Keywords
Computer Science and Digital Science
- A3.1.1. Modeling, representation
- A3.4.5. Bayesian methods
- A6.1.1. Continuous Modeling (PDE, ODE)
- A6.1.2. Stochastic Modeling
- A6.2.1. Numerical analysis of PDE and ODE
- A6.2.4. Statistical methods
- A6.3.1. Inverse problems
- A6.3.2. Data assimilation
- A6.3.3. Data processing
- A6.4.1. Deterministic control
Other Research Topics and Application Domains
- B1. Life sciences
- B1.1.2. Molecular and cellular biology
- B1.1.4. Genetics and genomics
- B1.1.7. Bioinformatics
- B1.1.8. Mathematical biology
- B1.1.10. Systems and synthetic biology
- B2.2.4. Infectious diseases, Virology
- B4.3.1. Biofuels
1 Team members, visitors, external collaborators
Research Scientists
- Delphine Ropers [Team leader, Inria, Researcher, HDR]
- Eugenio Cinquemani [Inria, Researcher, HDR]
- Aline Marguet [Inria, Researcher]
- Hidde de Jong [Inria, Senior Researcher, HDR]
Faculty Member
- Johannes Geiselmann [Univ Grenoble Alpes, Professor, HDR]
Post-Doctoral Fellow
- Thibault Etienne [Inria]
PhD Students
- Ignacia Cancino Aguirre [Inria, from Oct 2021]
- Charles Medous [Univ Grenoble Alpes, from Oct 2021]
- Antrea Pavlou [Inria]
- Emrys Reginato [Inria]
- Maaike Sangster [Inria]
Technical Staff
- Soraya Arias [Inria, Engineer]
- Tamas Muszbek [Inria, Engineer]
Interns and Apprentices
- Amélie Caddeo [Inria, Intern, from Apr 2021 until June 2021]
- Olivier Ulrich Feudjio [Inria, Intern, from Feb 2021 until July 2021]
- Charles Medous [Inria, Intern, from Apr until Sep 2021]
- Geoffrey Roudaut [Inria, Intern, from Apr until Sep 2021]
Administrative Assistant
- Diane Courtiol [Inria]
External Collaborator
- Muriel Cocaign-Bousquet [INRAE, senior researcher, from Oct. 2021, Toulouse, HDR]
2 Overall objectives
MICROCOSME combines computational and experimental approaches for the analysis, engineering, and control of the growth of microorganisms. Understanding and controlling the dynamics of bacterial growth is vitally important in health, medicine, biotechnology, and food industries, for instance to halt the growth of pathogens or stimulate the growth of probiotics or industrial microorganisms.
We develop multiscale models of growth, where the macroscopic observable, growth of a microbial population or community, depends on various metabolic pathways and regulatory mechanisms operating at microscopic scales within the cells. We use our (deterministic or stochastic) models to interpret experimental data or to infer the underlying growth processes from the data. This requires developing a platform for the automation of experiments, as well as methods and software for model estimation and data analysis. The analysis of microbial growth calls for new methodologies at the interface of microbiology, control theory, applied mathematics, computer science, biophysics, and molecular biology, which also leads to contributions in all of these fields. Our workhorse for the realization of this research program is the bacterium Escherichia coli pictured in Figure 1. Part of the microbiota of the human gut, E. coli is the model organism par excellence in microbiology and a popular platform for bio-based chemical production. We intend to extend approaches developed in-house for this specific microbe to other microorganisms including pathogens.
MICROCOSME has been created on October 1st, 2021. A recomposition and follow-up of former IBIS project-team, MICROCOSME unites researchers from Inria Grenoble – Rhône-Alpes and the Laboratoire Interdisciplinaire de Physique at Université Grenoble Alpes (CNRS UMR 5588).
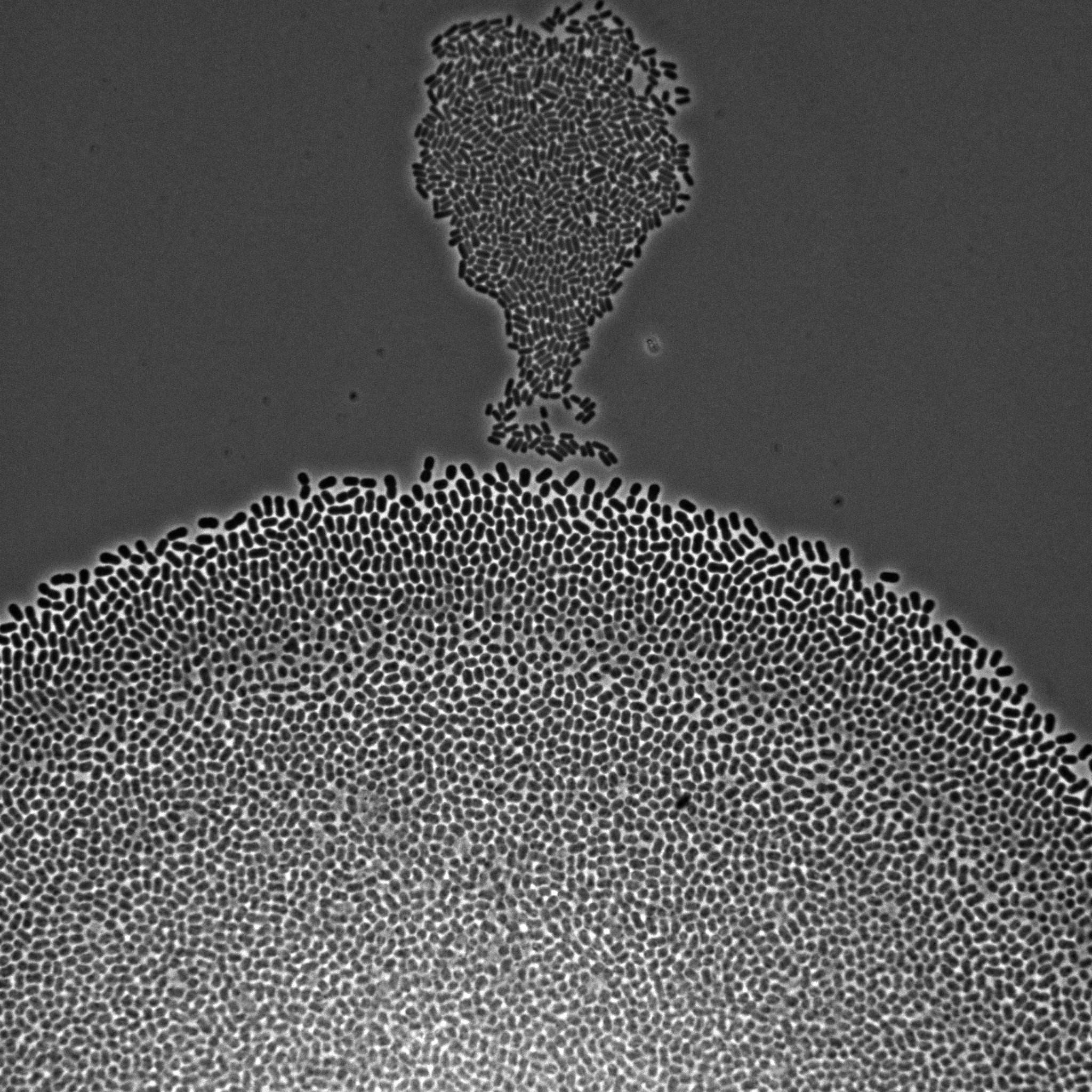
Figure 1: Microscopy image of Escherichia coli bacteria growing on a solid nutrient medium.
