for the algorithms running analysis;
- Intertwined: documents do not exist in isolation one from the other. There is more knowledge in a collection than carried by the sum of its individual documents and the relationships between documents also carry a lot of meaningful information. (Hyper)Links are a good support for materializing the relationships between documents, between parts of documents, and having analytic processes creating them automatically is challenging. Creating semantically rich typed links, linking elements at very different granularities is very hard to achieve. Furthermore, in addition to being disconnected, there is often no strong structure into each document, which makes even more difficult their analysis;
- Collections are very large: the scale of collections challenges any algorithm that runs analysis tasks, increasing the duration of the analysis processes, impacting quality as more irrelevant multimedia material gets in the way of relevant ones. Overall, scale challenges the complexity of algorithms as well as the quality of the result they produce;
- Hard to visualize: It is very difficult to facilitate humans getting insight on collections of multimedia documents because we hardly know how to display them due to their multimodal nature, or due to their number. We also do not know how to well present the complex relationships linking documents together: granularity matters here, as full documents can be linked with small parts from others. Furthermore, visualizing time-varying relationships is not straightforward. Data visualization for multimedia collections remains quite unexplored.
2.2 Scientific objectives
The ambition of Linkmedia is to propose foundations, methods, techniques and tools to help humans make sense of extremely large collections of multimedia material. Getting useful insight from multimedia is only possible if tools and users interact tightly. Accountability of the analysis processes is paramount in order to allow users understanding their outcome, to understand why some multimedia material was classified this way, why two fragments of documents are now linked. It is key for the acceptance of these tools, or for correcting errors that will exist. Interactions with users, facilitating analytics processes, taking into account the trust in the information and the possible adversarial behaviors are topics Linkmedia addresses.
3 Research program
3.1 Scientific background
Linkmedia is de facto a multidisciplinary research team in order to gather the multiple skills needed to enable humans to gain insight into extremely large collections of multimedia material. It is multimedia data which is at the core of the team and which drives the design of our scientific contributions, backed-up with solid experimental validations. Multimedia data, again, is the rationale for selecting problems, applicative fields and partners.
Our activities therefore include studying the following scientific fields:
- multimedia: content-based analysis; multimodal processing and fusion; multimedia applications;
- computer vision: compact description of images; object and event detection;
- machine learning: deep architectures; structured learning; adversarial learning;
- natural language processing: topic segmentation; information extraction;
- information retrieval: high-dimensional indexing; approximate k-nn search; embeddings;
- data mining: time series mining; knowledge extraction.
3.2 Workplan
Overall, Linkmedia follows two main directions of research that are (i) extracting and representing information from the documents in collections, from the relationships between the documents and from what user build from these documents, and (ii) facilitating the access to documents and to the information that has been elaborated from their processing.
3.3 Research Direction 1: Extracting and Representing Information
Linkmedia follows several research tracks for extracting knowledge from the collections and representing that knowledge to facilitate users acquiring gradual, long term, constructive insights. Automatically processing documents makes it crucial to consider the accountability of the algorithms, as well as understanding when and why algorithms make errors, and possibly invent techniques that compensate or reduce the impact of errors. It also includes dealing with malicious adversaries carefully manipulating the data in order to compromise the whole knowledge extraction effort. In other words, Linkmedia also investigates various aspects related to the security of the algorithms analyzing multimedia material for knowledge extraction and representation.
Knowledge is not solely extracted by algorithms, but also by humans as they gradually get insight. This human knowledge can be materialized in computer-friendly formats, allowing algorithms to use this knowledge. For example, humans can create or update ontologies and knowledge bases that are in relation with a particular collection, they can manually label specific data samples to facilitate their disambiguation, they can manually correct errors, etc. In turn, knowledge provided by humans may help algorithms to then better process the data collections, which provides higher quality knowledge to humans, which in turn can provide some better feedback to the system, and so on. This virtuous cycle where algorithms and humans cooperate in order to make the most of multimedia collections requires specific support and techniques, as detailed below.
Machine Learning for Multimedia Material.
Many approaches are used to extract relevant information from multimedia material, ranging from very low-level to higher-level descriptions (classes, captions, ...). That diversity of information is produced by algorithms that have varying degrees of supervision. Lately, fully supervised approaches based on deep learning proved to outperform most older techniques. This is particularly true for the latest developments of Recurrent Neural Networkds (RNN, such as LSTMs) or convolutional neural network (CNNs) for images that reach excellent performance 65. Linkmedia contributes to advancing the state of the art in computing representations for multimedia material by investigating the topics listed below. Some of them go beyond the very processing of multimedia material as they also question the fundamentals of machine learning procedures when applied to multimedia.
- Learning from few samples/weak supervisions. CNNs and RNNs need large collections of carefully annotated data. They are not fitted for analyzing datasets where few examples per category are available or only cheap image-level labels are provided. Linkmedia investigates low-shot, semi-supervised and weakly supervised learning processes: Augmenting scarce training data by automatically propagating labels 68, or transferring what was learned on few very well annotated samples to allow the precise processing of poorly annotated data 77. Note that this context also applies to the processing of heritage collections (paintings, illuminated manuscripts, ...) that strongly differ from contemporary natural images. Not only annotations are scarce, but the learning processes must cope with material departing from what standard CNNs deal with, as classes such as "planes", "cars", etc, are irrelevant in this case.
- Ubiquitous Training. NN (CNNs, LSTMs) are mainstream for producing representations suited for high-quality classification. Their training phase is ubiquitous because the same representations can be used for tasks that go beyond classification, such as retrieval, few-shot, meta- and incremental learning, all boiling down to some form of metric learning. We demonstrated that this ubiquitous training is relatively simpler 68 yet as powerful as ad-hoc strategies fitting specific tasks 81. We study the properties and the limitations of this ubiquitous training by casting metric learning as a classification problem.
- Beyond static learning. Multimedia collections are by nature continuously growing, and ML processes must adapt. It is not conceivable to re-train a full new model at every change, but rather to support continuous training and/or allowing categories to evolve as the time goes by. New classes may be defined from only very few samples, which links this need for dynamicity to the low-shot learning problem discussed here. Furthermore, active learning strategies determining which is the next sample to use to best improve classification must be considered to alleviate the annotation cost and the re-training process 72. Eventually, the learning process may need to manage an extremely large number of classes, up to millions. In this case, there is a unique opportunity of blending the expertise of Linkmedia on large scale indexing and retrieval with deep learning. Base classes can either be "summarized" e.g. as a multi-modal distribution, or their entire training set can be made accessible as an external associative memory 87.
- Learning and lightweight architectures. Multimedia is everywhere, it can be captured and processed on the mobile devices of users. It is necessary to study the design of lightweight ML architectures for mobile and embedded vision applications. Inspired by 91, we study the savings from quantizing hyper-parameters, pruning connections or other approximations, observing the trade-off between the footprint of the learning and the quality of the inference. Once strategy of choice is progressive learning which early aborts when confident enough 73.
- Multimodal embeddings. We pursue pioneering work of Linkmedia on multimodal embedding, i.e., representing multiple modalities or information sources in a single embedded space 85, 84, 86. Two main directions are explored: exploiting adversarial architectures (GANs) for embedding via translation from one modality to another, extending initial work in 86 to highly heterogeneous content; combining and constraining word and RDF graph embeddings to facilitate entity linking and explanation of lexical co-occurrences 62.
- Accountability of ML processes. ML processes achieve excellent results but it is mandatory to verify that accuracy results from having determined an adequate problem representation, and not from being abused by artifacts in the data. Linkmedia designs procedures for at least explaining and possibly interpreting and understanding what the models have learned. We consider heat-maps materializing which input (pixels, words) have the most importance in the decisions 80, Taylor decompositions to observe the individual contributions of each relevance scores or estimating LID 49 as a surrogate for accounting for the smoothness of the space.
- Extracting information. ML is good at extracting features from multimedia material, facilitating subsequent classification, indexing, or mining procedures. Linkmedia designs extraction processes for identifying parts in the images 78, 79, relationships between the various objects that are represented in images 55, learning to localizing objects in images with only weak, image-level supervision 80 or fine-grained semantic information in texts 60. One technique of choice is to rely on generative adversarial networks (GAN) for learning low-level representations. These representations can e.g. be based on the analysis of density 90, shading, albedo, depth, etc.
- Learning representations for time evolving multimedia material. Video and audio are time evolving material, and processing them requests to take their time line into account. In 74, 58 we demonstrated how shapelets can be used to transform time series into time-free high-dimensional vectors, preserving however similarities between time series. Representing time series in a metric space improves clustering, retrieval, indexing, metric learning, semi-supervised learning and many other machine learning related tasks. Research directions include adding localization information to the shapelets, fine-tuning them to best fit the task in which they are used as well as designing hierarchical representations.
Adversarial Machine Learning.
Systems based on ML take more and more decisions on our behalf, and maliciously influencing these decisions by crafting adversarial multimedia material is a potential source of dangers: a small amount of carefully crafted noise imperceptibly added to images corrupts classification and/or recognition. This can naturally impact the insight users get on the multimedia collection they work with, leading to taking erroneous decisions e.g.
This adversarial phenomenon is not particular to deep learning, and can be observed even when using other ML approaches 54. Furthermore, it has been demonstrated that adversarial samples generalize very well across classifiers, architectures, training sets. The reasons explaining why such tiny content modifications succeed in producing severe errors are still not well understood.
We are left with little choice: we must gain a better understanding of the weaknesses of ML processes, and in particular of deep learning. We must understand why attacks are possible as well as discover mechanisms protecting ML against adversarial attacks (with a special emphasis on convolutional neural networks). Some initial contributions have started exploring such research directions, mainly focusing on images and computer vision problems. Very little has been done for understanding adversarial ML from a multimedia perspective 59.
Linkmedia is in a unique position to throw at this problem new perspectives, by experimenting with other modalities, used in isolation one another, as well as experimenting with true multimodal inputs. This is very challenging, and far more complicated and interesting than just observing adversarial ML from a computer vision perspective. No one clearly knows what is at stake with adversarial audio samples, adversarial video sequences, adversarial ASR, adversarial NLP, adversarial OCR, all this being often part of a sophisticated multimedia processing pipeline.
Our ambition is to lead the way for initiating investigations where the full diversity of modalities we are used to work with in multimedia are considered from a perspective of adversarial attacks and defenses, both at learning and test time. In addition to what is described above, and in order to trust the multimedia material we analyze and/or the algorithms that are at play, Linkmedia investigates the following topics:
- Beyond classification. Most contributions in relation with adversarial ML focus on classification tasks. We started investigating the impact of adversarial techniques on more diverse tasks such as retrieval 48. This problem is related to the very nature of euclidean spaces where distances and neighborhoods can all be altered. Designing defensive mechanisms is a natural companion work.
- Detecting false information. We carry-on with earlier pioneering work of Linkmedia on false information detection in social media. Unlike traditional approaches in image forensics 63, we build on our expertise in content-based information retrieval to take advantage of the contextual information available in databases or on the web to identify out-of-context use of text or images which contributed to creating a false information 75.
- Deep fakes. Progress in deep ML and GANs allow systems to generate realistic images and are able to craft audio and video of existing people saying or doing things they never said or did 71. Gaining in sophistication, these machine learning-based "deep fakes" will eventually be almost indistinguishable from real documents, making their detection/rebutting very hard. Linkmedia develops deep learning based counter-measures to identify such modern forgeries. We also carry on with making use of external data in a provenance filtering perspective 92 in order to debunk such deep fakes.
- Distributions, frontiers, smoothness, outliers. Many factors that can possibly explain the adversarial nature of some samples are in relation with their distribution in space which strongly differs from the distribution of natural, genuine, non adversarial samples. We are investigating the use of various information theoretical tools that facilitate observing distributions, how they differ, how far adversarial samples are from benign manifolds, how smooth is the feature space, etc. In addition, we are designing original adversarial attacks and develop detection and curating mechanisms 49.
Multimedia Knowledge Extraction.
