Keywords
Computer Science and Digital Science
- A2.3.3. Real-time systems
- A2.4.2. Model-checking
- A3.4.1. Supervised learning
- A3.4.2. Unsupervised learning
- A3.4.6. Neural networks
- A5.4.2. Activity recognition
- A5.4.5. Object tracking and motion analysis
- A9.1. Knowledge
- A9.2. Machine learning
Other Research Topics and Application Domains
- B1.2.2. Cognitive science
- B2.1. Well being
- B7.1.1. Pedestrian traffic and crowds
- B8.1. Smart building/home
- B8.4. Security and personal assistance
1 Team members, visitors, external collaborators
Research Scientists
- Francois Bremond [Team leader, Inria, Senior Researcher, HDR]
- Antitza Dantcheva [Inria, Researcher]
- Esma Ismailova [Ecole Nationale Supérieure des Mines de Saint Etienne, Researcher, from Sep 2020]
- Alexandra Konig [Inria, Starting Research Position]
- Sabine Moisan [Inria, Researcher, HDR]
- Jean-Paul Rigault [Univ Côte d'Azur, Emeritus]
- Monique Thonnat [Inria, Senior Researcher, HDR]
- Susanne Thummler [Univ Côte d'Azur, Researcher, from Sep 2020]
Faculty Member
- Elisabetta De Maria [Univ Côte d'Azur, Associate Professor, until Aug 2020]
Post-Doctoral Fellows
- Michal Balazia [Univ Côte d'Azur]
- Abhijit Das [Inria, from Dec 2020]
- Laura Ferrari [Univ Côte d'Azur, from Feb 2020]
- Mohsen Tabejamaat [Inria, from Nov 2020]
- Leonard Torossian [Inria, from Sep 2020]
- Ujjwal Ujjwal [Inria]
PhD Students
- Abid Ali [Univ Côte d'Azur, from Nov 2020]
- Hao Chen [ES]
- Rui Dai [Univ Côte d'Azur]
- Srijan Das [Univ Côte d'Azur, until Nov 2020]
- Juan Diego Gonzales Zuniga [KONTRON]
- Mohammed Guermal [Inria, from Dec 2020]
- Jen Cheng Hou [Inria]
- Thibaud Lyvonnet [Inria]
- Yaohui Wang [Inria]
- Di Yang [Inria]
Technical Staff
- Tanay Agrawal [Inria, Engineer, from Oct 2020]
- Sebastien Gilabert [Inria, Engineer]
- Rachid Guerchouche [Inria, Engineer, until Apr 2020]
- Farhood Negin [Inria, Engineer, from Oct 2020]
- Tran Duc Tran [Inria, Engineer]
Interns and Apprentices
- Adit Vinay Deshmukh [Inria, until Jul 2020]
- Indu Joshi [Inria, from Feb 2020 until Jul 2020]
- Snehashis Majhi [Inria, until Feb 2020]
- Rupayan Mallick [Inria, from Feb 2020 until Aug 2020]
- Akankshya Mishra [Inria, until Jun 2020]
- Ritaban Roy [Inria, until Jul 2020]
- Carlotta Sanges [University of Genoa, from Oct 2020]
- Yi Xian Ye [Inria, until Jul 2020]
Administrative Assistant
- Sandrine Boute [Inria]
Visiting Scientist
- David Anghelone [Thales, until Jun 2020]
External Collaborators
- Daniel Gaffe [Univ Côte d'Azur]
- Valeria Manera [Univ Côte d'Azur]
- Minh Khue Phan Tran [Univ Côte d'Azur, until Apr 2020]
- Philippe Robert [Inria, CoBTeK]
2 Overall objectives
2.1 Presentation
The STARS (Spatio-Temporal Activity Recognition Systems) team focuses on the design of cognitive vision systems for Activity Recognition. More precisely, we are interested in the real-time semantic interpretation of dynamic scenes observed by video cameras and other sensors. We study long-term spatio-temporal activities performed by agents such as human beings, animals or vehicles in the physical world. The major issue in semantic interpretation of dynamic scenes is to bridge the gap between the subjective interpretation of data and the objective measures provided by sensors. To address this problem Stars develops new techniques in the field of computer vision, machine learning and cognitive systems for physical object detection, activity understanding, activity learning, vision system design and evaluation. We focus on two principal application domains: visual surveillance and healthcare monitoring.
2.2 Research Themes
Stars is focused on the design of cognitive systems for Activity Recognition. We aim at endowing cognitive systems with perceptual capabilities to reason about an observed environment, to provide a variety of services to people living in this environment while preserving their privacy. In today world, a huge amount of new sensors and new hardware devices are currently available, addressing potentially new needs of the modern society. However the lack of automated processes (with no human interaction) able to extract a meaningful and accurate information (i.e. a correct understanding of the situation) has often generated frustrations among the society and especially among older people. Therefore, Stars objective is to propose novel autonomous systems for the real-time semantic interpretation of dynamic scenes observed by sensors. We study long-term spatio-temporal activities performed by several interacting agents such as human beings, animals and vehicles in the physical world. Such systems also raise fundamental software engineering problems to specify them as well as to adapt them at run time.
We propose new techniques at the frontier between computer vision, knowledge engineering, machine learning and software engineering. The major challenge in semantic interpretation of dynamic scenes is to bridge the gap between the task dependent interpretation of data and the flood of measures provided by sensors. The problems we address range from physical object detection, activity understanding, activity learning to vision system design and evaluation. The two principal classes of human activities we focus on, are assistance to older adults and video analytic.
Typical examples of complex activity are shown in
Figure 1 and Figure 2 for a homecare application (See
Toyota Smarthome Dataset at https://
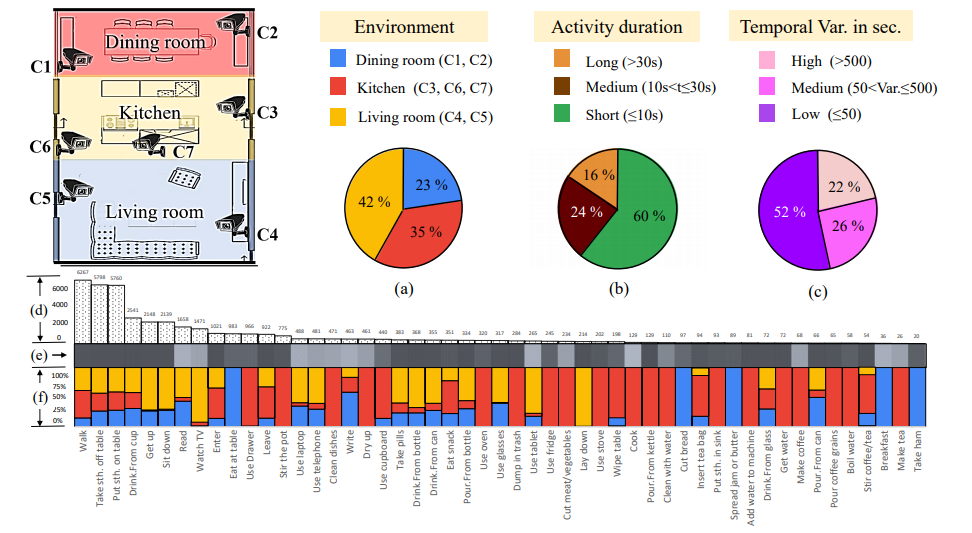
.png)
Activity (PrepareMeal, | |
PhysicalObjects( | (p : Person), (z : Zone), (eq : Equipment)) |
Components( | (s_inside : InsideKitchen(p, z)) |
(s_close : CloseToCountertop(p, eq)) | |
(s_stand : PersonStandingInKitchen(p, z))) | |
Constraints( | (z->Name = Kitchen) |
(eq->Name = Countertop) | |
(s_close->Duration >= 100) | |
(s_stand->Duration >= 100)) | |
Annotation( | AText("prepare meal"))) |
The ultimate goal is for cognitive systems to perceive and understand their environment to be able to provide appropriate services to a potential user. An important step is to propose a computational representation of people activities to adapt these services to them. Up to now, the most effective sensors have been video cameras due to the rich information they can provide on the observed environment. These sensors are currently perceived as intrusive ones. A key issue is to capture the pertinent raw data for adapting the services to the people while preserving their privacy. We plan to study different solutions including of course the local processing of the data without transmission of images and the utilization of new compact sensors developed for interaction (also called RGB-Depth sensors, an example being the Kinect) or networks of small non visual sensors.
2.3 International and Industrial Cooperation
Our work has been applied in the context of more than 10 European projects such as COFRIEND, ADVISOR, SERKET, CARETAKER, VANAHEIM, SUPPORT, DEM@CARE, VICOMO, EIT Health.
We had or have industrial collaborations in several domains: transportation (CCI Airport Toulouse Blagnac, SNCF, Inrets, Alstom, Ratp, Toyota, GTT (Italy), Turin GTT (Italy)), banking (Crédit Agricole Bank Corporation, Eurotelis and Ciel), security (Thales R&T FR, Thales Security Syst, EADS, Sagem, Bertin, Alcatel, Keeneo), multimedia (Thales Communications), civil engineering (Centre Scientifique et Technique du Bâtiment (CSTB)), computer industry (BULL), software industry (AKKA), hardware industry (ST-Microelectronics) and health industry (Philips, Link Care Services, Vistek).
We have international cooperations with research centers such as Reading University (UK), ENSI Tunis (Tunisia), Idiap (Switzerland), Multitel (Belgium), National Cheng Kung University, National Taiwan University (Taiwan), MICA (Vietnam), IPAL, I2R (Singapore), University of Southern California, University of South Florida (USA), Michigan State University (USA), Chinese Academy of Sciences (China), IIIT Delhi (India), Hochschule Darmstadt (Germany), Fraunhofer Institute for Computer Graphics Research IGD (Germany).
2.3.1 Industrial Contracts
-
Toyota: (Action Recognition System):
This project run from the 1st of August 2013 up to 2023. It aimed at detecting critical situations in the daily life of older adults living home alone. The system is intended to work with a Partner Robot (to send real-time information to the robot for assisted living) to better interact with older adults. The funding was 106 Keuros for the 1st period and more for the following years.
- Thales: This contract is a CIFRE PhD grant and runs from September 2018 until September 2021 within the French national initiative SafeCity. The main goal is to analyze faces and events in the invisible spectrum (i.e., low energy infrared waves, as well as ultraviolet waves). In this context models will be developed to efficiently extract identity, as well as event - information. This models will be employed in a school environment, with a goal of pseudo-anonymized identification, as well as event-detection. Expected challenges have to do with limited colorimetry and lower contrasts.
- Kontron: This contract is a CIFRE PhD grant and runs from April 2018 until April 2021 to embed CNN based people tracker within a video-camera.
- ESI: This contract is a CIFRE PhD grant and runs from September 2018 until March 2022 to develop a novel Re-Identification algorithm which can be easily set-up with low interaction.
3 Research program
3.1 Introduction
Stars follows three main research directions: perception for activity recognition, action recognition and semantic activity recognition. These three research directions are organized following the workflow of activity recognition systems: First, the perception and the action recognition directions provide new techniques to extract powerful features, whereas the semantic activity recognition research direction provides new paradigms to match these features with concrete video analytic and healthcare applications.
Transversely, we consider a new research axis in machine learning, combining a priori knowledge and learning techniques, to set up the various models of an activity recognition system. A major objective is to automate model building or model enrichment at the perception level and at the understanding level.
3.2 Perception for Activity Recognition
Participants: François Brémond, Antitza Dantcheva, Sabine Moisan, Monique Thonnat.
Keywords: Activity Recognition, Scene Understanding, Machine Learning, Computer Vision, Cognitive Vision Systems, Software Engineering.
3.2.1 Introduction
Our main goal in perception is to develop vision algorithms able to address the large variety of conditions characterizing real world scenes in terms of sensor conditions, hardware requirements, lighting conditions, physical objects, and application objectives. We have also several issues related to perception which combine machine learning and perception techniques: learning people appearance, parameters for system control and shape statistics.
3.2.2 Appearance Models and People Tracking
An important issue is to detect in real-time physical objects from perceptual features and predefined 3D models. It requires finding a good balance between efficient methods and precise spatio-temporal models. Many improvements and analysis need to be performed in order to tackle the large range of people detection scenarios.
Appearance models. In particular, we study the temporal variation of the features characterizing the appearance of a human. This task could be achieved by clustering potential candidates depending on their position and their reliability. This task can provide any people tracking algorithms with reliable features allowing for instance to (1) better track people or their body parts during occlusion, or to (2) model people appearance for re-identification purposes in mono and multi-camera networks, which is still an open issue. The underlying challenge of the person re-identification problem arises from significant differences in illumination, pose and camera parameters. The re-identification approaches have two aspects: (1) establishing correspondences between body parts and (2) generating signatures that are invariant to different color responses. As we have already several descriptors which are color invariant, we now focus more on aligning two people detection and on finding their corresponding body parts. Having detected body parts, the approach can handle pose variations. Further, different body parts might have different influence on finding the correct match among a whole gallery dataset. Thus, the re-identification approaches have to search for matching strategies. As the results of the re-identification are always given as the ranking list, re-identification focuses on learning to rank. "Learning to rank" is a type of machine learning problem, in which the goal is to automatically construct a ranking model from a training data.
Therefore, we work on information fusion to handle perceptual features coming from various sensors (several cameras covering a large scale area or heterogeneous sensors capturing more or less precise and rich information). New 3D RGB-D sensors are also investigated, to help in getting an accurate segmentation for specific scene conditions.
Long term tracking. For activity recognition we need robust and coherent object tracking over long periods of time (often several hours in video surveillance and several days in healthcare). To guarantee the long term coherence of tracked objects, spatio-temporal reasoning is required. Modeling and managing the uncertainty of these processes is also an open issue. In Stars we propose to add a reasoning layer to a classical Bayesian framework modeling the uncertainty of the tracked objects. This reasoning layer can take into account the a priori knowledge of the scene for outlier elimination and long-term coherency checking.
Controlling system parameters. Another research direction is to manage a library of video processing programs. We are building a perception library by selecting robust algorithms for feature extraction, by insuring they work efficiently with real time constraints and by formalizing their conditions of use within a program supervision model. In the case of video cameras, at least two problems are still open: robust image segmentation and meaningful feature extraction. For these issues, we are developing new learning techniques.
3.3 Action Recognition
Participants: François Brémond, Antitza Dantcheva, Monique Thonnat.
Keywords: Machine Learning, Computer Vision, Cognitive Vision Systems.
3.3.1 Introduction
Due to the recent development of high processing units, such as GPU, this is now possible to extract meaningful features directly from videos (e.g. video volume) to recognize reliably short actions. Action Recognition benefits also greatly from the huge progress made recently in Machine Learning (e.g. Deep Learning), especially for the study of human behavior. For instance, Action Recognition enables to measure objectively the behavior of humans by extracting powerful features characterizing their everyday activities, their emotion, eating habits and lifestyle, by learning models from a large number of data from a variety of sensors, to improve and optimize for example, the quality of life of people suffering from behavior disorders. However, Smart Homes and Partner Robots have been well advertised but remain laboratory prototypes, due to the poor capability of automated systems to perceive and reason about their environment. A hard problem is for an automated system to cope 24/7 with the variety and complexity of the real world. Another challenge is to extract people fine gestures and subtle facial expressions to better analyze behavior disorders, such as anxiety or apathy. Taking advantage of what is currently studied for self-driving cars or smart retails, there is a large avenue to design ambitious approaches for the healthcare domain. In particular, the advance made with Deep Learning algorithms has already enabled to recognize complex activities, such as cooking interactions with instruments, and from this analysis to differentiate healthy people from the ones suffering from dementia.
To address these issues, we propose to tackle several challenges:
3.3.2 Action recognition in the wild
The current Deep Learning techniques are mostly developed to work on few clipped videos, which have been recorded with students performing a limited set of predefined actions in front of a camera with high resolution. However, real life scenarios include actions performed in a spontaneous manner by older people (including people interactions with their environment or with other people), from different viewpoints, with varying framerate, partially occluded by furniture at different locations within an apartment depicted through long untrimmed videos. Therefore, a new dedicated dataset should be collected in a real-world setting to become a public benchmark video dataset and to design novel algorithms for ADL activity recognition. A special attention should be taken to anonymize the videos.
3.3.3 Attention mechanisms for action recognition
Activities of Daily Living (ADL) and video-surveillance activities are different from internet activities (e.g. Sports, Movies, YouTube), as they may have very similar context (e.g. same background kitchen) with high intra-variation (different people performing the same action in different manners), but in the same time low inter-variation, similar ways to perform two different actions (e.g. eating and drinking a glass of water). Consequently, fine-grained actions are badly recognized. So, we will design novel attention mechanisms for action recognition, for the algorithm being able to focus on a discriminative part of the person conducting the action. For instance, we will study attention algorithms, which could focus on the most appropriate body parts (e.g. full body, right hand). In particular, we plan to design a soft mechanism, learning the attention weights directly on the feature map of a 3DconvNet, a powerful convolutional network, which takes as input a batch of videos.
3.3.4 Action detection for untrimmed videos
Many approaches have been proposed to solve the problem of action recognition in short clipped 2D videos, which achieved impressive results with hand-crafted and deep features. However, these approaches cannot address real life situations, where cameras provide online and continuous video streams in applications such as robotics, video surveillance, and smart-homes. Here comes the importance of action detection to help recognizing and localizing each action happening in long videos. Action detection can be defined as the ability to localize starting and ending of each human action happening in the video, in addition to recognizing each action label. There have been few action detection algorithms designed for untrimmed videos, which are based on either sliding window, temporal pooling or frame-based labeling. However, their performance is too low to address real-word datasets. A first task consists in benchmarking the already published approaches to study their limitations on novel untrimmed video datasets, recorded following real-world settings. A second task could be to propose a new mechanism to improve either 1) the temporal pooling directly from the 3DconvNet architecture using for instance Temporal Convolution Networks (TCNs) or 2) frame-based labeling with a clustering technique (e.g. using Fisher Vectors) to discover the sub-activities of interest.
3.3.5 View invariant action recognition
The performance of current approaches strongly relies on the used camera angle: enforcing that the camera angle used in testing is the same (or extremely close to) as the camera angle used in training, is necessary for the approach performs well. On the contrary, the performance drops when a different camera view-point is used. Therefore, we aim at improving the performance of action recognition algorithms by relying on 3D human pose information. For the extraction of the 3D pose information, several open-source algorithms can be used, such as openpose or videopose3D (from CMU or Facebook research, https://
3.3.6 Uncertainty and action recognition
Another challenge is to combine the short-term actions recognized by powerful Deep Learning techniques with long-term activities defined by constraint-based descriptions and linked to user interest. To realize this objective, we have to compute the uncertainty (i.e. likelihood or confidence), with which the short-term actions are inferred. This research direction is linked to the next one, to Semantic Activity Recognition.
3.4 Semantic Activity Recognition
Participants: François Brémond, Sabine Moisan, Monique Thonnat.
Keywords: Activity Recognition, Scene Understanding, Computer Vision.