3 Research program
The research program of MICROCOSME is articulated around four research axes combining theory and experiments, which are illustrated in Figure 2 and detailed below.
3.1 Genome-scale analysis of microbial physiology
The molecular foundations of bacterial growth remain little understood today, because they involve large biochemical networks with physical and regulatory interactions across different levels of cellular organization. We investigate at the genome scale how the dynamics of gene expression and metabolism leads to microbial growth, using a combination of mathematical models and high-throughput data. The challenge is to integrate, in models of thousands of equations, multiple and heterogeneous datasets on the metabolic, transcriptomic, and proteomic level. We typically use constraint-based models to investigate the relations between microbial growth and metabolism, while the effect of growth on mRNA stability is analysed by means of non-linear mixed-effect models.
3.2 Natural and engineered resource allocation strategies in microorganisms
Microorganisms have evolved strategies to allocate their resources to different cellular functions and thus adjust their growth rate to fluctuating environments. We study these natural resource allocation strategies, by viewing cells as self-replicators that can be described using coarse-grained models and analysed by means of optimal and feedback control theory. The models take the form of systems of 5-10 nonlinear ordinary differential equations, with parameters estimated from published data or data obtained from dedicated experiments. Experimental work in the lab allows to validate model predictions on the single-cell and population level and to engineer new strategies for the reallocation of cell resources from growth to bioproduction.
3.3 Variability and robustness of microbial adaptation
The developments of experimental techniques and the use of video-microscopy have led to a growing number of high-quality data showing the heterogeneity among cells in a population. We combine these single-cell data with models describing the stochastic dynamics of individual cells, such as birth-death processes, branching processes, and mixed-effect models. The models allow to investigate the origins of heterogeneity and its role in the adaptation of microorganisms to environmental changes, and to leverage population heterogeneity for biotechnological applications. In practice, this requires the extension of modelling approaches by taking into account the specificities of heterogeneity, as well as the development of appropriate methods and software for the inference of models and of biological quantities from quantitative time-course profiles of the microbial response to environmental changes.
3.4 Analysis and control of microbial communities
Heterogeneity also arises within communities consisting of different microbial species. Understanding microbial interactions is a challenging task that goes well beyond the characterization of single species, and offers great opportunities for applications, such as the control of the community for bioproduction. Indeed, suitably constructed microbial consortia carry the potential to outperform single species in the accomplishment of processes of societal interest, such as biofuel synthesis. On the theoretical side, we develop (deterministic or stochastic) models of microbial dynamics similar to those in the three other research axes, which can be used to investigate new control approaches for microbial communities. On the experimental side, the application of control strategies for biotechnological applications requires the engineering of microbial strains and the automation of experiments. To that aim we have been developing a platform for feedback control experiments allowing the real-time monitoring, data processing, evaluation, and application of control laws.
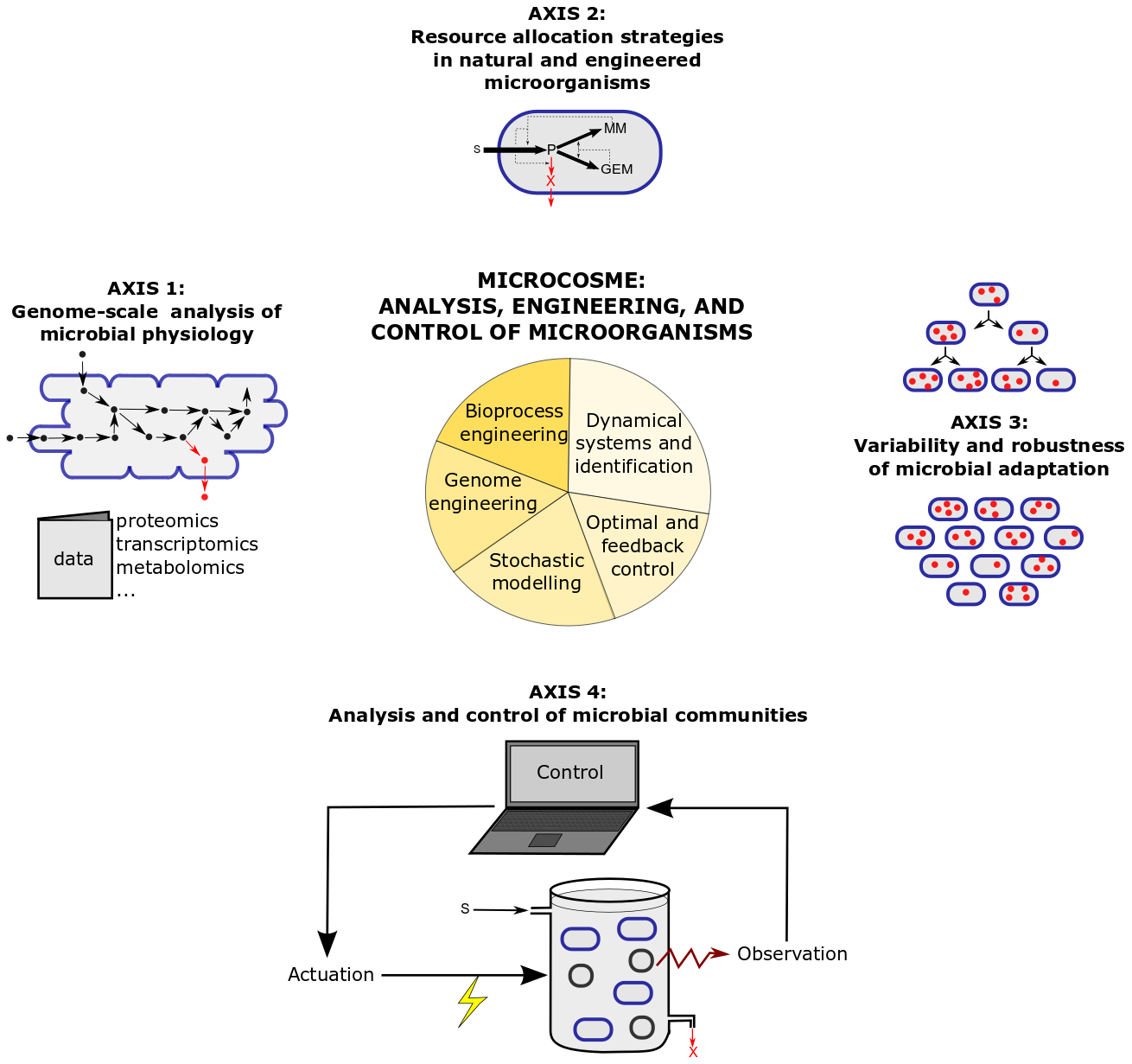
Figure 2: Research axes and methods in the MICROCOSME project-team.
