Keywords
Computer Science and Digital Science
- A2.5. Software engineering
- A3.1.1. Modeling, representation
- A3.1.4. Uncertain data
- A3.2.2. Knowledge extraction, cleaning
- A5.1. Human-Computer Interaction
- A5.3.4. Registration
- A5.4.4. 3D and spatio-temporal reconstruction
- A5.4.5. Object tracking and motion analysis
- A5.6. Virtual reality, augmented reality
- A6.1.1. Continuous Modeling (PDE, ODE)
- A6.1.2. Stochastic Modeling
- A6.1.5. Multiphysics modeling
- A6.2.3. Probabilistic methods
- A6.2.4. Statistical methods
- A6.2.5. Numerical Linear Algebra
- A6.2.8. Computational geometry and meshes
- A6.3.1. Inverse problems
- A6.3.2. Data assimilation
- A6.3.3. Data processing
- A6.3.4. Model reduction
- A9.2. Machine learning
- A9.10. Hybrid approaches for AI
Other Research Topics and Application Domains
- B1.2. Neuroscience and cognitive science
- B2.2.6. Neurodegenerative diseases
- B2.4. Therapies
- B2.4.3. Surgery
- B2.6. Biological and medical imaging
- B2.7. Medical devices
- B2.7.1. Surgical devices
1 Team members, visitors, external collaborators
Research Scientists
- Stéphane Cotin [Team leader, Inria, Senior Researcher, HDR]
- Hadrien Courtecuisse [CNRS, Researcher]
- Michel Duprez [Inria, Researcher, from Sep 2020]
- Axel Hutt [Inria, Senior Researcher, HDR]
- Didier Pinault [INSERM, Researcher, from Nov 2020, HDR]
Post-Doctoral Fellows
- Mohamed Ryadh Haferssas [Inria, until Nov 2020]
- Andréa Mendizabal [Assistance publique/Hôpitaux de Paris, from Nov 2020]
PhD Students
- Paul Baksic [Univ de Strasbourg]
- Jean-Nicolas Brunet [Inria, until May 2020]
- Andréa Mendizabal [Univ de Strasbourg, until Nov 2020]
- Guillaume Mestdagh [Univ de Strasbourg]
- Sergei Nikolaev [Inria]
- Alban Odot [Inria]
- Josephine Riedinger [Inria, from Dec 2020]
- Raffaella Trivisonne [Pole Emploi, from Feb 2020 until Oct 2020]
- Ziqiu Zeng [Univ de Strasbourg]
Technical Staff
- Mohamed Omar Boukhris [Inria, Engineer]
- Jean-Nicolas Brunet [Inria, Engineer, from Jun 2020]
- Sergei Nikolaev [Inria, Engineer, from May 2020 until Jun 2020]
- Pedro Suruagy Perrusi [Univ de Strasbourg, Engineer, from Sep 2020]
Interns and Apprentices
- Rayan Bounoua [Univ de Strasbourg, from Feb 2020 until Aug 2020]
- Robin Enjalbert [Inria, from Feb 2020 until Aug 2020]
- Francois Lecomte [Inria, from Mar 2020 until Aug 2020]
- Quentin Pubert [Inria, from Jul 2020 until Aug 2020]
- Pedro Suruagy Perrusi [Univ de Strasbourg, from Feb 2020 until Aug 2020]
Administrative Assistant
- Ouiza Herbi [Inria]
Visiting Scientists
- Anna Cazzaniga [Université de Verone - Italie, from Oct 2020 until Nov 2020]
- Nava Schulmann [Ecole Polytechnique Milan, Italie, From November 2020]
- Eleonora Tagliabue [Université de Verone - Italie, from Aug 2020]
External Collaborators
- Rémi Bessard Duparc [Institut Hospitalo-Universitaire de Strasbourg, until Jul 2020]
- Nazim Haouchine [Brigham and Women’s Hospital - Boston USA, from Jul 2020]
- Francois Lecomte [Institut Hospitalo-Universitaire de Strasbourg, from Sep 2020]
2 Overall objectives
2.1 Team Overview
The MIMESIS team develops numerical tools for advanced numerical simulations in the context of surgical training, planning and per-operative guidance (see Fig. 1). The underlying objectives include patient-specific biophysical and electrophysiological modeling, novel numerical techniques for real-time computation, data assimilation using Bayesian methods and more generally data-driven simulation. This last topic is a transverse research theme which raises several open problems, related to the field of machine learning. To pursue these directions we have assembled a team with a multidisciplinary background, and have established close collaborations with academic and clinical partners, in particular the IHU institute in Strasbourg. We also continue the development of the SOFA framework through the creation of a consortium, to better support the increasingly large community of users.
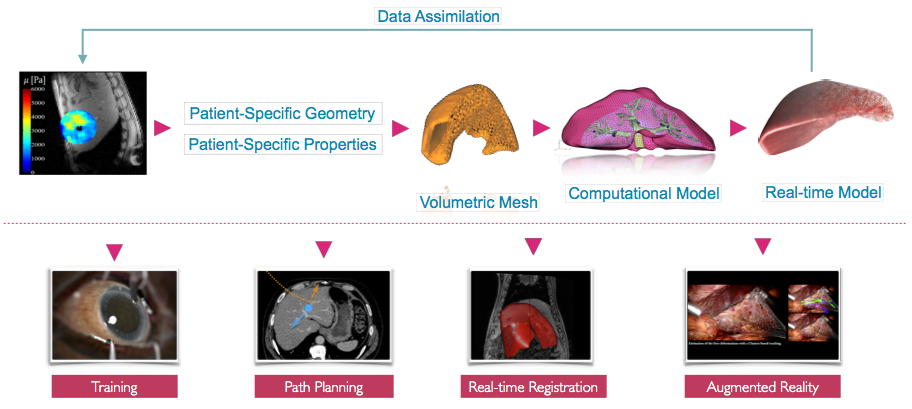
2.2 Challenges
Image- and signal-guided therapy has revolutionized medicine, in its ability to provide care that is both efficient and effective. However, images and multivariate signals acquired during an intervention are either incomplete, under-exploited or can induce adverse outcomes. This can be due, for instance, to the lack of dimensionality of X ray images and the associated radiation exposure for the patient or the spatial sub-sampling in multivariate signals by a too low number of measurement electrodes. We believe that by combining our expertise in real-time numerical simulation (of soft tissues, flexible medical devices, and complex interactions) with data extracted from intra-operative images and experimental multi-electrode signals, we could provide efficient per-operative guidance. To reach these objectives we need to solve challenges that lie at the intersection of several scientific domains. They include the development of novel numerical strategies (to enable real-time computation even with the increase in complexity of future models), and data-driven simulation (to link simulation with real world data).
3 Research program
3.1 Real-time computational models for interactive applications
The principal objective of this challenge is to improve, at the numerical level, the efficiency, robustness, and quality of the simulations (see Fig. 2). An important part of our research is dedicated to the development of computational models that remain compatible with real-time computation, i.e., which allow immediate visual or haptic feedback. This typically requires computation times below 50 ms and in some cases around 1 ms. Such advanced models can not only increase the realism of future training systems, but also act as a bridge toward the development of patient-specific solutions for computer-aided interventions. Additionally, such simulations should run on (high-end) consumer level computers (i.e. with a single multi-core CPU and a dedicated GPU).
