Keywords
Computer Science and Digital Science
- A5.4.2. Activity recognition
- A5.4.5. Object tracking and motion analysis
- A5.7.3. Speech
- A5.7.4. Analysis
- A5.10.2. Perception
- A5.10.4. Robot control
- A5.10.5. Robot interaction (with the environment, humans, other robots)
- A5.10.7. Learning
- A9.2. Machine learning
- A9.3. Signal analysis
- A9.5. Robotics
Other Research Topics and Application Domains
- B2. Health
- B5.6. Robotic systems
1 Team members, visitors, external collaborators
Research Scientists
- Xavier Alameda-Pineda [Team leader, Inria, Researcher, from Jul 2021, HDR]
- Patrice Horaud [Inria, Senior Researcher, from Jul 2021, HDR]
- Chris Reinke [Inria, Starting Research Position, from Jul 2021]
- Timothee Wintz [Inria, Starting Research Position, from Jul 2021]
PhD Students
- Louis Airale [Univ Grenoble Alpes, from Jul 2021]
- Anand Ballou [Univ Grenoble Alpes, from Jul 2021]
- Xiaoyu Bie [Univ Grenoble Alpes, from Jul 2021]
- Guillaume Delorme [Inria, from Jul 2021]
- Wen Guo [Université polytechnique de Catalogne - Espagne, from Jul 2021]
- Gaetan Lepage [Inria, from Jul 2021]
- Xiaoyu Lin [Inria, from Jul 2021]
- Yihong Xu [Inria, from Jul 2021]
Technical Staff
- Soraya Arias [Inria, Engineer, from Jul 2021]
- Alex Auternaud [Inria, Engineer, from Jul 2021]
- Luis Gomez Camara [Inria, Engineer, from Jul 2021]
- Zhiqi Kang [Inria, Engineer, from Jul 2021 until Sep 2021]
- Matthieu Py [Inria, Engineer, from Jul 2021]
- Nicolas Turro [Inria, Engineer, July 2021]
Interns and Apprentices
- Natanael Dubois–Quilici [Inria, from Jul 2021 until Sep 2021]
- David Emukpere [Inria, from Jul 2021 until Aug 2021]
Administrative Assistant
- Nathalie Gillot [Inria, from Jul 2021]
Visiting Scientists
- Timothee Dhaussy [Institut supérieur d'électronique de Paris, Sep 2021]
- Hanyu Xuan [Université normale de la Chine de l'Est (ECNU) Shanghai, from Jul 2021]
External Collaborator
- Laurent Girin [Institut polytechnique de Grenoble, from Jul 2021, HDR]
2 Overall objectives
In recent years, social robots have been introduced into public spaces, such as museums, airports, commercial malls, banks, show-rooms, schools, universities, hospitals, and retirement homes, to mention a few examples. In addition to classical robotic skills such as navigating in complex environments, grasping and manipulating objects, i.e. physical interactions, social robots must be able to communicate with people and to adopt appropriate behavior. Welcoming newcomers, providing various pieces of information, and entertaining groups of people are typical services that social robots are expected to provide in the near future.
Prominent examples of this type of robots, with great scientific, technological, economical and social impact, are Socially Assistive Robots (SARs). SARs are likely to play an important role in healthcare and psychological well-being, in particular during non-medical phases inherent to any hospital process 97, 82, 87, 96. It is well established that properly handling patients during these phases is of paramount importance, as crucial as the medical phases. It is worth to be noticed that non-medical phases represent a large portion of the total hospitalization time. It has been acknowledged that SARs could be well suited for explaining complex medical concepts to patients with limited health literacy 38. They can coordinate with medical staff and potentially reduce the amount of human resources required for instructing each individual patient 58. There is a consensus among physicians and psychotherapists that the use of robots in group settings has a positive impact on health, such as decreased stress and loneliness, and improved mood and sociability 39, 29. Therefore, one can confidently assert that social-robot research is likely to have a great potential for healthcare and that robot companionship is likely to improve both psychological well-being and the relationship between patients and hospital professionals. Beyond healthcare, socially intelligent robots will have applications in education, retail, public relationship and communication, etc. Thanks to the collaboration with industrial partners we can expect direct impact in tourism (PAL Robotics) and education (ERM Automatismes Industriels).