4 Application domains
The research agenda of MICROCOSME is interdisciplinary in nature, driven by fundamental questions in biology, which we address by a combination of mathematical, computational, and experimental tools. This enables us to develop and share with partners a know-how useful to address challenging problems in health, bioeconomy, and biotechnology.
4.1 Biotechnology and bioeconomy
Bioproduction imposes a strong metabolic burden on microorganisms, detrimental to their growth and the production yield. Our studies of natural resource allocation strategies lead us to explore and engineer various reallocation strategies to improve bioproduction through growth control. For instance, in the past, we have successfully implemented a growth switch in E. coli bacteria, aiming at shuttling resources, away from protein synthesis (key for bacterial growth) to the high-yield production of a metabolite of interest (glycerol) 6. We also develop and test control strategies for synthetic microbial communities, composed of populations of different E. coli strains or in consortia with other species. In the wake of our studies on the relation between growth and metabolism, we develop bioeconomy strategies for the valorisation of vegetal waste into value-added product. In one project, notably, we aim at designing E. coli strains able to efficiently degrade carbon sources derived from pre-treatment of agricultural and forestry residues (Section 9).
4.2 Health
Numerous Mycobacteria species pose serious threats to human and animal health. Mycobacteria tuberculosis strains are also known to withstand several of the antibiotics used to treat the infection. We have started to extend our microbial physiology analyses by means of constraint-based models to understand the molecular control of mycobacterial growth and characterize the relations between metabolism, pathogenicity, and growth phenotype of mycobacterial species. This may lead, in the long term, to the development of new treatments for curing tuberculosis and other mycobacterial infections.
5 Social and environmental responsibility
Several of our research activities have a direct societal impact: our work on Mycobacteria addresses important questions of public health, while the project on the degradation and valorisation of vegetal waste meet European efforts in Circular Bioeconomy to replace fossil feedstock with renewable resources.
6 Highlights of the year
The project-team IBIS "Modelling, Simulation, Measurement, and Control of Bacterial Regulatory Networks" that was headed by Hidde de Jong terminated its life after twelve years of existence. It has been replaced by MICROCOSME on October 1st, 2021, a project-team of the Inria Grenoble - Rhône-Alpes research centre, in partnership with the University Grenoble Alpes. Delphine Ropers is the team leader. The present activity report summarizes team contributions carried out within IBIS and MICROCOSME.
7 New software and platforms
7.1 New software
7.1.1 ODIN+
-
Name:
Platform for advanced monitoring, control and optimisation of bioprocesses
-
Keywords:
Systems Biology, Biotechnology, Automatic control, Monitoring
-
Functional Description:
This application proposes a framework for on-line supervision of bioreactors. It gathers the data sampled from different on-line and off-line sensors. ODIN+ is a distributed platform, enabling remote monitoring as well as remote data acquisition.More originally, it enables researchers and industrials to easily develop and deploy advanced control algorithms, optimisation strategies, together with estimates of state variables or process state. It also contains a process simulator which can be harnessed for experimentation and training purposes. It is modular in order to adapt to any plant and to run most of the algorithms, and it can handle the high level of uncertainties that characterises the biological processes. The architecture is based on Erlang, and communication between modules through a MQTT Broker with Python for running the algorithms. ODIN+ is developed in collaboration with the INRIA Ibis research team.
-
Authors:
Nicolas Niclausse, Nicolas Chleq, Jean-Luc Szpyrka, Pierre Fernique, Thibaud Kloczko, Tamas Muszbek, Amine Lahouel, Olivier Bernard, Eugenio Cinquemani
-
Contact:
Olivier Bernard
7.1.2 GNA
-
Name:
Genetic Network Analyzer
-
Keywords:
Model Checking, Bioinformatics, Gene regulatory networks, Qualitative simulation
-
Scientific Description:
Genetic Network Analyzer (GNA) is the implementation of methods for the qualitative modeling and simulation of gene regulatory networks developed in the IBIS project-team.
-
Functional Description:
The input of GNA consists of a model of the regulatory network in the form of a system of piecewise-linear differential equations (PLDEs), supplemented by inequality constraints on the parameters and initial conditions. From this information, GNA generates a state transition graph summarizing the qualitative dynamics of the system. In order to analyze large graphs, GNA allows the user to specify properties of the qualitative dynamics of a network in temporal logic, using high-level query templates, and to verify these properties on the state transition graph by means of standard model-checking tools, either locally installed or accessible through a remote web server.
-
Release Contributions:
(1) it supports the editing and visualization of regulatory networks, in an SBGN-compatible format, (2) it semi-automatically generates a prototype model from the network structure, thus accelerating the modeling process, and (3) it allows models to be exported in the SBML Qual standard.
-
News of the Year:
Tutorial on the use of the model formalism for analyzing synthetic genetic circuits, published in an edited book.
- URL:
- Publications:
-
Contact:
Hidde de Jong
-
Participants:
Hidde de Jong, Michel Page, Delphine Ropers
-
Partner:
UGA
7.1.3 WellInverter
-
Name:
WellInverter
-
Keywords:
Bioinformatics, Statistics, Data visualization, Data modeling
-
Scientific Description:
WellInverter is a web application that implements linear inversion methods for the reconstruction of gene expression profiles from fluorescent or luminescent reporter gene data. WellInverter makes the methods available to a broad audience of biologists and bioinformaticians. In particular, we have put in place a parallel computing architecture with a load balancer to distribute the analysis queries over several back-end servers, redesigned the graphical user interface, and developed a plug-in system for defining high-level routines for parsing data files produced by microplate readers from different manufacturers.
-
Functional Description:
As input, WellInverter reads the primary data file produced by a 96-well microplate reader, containing time-series measurements of the absorbance (optical density) as well as the fluorescence and luminescence intensities in each well (if available). Various modules exist to analyze the data, in particular for detecting outliers, subtracting background, estimating growth rates, promoter activities and protein concentrations, visualizing expression profiles, synchronizing replicate profiles, etc. The computational core of the web application consists of the Python library WellFARE.
-
News of the Year:
Publication in BMC Bioinformatics describing the new version of the application.
- URL:
- Publications:
-
Contact:
Hidde de Jong
-
Participants:
Delphine Ropers, Hidde de Jong, Johannes Geiselmann, Michel Page, Valentin Zulkower, Yannick Martin
-
Partner:
UGA
7.1.4 WellFARE
-
Name:
WellFARE
-
Keywords:
Bioinformatics, Statistics, Data visualization, Data modeling
-
Scientific Description:
WellFARE is a Python library implementing linear inversion methods for the reconstruction of gene expression profiles from fluorescent or luminescent reporter gene data. WellFARE form the computational core of the WellInverter web application.
-
Functional Description:
As input, WellFARE reads the primary data file produced by a 96-well microplate reader, containing time-series measurements of the absorbance (optical density) as well as the fluorescence and luminescence intensities in each well (if available). Various functions exist to analyze the data, in particular for detecting outliers, subtracting background, estimating growth rates, promoter activities and protein concentrations, visualizing expression profiles, synchronizing replicate profiles, etc. WellFARE is the computational core of the web application WellInverter.