To reach these goals, in a first topic we are investigating novel finite element techniques able to cope with complex, potentially ill-defined input data. After developing Smoothed FEM for real-time simulations, we are developing meshless techniques and immersed boundary methods. The first one is well suited for topological changes, which we sometimes need to account for in our simulations. The second is expected to lead to more stable, and numerically efficient, formulations of the finite element method. We are also developing numerical techniques to compute the complex interactions that can take place between anatomical structures or between medical devices and organs. Boundary conditions are known to also play an important role in the solution of such problems. Therefore we are investigating solutions to both identify and model the interactions that take place between the structure of interest and its anatomical environment.
In a different research topic, the team develops neuronal network models that describe the interactions between brain areas under neural stimulation. Each neuron exhibits stochastic spiking activity at the millisecond scale and each brain area comprises thousands of neurons. To achieve numerical real-time integration of the dynamical network models, we aim to reduce the dimensionality of the network models and to this end derive analytically dimension-reduced mean-field models. These models capture the major dynamics of the network models. Typically, such mean-field models are stochastic delayed differential equation systems. For evalution, the network models are simulated on multi-core CPU computers.
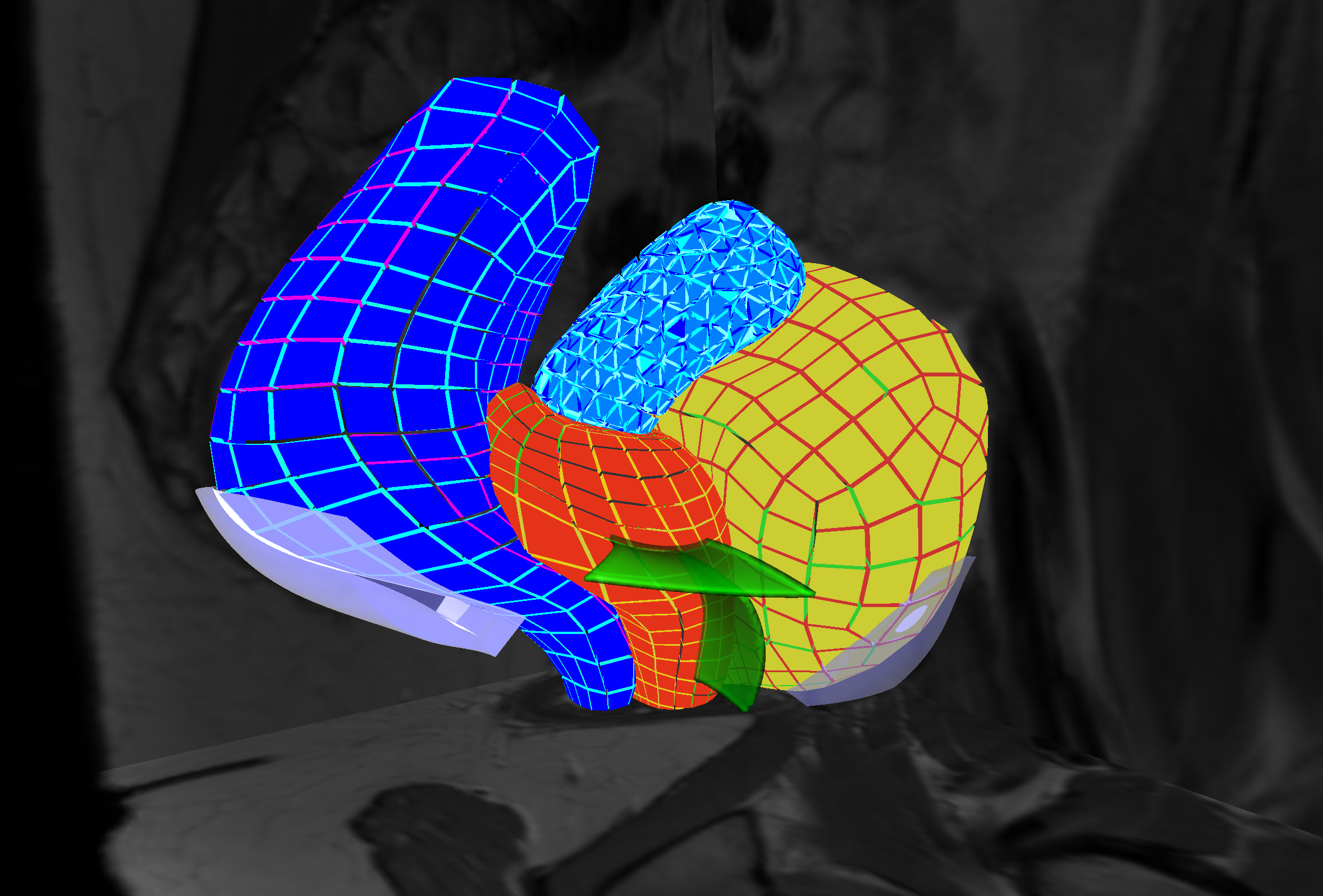
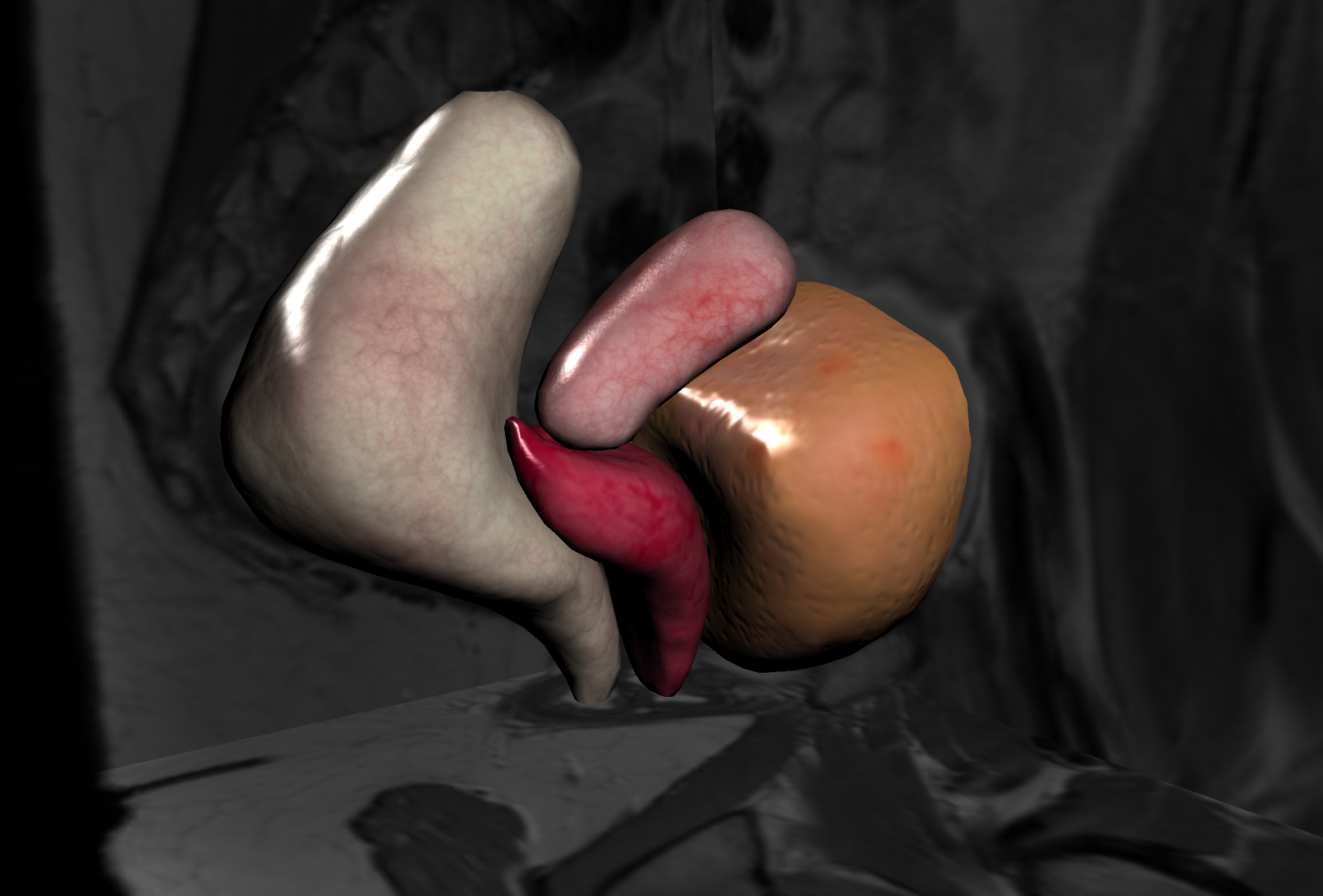
3.2 Data-driven simulations
Data-driven simulation has been a recent area of research in our team (see Fig. 3). We have demonstrated that it has the potential to bridge the gap between medical imaging and clinical routine by adapting pre-operative data to the time of the procedure. In the areas of non-rigid registration and augmented reality during surgery, we have demonstrated the benefit of our physics-based approaches with several key publications in major conferences (MICCAI, CVPR, IPCAI, ISMAR).
We have continued this work with an emphasis on robustness to uncertainty and outliers in the information extracted in real-time from image data, as well as real-time parameter estimation. This is currently done by combining Bayesian methods with advanced physics-based methods to handle uncertainties in image-driven simulations.
Finally, Bayesian or similar methods require to perform a large amount of simulations to sample the domain space, even when using efficient methods such as Reduced Order Unscented Kalman Filters. For this reason, we are investigating the use of neural networks to perform predictions instead of using full numerical simulations. A recent paper 31 shows it is possible to teach a neural network from numerical simulations and predict, with good accuracy, the deformation of an organ.
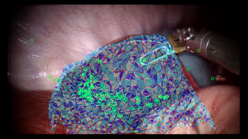
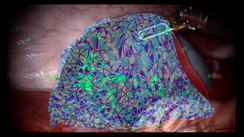
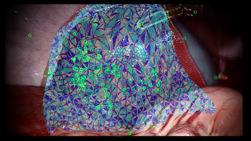
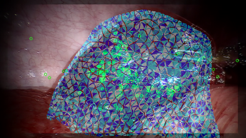
4 Application domains
4.1 Surgical training
Virtual training helps medical students to get familiar with surgical procedures before manipulation of real patients. The development of simulation used for medical training usually requires important computational power, since realistic behaviors are key to deliver a high-fidelity experience to the trainee. Further, the quality of interaction with the simulator (usually via visual and haptic rendering) is also of paramount importance. All these constraints make the development of training systems time-consuming, thus limiting the deployment of virtual simulators in standard medical curriculum.
4.2 Pre-operative planning
