Keywords
Computer Science and Digital Science
- A5.1.8. 3D User Interfaces
- A5.4. Computer vision
- A5.4.4. 3D and spatio-temporal reconstruction
- A5.4.5. Object tracking and motion analysis
- A5.5.1. Geometrical modeling
- A5.5.4. Animation
- A5.6. Virtual reality, augmented reality
- A6.2.8. Computational geometry and meshes
Other Research Topics and Application Domains
- B2.6.3. Biological Imaging
- B2.8. Sports, performance, motor skills
- B9.2.2. Cinema, Television
- B9.2.3. Video games
- B9.4. Sports
1 Team members, visitors, external collaborators
Research Scientists
- Edmond Boyer [Team leader, Inria, Senior Researcher, HDR]
- Stefanie Wuhrer [Inria, Researcher, HDR]
Faculty Members
- Jean-Sébastien Franco [Institut polytechnique de Grenoble, Associate Professor]
- Sergi Pujades Rocamora [Univ Grenoble Alpes, Associate Professor]
Post-Doctoral Fellows
- Joao Pedro Cova Regateiro [Univ Grenoble Alpes, from Apr 2020]
- Victoria Fernandez Abrevaya [Max Planck Institute, Tubingen, from Aug 2020]
PhD Students
- Matthieu Armando [Inria]
- Jean Basset [Inria]
- Nicolas Comte [ANATOSCOPE, from Apr 2020]
- Raphael Dang Nhu [Inria, from Oct 2020]
- Sanae Dariouche [Univ Grenoble Alpes]
- Victoria Fernandez Abrevaya [Inria, until Jul 2020]
- Roman Klokov [Inria, until Aug 2020]
- Mathieu Marsot [Inria]
- Di Meng [Inria]
- Abdullah Haroon Rasheed [Inria]
- Nitika Verma [Univ Grenoble Alpes]
- Boyao Zhou [Inria]
- Pierre Zins [Inria]
Technical Staff
- Laurence Boissieux [Inria, Engineer]
- Julien Pansiot [Inria, Engineer]
- Tomas Svaton [Inria, Engineer, until Sep 2020]
Interns and Apprentices
- Ilyass Hammouamri [Univ Grenoble Alpes, from Feb 2020 until Aug 2020]
- Eslam Mohammed [Inria, from Feb 2020 until Aug 2020]
- Ali Salman [Univ Grenoble Alpes, from Feb 2020 until Jun 2020]
Administrative Assistant
- Nathalie Gillot [Inria]
Visiting Scientist
- Stephane Durocher [Université de Manitoba, Canada, until Aug 2020]
2 Overall objectives
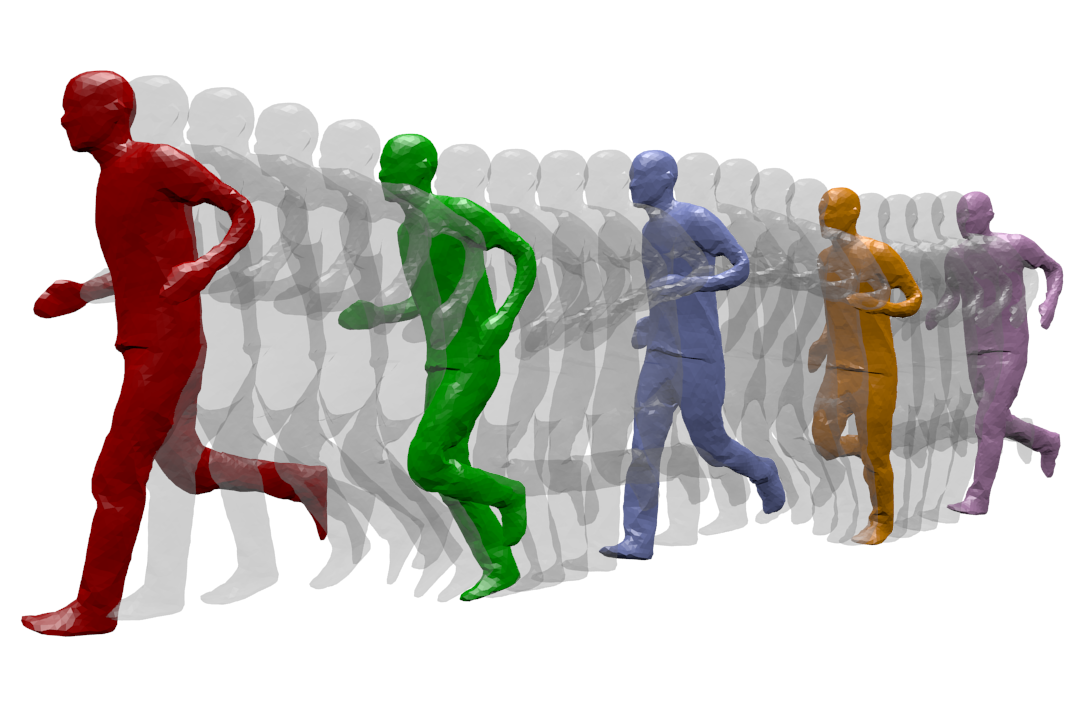
MORPHEO's ambition is to perceive and interpret shapes that move using multiple camera systems. Departing from standard motion capture systems, based on markers, that provide only sparse information on moving shapes, multiple camera systems allow dense information on both shapes and their motion to be recovered from visual cues. Such ability to perceive shapes in motion brings a rich domain for research investigations on how to model, understand and animate real dynamic shapes, and finds applications, for instance, in gait analysis, bio-metric and bio-mechanical analysis, animation, games and, more insistently in recent years, in the virtual and augmented reality domain. The MORPHEO team particularly focuses on three different axes within the overall theme of 3D dynamic scene vision or 4D vision:
- Shape and appearance models: how to build precise geometric and photometric models of shapes, including human bodies but not limited to, given temporal sequences.
- Dynamic shape vision: how to register and track moving shapes, build pose spaces and animate captured shapes.
- Inside shape vision: how to capture and model inside parts of moving shapes using combined color and X-ray imaging.
The strategy developed by MORPHEO to address the mentioned challenges is based on methodological tools that include in particular learning-based approaches, geometry, Bayesian inference and numerical optimization. The potential of machine learning tools in 4D vision is still to be fully investigated, and Morpheo contributes to exploring this direction.
3 Research program
3.1 Shape and Appearance Modeling
Standard acquisition platforms, including commercial solutions proposed by companies such as Microsoft, 3dMD or 4DViews, now give access to precise 3D models with geometry, e.g. meshes, and appearance information, e.g. textures. Still, state-of-the-art solutions are limited in many respects: They generally consider limited contexts and close setups with typically at most a few meter side lengths. As a result, many dynamic scenes, even a body running sequence, are still challenging situations; They also seldom exploit time redundancy; Additionally, data driven strategies are yet to be fully investigated in the field. The MORPHEO team builds on the Kinovis platform for data acquisition and has addressed these issues with, in particular, contributions on time integration, in order to increase the resolution for both shapes and appearances, on representations, as well as on exploiting machine learning tools when modeling dynamic scenes. Our originality lies, for a large part, in the larger scale of the dynamic scenes we consider as well as in the time super resolution strategy we investigate. Another particularity of our research is a strong experimental foundation with the multiple camera Kinovis platforms.
3.2 Dynamic Shape Vision
Dynamic Shape Vision refers to research themes that consider the motion of dynamic shapes, with e.g. shapes in different poses, or the deformation between different shapes, with e.g. different human bodies. This includes for instance shape tracking and shape registration, which are themes covered by MORPHEO. While progress has been made over the last decade in this domain, challenges remain, in particular due to the required essential task of shape correspondence that is still difficult to perform robustly. Strategies in this domain can be roughly classified into two categories: (i) data driven approaches that learn shape spaces and estimate shapes and their variations through space parameterizations; (ii) model based approaches that use more or less constrained prior models on shape evolutions, e.g. locally rigid structures, to recover correspondences. The MORPHEO team is substantially involved in both categories. The second one leaves more flexibility for shapes that can be modeled, an important feature with the Kinovis platform, while the first one is interesting for modeling classes of shapes that are more likely to evolve in spaces with reasonable dimensions, such as faces and bodies under clothing. The originality of MORPHEO in this axis is to go beyond static shape poses and to consider also the dynamics of shape over several frames when modeling moving shapes, and in particular with shape tracking, animation and, more recently, face registration.
3.3 Inside Shape Vision
Another research axis is concerned with the ability to perceive inside moving shapes. This is a more recent research theme in the MORPHEO team that has gained importance. It was originally the research associated to the Kinovis platform installed in the Grenoble Hospitals. This platform is equipped with two X-ray cameras and ten color cameras, enabling therefore simultaneous vision of inside and outside shapes. We believe this opens a new domain of investigation at the interface between computer vision and medical imaging. Interesting issues in this domain include the links between the outside surface of a shape and its inner parts, especially with the human body. These links are likely to help understanding and modeling human motions. Until now, numerous dynamic shape models, especially in the computer graphic domain, consist of a surface, typically a mesh, bound to a skeletal structure that is never observed in practice but that help anyway parameterizing human motion. Learning more accurate relationships using observations can therefore significantly impact the domain.
3.4 Shape Animation
3D animation is a crucial part of digital media production with numerous applications, in particular in the game and motion picture industry. Recent evolutions in computer animation consider real videos for both the creation and the animation of characters. The advantage of this strategy is twofold: it reduces the creation cost and increases realism by considering only real data. Furthermore, it allows to create new motions, for real characters, by recombining recorded elementary movements. In addition to enable new media contents to be produced, it also allows to automatically extend moving shape datasets with fully controllable new motions. This ability appears to be of great importance with the recent advent of deep learning techniques and the associated need for large learning datasets. In this research direction, we investigate how to create new dynamic scenes using recorded events. More recently, this also includes applying machine learning to datasets of recorded human motions to learn motion spaces that allow to synthesize novel realistic animations.
4 Application domains
4.1 4D modeling
Modeling shapes that evolve over time, analyzing and interpreting their motion has been a subject of increasing interest of many research communities including the computer vision, the computer graphics and the medical imaging communities. Recent evolutions in acquisition technologies including 3D depth cameras (Time-of-Flight and Kinect), multi-camera systems, marker based motion capture systems, ultrasound and CT scanners have made those communities consider capturing the real scene and their dynamics, create 4D spatio-temporal models, analyze and interpret them. A number of applications including dense motion capture, dynamic shape modeling and animation, temporally consistent 3D reconstruction, motion analysis and interpretation have therefore emerged.
4.2 Shape Analysis
Most existing shape analysis tools are local, in the sense that they give local insight about an object's geometry or purpose. The use of both geometry and motion cues makes it possible to recover more global information, in order to get extensive knowledge about a shape. For instance, motion can help to decompose a 3D model of a character into semantically significant parts, such as legs, arms, torso and head. Possible applications of such high-level shape understanding include accurate feature computation, comparison between models to detect defects or medical pathologies, and the design of new biometric models.
4.3 Human Motion Analysis
The recovery of dense motion information enables the combined analysis of shapes and their motions. Typical examples include the estimation of mean shapes given a set of 3D models or the identification of abnormal deformations of a shape given its typical evolutions. The interest arises in several application domains where temporal surface deformations need to be captured and analyzed. It includes human body analyses for which potential applications are anyway numerous and important, from the identification of pathologies to the design of new prostheses.
4.4 Virtual and Augmented Reality
This domain has actually seen new devices emerge that enable now full 3D visualization, for instance the HTC Vive, the Microsoft Hololens and the Magic leap one. These devices create a need for adapted animated 3D contents that can either be generated or captured. We believe that captured 4D models will gain interest in this context since they provide realistic visual information on moving shapes that tend to avoid negative perception effects such as the uncanny valley effect. Furthermore, captured 4D models can be exploited to learn motion models that allow for realistic generation, for instance in the important applicative case of generating realistically moving human avatars. Besides 3D visualization devices, many recent applications also rely on everyday devices, such as mobile phones, to display augmented reality contents with free viewpoint ability. In this case, 3D and 4D contents are also expected.