-
News of the Year:
Publication in BMC Bioinformatics describing the new version of WellFARE
- URL:
- Publication:
-
Contact:
Hidde de Jong
-
Participants:
Delphine Ropers, Johannes Geiselmann, Hidde de Jong, Michel Page, Valentin Zulkower, Yannick Martin
-
Partner:
UGA
8 New results
8.1 Analysis of fluorescent reporter genes
Participants: E. Cinquemani, H. de Jong, J. Geiselmann, A. Pavlou, D. Ropers.
The use of fluorescent and luminescent reporter genes allows real-time monitoring of gene expression, both at the level of individual cells and cell populations. Over the years, many useful resources have appeared, such as libraries of reporter strains for model organisms and computer tools for designing reporter plasmids. Moreover, the widespread adoption of thermostated microplate readers in experimental laboratories has made it possible to automate and multiplex reporter gene assays on the population level. This has resulted in large time-series data sets, typically comprising 100,000 - 1,000,000 measurements of absorbance, fluorescence, and luminescence for 96 wells on the microplate. In order to fully exploit these data sets, we need sound mathematical methods to infer biologically relevant quantities from the primary data and computer tools to apply the methods in an efficient and user-friendly manner.In the past few years we developed methods for the analysis of reporter gene data obtained in microplate experiments. These methods solve a range of estimation problems, notably the inference of growth rate, promoter activity, and protein concentration profiles. In the framework of her PhD thesis, Antrea Pavlou has continued this work by addressing a major issue in the interpretation of fluorescent data, namely that the inference of promoter activities is strongly dependent on the maturation properties of fluorescent proteins. We have therefore proposed an approach for incorporating maturation dynamics in the reconstruction of promoter activities. Our approach consists in developing and calibrating mechanistic maturation models for distinct fluorescent proteins. These models are then used alongside a Bayesian approach to estimate promoter activities from fluorescence data. We demonstrated by means of targeted experiments in Escherichia coli that our approach provides robust estimates, and that accounting for maturation is, in many cases, essential for the interpretation of gene expression data. This work has been submitted for publication.
8.2 Resource allocation models of bacterial growth
Participants: H. de Jong, J. Geiselmann, A. Pavlou, D. Ropers.
Various mathematical approaches have been used in the literature to describe the networks of biochemical reactions involved in microbial growth. With different levels of detail, the resulting models provide an integrated view of these reaction networks, including the transport of nutrients from the environment and the metabolism and gene expression allowing the conversion of these nutrients into biomass. Recent work has shown that coarse-grained models of resource allocation can account for a number of empirical regularities relating the macromolecular composition of the cell to the growth rate. Some of these models imply very specific control strategies enabling microorganisms to optimize growth. While these studies focus on steady-state growth, such conditions are rarely found in natural habitats, where microorganisms are continually challenged by environmental fluctuations.In recent years, in the framework of the PhD thesis of Nils Giordano, we extended the study of microbial growth strategies to dynamical environments, using a self-replicator model. In collaboration with the BIOCORE project-team, we formulated dynamical growth maximization as an optimal control problem that can be solved using Pontryagin’s Maximum Principle and we compared the theoretical results thus obtained with different possible implementations of growth control in bacterial cells 4. The experimental validation of some of these results are currently being carried out by Antrea Pavlou in the framework of her PhD project, funded by the ANR project Maximic (Section 9).
Also within the context of Maximic, we have worked on several extensions of the original self-replicator model. In collaboration with the BIOCORE and McTAO project-teams, we developed a model that accounts for additional classes of proteins, in particular a class of growth-rate-independent housekeeping proteins in addition to ribosomes and enzymes. Moreover, the model takes into account that the protein synthesis rate does not linearly depend on the total ribosome concentration, but only on its growth-rate-dependent fraction. In a paper accepted for publication in SIAM Journal on Applied Dynamical Systems 20, we perform an optimal control analysis of the extended model, using a combination of analytical and numerical tools. Our major conclusion is that, while the predicted dynamics of the system does not significantly change in comparison with the original model, the extended model more closely adheres to the experimental data. Moreover, it is capable of predicting the effects of metabolic load due to heterologous protein expression on the growth-rate dynamics.
In another follow-up work, carried out in collaboration with Francis Mairet (Ifremer Nantes) and Jean-Luc Gouzé (BIOCORE), we studied the effect of temperature on microbial growth, in particular the role of proteome allocation in bringing about temperature-induced changes. To tackle this problem, we propose an extended coarse-grained model of microbial growth that includes the processes of temperature-sensitive protein unfolding and chaperone-assisted (re)folding. We determine the proteome sector allocation that maximizes balanced growth rate as a function of nutrient limitation and temperature. Calibrated with quantitative proteomic data for Escherichia coli, the model allows us to bring out general principles of temperature-dependent proteome allocation and growth laws. The same activation energy for enzymes and ribosomes leads to an Arrhenius increase in growth rate at constant proteome composition over a large range of temperatures, whereas at extreme temperatures resources are diverted away from growth to chaperone-mediated stress responses. Our approach points at risks and possible remedies for the use of ribosome content to characterize complex ecosystems with temperature gradients. A paper based on this work was published in the journal npj Systems Biology and Applications this year 15.
8.3 Biotechnological applications of bacterial growth control
Participants: H. de Jong, J. Geiselmann, T. Clavier, D. Ropers.
The ability to experimentally control the growth rate is crucial for studying bacterial physiology. It is also of central importance for applications in biotechnology, where often the goal is to limit or even arrest growth. Growth-arrested cells with a functional metabolism open the possibility to channel resources into the production of a desired metabolite, instead of wasting nutrients on biomass production. In recent years we obtained a foundational result for growth control in bacteria 6, in that we engineered an E. coli strain where the transcription of a key component of the gene expression machinery, RNA polymerase, is under the control of an inducible promoter. By changing the inducer concentration in the medium, we can adjust the RNA polymerase concentration and thereby switch bacterial growth between zero and the maximal growth rate supported by the medium. The publication also presented a biotechnological application of the synthetic growth switch in which both the wild-type E. coli strain and our modified strain were endowed with the capacity to produce glycerol when growing on glucose. Cells in which growth has been switched off continue to be metabolically active and harness the energy gain to produce glycerol at a twofold higher yield than in cells with natural control of RNA polymerase expression, putting the yield very close to the theoretical maximum.
In an article published in ACS Synthetic Biology 18, we investigated the scalability of the growth switch in culture conditions that are closer to standard process conditions in biotechnology. Our results indicate that, in liter-scale bioreactors operating in fed-batch mode, growth-arrested Escherichia coli cells are able to convert glucose to glycerol at an increased yield. A multiomics quantification of the physiology of the cells shows that, apart from acetate production, few metabolic side effects occur. However, a number of specific responses to growth slow-down and growth arrest are launched at the transcriptional level. These notably include the downregulation of genes involved in growth-associated processes, such as amino acid and nucleotide metabolism and translation. Interestingly, the transcriptional responses are buffered at the proteome level, probably due to the strong decrease of the total mRNA concentration after the diminution of transcriptional activity and the absence of growth dilution of proteins. Growth arrest thus reduces the opportunities for dynamically adjusting the proteome composition, which poses constraints on the design of biotechnological production processes but may also avoid the initiation of deleterious stress responses.