Information obtained from collections via computer ran processes is not the only thing that needs to be represented. Humans are in the loop, and they gradually improve their level of understanding of the content and nature of the multimedia collection. Discovering knowledge and getting insight is involving multiple people across a long period of time, and what each understands, concludes and discovers must be recorded and made available to others. Collaboratively inspecting collections is crucial. Ontologies are an often preferred mechanism for modeling what is inside a collection, but this is probably limitative and narrow.
Linkmedia is concerned with making use of existing strategies in relation with ontologies and knowledge bases. In addition, Linkmedia uses mechanisms allowing to materialize the knowledge gradually acquired by humans and that might be subsequently used either by other humans or by computers in order to better and more precisely analyze collections. This line of work is instantiated at the core of the iCODA project Linkmedia coordinates.
We are therefore concerned with:
- Multimedia analysis and ontologies. We develop approaches for linking multimedia content to entities in ontologies for text and images, building on results in multimodal embedding to cast entity linking into a nearest neighbor search problem in a high-dimensional joint embedding of content and entities 84. We also investigate the use of ontological knowledge to facilitate information extraction from content 62.
- Explainability and accountability in information extraction. In relation with ontologies and entity linking, we develop innovative approaches to explain statistical relations found in data, in particular lexical or entity co-occurrences in textual data, for example using embeddings constrained with translation properties of RDF knowledge or path-based explanation within RDF graphs. We also work on confidence measures in entity linking and information extraction, studying how the notions of confidence and information source can be accounted for in knowledge basis and used in human-centric collaborative exploration of collections.
- Dynamic evolution of models for information extraction. In interactive exploration and information extraction, e.g., on cultural or educational material, knowledge progressively evolves as the process goes on, requiring on-the-fly design of new models for content-based information extractors from very few examples, as well as continuous adaptation of the models. Combining in a seamless way low-shot, active and incremental learning techniques is a key issue that we investigate to enable this dynamic mechanisms on selected applications.
3.4 Research Direction 2: Accessing Information
Linkmedia centers its activities on enabling humans to make good use of vast multimedia collections. This material takes all its cultural and economic value, all its artistic wonder when it can be accessed, watched, searched, browsed, visualized, summarized, classified, shared, ... This allows users to fully enjoy the incalculable richness of the collections. It also makes it possible for companies to create business rooted in this multimedia material.
Accessing the multimedia data that is inside a collection is complicated by the various type of data, their volume, their length, etc. But it is even more complicated to access the information that is not materialized in documents, such as the relationships between parts of different documents that however share some similarity. Linkmedia in its first four years of existence established itself as one of the leading teams in the field of multimedia analytics, contributing to the establishment of a dedicated community (refer to the various special sessions we organized with MMM, the iCODA and the LIMAH projects, as well as 69, 70, 66).
Overall, facilitating the access to the multimedia material, to the relevant information and the corresponding knowledge asks for algorithms that efficiently search collections in order to identify the elements of collections or of the acquired knowledge that are matching a query, or that efficiently allow navigating the collections or the acquired knowledge. Navigation is likely facilitated if techniques are able to handle information and knowledge according to hierarchical perspectives, that is, allow to reveal data according to various levels of details. Aggregating or summarizing multimedia elements is not trivial.
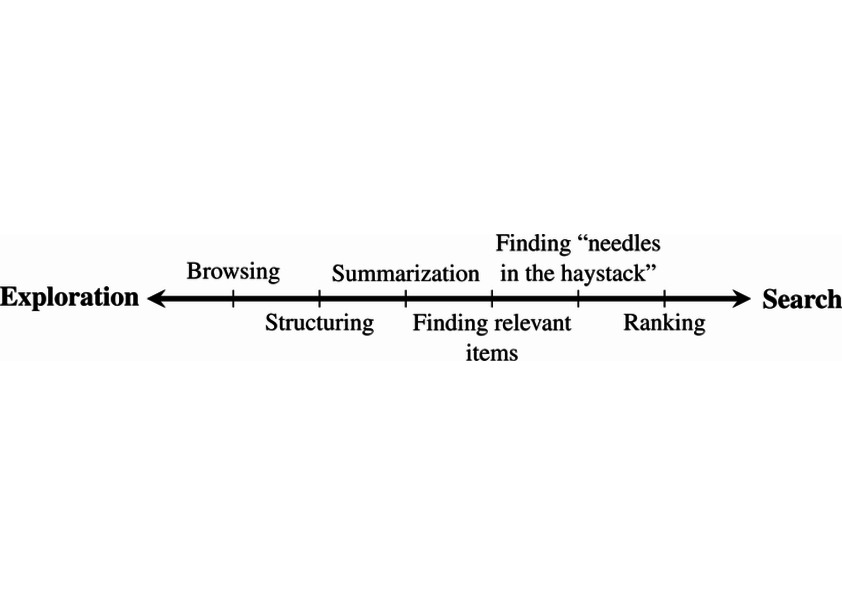
Three topics are therefore in relation with this second research direction. Linkmedia tackles the issues in relation to searching, to navigating and to summarizing multimedia information. Information needs when discovering the content of a multimedia collection can be conveniently mapped to the exploration-search axis, as first proposed by Zahálka and Worring in 89, and illustrated by Figure 1 where expert users typically work near the right end because their tasks involve precise queries probing search engines. In contrast, lay-users start near the exploration end of the axis. Overall, users may alternate searches and explorations by going back and forth along the axis. The underlying model and system must therefore be highly dynamic, support interactions with the users and propose means for easy refinements. Linkmedia contributes to advancing the state of the art in searching operations, in navigating operations (also referred to as browsing), and in summarizing operations.
Searching.
Search engines must run similarity searches very efficiently. High-dimensional indexing techniques therefore play a central role. Yet, recent contributions in ML suggest to revisit indexing in order to adapt to the specific properties of modern features describing contents.
- Advanced scalable indexing. High-dimensional indexing is one of the foundations of Linkmedia. Modern features extracted from the multimedia material with the most recent ML techniques shall be indexed as well. This, however, poses a series of difficulties due to the dimensionality of these features, their possible sparsity, the complex metrics in use, the task in which they are involved (instance search, -nn, class prototype identification, manifold search 68, time series retrieval, ...). Furthermore, truly large datasets require involving sketching 52, secondary storage and/or distribution 51, 50, alleviating the explosion of the number of features to consider due to their local nature or other innovative methods 67, all introducing complexities. Last, indexing multimodal embedded spaces poses a new series of challenges.
- Improving quality. Scalable indexing techniques are approximate, and what they return typically includes a fair amount of false positives. Linkmedia works on improving the quality of the results returned by indexing techniques. Approaches taking into account neighborhoods 61, manifold structures instead of pure distance based similarities 68 must be extended to cope with advanced indexing in order to enhance quality. This includes feature selection based on intrinsic dimensionality estimation 49.
- Dynamic indexing. Feature collections grow, and it is not an option to fully reindex from scratch an updated collection. This trivially applies to the features directly extracted from the media items, but also to the base class prototypes that can evolve due to the non-static nature of learning processes. Linkmedia will continue investigating what is at stake when designing dynamic indexing strategies.
Navigating.
Navigating a multimedia collection is very central to its understanding. It differs from searching as navigation is not driven by any specific query. Rather, it is mostly driven by the relationships that various documents have one another. Relationships are supported by the links between documents and/or parts of documents. Links rely on semantic similarity, depicting the fact that two documents share information on the same topic. But other aspects than semantics are also at stake, e.g., time with the dates of creation of the documents or geography with mentions or appearance in documents of some geographical landmarks or with geo-tagged data.
In multimedia collections, links can be either implicit or explicit, the latter being much easier to use for navigation. An example of an implicit link can be the name of someone existing in several different news articles; we, as humans, create a mental link between them. In some cases, the computer misses such configurations, leaving such links implicit. Implicit links are subject to human interpretation, hence they are sometimes hard to identify for any automatic analysis process. Implicit links not being materialized, they can therefore hardly be used for navigation or faceted search. Explicit links can typically be seen as hyperlinks, established either by content providers or, more aligned with Linkmedia, automatically determined from content analysis. Entity linking (linking content to an entity referenced in a knowledge base) is a good example of the creation of explicit links. Semantic similarity links, as investigated in the LIMAH project and as considered in the search and hyperlinking task at MediaEval and TRECVid, are also prototypical links that can be made explicit for navigation. Pursuing work, we investigate two main issues:
- Improving multimodal content-based linking. We exploit achievements in entity linking to go beyond lexical or lexico-visual similarity and to provide semantic links that are easy to interpret for humans; carrying on, we work on link characterization, in search of mechanisms addressing link explainability (i.e., what is the nature of the link), for instance using attention models so as to focus on the common parts of two documents or using natural language generation; a final topic that we address is that of linking textual content to external data sources in the field of journalism, e.g., leveraging topic models and cue phrases along with a short description of the external sources.
- Dynamicity and user-adaptation. One difficulty for explicit link creation is that links are often suited for one particular usage but not for another, thus requiring creating new links for each intended use; whereas link creation cannot be done online because of its computational cost, the alternative is to generate (almost) all possible links and provide users with selection mechanisms enabling personalization and user-adaptation in the exploration process; we design such strategies and investigate their impact on exploration tasks in search of a good trade-off between performance (few high-quality links) and genericity.
Summarizing.
Multimedia collections contain far too much information to allow any easy comprehension. It is mandatory to have facilities to aggregate and summarize a large body on information into a compact, concise and meaningful representation facilitating getting insight. Current technology suggests that multimedia content aggregation and story-telling are two complementary ways to provide users with such higher-level views. Yet, very few studies already investigated these issues. Recently, video or image captioning 88, 83 have been seen as a way to summarize visual content, opening the door to state-of-the-art multi-document text summarization 64 with text as a pivot modality. Automatic story-telling has been addressed for highly specific types of content, namely TV series 56 and news 76, 82, but still need a leap forward to be mostly automated, e.g., using constraint-based approaches for summarization 53, 82.
Furthermore, not only the original multimedia material has to be summarized, but the knowledge acquired from its analysis is also to summarize. It is important to be able to produce high-level views of the relationships between documents, emphasizing some structural distinguishing qualities. Graphs establishing such relationships need to be constructed at various level of granularity, providing some support for summarizing structural traits.
Summarizing multimedia information poses several scientific challenges that are:
- Choosing the most relevant multimedia aggregation type: Taking a multimedia collection into account, a same piece of information can be present in several modalities. The issue of selecting the most suitable one to express a given concept has thus to be considered together with the way to mix the various modalities into an acceptable production. Standard summarization algorithms have to be revisited so that they can handle continuous representation spaces, allowing them to benefit from the various modalities 57.
- Expressing user’s preferences: Different users may appreciate quite different forms of multimedia summaries, and convenient ways to express their preferences have to be proposed. We for example focus on the opportunities offered by the constraint-based framework.
- Evaluating multimedia summaries: Finding criteria to characterize what a good summary is remains challenging, e.g., how to measure the global relevance of a multimodal summary and how to compare information between and across two modalities. We tackle this issue particularly via a collaboration with A. Smeaton at DCU, comparing the automatic measures we will develop to human judgments obtained by crowd-sourcing;
- Taking into account structuring and dynamicity: Typed links between multimedia fragments, and hierarchical topical structures of documents obtained via work previously developed within the team are two types of knowledge which have seldom been considered as long as summarization is concerned. Knowing that the event present in a document is causally related to another event described in another document can however modify the ways summarization algorithms have to consider information. Moreover the question of producing coarse-to-fine grain summaries exploiting the topical structure of documents is still an open issue. Summarizing dynamic collections is also challenging and it is one of the questions we consider.
4 Application domains
4.1 Asset management in the entertainment business
Media asset management—archiving, describing and retrieving multimedia content—has turned into a key factor and a huge business for content and service providers. Most content providers, with television channels at the forefront, rely on multimedia asset management systems to annotate, describe, archive and search for content. So do archivists such as the Institut National de l'Audiovisuel, the bibliothèque Nationale de France, the Nederlands Instituut voor Beeld en Geluid or the British Broadcast Corporation, as well as media monitoring companies, such as Yacast in France. Protecting copyrighted content is another aspect of media asset management.