3.4.1 Introduction
Semantic activity recognition is a complex process where information is abstracted through four levels: signal (e.g. pixel, sound), perceptual features, physical objects and activities. The signal and the feature levels are characterized by strong noise, ambiguous, corrupted and missing data. The whole process of scene understanding consists in analyzing this information to bring forth pertinent insight of the scene and its dynamics while handling the low level noise. Moreover, to obtain a semantic abstraction, building activity models is a crucial point. A still open issue consists in determining whether these models should be given a priori or learned. Another challenge consists in organizing this knowledge in order to capitalize experience, share it with others and update it along with experimentation. To face this challenge, tools in knowledge engineering such as machine learning or ontology are needed.
Thus we work along the following research axes: high level understanding (to recognize the activities of physical objects based on high level activity models), learning (how to learn the models needed for activity recognition) and activity recognition and discrete event systems.
3.4.2 High Level Understanding
A challenging research axis is to recognize subjective activities of physical objects (i.e. human beings, animals, vehicles) based on a priori models and objective perceptual measures (e.g. robust and coherent object tracks).
To reach this goal, we have defined original activity recognition algorithms and activity models. Activity recognition algorithms include the computation of spatio-temporal relationships between physical objects. All the possible relationships may correspond to activities of interest and all have to be explored in an efficient way. The variety of these activities, generally called video events, is huge and depends on their spatial and temporal granularity, on the number of physical objects involved in the events, and on the event complexity (number of components constituting the event).
Concerning the modeling of activities, we are working towards two directions: the uncertainty management for representing probability distributions and knowledge acquisition facilities based on ontological engineering techniques. For the first direction, we are investigating classical statistical techniques and logical approaches. For the second direction, we built a language for video event modeling and a visual concept ontology (including color, texture and spatial concepts) to be extended with temporal concepts (motion, trajectories, events ...) and other perceptual concepts (physiological sensor concepts ...).
3.4.3 Learning for Activity Recognition
Given the difficulty of building an activity recognition system with a priori knowledge for a new application, we study how machine learning techniques can automate building or completing models at the perception level and at the understanding level.
At the understanding level, we are learning primitive event detectors. This can be done for example by learning visual concept detectors using SVMs (Support Vector Machines) with perceptual feature samples. An open question is how far can we go in weakly supervised learning for each type of perceptual concept (i.e. leveraging the human annotation task). A second direction is to learn typical composite event models for frequent activities using trajectory clustering or data mining techniques. We name composite event a particular combination of several primitive events.
3.4.4 Activity Recognition and Discrete Event Systems
The previous research axes are unavoidable to cope with the semantic interpretations. However they tend to let aside the pure event driven aspects of scenario recognition. These aspects have been studied for a long time at a theoretical level and led to methods and tools that may bring extra value to activity recognition, the most important being the possibility of formal analysis, verification and validation.
We have thus started to specify a formal model to define, analyze, simulate, and prove scenarios. This model deals with both absolute time (to be realistic and efficient in the analysis phase) and logical time (to benefit from well-known mathematical models providing re-usability, easy extension, and verification). Our purpose is to offer a generic tool to express and recognize activities associated with a concrete language to specify activities in the form of a set of scenarios with temporal constraints. The theoretical foundations and the tools being shared with Software Engineering aspects.
The results of the research performed in perception and semantic activity recognition (first and second research directions) produce new techniques for scene understanding and contribute to specify the needs for new software architectures (third research direction).
4 Application domains
4.1 Introduction
While in our research the focus is to develop techniques, models and platforms that are generic and reusable, we also make effort in the development of real applications. The motivation is twofold. The first is to validate the new ideas and approaches we introduce. The second is to demonstrate how to build working systems for real applications of various domains based on the techniques and tools developed. Indeed, Stars focuses on two main domains: video analytic and healthcare monitoring.
Domaine : Video Analytics
Our experience in video analytic (also referred to as visual surveillance) is a strong basis which ensures both a precise view of the research topics to develop and a network of industrial partners ranging from end-users, integrators and software editors to provide data, objectives, evaluation and funding.
For instance, the Keeneo start-up was created in July 2005 for the industrialization and exploitation of Orion and Pulsar results in video analytic (VSIP library, which was a previous version of SUP). Keeneo has been bought by Digital Barriers in August 2011 and is now independent from Inria. However, Stars continues to maintain a close cooperation with Keeneo for impact analysis of SUP and for exploitation of new results.
Moreover new challenges are arising from the visual surveillance community. For instance, people detection and tracking in a crowded environment are still open issues despite the high competition on these topics. Also detecting abnormal activities may require to discover rare events from very large video data bases often characterized by noise or incomplete data.
Domaine : Healthcare Monitoring
Since 2011, we have initiated a strategic partnership (called CobTek) with Nice hospital (CHU Nice, Prof P. Robert) to start ambitious research activities dedicated to healthcare monitoring and to assistive technologies. These new studies address the analysis of more complex spatio-temporal activities (e.g. complex interactions, long term activities).
4.1.1 Research
To achieve this objective, several topics need to be tackled. These topics can be summarized within two points: finer activity description and longitudinal experimentation. Finer activity description is needed for instance, to discriminate the activities (e.g. sitting, walking, eating) of Alzheimer patients from the ones of healthy older people. It is essential to be able to pre-diagnose dementia and to provide a better and more specialized care. Longer analysis is required when people monitoring aims at measuring the evolution of patient behavioral disorders. Setting up such long experimentation with dementia people has never been tried before but is necessary to have real-world validation. This is one of the challenge of the European FP7 project Dem@Care where several patient homes should be monitored over several months.
For this domain, a goal for Stars is to allow people with dementia to continue living in a self-sufficient manner in their own homes or residential centers, away from a hospital, as well as to allow clinicians and caregivers remotely provide effective care and management. For all this to become possible, comprehensive monitoring of the daily life of the person with dementia is deemed necessary, since caregivers and clinicians will need a comprehensive view of the person's daily activities, behavioral patterns, lifestyle, as well as changes in them, indicating the progression of their condition.
4.1.2 Ethical and Acceptability Issues
The development and ultimate use of novel assistive technologies by a vulnerable user group such as individuals with dementia, and the assessment methodologies planned by Stars are not free of ethical, or even legal concerns, even if many studies have shown how these Information and Communication Technologies (ICT) can be useful and well accepted by older people with or without impairments. Thus one goal of Stars team is to design the right technologies that can provide the appropriate information to the medical carers while preserving people privacy. Moreover, Stars will pay particular attention to ethical, acceptability, legal and privacy concerns that may arise, addressing them in a professional way following the corresponding established EU and national laws and regulations, especially when outside France. Now, Stars can benefit from the support of the COERLE (Comité Opérationnel d'Evaluation des Risques Légaux et Ethiques) to help it to respect ethical policies in its applications.
As presented in 2, Stars aims at designing cognitive vision systems with perceptual capabilities to monitor efficiently people activities. As a matter of fact, vision sensors can be seen as intrusive ones, even if no images are acquired or transmitted (only meta-data describing activities need to be collected). Therefore new communication paradigms and other sensors (e.g. accelerometers, RFID, and new sensors to come in the future) are also envisaged to provide the most appropriate services to the observed people, while preserving their privacy. To better understand ethical issues, Stars members are already involved in several ethical organizations. For instance, F. Brémond has been a member of the ODEGAM - “Commission Ethique et Droit” (a local association in Nice area for ethical issues related to older people) from 2010 to 2011 and a member of the French scientific council for the national seminar on “La maladie d'Alzheimer et les nouvelles technologies - Enjeux éthiques et questions de société” in 2011. This council has in particular proposed a chart and guidelines for conducting researches with dementia patients.
For addressing the acceptability issues, focus groups and HMI (Human Machine Interaction) experts, are consulted on the most adequate range of mechanisms to interact and display information to older people.
5 Social and environmental responsibility
5.1 Footprint of research activities
We have limited our travels by reducing our physical participation to conferences and to international collaborations.
5.2 Impact of research results
We have been involved for many years in promoting public transportation by improving safety onboard and in station. Moreover, we have been working on pedestrian detection for self-driving cars, which will help also reducing the number of individual cars.
6 Highlights of the year
Person Re-Identification
Person Re-Identification is a very challenging task, where current Computer Vision algorithms manage to obtain better results than humans. In previous years we obtained the best performance compared to the State-of-the-art approaches on the most popular benchmark datasets (e.g. Market-1501, CUHK03 and MARS), but it was in a supervised training scheme. This year we have obtained similar performance in a unsupervised training manner, using the Teacher-Student paradigm. We still have the State-of-the-art performance in this domain.
Action Recognition
This year, we have proposed several action recognition and action detection approaches able to outperform the State-of-the-art algorithms and get nearly maximal performance on most of ADL benchmark video datasets (e.g. Northwestern- UCLA Multiview Action 3D, NTU-RGB 120, Charades and SmartHome). These novel algorithms rely on novel attention mechanisms, either 3D Pose guided or self-supervised.
Video Generation
We have designed a novel spatio-temporal generative model, which seeks to capture the distribution of high dimensional video data in order to model appearance and motion in a disentangled manner. Thanks to this new model, more diverse videos can be generated by varying specifically the type of motion or emotion. This technique opens the path to new data augmentation approaches.
7 New software and platforms
Several experiences in technological projects have permitted to develop novel softwares and platforms. Some are provided as open-source code. Here are reported only the main ones.
7.1 New software
7.1.1 SUP
- Name: Scene Understanding Platform
- Keywords: Activity recognition, 3D, Dynamic scene
- Functional Description: SUP is a software platform for perceiving, analyzing and interpreting a 3D dynamic scene observed through a network of sensors. It encompasses algorithms allowing for the modeling of interesting activities for users to enable their recognition in real-world applications requiring high-throughput.
-
URL:
https://
team. inria. fr/ stars/ software - Contact: François Brémond
- Participants: Etienne Corvée, François Brémond, Hung Nguyen, Vasanth Bathrinarayanan
- Partners: CEA, CHU Nice, USC Californie, Université de Hamburg, I2R
7.1.2 VISEVAL
- Functional Description: ViSEval is a software dedicated to the evaluation and visualization of video processing algorithm outputs. The evaluation of video processing algorithm results is an important step in video analysis research. In video processing, we identify 4 different tasks to evaluate: detection, classification and tracking of physical objects of interest and event recognition.
-
URL:
http://
www-sop. inria. fr/ teams/ pulsar/ EvaluationTool/ ViSEvAl_Description. html - Contact: François Brémond
- Participants: Bernard Boulay, François Brémond
8 New results
8.1 Introduction
This year Stars has proposed new results related to its three main research axes: (i) perception for activity recognition, (ii) action recognition and (iii) semantic activity recognition.
Perception for Activity Recognition
Participants: François Brémond, Antitza Dantcheva, Juan Diego Gonzales Zuniga, Ujjwal Ujjwal, Hao Chen, David Anghelone, Monique Thonnat.
The new results for perception for activity recognition are:
- Deep Learning applied to Embedded Systems for People Tracking (see 8.2)
- Joint Detection and Tracking of Pedestrians in Real-time (see 8.3)
- Enhancing Diversity in Teacher-Student Networks via Asymmetric branches for Unsupervised Person Re-identification (see 8.4)
- Beyond the Visible - A survey on cross-spectral face recognition (see 8.5)
- Selective Spatio-Temporal Aggregation Based Pose Refinement System (see 8.6)
- Tattoo Fusion emotion recognition through a Tattoo-based wearable and multimodal Fusion (see 8.7)
- GAN: Disentangling Appearance and Motion for Video Generation (see 8.8)
- Comparing 3DCNN approaches for detecting deepfakes (see 8.9)
- Demographic Bias in Biometrics:A Survey on an Emerging Challenge (see 8.10)
Action Recognition
Participants: François Brémond, Antitza Dantcheva, Srijan Das, Rui Dai, Jen-Cheng Hou, Monique Thonnat.
The new results for action recognition are:
- Spatio-Temporal Attention Mechanism for Activity Recognition (see 8.11)
- VPN: Learning Video-Pose Embedding for Activities of Daily Living (see 8.12)
- PDAN: Pyramid Dilated Attention Network for Action Detection (see 8.13)
- TSU: Toyota Smarthome Untrimmed (see 8.14)
- Quantified Analysis for Epileptic Seizure Videos (see 8.15)
- Apathy Classification by Exploiting Task Relatedness (see 8.16)
- A weakly supervised learning technique for classifying facial expressions (see 8.17)
- Semi-supervised emotion recognition using inconsistently annotated data (see 8.18)
Semantic Activity Recognition
Participants: François Brémond, Elisabetta De Maria, Antitza Dantcheva, Srijan Das, Daniel Gaffé, Thibaud L'Yvonnet, Sabine Moisan, Jean-Paul Rigault, Yaohui Wang, Alexandra König, Michal Balazia, Philippe Robert, Monique Thonnat.
For this research axis, the contributions are:
- Probabilistic Model Checking for Activity Recognition in Medical Serious Games (see 8.19)
- MePheSTO – Digital Phenotyping 4 Psychiatric Disorders from Social Interaction (see 8.20)
- DeepSPA - Early detection of cognitive disorders such as dementia on the basis of speech analysis (see 8.21)
- Activis (see 8.22)
- E-Santé (see 8.23)
- An Investigative Study on Face Uniqueness (see 8.24)
- Using Artificial Intelligence for Diagnosis of Psychiatric Disorders (see 8.25)
8.2 Deep Learning applied to Embedded Systems for People Tracking
Participants: Juan Diego Gonzales Zuniga, Ujjwal Ujjwal, François Brémond, Serge Tissot.
Our work objective is two-fold: a) Perform tracking of multiple people in videos, which is an instance of Multiple Object Tracking (MOT) problem, and b) optimize this tracking on embedded and open source hardware platforms such as OpenVINO and ROCm.
People tracking is a challenging and relevant problem since it needs multiple additional modules to perform the data association between nodes. In addition, state-of-the-art solutions require intensive memory allocation and power consumption which are not available on embedded hardware. Most architectures either require great amounts of memory or large computing time to achieve a state-of-the-art performance, these results are mostly achieved with dedicated hardware at data centers.
8.2.1 Online Joint Detection and Tracking
In people tracking, we are questioning the main paradigm that is tracking-by-detection which heavily relies on the performance of the underlying detection method. This requires access to a highly accurate and robust people detector. On the other hand, few frameworks attempt detect and track people jointly. Our intent is to perform people tracking online and jointly with detection.
We are trying to determinate a manner in which a single model can both perform detection and tracking simultaneously. Along these lines, we experimented with a variation of I3D on the Posetrack data set that takes an input of 8 frames in order to create heatmaps along multiple frames as seen in Figure 4. Giving that the data of Posetrack or MOT cannot train a network as I3D, we are doing the pretraining with the synthetic JTA-Dataset.
This work is inspired by the less common methods of tracking-by-tracks and tracking-by-tracklets. Both 43 and 44 generate multi-frame bounding box tuplet proposals and extract detection scores and features with a CNN and LSTM, respectively. Recent researches improve object detection by applying optical flow to propagate scores between frames.
Another method we implemented is by using the detections of previous frames as proposal for the data association, it only uses the IOU between two objects as a distance metric. This approach is simple and efficient assuming the objects do not move drastically. An improved method increases the performance by using a siamese network to conserve identity across frames and predictions for death and birth of tracks.
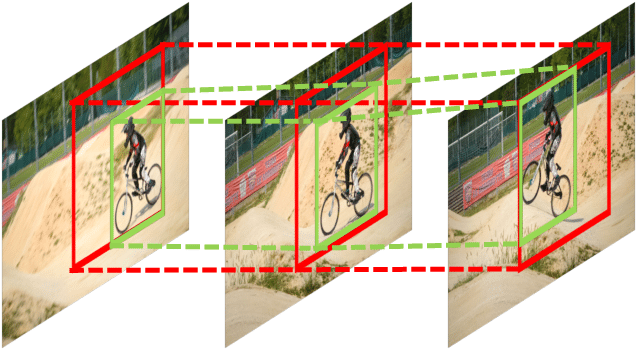
8.2.2 OpenVINO and ROCm
Regarding embedded hardware, we focus on enlarging both implementation and experimentation of two specific frameworks: OpenVINO and ROCm.
OpenVINO allows us to transfer deep learning models into Myriad and KeemBay chips, taking advantage of their capacity to compute multiple operations without the need of much power consumption. We have thoroughly tested their power consumption under different scenarios as well as implemented many qualitative algorithms with these two platforms, Figure 5 shows the Watt consumption and frame rate of the most popular backbone networks, making it viable to use on embedded applications with a reasonable 25FPS.
For ROCm, we have used the approach of 42 to optimize the compiler execution for a variety of CNN features and filters using a substitute GPU with similar computation capability as Nvidia but still remaining a low branch consumption around 15 Watts.
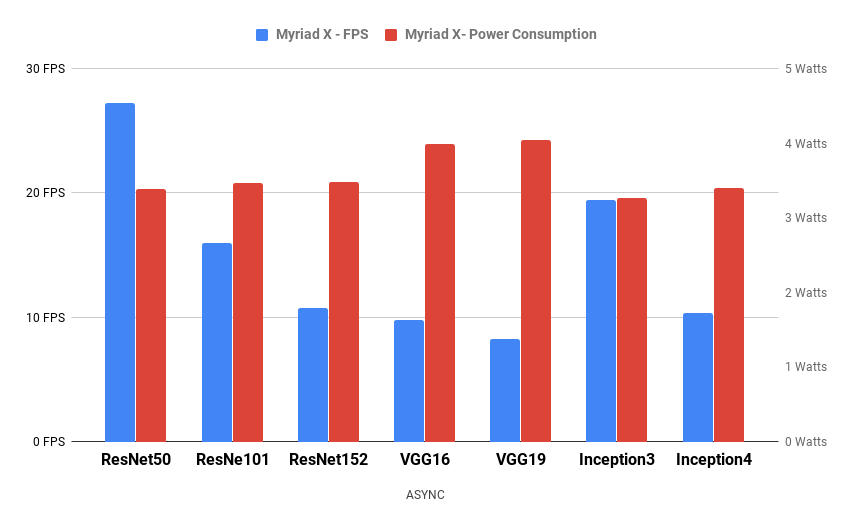
8.3 Joint Detection and Tracking of Pedestrians in Real-time
Participants: Ujjwal Ujjwal, Juan Diego Gonzalez Zuniga, François Brémond.
This work aims at performing an end-to-end detection and tracking of multiple moving targets in a video sequence.
8.3.1 Problem Statement
One of the fundamental ideas of “Joint Detection and Tracking” is to eliminate the period of waiting by the tracker. One other aspect of this problem is to jointly train the detector and tracker together to achieve better global optimization. End-to-end training in modern deep learning systems is preferred as it leads to multiple components of a computer vision system learning in parallel. This has been shown to result in considerable improvements in moden computer vision systems most importantly by reducing cases where early components perform worse resulting in a compromised performance by the whole system.
Another important problem worked upon has been to balance the speed accuracy trade-offs in pedestrian detection 36. This is especially important in autonomous vehicles where highly accurate detections at a high throughput are desired in real-time. Towards, this we choose a novel technique of selecting only the most relevant samples for inference which decrease computational costs by nearly and provide highly accurate pedestrian detection at a high throughput of 32 FPS. This comprises the state-of-art performance in pedestrian detection and is easily deployable in real-life scenarios.
8.3.2 Work Summary
We propose a joint approach to detection and tracking which is end-to-end trainable (see Figure6). Our approach is described in figure 6. Our approach first detects targets using a FPN based object detector called FCOS. These detections are further processed by a graph attention network (GAT). The performance of our method is comparable to state-of-art approaches and is outlined in table 1.