8.4 Analysis of synthetic microbial communities for bioproduction processes: real-time monitoring and optimality
Participants: E. Cinquemani, H. de Jong, J. Geiselmann, T. Muszbek, G. Roudaut, M. Sangster.
Modelling, analysis and control of microbial community dynamics is a fast-developing subject with great potential implications in the understanding of natural processes and the enhancement of biotechnological processes. Within the now-ended project IPL COSY (Section 9), we picked up the challenge to design and investigate the dynamics of synthetically engineered microbial communities with a consortium of Inria partners, and to test control strategies in vivo.
In IBIS/MICROCOSME, in particular, we have addressed the design of a bacterial community of two E. coli strains, mimicking mutualistic relationships found in nature, and with the potential to outperform a producer strain working in isolation in the production of a heterologous protein. We developed an ODE model of the key growth phenotypes of the community and their interactions, calibrated the model on literature data, and analysed the model for an in-depth understanding of the conditions supporting coexistence and of the tradeoffs encountered in this production process 10. The synthetic consortium is now at an advanced bioengineering stage. Ongoing model re-calibration based on our own data, including experiments on our automated platform (Section 3), now allows us to implement feedback control strategies for stabilization of biomasses to desirable bioproduction regimes. This work is currently being carried out by Maaike Sangster in the framework of her PhD thesis.
The developments in 10 have spurred a number of novel questions with theoretical and practical relevance. Model-based feedback control of microbial communities requires as a first step to be able to estimate the full system state in real time (abundance of the different species, but also current metabolite concentrations in the bioreactor). This is a nontrivial problem since typically only partial, indirect state measurements are available. In a publication in the International Journal of Robust and Nonlinear Control 14, in collaboration with VALSE, we have addressed observability for the (nonlinear dynamical) model in 10 and the development of real-time estimators under the realistic assumption that only biomass abundance is monitored, and that the contribution from different species is discerned via fluorescent reporters. We have notably shown that, while total biomass measurements may suffice to estimate individual biomasses with a perfectly known model, the simultaneous use of the reporter measurements allows one to do so in absence of specific knowledge of the interactions among species, and is essential for estimating substrate concentrations.
In parallel, we have pushed further the investigation of the synthetic community design for bioproduction optimization. In our previous work 10, bioproduction is accomplished by one species, while the second species grows on byproducts excreted by the first and eventually impairing the growth of the latter. Although this helps growth of the producing species, we investigated whether a modification of the second species so as to exploit the byproducts of the first species for additional bioproduction could improve the performance of the consortium as a whole. In work in collaboration with BIOCORE that we just submitted 25, in addition to a theoretical analysis of equilibria and coexistence regimes for this generalized community, we demonstrate that a second producing species can indeed outperform our original consortium design, thus shifting the interest from the commonly evoked concept of division of labor (different tasks for different species) to distribution of labor (same task across different species). The ANR Ctrl-AB puts these concepts to work for the synergistic growth of E. coli and microalgea (Section 9).
8.5 Modelling and inference of mRNA degradation
Participants: A. Caddeo, M. Cocaign-Bousquet, T. Etienne, O. Feudjio, D. Ropers.
Metabolic engineering strategies are crucial for the development of bacterial cell factories with improved performance. Until now, metabolic networks capable of (optimally) producing a certain compound of interest have been designed based on approaches integrating large-scale data on the steady-state concentrations of mRNA, protein and metabolites, sometimes with dynamic data on fluxes, but rarely with any information on mRNA degradation.
In a review published in Biotechnology Advances with colleagues at the Toulouse Biotechnology Institute and IBPC Paris 19, Thibault Etienne, Delphine Ropers, and Muriel Cocaign-Bousquet have compiled evidence that mRNA degradation is a key regulatory level in E. coli that metabolic engineering strategies should take into account. The degradation of mRNAs interacts with transcription and translation, two other gene expression processes, while mRNA degradation and cellular metabolism are intertwined: metabolic activity can be controlled by changes in mRNA degradation and in return, the activity of the mRNA degradation machinery is controlled by metabolic factors. This calls for mathematical models describing the crosstalk between mRNA degradation dynamics and other cellular processes that can be used to design novel mRNA degradation-based metabolic engineering strategies in biotechnology. While some efforts in this direction have started, model integration of different biological processus remains challenging. In the framework of the ANR project RIBECO (Section 9), Thibault Etienne, Muriel Cocaign-Bousquet and Delphine Ropers have developed a model of mRNA degradation that allows to analyse the regulation of mRNA degradation 3. The model is currently being used to interpret large data sets corresponding to the degradation kinetics of 4254 mRNAs in E. coli cells growing in different environmental and genetic backgrounds.
8.6 Inference of parameters on lineage trees
Participants: E. Cinquemani, A. Marguet, E. Reginato.
Recent technological developments have made it possible to obtain time-course single-cell measurements of gene expression as well as the associated lineage information. However, most of the existing methods for the identification of mathematical models of gene expression are not well-suited to single-cell data and make the simplifying assumptions that cells in a population are independent, thus ignoring cell lineages. The development of statistical tools taking into account the correlations between individual cells will allow in particular for the investigation of inheritance of traits in bacterial populations.Modelling and identification of gene expression models with mother-daughter inheritance have been investigated previously through a collaboration among Eugenio Cinquemani, Marc Lavielle (XPOP, Inria Saclay–Île-de-France) and Aline Marguet, which led to a publication in Bioinformatics 9. Here, a novel method generalizing the Mixed-Effects (ME) paradigm to tree-structured populations, called ARME, was developed for the inference of an Auto-Regressive (AR) inheritance model of kinetic gene expression parameters. The method assumes deterministic gene expression dynamics, which constitutes a limitation for systems with strong gene expression noise. Work this year addressed generalization of the method to stochastic gene expression dynamics. Focusing on noisy promoter activation dynamics in response to an environmental stimulus common to all cells, we considered a model where promoter activation is described by an Ornstein-Uhlenbeck process, and extended the ARME approach so as to cope with this additional noise source via Kalman filtering techniques. We demonstrated in simulation that the new method ourperforms its predecessor in case of significant promoter noise. The work was presented at the international IFAC conference SysId 2021 and published in the conference proceedings 16. Further exploration of the subject, including inference of inheritance dynamics from different types of data, constitutes the subject of the Ph.D. project of Emrys Reginato, started in the fall of 2020.
8.7 Mathematical analysis of structured branching populations
Participant: A. Marguet.