4.2 Multimedia Internet
One of the most visible application domains of linked multimedia content is that of multimedia portals on the Internet. Search engines now offer many features for image and video search. Video sharing sites also feature search engines as well as recommendation capabilities. All news sites provide multimedia content with links between related items. News sites also implement content aggregation, enriching proprietary content with user-generated content and reactions from social networks. Most public search engines and Internet service providers offer news aggregation portals. This also concerns TV on-demand and replay services as well as social TV services and multi-screen applications. Enriching multimedia content, with explicit links targeting either multimedia material or knowledge databases is central here.
4.3 Data journalism
Data journalism forms an application domain where most of the technology developed by Linkmedia can be used. On the one hand, data journalists often need to inspect multiple heterogeneous information sources, some being well structured, some other being fully unstructured. They need to access (possibly their own) archives with either searching or navigational means. To gradually construct insight, they need collaborative multimedia analytics processes as well as elements of trust in the information they use as foundations for their investigations. Trust in the information, watching for adversarial and/or (deep) fake material, accountability are all crucial here.
5 Social and environmental responsibility
5.1 Impact of research results
Mobile search
As part of our involvement in innovation project MobilAI, we have developed a novel knowledge transfer mechanism for metric learning 45, which can train a lightweight student network for image retrieval in a teacher-student setting, allowing it to outperform a large teacher network.
Our work is truly motivated by working together with a number of startup companies on mobile visual recognition. The companies have well-established technologies involving visual search, including for instance copyright protection by watermarking, worldwide identity document recognition and augmented reality in exhibitions.
However, solutions are mostly off-line or web-based; when mobile, they are mostly based on shallow representations, which still perform better than very small deep networks. Mobile and embedded computer vision applications are expected to have significant impact especially in developing countries, where access to computing is limited otherwise.
Despite the progress in efficient architectures, making small networks perform as well as large ones in different tasks is an enabling factor for mobile computing that is under-explored. While striving for scientific novelty, the interest of startup companies in our work for the development of innovative solutions is a direct indicator of socioeconomic impact to us.
6 Highlights of the year
- Teddy Furon: Chaire IA - SAIDA Security of Artificial Intelligence for Defense Applications.
- Best Student Paper for B. Bonnet, P. Bas, and T. Furon at IH&MMSEC Conference 19.
- Distinctive mention for B. Bonnet and T. Furon at MediaEval 2020 for their work on the Pixel Privacy challenge 18.
- Distinctive mention for V. Claveau at MediaEval 2020 for his work on the Fake News detection challenge 20.
7 New software and platforms
7.1 New software
7.1.1 TagEx
- Name: Yet another Part-of-Speech Tagger for French
- Keyword: Natural language processing
- Functional Description: TagEx is available as a web-service on https://allgo.inria.fr . Refer to Allgo for its usage.
-
URL:
https://
allgo. inria. fr/ app/ tagex - Contact: Vincent Claveau
7.1.2 NegDetect
- Name: Negation Detection
- Keyword: Natural language processing
- Functional Description: NegDetect relies on several layers of machine learning techniques (CRF, neural networks).
- Contacts: Vincent Claveau, Clément Dalloux
7.1.3 SurFree
- Name: A fast surrogate-free black-box attack against classifier
- Keywords: Computer vision, Classification, Cyber attack
-
Scientific Description:
Machine learning classifiers are critically prone to evasion attacks. Adversarial examples are slightly modified inputs that are then misclassified, while remaining perceptively close to their originals. Last couple of years have witnessed a striking decrease in the amount of queries a black box attack submits to the target classifier, in order to forge adversarials. This particularly concerns the blackbox score-based setup, where the attacker has access to top predicted probabilites: the amount of queries went from to millions of to less than a thousand.
This paper presents SurFree, a geometrical approach that achieves a similar drastic reduction in the amount of queries in the hardest setup: black box decision-based attacks (only the top-1 label is available). We first highlight that the most recent attacks in that setup, HSJA, QEBA and GeoDA all perform costly gradient surrogate estimations. SurFree proposes to bypass these, by instead focusing on careful trials along diverse directions, guided by precise indications of geometrical properties of the classifier decision boundaries. We motivate this geometric approach before performing a head-to-head comparison with previous attacks with the amount of queries as a first class citizen. We exhibit a faster distortion decay under low query amounts (few hundreds to a thousand), while remaining competitive at higher query budgets.
Paper : https://arxiv.org/abs/2011.12807
- Functional Description: This software is the implementation in python of the attack SurFree. This is an attack against a black-box classifier. It finds an input close to the reference input (Euclidean distance) yet not classified with the same predicted label as the reference input. This attack has been tested against image classifier in computer vision.
-
URL:
https://
github. com/ t-maho/ SurFree - Authors: Thibault Maho, Erwan Le Merrer, Teddy Furon
- Contacts: Teddy Furon, Thibault Maho, Erwan Le Merrer
7.1.4 GrowAndPrune
- Name: Neural architecture growing, pruning and search
- Keywords: Deep learning, Neural architecture search
- Functional Description: This is the official code that enables the reproduction of the results of our work https://avrithis.net/data/cv/pdf/msc/2020.neitthoffer.pdf
-
URL:
https://
github. com/ shymine/ neural-architecture-growing-pruning-and-search - Contacts: Timothee Neitthoffer, Ioannis Avrithis
7.1.5 AML
- Name: Asymmetric Metric Learning
- Keywords: Knowledge transfer, Metric learning, Image retrieval
- Functional Description: This is the official code and a set of pre-trained models that enable the reproduction of the results of our paper https://hal.inria.fr/hal-03047591.
-
URL:
https://
github. com/ budnikm/ asymmetric_metric_learning - Contacts: Mateusz Budnik, Ioannis Avrithis
7.1.6 NFSL
- Name: Noisy Few-Shot Learning
- Keywords: Few-shot learning, Deep learning
- Functional Description: This is the official code that enables the reproduction of the results of our paper https://hal.inria.fr/hal-03047513.
-
URL:
https://
github. com/ google-research/ noisy-fewshot-learning - Contacts: Ahmet Iscen, Ioannis Avrithis
7.1.7 DSM
- Name: Deep Spatial Matching
- Keywords: Spatial matching, Content-based Image Retrieval, Deep learning
- Functional Description: This is the official code that enables the reproduction of the results of our paper https://hal.inria.fr/hal-02374156.
-
URL:
https://
github. com/ osimeoni/ DSM - Contacts: Oriane Simeoni, Ioannis Avrithis
7.1.8 DAL
- Name: Rethinking Deep Active Learning
- Keywords: Active Learning, Deep learning
- Functional Description: This is the official code that enables the reproduction of the results of our paper https://hal.inria.fr/hal-02372102.
-
URL:
https://
github. com/ osimeoni/ RethinkingDeepActiveLearning - Contacts: Oriane Simeoni, Mateusz Budnik, Ioannis Avrithis
8 New results
8.1 Extracting and Representing Information
8.1.1 Building Medical concept embeddings without texts
Participants: Vincent Claveau.
In the medical field, many TAL tools are now based on embeddings of concepts from the UMLS.Existing approaches to generate these embeddings require large amounts of medical data. Contrary to these approaches, we propose in this article (21) to rely on Japanese translations of the concepts,more precisely in Kanjis, available in the UMLS to generate these embeddings. Tested on different evaluation tasks proposed in the literature, our approach, which therefore requires no text, yields goodresults compared to the state of the art. Moreover, we show that it is interesting to combine them with existing – contextual-based – embeddings.8.1.2 CAS: corpus of clinical cases in French
Participants: Clément Dalloux, Vincent Claveau, Natalia Grabar.
Background: Textual corpora are extremely important for various NLP applications as they provide information necessary for creating, setting and testing those applications and the corresponding tools. They are also crucial for designing reliable methods and reproducible results. Yet, in some areas, such as the medical area, due to confidentiality or to ethical reasons, it is complicated or even impossible to access representative textual data. We propose the CAS corpus built with clinical cases, such as they are reported in the published scientific literature in French. Results: Currently, the corpus contains 4,900 clinical cases in French, totaling nearly 1.7M word occurrences. Some clinical cases are associated with discussions. A subset of the whole set of cases is enriched with morpho-syntactic (PoS-tagging, lemmatization) and semantic (the UMLS concepts, negation, uncertainty) annotations. The corpus is being continuously enriched with new clinical cases and annotations. The CAS corpus has been compared with similar clinical narratives. When computed on tokenized and lowercase words, the Jaccard index indicates that the similarity between clinical cases and narratives reaches up to 0.9727. Conclusion: We assume that the CAS corpus can be effectively exploited for the development and testing of NLP tools and methods. Besides, the corpus will be used in NLP challenges and distributed to the research community 14.8.1.3 On the Correlation of Word Embedding Evaluation Metrics
Participants: François Torregrossa, Vincent Claveau, Nihel Kooli, Guillaume Gravier, Robin Allesiardo.
Word embeddings intervene in a wide range of natural language processing tasks. These geometrical representations are easy to manipulate for automatic systems. Therefore, they quickly invaded all areas of language processing. While they surpass all predecessors, it is still not straightforward why and how they do so. In this work, we propose to investigate all kind of evaluation metrics on various datasets in order to discover how they correlate with each other 35. Those correlations lead to 1) a fast solution to select the best word embeddings among many others, 2) a new criterion that may improve the current state of static Euclidean word embeddings, and 3) a way to create a set of complementary datasets, i.e. each dataset quantifies a different aspect of word embeddings.8.1.4 HierarX: a tool for discovering hierarchies in hyperbolic spaces
Participants: François Torregrossa, Guillaume Gravier, Vincent Claveau, Nihel Kooli.
This work 36 introduces the HierarX tool which projects multiple datasources into hyperbolicmanifolds : Lorentz or Poincaré. From similarities between word pairs or continuous wordrepresentations in high dimensional spaces, HierarX is able to embed knowledge in hyperbolicgeometries with small dimensionality. Those shape information into continuous hierarchies.This work presents the HierarX workflow as well as its main use-cases.
8.1.5 Few-Shot Few-Shot Learning and the role of Spatial Attention
Participants: Yann Lifchitz, Yannis Avrithis, Sylvaine Picard.
Few-shot learning is often motivated by the ability of humans to learn new tasks from few examples. However, standard few-shot classification benchmarks assume that the representation is learned on a limited amount of base class data, ignoring the amount of prior knowledge that a human may have accumulated before learning new tasks. At the same time, even if a powerful representation is available, it may happen in some domain that base class data are limited or non-existent. This motivates us to study a problem where the representation is obtained from a classifier pre-trained on a large-scale dataset of a different domain, assuming no access to its training process, while the base class data are limited to few examples per class and their role is to adapt the representation to the domain at hand rather than learn from scratch. We adapt the representation in two stages, namely on the few base class data if available and on the even fewer data of new tasks. In doing so, we obtain from the pre-trained classifier a spatial attention map that allows focusing on objects and suppressing background clutter. This is important in the new problem, because when base class data are few, the network cannot learn where to focus implicitly. We also show that a pre-trained network may be easily adapted to novel classes, without meta-learning 29.
8.1.6 Local Propagation for Few-Shot Learning
Participants: Yann Lifchitz, Yannis Avrithis, Sylvaine Picard.
The challenge in few-shot learning is that available data is not enough to capture the underlying distribution. To mitigate this, two emerging directions are (a) using local image representations, essentially multiplying the amount of data by a constant factor, and (b) using more unlabeled data, for instance by transductive inference, jointly on a number of queries. In this work, we bring these two ideas together, introducing local propagation. We treat local image features as independent examples, we build a graph on them and we use it to propagate both the features themselves and the labels, known and unknown. Interestingly, since there is a number of features per image, even a single query gives rise to transductive inference. As a result, we provide a universally safe choice for few-shot inference under both non-transductive and transductive settings, improving accuracy over corresponding methods. This is in contrast to existing solutions, where one needs to choose the method depending on the quantity of available data 30.8.1.7 Iterative label cleaning for transductive and semi-supervised few-shot learning
Participants: Michalis Lazarou, Yannis Avrithis, Tania Stathaki.