Beyond training, clinicians ask for innovative tools that can assist them in the pre-operative planning of an intervention. Using the patient information acquired before the operation, physics-based simulations allow to simulate the effect of therapy with no risk to the patient. The clinicians can thus virtually assess different strategies and select the optimal procedure. Compared to a training simulation, a planning system requires a high accuracy to ensure reliability. Constrained by the time elapsed between the preoperative acquisition and the intervention, the computation must also be efficient.
4.3 Intra-operative guidance
Besides the surgery training and planning, another major need from clinicians is surgical guidance. While the clinician is performing the operation, a guidance system provides enriched visual feedback. This is especially useful with the emergence of minimally invasive surgery (MIS) where the visual information is often strongly limited. It can be used for example to avoid critical areas such as vessels or to highlight the position of a tumor during its resection. In the MIS technique, the clinician does not interact with organs directly as in the open surgery, but manipulates instruments inserted through trocars placed in small incisions in the wall of the abdominal cavity. The surgeon can observe these instruments on a display showing a video stream captured by an endoscopic camera inserted through the navel. The main advantage of the method resides in reducing pain and time recovery, in addition to reducing bleeding and risks of infection. However, from a surgical standpoint, the procedure is quite complex since the field of view is considerably reduced and the direct manipulation of organs is not possible. For an example, see 18.
5 Social and environmental responsibility
MaskDecath: diving masks converted into respirators
Patients who require ventilatory support are usually connected to ventilation systems (Continuous Positive Airway Pressure (CPAP)). Faced with the lack of respiratory assistance equipment and the large number of patients affected by COVID 19, the MaskDecath project aimed to print in 3D an adapter (and if necessary a valve) to transform a Decathlon diving mask into a low-cost CPAP device that could be distributed quickly. To make these adapters, we were inspired by a similar Italian initiative, and then optimized their design through numerous tests to make them more functional. Within a few days, many parts were printed with the on-board means available to our team and a partner team at Twente University.
To make sure that the masks were suitable for use on patients, and in particular that with our modifications the contaminated air exhaled by the patient was well filtered before being discharged outside, we worked with Dr. Silvana Perretta, from the civil hospital in Strasbourg. Once the tests were passed, around 100 adapters were printed, with 30 of them used at the hospital in Strasbourg and the rest of the modified masks were sent to Colmar and Mulhouse. More information on this project can be found under https://
6 Highlights of the year
Neurostimulation stabilizes spiking neural networks by disrupting seizure-like oscillatory transitions
An improved understanding of the mechanisms underlying neuromodulatory approaches to mitigate seizure onset is needed to identify clinical targets for the treatment of epilepsy. We examined the role played by intrinsic and extrinsic stimuli on a network’s predisposition to sudden transitions into oscillatory dynamics, similar to the transition to the seizure state 24. Our analyses revealed that such stimuli, be they noisy or periodic in nature, exert a stabilizing influence on network responses, disrupting the development of such oscillations. Our research shows that such stabilization of neural activity occurs through a selective recruitment of inhibitory cells, providing a theoretical undergird for the known key role these cells play in both the healthy and diseased brain.