5 Social and environmental responsibility
5.1 Footprint of research activities
We currently have no precise measurements of the environmental footprint related to Morpheo's research activities, also due to a lack of tools to conduct such measurements. However, we believe that our dissemination strategy and our data management have the highest impacts.
Dissemination strategy: Traditionally, Morpheo's dissemination strategy has been to publish in the top conferences of the field (CVPR, ECCV, ICCV, MICCAI), leading to long-distance work trips to attend these conferences and present our works, which is not sustainable. As a result of the Covid-19 crisis, our research community has started in 2020 to experiment with virtual conference systems. These digital meetings have some merits: beyond the obvious advantage of reduced travel (leading to fewer emissions and improved work-life balance), they democratize attendance as attending has a fraction of the previous cost. However, the major drawback is that digital attendance leads to significantly lower and lower-quality exchange between participants as “off-line” discussions are impossible through these systems and “on-line” ones often partially through written chat or disrupted by technical difficulties. In the future, we will consider more often to publish works in the top journals of the field directly to reduce travel, while still publishing and attending some in-person conferences or seminars to allow for networking.
Data management: The data on which Morpheo's research is based is large. The Kinovis platform at Inria Grenoble produces around 1,5GB of data when capturing one actor for one second at 50fps (using masked images). The platform that also captures X-ray images at CHU produces around 1.3GB of data per second at 30fps for video and X-rays (without masking the images). For practical reasons, we reduce the data as much as possible for the targeted application by only keeping e.g. 3D reconstructions or down-sampled spatial or temporal camera images. Yet, acquiring, processing and storing these data is costly in terms of resources. In addition, the data captured by these platforms are personal data and highly regulated. Our data management therefore needs to consider multiple aspects: data encryption to protect personal information, data backup to allow for reproducibility, and the environmental impact of data storage and processing. For all these aspects, we are constantly looking for more satisfactory solutions, which seem to be hard to find in our case.
For data processing, we rely heavily on cluster use, and since a few years on neural networks, which are known to have a heavy carbon footprint. Yet, in our research field, these types of processing methods have been shown to lead to significant performance gains. For this reason, we continue to use neural networks as tools, while attempting to use architectures that allow for faster and more energy efficient training, and simple test cases that can often be trained on local machines (with GPU) to allow catching (hopefully most) bugs without having to train the full network on the cluster.
5.2 Impact of research results
Morpheo's main research topic is not related to sustainability. Yet, some of our research directions could be applied to solve problems relevant to sustainability such as:
- Realistic virtual avatar creation holds the potential to allow for realistic interactions through large geographic distances, leading to more realistic and satisfactory teleconferencing systems. As has been observed during the Covid-19 crisis, such systems can remove the need for traveling, thereby preventing costs and pollution and preserving resources. If avatars were to allow for more realistic interactions than existing teleconferencing systems, there is hope that the need to travel can be reduced even in times without health emergency. Morpheo captures and analyzes humans in 4D, thereby allowing to capture both their shape and movement patterns, and actively works on human body and body part (in particular face) modeling. These research directions tackle problems that are highly relevant for the creation of digital avatars that do not fall into the uncanny valley (even during movement). In this line of work, Morpheo currently participates in the IPL Avatar project, in particular the works of Ph.D. student Jean Basset.
- Modeling and analyzing humans in clothing holds potential to reduce the rate of returns of online sales in the clothing industry, which are currently high. In particular, highly realistic dynamic clothing models could potentially help a consumer to better evaluate the fit and dynamic aspects of the clothing prior to acquisition. Morpheo's interest includes the analysis of clothing deformations based on 4D captures. Preliminary results have been achieved in the past (works of former Ph.D. student Jinlong Yang), and we plan to continue investigating this line of work. To this end, our plans for future work include the capture a large-scale dataset of humans in different clothing items performing different motions in collaboration with NaverLabs Research. No such dataset exists to date, and it would allow the research community to study the dynamic effects of clothing.
Of course, as with any research direction, ours can also be used to generate technologies that are resource-hungry and whose necessity may be questionable, such as inserting animated 3D avatars in scenarios where simple voice communication would suffice, for instance.
6 New software and platforms
6.1 New software
6.1.1 Lucy Viewer
- Keywords: Data visualization, 4D, Multi-Cameras
-
Scientific Description:
Lucy Viewer is an interactive viewing software for 4D models, i.e, dynamic three-dimensional scenes that evolve over time. Each 4D model is a sequence of meshes with associated texture information, in terms of images captured from multiple cameras at each frame. Such data is available from the 4D repository website hosted by INRIA Grenoble.
With Lucy Viewer, the user can use the mouse to zoom in onto the 4D models, zoom out, rotate, translate and view from an arbitrary angle as the 4D sequence is being played. The texture information is read from the images at each frame in the sequence and applied onto the meshes. This helps the user visualize the 3D scene in a realistic manner. The user can also freeze the motion at a particular frame and inspect a mesh in detail. Lucy Viewer lets the user to also select a subset of cameras from which to apply texture information onto the meshes. The supported formats are meshes in .OFF format and associated images in .PNG or .JPG format.
- Functional Description: Lucy Viewer is an interactive viewing software for 4D models, i.e, dynamic three-dimensional scenes that evolve over time. Each 4D model is a sequence of meshes with associated texture information, in terms of images captured from multiple cameras at each frame.
-
URL:
https://
kinovis. inria. fr/ lucyviewer/ - Authors: Edmond Boyer, Matthieu Armando, Jean-Sébastien Franco, Thomas Pasquier, Eymeric Amselem
- Contacts: Edmond Boyer, Eymeric Amselem
- Participants: Edmond Boyer, Jean-Sébastien Franco, Matthieu Armando, Eymeric Amselem
6.1.2 Shape Tracking
- Functional Description: We are developing a software suite to track shapes over temporal sequences. The motivation is to provide temporally coherent 4D Models, i.e. 3D models and their evolutions over time , as required by motion related applications such as motion analysis. This software takes as input a temporal sequence of 3D models in addition to a template and estimate the template deformations over the sequence that fit the observed 3D models.
- Contact: Edmond Boyer
6.1.3 QuickCSG V2
- Keywords: 3D modeling, CAD, 3D reconstruction, Geometric algorithms
-
Scientific Description:
See the technical report "QuickCSG: Arbitrary and Faster Boolean Combinations of N Solids", Douze, Franco, Raffin.
The extension of the algorithm to self-intersecting meshes is described in "QuickCSG with self-intersections", a document inside the package.
- Functional Description: QuickCSG is a library and command-line application that computes Boolean operations between polyhedra. The basic algorithm is described in the research report "QuickCSG: Arbitrary and Faster Boolean Combinations of N Solids", Douze, Franco, Raffin. The input and output polyhedra are defined as indexed meshes. In version 2, that was developed in the context of a software transfer contract, the meshes can be self-intersecting, in which case the inside and outside are defined by the non-zero winding rule. The operation can be any arbitrary Boolean function, including one that is defined as a CSG tree. The focus of QuickCSG is speed. Robustness to degeneracies is obtained by carefully applied random perturbations.
-
URL:
https://
kinovis. inria. fr/ quickcsg/ - Authors: Matthys Douze, Jean-Sébastien Franco, Bruno Raffin
- Contacts: Matthys Douze, Jean-Sébastien Franco, Bruno Raffin
6.1.4 CVTGenerator
- Name: CVTGenerator
- Keywords: Mesh, Centroidal Voronoi tessellation, Implicit surface
- Functional Description: CVTGenerator is a program to build Centroidal Voronoi Tessellations of any 3D meshes and implicit surfaces.
-
URL:
http://
cvt. gforge. inria. fr/ - Contacts: Franck Hétroy, Edmond Boyer, Li WANG
- Partner: INP Grenoble
6.1.5 Adaptive mesh texture
- Keywords: 3D, Geometry Processing, Texturing
- Functional Description: Tool for computing appearance information on a 3D scene acquired with a multi-view stereo (MVS) pipeline. Appearance information is sampled in an adaptive way so as to maximize the entropy of stored information. This is made possible through a homemade representation of appearance, different from the more traditional texture maps. This tool laso includes a compression module, so as to optimize disk space.
- Release Contributions: 1st version
-
URL:
https://
gitlab. inria. fr/ marmando/ adaptive-mesh-texture - Authors: Matthieu Armando, Edmond Bover, Jean-Sébastien Franco, Vincent Leroy
- Contact: Matthieu Armando
- Partner: Microsoft
7 New results
7.1 Volume Sweeping: Learning Photoconsistency for Multi-View Shape Reconstruction
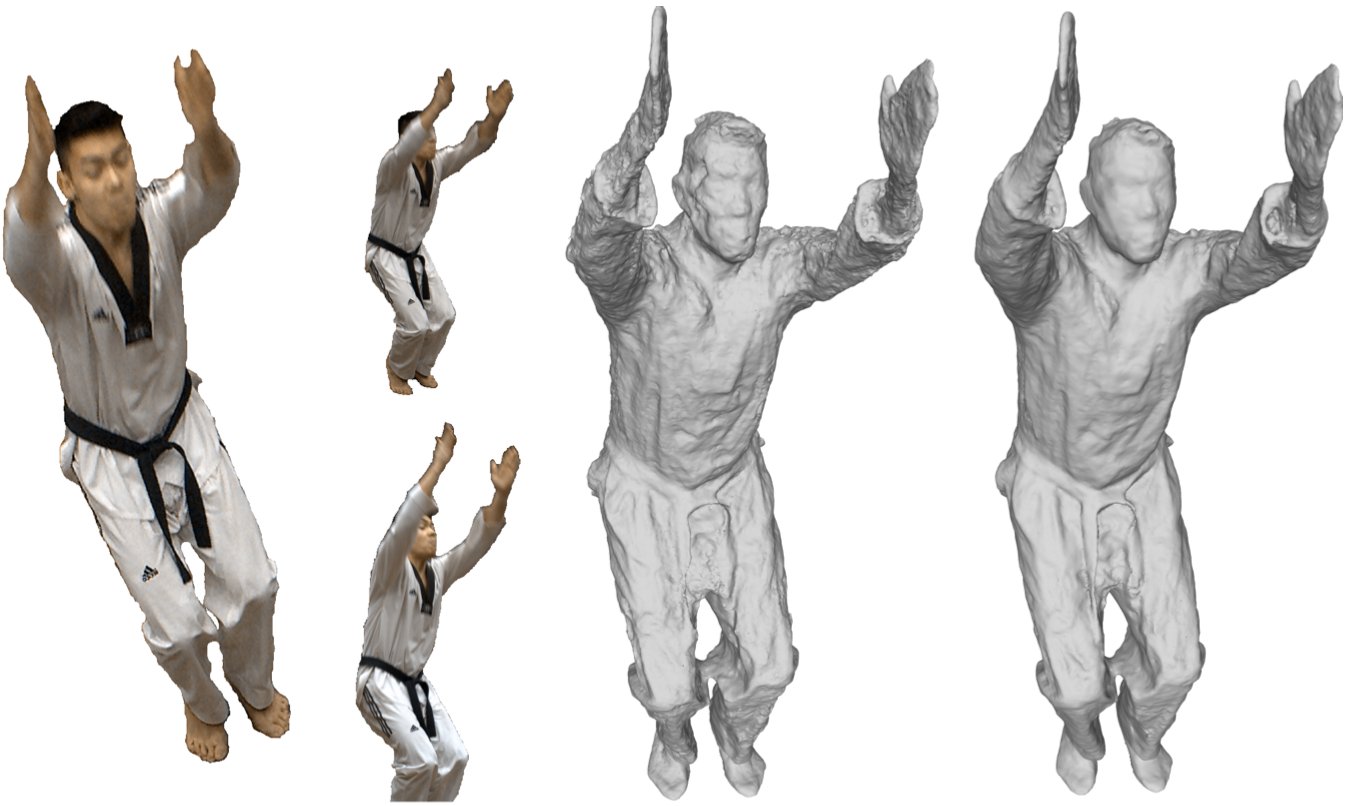
We propose a full study and methodology for multi-view stereo reconstruction with performance capture data. Multi-view 3D reconstruction has largely been studied with general, high resolution and high texture content inputs, where classic low-level feature extraction and matching are generally successful. However in performance capture scenarios, texture content is limited by wider angle shots resulting in smaller subject projection areas, and intrinsically low image content of casual clothing. We present a dedicated pipeline, based on a per-camera depth map sweeping strategy, analyzing in particular how recent deep network advances allow to replace classic multi-view photoconsistency functions with one that is learned. We show that learning based on a volumetric receptive field around a 3D depth candidate improves over using per-view 2D windows, giving the photoconsistency inference more visibility over local 3D correlations in viewpoint color aggregation. Despite being trained on a standard dataset of scanned static objects, the proposed method is shown to generalize and significantly outperform existing approaches on performance capture data, while achieving competitive results on recent benchmarks.