Few-shot learning amounts to learning representations and acquiring knowledge such that novel tasks may be solved with both supervision and data being limited. Improved performance is possible by transductive inference, where the entire test set is available concurrently, and semi-supervised learning, where more unlabeled data is available. These problems are closely related because there is little or no adaptation of the representation in novel tasks.Focusing on these two settings, we introduce a new algorithm that leverages the manifold structure of the labeled and unlabeled data distribution to predict pseudo-labels, while balancing over classes and using the loss value distribution of a limited-capacity classifier to select the cleanest labels, iterately improving the quality of pseudo-labels 47. Our solution sets new state of the art on four benchmark datasets, namely miniImageNet, tieredImageNet, CUB and CIFAR-FS, while being robust over feature space pre-processing and the quantity of available data.
8.1.8 Graph Convolutional Networks for Learning with Few Clean and Many Noisy Labels
Participants: Ahmet Iscen, Giorgos Tolias, Yannis Avrithis, Ondra Chum, Cordelia Schmid.
In this work we consider the problem of learning a classifier from noisy labels when a few clean labeled examples are given 27. The structure of clean and noisy data is modeled by a graph per class and Graph Convolutional Networks (GCN) are used to predict class relevance of noisy examples. For each class, the GCN is treated as a binary classifier, which learns to discriminate clean from noisy examples using a weighted binary cross-entropy loss function. The GCN-inferred "clean" probability is then exploited as a relevance measure. Each noisy example is weighted by its relevance when learning a classifier for the end task. We evaluate our method on an extended version of a few-shot learning problem, where the few clean examples of novel classes are supplemented with additional noisy data. Experimental results show that our GCNbased cleaning process significantly improves the classification accuracy over not cleaning the noisy data, as well as standard few-shot classification where only few clean examples are used.8.1.9 Joint Learning of Assignment and Representation for Biometric Group Membership
Participants: Marzieh Gheisari Khorasgani, Teddy Furon, Laurent Amsaleg.
This work proposes a framework for group membership protocols preventing the curious but honest server from reconstructing the enrolled biometric signatures and inferring the identity of querying clients. This framework learns the embedding parameters, group representations and assignments simultaneously. Experiments show the trade-off between security/privacy and verification/identification performances 26.8.1.10 Interactive Learning for Multimedia at Large
Participants: Omar Shahbaz Khan, Björn Þór Jónsson, Stevan Rudinac, Jan Zahálka, Hanna Ragnarsdóttir, Þórhildur Þorleiksdóttir, Gylfi Þór Guðmundsson, Laurent Amsaleg, Marcel Worring.
Interactive learning has been suggested as a key method for addressing analytic multimedia tasks arising in several domains. Until recently, however, methods to maintain interactive performance at the scale of today's media collections have not been addressed. We propose an interactive learning approach that builds on and extends the state of the art in user relevance feedback systems and high-dimensional indexing for multimedia. We report on a detailed experimental study using the ImageNet and YFCC100M collections, containing 14 million and 100 million images respectively. The proposed approach outperforms the relevant state-of-the-art approaches in terms of interactive performance, while improving suggestion relevance in some cases. In particular, even on YFCC100M, our approach requires less than 0.3 s per interaction round to generate suggestions, using a single computing core and less than 7 GB of main memory 398.1.11 Asymmetric Metric Learning for Knowledge Transfer
Participants: Mateusz Budnik, Yannis Avrithis.
Knowledge transfer from large teacher models to smaller student models has recently been studied for metric learning, focusing on fine-grained classification. In this work, focusing on instance-level image retrieval, we study an asymmetric testing task, where the database is represented by the teacher and queries by the student. Inspired by this task, we introduce asymmetric metric learning, a novel paradigm of using asymmetric representations at training. This acts as a simple combination of knowledge transfer with the original metric learning task. We systematically evaluate different teacher and student models, metric learning and knowledge transfer loss functions on the new asymmetric testing as well as the standard symmetric testing task, where database and queries are represented by the same model. We find that plain regression is surprisingly effective compared to more complex knowledge transfer mechanisms, working best in asymmetric testing. Interestingly, our asymmetric metric learning approach works best in symmetric testing, allowing the student to even outperform the teacher 45.8.1.12 Exploring Quality Camouflage for Social Images
Participants: Zhuoran Liu, Zhengyu Zhao, Martha Larson, Laurent Amsaleg.
Social images can be misused in ways not anticipated or intended by the people who share them online. In particular, high-quality images can be driven to unwanted prominence by search engines or used to train unscrupulous AI. The risk of misuse can be reduced if photos can evade quality filtering, which is commonly carried out by automatic Blind Image Quality Assessment (BIQA) algorithms. The Pixel Privacy Task benchmarks privacy-protective approaches that shield images against unethical computer vision algorithms. In the 2020 task, participants are asked to develop quality camouflage methods that can effectively decrease the BIQA score of high-quality images while maintaining image appeal. The camouflage should not damage the image from the point of view of the user: it needs to be either imperceptible, or else to enhance the image visibly, to the human eye. We report on this initiative in the following publication: 32.8.1.13 Fooling an Automatic Image Quality Estimator
Participants: Benoît Bonnet, Teddy Furon, Patrick Bas.
We present our work on the 2020 MediaEval task: "Pixel Privacy: Quality Camouflage for Social Images". Blind Image Quality Assessment (BIQA) is an algorithm predicting a quality score for any given image. Our task is to modify an image to decrease its BIQA score while maintaining a good perceived quality. Since BIQA is a deep neural network, we worked on an adversarial attack approach of the problem 18.8.1.14 High Intrinsic Dimensionality Facilitates Adversarial Attack: Theoretical Evidence
Participants: Laurent Amsaleg, James Bailey, Amélie Barbe, Sarah Erfani, Teddy Furon, Michael Houle, Miloš Radovanović, Vinh Nguyen Xuan.
Machine learning systems are vulnerable to adversarial attack. By applying to the input object a small, carefully-designed perturbation, a classifier can be tricked into making an incorrect prediction. This phenomenon has drawn wide interest, with many attempts made to explain it. However, a complete understanding is yet to emerge. In this work we adopt a slightly different perspective, still relevant to classification 8. We consider retrieval, where the output is a set of objects most similar to a user-supplied query object, corresponding to the set of k-nearest neighbors. We investigate the effect of adversarial perturbation on the ranking of objects with respect to a query. Through theoretical analysis, supported by experiments, we demonstrate that as the intrinsic dimensionality of the data domain rises, the amount of perturbation required to subvert neighborhood rankings diminishes, and the vulnerability to adversarial attack rises. We examine two modes of perturbation of the query: either 'closer' to the target point, or 'farther' from it. We also consider two perspectives: 'query-centric', examining the effect of perturbation on the query's own neighborhood ranking, and 'target-centric', considering the ranking of the query point in the target's neighborhood set. All four cases correspond to practical scenarios involving classification and retrieval.
8.1.15 An alternative proof of the vulnerability of k-NN classifiers in high intrinsic dimensionality regions
Participants: Teddy Furon.
This document proposes an alternative proof of the result contained in article "High intrinsic dimensionality facilitates adversarial attack: Theoretical evidence" 8. The proof is simpler to understand and leads to a more precise statement about the asymptotical distribution of the relative amount of perturbation 46.8.1.16 Defending Adversarial Examples via DNN Bottleneck Reinforcement
Participants: Wenqing Liu, Miaojing Shi, Teddy Furon, Li Li.
This work presents a DNN bottleneck reinforcement scheme to alleviate the vulnerability of Deep Neural Networks (DNN) against adversarial attacks 31. Typical DNN classifiers encode the input image into a compressed latent representation more suitable for inference. This information bottleneck makes a trade-off between the image-specific structure and class-specific information in an image. By reinforcing the former while maintaining the latter, any redundant information, be it adversarial or not, should be removed from the latent representation. Hence, this paper proposes to jointly train an auto-encoder (AE) sharing the same encoding weights with the visual classifier. In order to reinforce the information bottleneck, we introduce the multi-scale low-pass objective and multi-scale high-frequency communication for better frequency steering in the network. Unlike existing approaches, our scheme is the first reforming defense per se which keeps the classifier structure untouched without appending any pre-processing head and is trained with clean images only. Extensive experiments on MNIST, CIFAR-10 and ImageNet demonstrate the strong defense of our method against various adversarial attacks.8.1.17 What if Adversarial Samples were Digital Images?
Participants: Benoît Bonnet, Teddy Furon, Patrick Bas.
Although adversarial sampling is a trendy topic in computer vision, very few works consider the integral constraint: The result of the attack is a digital image whose pixel values are integers. This is not an issue at first sight since applying a rounding after forging an adversarial sample trivially does the job. Yet, this work shows theoretically and experimentally that this operation has a big impact. The adversarial perturbations are fragile signals whose quantization destroys its ability to delude an image classifier. This paper presents a new quantization mechanism which preserves the adversariality of the perturbation. Its application outcomes to a new look at the lessons learnt in adversarial sampling 19.8.1.18 Smooth Adversarial Examples
Participants: Hanwei Zhang, Yannis Avrithis, Teddy Furon, Laurent Amsaleg.
This paper investigates the visual quality of the adversarial examples. Recent papers propose to smooth the perturbations to get rid of high frequency artefacts. In this work, smoothing has a different meaning as it perceptually shapes the perturbation according to the visual content of the image to be attacked 16. The perturbation becomes locally smooth on the flat areas of the input image, but it may be noisy on its textured areas and sharp across its edges. This operation relies on Laplacian smoothing, well-known in graph signal processing, which we integrate in the attack pipeline. We benchmark several attacks with and without smoothing under a white-box scenario and evaluate their transferability. Despite the additional constraint of smoothness, our attack has the same probability of success at lower distortion.8.1.19 Walking on the Edge: Fast, Low-Distortion Adversarial Examples
Participants: Hanwei Zhang, Yannis Avrithis, Teddy Furon, Laurent Amsaleg.
Adversarial examples of deep neural networks are receiving ever increasing attention because they help in understanding and reducing the sensitivity to their input. This is natural given the increasing applications of deep neural networks in our everyday lives. When white-box attacks are almost always successful, it is typically only the distortion of the perturbations that matters in their evaluation. In this work 17, we argue that speed is important as well, especially when considering that fast attacks are required by adversarial training. Given more time, iterative methods can always find better solutions. We investigate this speed-distortion trade-off in some depth and introduce a new attack called boundary projection (BP) that improves upon existing methods by a large margin. Our key idea is that the classification boundary is a manifold in the image space: we therefore quickly reach the boundary and then optimize distortion on this manifold.8.1.20 Adversarial Regularization for Explainable-by-Design Time Series Classification
Participants: Yichang Wang, Rémi Emonet, Elisa Fromont, Simon Malinowski, Romain Tavenard.
Times series classification can be successfully tackled by jointly learning a shapelet-based representation of the series in the dataset and classifying the series according to this representation. This shapelet-based classification is both accurate and explainable since the shapelets are time series themselves and thus can be visualized and be provided as a classification explanation. In this work, we claim that not all shapelets are good visual explanations and we propose a simple, yet also accurate, adversarily regularized EXplainable Convolutional Neural Network, XCNN, that can learn shapelets that are, by design, suited for explanations. We validate our method on the usual univariate time series benchmarks of the UCR repository 38.8.1.21 Detecting Human-Object Interaction with Mixed Supervision
Participants: Suresh Kumaraswamy, Miaojing Shi, Ewa Kijak.