Nevertheless, today's state-of-the-art in robotics is not well-suited to fulfill these needs. Indeed, social-robot platforms that are currently available, whether laboratory prototypes or commercial systems, are based on interface technologies borrowed from smartphones, namely touch-screens and voice commands. This creates two bottlenecks: (i) it limits the use of robots to a handful of simple scenarios which leads to (ii) social robots not being well accepted by a large percentage of users such as the elderly. While there are research programs and projects which have tackled some of these challenges, existing commercially available robots cannot (or only to a very limited extent) recognize individual behaviors (e.g. facial expressions, hand- and body-gestures, head- and eye-gaze) or group behaviors (e.g. who looks at whom, who speaks to whom, who needs robot assistance, etc.). They cannot distinguish between patients, family members, and carers in order to adopt proper attitudes and to exchange adequate pieces of information. They do not have the ability to take social (or non-verbal) signals into account while they are engaged in spoken dialogue and they cannot connect the dialogue with the persons and objects that are physically present in their surroundings. These limitations are largely due to the fact that human-robot interaction technologies are based on algorithms that have been designed for reactive single-user dialog, mostly based on keyword spotting where the robot waits to be instructed what to do based on a limited set of scripted actions. In some cases, the user even has to resort to a handheld microphone or smartphone to overcome the limitations of the built-in microphones and speech recognition systems. We would like to develop robots that are responsible for their perception, and act to enhance the quality of the signals they receive, instead of asking the users to adapt their behavior to the robotic platform.
The scientific ambition of RobotLearn is to train robots to acquire the capacity to look , listen , learn , move and speak in a socially acceptable manner.
The scientific ambition of RobotLearn, outlined above, may be broken down into the following three objectives:
- Develop deep probabilistic models and methods that allow the fusion of audio and visual data, possibly sequential, recorded with cameras and microphones, and in particular with sensors onboard of robots.
- Increase the performance of human behaviour understanding using deep probabilistic models and jointly exploiting auditory and visual information.
- Learn robot-action policies that are socially acceptable and that enable robots to better perceive humans and the physical environment.
This will require several new scientific and technological developments. The scientific objectives of RobotLearn stand at the cross-roads of several topics: computer vision, audio signal processing, speech technology, statistical learning, deep learning, and robotics. In partnership with several companies (e.g. PAL Robotics and ERM Automatismes Industriels), the technological objective is to launch a brand new generation of robots that are flexible enough to adapt to the needs of the users, and not the other way around. The experimental objective is to validate the scientific and technological progress in the real world. Furthermore, we believe that RobotLearn will contribute with tools and methods able to process robotic data (perception and action signals) in such a way that connections with more abstract representations (semantics, knowledge) are possible. The developments needed to discover and use such connections could be addressed through collaborations. Similarly, aspects related to robot deployment in the consumer world, such as ethics and acceptability will be addressed in collaboration, for instance, with the Broca day-care hospital in Paris.
RobotLearn will build on the scientific expertise that has been developed over the past years by the Perception team. The main emphasis of the Perception team has been the development of audio-visual machine perception, from fundamental principles to the implementation of human-robot interaction algorithms and of software based on these principles.
Particular emphasis has been put on statistical learning and inference principles, and their implementation in terms of practical solvers. For the past five years, the following problems were addressed: separation and localization of multiple (static or moving) audio sources, speech enhancement and separation4, multiple person tracking using visual, audio, and audio-visual observations, head-pose and eye-gaze estimation and tracking for understanding human-human and human-robot social interactions, and visually- and audio-guided robot control.