Alternating quarantine for sustainable epidemic mitigation
Absent pharmaceutical interventions, social distancing, lock-downs and mobility restrictions remain our prime response in the face of epidemic outbreaks. To ease their potentially devastating socioeconomic consequences, we proposed
22
an alternating quarantine strategy: at every instance, half of the population remains under lockdown while the other half continues to be active - maintaining a routine of weekly succession between activity and quarantine. As a result it provides a dramatic reduction in transmission, comparable to that achieved by a population-wide lockdown, despite sustaining socioeconomic continuity at
7 New software and platforms
7.1 New software
7.1.1 SOFA
- Name: Simulation Open Framework Architecture
- Keywords: Real time, Multi-physics simulation, Medical applications
- Functional Description: SOFA is an Open Source framework primarily targeted at real-time simulation, with an emphasis on medical simulation. It is mostly intended for the research community to help develop new algorithms, but can also be used as an efficient prototyping tool. Based on an advanced software architecture, it allows : the creation of complex and evolving simulations by combining new algorithms with algorithms already included in SOFA, the modification of most parameters of the simulation (deformable behavior, surface representation, solver, constraints, collision algorithm, etc. ) by simply editing an XML file, the building of complex models from simpler ones using a scene-graph description, the efficient simulation of the dynamics of interacting objects using abstract equation solvers, the reuse and easy comparison of a variety of available methods.
- News of the Year: The new version v20.06 has been released including new elements on SoftRobots + ModelOrderReduction integration, in addition to an improved architecture and lots of cleans and bugfixes.
-
URL:
http://
www. sofa-framework. org - Contacts: Hugo Talbot, Stéphane Cotin, Christian Duriez, Hervé Delingette, François Faure, Maud Marchal
- Participants: Christian Duriez, François Faure, Hervé Delingette, Stéphane Cotin, Hugo Talbot, Maud Marchal
- Partner: IGG
7.1.2 SofaPython3
- Name: SofaPython3
- Keywords: Python, Numerical simulations, Sofa
- Functional Description: This plugin allows to use Sofa as a library from any python3 program. It also allows to write new mechanical component for a Sofa simulation in python3.
- Release Contributions: Python3 and SOFA are meeting in one plugin: SofaPython3. In 2020, the integration of this plugin within SOFA opens up new perspectives. Not only can a SOFA simulation be described using a Python script with the embedded Python interpreter, but one or several SOFA instances can now created from a Python environment. Even more powerful, you can create new components (e.g. forcefields) in Python using the bindings provided in the plugin. Python-scripted simulations have no limit anymore!
- News of the Year: Python3 and SOFA are meeting in one plugin: SofaPython3. In 2020, the integration of this plugin within SOFA opens up new perspectives. Not only can a SOFA simulation be described using a Python script with the embedded Python interpreter, but one or several SOFA instances can now created from a Python environment. Even more powerful, you can create new components (e.g. forcefields) in Python using the bindings provided in the plugin. Python-scripted simulations have no limit anymore!
-
URL:
https://
github. com/ sofa-framework/ SofaPython3/ - Contacts: Bruno Marques, Damien Marchal, Jean-Nicolas Brunet, Thierry Gaugry, Frédérick Roy, Guillaume Paran, Hugo Talbot
- Participants: Jean-Nicolas Brunet, Damien Marchal, Bruno Marques, Thierry Gaugry, Frédérick Roy, Guillaume Paran, Hugo Talbot
7.1.3 Caribou
- Name: Caribou - SofaCaribou
- Keywords: Sofa, Multiphysics modelling, Biomechanics, Geometric algorithms, Plugin SOFA
-
Functional Description:
The Caribou project is aimed at multiphysics computation. This plugin complements the FEM-based models existing in SOFA with a more modularized approach. For continuum solid mechanics, the mechanical law, the type of finite element and the quadrature method can be selected separately. This method therefore eases the reading and understanding of the code and the associated theory. The Caribou project also provides generic C++ utilities, and SOFA components such as solvers.
The project is composed of two modules: (i) Caribou library brings multiple geometric, linear analysis and topological tools that are designed to be as independent as possible from external projects. (ii) SofaCaribou library is built on top of the caribou library, but brings new components to the SOFA project as a plugin.
- News of the Year: The project is new and aims at multiphysics computation.
- Publication: 02158862
- Authors: Jean-Nicolas Brunet, Stéphane Cotin
- Contacts: Jean-Nicolas Brunet, Stéphane Cotin
7.1.4 SOFA Optimus
- Name: Optimization methods in SOFA: stochastic filtering and data assimilation.
- Keywords: Data assimilation, Kalman filter, Stochastic optimization
- Functional Description: Optimus is a plugin to work with advanced methods of state estimation and parameter identification. It was created to provide a testing environment for data-driven physics-based modeling (typically finite elements). While currently the plugin implements only stochastic methods based on Kalman filtering, its architecture allows for the implementation of generic prediction–correction schemes where the model is employed as a predictor and correction is performed using given observation data.
- News of the Year: Late 2020, the repository has been cleaned and prepared for its open-source release.
-
URL:
https://
gitlab. inria. fr/ mimesis/ Optimus - Authors: Igor Peterlik, Sergei Nikolaev, Stéphane Cotin, Raffaella Trivisonne, Nava Schulmann
- Contacts: Stéphane Cotin, Raffaella Trivisonne, Sergei Nikolaev, Nava Schulmann
- Participant: Sergei Nikolaev
8 New results
8.1 Real-time simulation of hyperelastic materials using Deep Learning
Participants: Andrea Mendizabal, Eleonora Tagliabue, Tristan Hoellinger, Jean-Nicolas Brunet, Sergei Nikolaev, Stéphane Cotin.
The finite element method (FEM) is among the most commonly used numerical methods for solving engineering problems. Due to its computational cost, various ideas have been introduced to reduce computation times, such as domain decomposition, parallel computing, adaptive meshing, and model order reduction. In an article 31 and in a thesis 33 we have demonstrated how the use of machine learning algorithms in combination with conventional numerical methods could improve computer-assisted interventions. In particular, we have presented the U-Mesh method based on deep neural networks, which models the biomechanical behavior of an organ, respecting the specificities of each patient and satisfying the real-time constraint inherent in image-guided surgery. In the pre-operative phase, we built a finite element model of the organ considered with average parameters, in order to generate a training dataset to train the network. Then, during surgery, the mechanical properties of organs are identified with Bayesian Inference and new patient-specific data can be generated. The weights of networks previously trained can be updated using the new simulated data.8.2 Three‐dimensional registration using 2D dynamic magnetic resonance imaging slices
Participants: Hadrien Courtecuisse, Stéphane Cotin.
The aim of this work is to provide a better knowledge and understanding of pathologies such as prolapsus or abnormal mobility of tissues. 2D dynamic MRI sequences are commonly used in clinical routines in order to evaluate the dynamic of organs, but due to the limited field of view, subjectivity related to human perception cannot be avoided in the diagnoses. To this end, a novel method for 2D/3D registration is proposed 16 combining 3D finite element models with a priori knowledge of boundary conditions, in order to provide a 3D extrapolation of the dynamic of the organs observed in a single 2D MRI slice. The method is applied to the four main structures of the female pelvic floor (bladder, vagina, uterus and rectum), providing a full 3D visualisation of the organs' displacements. The methodology is evaluated with two patient‐specific data sets of volunteers presenting no pelvic pathology, and a sensitivity study is performed using synthetic data. The resulting simulations provide an estimation of the dynamic 3D shape of the organs which facilitates the diagnosis compared with 2D sequences.8.3 Methods to interweave observations and models
Participants: Axel Hutt, Nava Schulmann, Stéphane Cotin.