Human object interaction (HOI) detection is an important task in image understanding and reasoning. It is in a form of HOI triplet human, verb, object, requiring bounding boxes for human and object, and action between them for the task completion. In other words, this task requires strong supervision for training that is however hard to procure. A natural solution to overcome this is to pursue weakly-supervised learning, where we only know the presence of certain HOI triplets in images but their exact location is unknown. Most weakly-supervised learning methods do not make provision for leveraging data with strong supervision, when they are available; and indeed a naive combination of this two paradigms in HOI detection fails to make contributions to each other. In this regard we propose a mixed-supervised HOI detection pipeline: thanks to a specific design of momentum-independent learning that learns seamlessly across these two types of supervision 28. Moreover, in light of the annotation insufficiency in mixed supervision, we introduce an HOI element swapping technique to synthesize diverse and hard negatives across images and improve the robustness of the model. Our method is evaluated on the challenging HICO-DET dataset. It performs close to or even better than many fully-supervised methods by using a mixed amount of strong and weak annotations; furthermore, it outperforms representative state of the art weakly and fully-supervised methods under the same supervision.8.1.22 A correlation-based entity embedding approach for robust entity linking
Participants: Cheikh Brahim El Vaigh, François Torregrossa, Robin Allesiardo, Guillaume Gravier, Pascale Sébillot.
Done as part of the IPL iCODA.
Entity alignment is a crucial tool in knowledge discovery to reconcile knowledge from different sources. Recent state-of-the-art approaches leverage joint embedding of knowledge graphs (KGs) so that similar entities from different KGs are close in the embedded space. Whatever the joint embedding technique used, a seed set of aligned entities, often provided by (time-consuming) human expertise, is required to learn the joint KG embedding and/or a mapping between KG embeddings. In this context, a key issue is to limit the size and quality requirement for the seed. State-of-the-art methods usually learn the embedding by explicitly minimizing the distance between aligned entities from the seed and uniformly maximizing the distance for entities not in the seed. In contrast, we design a less restrictive optimization criterion that indirectly minimizes the distance between aligned entities in the seed by globally maximizing the dimension-wise correlation among all the embeddings of seed entities. Within an iterative entity alignment system, the correlation-based entity embedding function achieves state-of-the-art results and is shown to significantly increase robustness to the seed's size and accuracy. It ultimately enables fully unsupervised entity alignment using a seed automatically generated with a symbolic alignment method based on entities' names 25.8.1.23 IRISA System for Entity Detection and Linking at CLEF HIPE 2020
Participants: Cheikh Brahim El Vaigh, Guillaume Le Noé-Bienvenu, Guillaume Gravier, Pascale Sébillot.
This note describes IRISA's system for the task of named entity processing on historical newspapers in French 24. Following a standard entity detection and linking pipeline, our system implements three steps to solve the named entity linking task. Named Entity Recognition (NER) is first performed to identify the entity mentions in a document based on a Conditional Random Fields classifier. Candidate entities from Wikidata are then generated for each mention found, using simple search. Finally, every mention is linked to one of its candidate entities in a so-called linking step leveraging various string metrics and the semantic structure of Wikidata to improve on the linking decisions.8.1.24 Relation, es-tu là ? Détection de relations par LSTM pour améliorer l’extraction de relations
Participants: Cyrielle Mallart, Michel Le Nouy, Guillaume Gravier, Pascale Sébillot.
De nombreuses méthodes d’extraction et de classification de relations ont été proposées et testées sur des données de référence. Cependant, dans des données réelles, le nombre de relations potentielles est énorme et les heuristiques souvent utilisées pour distinguer de vraies relations de co-occurrences fortuites ne détectent pas les signaux faibles pourtant importants. Dans cet article, nous étudions l’apport d’un modèle de détection de relations, identifiant si un couple d’entités dans une phrase exprime ou non une relation, en tant qu’étape préliminaire à la classification des relations. Notre modèle s’appuie sur le plus court chemin de dépendances entre deux entités, modélisé par un LSTM et combiné avec les types des entités. Sur la tâche de détection de relations, nous obtenons de meilleurs résultats qu’un modèle état de l’art pour la classification de relations, avec une robustesse accrue aux relations inédites. Nous montrons aussi qu’une détection binaire en amont d’un modèle de classification améliore significativement ce dernier 338.1.25 Understanding the phenomenology of reading through modelling
Participants: Alessio Antonini, Mari Carmen Suárez-Figueroa, Alessandro Adamou, Francesca Benatti, François Vignale, Guillaume Gravier, Lucia Lupi.
Large scale cultural heritage datasets and computational methods for the humanities research framework are the two pillars of digital humanities, a research field aiming to expand humanities studies beyond specific sources and periods to address macroscope research questions on broad human phenomena. In this regard, the development of machine-readable semantically enriched data models based on a cross-disciplinary "language" of phenomena is critical for achieving the interoperabil-ity of research data. This contribution reports, documents, and discusses the development of a model for the study of reading experiences as part of the EU JPI-CH project Reading Europe Advanced Data Investigation Tool (READ-IT). Through the discussion of the READ-IT ontology of reading experience, this contribution will highlight and address three challenges emerging from the development of a conceptual model for the support of research on cultural heritage. Firstly, this contribution addresses modelling for multidisciplinary research. Secondly, this work addresses the development of an ontology of reading experience, under the light of the experience of previous projects, and of ongoing and future research developments 37. Lastly, this contribution addresses the validation of a conceptual model in the context of ongoing research, the lack of a consolidated set of theories and of a consensus of domain experts 9.8.1.26 Rethinking deep active learning: Using unlabeled data at model training
Participants: Oriane Siméoni, Mateusz Budnik, Yannis Avrithis, Guillaume Gravier.
Active learning typically focuses on training a model on few labeled examples alone, while unlabeled ones are only used for acquisition. In this work we depart from this setting by using both labeled and unlabeled data during model training across active learning cycles 34. We do so by using unsupervised feature learning at the beginning of the active learning pipeline and semi-supervised learning at every active learning cycle, on all available data. The former has not been investigated before in active learning, while the study of latter in the context of deep learning is scarce and recent findings are not conclusive with respect to its benefit. Our idea is orthogonal to acquisition strategies by using more data, much like ensemble methods use more models. By systematically evaluating on a number of popular acquisition strategies and datasets, we find that the use of unlabeled data during model training brings a spectacular accuracy improvement in image classification, compared to the differences between acquisition strategies. We thus explore smaller label budgets, even one label per class.8.1.27 Improving topic modeling through homophily for legal documents
Participants: Kazuki Ashihara, Cheikh Brahim El Vaigh, Chenhui Chu, Benjamin Renoust, Noriko Okubo, Noriko Takemura, Yuta Nakashima, Hajime Nagahara.
Topic modeling that can automatically assign topics to legal documents is very important in the domain of computational law. The relevance of the modeled topics strongly depends on the legal context they are used in. On the other hand, references to laws and prior cases are key elements for judges to rule on a case. Taken together, these references form a network, whose structure can be analysed with network analysis. However, the content of the referenced documents may not be always accessed. Even in that case, the reference structure itself shows that documents share latent similar characteristics. We propose to use this latent structure to improve topic modeling of law cases using document homophily. In this paper, we explore the use of homophily networks extracted from two types of references: prior cases and statute laws, to enhance topic modeling on legal case documents. We conduct in detail, an analysis on a dataset consisting of rich legal cases, i.e., the COLIEE dataset, to create these networks. The homophily networks consist of nodes for legal cases, and edges with weights for the two families of references between the case nodes. We further propose models to use the edge weights for topic modeling. In particular, we propose a cutting model and a weighting model to improve the relational topic model (RTM). The cutting model uses edges with weights higher than a threshold as document links in RTM; the weighting model uses the edge weights to weight the link probability function in RTM. The weights can be obtained either from the co-citations or from the cosine similarity based on an embedding of the homophily networks. Experiments show that the use of the homophily networks for topic modeling significantly outperforms previous studies, and the weighting model is more effective than the cutting model 10.8.2 Accessing Information
8.2.1 Detection of Fake News in Social Networks: the MediaEval2020 challenge
Participants: Vincent Claveau.
In 20 we present the participation of IRISA to the task of fake news detection from tweets, relying either on the text or on propagation information. For the text based detection, variants of BERT-based classification are proposed. In order to improve this standard approach, we investigate the interest of augmenting the dataset by creating tweets with fine-tuned generative models. For the graph based detection, we have proposed models characterizing the propagation of the news or the users' reputation. With these approaches, we obtained very good results and respectively ranked 2nd and 1st among the participants.8.2.2 Supervised learning for the detection of negation and of its scope in French and Brazilian Portuguese biomedical corpora
Participants: Clément Dalloux, Vincent Claveau, Natalia Grabar, Lucas Emanuel Silva Oliveira, Claudia Maria Cabral Moro, Yohan Bonescki Gumiel, Deborah Ribeiro Carvalho.
Automatic detection of negated content is often a prerequisite in information extraction systems in various domains. In the biomedical domain especially, this task is important because negation plays an important role. In this work, two main contributions are proposed. First, we work with languages which have been poorly addressed up to now: Brazilian Portuguese and French. Thus, we developed new corpora for these two languages which have been manually annotated for marking up the negation cues and their scope. Second, we propose automatic methods based on supervised machine learning approaches for the automatic detection of negation marks and of their scopes. The methods show to be robust in both languages (Brazilian Portuguese and French) and in cross-domain (general and biomedical languages) contexts. The approach is also validated on English data from the state of the art: it yields very good results and outperforms other existing approaches. Besides, the application is accessible and usable online. We assume that, through these issues (new annotated corpora, application accessible online, and cross-domain robustness), the reproducibility of the results and the robustness of the NLP applications will be augmented 13, 43.8.2.3 Supervised Learning for the ICD-10 Coding of French Clinical Narratives
Participants: Clément Dalloux, Vincent Claveau, Marc Cuggia, Guillaume Bouzillé, Natalia Grabar.
Automatic detection of ICD-10 codes in clinical documents has become a necessity. In this article, after a brief reminder of the existing work, we present a corpus of French clinical narratives annotated with the ICD-10 codes. Then, we propose automatic methods based on neural network approaches for the automatic detection of the ICD-10 codes. The results show that we need 1) more examples per class given the number of classes to assign, and 2) a better word/concept vector representation of documents in order to accurately assign codes 22, 43.8.2.4 Hierarchical Multi-Label Propagation using Speaking Face Graphs for Multimodal Person Discovery
Participants: Gabriel Barbosa da Fonseca, Gabriel Sargent, Ronan Sicre, Zenilton Kleber Gonçalves Do Patrocinio, Guillaume Gravier, Silvio Jamil Guimarães.
TV archives are growing in size so fast that manually indexing becomes unfeasible. Automatic indexing techniques can be applied to overcome this issue, and this work proposes an unsupervised technique for multimodal person discovery. To achieve this goal, we propose a hierarchical label propagation technique based on quasi-flat zones theory, that learns from labeled and unlabeled data and propagates names through a multimodal graph representation. In this representation, we combine audio, video, and text processing techniques to model the data as a graph of speaking faces. In the proposed mod-eling, we extract names via optical character recognition and propagate them through the graph using audiovisual relationships between speaking faces. We also use a random walk label propagation and two graph clustering strategies to serve as baselines. The proposed label propagation techniques always outper-form the clustering baselines on the quantitative assessments. Our approach also outperforms all literature methods tested on the same dataset except for one, which uses a different preprocessing step. The proposed hierarchical label propagation and the random walk baseline produce highly equivalent results according to the Kappa coefficient, but the hierarchical propagation is parameter-free and over 9 times faster than the random walk under the same configurations 11.8.2.5 A Novel Path-based Entity Relatedness Measure for Efficient Collective Entity Linking
Participants: Cheikh Brahim El Vaigh, François Goasdoué, Guillaume Gravier, Pascale Sébillot.