The formulations of choice have been latent variable mixture models, dynamic Bayesian networks (DBNs), and their extensions. Robust mixture models were developed, e.g. for clustering audio-visual data 55, for modeling the acoustic-articulatory tract 57, or for registering multiple point sets 50. DBNs may well be viewed as hybrid state-space models, i.e. models that combine continuous and discrete latent variables. DBNs often lead to intractable maximum a posteriori (MAP) problems. For this reason, approximate inference has been thoroughly investigated. In particular a number of variational expectation maximization (VEM) algorithms were developed, such as high-dimensional regression with latent output 45 and with spatial Markov dependencies 43, sound-source separation and localization 44, 63, multiple person tracking using visual observations 35, audio observations 37, 72, or audio-visual fusion 56, 15, head-pose and eye-gaze estimation and tracking 48, 83, 14. Variational approximation has also been combined with generative deep neural networks for audio-visual speech enhancement 93. Very recently, we have reviewed the literature on deep probabilistic sequential modeling, and proposed a model class called dynamical variational autoencoders, see 16 (preprint).
In parallel, we addressed the problems of speech localization, speech separation and speech enhancement in reverberant environments. This is an extremely important topic in the framework of robot audition. Nonetheless, the formulation that we proposed and the associated algorithms can be used in the general case of multi-channel audio signal processing in adverse acoustic conditions. Traditionally, audio signals are represented as spectrograms using the short-time Fourier transform (STFT). In the case of multiple channels, one has to combine spectrograms associated with different microphones and the multiplicative transfer function (MTF) is often used for this purpose. The multiplicative model is not well suited when the task consists of distinguishing between the direct-path sound, on one side, and early and late reverberations, on the other side. Instead we proposed to use the convolutive transfer function (CTF). The CTF model was combined with supervised localization 46 to yield a sound localization method that is immune to the presence of reverberation 78. We used a probabilistic setting to extend this method to multiple sound sources 79, to online localization and tracking 72, to dereverberation 73, and to speech separation and enhancement 77, 76. We also developed a method for online speech dereverberation 75.
The use of audio signal processing in robotics – robot audition – has received less attention, compared to the long history of research in robot vision. We contributed to this new research topic in several ways. We thoroughly studied the geometry of multiple microphones for the purpose of sound localization from time delays 32 and for fusing audio and visual data 33. The use of the CTF mentioned above, in conjunction with microphones embedded into robot heads, has been thoroughly investigated and implemented onto our robotic platforms 74, 71, 31. In parallel, we investigated novel approaches to sensor-based robot control based on reinforcement learning 65, 66.
3 Research program
RobotLearn will be structured in three research axes, allowing to develop socially intelligent robots, as depicted in the accompanying figure. First, on deep probabilistic models, which include the large family of deep neural network architectures, the large family of probabilistic models, and their intersection. Briefly, we will investigate how to jointly exploit the representation power of deep network together with the flexibility of probabilistic models. A well-known example of such combination are variational autoencoders. Deep probabilistic models are the methodological backbone of the proposed projet, and set the foundations of the two other research axes. Second, we will develop methods for the automatic understanding of human behavior from both auditory and visual data. To this aim we will design our algorithms to exploit the complementary nature of these two modalities, and adapt their inference and on-line update procedures to the computational resources available when operating with robotic platforms. Third, we will investigate models and tools allowing a robot to automatically learn the optimal social action policies. In other words, learn to select the best actions according to the social environment. Importantly, these action policies should also allow us to improve the robotic perception, in case this is needed to better understand the ongoing interaction. We believe that these two research axes, grounded on deep and probabilistic models, will ultimately enable us to train robots to acquire social intelligence, meaning, as discussed in the introduction, the capacity to look, listen, learn, move and speak.
3.1 Deep probabilistic models
A large number of perception and interaction processes require temporal modeling. Consider for example the task of extracting a clean speech signal from visual and audio data. Both modalities live in high-dimensional observation spaces and one challenge is to extract low-dimensional embeddings that encode information in a compact way and to update it over time. These high-dimensional to low-dimensional mappings are nonlinear in the general case. Moreover, audio and visual data are corrupted by various perturbations, e.g. by the presence of background noise which is mixed up with the speech signal uttered by a person of interest, or by head movements that overlap with lip movements. Finally, for robotics applications, the available data is scarce, and datasets captured in other settings can only serve as proxies, thus requiring either adaptation 99 or the use of unsupervised models 36. Therefore, the problem is manyfold: to extract low-dimensional compact representations from high-dimensional inputs, to disregard useless data in order to retain information that is relevant for the task at hand, to update and maintain reliable information over time, and to do so in without (or with very few) annotated data from the robot.