Observations may improve theoretical models and several techniques are known to merge both in an optimal way. In this context, we have been working on recurrence analysis 14, that is a data analysis technique applied to extract an underlying dynamical model. Moreover, we also have been working on mathematical models 19, 24, 40 that aim to reproduce experimental data. Data assimilation combines both observations and models in one technique. We have employed the Localized Ensemble Transform Kalman Filter (LETKF) to estimate short-term predictions in the presence of nonlocal observations 21, 25. Future studies on the LETKF aim to understand better how nonlocal observations affect predictions and how they should be assimilated to achieve optimum predictions. In this context, Axel Hutt has co-edited the book “Synergetics” 30 published in the Springer series “Encyclopedia of Complexity and Systems”, together with Hermann Haken (emeritus at University of Stuttgart).8.4 Estimation of boundary conditions for patient-specific liver simulation during augmented surgery
Participants: Sergei Nikolaev, Stéphane Cotin.
The liver is the largest solid organ in the human body. It plays a fundamental role in various vital functions. Therefore, liver diseases might cause severe problems that lead to a variety of abnormalities and shorter life expectancy. Together with liver transplantation, the typical treatment of liver cancer is liver resection. A possible solution to support the medical surgery is to use a model that simulates the behavior of the real organ, cf. Fig. 4. Matched to the visible part, it predicts the positions of invisible parts and shows the locations of initially registered tumors. While researchers are focused on patient-specific liver properties, very few have addressed the question of boundary conditions. Resulting mainly from ligaments attached to the liver, they are not visible in pre-operative images, yet play a key role in the computation of the deformation. We have proposed 23 to estimate both the location and stiffness of ligaments by using a combination of a statistical atlas, numerical simulation, and Bayesian inference. Our approach is evaluated using synthetic data and phantom data. Results show that our estimation of the boundary conditions improves the accuracy of the simulation by 75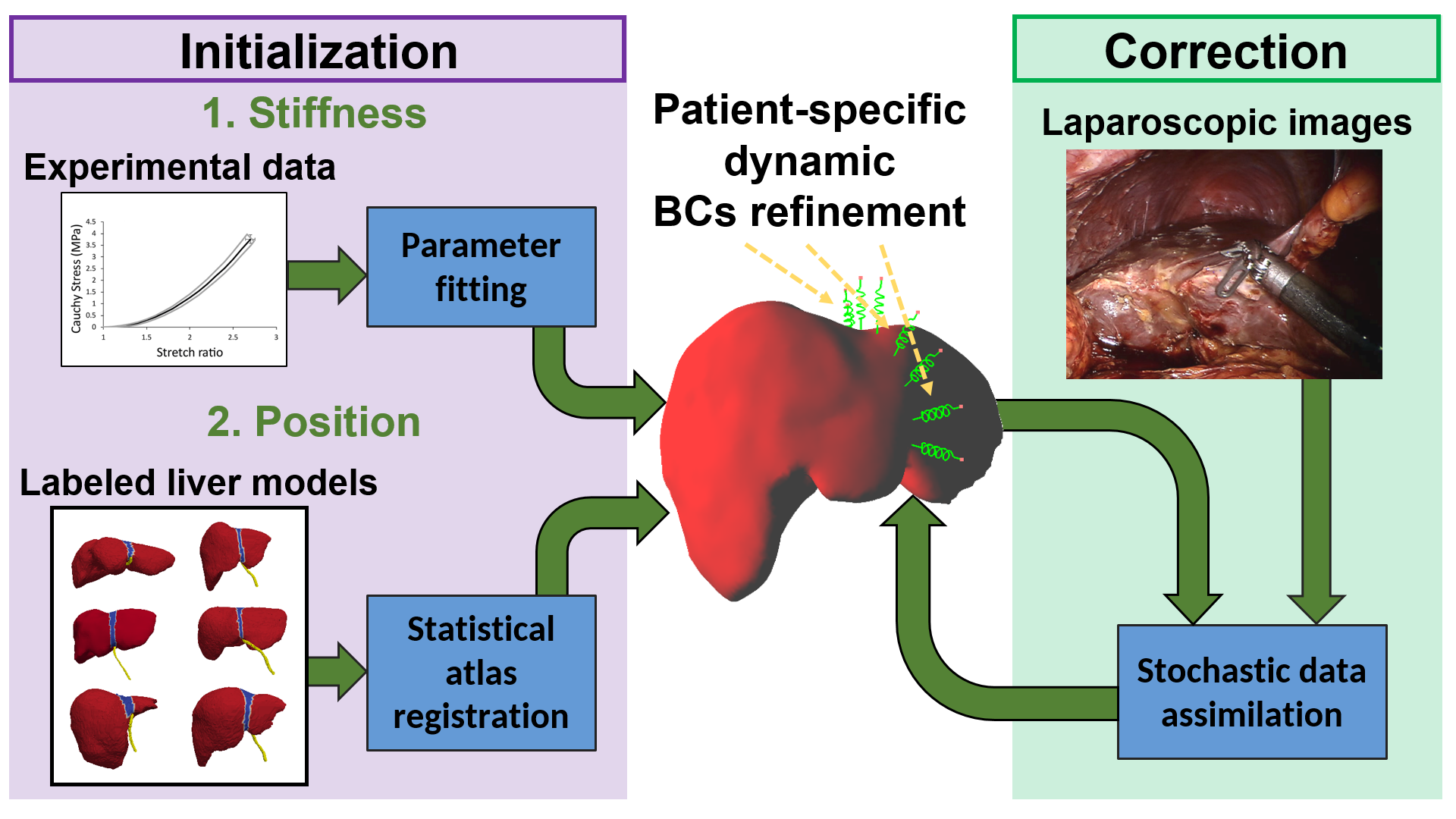
8.5 New Exploratory Action “A/D Drugs”
Participants: Axel Hutt, Josephine Riedinger, Didier Pinault.
When it comes to treating mental disorders, the emergence of resistance to medication is a major problem. Replacing chemical medicine with digital medicine (neural stimulation) could be one way of getting around the problem (https://
8.6 Deformation aware augmented reality for craniotomy using 3D/2D non-rigid registration of cortical vessels
Participants: Nazim Haouchine, Stéphane Cotin.
Intra-operative brain shift is a well-known phenomenon that describes non-rigid deformation of brain tissues due to gravity and loss of cerebrospinal fluid among other phenomena. This has a negative influence on surgical outcome that is often based on pre-operative planning where the brain shift is not considered. We present a novel brain-shift aware Augmented Reality method to align pre-operative 3D data onto the deformed brain surface viewed through a surgical microscope 27, 28. We formulate our non-rigid registration as a Shape-from-Template problem. A pre-operative 3D wire-like deformable model is registered onto a single 2D image of the cortical vessels, which is automatically segmented. This 3D/2D registration drives the underlying brain structures, such as tumors, and compensates for the brain shift in sub-cortical regions. We evaluated our approach on simulated and real data composed of 6 patients. It achieved good quantitative and qualitative results making it suitable for neurosurgical guidance.8.7 Numerical schemes and model control
Participants: Michel Duprez.