Collective entity linking is a core natural language processing task, which consists in jointly identifying the entities of a knowledge base (KB) that are mentioned in a text exploiting existing relations between entities within the KB. State-of-the-art methods typically combine local scores accounting for the similarity between mentions and entities, with a global score measuring the coherence of the set of selected entities. The latter relies on the structure of a KB: the hyperlink graph of Wikipedia in most cases or the graph of an RDF KB, e.g., BaseKB or Yago, to benefit from the precise semantics of relationships between entities. In this paper, we devise a novel RDF-based entity relatedness measure for global scores with important properties: (i) it has a clear semantics, (ii) it can be calculated at reasonable computational cost, and (iii) it accounts for the transitive aspects of entity relatedness through existing (bounded length) property paths between entities in an RDF KB. Further, we experimentally show on the TAC-KBP2017 dataset, both with BaseKB and Yago, that it provides significant improvement over state-of-the-art entity relatedness measures for the collective entity linking task 23.9 Bilateral contracts and grants with industry
9.1 Bilateral contracts with industry
CIFRE PhD: Incremental dynamic construction of knowledge bases from text mining
Participants: Guillaume Gravier, Cyrielle Mallart, Pascale Sébillot.
Duration: 3 years, started in Dec. 2018
In the context of a newspaper, the thesis explores the combination of text mining and knowledge representation techniques to assist the extraction, interpretation and validation of valuable pieces of information from the journal’s content so as to incrementally build a full-scale knowledge base. This thesis is in close relation with the iCODA Inria Project Lab, with direct contribution to the project’s results.
CIFRE PhD: Embedding heterogeneous data for directory search
Participants: Vincent Claveau, Guillaume Gravier, François Torregrossa.
Duration: 3 years, started in Dec. 2018
The thesis aims at learning how to jointly exploit heterogeneous sources of information (e.g., names, activity sector, user profiles, queries, etc.) in the design of neural network embeddings for information retrieval and language understanding. Applications cover natural language query analysis and personalized information retrieval in Pagesjaunes’ directory.
CIFRE PhD: Few shot learning for object recognition in aerial images
Participants: Yannis Avrithis, Yann Lifchitz.
Duration: 3 years, started in March 2018
This is a CIFRE PhD thesis project aiming to study architectures and learning techniques most suitable for object recognition from few samples and to validate these approaches on multiple recognition tasks and use-cases related to aerial images.
CIFRE PhD: Deep Learning and Homomorphic encryption
Participants: Teddy Furon, Samuel Tap.
Duration: 3 years, started in December 2020
This is a CIFRE PhD thesis project aiming to study inference and training of neural networks in the encrypted domain. This means that inputs (test or training data) are encrypted to protect confidentiality.
CIFRE PhD: Robustness of machine learning against uncertainties
Participants: Teddy Furon, Karim Tit.
Duration: 3 years, started in December 2020
This is a CIFRE PhD thesis project aiming to study the robustness of machine learning algorithm facing uncertainties in the acquisition chain of the data.
CIFRE PhD: Semantic multimodal question answering in domestic environments
Participants: Teddy Furon, Deniz Engin.
Duration: 3 years, started in June 2020
This is a CIFRE PhD thesis project aiming at designing novel deep learning based MQA techniques that takes in to account rich information from different sensors to facilitate living condition at home. Advances in artificial intelligence boost research towards VQA as well as multimodal analysis.
CIFRE PhD: Multimodal detection of fake news
Participants: Vincent Claveau, Ewa Kijak, Antoine Chaffin.
Duration: 3 years, started in November 2020
This is a CIFRE PhD thesis project aiming at designing multimodal models able to detect fake news, like repurposing techniques, based on joint analysis of visual and textual modalities.
CIFRE PhD: Semantic multimodal question answering (MQA) in domestic environments
Participants: Yannis Avrithis, Teddy Furon, Deniz Engin.
Duration: 3 years, started in September 2020
This is a CIFRE PhD thesis project aiming at designing novel question answering methods based on deep learning to facilitate living conditions in home environments. It investigates moving from image understanding towards multimodal context understanding in video of long duration. This may allow answering questions based on what has happened in the past.
10 Partnerships and cooperations
10.1 International initiatives
10.1.1 Inria associate team not involved in an IIL
LOGIC
- Title: Learning on graph-based hierarchical methods for image and multimedia data
- Duration: 2020 - 2022
- Coordinator: Simon Malinowski
-
Partners:
- VIPLAB, Pontifícia Universidade Católica de Minas Gerais (Brazil)
- Inria contact: Simon Malinowski
- Summary: The main goal of this project is related to learning graph-based hierarchical methods to be applied on image and multimedia data. Regarding image data, we aim at advancing in the state-of-the-art on hierarchy of partitions taking into account aspects of efficiency, quality, and interactivity, as well as the use of hierarchical information to help the information extraction process. Research on graph-based multimedia label/information propagation will be developed within this project along two main lines of research : - construction of multimedia graphs where links should depict semantic proximity between documents or fragments of documents - how different graph structures can be used to propagate information (usually tags or labels) from one document to another and across modalities
10.1.2 Inria international partners
Informal international partners
- Michael Houle, NII, Japan
- Marcel Worring, UvA, Netherlands
- Martha Larson, Radboud U., Netherlands
10.1.3 Participation in other international programs
CAPES COFECUB HIMMD
- Title: Hierarchical Graph-based Analysis of Image, Video and Multimedia Data
- Duration: 2019 - 2022
-
Partners:
- Pontifícia Universidade Católica de Minas Gerais (Brazil)
- Laboratoire d'Informatique Gaspard Monge (France)
- Universidade Federal de Minas Gerais (Brazil)
- Univeristy of Campinas (Brazil)
- Grenoble Institute of Technology (France)
- Institut de Recherche en Informatique et Systèmes Aléatoires (France)
- Contact: Guillaume Gravier
- Summary: The main goal of the project is to advance in the state- of-the-art on hierarchy of partitions taking into account aspects of efficiency, quality, making hierarchical and interactivity, as well as the use of hierarchical information to help in the information extraction and the label propagation. Moreover, we will inves- tigate hierarchical visualization of all, image, video and multimedia, by using countour saliency maps. Finally, we will explore the criteria for hierarchical comparison and for hierarchical combination taking into account their contour saliency maps and learn- ing methods. The results of these studies will be used for solving several applications like human action recognition, pornography detection, image and video region label- ing, multimedia label propagation, image and video inpainting, among others.
10.2 International research visitors
10.2.1 Visits of international scientists
Michalis Lazarou, PhD student at Imperial College, University of London. Planned to stay 5 months (November 2020 - January 2021), but left in November 2020 (stayed 2 months) due to health crisis.
Philip Bellos, MSc student at National and Kapodistrian University of Athens. Planned to stay 4 months (October 2020 - January 2021), but left in November 2020 (stayed 1 month) due to health crisis.
Vasileios Psomas, MSc student at National and Kapodistrian University of Athens. Stayed 4 months (February-May 2020).
Amaia Abanda, PhD Student at BCAM, Spain. She stayed from mid-September to end of October (3 months were planned). Josu Ircio Fernandez, PhD Student at the Center for Technological Research, Spain stayed in October (3 months were planned, but the stay was shortened due to health crisis).
10.3 European initiatives
10.3.1 Collaborations in European programs, except FP7 and H2020
JPI CH READ-IT (Joint Programming Initiative on Cultural Heritage)
Participants: Vincent Claveau, Guillaume Gravier, Ewa Kijak, Suresh Kirthi Kumaraswamy, Guillaume Le Noé-Bienvenu, Pascale Sébillot.
Duration: 3.5 years, started in May 2018
READ-IT is a transnational, interdisciplinary R&D project that will build a unique large-scale, user- friendly, open access, semantically-enriched investigation tool to identify and share groundbreaking evidence about 18th-21st century Cultural Heritage of reading in Europe. READ-IT will ensure the sustainable and reusable aggregation of qualitative data allowing an in-depth analysis of the Cultural Heritage of reading. State-of-the art technology in Semantic Web and information systems will provide a versatile, end-users oriented environment enabling scholars and ordinary readers to retrieve information from a vast amount of community-generated digital data leading to new understanding about the circumstances and effects of reading in Europe.
learninG, pRocessing And oPtimizing shapES (GRAPES)
Participants: Yannis Avrithis.
Duration: 4 years, started in December 2019
GRAPES aims at considerably advancing the state of the art in Mathematics, Computer-Aided Design, and Machine Learning in order to promote game changing approaches for generating, optimizing, and learning 3D shapes, along with a multisectoral training for young researchers. Recent advances in the above domains have solved numerous tasks concerning multimedia and 2D data. However, automation of 3D geometry processing and analysis lags severely behind, despite their importance in science, technology and everyday life, and the well-understood underlying mathematical principles. The CAD industry, although well established for more than 20 years, urgently requires advanced methods and tools for addressing new challenges.
The scientific goal of GRAPES is to bridge this gap based on a multidisciplinary consortium composed of leaders in their respective fields. Top-notch research is also instrumental in forming the new generation of European scientists and engineers. Their disciplines span the spectrum from Computational Mathematics, Numerical Analysis, and Algorithm Design, up to Geometric Modeling, Shape Optimization, and Deep Learning. This allows the 15 PhD candidates to follow either a theoretical or an applied track and to gain knowledge from both research and innovation through a nexus of inter-sectoral secondments and Network-wide workshops.
Horizontally, our results lead to open-source, prototype implementations, software integrated into commercial libraries as well as open benchmark datasets. These are indispensable for dissemination and training but also to promote innovation and technology transfer. Innovation relies on the active participation of SMEs, either as a beneficiary hosting an ESR or as associate partners hosting secondments. Concrete applications include simulation and fabrication, hydrodynamics and marine design, manufacturing and 3D printing, retrieval and mining, reconstruction and visualization, urban planning and autonomous driving.
10.4 National initiatives
Chaire Security of AI for Defense Applications (SAIDA)
Participants: Teddy Furon, Laurent Amsaleg, Erwan Le Merrer, Mathias Rousset, Benoit Bonnet, Thibault Maho, Patrick Bas, Samuel Tap, Karim Tit.
Duration: 4 years, started Sept 2020
Inria Project Lab Knowledge-driven data and content collaborative analytics (iCODA)
Participants: Laurent Amsaleg, Cheikh Brahim El Vaigh, Guillaume Gravier, Cyrielle Mallart, Pascale Sébillot.
Duration: 4.5 years, started in April 2017
One of today’s major issues in data science is the design of algorithms that allow analysts to efficiently infer useful information and knowledge by collaboratively inspecting heterogeneous information sources, from structured data to unstructured content. Taking data journalism as an emblematic use-case, the goal of the project is to develop the scientific and technological foundations for knowledge- mediated user-in-the-loop collaborative data analytics on heterogeneous information sources, and to demonstrate the effectiveness of the approach in realistic, high-visibility use-cases. The project stands at the crossroad of multiple research fields—content analysis, data management, knowledge representation, visualization—that span multiple Inria themes, and counts on a club of major press partners to define usage scenarios, provide data and demonstrate achievements.
INRIA-BNF: Classification d'images patrimoniales (CIP)
Participants: Florent Michel, Laurent Amsaleg, Guillaume Gravier, Ewa Kijak, Yannis Avrithis.
Duration: 1 year, started in Dec 2018. Extended to May 2020.
This project is within the context of the collaborations between INRIA and the French Ministry of Culture. In that context, we have started a collaboration with the French National Library (BNF) which collects, preserves and makes known the national documentary heritage. This collaboration aims at facilitating the automatic classification of heritage images through the use of recent deep-learning techniques. Such images are quite specific: they are not at all similar with what deep-learning techniques are used to work with, that is, the classification of heritage images does not target modern categories such as planes, cars, cats and dogs because this is irrelevant and because heritage collections do not include images of contemporary objects. Furthermore, heritage images come in vast quantities, but they are little annotated and deep-learning techniques can hardly rely on massive annotations to easily learn. Last, the learning has to be continuous as curators may need to add or modify existing classes, without re-learning everything from scratch.
The techniques of choice to reach that goal include the semi-supervised learning, low-shot learning techniques, knowledge transfer, fine tuning existing models, etc.
ANR Archival: Multimodal machine comprehension of language for new intelligent interfaces of scientific and cultural mediation
Participants: Laurent Amsaleg, Guillaume Gravier, Duc Hau Nguyen, Pascale Sébillot.