We also summarize our results on pedestrian detection with both speed and accuracy measurements in table 2.
Method | Detr | MOTA | IDF | MT | ML | FP | FN | IDS |
SCNet | Priv | 60.0 | 54.4 | 34.4 | 16.2 | 72230 | 145851 | 7611 |
LSST | Pub | 54.7 | 62.3 | 20.4 | 40.1 | 26091 | 228434 | 1243 |
Tracktor | Pub | 53.5 | 52.3 | 19.5 | 36.3 | 12201 | 248047 | 2072 |
Tracktor++V2 | Pub | 56.3 | 55.1 | 21.1 | 36.3 | 8866 | 235,449 | 1987 |
JBNOT | Pub | 52.6 | 50.8 | 19.7 | 25.8 | 31572 | 232659 | 3050 |
FAMNet | Pub | 52.0 | 48.7 | 19.1 | 33.4 | 14138 | 253616 | 3072 |
TubeTK | w/o | 63.0 | 58.6 | 31.2 | 19.9 | 27060 | 177483 | 4137 |
JDMOT | w/o | 56.4 | 42.0 | 16.7 | 40.8 | 17421 | 223974 | 4572 |
Ours | w/o | 58.7 | 56.9 | 28.7 | 20.2 | 38556 | 189612 | 4830 |
Method | Stages | LAMR | Speed | |||||||
|
|
|||||||||
Faster-RCNN | 2 | 12.10 | 15.4 | 7 | ||||||
SSD | 1 | 17.78 (16.36) | 19.69 | 48 | ||||||
YOLOv2 | 1 | 21.62 (20.83) | NA | 60 | ||||||
RPN-BF | 2 | 9.6 (NA) | NA | 7 | ||||||
MS-CNN | 2 | 10.0 (NA) | NA | 8 | ||||||
SDS-RCNN | 2 | 7.6 (NA) | NA | 5 | ||||||
ALF-Net | 1 | 4.5 (NA) | 12.0 | 20 | ||||||
Rep-Loss | 2 | 5.0 (4.0) | 13.2 | - | ||||||
Ours | 1.5 | 4.76 (3.99) | 8.12 | 32 |
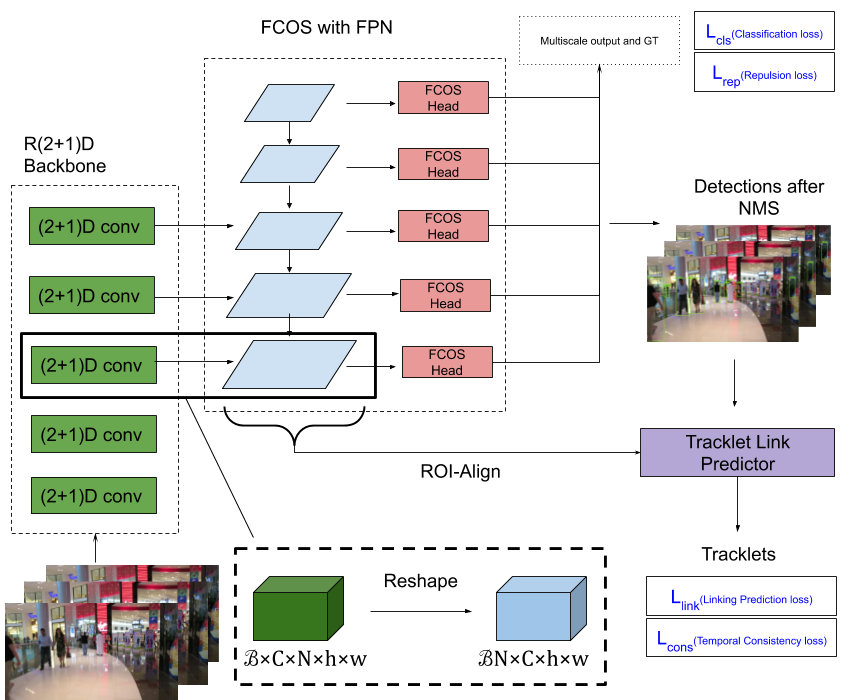
8.4 Enhancing Diversity in Teacher-Student Networks via Asymmetric branches for Unsupervised Person Re-identification
Participants: Hao Chen, Benoit Lagadec, François Brémond.
The objective of unsupervised person re-identification (Re-ID) is to learn discriminative features without labor-intensive identity annotations. State-of-the-art unsupervised Re-ID methods assign pseudo labels to unlabeled images in the target domain and learn from these noisy pseudo labels. Recently introduced Mean Teacher Model is a promising way to mitigate the label noise. However, during the training, self-ensembled teacher-student networks quickly converge to a consensus which leads to a local minimum. We explore the possibility of using an asymmetric structure inside neural network to address this problem. First, symmetric branches are proposed to extract features in different manners, which enhances the feature diversity in appearance signatures. Then, our proposed cross-branch supervision allows one branch to get supervision from the other branch, which transfers distinct knowledge and enhances the weight diversity between teacher and student networks. Extensive experiments show that our proposed method 30 can significantly surpass the performance of previous work on both unsupervised domain adaptation and fully unsupervised Re-ID tasks.
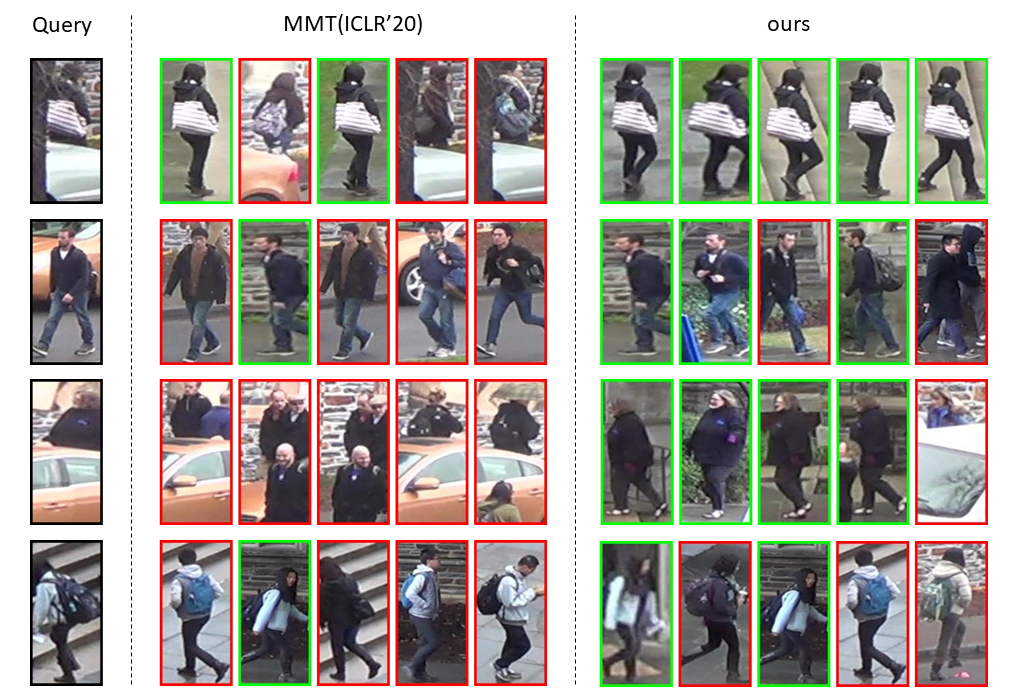
8.5 Beyond the Visible - A survey on cross-spectral face recognition
Participants: David Anghelone, Antitza Dantcheva.
This subject is within the framework of the national project SafeCity: Security of Smart Cities.
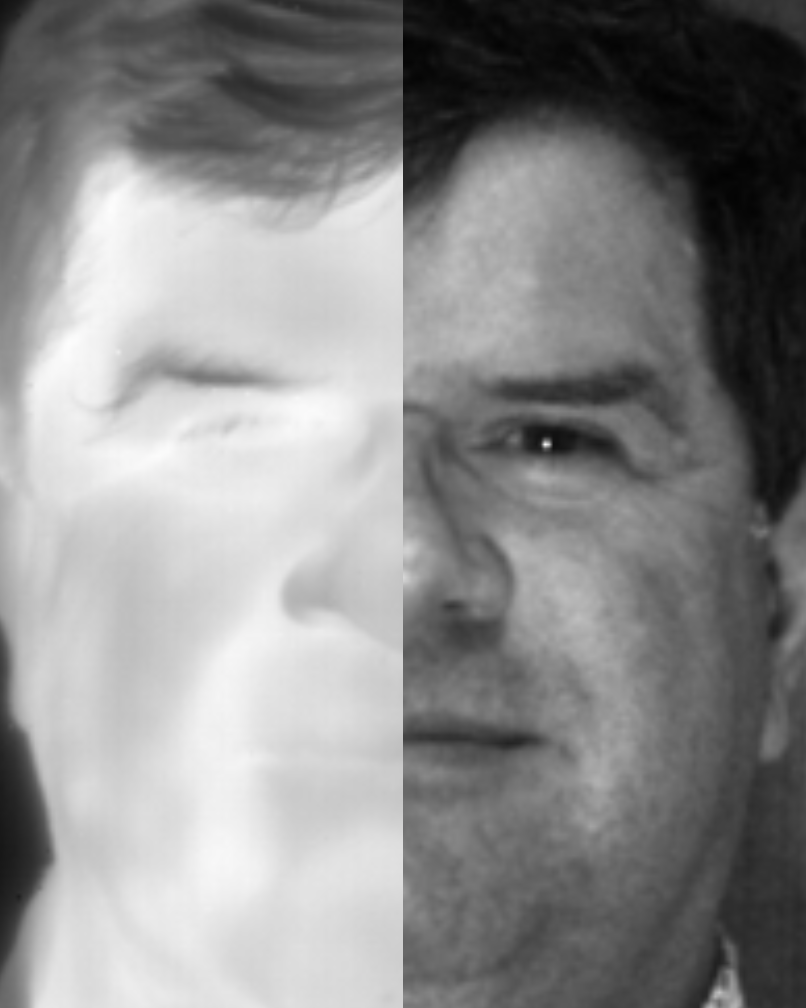
Face recognition has been a highly active area for decades and has witnessed increased interest in the scientific community. In addition, these technologies are being widely deployed, becoming part of our daily life. So far, these systems operate mainly in the visible spectrum as RGB-imagery, due to the ubiquity of advanced sensor technologies. However, limitations encountered in the visible spectrum such as illumination-restriction, variation in poses, noise as well as occlusion significantly degrades the recognition performance. In order to overcome such limitations, recent research has explored face recognition based on spectral bands beyond the visible. In this context, one pertinent scenario has been the matching of facial images that are sensed in different modalities - infrared vs. visible. Challenging in this recognition process has been the significant variation in facial appearance caused by the modality gap, this is depicted on Figure 8. Motivated by this, we conduced a survey on cross-spectral face recognition by providing an overview of recent advance and placing emphasis on deep learning methods.
8.6 Selective Spatio-Temporal Aggregation Based Pose Refinement System
Participants: Di Yang, Rui Dai, Yaohui Wang, Rupayan Mallick, Luca Minciullo, Francesca Gianpiero, François Brémond.
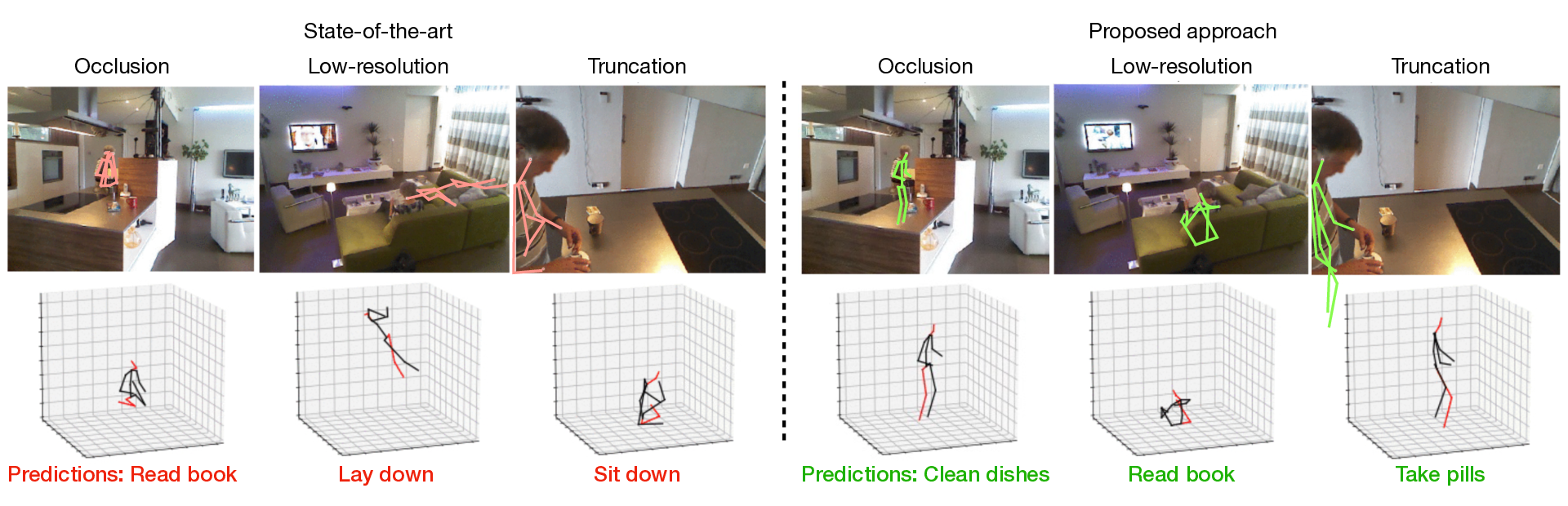
As shown in Fig. 9, taking advantage of human pose data for understanding human activities has attracted much attention these days. However, state-of-the-art pose estimators struggle in obtaining high-quality 2D or 3D pose data due to occlusion, truncation and low-resolution in real-world un-annotated videos. Hence, in this work 40 we propose 1) a selective Spatio-Temporal Aggregation mechanism, named SST-A, that refines and smooths the keypoint locations extracted by several expert pose estimators, 2) an effective weakly-supervised self-training framework which leverages the aggregated poses as pseudo ground-truth instead of handcrafted annotations for real-world pose estimation. Extensive experiments are conducted for evaluating not only the upstream pose refinement but also the downstream action recognition performance on four real-world datasets, NTU-Pose, Toyota Smarthome, Charades, and Kinetics50. We demonstrate that the skeleton data refined by our Pose-Refinement system (SSTA-PRS) is effective at boosting various existing action recognition models, which achieves competitive or state-of-the-art performance.
8.6.1 Results
The quantitative evaluation results with pose ground-truth (Tab. 3) and with action ground-truth (Tab. 4) demonstrate the effectiveness of the proposed method.
Methods | NTU-Pose | Smarthome-Pose |
PCKh (@%) | PCKh (@%) | |
LCRNet++ | 54.1 | 64.4 |
AlphaPose | 53.2 | 55.5 |
OpenPose | 45.4 | 58.9 |
SST-A only(ours) | 61.8 | 65.7 |
SSTA-PRS(ours) | 68.0 | 73.7 |
Methods | RGB | Pose | Smarthome | ||
CS(%) | CV1(%) | CV2(%) | |||
DT |
|
41.9 | 20.9 | 23.7 | |
I3D |
|
53.4 | 34.9 | 45.1 | |
I3D+NL |
|
53.6 | 34.3 | 43.9 | |
AssembleNet++(+object) |
|
63.6 | - | - | |
P-I3D |
|
|
54.2 | 35.1 | 50.3 |
Separable STA |
|
|
54.2 | 35.2 | 50.3 |
VPN |
|
|
60.8 | 43.8 | 53.5 |
VPN+SSTA-PRS(ours) |
|
|
65.2 | - | 54.1 |
LSTM |
|
42.5 | 13.4 | 17.2 | |
MS-AAGCN |
|
56.5 | - | - | |
2s-AGCN |
|
57.1 | 22.1 | 49.7 | |
2s-AGCN+SSTA-PRS(ours) |
|
60.9 | 22.5 | 53.5 |
8.7 Tattoo Fusion emotion recognition through a Tattoo-based wearable and multimodal Fusion
Participants: Laura M. Ferrari, François Brémond, Esma Ismailova, Susanne Thummler.
The Tattoo Fusion is a highly multidisciplinary project to foster emotion recognition. It bridges together biomedical engineering, material science, deep learning and psychology. The main goal is the implementation of next-generation fusion model, on multimodal data. The first objective is the development of a multimodal acquisition platform made of a seamless tattoo-based wearable, for biosignals recording, and cameras. This approach will improve the state-of-the-art in emotions recognition in terms of accuracy and experimental paradigm. The experimental paradigm is detailed in the ethical protocol where multiple Use Cases (UCs) are defined in order to elicit and record emotions. In the design thinking approach, together with the UCs, we define the User Requirements (UR) and stakeholders need, through a questionary and proficient discussion with clinicians, with respect to the tattoo wearable. Tattoo electronics is a cutting edge approach in the field of cutaneous sensing. It offers an imperceptible interface with the skin, while guaranteeing high signal quality, long-lasting recording and being easy to use. Tattoo-based devices have shown their capabilities in multiple fields, with the main application in human health biomonitoring 19. Here the innovative approach is to integrate novel human biomonitoring technology with advanced signal processing on a same platform to translate lab-scale development in real-life usefulness.