This work was published at International Journal of Computer Vision, one of the top journals in computer vision 9.
7.2 Mesh Denoising with Facet Graph Convolutions
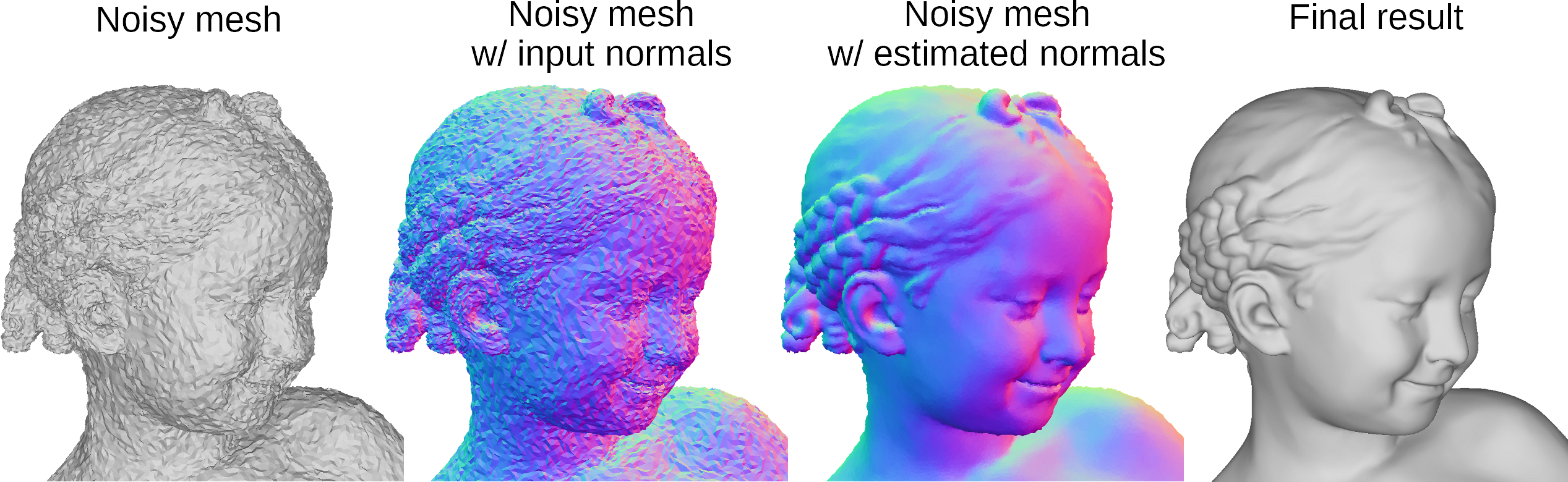
We examine the problem of mesh denoising, which consists of removing noise from corrupted 3D meshes while preserving existing geometric features. Most mesh denoising methods require a lot of mesh-specific parameter fine-tuning, to account for specific features and noise types. In recent years, data-driven methods have demonstrated their robustness and effectiveness with respect to noise and feature properties on a wide variety of geometry and image problems. Most existing mesh denoising methods still use hand-crafted features, and locally denoise facets rather than examine the mesh globally. In this work, we propose the use of a fully end-to-end learning strategy based on graph convolutions, where meaningful features are learned directly by our network. It operates on a graph of facets, directly on the existing topology of the mesh, without resampling, and follows a multi-scale design to extract geometric features at different resolution levels. Similar to most recent pipelines, given a noisy mesh, we first denoise face normals with our novel approach, then update vertex positions accordingly. Our method performs significantly better than the current state-of-the-art learning-based methods. Additionally, we show that it can be trained on noisy data, without explicit correspondence between noisy and ground-truth facets. We also propose a multi-scale denoising strategy, better suited to correct noise with a low spatial frequency.
This result was published in a prominent computer graphics journal, IEEE Transactions on Visualization and Computer Graphics 5.
7.3 Learning and Tracking the 3D Body Shape of Freely Moving Infants from RGB-D sequences for early diagnosis of motor development disorders.
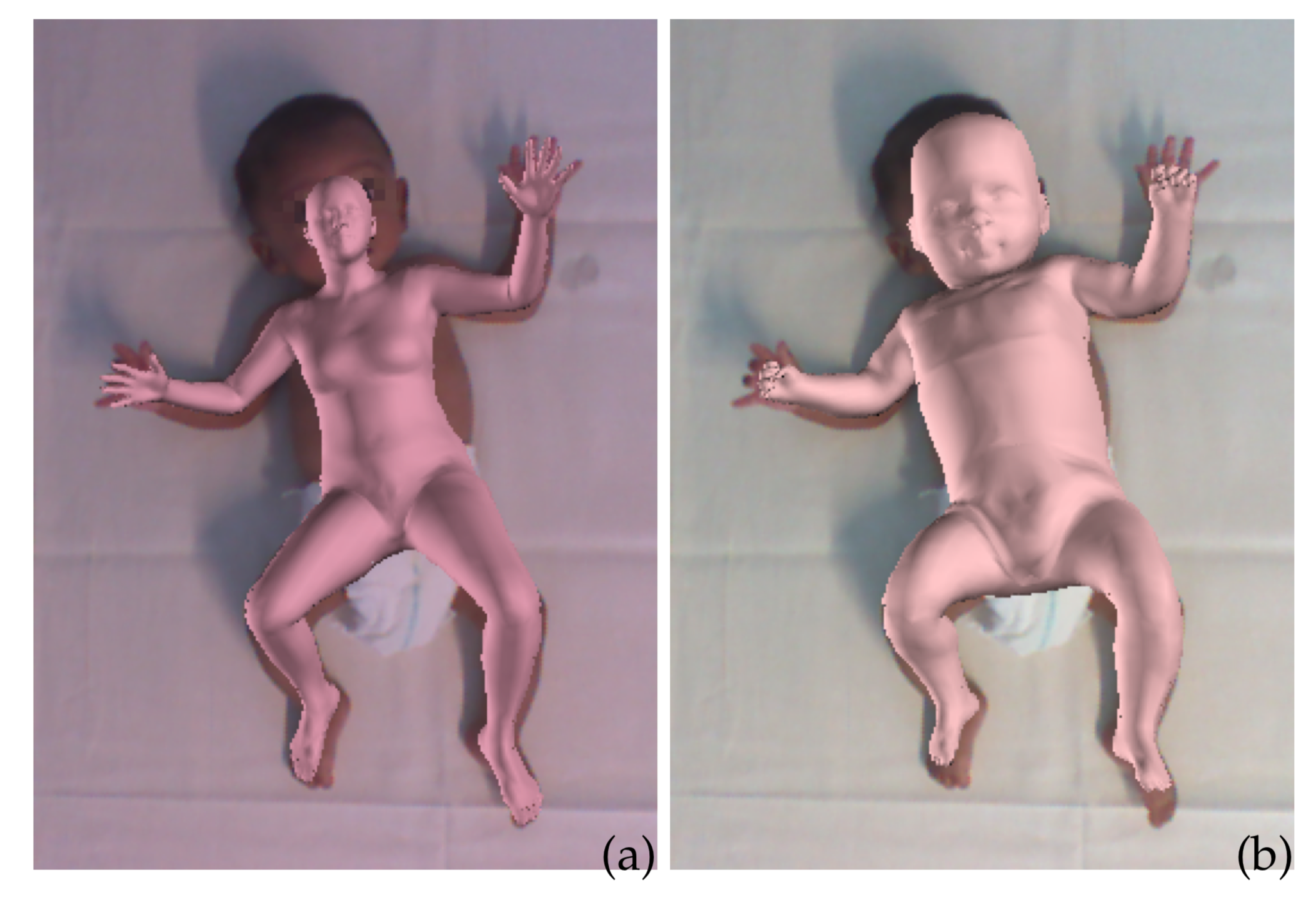
Children with motor development disorders benefit greatly from early interventions. An early diagnosis in pediatric preventive care can be improved by automated screening. Current approaches to automated motion analysis, however, are expensive,require lots of technical support, and cannot be used in broad clinical application.
In these three works we developped steps towards a fully automatic screening method. In the first one an infant statistical body model was learned from RGB-D data.
Statistical models of the human body surface are generally learned from thousands of high-quality 3D scans in predefined poses to cover the wide variety of human body shapes and articulations. Acquisition of such data requires expensive equipment, calibration procedures, and is limited to cooperative subjects who can understand and follow instructions, such as adults. We present a method for learning a statistical 3D Skinned Multi-Infant Linear body model (SMIL) from incomplete, low-quality RGB-D sequences of freely moving infants. Quantitative experiments show that SMIL faithfully represents the RGB-D data and properly factorizes the shape and pose of the infants. To demonstrate the applicability of SMIL, we fit the model to RGB-D sequences of freely moving infants and show, with a case study, that our method captures enough motion detail for General Movements Assessment (GMA), a method used in clinical practice for early detection of neurodevelopmental disorders in infants. SMIL provides a new tool for analyzing infant shape and movement and is a step towards an automated system for GMA.
This result was published in a prominent computer vision journal, IEEE Transactions on Pattern Analysis and Machine Intelligence 8.
Based on this model, we could further quantify that the model was able to capture the essential information for the diagnosis by rendering new videos from the model parameters by asking expert raters to diagnose from these only. Results were published at the clinical international journal Early human development 11 .
The third publication provides a first step towards the automatic diagnosis, namely KineMAT. A selection of possible movement parameters was quantified and aligned with diagnosis-specific movement characteristics. KineMAT and the SMIL model allow reliable, three-dimensional measurements of sponta-neous activity in infants with a very low errorrate. Based on machine-learning algorithms,KineMAT can be trained to automatically recognize pathological spontaneous motorskills. It is inexpensive and easy to use and can be developed into a screening tool for preventive care for children. The tool was published at a national Bundesgesundheitsblatt-Gesundheitsforschung-Gesundheitsschutz german journal 10.