In our work, we consider the major challenges in taking into account the complexity of the geometries on which finite element simulations are carried out. In the research context of fictitious domains 39, we have developed a numerical scheme taking into account the boundary data using a levelset function which is an object that can be obtained during the segmentation of the images. Concerning studies related control 35, 38, 36, 37, 15, the qualitative analysis of optimal strategies allows a better understanding of the biological and physical phenomena in question and the development of faster numerical approximation techniques. We have demonstrated the feasibility of such commands and studied the impact of temporal and spatial influence. We have sometimes even given an explicit characterization of optimal strategies. In most of these studies, we numerically illustrate the validity of the theoretical results obtained.8.8 Predicted microscopic cortical brain images for optimal craniotomy positioning and visualisation
Participants: Nazim Haouchine.
During a craniotomy, the skull is opened to allow surgeons to access the brain and perform the procedure. The position and size of this opening are chosen in a way to avoid critical structures, such as vessels, and facilitate the access to tumours. Planning the operation is done based on pre-operative images and does not account for intra-operative surgical events. We present a novel image-guided neurosurgical system 20 to optimise the craniotomy opening. Using physics-based modelling we define a cortical deformation map that estimates the displacement field at candidate craniotomy locations. This deformation map is coupled with an image analogy algorithm that produces realistic synthetic images that can be used to predict both the geometry and the appearance of the brain surface before opening the skull. These images account for cortical vessel deformations that may occur after opening the skull and is rendered in a way that increases the surgeon’s understanding and assimilation. Our method was tested retrospectively on patients data showing good results and demonstrating the feasibility of practical use of our system.8.9 Use of stereo-laparoscopic liver surface reconstruction to compensate for pneumoperitoneum deformation through biomechanical modeling
Participants: Jean-Nicolas Brunet, Sergei Nikolaev, Stéphane Cotin.
Abdominal organs undergo large deformations due to intra-abdominal pressure (pneumoperitoneum) during laparoscopic surgery, especially large organs such as the liver. These deformations cause large inaccuracies when using surgical navigation systems. Fortunately, intra-operative imaging through CT/MRI can be acquired in modern hybrid ORs as well as laparoscopic ultrasound and can both be used to provide updated organ models. However, these medical imaging modalities are expensive and may extend the surgical workflow, hence, biomechanical models could be used as a solution for intra-operative registration, also to account for organ deformations due to surgical manipulation. Within our study 32, we propose a solution to compensate for pneumoperitoneum, which could greatly increase the accuracy of liver surgical navigation systems.9 Bilateral contracts and grants with industry
9.1 Bilateral contracts with industry
- Company: Siemens (France)
- Duration: 3 years (ended in Dec 2020)
- Participants: Stéphane Cotin and Francois Lecomte
- Description: Siemens is a global leader in healthcare industry. Via IHU, we collaborate with Siemens in the context of the IHU project CIOS Alpha Fusion dealing with augmentation of the intra-operative image provided by a fluoroscopic imaging modality with pre-operative data.
10 Partnerships and cooperations
10.1 International research visitors
10.1.1 Visits of international scientists
- Micha Pfeiffer from the German Cancer Research Center in Dresden (Germany) has visited us in September.
- Anna Cazzaniga from the University of Verona (Italy) has visited us from Oct 2020 until Nov 2020.
- Eleonora Tagliabue from the University of Verona (Italy) has visited us from Aug 2020
10.2 European initiatives
10.2.1 FP7 & H2020 Projects
HiPerNav
- Title: High Performance Soft-tissue Navigation
-
Partners:
- CASCINATION AG (Switzerland)
- Innovasjon Norge (Norway)
- NORGES TEKNISK-NATURVITENSKAPELIGE UNIVERSITET NTNU (Norway)
- OSLO UNIVERSITETSSYKEHUS HF (Norway)
- SIEMENS HEALTHCARE GMBH (Germany)
- SINTEF AS (Norway)
- TECHNISCHE UNIVERSITEIT DELFT (Netherlands)
- UNIVERSIDAD DE CORDOBA (Spain)
- UNIVERSITE PARIS 13 (France)
- YES!Delft BV (Netherlands)
DRIVEN
- Title: Increasing the scientific excellence and innovation capacity in Data-Driven Simulation of the University of Luxembourg
-
Partners:
- Université du Luxembourg (Luxembourg)
- University of Limerick (Ireland)
10.3 National initiatives
ADT (Action de Développement Technologique)
MIMESIS had received a support for the development of the project LOSAR: Liver Open Surgery with Augmented Reality which has been finalized in 2020. This project had been done in collaboration with Paul Brousse Hospital in Paris. The goal of Losar was to develop the first augmented reality software usable in the operating room in order to assist surgeons during open liver surgery. To do this, we have developed a stand-alone application to process and automate the generation of preoperative data, as well as to improve the ergonomics of the current software to better meet clinical constraints. The ADT enabled the development of methods for RGBD cameras which facilitate the use of the method in the OR (no markers) and reduce the constraints with the workspace of medical staff. Finally, we worked on solutions to improve the stability of the solution and make more robust the registration algorithms based on real-time biomechanical models. Several experiments have been carried out to validate the system in vivo and ex vivo.
MIMESIS has received support for the development of the project DeepPhysX: Data-driven simulation.This project aims to develop new tools for real-time navigation and registration in image-guided surgery. It aims to integrate learning methods with numerical simulations in order to obtain robust predictions adapted to the patient. At first, the project considers previous calculation codes, e.g. PyTorch, SOFA and its Python3 interface and the Caribou plugin of Sofa. Then the development of the Kromagon plugin of Sofa dedicated to deep learning (Python and C ++ development) will continue. Essentially, a new Newton algorithm will be developed that combines AI-based prediction and numerical computation. Performance and accuracy evaluation and documentation and provision of the plugin (binary or open) will close the project. This work will be performed in close collaboration to the “surgical guidance” project (Hopital Paul Brousse and / or IHU Strasbourg).
ANR (Agence Nationale de la Recherche)
MIMESIS coordinates the ANR project entitled SPERRY: SuPervisEd Robotic suRgerY - application to needle insertion. Percutaneous medical procedures (using surgical needles) are among the least invasive approaches to accessing deep internal structures of organs without damaging surrounding tissues. Today, many surgical procedures rely on the use of needles allowing for complex interventions such as curie-therapies or thermo-ablations of tumors (cryoablation, radio frequencies). Unlike traditional open surgery, these approaches only affect a localized area around the needle, reducing trauma and risks of complications. These treatments also offer new solutions for tumors or for metastases for which traditional methods may be contraindicated due to the age of the patient and the extent or location of the disease. In this project, we want to develop new solutions for the control of medical robots interacting with soft tissues. This work is motivated by recent advances in the field of medical simulation achieving a sufficient level of realism to help surgeons during the operation. The maturity of these techniques now suggests the ability to use a simulation intra-operatively to control the motion of a robotic system for needle insertion. This is really a challenge, because in general, little information can be extracted in real time from images during an intervention. We believe that even minimal knowledge of the mechanical behavior of structures, associated with the use of images can make it possible and allow a robot to reach a pre-identified target during a planning stage, without human intervention.