This class of problems can be addressed in the framework of state-space models (SSMs). In their most general form, SSMs are stochastic nonlinear systems with latent variables. Such a system is composed of a state equation, that describes the dynamics of the latent (or state) variables, and observation equations (an observation equation for each sensorial modality ) that predict observations from the state of the system, namely:
where the latent vector evolves according to a nonlinear stationary Markov dynamic model driven by the observed control variable and corrupted by the noise . Similarly, the observed vectors are modeled with nonlinear stationary functions of the current state and current input, affected by noise . Models of this kind have been examined for decades and their complexity increases from linear-Gaussian models to nonlinear and non-Gaussian ones. Interestingly, they can also be viewed in the framework of probabilistic graphical models to represent the conditional dependencies between the variables. The objective of an SSM is to infer the sequence of latent variables by computing the posterior distribution of the latent variable, conditioned by the sequence of observations, .
When both and are linear and when the noise processes and are both Gaussian, this becomes a linear dynamical system (LDS), also well known as the Kalman filter (KF), which is usually solved in the framework of probabilistic latent variable models. Things become more complex when both and are nonlinear, as the integrals required by the evaluation of the posterior become intractable. Several methods were proposed to deal with nonlinear SSMs, e.g. Bayesian tracking with particle filters, the extended Kalman filter (EKF), and the unscented Kalman filter (UKF).
Outcomes of nonlinear and non-Gaussian Bayesian trackers based on sampling were reviewed and discussed 34, most notably the problems of degeneracy, choice of importance density, and resampling. The basic idea of EKF is to linearize the equations using a first-order Taylor expansion and to apply the standard KF to the linearized model. The additional error due to linearization is not taken into account which may lead to sub-optimal performance. Rather than approximating a nonlinear dynamical system with a linear one, UKF specifies the state distribution using a minimal set of deterministically selected sample points. The sample points, when propagated through the true nonlinear system, capture the posterior state distribution accurately up to the third-order Taylor expansion. An expectation-maximization (EM) algorithm was proposed in 88 that alternates between an extended Kalman smoother which estimates an approximate posterior distribution (E-step), and nonlinear regression using a Gaussian radial basis function network to approximate and (M-step).
An alternative to nonlinear SSMs is to consider different linear dynamic regimes and to introduce an additional discrete variable, a switch, that can take one out of values – the switching Kalman filter (SKF). The drawback of SKFs is the exponential increase of the number of mixture components of the posterior distribution over time, namely , hence an approximate posterior must be evaluated at each time step, e.g. the generalized pseudo-Bayes of order 2 (GPB2) algorithm 83.
A similar type of intractability (exponential increase of the number of mixture components of the posterior distribution) appears in the case when SSMs are used to track several objects and when there are several possible observations that are likely to be associated with each object. In such cases, additional discrete hidden variables are necessary, namely a variable that associates the -th observation with the -th object at time . Let these variables be denoted with , e.g. means that observation at is assigned to object . The number of mixture components of the posterior distribution after time steps is , where is the number of state variables (objects to be tracked) and is the number of observed variables. Problems like these can be solved in the framework of Bayesian variational inference. We developed a general framework for variational multiple object tracking and proposed several tractable variational expectation-maximization algorithms (VEM) for visual, audio, and audio-visual multiple-object tracking, 35, 72, 37, 15.
Very recently, there has been strong interest into building SSMs in the framework of deep neural networks (DNNs). This is a very promising topic of research for several reasons. It allows the representation of arbitrary nonlinear state and observation functions, and , using a plethora of feedforward and recurrent neural network architectures and hence to develop practical discriminative and generative deep filters, without the limitations of linear-Gaussian models that have been the state-of-the-art for several decades. In its general form, an RNN replaces eq. (1) with (for simplicity, we consider a single modality and hence we omit the modality index ):
where , , are hidden-to-hidden, input-to-hidden and hidden-to-output weight matrices, , are bias vectors, and , are activation functions. The discriminative formulation allows end-to-end learning using a loss and simple and scalable stochastic gradient descent methods, thus exploiting the power of deep neural networks to represent data. It opens the door to devising SSMs with high-dimensional observation spaces. Nevertheless, while discriminative recurrent neural network (RNN) learning is well understood and efficient training methods are available, they are strongly dependent on the availability of large corpora of annotated data. In some cases, data annotation can be done relatively easily, e.g. adding various noise types to speech signals, in many other cases, augmenting the data with the corresponding ground-truth annotations is cumbersome.