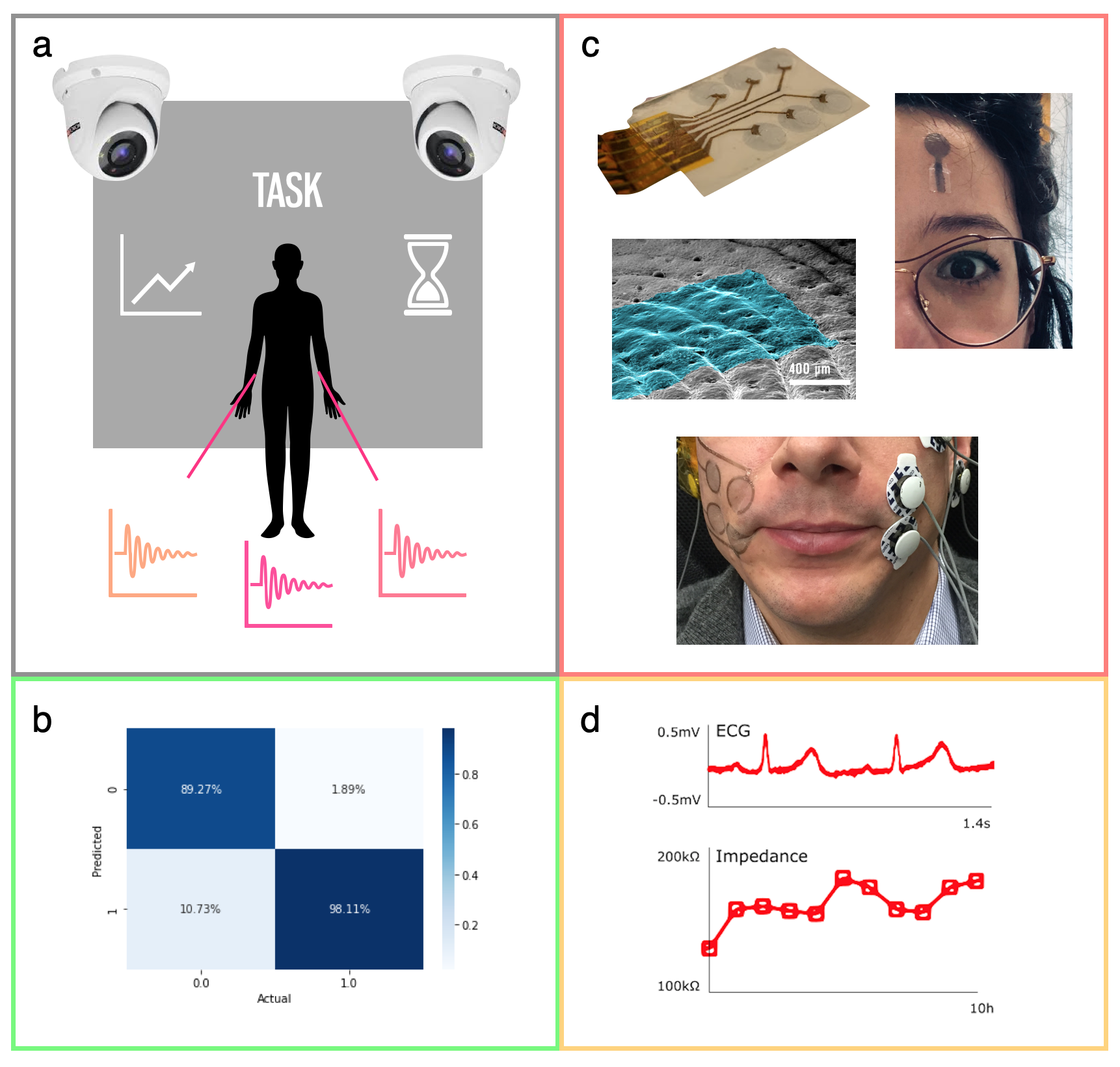
8.8 GAN: Disentangling Appearance and Motion for Video Generation
Participants: Yaohui Wang, François Brémond, Antitza Dantcheva.
Creating realistic human videos entails the challenge of being able to simultaneously generate both appearance, as well as motion. To tackle this challenge, we introduced in 37 GAN (see Fig. 11), a novel spatio-temporal generative model, which seeks to capture the distribution of high dimensional video data and to model appearance and motion in disentangled manner. The latter is achieved in an extension of our work 38 by decomposing appearance and motion in a three-stream Generator, where the main stream aims to model spatio-temporal consistency, whereas the two auxiliary streams augment the main stream with multi-scale appearance and motion features, respectively. An extensive quantitative and qualitative analysis are shown in Tab. 5 that our model systematically and significantly outperforms state-of-the-art methods on the facial expression datasets MUG and UvA-NEMO, as well as the Weizmann and UCF101 datasets on human action. Additional analysis on the learned latent representations confirms the successful decomposition of appearance and motion.
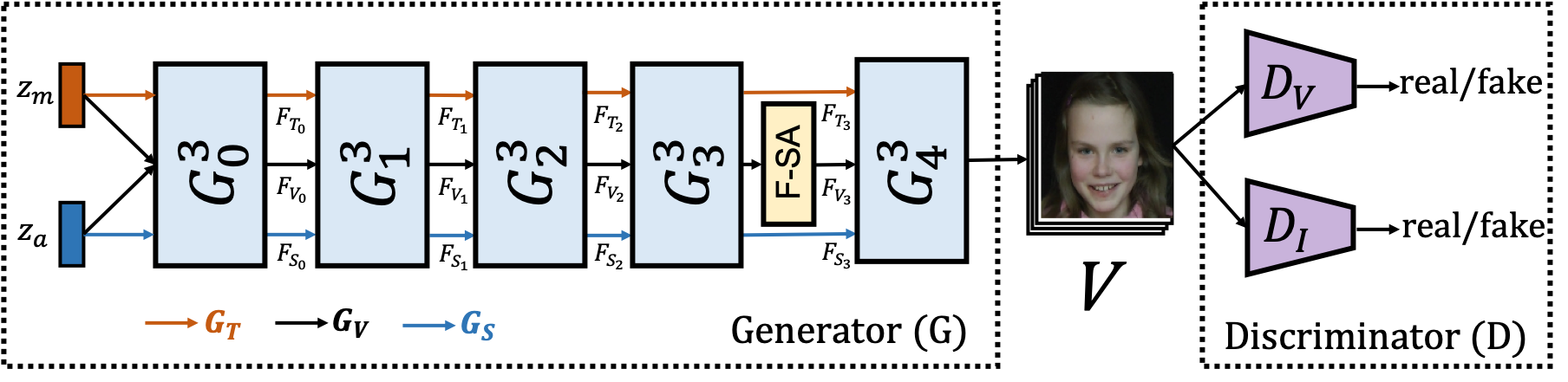
MUG | UvA | Weizmann | UCF101 | ||
FID | FID | FID | FID | IS | |
VGAN | 160.76 | 235.01 | 158.04 | 115.06 | 2.94 |
TGAN | 97.07 | 216.41 | 99.85 | 110.58 | 2.74 |
MoCoGAN | 87.11 | 197.32 | 92.18 | 104.14 | 3.06 |
GAN | 67.12 | 119.22 | 86.01 | 91.21 | 3.62 |
8.9 Comparing 3DCNN approaches for detecting deepfakes
Participants: Yaohui Wang, Antitza Dantcheva.
Manipulated images and videos have become increasingly realistic due to the tremendous progress of deep convolutional neural networks (CNNs). While technically intriguing, such progress raises a number of social concerns related to the advent and spread of fake information and fake news. Such concerns necessitate the introduction of robust and reliable methods for fake image and video detection. Towards this in this work 39, we study the ability of state of the art video CNNs including 3D ResNet, 3D ResNeXt, and I3D in detecting manipulated videos. We present related experimental results on videos tampered by four manipulation techniques, as included in the FaceForensics++ dataset. We investigate three scenarios, where the networks are trained to detect (a) all manipulated videos, as well as (b) separately each manipulation technique individually. Finally and deviating from previous works, we conduct cross-manipulation results, where we (c) detect the veracity of videos pertaining to manipulation-techniques not included in the train set. Our findings clearly indicate the need for a better understanding of manipulation methods and the importance of designing algorithms that can successfully generalize onto unknown manipulations.
8.10 Demographic Bias in Biometrics: A Survey on an Emerging Challenge
Participants: Antitza Dantcheva.
Systems incorporating biometric technologies have become ubiquitous in personal, commercial, and governmental identity management applications. Both cooperative (e.g., access control) and non cooperative (e.g., surveillance and forensics) systems have benefited from biometrics. Such systems rely on the uniqueness of certain biological or behavioral characteristics of human beings, which enable for individuals to be reliably recognized using automated algorithms. Recently, however, there has been a wave of public and academic concerns regarding the existence of systemic bias in automated decision systems (including biometrics). Most prominently, face recognition algorithms have often been labeled as “racist” or “biased” by the media, non governmental organizations, and researchers alike. The main contributions of this article 18 are: 1) an overview of the topic of algorithmic bias in the context of biometrics; 2) a comprehensive survey of the existing literature on biometric bias estimation and mitigation; 3) a discussion of the pertinent technical and social matters; and 4) an outline of the remaining challenges and future work items, both from technological and social points of view.
8.11 Spatio-Temporal Attention Mechanism for Activity Recognition
Participants: Srijan Das, François Brémond, Monique Thonnat.
This is a thesis work that targets recognition of human actions in videos. Action recognition is a complicated task in the field of computer vision due to its high complex challenges. With the emergence of deep learning and large scale datasets from internet sources, substantial improvements have been made in video understanding. For instance, state-of-the-art 3D convolutional networks like I3D pre-trained on huge datasets like Kinetics have successfully boosted the recognition of actions from internet videos. But, these networks with rigid kernels applied across the whole space-time volume cannot address the challenges exhibited by Activities of Daily Living (ADL).
We are particularly interested in discriminative video representation for ADL. Besides the challenges in generic videos, ADL exhibits - (i) fine-grained actions with short and subtle motion like pouring grain and pouring water, (ii) actions with similar visual patterns differing in motion patterns like rubbing hands and clapping, and finally (iii) long complex actions like cooking. In order to address these challenges, we have made three key contributions.
The first contribution includes - a multi-modal fusion strategy to take the benefits of multiple modalities into account for classifying actions. However the question remains, how to combine multiple modalities in an end-to-end manner? How can we make use of the 3D information to guide the current state-of-the-art RGB networks for action classification? To this end, we propose articulated pose driven attention mechanisms for action classification. We propose, three variants of spatio-temporal attention mechanisms exploiting RGB and 3D pose modalities to address the aforementioned challenges (i) and (ii) for short actions. Our third main contribution is a Temporal Model on top of our attention based model. The video representation retaining dense temporal information enables the temporal model to model long complex actions which is crucial for ADL. This third contribution has been published in WACV 2020 33.
We have evaluated our first contribution on three small-scale public datasets: CAD-60, CAD-120 and MSRDailyActivity3D. On the other hand, we have evaluated our remaining two contributions on four public datasets: a large scale human activity dataset: NTU-RGB+D 120, its subset NTU-RGB+D 60, a real-world challenging human activity dataset: Toyota Smarthome and a small scale human-object interaction dataset Northwestern UCLA. Our experiments show that the methods proposed in this thesis outperform the state-of-the-art results. This Thesis has been defended on 1 October 2020 and is available online 41.
8.12 VPN: Learning Video-Pose Embedding for Activities of Daily Living
Participants: Srijan Das, Saurav Sharma, Rui Dai, François Brémond, Monique Thonnat.
In this work, we focus on the spatio-temporal aspect of recognizing Activities of Daily Living (ADL). ADL have two specific properties (i) subtle spatio-temporal patterns and (ii) similar visual patterns varying with time. Therefore, ADL may look very similar and often necessitate to look at their fine-grained details to distinguish them. Because the recent spatio-temporal 3D ConvNets are too rigid to capture the subtle visual patterns across an action, we propose a novel Video-Pose Network: VPN as depicted in fig. 12. The 2 key components of this VPN are a spatial embedding and an attention network. The spatial embedding projects the 3D poses and RGB cues in a common semantic space. This enables the action recognition framework to learn better spatio-temporal features exploiting both modalities. In order to discriminate similar actions, the attention network provides two functionalities - (i) an end-to-end learnable pose backbone exploiting the topology of human body, and (ii) a coupler to provide joint spatio-temporal attention weights across a video. Experiments1 show that VPN outperforms the state-of-the-art results for action classification on a large scale human activity dataset: NTU-RGB+D 120, its subset NTU-RGB+D 60, a real-world challenging human activity dataset: Toyota Smarthome and a small scale human-object interaction dataset Northwestern UCLA. This work has been accepted in ECCV 2020 32.
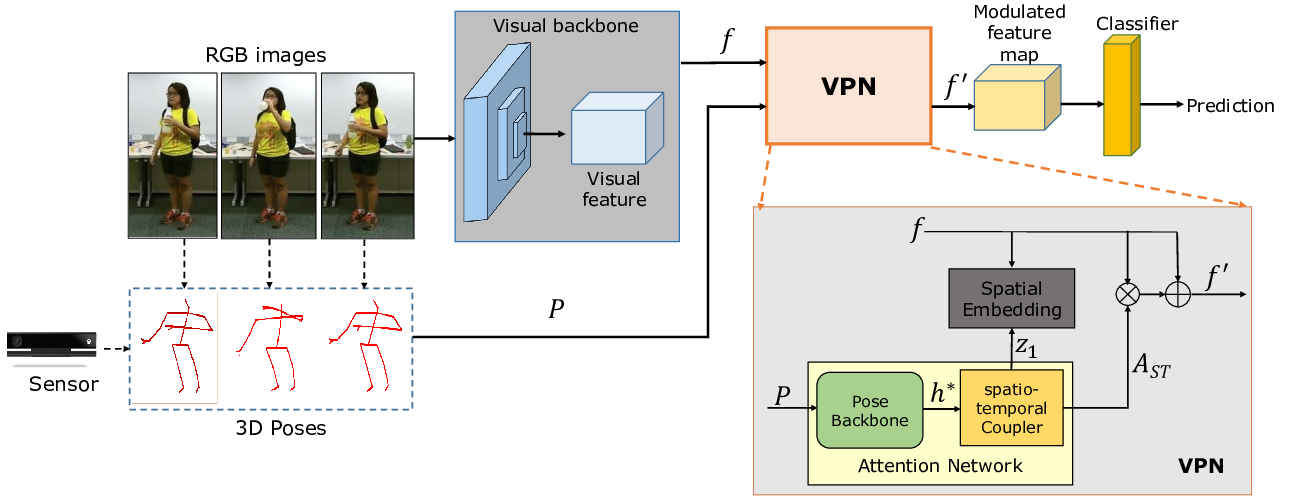
8.13 PDAN: Pyramid Dilated Attention Network for Action Detection
Participants: Rui Dai, Srijan Das, François Brémond.
Handling long and complex temporal information is an important challenge for action detection tasks. This challenge is further aggravated by densely distributed actions in untrimmed videos. Previous action detection methods fail in selecting the key temporal information in long videos. To this end, we introduce the Dilated Attention Layer (DAL), see Fig. 13. Compared to previous temporal convolution layer, DAL allocates attentional weights to local frames in the kernel, which enables it to learn better local representation across time. Furthermore, we introduce Pyramid Dilated Attention Network (PDAN) which is built upon DAL, see Fig. 14. With the help of multiple DALs with different dilation rates, PDAN can model short-term and long-term temporal relations simultaneously by focusing on local segments at the level of low and high temporal receptive fields. This property enables PDAN to handle complex temporal relations between different action instances in long untrimmed videos. To corroborate the effectiveness and robustness of our method, we evaluate it on three densely annotated, multi-label datasets: MultiTHUMOS, Charades and Toyota Smarthome Untrimmed (TSU) dataset. PDAN is able to outperform previous state-of-the-art methods on all these datasets. This work is accepted in Winter Conference on Applications of Computer Vision 2021 (WACV 2021) 31.
8.14 TSU: Toyota Smarthome Untrimmed
Participants: Rui Dai, Srijan Das, François Brémond.
Designing activity detection systems that can be successfully deployed in daily-living environments require datasets that characterize the challenges typical of real-world settings. In this work, we introduce a new untrimmed daily-living dataset that features several real-world challenges: Toyota Smarthome Untrimmed (TSU). TSU contains a wide variety of activities performed in a spontaneous manner. Activities are collected in real-world settings, which results in non-optimal viewpoints. The dataset contains dense annotations including elementary, composite activities and activities involving interaction with objects. Fig. 15 provides an analysis of activities in TSU. We provide an analysis of the real-world challenges featured by TSU dataset, highlighting the open issues for detection algorithms. We show that the current state-of-the-art methods fail to achieve satisfactory performance on TSU dataset. We release the dataset for research use at https://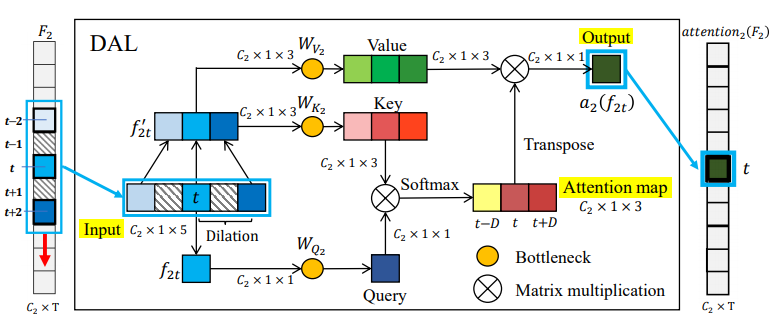
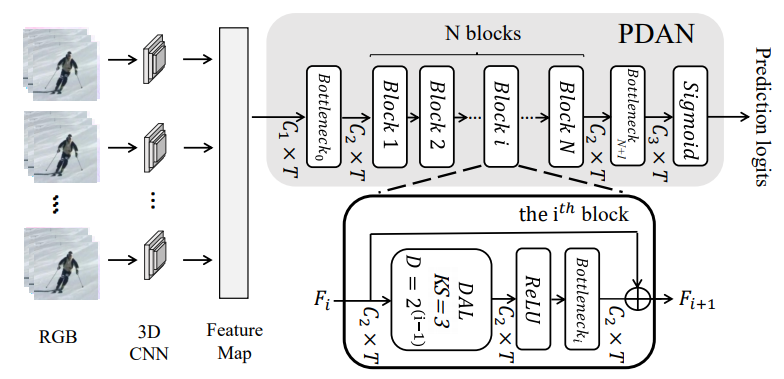
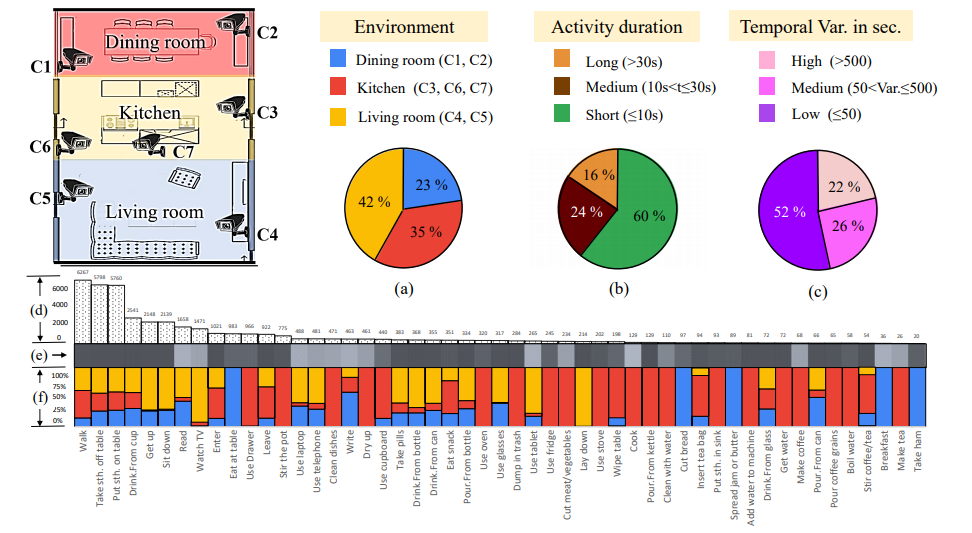
8.15 Quantified Analysis for Epileptic Seizure Videos
Participants: Jen-Cheng Hou, Monique Thonnat.
Epilepsy is a type of neurological disorder, affecting around 50 million people worldwide. Epilepsy's main symptoms are seizures, which are caused by abnormal neuronal activities in the brain. To determine appropriate treatments, neurologists assess manifestation of patients' behavior when seizures occur. Nevertheless, there are few objective criteria regarding the procedure, and diagnosis could be biased due to subjective evaluation. Hence it is important to quantify patients' ictal behaviors for better assessment of the disorder. In collaboration with Prof. Fabrice Bartolomei and Dr. Aileen McGonigal from Timone Hospital, Marseille, we have access to video recordings from epilepsy monitoring unit for analysis, with consent from ethics committee (IRB) and the patients involved.