The investigation of cellular populations at a single-cell level has already led to the discovery of important phenomena, such as the occurrence of different phenotypes in an isogenic population. Nowadays, several experimental techniques, such as microscopy combined with the use of microfluidic devices, enable one to take investigation further by providing time-profiles of the dynamics of individual cells over entire lineage trees. The development of models that take into account the genealogy is an important step in the study of inheritance in bacterial population. And the efficient analysis of single-cell data relies on the mathematical analysis of those models.Structured branching processes allows for the study of populations, where the lifecycle of each cell is governed by a given characteristic or trait, such as the internal concentration of proteins. The dependence on this characteristic of cellular mechanisms, such as division or ageing, has been investigated by Aline Marguet via the mathematical analysis of those processes. In collaboration with Charline Smadi (INRAE Grenoble), Aline Marguet investigated the long-time behavior of continuous-state nonlinear branching processes with catastrophes. This work, which has been published in the Electronic Journal of Probability 17, is motivated by the study of parasite infection in a cell line. The continuous-state branching processes model the dynamic of the parasites inside a cell and the catastrophes model cellular divisions that dilute the number of parasites in the cell. We pinpoint conditions on the dynamics of the parasites to explode or get extinct in a cell line. Combining some results of 17 and the Many-to-one formula proved in 27 that characterizes the dynamic of a typical individual, we investigated the long-time behaviour of an infected cell population. In this companion work, submitted for publication 26, we model the dynamic of the cell population using a structured branching process where the cell cycle depends on the dynamics of the parasites contained in the cell. We investigate the role of different sharing strategies at division on the fate of the cell population and give conditions for recovery or proliferation of the infection. In particular, we proved that for a constant division rate, according to our model, the more asymetric the repartition of the parasites at division, the more the cell population can survive strong parasite infections.
The study of the asymptotic behavior of general non-conservative semigroups is important for several aspects of branching processes, especially to prove the efficiency of statistical procedures. Vincent Bansaye from École Polytechnique, Bertrand Cloez from INRAE Montpellier, Pierre Gabriel from Université Versailles Saint-Quentin, and Aline Marguet, obtained necessary and sufficient conditions for uniform exponential contraction in weighted total variation norm of non-conservative semigroups. It ensures the existence of Perron eigenelements and provides quantitative estimates of spectral gaps, complementing Krein-Rutman theorems and generalizing recent results relying on probabilistic approaches. This work has been submitted for publication 24.
8.8 Qualitative modelling of synthetic gene circuits
Participant: H. de Jong.
Qualitative modelling approaches are promising and still underexploited tools for the analysis and design of synthetic gene circuits. They can make predictions of circuit behavior in the absence of precise, quantitative information. Moreover, they provide direct insight into the relation between the feedback structure and the dynamical properties of a network. In a book chapter for an edited volume 21, co-written with Madalena Chaves (BIOCORE), we review qualitative modelling approaches by focusing on two specific formalisms, Boolean networks and piecewise-linear differential equations, and illustrate their application by means of three well-known synthetic circuits. We describe various methods for the analysis of state transition graphs, the discrete representations of the network dynamics that are generated in both modelling frameworks. We also briefly present the problem of controlling synthetic circuits, an emerging topic that could profit from the capacity of qualitative modelling approaches to rapidly scan a space of design alternatives.
9 Partnerships and cooperations
Participants: I. Cancino Aguirre, E. Cinquemani, M. Cocaign-Bousquet, H. de Jong, T. Etienne, J. Geiselmann, A. Marguet, C. Medous, T. Muszbek, A. Pavlou, D. Ropers, M. Sangster.
9.1 International initiatives
H. de Jong and D. Ropers collaborate with T. Gedeon (Montana State University), former invited researcher in our former team IBIS, on research allocation strategies in microorganisms.
I. Cancino Aguirre, H. de Jong and D. Ropers have started a collaboration with L. Carvalho (Francis Crick Institute, London) on the molecular control of the growth and pathogenicity of mycobacterium species.
9.2 National initiatives
Project name | MEMIP – Modèles à effets mixtes de processus intracellulaires : méthodes, outils et applications |
Coordinator | G. Batt |
MICROCOSME participants | E. Cinquemani, A. Marguet, D. Ropers |
Type | ANR project (2016-2021) |
Project name | MAXIMIC: Optimal control of microbial cells by natural and synthetic strategies |
Coordinator | H. de Jong |
MICROCOSME participants | C. Boyat, E. Cinquemani, J. Geiselmann, H. de Jong, A. Pavlou, C. Pinel, D. Ropers |
Type | ANR project (2017-2021) |
Web page | Link to project description |
Project name | RIBECO (RIBonucleotide ECOnomy): Engineering RNA life cycle to optimize economy of microbial energy |
Coordinator | M. Cocaign-Bousquet |
MICROCOSME participants | E. Cinquemani, T. Etienne, D. Ropers |
Type | ANR project (2018-2023) |
Web page | Link to project description |
Project name | Ctrl-AB : Optimization and control of the productivity of an algal-bacterial consortium |
Coordinator | J.-L. Gouzé |
MICROCOSME participants | E. Cinquemani, H. de Jong, J. Geiselmann, A. Marguet, T. Muszbek, C. Pinel, M. Sangster |
Type | ANR project (2020-2024) |
Project name | COSY: real-time COntrol of SYnthetic microbial communities |
Coordinator | E. Cinquemani |
MICROCOSME participants | E. Cinquemani, H. de Jong, J. Geiselmann, M. Mauri, T. Muszbek, C. Pinel, D. Ropers, M. Sangster |
Type | Inria Project Lab (2017-2021) |
Web page | Link to project description |
Project name | OPTICO : OPTImal COntrol software for microbial communities in a system of minibioreactors |
Coordinator | E. Cinquemani |
MICROCOSME participants | E. Cinquemani, H. de Jong, J. Geiselmann, T. Muszbek |
Type | Inria ADT (2019-2021) |
In addition to the above projects, A. Marguet contributes to the ANR JCJC NOLO of Bertrand Cloez (INRAE Montpellier) on non-local branching processes. J. Geiselmann and H. de Jong work with P. Hersen (Unité Physico-chimique, Institut Curie) on growth control in microorganisms.
9.3 Regional initiatives
Project name | MOSTIC: Stochastic modelling and inference for cell communities in interaction |
Coordinator | A. Marguet |
MICROCOSME participants | E. Cinquemani, J. Geiselmann, A. Marguet, C. Medous |
Type | IXXI/BioSyl project (2020-2022) |
Web page | Link to project description |
Project name | AnaComBa: Analyse de Communautés Bactériennes : modélisation stochastique |
Coordinator | A. Marguet & L. Coquille |
MICROCOSME participants | E. Cinquemani, A. Marguet, C. Medous |
Type | Equipe-Action du LABEX Persyval (2021-2024) |
10 Dissemination
Participants: E. Cinquemani, T. Clavier, H. de Jong, T. Etienne, J. Geiselmann, A. Marguet, C. Medous, T. Muszbek, A. Pavlou, E. Reginato, D. Ropers, M. Sangster.