7.4 Contact preserving shape transfer: Retargeting motion from one shape to another
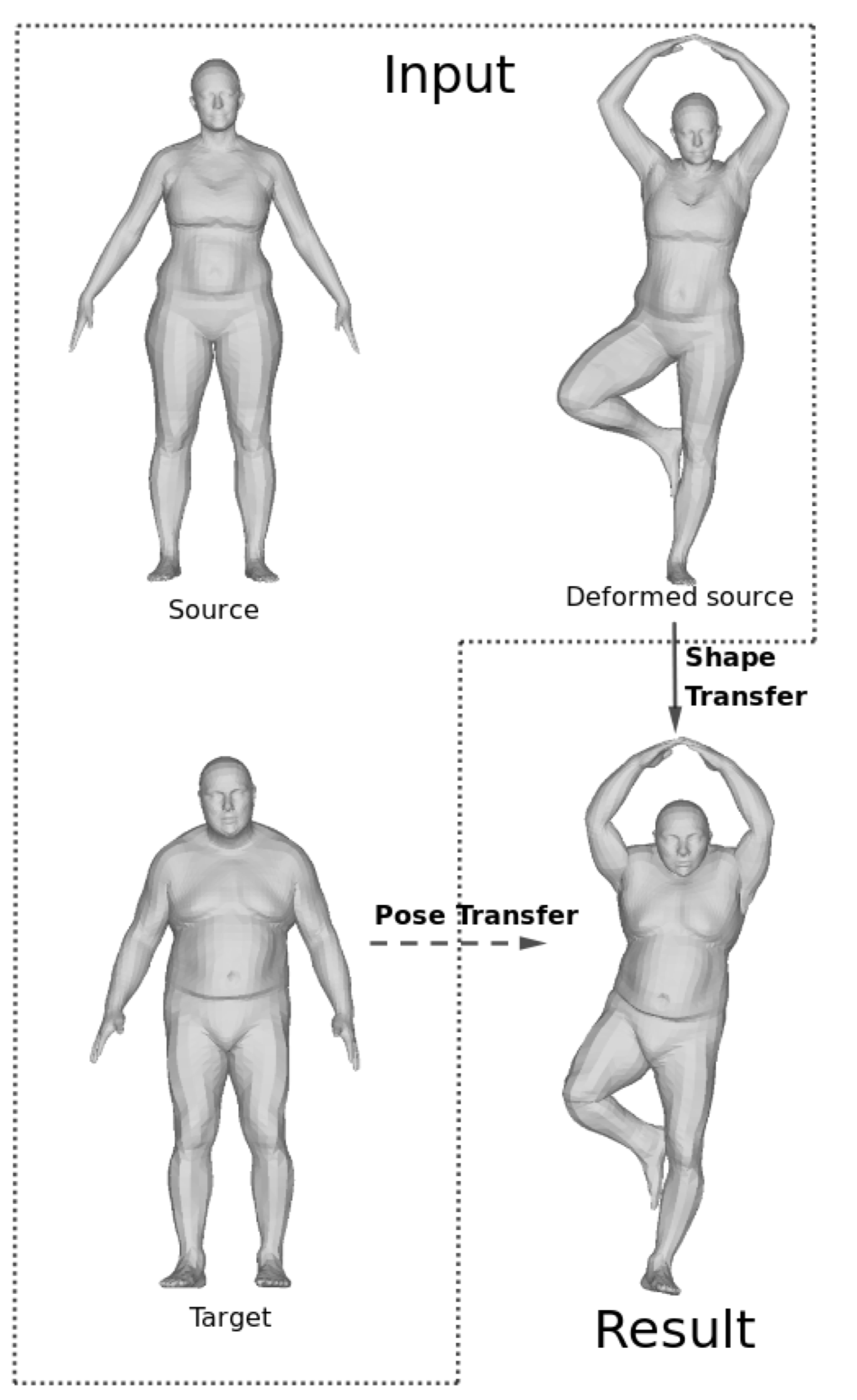
We present an automatic method that allows to retarget poses from a source to a target character by transferring the shape of the target character onto the desired pose of the source character. By considering shape instead of pose transfer our method allows to better preserve the contextual meaning of the source pose, typically contacts between body parts, than pose-based strategies. To this end, we propose an optimization-based method to deform the source shape in the desired pose using three main energy functions: similarity to the target shape, body part volume preservation, and collision management to preserve existing contacts and prevent penetrations. The results show that our method allows to retarget complex poses with several contacts to different morphologies , and is even able to create new contacts when morphology changes require them, such as increases in body size. To demonstrate the robustness of our approach to different types of shapes, we successfully apply it to basic and dressed human characters as well as wild animal models, without the need to adjust parameters.
This result was published in the journal Computers and Graphics 6.
7.5 Learning to Dress 3D People in Generative Clothing
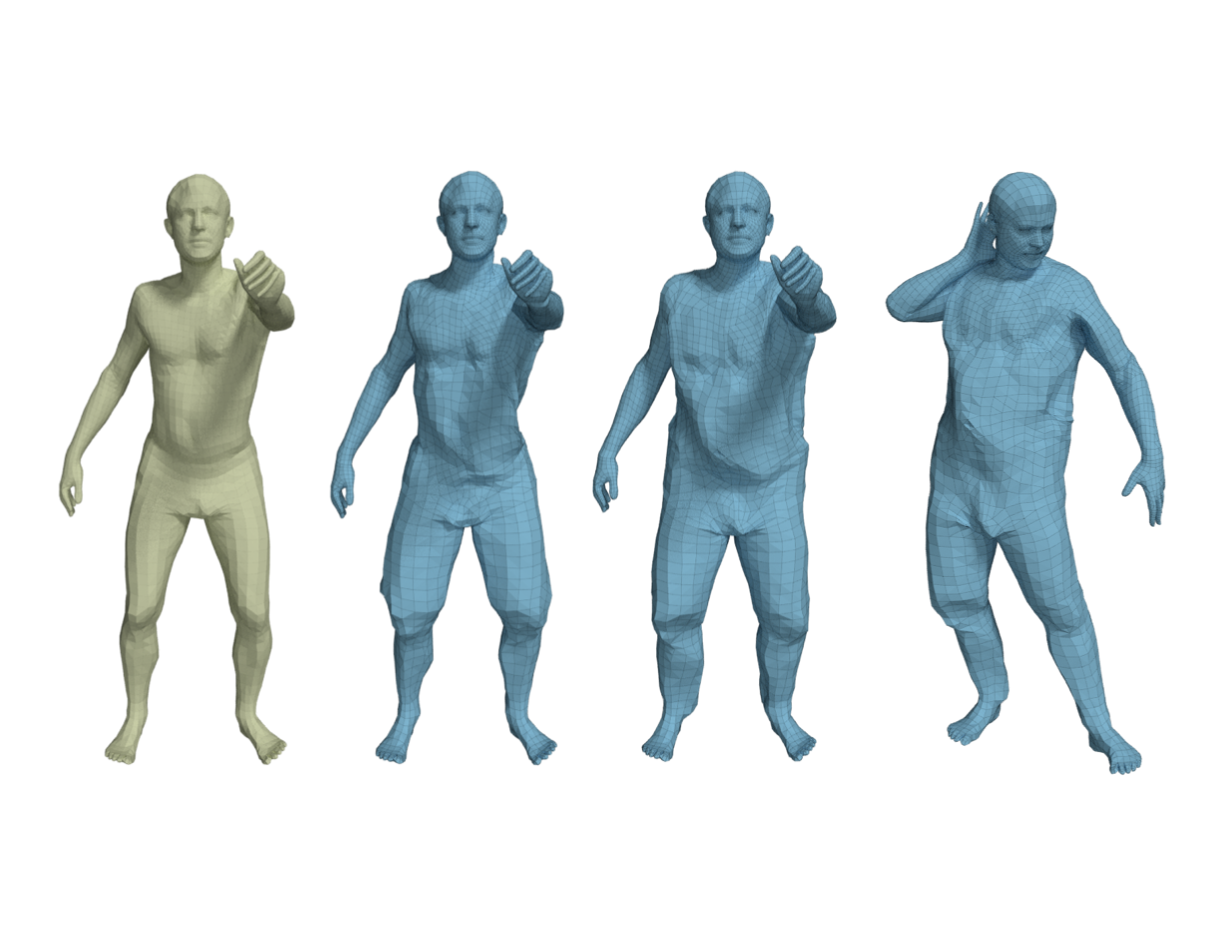
Three-dimensional human body models are widely usedin the analysis of human pose and motion. Existing models, however, are learned from minimally-clothed 3D scansand thus do not generalize to the complexity of dressed people in common images and videos. Additionally, current models lack the expressive power needed to represent the complex non-linear geometry of pose-dependent clothing shapes. To address this, we learn a generative 3D meshmodel of clothed people from 3D scans with varying poseand clothing. Specifically, we train a conditional Mesh-VAE-GAN to learn the clothing deformation from the SMPL body model, making clothing an additional term in SMPL. Our model is conditioned on both pose and clothing type, giving the ability to draw samples of clothing to dress different body shapes in a variety of styles and poses. To preserve wrinkle detail, our Mesh-VAE-GAN extends patchwise discriminators to 3D meshes. Our model, named CAPE, represents global shape and fine local structure, effectively extending the SMPL body model to clothing. To our knowledge, this is the first generative model that directly dresses 3D human body meshes and generalizes to different poses.
This work was published at Conference on Computer Vision and Pattern Recognition, one of the top computer vision conferences 14.
7.6 Learning to Measure the Static Friction Coefficient in Cloth Contact
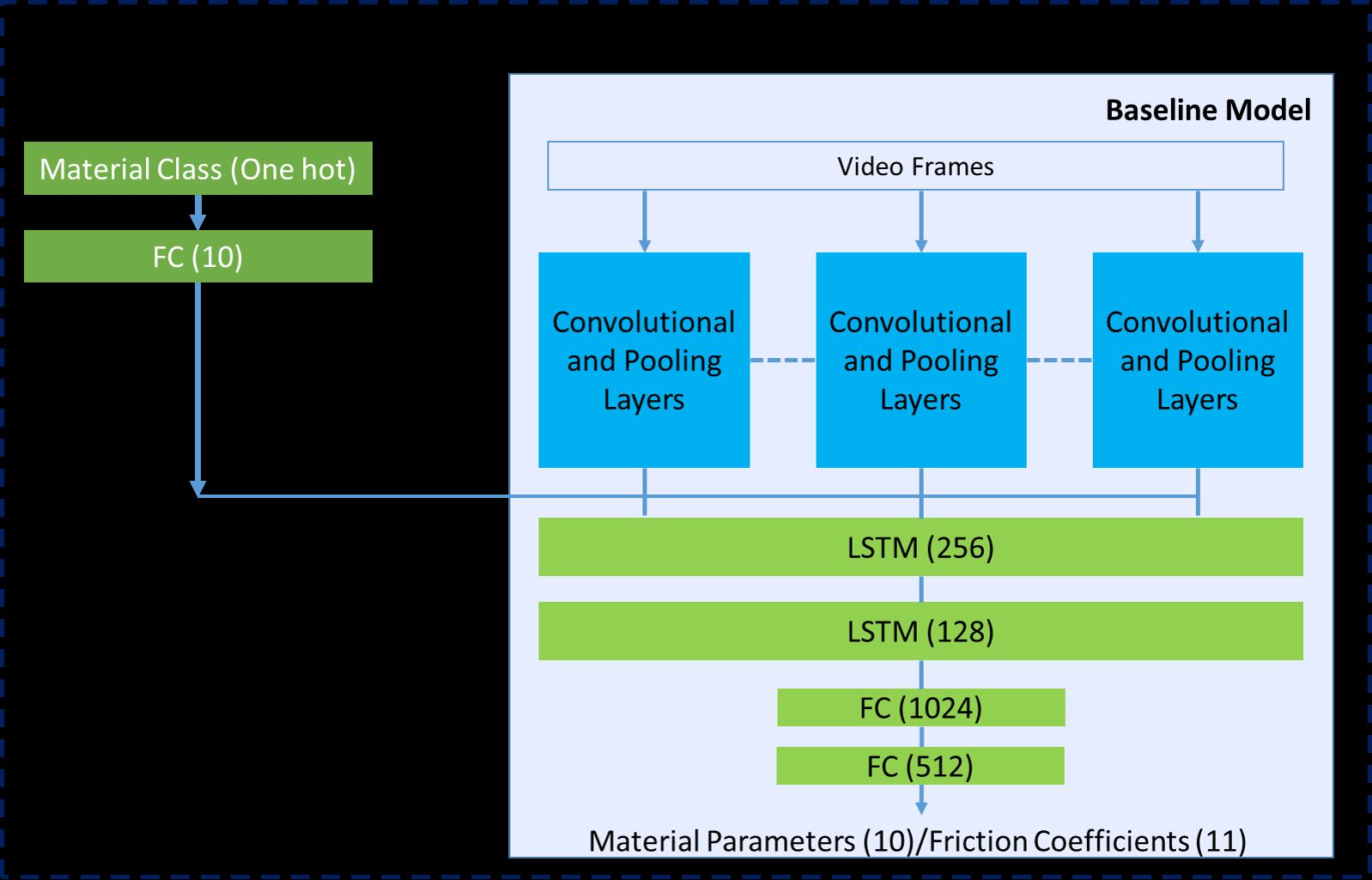
Measuring friction coefficients between cloth and an external body is a longstanding issue in mechanical engineering, never yet addressed with a pure vision-based system. The latter offers the prospect of simpler, less invasive friction measurement protocols compared to traditional ones,and can vastly benefit from recent deep learning advances. Such a novel measurement strategy however proves challenging, as no large labelled dataset for cloth contact exists, and creating one would require thousands of physics work-bench measurements with broad coverage of cloth-material pairs. Using synthetic data instead is only possible assuming the availability of a soft-body mechanical simulator with true-to-life friction physics accuracy, yet to be verified. We propose a first vision-based measurement network for friction between cloth and a substrate, using a simple and repeatable video acquisition protocol, shown in Figure 7. We train our network on purely synthetic data generated by a state-of-the-art frictional contact simulator, which we carefully calibrate and validate against real experiments under controlled conditions. We show promising results on a large set of contact pairs between real cloth samples and various kinds of substrates, with
This work was published as an oral (<5% acceptance) at Conference on Computer Vision and Pattern Recognition, one of the top computer vision conferences 15.