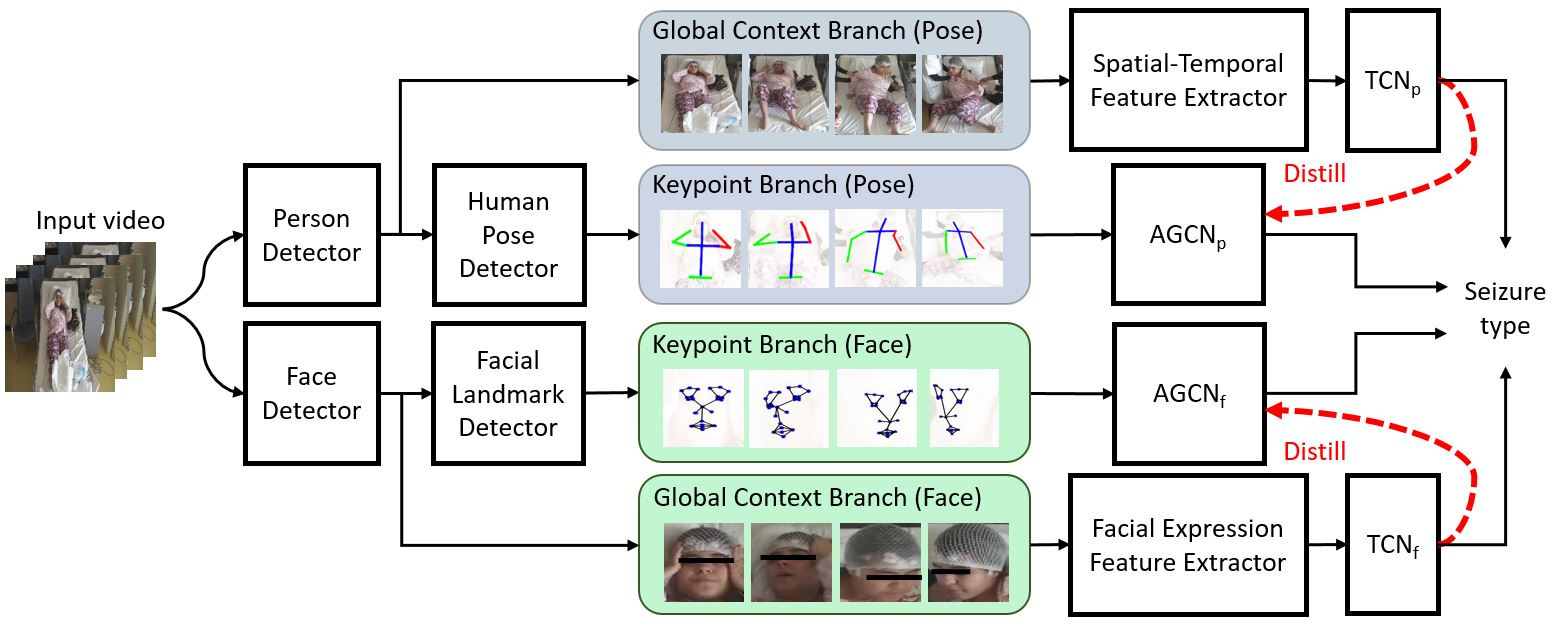
8.15.1 A Multimodal Approach for Seizure Classification with Knowledge Distillation
In clinical epilepsy practice, a common diagnostic challenge is distinguishing between patients with epileptic seizures and those with psychogenic non-epileptic seizures. Accurate diagnosis is important in order to implement appropriate clinical management, and often relies on visual analysis of seizures recorded on video in the hospital setting. In this work, we propose a multimodal approach with knowledge distillation to classify these two types of seizures. As shown in Figure 16, the proposed framework is based on deep learning models and utilizes multimodal information from keypoints and appearance from both body and face. Inspired by recent success of graph convolutional networks (GCNs) in various tasks, we take the detected keypoints through time as spatio-temporal graph and train it with an adaptive GCN method (AGCN) to model the spatio-temporal dynamics throughout the seizure event. Besides, we regularize the keypoint features with complementary information from the appearance stream by imposing a knowledge distillation mechanism (KD). We demonstrate the effectiveness of our approach by conducting experiments on real-world seizure videos and compare it with several baseline methods from related works. We performed experiments on real patients with 61 seizures. The experiments are conducted by seizure-wise cross validation, and with the proposed model, the performances of the F1-score and the accuracy are 0.94 and 0.92, respectively, as shown in Table 6. This work is currently under review.
model | F1-score | accuracy | |
Karacsony et al.,2020 | 0.81 | 0.77 | |
Ahmedt-Aristizabal et al., 2018 (pose) | 0.87 | 0.84 | |
Ahmedt-Aristizabal et al., 2018 (face) | 0.85 | 0.79 | |
0.85 | 0.80 | ||
0.84 | 0.77 | ||
0.80 | 0.74 | ||
0.85 | 0.79 | ||
+KD | 0.91 | 0.89 | |
+KD | 0.89 | 0.87 | |
Ensemble | 0.94 | 0.92 |
8.15.2 Neural correlates of rhythmic rocking in prefrontal seizures
In our previous study 22, we analyzed several prefrontal seizures with rhythmic rocking features based on their head movement trajectories. In collaboration with researchers from Aix Marseille University, neural correlates was found on the same study cases by analyzing the frequencies between EEG signals and patients' rocking movement. This work has been published in 26.
8.16 Apathy Classification by Exploiting Task Relatedness
Participants: Antitza Dantcheva, Philippe Robert, François Brémond.
Apathy is characterized by symptoms such as reduced emotional response, lack of motivation, and limited social interaction. Current methods for apathy diagnosis require the patient's presence in a clinic and time consuming clinical interviews, which are costly and inconvenient for both patients and clinical staff, hindering among others large-scale diagnostics. In this work 35 we propose a multi-task learning (MTL) framework for apathy classification based on facial analysis, entailing both emotion and facial movements. In addition, it leverages information from other auxiliary tasks (i.e., clinical scores), which might be closely or distantly related to the main task of apathy classification. Our proposed MTL approach (termed MTL+) improves apathy classification by jointly learning model weights and the relatedness of the auxiliary tasks to the main task in an iterative manner. Instead of subjective assignment of weights for each task, MTL+ learns the task relatedness directly from the data. Our approach caters to the challenging setting of current apathy assessment interviews, which include short video clips with wide face pose variations, very low-intensity expressions, and insignificant inter-class variations. Our results on 90 video sequences acquired from 45 subjects obtained an apathy classification accuracy of up to , using the concatenated emotion and motion features. Our results further demonstrate the improved performance of MTL+ over MTL.
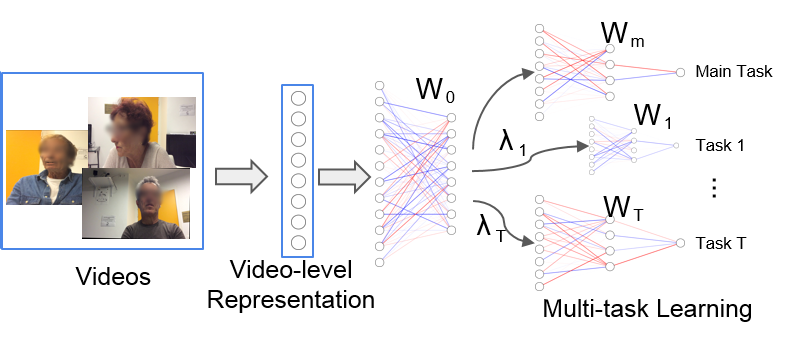
Features used | Accuracy | F1-score |
MTL with Motion Features | 62.22 | 0.582 |
MTL with Emotion features | 66.66 | 0.638 |
MTL with Emotion + Motion Features | 71.11 | 0.716 |
MTL+ with Motion Features | 71.11 | 0.679 |
MTL+ with Emotion features | 77.77 | 0.776 |
MTL+ with Emotion + Motion Features | 80.00 | 0.786 |
8.17 A weakly supervised learning technique for classifying facial expressions
Participants: Antitza Dantcheva, François Brémond.
Expression recognition remains challenging, predominantly due to (a) lack of sufficient data, (b) subtle emotion intensity, (c) subjective and inconsistent annotation, as well as due to (d) in-the-wild data containing variations in pose, intensity, and occlusion. To address such challenges in a unified framework 34, we propose a self-training based semi-supervised convolutional neural network (CNN) framework, which directly addresses the problem of (a) limited data by leveraging information from unannotated samples. Our method uses ‘successive label smoothing’ to adapt to the subtle expressions and improve the model performance for (b) low-intensity expression samples. Further, we address (c) inconsistent annotations by assigning sample weights during loss computation, thereby ignoring the effect of incorrect ground truth. We observe significant performance improvement in in-the-wild datasets by leveraging the information from the in-the-lab datasets, related to challenge (d). Associated to that, experiments on four publicly available datasets demonstrate large performance gains in cross-database performance, as well as show that the proposed method achieves to learn different expression intensities, even when trained with categorical samples.
8.18 Expression recognition with deep features extracted from holistic and part-based models
Participants: Antitza Dantcheva, François Brémond.
Facial expression recognition aims to accurately interpret facial muscle movements in affective states (emotions). Previous studies have proposed holistic analysis of the face, as well as the extraction of features pertained only to specific facial regions towards expression recognition. While classically the latter have shown better performances, we here explore this in the context of deep learning. In particular, this work 21 provides a performance comparison of holistic and part-based deep learning models for expression recognition. In addition, we showcase the effectiveness of skip connections, which allow a network to infer from both low and high-level feature maps. Our results suggest that holistic models outperform part-based models, in the absence of skip connections. Finally, based on our findings, we propose a data augmentation scheme, which we incorporate in a part-based model. The proposed multi-face multi-part (MFMP) model leverages the wide information from part-based data augmentation, where we train the network using the facial parts extracted from different face samples of the same expression class. Extensive experiments on publicly available datasets show a significant improvement of facial expression classification with the proposed MFMP framework.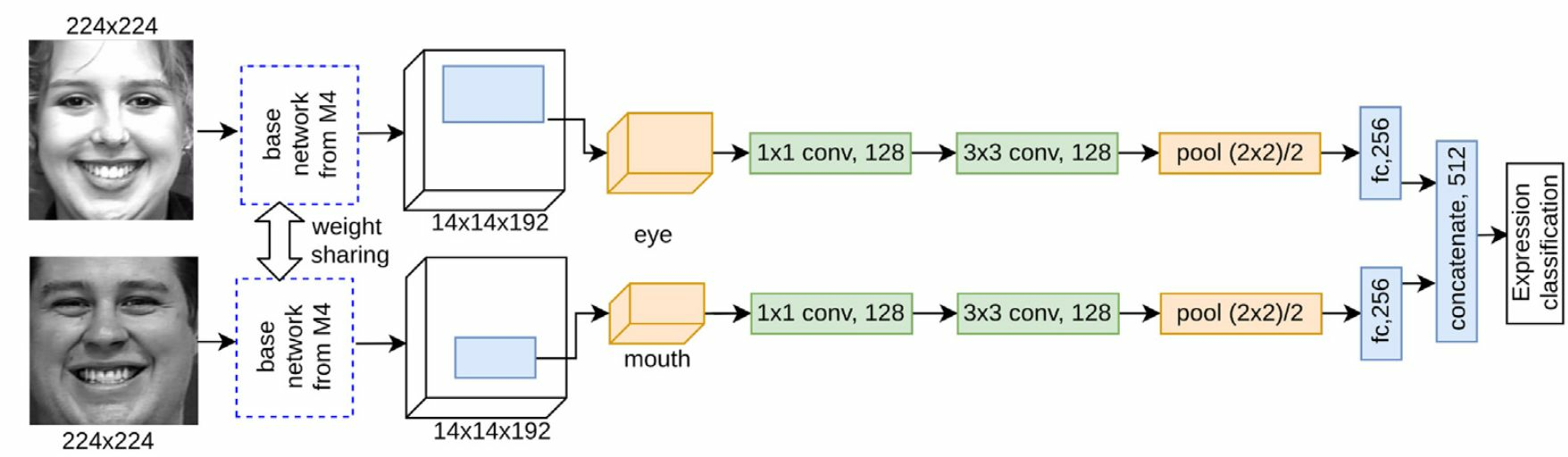
8.19 Probabilistic Model Checking for Activity Recognition in Medical Serious Games
Participants: Elisabetta De Maria, Sabine Moisan, Jean-Paul Rigault, Thibaud L'Yvonnet.
We propose a formal approach based on probabilistic discrete-time Markov chains (DTMC) to model human activities 17. Important properties of such models can be automatically verified thanks to model checking. We applied this approach to patients playing serious games in the medical field. The goal is to differentiate their level of damages among different cognitive functions.
We identified three prospective applications of our approach. First, to evaluate a new patient before the first diagnosis of doctors, we can compare her game performance to a reference model representing a "healthy" behavior. Second, to monitor known patients, a customized model can be created according to their first results, and, over time, their health improvement or deterioration can be monitored. Finally, to pre-select a cohort of patients, a reference model can determine, in a fast way, whether a new group of patients belongs to a specific category.
We selected three serious games for Alzheimer patients. 1) The Code game tests visual attention. After a first version with fixed probabilities associated with patient actions along a whole game session, we implemented another version in which time dependent probabilities are pre-computed to take into account the patient's fatigue over time. This version is more realistic to represent the behavior of a patient. 2) The Recognition game targets episodic memory. Its model was directly implemented with pre-computed time dependent probabilities and we also divided it into several modules to facilitate the modeling task. This model requires the use of the explicit model-checking engine of PRISM, the only one able to cope with its big state space (because not all states are attainable). Regardless of this constraint, we succeeded in obtaining meaningful properties for medical monitoring. 3) The third game, which addresses the inhibitory control function, was the most difficult to model. This difficulty also pushed PRISM to its limits as the time for model-checking is rather longer than for the two first games. However, we obtained readable and meaningful results depicting the variants that can be found in a patient behavior.
We encoded the three games as DTMCs in PRISM, and we tested meaningful PCTL* (Probabilistic Computation Tree Logic) properties thanks to the PRISM model checkers. As an example, Figure 19 displays a diagram obtained with the "run experiment" tool of PRISM on a temporal logic property of the last game. This diagram summarizes the evolution of the modeled patient's good or bad actions in time. At the beginning of the game (between action 0 and 2), a short training phase is proposed. The diagram clearly shows the good effect of this training phase (between action 2 and 6) but, starting from action 10, the modeled patient does more bad actions than good ones.
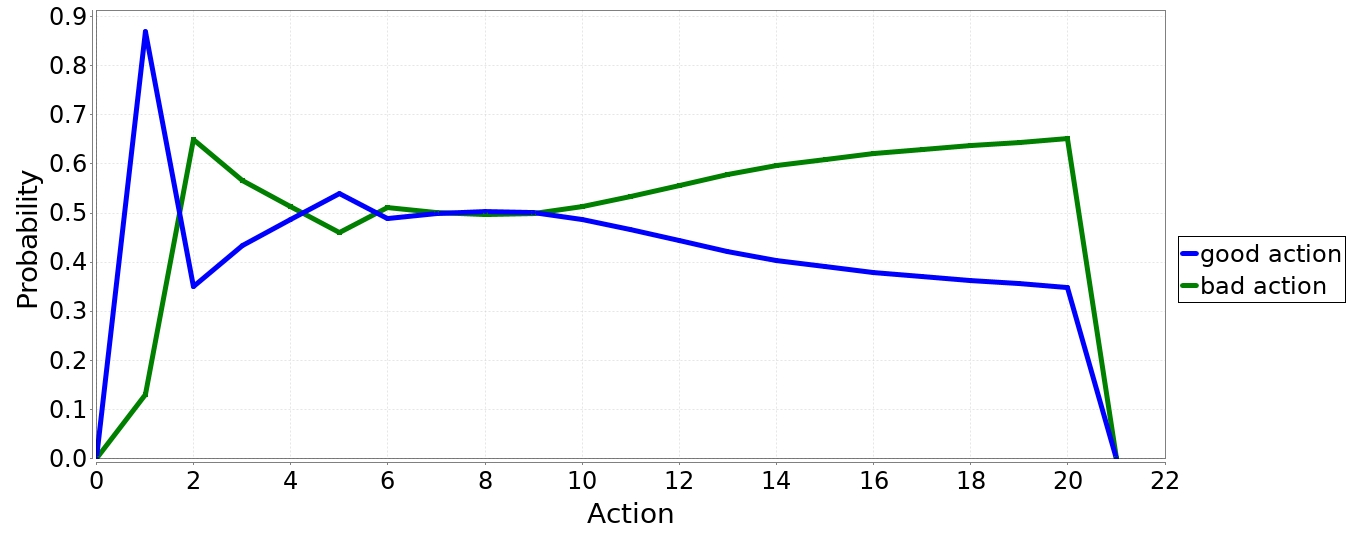
Modeling these games allowed us to validate our approach and to test its scalability for rather different applications. The results encourage us to pursue our research on behavior modeling for patient analysis.
The probabilities in our models are initially given by medical practitioners and need to be updated according to real clinical experimentation results, in order to obtain a more realistic model and to provide a more accurate prediction. To this end, we set up models for different profiles (such as mild, moderate or severe Alzheimer) with the help of clinicians and we proposed a medical protocol that started a few months ago. Two groups of people will play the three games over a period of nine months: a control group with no known cognition deficit, and a patient group with an identified medium cognition deficit. All game data (scores, answers, and response times) as well as video recordings (focused only on the hands of the participants) will be recorded and anonymized. These results will be used to adjust probabilities in the models in order to obtain a better representation of the behavior variants and to make our models effective.
These results will be published in the Science of Computer Programming (SCP) Journal.
8.20 MePheSTO – Digital Phenotyping 4 Psychiatric Disorders from Social Interaction
Participants: Alexandra König, Tanay Agrawal, Michal Balazia, Philippe Robert, François Brémond.
MePheSTO is an interdisciplinary research project that envisions a scientifically sound methodology based on artificial intelligence methods for the identification and classification of objective, and thus measurable, digital phenotypes of psychiatric disorders. MePheSTO has a solid foundation of clinically motivated scenarios and use-cases synthesized jointly with clinical partners. Important to MePheSTO is the creation of a multimodal corpus including speech, video, and biosensors of social patient-clinician interactions, which serves as the basis for deriving methods, models and knowledge. Important project outcomes include technical tools and organizational methods for the management of medical data that implement both ELSI and GDPR requirements, demonstration scenarios covering patients' journeys including early detection, diagnosis support, relapse prediction, therapy support, an annotated corpus, Ph.D. theses, and publications. MePheSTO builds a joint DFKI-Inria workforce – the foundation for future RD and innovation projects.
8.21 DeepSPA - Early detection of cognitive disorders such as dementia on the basis of speech analysis
Participants: Alexandra König, Philippe Robert, François Brémond.
Hinging on recent advances in automatic speech analysis and computational linguistics as well as image-based behavioral analysis, the DeepSpa project aims to explore the use of a telecommunication-based system empowered by artificial intelligence (AI) to facilitate large scale population-based pre-screening and monitoring of potential trial participants. For this, the objective is to validate a semi-automated telephone tool for neurocognitive pre-screening and pre-selection of participants of clinical trials targeting various neurodegenerative diseases (Use case 1). In addition, a videoconference system is used for remote disease monitoring, e.g. patients will be assessed at their own homes (Use case 2).