Duration: 3.5 year, started in Dec. 2019
The multidisciplinary and multi-actor ARCHIVAL project aims at yielding collaborations between researchers from the fields of Information and Communication Sciences as well as Computer Sciences around archive value enhancing and knowledge sharing for arts, culture and heritage. The project is structured around the following questionings: What part can machine comprehension methods play towards the reinterpretation of thematic archive collections? How can content mediation interfaces exploit results generated by current AI approaches?
ARCHIVAL teams will explore heterogeneous document collection structuration in order to explicitly reveal implicit links, to explain the nature of these links and to promote them in an intelligible way towards ergonomic mediation interfaces that will guarantee a successful appropriation of contents. A corpus has been delimited from the FMSH “self-management” collection, recently awarded as Collex, which will be completed from the large Canal-U academic audiovisual portal. The analysis and enhancement of this collection is of particular interest for Humanities and Social Sciences in a context where it becomes a necessity to structurally reconsider new models of socioeconomic development (democratic autonomy, social and solidarity-based economy, alternative development,…).
ANR MEERQAT: MultimEdia Entity Representation and Question Answering Tasks
Participants: Laurent Amsaleg, Yannis Avrithis, Ewa Kijak, Shashanka Venkataramanan.
Duration: 3.5 year, started in April 2020
The overall goal of the project is to tackle the problem of ambiguities of visual and textual content by learning then combining their representations. As a final use case, we propose to solve a Multimedia Question Answering task, that requires to rely on three different sources of information to answer a (textual) question with regard to visual data as well as an external knowledge base containing millions of unique entities, each being represetd by textual and visual content as well as some links to other entities. An important work will deal with the representation of entities into a common tri-modal space, in which one should determine the content to associate to an entity to adequately represent it. The challenge consists in defining a representation that is compact (for performance) while still expressive enough to reflect the potential links between the entity and a variety of others.
MinArm: EVE3
Participants: Teddy Furon.
Duration: 3 year, started in April 2019
Teaching and technology survey on steganography and steganalysis in the real world.
ANR UNLIR: Unsupervised Representation Learning for Image Recognition
Participants: Yannis Avrithis.
Duration: 4 years, started in January 2020
The project lies in the field of computer vision, pattern recognition, and machine learning. We study two problems of image recognition: image classification and image retrieval. Like machine learning, computer vision has witnessed a core change with the recent repopularization of Deep Neural Networks (DNN). Despite the success of DNN, several limitations are to be investigated.
- Complex recognition problems such as fine grained classification (highly similar categories e.g. bird species, airplane/car models, etc.) show that state of the art DNNs are still improved by better objective functions and more discriminative intermediate representations.
- Despite progress in using less annotated data, DNN can hardly cope with learning from few examples.
- DNNs have so many parameters and complex structures that it is extremely hard to understand what happens in every layer in producing the final decision.
This project aims to address these limitations. In particular, we will work towards building networks capable of solving fine-grained visual recognition tasks. We will improve the capabilities of networks to learn from few to no data, building highly discriminative representations that can address complex recognition problems. Following that, we will provide insight on how such models take their decisions.
10.5 Regional initiatives
Computer vision for smart phones (MobilAI)
Participants: Yannis Avrithis, Mateusz Budnik.
Duration: 2 years, started in September 2018
The ability of our mobile devices to process visual information is currently not limited by their camera or computing power but by the network. Many mobile apps suffer from long latency due to data transmitted over the network for visual search. MobilAI aims to provide fast visual recognition on mobile devices, offering quality user experience whatever the network conditions. The idea is to transfer efficient deep learning solutions for image classification and retrieval onto embedded platforms such as smart phones. The intention is to use such solutions in B2B and B2C application contexts, for instance recognizing products and ordering online, accessing information about artifacts in exhibitions, or identifying identity documents. In all cases, visual recognition is performed on the device, with minimal or no access to the network.
11 Dissemination
11.1 Promoting scientific activities
11.1.1 Scientific events: organisation
Member of the organizing committees
- Vincent Claveau, as finance head of ARIA, was involved in the organization of CIRCLE 2020
- Guillaume Gravier was area chair for ACM Multimedia 2020
- Laurent Amsaleg was area chair for ACM Multimedia 2020
- Simon Malinowski co-organized the Workshop on Advanced Learning and Analytics on Temporal Data in September 2020, colocated with ECML/PKDD Conference (virtual event due do health crisis)
11.1.2 Scientific events: selection
Member of the conference program committees
- Laurent Amsaleg was a PC member of: ACM International Conference on Multimedia, ACM International Conference on Multimedia Retrieval, Multimedia Modeling, Content-Based Multimedia Indexing, IEEE International Conference on Multimedia & Expo, International Conference on Similarity Search and Applications.
- Vincent Claveau was a PC member of: CIRCLE, COLING, ECIR, LREC, TALN, workshop 'Ethique et TAL', workshop 'TextMine'
- Guillaume Gravier was PC member of: European Conference on Information Retrieval (ECIR), Intl. Conf. on Multimedia Retrieval (ICMR).
- Ewa Kijak was PC member of: ACM International Conference on Multimedia, IEEE International Conference on Content-Based Multimedia Indexing.
- Pascale Sébillot was a PC member of: International Joint Conference on Artificial Intelligence and Pacific Rim International Conference on Artificial Intelligence (IJCAI-PRICAI), European Conference on Information Retrieval (ECIR), Language Resources and Evaluation Conference (LREC).
- Pascale Sébillot was a reviewer for: Conference Traitement Automatique des Langues Naturelles (TALN).
- Teddy Furon was a reviewer for: Int. Work. on Digital Watermarking (IWDW), IEEE Work. on Information and Forensics (WIFS).
- Yannis Avrithis was a PC member of European Conference on Computer Vision (ECCV).
- Simon Malinowski was a PC member for Workshop on Advanced Learning and Analytics on Temporal Data in September 2020.
11.1.3 Journal
Member of the editorial boards
- Vincent Claveau is a member of the editorial board of Traitement Automatique des Langues (TAL)
- Pascale Sébillot is editor of the Journal Traitement Automatique des Langues (TAL).
- Pascale Sébillot is member of the editorial board of the Journal Traitement Automatique des Langues (TAL).
Reviewer - reviewing activities
- Laurent Amsaleg was a reviewer for: IEEE Transactions on Information Forensics and Security, IEEE Transactions on Signal Processing.
- Vincent Claveau was a reviewer for: Multimedia Tools and Applications (MTAP), IMIA Yearbook of Medical Informatics, Traitement Automatique des Langues (TAL).
- Pascale Sébillot was a reviewer for: Traitement Automatique des Langues (TAL).
- Teddy Furon was a reviewer for: IEEE Trans. on Information and Forensics, IEEE Trans. on Image Processing, IEEE Trans. on Signal Processing.
- Yannis Avrithis was a reviewer for: IEEE Transactions on Pattern Analysis and Machine Intelligence (TPAMI).
- Simon Malinowski was a reviewer for Data Mining and Knowledge Discovery Journal (DAMI).
11.1.4 Invited talks
- Teddy Furon at THALES internal seminar
- Teddy Furon at HUBDAY DS&IA, Pôle de Compétitivité Systematic
11.1.5 Leadership within the scientific community
- Laurent Amsaleg is a member of the Steering Committee of SISAP for the 2016-2020 term
- Laurent Amsaleg is a member of the Steering Committee of ACM Multimedia for the 2020-2023 term
- Vincent Claveau is a member of the Steering Committee of TALN conference (2018-2020)
- Guillaume Gravier is a member of the scientific committee of the GDR Traitement Automatique du Langage Naturel
- Guillaume Gravier is a member of Allistene's national network of AI referents
- Guillaume Gravier is a member of the steering committee of the graduate school (EUR) Digisport
- Guillaume Gravier is coordinating the AI doctoral program (ANR CDIA call) for Rennes
- Pascale Sébillot was a member of the permanent steering committee of Conf. francophone Traitement Automatique des Langues Naturelles (July 2013-July 2020).
- Pascale Sébillot is a member of the board of the pre-GDR Traitement Automatique des Langues; leader of the Intermodality and Multimodality working group till Dec. 2020.
11.1.6 Scientific expertise
- Vincent Claveau served as expert for the evaluation of DATAIA/MSH Paris-Saclay 'Projets excellence'
- Vincent Claveau served as expert for the evaluation of CIFRE PhD projects
- Guillaume Gravier served as an evaluator for the Belgian FED - tWIN program
- Guillaume Gravier evaluated tenure tracks application for UChile
- Guillaume Gravier was a member of the selection committee for INA's call for research projects
- Teddy Furon is Scientific advisor for the Imatag company
- Yannis Avrithis served as expert for the evaluation of Post-Doctoral Research projects for State Education Development Agency, Latvia
- Yannis Avrithis appointed as member of the Advisory Board of European H2020 project DeepCube
11.1.7 Research administration
- Guillaume Gravier is deputy director of IRISA
- Pascale Sébillot is the director of the Computer Science Laboratory, INSA Rennes, France.
- Pascale Sébillot is the deputy director of the Scientific Advisory Committee of IRISA UMR 6074.
- Pascale Sébillot is a member of the theses advisory committee of the MathSTIC doctoral school.
- Pascale Sébillot is a member of the board of the MathSTIC doctoral school.
- Teddy Furon is a member of Commission du Personnel Inria Rennes Bretagne Atlantique
- Laurent Amsaleg is a member of Commission formation permanente, Inria
- Laurent Amsaleg is a member of Commission des moyens incitatifs, Inria
11.2 Teaching - Supervision - Juries
11.2.1 Teaching
- Licence: Laurent Amsaleg, Bases de données avancées, 2h, L3-option génie mathématique, INSA Rennes, France
- Licence: Guillaume Gravier, Base de données, 26h, L2, INSA Rennes
- Licence: Guillaume Gravier, Natural language processing, 12h, L3, INSA Rennes
- Licence: Guillaume Gravier, Probability and statistics, 16h, L3, INSA Rennes, France
- Licence: Pascale Sébillot, Natural Language Processing, 10h, L3, INSA Rennes, France
- Licence: Simon Malinowski, Data Analysis, 32h, L3, ISTIC, Rennes, France
- Master: Laurent Amsaleg, Bases de données avancées, 25h, M2, INSA Rennes, France
- Engineering school: Vincent Claveau, Machine Learning, 18h, 3rd year, INSA Rennes, France
- Master: Vincent Claveau, Information Retrieval, 10h, M2 MIAGE, Univ. Rennes, France
- Master: Pascale Sébillot, Natural Language Processing, 6h, M1, INSA Rennes, France
- Master: Teddy Furon, Rare Event Simulations, INSA Rennes, France
- Master: Guillaume Gravier, Natural Language Processing, 6h, M1, INSA Rennes
- Master: Guillaume Gravier, Natural Language Processing, 21h, M2, ENSAI
- Master: Guillaume Gravier, Data analysis and probabilistic modeling, 30h, M2, Univ. Rennes 1
- Master: Ewa Kijak, Image processing, 55h, M1, ESIR, France
- Master: Ewa Kijak, Supervised machine learning, 15h, M2R, University Rennes 1, France
- Master: Ewa Kijak, Image classification, 45h, M1, ESIR, France
- Master: Ewa Kijak, Computer vision, 22h, M2, ESIR, France
- Engineering school: Vincent Claveau, Machine Learning, 18h, 3rd year, INSA Rennes, France
- Master: Vincent Claveau, Information Retrieval, 10h, M2 MIAGE, Univ. Rennes, France
- Master: Yannis Avrithis, Deep learning for vision, 20h, M2 SIF France
- Master: Yannis Avrithis, Computer vision, 30h, National and Kapodistrian University of Athens, Greece
- Master: Simon Malinowski, Basics of Data Analytics for Data Science, 24h, EIT Data Science Master 1, Rennes
- Master: Simon Malinowski, Prediction Methods, 30h, M1 MIAGE and Data Science EIT Master 1, Rennes
- Master: Simon Malinowski, Statisical Data Mining, 24h, M2 MIAGE, ISTIC, Rennes
- Master: Simon Malinowski, Symbolic Data Mining, 12h, M2 MIAGE, ISTIC, Rennes
- Simon Malinowski is responsible for the Master 2 MIAGE parcours Classique
- Simon Malinowski is responsible for the M2 studies within the DataScience track of the EIT-digital master school.