7.7 Cross-modal Deep Face Normals with Deactivable Skip Connections
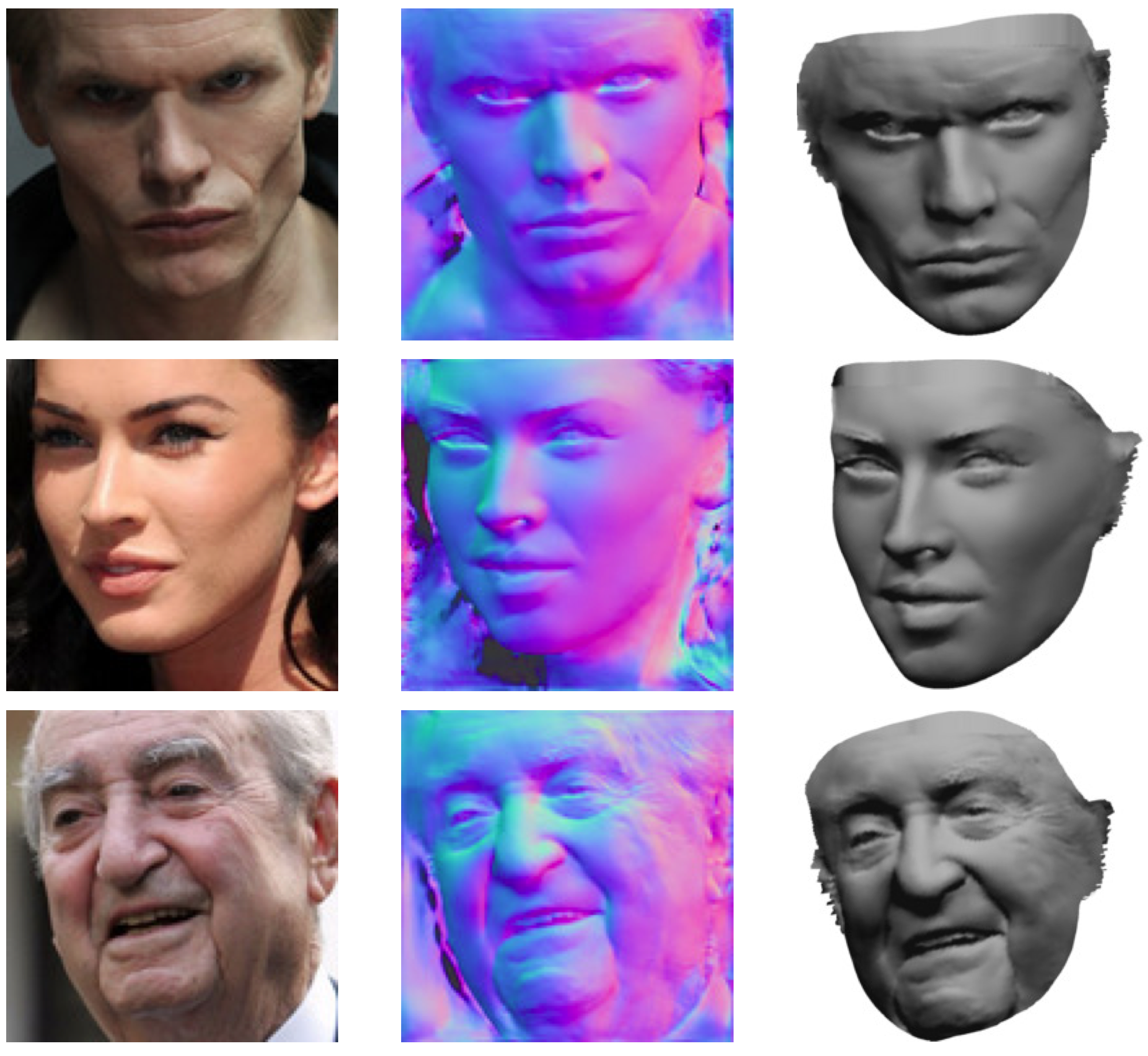
We present an approach for estimating surface normals from in-the-wild color images of faces. While datadriven strategies have been proposed for single face images, limited available ground truth data makes this problem difficult. To alleviate this issue, we propose a method that can leverage all available image and normal data, whether paired or not, thanks to a novel cross-modal learning architecture. In particular, we enable additional training with single modality data, either color or normal, by using two encoder-decoder networks with a shared latent space. The proposed architecture also enables face details to be transferred between the image and normal domains, given paired data, through skip connections between the image encoder and normal decoder. Core to our approach is a novel module that we call deactivable skip connections, which allows integrating both the auto-encoded and imageto-normal branches within the same architecture that can be trained end-to-end. This allows learning of a rich latent space that can accurately capture the normal information. We compare against state-of-the-art methods and show that our approach can achieve significant improvements, both quantitative and qualitative, with natural face images.
This work was published at Conference on Computer Vision and Pattern Recognition, one of the top computer vision conferences 12.
7.8 GENTEL : GENerating Training data Efficiently for Learning to segment medical images
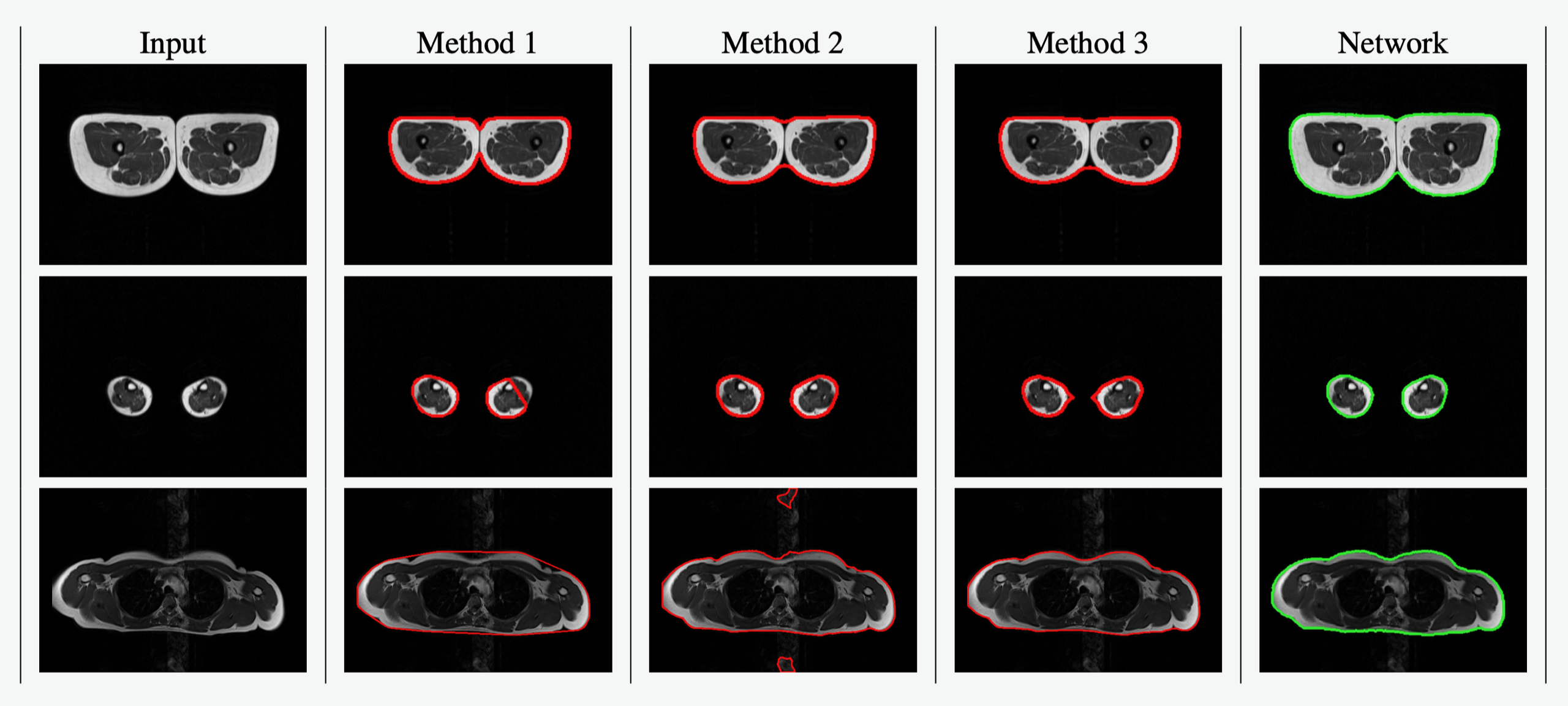
Accurately segmenting MRI images is crucial for many clinical applications. However, manually segmenting images with accurate pixel precision is a tedious and time consuming task. In this paper we present a simple, yet effective method to improve the efficiency of the image segmentation process. We propose to transform the image annotation task into a binary choice task. We start by using classical image processing algorithms with different parameter values to generate multiple, different segmentation masks for each input MRI image. Then, the user, instead of segmenting the pixels of the images, she/he only needs to decide if a segmentation is acceptable or not. This method allows us to efficiently obtain high quality segmentations with minor human intervention. With the selected segmentations we train a state-of-the-art neural network model. For the evaluation, we use a second MRI dataset (1.5T Dataset), acquired with a different protocol and containing annotations. We show that the trained network i) is capable to automatically segment cases where none of the classical methods obtained a high quality result ii) generalizes to the second MRI dataset, which was acquired with a different protocol and never seen at training time ; and iii) allows to detect miss-annotations in this second dataset. Quantitatively, the trained network obtains very good results : DICE score - mean 0.98, median 0.99- and Hausdorff distance (in pixels) - mean 4.7, median 2.0-.