One can build on the analogy between SSMs, i.e. (1) and RNNs, i.e. (2). Roughly speaking, is the network input, is the internal (or hidden) state and is the output. A large number of combinations of feedforward and recurrent network architectures are possible in order to build the two functions. These combinations must be carefully studied as there is no universal solution to solve all the problems raised by processing complex audio and visual data. For example, the back-propagation Kalman filter (BKF) 59 combines a feedforward convolutional neural network (CNN) that transforms the input into into a low-dimensional vector which serves then as input for an RNN, improperly named Kalman filter.
In addition to require large amounts of annotated data for training their parameters, RNNs suffer from another main drawback: they are deterministic. Therefore, it is not possible to learn, exploit and track over time the uncertainty associated with the underlying temporal processes. Moreover, it is unclear how to use such models in unsupervised settings where the test data might be scarce and from a slighly different statistical distribution (typical case in robotic applications). Recently, there has been a burgeoning literature that addresses these issues, at the cross-roads of deep recurrent neural networks and probabilistic models. We recently released an extensive and comprehensive review of these model and methods and proposed several promising research avenues 16. In more detail, we proposed a novel class of models that may well be viewed as an umbrella for several methodologies that were recently proposed in the literature, we unified the notations, and we identified a number of promising research lines. We termed this class of models Dynamic Variational Autoencoders (DVAE). In one sentence, this means that we aim at modeling recurrent processes and the associated uncertainty by means of deep neural networks and probabilistic models. We name the larger family of all these methods as Deep Probabilistic Models (DPMs), which form a backbone among the methodological foundations of RobotLearn.
Learning DPMs is challenging from the theoretical, methodological and computational points of view. Indeed, the problem of learning, for instance, deep generative Bayesian filters in the framework of nonlinear and non-Gaussian SSMs remains intractable and approximate solutions, that are both optimal from a theoretical point of view and efficient from a computational point of view, remain to be proposed. We plan to investigate both discriminative and generative deep recurrent Bayesian networks and to apply them to audio, visual and audio-visual processing tasks.
Exemplar application: audio-visual speech enhancement
Speech enhancement is the task of filtering a noisy speech signal, e.g. speech corrupted by the ambient acoustics. In the recent past we have developed a handful of methods to address this task in challenging scenarios (e.g. high reverberation or very low signal-to-noise ratios).
We first proposed an architecture based on LSTMs to perform spectral-noise estimation 81 and speech enhancement 80. The idea of the latter is to map speech signals into the spectral domain using the short-time Fourier transform (STFT) and hence to represent audio signals in a time-frequency space. The input of the proposed LSTM-based narrow-band filter is a noisy signal while the target used for network training is a noise-free signal. This discriminative deep filter formulation yields excellent results when applied to speech enhancement. Since the filter processes the STFT input frequency-wise (hence the name narrow band) it is generalizable to other types of temporal data. For example we can use this same concept to process human gestures and facial expressions over time.
In order to capture and exploit the uncertainty, we also exploited variational auto-encoders (VAEs) 62 which are feed-forward encoder-decoder latent variable networks, that have recently gained an immense popularity. We developed a VAE-based speech enhancement method which learns a speech model. At test time, this pre-trained speech model is combined with a nonnegative matrix factorization (NMF) noise model whose parameters are estimated from an observed noise-corrupted speech signal 69, 70. This formulation has two distinctive features: (i) there is no need to learn in the presence of various noise types, since the VAE network learns a clean-speech model, and (ii) pairs of noisy- and clean-speech signals are not necessary for training, as it is the case with discriminative approaches. Currently the use of NMF techniques limits the representation power of the noise signal. More powerful models, such as DVAEs could also be used within the same general-purpose formulation.