11.2.2 Supervision
- PhD in progress: Hanwei Zhang, Deep Learning in Adversarial Contexts, October 2017, Laurent Amsaleg, Yannis Avrithis, Teddy Furon
- PhD in progress: Yichang Wang, Adversarial methods for explainable time series classification. Started in April 2018. Simon Malinowski, Elisa Fromont, Romain Tavenard, Rémi Emonet.
- PhD in progress: Marzieh Gheisari Khorasgani, Secure identification in the Internet of Things, January 2018, Laurent Amsaleg & Teddy Furon
- PhD in progress: Antoine Perquin, Universal speech synthesis through embeddings of massive heterogeneous data, October 2017, Laurent Amsaleg, Gwénolé Lecorvé & Damien Lolive (with Expression, IRISA team)
- PhD in progress: Benoit Bonnet, Adversarial images, November 2019, Teddy Furon & Patrick Bas
- PhD in progress: Cheikh Brahim El Vaigh, Incremental content to data linking leveraging ontological knowledge in data journalism, started October 2017, Guillaume Gravier, Pascale Sébillot and François Goasdoué (with CEDAR, Inria team)
- PhD in progress: Cyrielle Mallart, Incremental dynamic construction of knowledge graphs from text mining, started December 2018, Guillaume Gravier, Michel Le Nouy (Ouest-France), Pascale Sébillot
- PhD in progress: Duc Hau Nguyen, Multimodal space for the generation and justification of semantic links between documents, started September 2020, Guillaume Gravier, Pascale Sébillot
- PhD in progress: François Torregrossa, Heterogeneous data embedding for professional search, started November 2018, Robin Allessiardo (So Local), Vincent Claveau, Guillaume Gravier
- PhD in progress: Yann Lifchitz, Few shot learning for object recognition in aerial images. Started March 2018, Yannis Avrithis & Sylvaine Picard (Safran Tech).
- PhD in progress: Raquel Almeida, Learning hierarchichal models for multimedia data, started January 2019, Ewa Kijak & Simon Malinowski & Laurent Amsaleg
- PhD in progress: Shashanka Venkataramanan, Metric learning for instance- and category-level visual representations. Started in December 2020. Yannis Avrithis, Ewa Kijak & Laurent Amsaleg
- PhD in progress: Thibault Maho, Black box attacks, Teddy Furon & Erwan Le Merrer
- PhD in progress: Samuel Tap, Deep learning in the encrypted domain, Teddy Furon
- PhD in progress: Karim Tit, Deep learning and uncertainties, Teddy Furon
- PhD in progress: Deniz Engin, Video query answering in domestic environments. Started in September 2020. Teddy Furon, Yannis Avrithis, Laurent Amsaleg
- PhD in progress: Antoine Chaffin, Multimodal detection of fake news, started November 2020, Ewa Kijak, Vincent Claveau
- PhD: Oriane Siméoni, Robust image representation for classification, retrieval and object discovery, defended Nov. 2020, Yannis Avrithis, Guillaume Gravier
- PhD: Colin Leverger, Probabilistic forecasting of seasonal time series, defended Nov. 2020, Simon Malinowski, Thomas Guyet, Laurence Rozé, Alexandre Termier
- PhD: Clément Dalloux, Text-mining and information extraction in clinical texts, Sup.: Vincent Claveau. Defended in Dec. 2020 43
11.2.3 Juries
- Vincent Claveau was reviewer for the mid-term PhD auditions of Ygor Gallina (LS2N)
- Vincent Claveau was reviewer for the PhD of Paul Mousset (Univ. Toulouse - IRIT)
- Vincent Claveau was reviewer for the PhD of Faneva Ramiandrisoa (Univ. Toulouse - IRIT)
- Guillaume Gravier was president of the HDR juries of Ngoc Quong (Univ. Rennes 1) and Aurelie Lemaitre (Univ. Rennes 2)
- Guillaume Gravier was a reviewer of the PhD thesis of Y. Le Gacheux, CNAM
- Pascale Sébillot was involved in the following juries:
- HDR Peggy Cellier, Université Rennes 1, October 2020, member
- HDR Richard Dufour, Avignon Université, December 2020, reviewer
- Teddy Furon was reviewer for the PhD of Alexandre Sablayrolles (Facebook - Inria Grenoble)
- Teddy Furon was a member of Comité de sélection for IUT St Dié, Univ. Lorraine
- Yannis Avrithis was involved in the following juries:
- PhD Martin Engilberge, Paris-Sorbonne Université, June 2020, reviewer
- PhD Patrick Bordes, Paris-Sorbonne Université, Nov. 2020, reviewer
11.3 Popularization
11.3.1 Articles and contents
- interview for the journal 'Techniques pour l'ingénieur', March 2020
- Vincent Claveau: interview for the journal 'Sciences et vie'
- Vincent Claveau: interview for RFI Brazil, Feb. 2020
- Vincent Claveau: interview for Atlantico (Press), Oct. 2020
11.3.2 Education
- Vincent Claveau: Virtual conference/interview for students of Sciences Po Presse écrite about "Culture et enjeux du numérique"
11.3.3 Interventions
- Vincent Claveau: Public conference Table ronde "Vannes Territoire apprenant"
12 Scientific production
12.1 Major publications
- 1 articleHigh Intrinsic Dimensionality Facilitates Adversarial Attack: Theoretical EvidenceIEEE Transactions on Information Forensics and Security16September 2020, 1-12
- 2 inproceedingsEstimating Local Intrinsic Dimensionality21th ACM SIGKDD International Conference on Knowledge Discovery and Data Mining, KDD'15ACMSidney, AustraliaACMAugust 2015, 29-38
- 3 inproceedingsWhat if Adversarial Samples were Digital Images?IH&MMSEC 2020 - 8th ACM Workshop on Information Hiding and Multimedia SecurityIH&MMSec '20: Proceedings of the 2020 ACM Workshop on Information Hiding and Multimedia SecurityDenver, FranceACMJune 2020, 1-11
- 4 inproceedingsIndiscriminateness in representation spaces of terms and documentsECIR 2018 - 40th European Conference in Information Retrieval10772LNCSGrenoble, FranceSpringerMarch 2018, 251-262
- 5 inproceedings Efficient Diffusion on Region Manifolds: Recovering Small Objects with Compact CNN Representations 2017 IEEE Conference on Computer Vision and Pattern Recognition (CVPR) Honolulu, United States July 2017
- 6 articleImage search with selective match kernels: aggregation across single and multiple imagesInternational Journal of Computer Vision1163Erratum : DOI : 10.1007/s11263-015-0810-42015, 247-261
- 7 articleA Crossmodal Approach to Multimodal Fusion in Video HyperlinkingIEEE MultiMedia2522018, 11-23
12.2 Publications of the year
International journals
International peer-reviewed conferences
Scientific book chapters
Edition (books, proceedings, special issue of a journal)
Doctoral dissertations and habilitation theses
Reports & preprints
12.3 Cited publications
- 48 inproceedings The Vulnerability of Learning to Adversarial Perturbation Increases with Intrinsic Dimensionality WIFS 2017
- 49 inproceedings Estimating Local Intrinsic Dimensionality KDD 2015
- 50 article Prototyping a Web-Scale Multimedia Retrieval Service Using Spark ACM TOMCCAP 14 3s 2018
- 51 inproceedings Scalability of the NV-tree: Three Experiments SISAP 2018
- 52 inproceedings Sketching techniques for very large matrix factorization ECIR 2016
- 53 inproceedings Constraint Satisfaction Programming for Video Summarization ISM 2013
- 54 article Wild Patterns: Ten Years After the Rise of Adversarial Machine Learning Pattern Recognition 2018
- 55 phdthesis Image indexing and retrieval using component trees Université de Bretagne Sud 2016
- 56 phdthesis A storytelling machine? : Automatic video summarization: the case of TV series University of Avignon, France 2016
- 57 inproceedings A Study on Multimodal Video Hyperlinking with Visual Aggregation ICME 2018
- 58 inproceedings Time Series Retrieval using DTW-Preserving Shapelets SISAP 2018
- 59 article Audio Adversarial Examples: Targeted Attacks on Speech-to-Text CoRR abs/1801.01944 2018
- 60 inproceedings Numerical eligibility criteria in clinical protocols: annotation, automatic detection and interpretation AIME 2017
- 61 inproceedings Image Retrieval with Reciprocal and shared Nearest Neighbors VISAPP 2014
- 62 inproceedingsUsing Knowledge Base Semantics in Context-Aware Entity LinkingDocEng 2019 - 19th ACM Symposium on Document EngineeringBerlin, GermanyACMSeptember 2019, 1-10
- 63 book Photo Forensics The MIT Press 2016
- 64 article Recent automatic text summarization techniques: a survey Artif. Intell. Rev. 47 1 2017
- 65 book Deep Learning MIT Press 2016
- 66 inproceedings Shaping-Up Multimedia Analytics: Needs and Expectations of Media Professionals MMM, Special Session Perspectives on Multimedia Analytics 2016
- 67 inproceedings Scaling Group Testing Similarity Search ICMR 2016
- 68 inproceedings Mining on Manifolds: Metric Learning without Labels CVPR 2018
- 69 inproceedings A Multi-Dimensional Data Model for Personal Photo Browsing MMM 2015
- 70 inproceedings Ten Research Questions for Scalable Multimedia Analytics MMM, Special Session Perspectives on Multimedia Analytics 2016
- 71 article Deep Video Portraits ACM TOG 2018
- 72 inproceedings Active Learning to Assist Annotation of Aerial Images in Environmental Surveys CBMI 2018
- 73 article IamNN: Iterative and Adaptive Mobile Neural Network for Efficient Image Classification CoRR abs/1804.10123 2018
- 74 inproceedings Learning DTW-Preserving Shapelets IDA 2017
- 75 inproceedings Context-Aware Forgery Localization in Social-Media Images: A Feature-Based Approach Evaluation ICIP 2018
- 76 inproceedings Connecting the dots between news articles KDD 2010
- 77 inproceedings Weakly Supervised Object Localization Using Things and Stuff Transfer ICCV 2017
- 78 inproceedings Unsupervised part learning for visual recognition CVPR 2017
- 79 inproceedings Memory Vectors for Particular Object Retrieval with Multiple Queries ICMR 2015
- 80 inproceedings Unsupervised Object Discovery for Instance Recognition WACV 2018
- 81 inproceedings Deep Metric Learning via Lifted Structured Feature Embedding CVPR 2016
- 82 inproceedings Highly Efficient Multimedia Event Recounting from User Semantic Preferences ICMR 2014
- 83 article Show and Tell: Lessons Learned from the 2015 MSCOCO Image Captioning Challenge TPAMI 39 4 2017
- 84 phdthesis Deep Neural Architectures for Automatic Representation Learning from Multimedia Multimodal Data INSA de Rennes 2017
- 85 inproceedings Bidirectional Joint Representation Learning with Symmetrical Deep Neural Networks for Multimodal and Crossmodal Applications ICMR 2016
- 86 inproceedings Generative Adversarial Networks for Multimodal Representation Learning in Video Hyperlinking ICMR 2017
- 87 article Memory Networks CoRR abs/1410.3916 2014
- 88 inproceedings Video Paragraph Captioning Using Hierarchical Recurrent Neural Networks CVPR 2016
- 89 inproceedings Towards interactive, intelligent, and integrated multimedia analytics VAST 2014
- 90 inproceedings Crowd Counting via Scale-Adaptive Convolutional Neural Network WACV 2018
- 91 article ShuffleNet: An Extremely Efficient Convolutional Neural Network for Mobile Devices CoRR abs/1707.01083 2017
- 92 inproceedings Provenance filtering for multimedia phylogeny ICIP 2017