This work was published at Congrès Reconnaissance des Formes, Image, Apprentissage et Perception 16.
7.9 Discrete Point Flow Networks for Efficient Point Cloud Generation
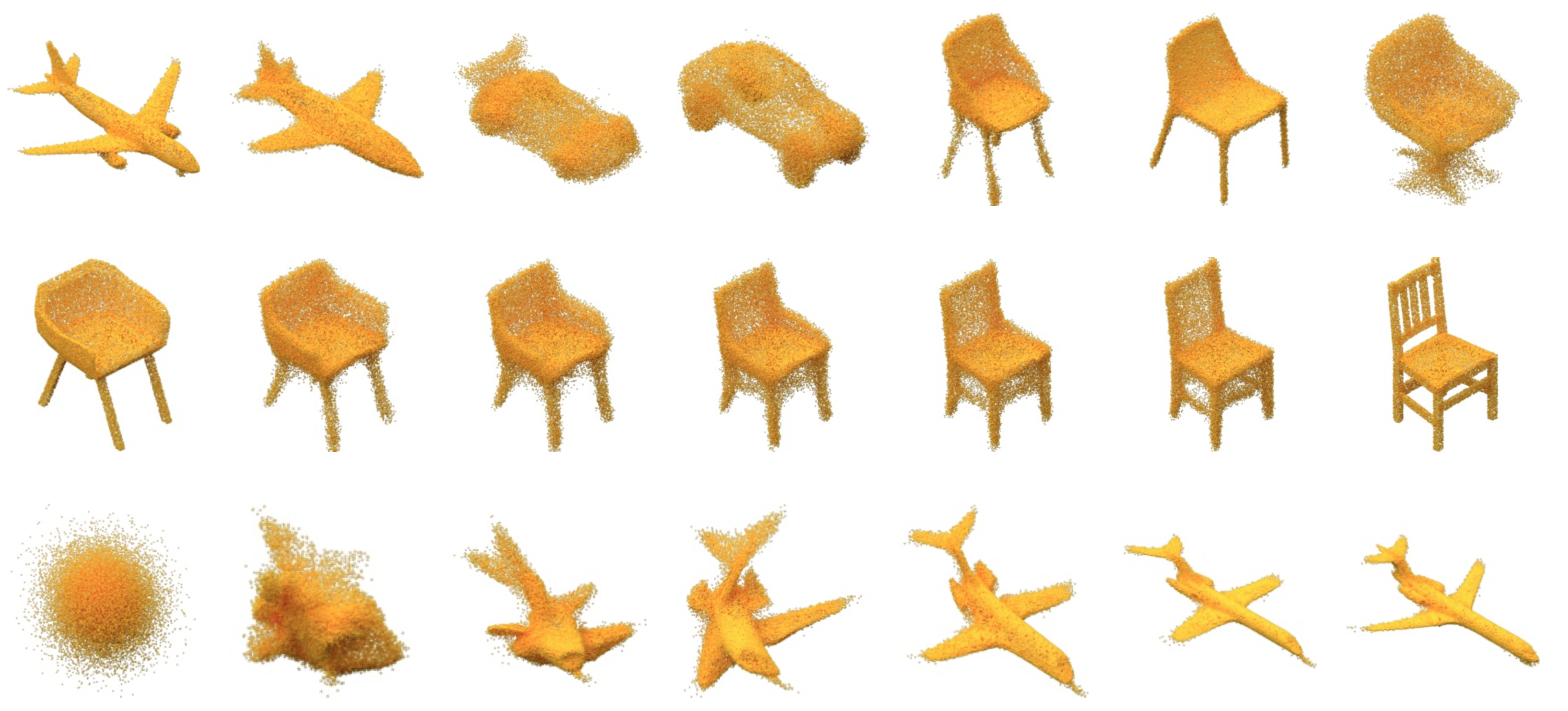
Generative models have proven effective at modeling 3D shapes and their statistical variations. In this paper we investigate their application to point clouds, a 3D shape representation widely used in computer vision for which, however, only few generative models have yet been proposed. We introduce a latent variable model that builds on normalizing flows with affine coupling layers to generate 3D point clouds of an arbitrary size given a latent shape representation. To evaluate its benefits for shape modeling we apply this model for generation, autoencoding, and single-view shape reconstruction tasks. We improve over recent GAN-based models in terms of most metrics that assess generation and autoencoding. Compared to recent work based on continuous flows, our model offers a significant speedup in both training and inference times for similar or better performance. For single-view shape reconstruction we also obtain results on par with state-of-the-art voxel, point cloud, and mesh-based methods.
This work was published at European Conference on Computer Vision, one of the top computer vision conferences 13.
7.10 Reconstructing Human Body Mesh from Point Clouds by Adversarial GP Network
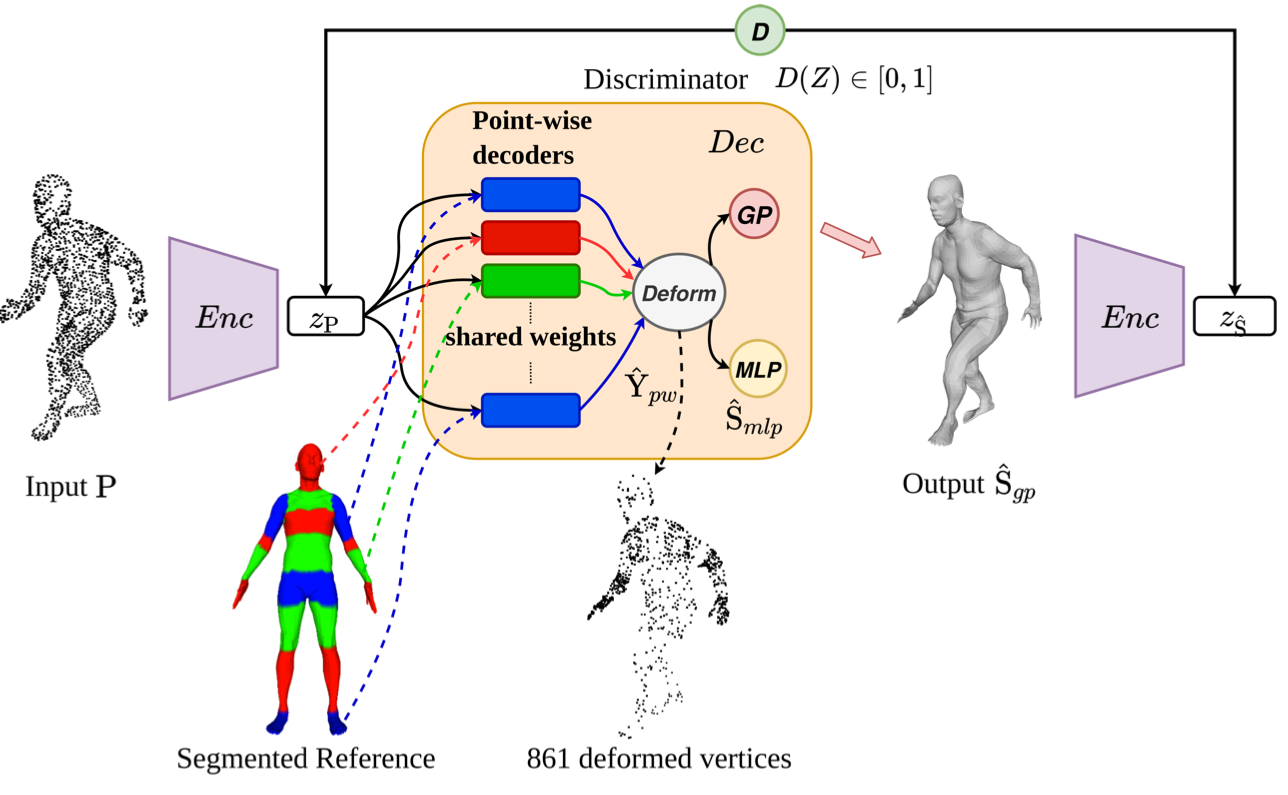
We study the problem of reconstructing the template-aligned mesh for human body estimation from unstructured point cloud data. Recently proposed approaches for shape matching that rely on Deep Neural Networks (DNNs) achieve state-of-the-art results with generic point-wise architectures; but in doing so, they exploit much weaker human body shape and surface priors with respect to methods that explicitly model the body surface with 3D templates. We investigate the impact of adding back such stronger shape priors by proposing a novel dedicated human template matching process, which relies on a point-based, deep autoencoder architecture. We encode surface smoothness and shape coherence with a specialized Gaussian Process layer. Furthermore, we enforce global consistency and improve the generalization capabilities of the model by introducing an adversarial training phase. The choice of these elements is grounded on an extensive analysis of DNNs failure modes in widely used datasets like SURREAL and FAUST. We validate and evaluate the impact of our novel components on these datasets, showing a quantitative improvement over state-of-the-art DNN-based methods, and qualitatively better results.
This work was published at Asian Conference on Computer Vision 17.
7.11 Morphology-Based Individual Vertebrae Classification
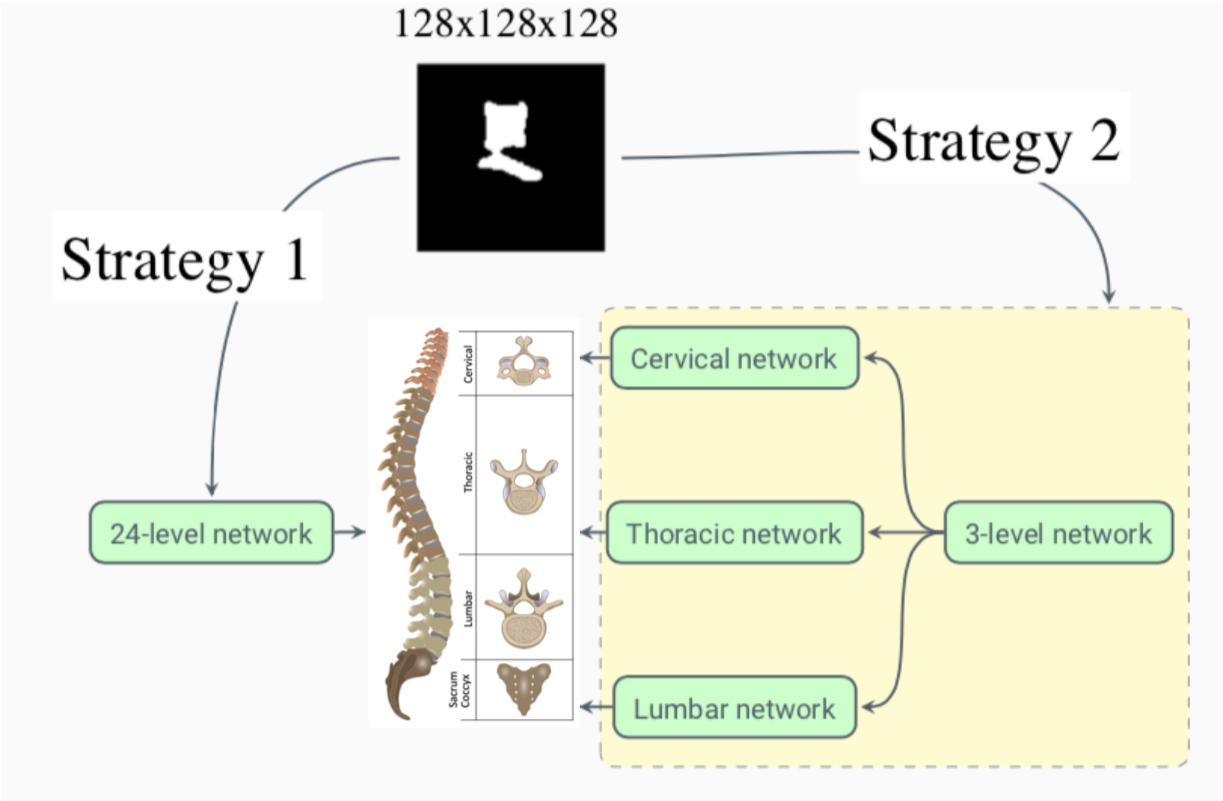
The human spine is composed, in non-pathological cases, of 24 vertebrae. Most vertebrae are morphologically distinct from the others, such as C1 (Atlas) or C2 (Axis), but some are morphologically closer, such as neighboring thoracic or lumbar vertebrae. In this work, we aim at quantifying to which extent the shape of a single vertebra is discriminating. We use a publicly available MICCAI VerSe 2019 Challenge dataset containing individually segmented vertebrae from CT images. We train several variants of a baseline 3D convolutional neural network (CNN) taking a binary volumetric representation of an isolated vertebra as input and regressing the vertebra class. We start by predicting the probability of the vertebrae to belong to each of the 24 classes. Then we study a second approach based on a two-stage aggregated classification which first identifies the anatomic group (cervical, thoracic or lumbar) then uses a group-specific network for the individual classification. Our results show that: i) the shape of an individual vertebra can be used to faithfully identify its group (cervical, thoracic or lumbar), ii) the shape of the cervical and lumbar seems to have enough information for a reliable individual identification, and iii) the thoracic vertebrae seem to have the highest similarity and are the ones where the network is confused the most. Future work will study if other representations (such as meshes or pointclouds) obtain similar results, i.e. does the representation have an impact in the prediction accuracy?