In two different sites (Maastricht University-Use case 1 /Inria- Use case 2) clinical validation studies were performed with 180 participants in total to assess the feasibility and usability of such phone and telecommunication-based remote neurocognitive assessment. It consists of a short interview on how the participants perceive their memory, and overall mental state, a verbal (visual) memory task, and fluency tasks. The predictive potential of information extracted from the participant’s speech and image during cognitive and narrative tasks are examined. Longitudinal and cross-sectional data is collected and results extracted remotely validated against face-to-face results. Moreover, the degree to which participants experience the phone/teleconference system-based as satisfactory as a F2F assessment will be evaluated with the help of qualitative interview at the end of the study.
We were mainly in charge of use case 2 and its study which took place in Digne-les-bains. In the tables below the demographic characteristics of the included participants are shown and their comparison results between face to face and video-conference -based assessments results.
Total | |
Number | 39 |
Age, M SD | 73.44 10.34 |
Sex. % Male | 36% |
Education | 27.6 2.3 |
Primary, n (%) | 14; 36% |
Secondary, n (%) | 12; 36% |
High, n (%) | 13; 31% |
Average period between assessments, day (sd) | 16.5 3.71 |
Cognitive functions and tests | VC | F2F | Correlation | Sig. | |||
M | SD | M | |||||
Global cognitive | MMSE | 26.46 | 3.66 | 28.23 | 2.22 | 0.555 | 0.000 |
functioning | |||||||
Memory (FSCRT) | Total recall | 43.74 | 4.79 | 43.45 | 5.7 | 0.657 | 0.000 |
Delayed recall | 15.09 | 1.71 | 15.00 | 2.10 | 0.278 | 0.136 | |
Recognition score | 15.62 | 1.72 | 15.63 | 1.18 | -0.068 | 0.726 | |
Naming task | Lexis total score | 56.40 | 6.12 | 58.37 | 5.21 | 0.893 | 0.000 |
Inhibition (Stroop) | Color, duration(s) | 76.78 | 19.87 | 71.28 | 13.84 | 0.370 | 0.048 |
Verbal Fluencies | P | 19.61 | 7.46 | 22.37 | 8.08 | 0.695 | 0.000 |
Animals | 25.29 | 9.56 | 26.42 | 7.91 | 0.861 | 0.006 | |
SVFz | -0.20 | 1.34 | 0.55 | 3.85 | -0.021 | 0.909 | |
PVFz | -0.05 | 1.15 | 0.21 | 0.92 | 0.323 | 0.062 | |
Praxis | Total score | 20.15 | 2.42 | 22.24 | 1.58 | 0.650 | 0.000 |
Preliminary findings
Similar results were obtained on the several cognitive test measures when comparing the remote to the face-to-face administration method. Picture naming task (r=,893), semantic verbal fluency (animals) (r=,861) and total word recall (r=,657) showed the strongest correlations. Acceptability of the tool was relatively high with preference for certain participants even in the remote method for more convenience.
User experience
Based on the System Usability Scale (SUS), a questionnaire was developed and administered to all participants in order to assess their level of satisfaction and comfort during the video conference-based testing administration. Moreover, several conversations between the research nurse; the patients and the psychologists revealed how the participants experienced this new method. We did a first preliminary analysis of 21 of the acceptability questionnaires with rather encouraging results. Overall, 16 out of 21 participants rated that they were satisfied with the experience of the video-conference based assessment. Most participants find the system easy to use. When we ask what type of method the participants prefer 41,7
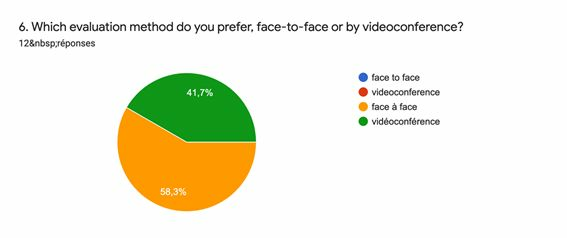
Some more qualitative comments were:
- ”Strangely, I feel more free in front of the computer to express myself”
- “It still feels intimate and personal but this might depend on the clinician”
- “For me it is not different than face to face and I'm used to it.”
- “Not rather pleasantly surprised, puts a distance that is rather facilitating”
Overall, results support the feasibility and reliability of remote cognitive testing through administration via a telemedicine tool. These systems can be used for remote disease monitoring, enabling patients to be assessed in their own homes and improve utilization of expert assessors allowing them to conduct neurocognitive testing remotely.
Demonstration of the effectiveness of this technology may later make it possible to diffuse its use across all rural areas (‘medical deserts’) in France and thus to improve the early diagnosis of neurodegenerative pathologies, while providing data crucial for basic research. Ultimately, it will lead to an improvement of health care access and care of isolated seniors in these regions. Furthermore, recruitment, onboarding and monitoring of potential candidates in these regions for clinical trials will be facilitated.
Related results can be found in the publications 23, 27, 20.
8.22 Activis
Participants: Abid Ali, Sebastien Gilabert, Suzanne Thummler, François Brémond, Monique Thonnat.
In computer science and artificial intelligence, ontology languages are formal languages used to construct ontology. They allow the encoding of knowledge about specific domains and often include reasoning rules that support the processing of that knowledge. An information flow is best described in figure 21. Ontology languages are usually declarative languages, are almost always generalizations of frame languages, and are commonly based on either first-order logic or on description logic. Figure 22 shows information based on event complexity. In our domain we use an ontology language approach for modeling some usual events like Make_Tea or specific medical events as stereotype for autism patients. Our final goal is to use the SUP ontology language (Scene Understanding Platform) combined with deep learning methodologies to develop an automatic ADOS protocol, helping clinical people in diagnosis of autism.
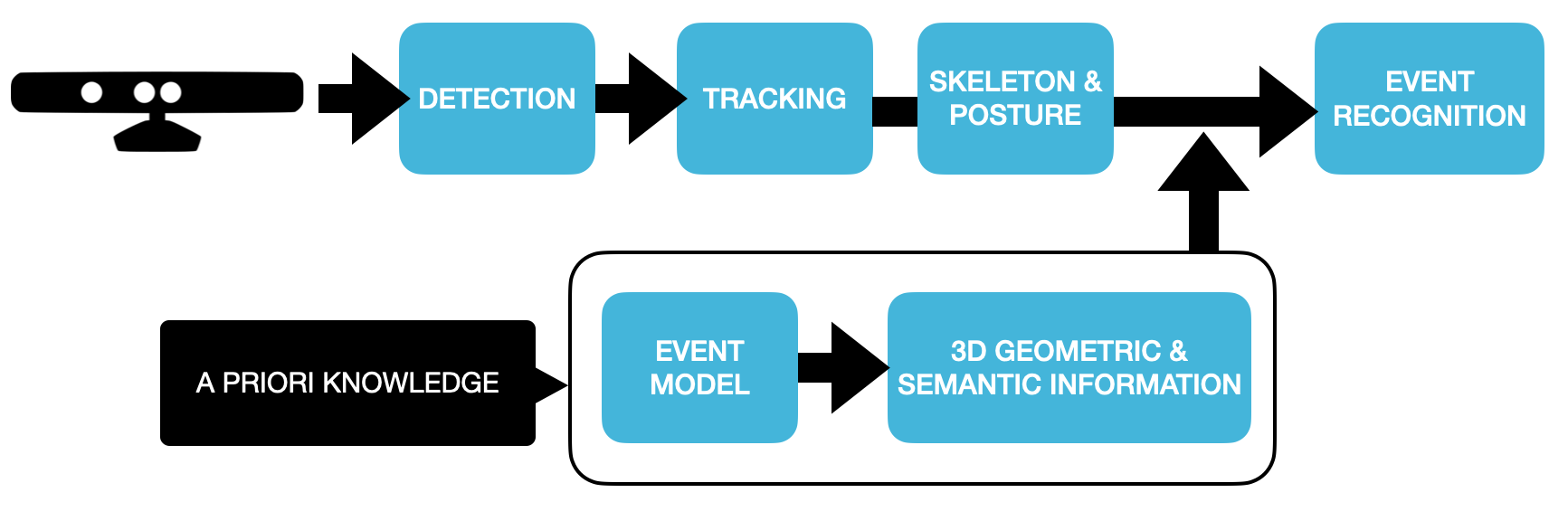
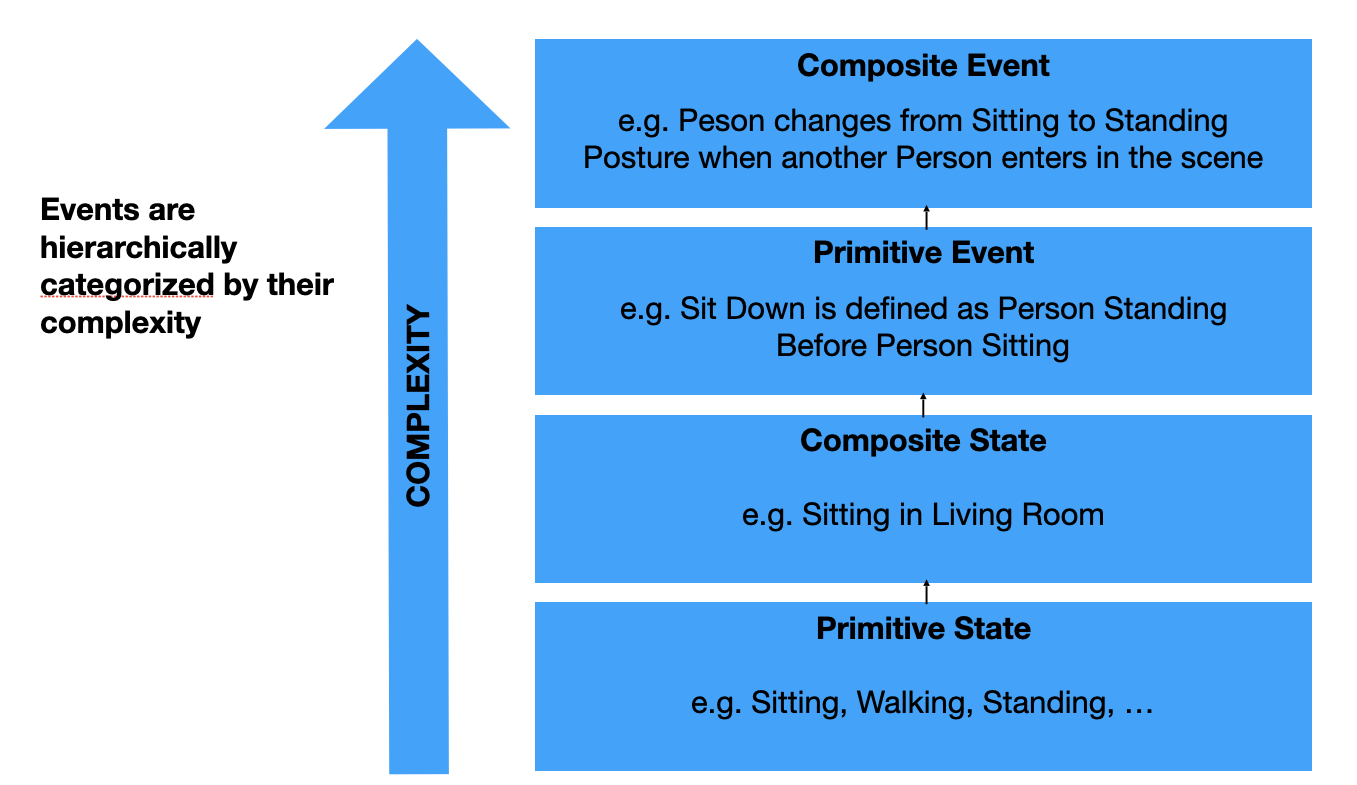
8.22.1 Work Description
Dataset
Initially we collect raw data including approximately 50 videos for Activis project. Each video is 40-50 mins long. Currently, we have 25 total action classes as shown in the figure 23. Based on these action labels we clipped the videos, achieving 586 videos in total, this includes a total of 1001945 frames. At the moment we have an unbalanced dataset, which will be improved later with more data collection and annotations.
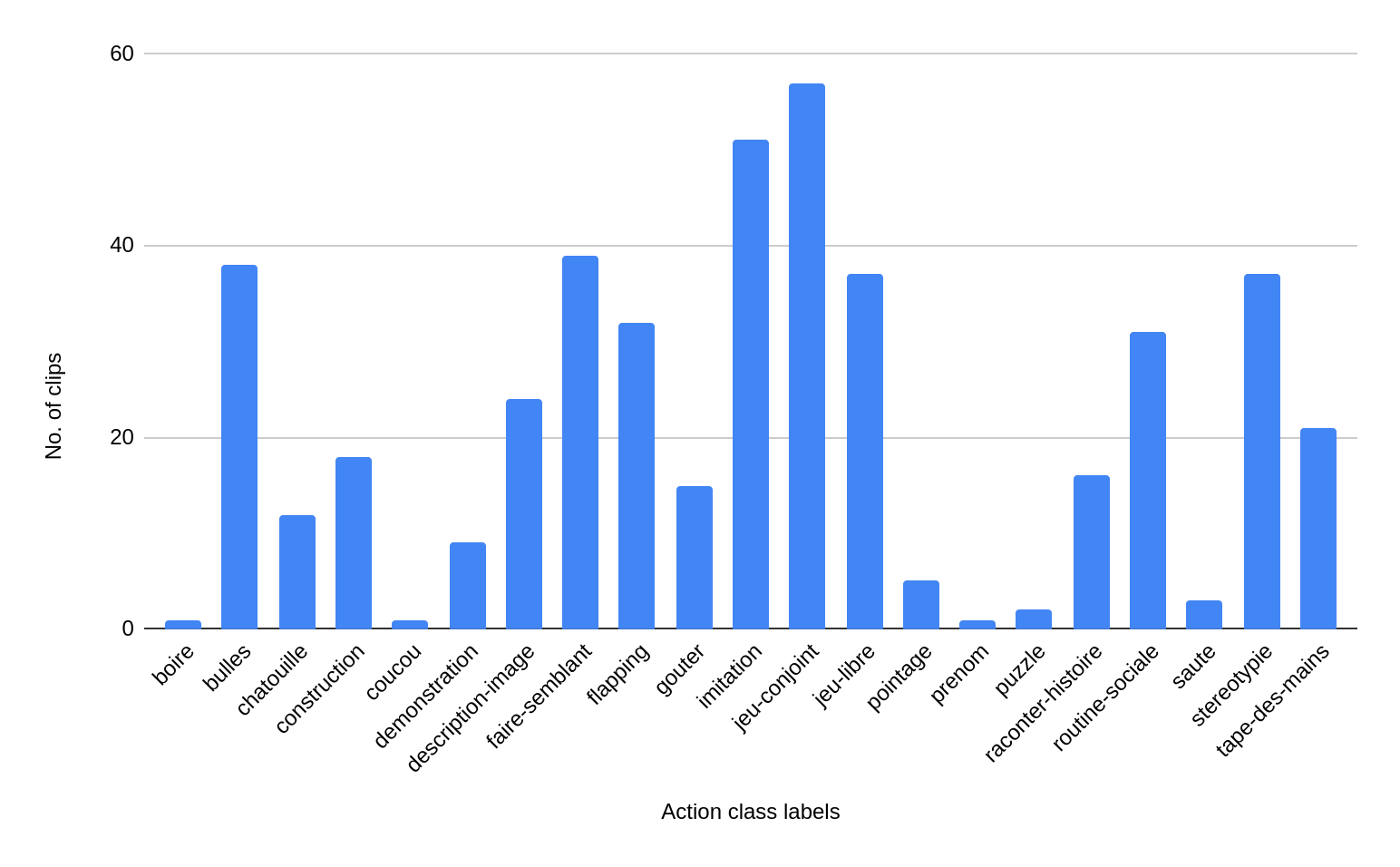
8.22.2 Preprocessing
Before training a good action classifier, the data needs to be preprocessed. Our preprocessing steps involve pose estimation, bounding box generation, tracking and extraction. Currently, we perform person extraction on few clips from the data to perform certain tests. Firstly, we perform 2D and 3D pose estimation using LCRNet9 45 architecture. Bounding boxes were created based on detected poses. These bounding boxes were used to track each person within the video. We used SORT 46 algorithm to track each person (in our case clinician and kid) in each clip. Since, we were interested to use both the clinician and kid for training, both of the persons were extracted.
8.23 E-Santé
Participants: Rachid Guerchouche, Tran Duc Minh, Valeria Manera, Monique Thonnat, François Brémond.
The E-Santé Silver Economy project is a collaborative project within the framework of the FEDER European cross-border cooperation program between France and Italy Interreg ALCOTRA. The aim is to increase innovation projects (especially clusters, centers and companies) - and to develop innovative services at cross-border level.
The E-Santé Silver Economy project tackles the issues of frailty among seniors, more particularly in rural and isolated areas; as well as access to innovations for the area of the ALCOTRA territory, which presents an imbalance in terms of innovation, and access to public services between urban and rural areas. The majority of the population, services and economic activities are concentrated in cities. The aim of the project is therefore to experiment with innovative e-health tools to increase the accessibility of isolated people to care (screening, diagnosis and follow-up), as well as keeping the elderly at home as long as possible, by proposing solutions to delay the decrease in their mental, cognitive and physical capacities. Inria is involved in many tasks in the project:
- Participation in needs definition, including through Focus Groups.
- Proposing technological solutions fitting the needs of the project.
- Organizing the peer review of the project.
- Participation in the Living-Labs.
- Participation in organizing, planning, and executing the experiments on rural areas.
STARS participated in the definition of needs in terms of fighting against the physical and mental frailties of the elderly. Inria proposed a comprehensive classification, which focuses on how to prevent cognitive and physical impairments in elderly. The classification is not only focusing on screening and diagnostic, but also on the follow-up and the delay of autonomy loss. In addition, STARS worked extensively on benchmarking the existing technologies, which can help in answering the defined needs. For each category of needs, a set of representative existing technological solutions were listed and described. Commercialized and on-going research solutions were both investigated. STARS also proposed three technological solutions, which could be experimented in the Living-Labs and/or the field-experiments. Inria worked on adapting its solutions for possible deployment.