In addition, we participate in the ANR Project VATSOP: Images and models for computer guidance during Video Assisted Thoracic Surgery (VATS). In France, lung cancer is the most common cause of death by cancer before breast and colorectal cancers. Video-Assisted Thoracic Surgery (VATS) is a minimally-invasive trans-thoracic procedure that has become prominent for the treatment of lung cancer. However, localizing the nodules during VATS is extremely challenging due to the technical difficulties inherent to endoscopic surgery, and due to the collapse of the lung during the procedure. The main objective of our project is to develop and integrate, in an operating room, an image-based guidance solution which would: 1) estimate the position of the patient’s nodule after pneumothorax from CBCT images; 2) propose an augmented reality-based guidance to this location on the endoscopic view; 3) allow to follow this location in real-time on the endoscopic view, during manipulation of the lung by the surgeon. Partners of the project are TIMC Grenoble and LTSI Rennes.
Sofa Consortium
MIMESIS is closely connected to the SOFA Consortium, created by Inria in November 2015 with the objective to support the SOFA community and encourage contributions from new SOFA users. The consortium should also be a way to better answer to the needs of academic or industrial partners. MIMESIS actively participates at the development of SOFA and contributes to the evolution of the framework. Moreover, MIMESIS also participates in an initiative aiming at verification and validation of codes and algorithms of SOFA.
National Exploratory Action
- Title: A/D Drugs
- Duration: April 2020 - December 2023
- Coordinator: Axel Hutt
- Partners: Didier Pinault (INSERM 1114)
- Participants: Axel Hutt, Joséphine Riedinger, Didier Pinault
-
Summary:
When it comes to treating mental disorders, the emergence of resistance to medication is a major problem. Replacing chemical medicine with digital medicine (neural stimulation) could be one way of getting around the problem.
This is the concept behind "A/D Drugs" (PI : Axel Hutt) which will deploy a process of data assimilation and control in order to adapt stimulation to each patient.
But this research is not without its risks - very little is known about the links between the effect of the chemical molecules and neurostimulation (https://
www. ).inria. fr/ en/ ad-drugs-exploratory-action-aimed-optimising-neurostimulation
11 Dissemination
11.1 Promoting scientific activities
11.1.1 Scientific events: organisation
General chair, scientific chair
Stephane Cotin is part of the program committee of IPCAI 2021 (as Area Chair)
11.1.2 Scientific events: selection
Chair of conference program committees
Stephane Cotin is co-Program chair for MICCAI 2021
Member of the conference program committees
Axel Hutt is member of the PC of MIMESIP 2021.
11.1.3 Journal
Member of the editorial boards
- Axel Hutt is Chief Editor of the section "Dynamical Systems" of the journal Frontiers in Applied Mathematics and Statistics.
- Axel Hutt is Associate Editor for the Journal of Clinical Medicine
- Axel Hutt was Guest Editor of the Frontiers Research Topic Recurrence Analysis of Complex Systems
- Axel Hutt was Guest Editor of the Frontiers Research Topic Stability: A current overview
- Axel Hutt was Guest Editor of the Frontiers Research Topic Data Assimilation of Nonlocal Observations in Complex Systems
- Axel Hutt is Guest Editor of the still running Frontiers Research Topic Machine Learning in Natural Complex Systems
- Axel Hutt is Guest Editor of the still running Frontiers Research Topic Modelling collective motion across scales
Reviewer - reviewing activities
Axel Hutt has reviewed manuscripts for Frontiers in Human Neuroscience, Frontiers in Applied Mathematics and Statistics, PLoS Computational Biology and Scientific Reports.
11.1.4 Invited talks
- Stephane Cotin gave the talk “Vers un jumeau numérique” at the Inauguration of the “Chaire d’innovation BOPA” in Villejuif (June 2020)
- Stephane Cotin was invited speaker at the French-African seminar on Computer Science (July 2020)
- Axel Hutt has given a talk at the online “Conference on Complex Systems 2020” (December 4-11, 2020)
- Axel Hutt has given a talk at the online “Dynamics Days 2020” (August 24-27, 2020)
- Axel Hutt has given a talk at the online “International Conference on Mathematical Neuroscience 2020” (July 6-7, 2020)
- Axel Hutt has given a seminar talk in the Exeter Dynamics Group, University of Exeter, UK (December 1, 2020)
- Axel Hutt has given a seminar talk in the Institute of Metabolism and Systems Research, University of Birmingham, UK (October 5, 2020)
11.1.5 Leadership within the scientific community
Stéphane Cotin has been elected as a member of the National Academy of Surgery (in November 2020).
11.2 Teaching - Supervision - Juries
Teaching
- Master: Hadrien Courtecuisse, Real-time simulation, 80h, M2, University of Strasbourg
- License: Michel Duprez, Informatics, 34h, L3, University of Strasbourg
- License: Michel Duprez, Numerical analysis techniques, 17h+15h, L3, University of Strasbourg
- Master: Michel Duprez, Incertitude quantification, 14h, M2, University of Strasbourg
Supervision
- PhD : Raffaella Trivisonne, "Computer-aided vascular interventions", supervised by Stéphane Cotin and Erwan Kerrien. Defended on Octobre 15, 2020 34.
- PhD : Jean-Nicolas Brunet, "Characterization of boundary conditions for biomechanical modeling of liver", supervised by Stéphane Cotin. Defended on November 4, 2020 32
- PhD : Andrea Mendizabal, "Numerical simulation of soft tissues and machine learning", supervised by Stéphane Cotin. Defended on December 3, 2020 33.
- PhD : Sebastian Rimbert, "Contribution of median nerve stimulation in the design of a BCI based on cerebral motor activity : towards improvement in the detection of intraoperative awareness during general anaesthesia", supervised by Axel Hutt and Laurent Bougrain. Defended on Octobre 19, 2020.
- PhD in progress: Nicolas Golse, "Navigation using the augmented reality during hepatic surgery", started 01/09/2016, supervised by Stéphane Cotin.
- PhD in progress: Sergei Nikolaev, "Characterization of boundary conditions for biomechanical modeling of liver", started 01/05/2017, supervised by Stéphane Cotin, co-supervised by Igor Peterlik and Hadrien Courtecuisse.
- PhD in progress: Paul Baksic, "Robotic assistance for percutaneous surgical interventions in deformable structures – Application to radiofrequency ablation", started 01/10/2018, supervised by Hadrien Courtecuisse.
- PhD in progress: Ziqiu Zeng, "SPERRY - Supervised Robotic Surgery - Application to needle insertion", started 01/07/2019, supervised by Hadrien Courtecuisse.
- PhD in progress: Guillaume Mestdagh, "Real-Time Tumor Tracking as an Optimization Problem", started 01/09/2019,co-supervised by Stéphane Cotin.