10.1 Promoting scientific activities
10.1.1 Scientific events: organisation
Member of organizing committees
MICROCOSME members | Conference, workshop, school | Date |
Eugenio Cinquemani | Modelling Heterogeneous Populations with applications in Biology, online | May 2021 |
Eugenio Cinquemani | 19th International Conference on Computational Methods in Systems Biology (CMSB 2021), Bordeaux/online | Sep 2021 |
Hidde de Jong | CompSysBio: Advanced Lecture Course on Computational Systems Biology, Aussois | Nov 2021 |
Aline Marguet | Biohasard 2021: Stochastic Models for Biology, online | May-Jun 2021 |
10.1.2 Scientific events: selection
Chair of conference program committees
MICROCOSME members | Conference, workshop, school | Role |
Eugenio Cinquemani | European Control Conference (ECC 2021) | Associate editor |
Eugenio Cinquemani | 19th International Conference on Computational Methods in Systems Biology (CMSB 2021), Bordeaux/online 22 | Program co-chair |
Member of conference program committees
MICROCOSME member | Conference, workshop, program |
Eugenio Cinquemani | ECC 2021, ECC 2022, CMSB 2021 |
Hidde de Jong | IFAC DYCOPS-CAB 2022 |
Delphine Ropers | JOBIM2021, CSBio 2021 |
10.1.3 Journal
Member of Editorial Boards
MICROCOSME member | Journal |
Johannes Geiselmann | Frontiers in Microbiology (reviews editor) |
Hidde de Jong | Journal of Mathematical Biology |
Hidde de Jong | Biosystems (reviews editor) |
10.1.4 Invited talks and other presentations
Eugenio Cinquemani
Title | Event and location | Date |
Inference of the statistics of a promoter process from population-snapshot gene expression data | Invited presentation at Biohasard: Stochastic models for biology, online | Jun 2021 |
Inheritance and variability of kinetic gene expression parameters in microbial cells: Modelling and inference from lineage tree data | Invited seminar at University of Edinburgh, Theoretical Biophysics (Rosalind Allen's group), online | Feb 2021 |
Hidde de Jong
Title | Event and location | Date |
Dynamic models integrating metabolism and gene expression | Blackboard course at CompSysBio 2022, Aussois | Nov 2021 |
Natural and synthetic control of resource allocation in microorganisms | Invited virtual talk in "Population Dynamics Seminars, University of Edinburgh | Mar 2021 |
Mathematical modeling and control of resource allocation in microorganisms | Invited virtual presentation at Journées nationales du GDR IM | Mar 2021 |
Aline Marguet
Title | Event and location | Date |
Stochastic gene expression: modelling and inference from lineage trees | Séminaire de Probabilités et Statistiques, Montpellier | Feb 2021 |
Stochastic gene expression: modelling and inference from lineage trees | Séminaire de Modélisation aléatoire du vivant, Paris | June 2021 |
Identification of stochastic gene expression models over lineage trees | 19th IFAC symposium on System Identification, Padova | Jul 2021 |
Comportement asymptotique d'une population de cellules infectée par des parasites | Invited talk at Journées MAS 2021, online | Aug 2021 |
Antrea Pavlou
Title | Event and location | Date |
Insights into bacterial resource allocation in changing environments using a combination of experimental and computational approaches | GDR BIOSS seminar, online | Apr 2021 |
Delphine Ropers
Title | Event and location | Date |
Model-based interpretation of high-throughput data: Integrative analysis of bacterial mRNA decay | Invited talk at Computational and Mathematical Biology seminars, Grenoble | Oct 2021 |
Model-based interpretation of high-throughput data: Integrative analysis of bacterial mRNA decay | Invited talk at Journée nationale du GT BIOSS, Lyon | Nov 2021 |
Maaike Sangster
Title | Event and location | Date |
Development, characterization and control of E.coli communities on an automated experimental platform | CompSysBio, Aussois | Nov 2021 |
10.1.5 Scientific evaluation and expertise
MICROCOSME member | Organism | Role |
Johannes Geiselmann | UMR5240 CNRS-UCBL-INSA-BayerCropScience | Member scientific council |
Hidde de Jong | Microbiology and Food Chain Department, Inrae | Member scientific council |
Hidde de Jong | Univ Grenoble Alpes | Member scientific council of Pôle MSTIC |
Hidde de Jong | Univ Grenoble Alpes | Member of Vice-Présidence Recherche & Innovation élargie |
Hidde de Jong | Univ Grenoble Alpes | Member of Collège des Ecoles Doctorales |
Hidde de Jong | Fédération QuantAlps, Grenoble | Board member |
Delphine Ropers | INRA-Inria | Member selection committee PhD grants |
Delphine Ropers | Elixir | Member of Microbial Biotechnology Community |
10.1.6 Research administration
MICROCOSME member | Committee | Role |
Eugenio Cinquemani | Inria Grenoble - Rhône-Alpes | Member Comité des Emplois Scientifiques (CES) |
Eugenio Cinquemani | Inria Grenoble - Rhône-Alpes | Member Comité des Utilisateurs des Moyens Informatiques (CUMI) |
Eugenio Cinquemani | Inria | Member Comité Administrative Paritaire (CAP) |
Eugenio Cinquemani | Inria Grenoble - Rhône-Alpes | Member Comité Développement Technologique (CDT) |
Hidde de Jong | Inria Grenoble - Rhône-Alpes | Scientific director (délégué scientifique), member of board of research center |
Hidde de Jong | Inria Grenoble - Rhône-Alpes | President Comité des équipes-projets (CEP) |
Hidde de Jong | Inria Grenoble - Rhône-Alpes | Member scientific council (COS) |
Hidde de Jong | Inria Grenoble - Rhône-Alpes | Member Comité des Emplois Scientifiques (CES) |
Hidde de Jong | Inria Grenoble - Rhône-Alpes | Member Comité Développement Technologique (CDT) |
Hidde de Jong | Inria Grenoble - Rhône-Alpes | Member Comité des Etudes Doctorales (CED) |
Aline Marguet | Inria Grenoble - Rhône-Alpes | Member Comité du centre |
Aline Marguet | Inria Grenoble - Rhône-Alpes | Member Comité des études doctorales |
Aline Marguet | Inria Grenoble - Rhône-Alpes | Correspondent Sciences Environnements Sociétés |
Delphine Ropers | Inria Grenoble - Rhône-Alpes | Référente chercheurs |
Delphine Ropers | Inria Grenoble - Rhône-Alpes | Co-coordinator of mentoring program |
10.1.7 Recruitment committees
MICROCOSME member | Organism | Recruitment |
Eugenio Cinquemani | Inria Grenoble - Rhône-Alpes | Chargés de recherche (jury d'admissibilité) |
Hidde de Jong | Inria Grenoble - Rhône-Alpes | IFSP 2021 (jury d'admission) |
Johannes Geiselmann | Univ Grenoble - Alpes | Professor |
Hidde de Jong | Inria Grenoble - Rhône-Alpes | CRCN & IFSP 2022 (jury d'admissibilité) |
Delphine Ropers | Inria national | SRP & ARP (jury d'admissibilité) |
10.2 Teaching - Supervision - Juries
10.2.1 Teaching
Eugenio Cinquemani
- Course: Statistics for systems biology, M1, Master Approches Interdisciplinaires du Vivant, CRI/Univ Paris Descartes (20 h, also in charge of 20 h of practicals)
- Course: Modelling and identification of metabolic networks, M1, Phelma, INP Grenoble (4 h)
Hidde de Jong