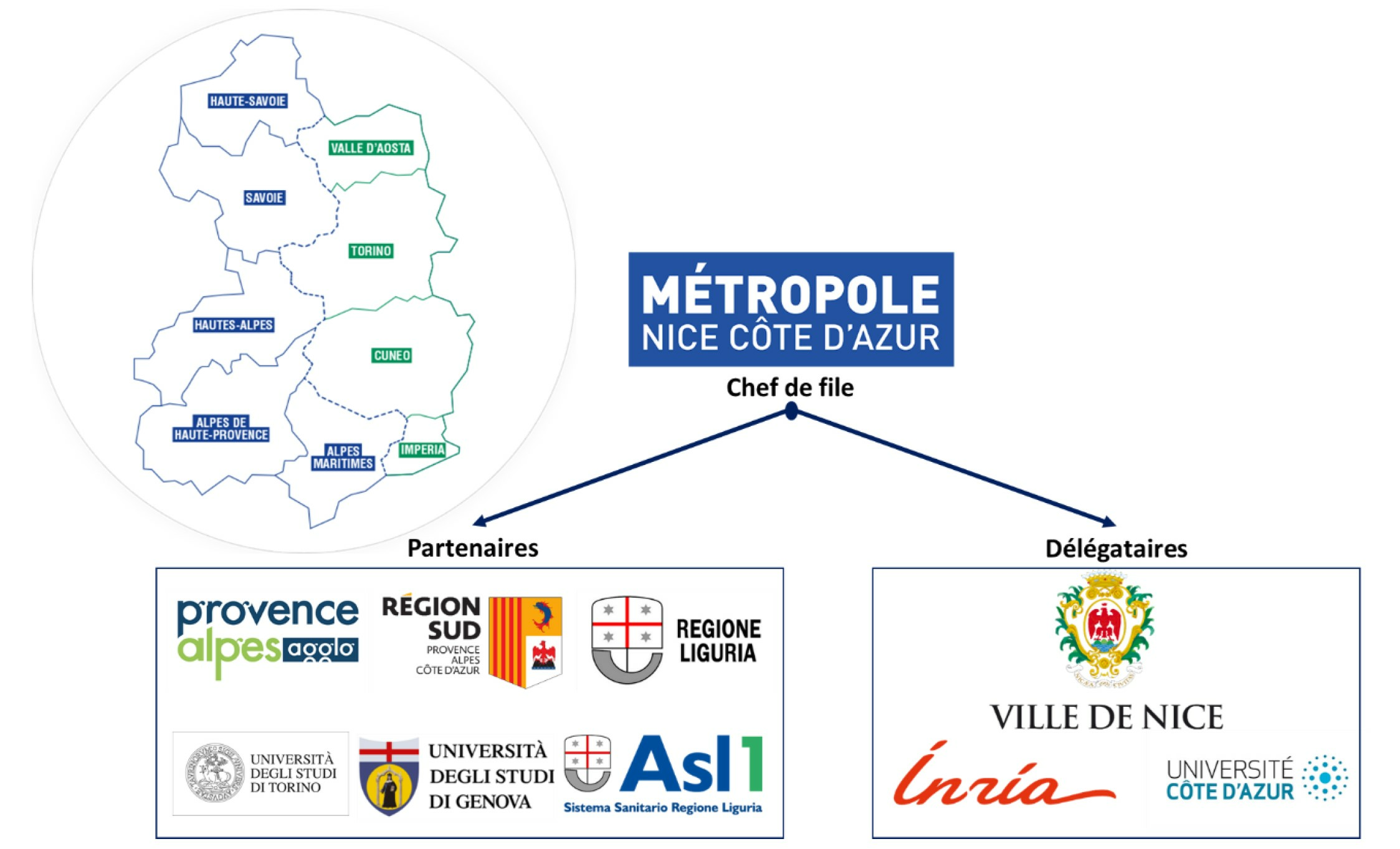
8.24 An Investigative Study on Face Uniqueness
Participants: Michal Balazia, Antitza Dantcheva, François Brémond.
Face recognition has been widely accepted as a means of identification in applications ranging from border control to security in the banking sector. Surprisingly, while widely accepted, we still lack the understanding of uniqueness or distinctiveness of faces as biometric modality. In this work, we study the impact of factors such as image resolution, feature representation, database size, age and gender on uniqueness denoted by the Kullback-Leibler divergence between genuine and impostor distributions (see Figure 25). Towards understanding the impact, we evaluate the datasets AT&T, LFW, IMDb-Face, as well as ND-TWINS, with the feature extraction algorithms VGGFace, VGG16, ResNet50, InceptionV3, MobileNet and DenseNet121, that reveal the quantitative impact of the named factors.
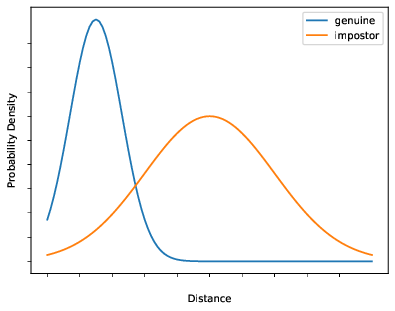
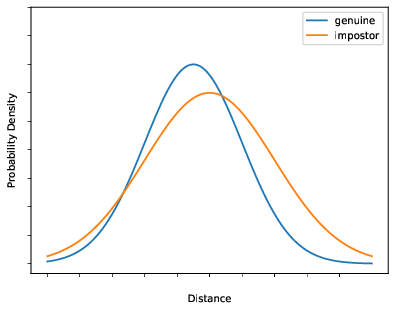
At ICPR'20 28, we presented preliminary results on the impact of these factors on facial uniqueness. We provided clear experimental evidence of decrease in the uniqueness score, in the case that (a) image resolution decreases, (b) a single gender is observed, (c) a smaller age group is observed, (d) a larger dataset is used, as well as (e) different feature extractors are used. We illustrated that while feature representation and dataset size significantly affect the uniqueness score, image resolution has a negligible impact. Further, we proposed an alternative uniqueness estimate, which reflects on the presence of twins. While these are early results, our findings indicate the need for a better understanding of the concept of biometric uniqueness and its implication on face recognition.
8.25 Using Artificial Intelligence for Diagnosis of Psychiatric Disorders
Participants: Michal Balazia, Antitza Dantcheva, François Brémond.
People with psychiatric conditions such as schizophrenia, bipolar disorder, or depression are often disabled for life, have a lower life expectancy, and a higher suicide rate. Anxiety or obsessive-compulsive disorders and substance consumption are common comorbidities. Mission of this research is to develop a digital framework for identification and classification of objective, and thus measurable, digital phenotypes of psychiatric disorders. The framework uses social patient-clinician interaction data to detect acute episodes of illness, to predict the course of the disease, and to support therapy.
Within the whole framework, we specifically focus on developing a computer vision and AI technology for detecting specific visual behaviors of patients communicating with clinicians via video conference calls. The system incorporates multiple domain-specific models, each trained for a particular behavior. In particular, we are now able to extract a large number of low and middle level features including gaze, eye contact and looking in 4 other directions, head orientation and tilt, emotions encoded in the space of valence and arousal, and 17 specific action units. These features are visualized (see Figure 26) in the form of augmentations to the recorded videos to be further used to improve diagnostics of various neurocognitive disorders: anxiety, depression, apathy, and ultimately Alzheimer's disease.
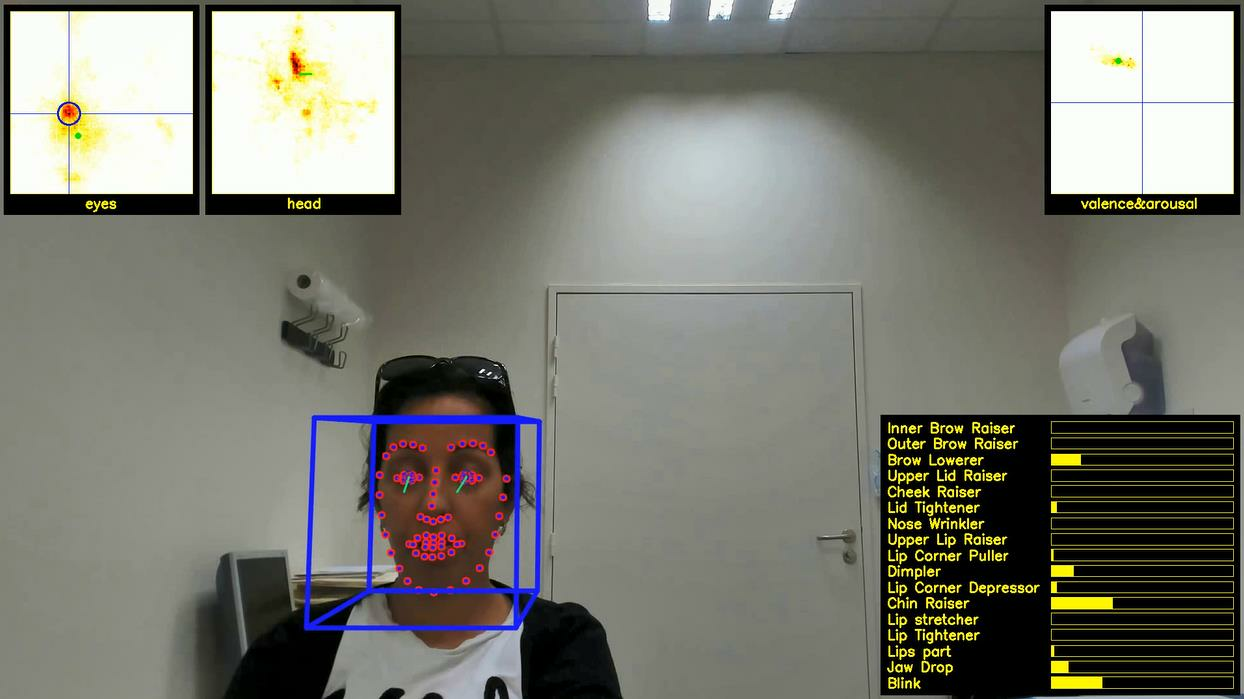
9 Bilateral contracts and grants with industry
Stars team has currently several experiences in technological transfer towards industrials, which have permitted to exploit research result.
9.1 Bilateral contracts with industry
Toyota
Toyota is working with Stars on action recognition software to be integrated on their robot platform. This project aims at detecting critical situations in the daily life of older adults alone at home. This will require not only recognition of ADLs but also an evaluation of the way and timing in which they are being carried out. The system we want to develop is intended to help them and their relatives to feel more comfortable because they know that potential dangerous situations will be detected and reported to caregivers if necessary. The system is intended to work with a Partner Robot - HSR - (to send real-time information to the robot) to better interact with the older adult.
Thales
Thales and Inria jointly explore facial analysis in the invisible spectrum. Among the different spectra low energy infrared waves, as well as ultraviolet waves will be studied. In this context following tasks will be included: We are designing a model to extract biometric features from the acquired data. Analysis of the data related to contours, shape, etc. will be performed. Current methodology cannot be adopted, since colorimetry in the invisible spectrum is more restricted with less diffuse variations and is less nuanced. Then facial recognition will be performed in the invisible spectrum. Expected challenges have to do with limited colorimetry and lower contrasts. In addition to the first milestone (face recognition in the invisible spectrum), there are two other major milestones: 2. Implementation of such a face recognition system, to be tested at the passage of the access portal to a school. 3. Pseudo-anonymized identification within a school (outdoor courtyards, interior buildings). Combining biometrics in the invisible spectra and anonymisation within an established group requires removing certain additional barriers that are specific to biometrics but also the use of statistical methods associated with biometrics. This pseudo-anonymized identification must also incorporate elements of information provided by the proposed electronic school IDs.
Kontron
Kontron has a collaboration with Stars, which runs from April 2018 until July 2021 to embed CNN based people tracker within a video-camera. Their system uses Intel VPU modules, such as Myriad X (MA2485), based on OpenVino library.
European System Integration
The company ESI (European System Integration) has a collaboration with Stars, which runs from September 2018 until March 2022 to develop a novel Re-Identification algorithm which can be easily set-up with low interaction for videosurveillance applications. ESI provides software solutions for remote monitoring stations, remote assistance, video surveillance, and call centers. It was created in 1999 and ESI is a leader in the French remote monitoring market. Nowadays, ensuring the safety of goods and people is a major problem. For this reason, surveillance technologies are attracting growing interest and their objectives are constantly evolving: it is now a question of automating surveillance systems and helping video surveillance operators in order to limit interventions and staff. One of the current difficulties is the human processing of video, as the multiplication of video streams makes it difficult to understand meaningful events. It is therefore necessary to give video surveillance operators suitable tools to assist them with tasks that can be automated. The integration of video analytics modules will allow surveillance technologies to gain in efficiency and precision. In recent times, deep learning techniques have been made possible by the advent of GPU processors, which offer significant processing possibilities. This leads to the development of automatic video processing.
Fantastic Sourcing
Fantastic Sourcing is a French SME specialized in micro-electronics, it develops e-health technologies. Fantastic Sourcing is collaborating with Stars through the UCA Solitaria project, by providing their Nodeus system. Nodeus is a IoT (Internet of Things) system for home support for the elderly, which consists of a set of small sensors (without video cameras) to collect precious data on the habits of isolated people. Solitaria project performs a multi-sensor activity analysis for monitoring and safety of older and isolated people. With the increase of the ageing population in Europe and in the rest of the world, keeping elderly people at home, in their usual environment, as long as possible, becomes a priority and a challenge of modern society. A system for monitoring activities and alerting in case of danger, in permanent connection with a device (an application on a phone, a surveillance system ...) to warn relatives (family, neighbours, friends ...) of isolated people still living in their natural environment could save lives and avoid incidents that cause or worsen the loss of autonomy. In this RD project, we propose to study a solution allowing the use of a set of innovative heterogeneous sensors in order to: 1) detect emergencies (falls, crises, etc.) and call relatives (neighbours, family, etc.); 2) detect, over short or longer predefined.
Nively - WITA SRL
Nively is a French SME specialized in e-health technologies, it develops position and activity monitoring of activities of daily living platforms based on video technology. Nively’s mission is to use technological tools to put people back at the center of their interests, with their emotions, identity and behavior. Nively is collaborating with Stars through the UCA Solitaria project, by providing their MentorAge system. This software allows the monitoring of elderly people in nursing homes in order to detect all the abnormal events in the lives of residents (falls, runaways, strolls, etc.). Nively’s technology is based on RGBD video sensors (Kinects type) and a software platform for event detection and data visualization. Nively is also in charge of Software distribution for the ANR Activis project. This project is based on an objective quantification of the atypical behaviors on which the diagnosis of autism is based, with medical (diagnostic assistance and evaluation of therapeutic programs) and computer scientific (by allowing a more objective description of atypical behaviors in autism) objectives. This quantification requires video analysis of the behavior of people with autism. In particular, we propose to explore the issues related to the analysis of ocular movement, gestures and posture to characterize the behavior of a child with autism. Thus, Nively will add autistic behavior analysis software to its product range.
ARECO
ARECO is a French SME specialized in the field of nebulization and misting technologies. It manufactures and sells nebulization devices and dynamic display processes. In this study, an algorithm will be designed for the analysis of customer behaviors when approaching the Fruits & Vegetables department. The analysis should be done on an offline video tape in the evening to store the analyzed interactions. The algorithm will be able to extract the recognized actions and the corresponding time information from a video and store them in a separate file.
9.2 Bilateral grants with industry
LiChIE Project
The LiChIE project (Lion Chaine Image Elargie) is conducted in collaboration with AirBus and BPI to found nine topics including six on the theme of In-flight imagery and three on the robotics theme for the assembly of satellites. The two topics involving STARS are :
- Mohammed Guermal's PhD thesis on Visual Understanding of Activities for an improved collaboration between humans and robots. He began on December 1, 2020.
- Farhood Negin post-doctoral studies on detection and tracking of vehicles from satellite videos and abnormal activity detection. He started in Oct 2020 for 2 years.
10 Partnerships and cooperations
10.1 International initiatives
10.1.1 Inria associate team not involved in an IIL
SafEE (Safe and Easy Environment)
- Title: Safe and Easy Environment for Alzheimer Disease and related disorders
- Duration: 2018 - 2020
- Coordinator: Inria - Taipei Tech
-
Partners:
- Dept. of Electrical Engineering, National Taipei University of Technology Taipei (Taiwan)
- National Cheng Kung University (Taiwan)
- Taichung Veterans General Hospital (Taiwan)
- Nice Hospital
- Inria contact: François Brémond
- Summary: SafEE (Safe Easy Environment) investigates technologies for the evaluation, stimulation and intervention for Alzheimer patients. The SafEE project aims at improving the safety, autonomy and quality of life of older people at risk or suffering from Alzheimer's disease and related disorders. More specifically the SafEE project : 1) focuses on specific clinical targets in three domains: behavior, motricity and cognition 2) merges assessment and non pharmacological help/intervention and 3) proposes easy ICT device solutions for the end users. In this project, experimental studies will be conducted both in France (at Hospital and Nursery Home) and in Taiwan.
10.2 European initiatives
10.2.1 FP7 & H2020 Projects
BIM2TWIN
- Title: BIM2TWIN: Optimal Construction Management Production Control
- Duration: November 2020 - November 2024
- Coordinator: CSTB
-
Partners:
- CENTRE SCIENTIFIQUE ET TECHNIQUE DU BATIMENT
- TECHNION - ISRAEL INSTITUTE OF TECHNOLOGY
- THE CHANCELLOR MASTERS AND SCHOLARSOF THE UNIVERSITY OF CAMBRIDGE
- TECHNISCHE UNIVERSITAET MUENCHEN
- Inria contact: Pierre Alliez and Francois Bremond
-
Summary:
BIM2TWIN aims to build a Digital Building Twin (DBT) platform for construction management that implements lean principles to reduce operational waste of all kinds, shortening schedules, reducing costs, enhancing quality and safety and reducing carbon footprint. BIM2TWIN proposes a comprehensive, holistic approach. It consists of a (DBT) platform that provides full situational awareness and an extensible set of construction management applications. It supports a closed loop Plan-Do- Check-Act mode of construction. Its key features are:
- Grounded conceptual analysis of data, information and knowledge in the context of DBTs, which underpins a robust system architecture
- A common platform for data acquisition and complex event processing to interpret multiple monitored data streams from construction site and supply chain to establish real-time project status in a Project Status Model (PSM)
- Exposure of the PSM to a suite of construction management applications through an easily accessible application programming interface (API) and directly to users through a visual information dashboard
- Applications include monitoring of schedule, quantities budget, quality, safety, and environmental impact.
- PSM representation based on property graph semanticaly linked to the Building Information Model (BIM) and all project management data. The property graph enables flexible, scalable storage of raw monitoring data in different formats, as well as storage of interpreted information. It enables smooth transition from construction to operation.
BIM2TWIN is a broad, multidisciplinary consortium with hand-picked partners who together provide an optimal combination of knowledge, expertise and experience in a variety of monitoring technologies, artificial intelligence, computer vision, information schema and graph databases, construction management, equipment automation and occupational safety. The DBT platform will be experimented on 3 demo sites (SP, FR, FI).
10.2.2 Collaborations in European programs, except FP7 and H2020
MePheSTO
- Title: MePheSTO: Digital Phenotyping 4 Psychiatric Disorders from Social Interaction
- Duration: September 2020 - August 2023
- Coordinator: Inria-DFKI joint project
-
Partners:
- François Brémond (Inria-STARS team)
- Maxime Amblard (Inria-SEMAGRAMME team)
- Jan Alexandersson (DFKI-COS, Saarbruecken)
- Johannes Tröger (DFKI-COS, Saarbruecken)
- Inria contact: Maxime Amblard and Francois Brémond
- Summary: MePheSTO is an interdisciplinary research project that envisions a scientifically sound methodology based on artificial intelligence methods for the identification and classification of objective, and thus measurable, digital phenotypes of psychiatric disorders. MePheSTO has a solid foundation of clinically motivated scenarios and use-cases synthesized jointly with clinical partners. Important to MePheSTO is the creation of a multimodal corpus including speech, video, and biosensors of social patient-clinician interactions, which serves as the basis for deriving methods, models and knowledge. Important project outcomes include technical tools and organizational methods for the management of medical data that implement both ELSI and GDPR requirements, demonstration scenarios covering patients' journeys including early detection, diagnosis support, relapse prediction, therapy support, an annotated corpus, Ph.D. theses, and publications. MePheSTO builds a joint DFKI-Inria workforce – the foundation for future R&D and innovation projects.