- PhD in progress: Joséphine Riedinger, "Closed-loop Transcranial Electric Stimulation of Neural Networks in a Rodent Model of Psychosis Transition" , started 01/12/2020, supervised by Axel Hutt and Didier Pinault.
11.2.1 Juries
- Stephane Cotin was reviewer and member of the jury for the PhD thesis of Pablo ALVAREZ (Univ Rennes)
- Stephane Cotin was reviewer and member of the jury for the PhD thesis of Charles BARNOUIN (Univ Lyon),
- Stephane Cotin was member of the PhD committee of Agniva SENGUPTA (Univ Rennes).
- Stephane Cotin was reviewer and committee member for the Habilitation thesis of Florence ZARA (Univ Lyon).
11.3 Popularization
11.3.1 Articles and contents
The team’s research has been mentioned in “Sciences et Avenir” (No.887, January 2021).
12 Scientific production
12.1 Major publications
- 1 articleControlling the error on target motion through real-time mesh adaptation: applications to Deep Brain StimulationInternational Journal for Numerical Methods in Biomedical EngineeringDecember 2017, 1-26
- 2 articleReal-time simulation of contact and cutting of heterogeneous soft-tissuesMedical Image AnalysisFebruary 2014, 20
- 3 articleBiomechanics-based graph matching for augmented CT-CBCTIJCARSApr 2018, URL: https://doi.org/10.1007/s11548-018-1755-1
- 4 articleElastic Registration Based on Compliance Analysis and Biomechanical Graph MatchingAnnals of Biomedical Engineering2019, 1--16
- 5 article Vision-based Force Feedback Estimation for Robot-assisted Surgery using Instrument-constrained Biomechanical 3D Maps IEEE Robotics and Automation Letters Proceedings of International Conference on Robotics and Automation ICRA 2018 February 2018
- 6 articleBlood vessel modeling for interactive simulation of interventional neuroradiology proceduresMedical Image Analysis35January 2017, 685 - 698
- 7 inproceedings A Combined Simulation & Machine Learning Approach for Image-based Force Classification during Robotized Intravitreal Injections MICCAI 2018 - 21st International Conference on Medical Image Computing and Computer Assisted Intervention Granada, Spain September 2018
- 8 articleSimulation of hyperelastic materials in real-time using deep learningMedical Image Analysis592019, 101569
- 9 articleBrain-shift compensation using intraoperative ultrasound and constraint-based biomechanical simulationMedical Image Analysis402017, 133 - 153
- 10 article Fast Elastic Registration of Soft Tissues under Large Deformations Medical Image Analysis 2017
- 11 inproceedings Capturing Deformations of Interacting Non-rigid Objects Using RGB-D Data IROS 2018 - IEEE/RSJ International Conference on Intelligent Robots and Systems Madrid, Spain October 2018
- 12 articleFiloGen: A Model-Based Generator of Synthetic 3D Time-Lapse Sequences of Single Motile Cells with Growing and Branching FilopodiaIEEE Transactions on Medical ImagingJune 2018, 1-12
- 13 articleInteractive training system for interventional electrocardiology proceduresMedical image analysis352017, 225--237
12.2 Publications of the year
International journals
- 14 article Editorial: Recurrence Analysis of Complex Systems Dynamics Frontiers in Applied Mathematics and Statistics 6 August 2020
- 15 articleHow Best Can Finite-Time Social Distancing Reduce Epidemic Final Size?Journal of Theoretical BiologyDecember 2020, 110557
- 16 article Three‐dimensional physics‐based registration of pelvic system using 2D dynamic magnetic resonance imaging slices Strain 56 3 June 2020
- 17 articleElastic registration based on compliance analysis and biomechanical graph matchingAnnals of Biomedical Engineering482020, 447–462
- 18 article Augmented Reality during Open Liver Surgery Using a Markerless Non-rigid Registration System Journal of Gastrointestinal Surgery January 2020
- 19 article Editorial: Stability: A Current Overview Frontiers in Applied Mathematics and Statistics 6 November 2020
- 20 article Predicted Microscopic Cortical Brain Images for Optimal Craniotomy Positioning and Visualization Computer Methods in Biomechanics and Biomedical Engineering: Imaging & Visualization October 2020
- 21 article Divergence of the Ensemble Transform Kalman Filter (LETKF) by nonlocal observations Frontiers in Applied Mathematics and Statistics 2020
- 22 article Alternating quarantine for sustainable epidemic mitigation Nature Communications 12 1 December 2021
- 23 articleEstimation of boundary conditions for patient-specific liver simulation during augmented surgeryInternational Journal of Computer Assisted Radiology and Surgery157May 2020, 1107-1115
- 24 article Neurostimulation stabilizes spiking neural networks by disrupting seizure-like oscillatory transitions Scientific Reports September 2020
- 25 article Assimilating visible and infrared radiances in idealized simulations of deep convection Monthly Weather Review 2020
- 26 article Constrained Stochastic State Estimation of Deformable 1D Objects: Application to Single-view 3D Reconstruction of Catheters with Radio-opaque Markers Computerized Medical Imaging and Graphics 81 February 2020
International peer-reviewed conferences
- 27 inproceedings Alignment of Cortical Vessels viewed through the Surgical Microscope with Preoperative Imaging to Compensate for Brain Shift Medical Imaging 2020: Image-Guided Procedures, Robotic Interventions, and Modeling Houston, United States February 2020
Conferences without proceedings
- 28 inproceedings Deformation Aware Augmented Reality for Craniotomy using 3D/2D Non-rigid Registration of Cortical Vessels Medical Image Computing and Computer Assisted Intervention Lima, Peru October 2020
- 29 inproceedings Use of stereo-laparoscopic liver surface reconstruction to compensate for pneumoperitoneum deformation through biomechanical modeling. VPH2020 - Virtual Physiological Human Paris, France August 2020
Scientific books
- 30 book Synergetics 2020
Scientific book chapters
- 31 inbookData-driven simulation for augmented surgery132Developments and Novel Approaches in Biomechanics and MetamaterialsJuly 2020, 71-96
Doctoral dissertations and habilitation theses
- 32 thesis Exploring new numerical methods for the simulation of soft tissue deformations in surgery assistance Université de Strasbourg November 2020
- 33 thesis Machine Learning meets real-time Numerical Simulation - Application to surgical training, preoperative planning and surgical assistance Université de Strasbourg December 2020
- 34 thesis Image-guided Interactive Simulation for Endovascular Surgery Université de Strasbourg October 2020
Reports & preprints
- 35 misc Optimal control strategies for the sterile mosquitoes technique 2020
- 36 misc Optimal Immunity Control and Final Size Minimization by Social Distancing for the SIR Epidemic Model January 2021
- 37 misc Optimization of spatial control strategies for population replacement, application to Wolbachia December 2020
- 38 misc Bilinear local controllability to the trajectories of the Fokker-Planck equation with a localized control November 2020
-
39
misc
A new
-FEM approach for problems with natural boundary conditions' March 2020 - 40 misc Arousal fluctuations govern oscillatory transitions between dominant gamma and alpha occipital activity during eyes open/closed conditions December 2020