This work was published at Shape in Medical Imaging 19.
7.12 Learning a Statistical Full Spine Model from Partial Observations
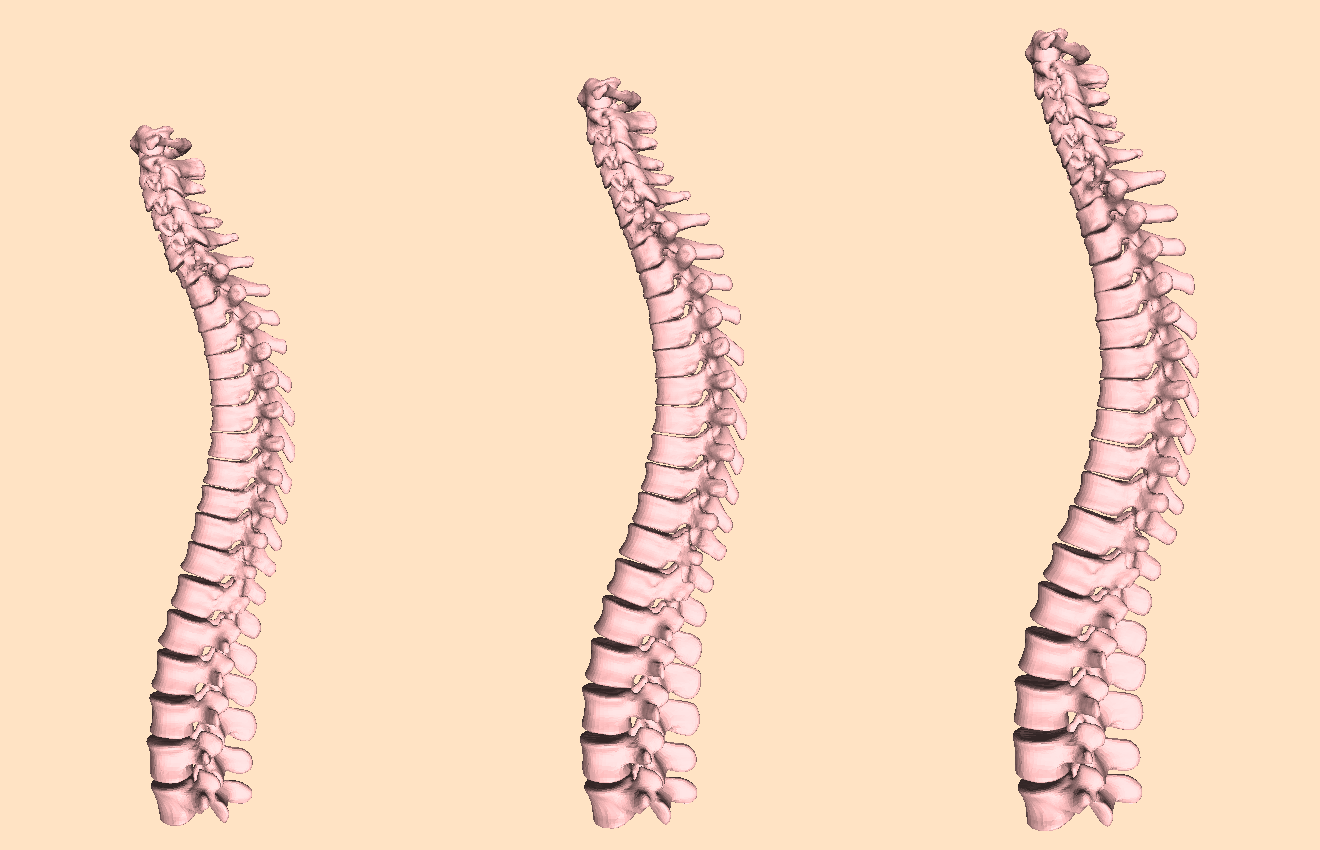
The study of the morphology of the human spine has attracted research attention for its many potential applications, such as image segmentation, bio-mechanics or pathology detection. However, as of today there is no publicly available statistical model of the 3D surface of the full spine. This is mainly due to the lack of openly available 3D data where the full spine is imaged and segmented. In this paper we propose to learn a statistical surface model of the full-spine (7 cervical, 12 thoracic and 5 lumbar vertebrae) from partial and incomplete views of the spine. In order to deal with the partial observations we use probabilistic principal component analysis (PPCA) to learn a surface shape model of the full spine. Quantitative evaluation demonstrates that the obtained model faithfully captures the shape of the population in a low dimensional space and generalizes to left out data. Furthermore, we show that the model faithfully captures the global correlations among the vertebrae shape. Given a partial observation of the spine, i.e. a few vertebrae, the model can predict the shape of unseen vertebrae with a mean error under 3 mm. The full-spine statistical model is trained on the VerSe 2019 public dataset and is publicly made available to the community for non-commercial purposes.
This work was published at Shape in Medical Imaging 18.
8 Bilateral contracts and grants with industry
8.1 Bilateral contracts with industry
- The Morpheo INRIA team and Microsoft research set up a collaboration on the capture and modelling of moving shapes using multiple videos. Two PhD works are part of this collaboration with the objective to make contributions on 4D Modeling. The PhDs take place at Inria Grenoble Rhône-Alpes and involve visits and stays at Microsoft in Cambridge (UK) and Zurich (CH). The collaboration is part of the Microsoft Research - Inria Joint Centre.
- The Morpheo INRIA team has another collaboration with Facebook reality lab in San Francisco. The collaboration involves one PhD who is currently at the INRIA Grenoble Rhône-Alpes working on the estimation of shape and appearance from a single image. The collaboration started in 2019.
8.2 Bilateral grants with industry
- The Morpheo INRIA team also collaborates with the local start-up Anatoscope created by former researchers at INRIA Grenoble. The collaboration involves one PhD (CIFRE) who shares his time between Morpheo and Anatoscope offices. He is working on new classifications methods of the scoliose disease that take the motion of the patient into account in addition to their morphology. The collaboration started in 2020.
9 Partnerships and cooperations
9.1 International research visitors
9.1.1 Visits of international scientists
Stephane Durocher, professor at University of Manitoba, spent his sabbatical at Morpheo. The collaborations initiated during his stay led to two submitted articles.
9.2 National initiatives
9.2.1 ANR
ANR PRCE CaMoPi – Capture and Modelling of the Shod Foot in Motion
The main objective of the CaMoPi project is to capture and model dynamic aspects of the human foot with and without shoes. To this purpose, video and X-ray imagery will be combined to generate novel types of data from which major breakthroughs in foot motion modelling are expected. Given the complexity of the internal foot structure, little is known about the exact motion of its inner structure and the relationship with the shoe. Hence the current state-of-the art shoe conception process still relies largely on ad-hoc know-how. This project aims at better understanding the inner mechanisms of the shod foot in motion in order to rationalise and therefore speed up and improve shoe design in terms of comfort, performance, and cost. This requires the development of capture technologies that do not yet exist in order to provide full dense models of the foot in motion. To reach its goals, the CaMoPi consortium comprises complementary expertise from academic partners : Inria (combined video and X-ray capture and modeling) and Mines St Etienne (finite element modeling), as well as industrial : CTC Lyon (shoe conception and manufacturing, dissemination). The project has effectively started in October 2017 and is currently handled by Tomas Svaton, recruited as an engineer in April 2018.
ANR JCJC SEMBA – Shape, Motion and Body composition to Anatomy
Existing medical imaging techniques, such as Computed Tomography (CT), Dual Energy X-Ray Absorption (DEXA) and Magnetic Resonance Imaging (MRI), allow to observe internal tissues (such as adipose, muscle, and bone tissues) of in-vivo patients. However, these imaging modalities involve heavy and expensive equipment as well as time consuming procedures. External dynamic measurements can be acquired with optical scanning equipment, e.g. cameras or depth sensors. These allow high spatial and temporal resolution acquisitions of the surface of living moving bodies. The main research question of SEMBA is: "can the internal observations be inferred from the dynamic external ones only?". SEMBA’s first hypothesis is that the quantity and distribution of adipose, muscle and bone tissues determine the shape of the surface of a person. However, two subjects with a similar shape may have different quantities and distributions of these tissues. Quantifying adipose, bone and muscle tissue from only a static observation of the surface of the human might be ambiguous. SEMBA's second hypothesis is that the shape deformations observed while the body performs highly dynamic motions will help disambiguating the amount and distribution of the different tissues. The dynamics contain key information that is not present in the static shape. SEMBA’s first objective is to learn statistical anatomic models with accurate distributions of adipose, muscle, and bone tissue. These models are going to be learned by leveraging medical dataset containing MRI and DEXA images. SEMBA's second objective will be to develop computational models to obtain a subject-specific anatomic model with an accurate distribution of adipose, muscle, and bone tissue from external dynamic measurements only.
ANR JCJC 3DMOVE - Learning to synthesize 3D dynamic human motion
It is now possible to capture time-varying 3D point clouds at high spatial and temporal resolution. This allows for high-quality acquisitions of human bodies and faces in motion. However, tools to process and analyze these data robustly and automatically are missing. Such tools are critical to learning generative models of human motion, which can be leveraged to create plausible synthetic human motion sequences. This has the potential to influence virtual reality applications such as virtual change rooms or crowd simulations. Developing such tools is challenging due to the high variability in human shape and motion and due to significant geometric and topological acquisition noise present in state-of-the-art acquisitions. The main objective of 3DMOVE is to automatically compute high-quality generative models from a database of raw dense 3D motion sequences for human bodies and faces. To achieve this objective, 3DMOVE will leverage recently developed deep learning techniques. The project also involves developing tools to assess the quality of the generated motions using perceptual studies. This project currently involves two Ph.D. students: Mathieu Marsot hired in November 2019 and Raphaël Dang-Nhu hired October 2020.