- Course and practicals: Modeling and simulation of gene regulatory networks, M2, BIM, INSA de Lyon (25 h)
Aline Marguet
- Practicals: Biostatistics, M2, Univ Grenoble Alpes (27 h)
Charles Medous
- Course and practicals: Introduction to mathematical tools, L1, Life sciences, Univ Grenoble Alpes (32 h)
Antrea Pavlou
- Course: Statistics for biology, L2, Mathematics, Univ Grenoble Alpes (30h)
Emrys Reginato
- Course and practicals: Algorithmic and Python programming, L1, Earth Sciences, Univ Grenoble Alpes (37h)
- Practicals: Algorithmic and Python programming, L1, Physics, Univ Grenoble Alpes (22h)
- Tutorial: Introduction to linear algebra, L1, Physics, Univ Grenoble Alpes (18h)
Delphine Ropers
- Course and practicals: Modelling in systems biology, M1, Phelma, INP Grenoble (16 h)
- Course and practicals: Cell systems biology and modelling cell functions, M1, Master ingéniérie de la santé, Univ Grenoble Alpes (24 h)
- Course: Modeling and simulation of genetic regulatory networks, M2, INSA de Toulouse (4 h)
- Course: Metabolic modelling with omics data, M2, IA4 Health International master course, Univ Grenoble Alpes (6h)
Maaike Sangster
- Practicals: Cellular biology, L2, Univ Grenoble Alpes (28 h)
10.2.2 Supervision
- HdR: Delphine Ropers, Modelling biochemical reaction networks in bacteria – From data to models and back 23, Univ Claude Bernard, Lyon I, May 2021
- PhD in progress: Antrea Pavlou, Experimental and computational analysis of bacterial self-replicators. Supervisors: Hidde de Jong and Johannes Geiselmann
- PhD in progress: Maaike Sangster, Development, characterization and control of E. coli communities on an automated experimental platform. Supervisors: Eugenio Cinquemani and Johannes Geiselmann
- PhD in progress: Emrys Reginato, Development, analysis, and inference of stochastic models of gene expression in growing cell populations. Supervisors: Eugenio Cinquemani and Aline Marguet
- PhD in progress: Thibault Clavier, Genetic growth control to maximize the bioproduction in E. coli. Supervisors: Johannes Geiselmann and Hidde de Jong
- PhD in progress: Ignacia Cancino Aguirre, Computational analysis of metabolic strategies in pathogenic bacteria. Supervisors: Delphine Ropers and Hidde de Jong
- PhD in progress: Charles Medous, Analysis of bacterial communities : stochastic modelling. Supervisors: Loren Coquille (Institut Fourier, Grenoble), Aline Marguet, Charline Smadi (Inrae Grenoble).
10.2.3 Juries
PhD thesis committees
MICROCOSME member | Role | PhD student | University | Date |
Eugenio Cinquemani | Examinateur | Hussein Kanso | Univ d'Avignon | May 2021 |
Eugenio Cinquemani | Examinateur | Alex dos Reis de Souza | Univ Lille | Sept 2021 |
Eugenio Cinquemani | Examinateur | Arthur Carcano | Univ de Paris | Dec 2021 |
Johannes Geiselmann | Rapporteur | Niel Cox | Univ Paul Sabatier, Toulouse | Oct 2021 |
Johannes Geiselmann | Rapporteur | Elsa de Val | INSA Lyon | Nov 2021 |
Johannes Geiselmann | Examinateur | Sunil Nahata | ENS Lyon and Univ Grenoble Alpes | Dec 2021 |
Johannes Geiselmann | Rapporteur | Isabelle Simon | Univ Orléans | Dec 2021 |
Hidde de Jong | Examinateur | Agustín Yabo | Univ Côte d'Azur, Nice | Dec 2021 |
Delphine Ropers | Examinatrice | Chetan Aditya | Univ Paris | Oct 2021 |
Delphine Ropers | Rapportrice | Alexey Koshkin | Univ de Lyon | Dec 2021 |
Habilitation (HDR) committees
MICROCOSME member | Role | HDR candidate | University | Date |
Hidde de Jong | Rapporteur | Carole Knibbe | INSA de Lyon/Univ Lyon Claude Bernard | Jan 2021 |
Johannes Geiselmann | Examinateur | Delphine Ropers | Univ Lyon | May 2021 |
Johannes Geiselmann | Examinateur | Audrey Le Gouëllec | Univ Grenoble Alpes | Oct 2021 |
PhD advisory committees
MICROCOSME member | PhD student | University |
Eugenio Cinquemani | Arthur Carcano | Institut Pasteur/Inria |
Eugenio Cinquemani | Marielle Peré | Univ Côte d'Azur |
Johannes Geiselmann | Clément Caffaratti | Univ Grenoble Alpes |
Hidde de Jong | Kapil Newar | Univ Grenoble Alpes |
Delphine Ropers | Charlotte Roux | Univ Paul Sabatier, Toulouse |
Delphine Ropers | Zélia Bontemps | Univ de Lyon |
10.2.4 Teaching administration
- Eugenio Cinquemani organizes a module on statistics in systems biology at CRI/Univ Paris Descartes.
- Delphine Ropers organizes a module on the mathematical modelling of biological systems at PHELMA, INP Grenoble.
- Hidde de Jong organizes a module on the modelling of genetic and metabolic networks at INSA de Lyon.
11 Scientific production
11.1 Major publications
- 1 articleEstimation of time-varying growth, uptake and excretion rates from dynamic metabolomics data.Bioinformatics33142017, i301-i310
- 2 articleStochastic reaction networks with input processes: Analysis and application to gene expression inference.Automatica1012019, 150-156
- 3 articleCompetitive effects in bacterial mRNA decay.Journal of Theoretical Biology504November 2020
- 4 articleDynamical allocation of cellular resources as an optimal control problem: Novel insights into microbial growth strategies.PLoS Computational Biology123March 2016, e1004802
- 5 articleStatistical estimation in a randomly structured branching population.Stochastic Processes and their Applications129122019, 5236-5277
- 6 articleA synthetic growth switch based on controlled expression of RNA polymerase.Molecular Systems Biology1111November 2015, 840
- 7 articleWhat population reveals about individual cell identity: Single-cell parameter estimation of models of gene expression in yeast.PLoS Computational Biology122February 2016, e1004706
- 8 articleOptimal proteome allocation and the temperature dependence of microbial growth laws.npj Systems Biology and Applications7142021
- 9 articleInheritance and variability of kinetic gene expression parameters in microbial cells: modeling and inference from lineage tree data.Bioinformatics35142019, i586-i595
- 10 articleEnhanced production of heterologous proteins by a synthetic microbial community: Conditions and trade-offs.PLoS Computational Biology1642020, e1007795
- 11 articleThe Csr System Regulates Escherichia coli Fitness by Controlling Glycogen Accumulation and Energy Levels.mBio85October 2017, 1-14
- 12 articleThe post-transcriptional regulatory system CSR controls the balance of metabolic pools in upper glycolysis of Escherichia coli.Molecular Microbiology1004May 2016, 686-700
- 13 articleAcetate metabolism and the inhibition of bacterial growth by acetate.Journal of Bacteriology20113July 2019, 147 - 166
11.2 Publications of the year
International journals
Scientific book chapters
Edition (books, proceedings, special issue of a journal)
Doctoral dissertations and habilitation theses
Reports & preprints
11.3 Cited publications
- 26 unpublishedParasite infection in a cell population with deaths. 2020, Preprint
- 27 articleUniform sampling in a structured branching population.Bernoulli254A2019, 2649-2695