DeepSpa
- Title: DeepSpa: Deep Speech Analysis
- Duration: January 2019 - June 2021.
- Coordinator: Inria
-
Partners:
- Inria: technical partner and project coordinator
- University of Maastricht: clinical partner
- Jansen and Jansen: pharma partner and business champion
- Association Innovation Alzheimer: subgranted clinical partner
- Ki-element: subgranted technical partner.
- Inria contact: Alexandra König (STARS)
- Summary: The DeepSpa is a EIT Health project, which aims to deliver telecommunication based neurocognitive assessment tools for early screening, early diagnostic and follow-up of cognitive disorders, mainly in elderly. The target is also clinical trials addressing Alzheimer’s and other neurodegenerative diseases. By combining AI in speech recognition and video analysis for facial expression recognition, the proposed tools allow remote cognitive and psychological testing, thereby saving time and money.
E-Santé Silver Economy - Alcotra
- Title: E-Santé
- Duration: February 2020 - June 2022.
- Coordinator: Nice Metropole
-
Partners:
- Nice Metropole
- Inria (Stars)
- CoBTek
- Nice hospital
- University of Genova
- University of Torino
- Liguria Region
- Liguria Digitale
- Provence Alpes Agglomération
- University of Côte d'Azur
- Inria contact: François Brémond (STARS)
- Summary: E-Santé Silver Economy is a Alcotra project, which performs a multi-sensor activity analysis for the monitoring and safety of older and isolated people. The E-Health (E-Santé in French and E-Sanità in Italian) / Silver Economy project is a collaborative project within the framework of the European cross-border cooperation program between France and Italy Interreg ALCOTRA . The aim is to increase innovation projects (in particular clusters, poles and companies) - and to develop innovative services at cross-border level. The E-Health / Silver Economy project tackles the problems of frailty among elderly, more particularly in rural and isolated areas; as well as access to innovations for the ALCOTRA regions, where there is an imbalance in terms of innovation and access to public services between urban and rural areas. The majority of the population, services and economic activities are concentrated in cities. The aims of the project are therefore: to experiment innovative e-health tools and to increase the accessibility of isolated people to care (screening, diagnosis and follow-up); in order to keep elderly people at their own houses as long as possible, by proposing solutions to delay the decrease in their mental, cognitive and physical capacities.
10.3 National initiatives
10.3.1 ANR
ENVISION
- Title: ENVISION: Computer Vision for automated holistic analysis of humans
- Duration: 2017 - 2021.
- Coordinator: Inria
- Inria contact: Antitza Dantcheva
- Summary: The main objective of ENVISION is to develop the computer vision and theoretical foundations of efficient biometric systems that analyze appearance and dynamics of both face and body, towards recognition of identity, gender, age, as well as mental and social states of humans in the presence of operational randomness and data uncertainty. Such dynamics - which will include facial expressions, visual focus of attention, hand and body movement, and others, constitute a new class of tools that have the potential to allow for successful holistic analysis of humans, beneficial in two key settings: (a) biometric identification in the presence of difficult operational settings that cause traditional traits to fail, (b) early detection of frailty symptoms for health care.
RESPECT
- Title: RESPECT: Computer Vision for automated holistic analysis of humans
- Duration: 2018 - 2021.
- Coordinator: Hochschule Darmstadt
-
Partners:
- Inria
- Hochschule Darmstadt
- EURECOM
- Inria contact: Antitza Dantcheva
- Summary: In spite of the numerous advantages of biometric recognition systems over traditional authentication systems based on PINs or passwords, these systems are vulnerable to external attacks and can leak data. Presentations attacks (PAs) – impostors who manipulate biometric samples to masquerade as other people – pose serious threats to security. Privacy concerns involve the use of personal and sensitive biometric information, as classified by the GDPR, for purposes other than those intended. Multi-biometric systems, explored extensively as a means of improving recognition reliability, also offer potential to improve PA detection (PAD) generalisation. Multi-biometric systems offer natural protection against spoofing since an impostor is less likely to succeed in fooling multiple systems simultaneously. For the same reason, previously unseen PAs are less likely to fool multi-biometric systems protected by PAD. RESPECT, a Franco-German collaborative project, explores the potential of using multi-biometrics as a means to defend against diverse PAs and improve generalisation while still preserving privacy. Central to this idea is the use of (i) biometric characteristics that can be captured easily and reliably using ubiquitous smart devices and, (ii) biometric characteristics which facilitate computationally manageable privacy preserving, homomorphic encryption. The research focuses on characteristics readily captured with consumer-grade microphones and video cameras, specifically face, iris and voice. Further advances beyond the current state of the art involve the consideration of dynamic characteristics, namely utterance verification and lip dynamics. The core research objective is to determine which combination of biometrics characteristics gives the best biometric authentication reliability and PAD generalisation while remaining compatible with computationally efficient privacy preserving BTP schemes.
ACTIVIS
- Title: ACTIVIS: Video-based analysis of autism behavior
- Duration: 2020 - 2023
- Coordinator: Aix-Marseille Université - LIS
-
Partners:
- Inria
- Aix-Marseille Université - LIS
- Hôpitaux Pédiatriques Nice CHU-Lenval - CoBTeK
- Nively
- Inria contact: François Brémond
- Summary: The ACTIVIS project is an ANR project (CES19: Technologies pour la santé) started in January 2020 and will end in December 2023 (48 months). This project is based on an objective quantification of the atypical behaviors on which the diagnosis of autism is based, with medical (diagnostic assistance and evaluation of therapeutic programs) and computer scientific (by allowing a more objective description of atypical behaviors in autism) objectives. This quantification requires video analysis of the behavior of people with autism. In particular, we propose to explore the issues related to the analysis of ocular movement, gestures and posture to characterize the behavior of a child with autism.
10.3.2 FUI
ReMinAry
- Title: ReMinAry
- Duration: September 2016 - June 2020.
- Coordinato: GENIOUS Systèmes
-
Partners:
- GENIOUS Systèmes,
- Inria (Stars),
- MENSIA technologies - Mindmaze,
- Institut du Cerveau et de la Moelle épinière,
- la Pitié-Salpêtrière hospital.
- Inria contact: François Brémond (STARS)
- Summary: This project is based on the use of motor imagery (MI), a cognitive process consisting of the mental representation of an action without concomitant movement production. This technique consists in imagining a movement without realizing it, which entails an activation of the brain circuits identical to those activated during the real movement. By starting rehabilitation before the end of immobilization, a patient operated on after a trauma will gain rehabilitation time and function after immobilization is over. The project therefore consists in designing therapeutic video games to encourage the patient to re-educate in a playful, autonomous and active way in a phase where the patient is usually passive. The objective will be to measure the usability and the efficiency of the re-educative approach, through clinical trials centered on two pathologies with immobilization: post-traumatic (surgery of the shoulder) and neurodegenerative (amyotrophic lateral sclerosis).
10.4 Regional initiatives
Solitaria - Multi-sensor activity monitoring
- Title: Solitaria
- Duration: October 2019 - April 2020.
- Coordinator: Idex UCA
-
Partners:
- Inria (Stars): technical partner and project coordinator
- CoBTek,
- Fantastic Sourcing.
- Inria contact: François Brémond (STARS)
-
Summary:
Solitaria is a UCA project, which performs a multi-sensor activity analysis for monitoring and safety of older and isolated people. With the increase of the ageing population in Europe and in the rest of the world, keeping elderly people at home, in their usual environment, as long as possible, becomes a priority and a challenge of modern society. A system for monitoring activities and alerting in case of danger, in permanent connection with a device (an application on a phone, a surveillance system ...) to warn relatives (family, neighbours, friends ...) of isolated people still living in their natural environment could save lives and avoid incidents that cause or worsen the loss of autonomy. In this R&D project, we propose to study a solution allowing the use of a set of innovative heterogeneous sensors in order to:
- 1) detect emergencies (falls, crises, etc.) and call relatives (neighbours, family, etc.);
- 2) detect, over short or longer predefined periods, behavioural changes in the elderly through an intelligent analysis of data from sensors.
- Fantastic Sourcing is collaborating with Stars through the UCA Solitaria project, by providing their Nodeus system. Nodeus is a IoT (Internet of Things) system for home support for the elderly, which consists of a set of small sensors (without video cameras) to collect precious data on the habits of isolated people. Fantastic Sourcing is a French SME specialized in micro-electronics, it develops e-health technologies.
11 Dissemination
11.1 Promoting scientific activities
11.1.1 Scientific events: organisation
General chair, scientific chair
François Brémond was General Chair at IPAS 22020
(https://
Member of the organizing committees
Antitza Dantcheva served in following organizing committees.
- Publication Chair at the International Joint Conference on Biometrics (IJCB'20), October 2020
- Co-Organizer of the Workshop and challenge on “Computer Vision for Physiological Measurement” in conjunction with the Conference on Computer Vision and Pattern Recognition (CVPR), June 2020
- Co-Organizer of the Special Session on “Bias in Biometrics” at the European Signal Processing Conference (EUSIPCO), August 2020
- Co-Organizer of the Special Session on “Advances and Challenges in Face and Gesture based Security Systems (ACFGSS)” at the IEEE Conference on Automatic Face and Gesture Recognition (FG), May 2020
- Co-Organizer of the Special Session on “Human Health Monitoring Based on Computer Vision” at the IEEE Conference on Automatic Face and Gesture Recognition (FG), May 2020
- Co-Organizer of the Workshop on “Deepfakes and Presentation Attacks in Biometrics” in conjunction with the Winter Conference on Computer Vision (WACV), March 2020
11.1.2 Scientific events: selection
Chair of conference program committees
- François Brémond was in the Program Committees of ICDP‘20 and AVSS’20.
- Antitza Dantcheva was Program Chair of the International Conference of the Biometrics Special Interest Group (BIOSIG) 2020.
Member of the conference program committees
- Monique Thonnat was program committee member of the conference IJCAI-PRICAI 2020
- Monique Thonnat was program committee member of the conference ICPRAM 2021
Reviewer
- François Brémond was reviewer for WACV’20, ECCV’20, CVPR’20.
- Antitza Dantcheva was reviewer for IJCB'20, FG'20.
- David Anghelone was reviewer for the Fourth IEEE International Conference on Image Processing (ICIP), Applications and Systems (IPASS2020).
- Sjrijan Das was reviewer for WACV 2021, CVPR 2021, and KCST 2021.
- Michal Balazia was reviewer for IEEE International Conference on Pattern Recognition (ICPR).
11.1.3 Journal
Member of the editorial boards
- François Brémond was handling editor of MVA journal - the international journal “Machine Vision and Application” 2020.
- Antitza Dantcheva is Associate Editor of Pattern Recognition (PR), and in the editorial board of the Journal Multimedia Tools and Applications (MTAP).
Reviewer - reviewing activities
- Michal Balazia was review for MDPI Sensors journal, Pattern Recognition journal, Pattern Recognition Letters journal, IET Biometrics journal, ACM Computing Surveys.
11.1.4 Invited talks
François Brémond gave an invited talk at IPAS 2020 (https://
11.1.5 Scientific expertise
- Antitza Dantcheva was member of the evaluation committee of ANR AAPG 2020 - Comité Sécurité Globale et Cybersécurité.
- Antitza Dantcheva was in the Technical Activities Committee of IEEE Biometrics Council.
- Antitza Dantcheva served in the EURASIP Biomedical Image & Signal Analytics (BISA) special area team.
- Srijan Das was mentor for the Emerging Technology Business Incubator (ETBI) Led by NIT Rourkela, a platform envisaged transforming the start-up ecosystem of the region.
- Monique Thonnat was expert for international evaluation: CONACYT Ciencia de Frontera 2019 (Mexico) and ECOS-Sud Chili 2020 (Chili).
11.1.6 Research administration
Monique Thonnat is member of the scientific board of ENPC, Ecole Nationale des Ponts et Chaussées.
11.2 Teaching - Supervision - Juries
11.2.1 Teaching
- François Brémond organized and lectured the Master MSc Data Science and Artificial Intelligence (Computer Vision and Deep Learning) 30h class at Université Côte d'Azur in 2020 and 2021.
Web-site: http://
www-sop. inria. fr/ members/ Francois. Bremond/ MSclass/ deepLearningWinterSchool/ UCA_master/ index. html - Antitza Dantcheva taught 2 classes at Polytech Nice Sophia - Univ Côte d'Azur (Applied Artificial Intelligence, Master 2) in Oct.2020.
- David Anghelone was Teaching assistant at Polytech Nice Sophia - Univ Côte d'Azur (Applied Artificial Intelligence, Master 2) in Oct.2020
11.2.2 Supervision
- PhD: Srijan DAS "Spatio-temporal Attention Mechanisms for Activity Recognition" (defended 1rst of October 2020) supervisors Monique Thonnat and François Brémond.
- PhD in progress: Jen-Cheng HOU "Quantified Analysis for Seizure Videos", supervisor Monique Thonnat.
- PhD in progress: Rui Dai, 2019, "Action Detection for Untrimmed Videos based on Deep Neural Networks", François Brémond.
- PhD in progress: Abdorrahim Bahrami, "Modelling and verifying dynamical properties of biological neural networks in Coq", Elisabetta De Maria.
- PhD in progress: Thibaud L'Yvonnet, "Relations between human behaviour models and brain models - Application to serious games", 2018, Sabine Moisan and Elisabetta De Maria.
- PhD in progress: Hao Chen, 2019, “People Re-identification using Deep Learning methods”, François Brémond and Benoit Lagadec (Fellowship CIFRE - ESI).
- PhD in progress: Yaohui Wang, "Learning to generate human videos", supervisor Antitza Dantcheva and François Brémond.
- PhD in progress: David Anghelone, "Computer Vision and Deep Learning applied to Facial analysis in the invisible spectra", supervisor Antitza Dantcheva.
11.2.3 Juries
François Brémond was reviewer of the HDR (habilitation) jury of Stéphane Herbin, ONERA, 6 Jul 2020.
- François Brémond was in following PhD committees.
- Cristiano Massaroni, Sapienza University, 16 February 2020 (Reviewer)
- Konstantinos Papadopoulos, Université du Luxembourg, 20th April 2020
- Lucas Pascal, Eurecom, 12 June 2020 (comité de suivi de thèse)
- Florent Jousse, Université Côte d'Azur, 27 May 2020 (comité de suivi de thèse)
- Melissa Sanabria, Université Côte d'Azur, 20 July 2020 (comité de suivi de thèse)
- Magali Payne, Université Côte d'Azur, 4 September 2020 (comité de suivi de thèse)
- Claire Labit Bonis, LAAS (comité de suivi de thèse)
- Nicolas Girard, Université Côte d'Azur, 16 October 2020 (Président)
- Thibault Blanc-Beyne, IRIT, 9 November 2020 (Reviewer)
- Hani Javan Hemmat, Eindhoven University of Technology, 24 November 2020 (Reviewer)
- Renato BAPTISTA, Université du Luxembourg, 13th January 2021
- Monique Thonnat was in the following PhD committees.
- Nicolas Foulquier, Université Bretagne Occidentale, 26th of February 2020 (Reviewer)
- Adrien Malaisé, Université de Lorraine, 7th of July 2020 (Reviewer)
- Francois Lasson, ENIB, 5th of October 2020 (Reviewer)
- Srijan Das, Université Côte d'Azur, 1st of October 2020 (Examiner)
- Antitza Dantcheva was in the comité de suivi de thèse of following PhD students.
- Santiago Smith Silva, Université Côte d'Azur, 19th March 2020 and 1st December 2020
- Julien Aubert, Eurecom, 7th May 2020
12 Scientific production
12.1 Major publications
- 1 inproceedingsLearning to match appearances by correlations in a covariance metric spaceEuropean Conference on Computer VisionSpringer2012, 806--820
- 2 article Exploiting Feature Correlations by Brownian Statistics for People Detection and Recognition IEEE transactions on systems, man, and cybernetics 2016
- 3 inproceedings Video Covariance Matrix Logarithm for Human Action Recognition in Videos IJCAI 2015 - 24th International Joint Conference on Artificial Intelligence (IJCAI) Buenos Aires, Argentina July 2015
- 4 articleSemantic Event Fusion of Different Visual Modality Concepts for Activity RecognitionIEEE Transactions on Pattern Analysis and Machine Intelligence382016, 1598 - 1611
- 5 articleGender estimation based on smile-dynamicsIEEE Transactions on Information Forensics and Security2016, 11
- 6 inproceedings Toyota Smarthome: Real-World Activities of Daily Living ICCV 2019 -17th International Conference on Computer Vision Seoul, South Korea October 2019
- 7 inproceedings VPN: Learning Video-Pose Embedding for Activities of Daily Living ECCV 2020 - 16th European Conference on Computer Vision Glasgow (Virtual), United Kingdom August 2020
- 8 inproceedings Gesture Recognition by Learning Local Motion Signatures CVPR 2010 : IEEE Conference on Computer Vision and Pattern Recognition San Franscico, CA, United States IEEE Computer Society Press June 2010
- 9 article Recognizing Gestures by Learning Local Motion Signatures of HOG Descriptors IEEE Transactions on Pattern Analysis and Machine Intelligence 2012
- 10 inproceedingsKnowledge Representation for Program ReuseEuropean Conference on Artificial Intelligence (ECAI)Lyon, FranceJuly 2002, 240-244
- 11 articleBlocks, a Component Framework with Checking Facilities for Knowledge-Based SystemsInformatica, Special Issue on Component Based Software Development254November 2001, 501-507
- 12 articleCompilation Modulaire d'un Langage SynchroneRevue des sciences et technologies de l'information, série Théorie et Science Informatique430June 2011, 441-471URL: http://hal.inria.fr/inria-00524499/en
- 13 article What Can Program Supervision Do for Software Re-use? IEE Proceedings - Software Special Issue on Knowledge Modelling for Software Components Reuse 147 5 2000
- 14 inproceedings Automatic Video Interpretation: A Novel Algorithm based for Temporal Scenario Recognition The Eighteenth International Joint Conference on Artificial Intelligence (IJCAI'03) Acapulco, Mexico 9-15 September 2003
- 15 inproceedings G3AN: Disentangling Appearance and Motion for Video Generation CVPR 2020 - IEEE Conference on Computer Vision and Pattern Recognition Seattle / Virtual, United States June 2020
12.2 Publications of the year
International journals
International peer-reviewed conferences
Conferences without proceedings
Doctoral dissertations and habilitation theses
12.3 Cited publications
- 42 inproceedingsTVM: An Automated End-to-end Optimizing Compiler for Deep LearningProceedings of the 12th USENIX Conference on Operating Systems Design and ImplementationOSDI'18Berkeley, CA, USACarlsbad, CA, USAUSENIX Association2018, 579--594URL: https://dl.acm.org/citation.cfm?id=3291168.3291211
- 43 inproceedingsObject Detection in Videos with Tubelet Proposal Networks07 2017, 889-897
- 44 inproceedingsObject Detection from Video Tubelets with Convolutional Neural Networks06 2016, 817-825
- 45 inproceedingsLcr-net: Localization-classification-regression for human poseProceedings of the IEEE Conference on Computer Vision and Pattern Recognition2017, 3433--3441
- 46 inproceedingsSimple online and realtime tracking with a deep association metric2017 IEEE international conference on image processing (ICIP)IEEE2017, 3645--3649