9.2.2 Competitivity Clusters
FUI24 SPINE-PDCA
The goal of the SPINE-PDCA project is to develop a unique medical platform that will streamline the medical procedure and achieve all the steps of a minimally invasive surgery intervention with great precision through a complete integration of two complementary systems for pre-operative planning (EOS platform from EOS IMAGING) and imaging/intra-operative navigation (SGV3D system from SURGIVISIO). Innovative low-dose tracking and reconstruction algorithms will be developed by Inria, and collaboration with two hospitals (APHP Trousseau and CHU Grenoble) will ensure clinical feasibility. The medical need is particularly strong in the field of spinal deformity surgery which can, in case of incorrect positioning of the implants, result in serious musculoskeletal injury, a high repeat rate (10 to 40% of implants are poorly positioned in spine surgery) and important care costs. In paediatric surgery (e. g. idiopathic scoliosis), the rate of exposure to X-rays is an additional major consideration in choosing the surgical approach to engage. For these interventions, advanced linkage between planning, navigation and postoperative verification is essential to ensure accurate patient assessment, appropriate surgical procedure and outcome consistent with clinical objectives. The project has effectively started in October 2018 with Di Meng's recruitment as a PhD candidate.
MIAI Chair 3D Vision
9.3 Regional initiatives
Data Driven 3D Vision Edmond Boyer obtained a chair in the new Multidisciplinary Institute in Artificial Intelligence (MIAI) of Grenoble Alpes University. The chair entitled Data Driven 3D Vision is for 4 years and aims at investigating deep learning for 3D artificial vision in order to break some of the limitations in this domain. Applications are especially related to humans and to the ability to capture and analyze their shapes, appearances and motions, for upcoming new media devices, sport and medical applications. A post doc, Joao Regateiro, was recruited in 2020 within the cahir.
10 Dissemination
10.1 Promoting scientific activities
10.1.1 Scientific events: organisation
Member of the organizing committees
- Edmond Boyer is member of the steering committee of the 3DV conference.
10.1.2 Scientific events: selection
Member of the conference program committees
- Edmond Boyer was area chair for CVPR 2020 and ECCV 2020.
Reviewer
- Stefanie Wuhrer reviewed for CVPR, ECCV, 3DV, SIGGRAPH and SIGGRAPH Asia
- Sergi Pujades reviewed for CVPR, MICCAI and 3DV.
- Jean-Sébastien Franco reviewed for CVPR, ECCV, 3DV
10.1.3 Journal
Member of the editorial boards
- Edmond Boyer is associate editor of the International Journal of Computer Vision (IJCV, Springer).
Reviewer - reviewing activities
- Sergi Pujades reviewed for Computer & Graphics and eLife.
- Jean-Sébastien Franco reviewed for International Journal on Computer vision and Computer & Graphics.
- Edmond Boyer reviewed for International Journal on Computer vision and IEEE PAMI.
10.1.4 Scientific expertise
- Stefanie Wuhrer reviewed a research proposal for ANR
- Stefanie Wuhrer was part of the CRCN / ISFP hiring committee
- Sergi Pujades was part of the Labex IPU hiring committee
- Sergi Pujades was part of the Persival IPU hiring committee
- Jean-Sébastien Franco is part of the Ensimag ATER hiring comittee
- Edmond Boyer reviewed a consolidator ERC research proposal.
10.1.5 Research administration
- Edmond Boyer is auditor for the Computer Vision European Association.
10.2 Teaching - Supervision - Juries
10.2.1 Teaching
- Licence : Sergi Pujades, Algorithmique et programmation fonctionnelle, 41H équivalent TD, L1, Université Grenoble Alpes, France.
- Licence : Sergi Pujades, Modélisation des structures informatiques: aspects formels, 45H équivalent TD, L1, Université Grenoble Alpes, France.
- Master : Sergi Pujades, Numerical Geometry, 15H équivalent TD, M1, Université Grenoble Alpes, France
- Master: Sergi Pujades, Introduction to Visual Computing, 18h, M1 MoSig, Université Grenoble Alpes.
- Master: Sergi Pujades, 3D Graphics, 13h, M1 Ensimag 2nd year.
- Master: Jean-Sébastien Franco, Supervision of the 2nd year program (300 students), 36h, Ensimag 2nd year, Grenoble INP.
- Master: Jean-Sébastien Franco, Introduction to Computer Graphics, 45h, Ensimag 2nd year, Grenoble INP.
- Master: Jean-Sébastien Franco, Introduction to Computer Vision, 27h, Ensimag 3rd year, Grenoble INP.
- Master: Edmond Boyer, 3D Modeling, 23h, M2R Mosig GVR, Grenoble INP.
- Master: Edmond Boyer, Introduction to Visual Computing, 42h, M1 MoSig, Université Grenoble Alpes.
- Master: Stefanie Wuhrer, 3D Graphics, 11h, M1 MoSig and MSIAM, Université Grenoble Alpes.
10.2.2 Supervision
- PhD: Victoria Fernandez Abrevaya, Large Scale Learning of Shape and Motion Models for the 3D Face, Université Grenoble Alpes (France), November 2020, supervised by Edmond Boyer and Stefanie Wuhrer.
- PhD in progress : Sanae Dariouche, Anatomic Statistical Models of adipose, bone and muscle tissue, Université Grenoble Alpes (France), 01/11/2019, Sergi Pujades and Edmond Boyer
- PhD in progress : Marilyn Keller, Ribs motion models for personalized 3D printed implants, 23/09/2019, Sergi Pujades and Michael Black
- PhD in progress : Nicolas Conte, Scoliosis classification, 01/04/2020, Sergi Pujades and François Faure and Edmond Boyer and Aurélien Courvoisier
- PhD in progress : Di Meng, Deep learning for low-dose CBCT(Cone-Beam Computed Tomography) reconstruction and registration,Université Grenoble Alpes (France), 23/09/2019, Edmond Boyer and Sergi Pujades
- PhD in progress: Matthieu Armando, Temporal Integration for Shape and Appearance Modeling, Université Grenoble Alpes (France), started 01/01/2018, supervised by Edmond Boyer and Jean-Sébastien Franco.
- PhD in progress: Boyao Zhou, Augmenting User Self-Representation in VR Environments, Université Grenoble Alpes (France), started 01/02/2019, supervised by Edmond Boyer and Jean-Sébastien Franco.
- PhD in progress: Jean Basset, Learning Morphologically Plausible Pose Transfer, Université Grenoble Alpes (France), started 01/10/2018, supervised by Edmond Boyer, Franck Multon and Stefanie Wuhrer.
- PhD in progress: Abdullah Haroon Rasheed, Cloth Modeling and Simulation, Université Grenoble Alpes (France), started 01/11/2017, supervised by Florence Bertails-Descoubes, Jean-Sébastien Franco and Stefanie Wuhrer.
- PhD in progress: Pierre Zins, Learning to infer human motion, Université Grenoble Alpes (France), started 01/10/2019, supervised by Edmond Boyer, Tony Tung (Facebook) and Stefanie Wuhrer.
- PhD in progress: Mathieu Marsot, Generative modeling of 3D human motion, Université Grenoble Alpes (France), started 01/11/2019, supervised by Jean-Sébastien Franco and Stefanie Wuhrer.
- PhD in progress: Raphaël Dang-Nhu, Generating and evaluating human motion, Université Grenoble Alpes (France), started 01/10/2020, supervised by Anne-Hélène Olivier (Université Rennes) and Stefanie Wuhrer.
10.2.3 Juries
- Stefanie Wuhrer was jury member for the PhD defense of Adrien Poulenard, LIX, March 2020.
- Stefanie Wuhrer was mid-thesis expert for PhD candidate Marie-Julie Rakotosaona, LIX, October 2020.
- Edmond Boyer was jury member for the PhD defense of Moussa Issa, INSEP Paris, January 2020.
- Edmond Boyer was reviewer for the HDR of Hassen Drida, , June 2020.
- Edmond Boyer was president for the HDR jury of Xavier Alameda, INRIA Grenoble, December 2020.
10.3 Popularization
10.3.1 Internal or external Inria responsibilities
- Edmond Boyer is member of the INRIA Grenoble Rhône-Alpes COS (scientific committee).
10.3.2 Interventions
- Edmond Boyer organized (October 2020) a seminar for the Inria students at the early stage of a PhD that aims at informing and clarifying on aspects of the PhD thesis execution.
11 Scientific production
11.1 Major publications
- 1 articleLearning and Tracking the 3D Body Shape of Freely Moving Infants from RGB-D sequencesIEEE Transactions on Pattern Analysis and Machine Intelligence4210October 2020, 2540-2551
- 2 inproceedings Discrete Point Flow Networks for Efficient Point Cloud Generation 16th European Conference on Computer Vision Glasgow, United Kingdom August 2020
- 3 article Volume Sweeping: Learning Photoconsistency for Multi-View Shape Reconstruction International Journal of Computer Vision September 2020
- 4 inproceedingsLearning to Measure the Static Friction Coefficient in Cloth ContactCVPR 2020 - IEEE Conference on Computer Vision and Pattern RecognitionSeattle, United StatesJune 2020, 1-10
11.2 Publications of the year
International journals
- 5 article Mesh Denoising with Facet Graph Convolutions IEEE Transactions on Visualization and Computer Graphics 2020
- 6 articleContact preserving shape transfer: Retargeting motion from one shape to anotherComputers and Graphics892020, 11-23
- 7 article3D Morphable Face Models - Past, Present and FutureACM Transactions on Graphics395June 2020, 157:1-38
- 8 articleLearning and Tracking the 3D Body Shape of Freely Moving Infants from RGB-D sequencesIEEE Transactions on Pattern Analysis and Machine Intelligence4210October 2020, 2540-2551
- 9 article Volume Sweeping: Learning Photoconsistency for Multi-View Shape Reconstruction International Journal of Computer Vision September 2020
- 10 articleAnalysis of motor development within the first year of life: 3-D motion tracking without markers for early detection of developmental disordersBundesgesundheitsblatt - Gesundheitsforschung - Gesundheitsschutz637July 2020, 881-890
- 11 articleGeneral Movement Assessment from videos of computed 3D infant body models is equally effective compared to conventional RGB Video ratingEarly Human Development144May 2020, 104967
International peer-reviewed conferences
- 12 inproceedingsCross-modal Deep Face Normals with Deactivable Skip ConnectionsCVPR 2020 - IEEE Conference on Computer Vision and Pattern RecognitionSeattle, United StatesJune 2020, 1-11
- 13 inproceedings Discrete Point Flow Networks for Efficient Point Cloud Generation 16th European Conference on Computer Vision Glasgow, United Kingdom August 2020
- 14 inproceedingsLearning to Dress 3D People in Generative ClothingCVPR 2020 - IEEE/CVF Conference on Computer Vision and Pattern RecognitionSeattle, United StatesJune 2020, 6468-6477
- 15 inproceedingsLearning to Measure the Static Friction Coefficient in Cloth ContactCVPR 2020 - IEEE Conference on Computer Vision and Pattern RecognitionSeattle, United StatesJune 2020, 9909-9918
- 16 inproceedingsGENTEL : GENerating Training data Efficiently for Learning to segment medical imagesRFIAP 2020 - Congrés Reconnaissance des Formes, Image, Apprentissage et PerceptionVannes, FranceJune 2020, 1-7
- 17 inproceedings Reconstructing Human Body Mesh from Point Clouds by Adversarial GP Network ACCV - Asian Conference on Computer Vision Kyoto, Japan November 2020
Scientific book chapters
- 18 inbookLearning a Statistical Full Spine Model from Partial Observations12474Shape in Medical Imaging. ShapeMI 2020Lecture Notes in Computer ScienceOctober 2020, 122-133
- 19 inbookMorphology-Based Individual Vertebrae Classification12474Shape in Medical Imaging. ShapeMI 2020Lecture Notes in Computer ScienceOctober 2020, 134-144
Doctoral dissertations and habilitation theses
- 20 thesis Large-scale learning of shape and motion models for the 3D face Grenoble UGA - Université Grenoble Alpes November 2020