We have also started to investigate the extension of unimodal (audio) VAE-based speech enhancement method to multimodal (audio-visual) speech enhancement. It is well established that audio and visual data convey complementary information for the processing of speech. In particular the two modalities are affected by completely different sources of noise. Indeed, audio-speech is contaminated by additive noise due to the presence of other audio sources, while visual-speech is contaminated by occlusions and by head movements. Currently, audio-visual processing methods assume clean visual information and it is absolutely not clear how to deal with noisy visual data in the framework of speech processing.
Along this line of research, we have proposed an audio-visual VAE that is trained using synchronized audio-speech and visual-speech data, thus yielding an audio-visual prior model for speech. At test time, the approach follows the same idea as in the case of audio speech enhancement: NMF for audio-noise estimation and speech reconstruction 93. Very recently we have started to develop the concept of mixture of variational auto-encoders (MVAE) which is an attempt to put the two modalities on an equal footing 89, 90, as well as their temporal extension 91. The central idea is to consider an audio encoder and a visual encoder that are jointly trained with a shared decoder. The general architecture of proposed MVAE formulation is shown on Figure 1. As is the case with VAEs, this leads to an intractable posterior distribution and we resort to variational inference to devise a tractable solver.
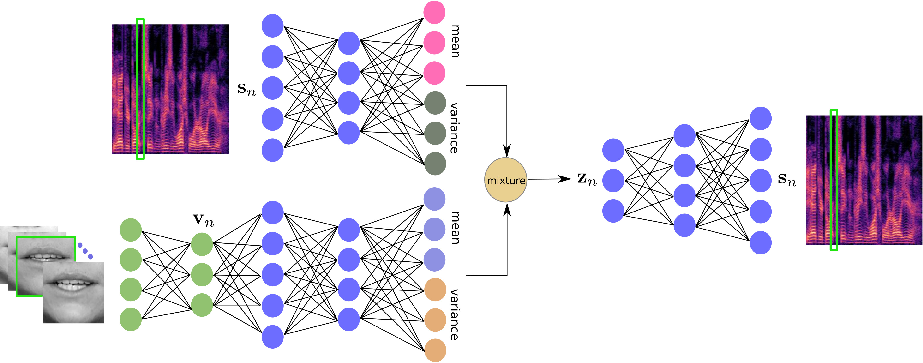
The proposed mixture VAE architecture for learning a speech generative model using audio and visual information (from ).
The proposed mixture VAE architecture for learning a speech generative model using audio and visual information (from 89).
Research directions
We will investigate the following topics on deep probabilistic models:
-
Discriminative deep filters. We plan to build on our recent work on discriminative deep filtering for speech enhancement 80, in order to address challenging problems associated with the temporal modeling and data fusion for robot perception and action. In particular we plan to devise novel algorithms that enable the robotic platform to, for instance, robustly track the visual focus of attention, or appropriately react to its changes. Such tasks require end-to-end learning, from the detection of facial and body landmarks to the prediction of their trajectories and activity recognition. In particular, we will address the task of characterizing temporal patterns of behavior in flexible settings, e.g. users not facing the camera. For example, lip reading for speech enhancement and speech recognition must be performed in unconstrained settings, e.g. in the presence of rigid head motions or when the user's face is partially occluded. Discriminative deep filters will also be investigated, within the framework of reinforcement learning, to devise optimal action policies exploiting sequential multi-modal data.
- Generative deep recurrent neural networks. Most of the VAE-based methods in the literature are tailored to use uni-modal data. VAE models for multimodal data are merely available and we are among the first to propose an audio-visual VAE model for speech enhancement 93. Nevertheless, the proposed framework treats the two modalities unevenly. We started to investigate the use of mixture models in an attempt to put the two modalities on an equal footing 89, 90, 91. However, this is a long term endeavor since it raises many difficult questions from both theoretical and algorithmic points of view. Indeed, while the concept of noisy speech is well formalized in the audio signal processing domain, it is not understood in the computer vision domain. We plan to thoroughly address the combination of generative deep networks with robust mixture modeling, using for instance heavy-tailed Student-t distributions, and coping with the added complexity by means of variational approximations. Eventually, we will consider combinations of VAEs with sequential models such as for instance RNNs, and with attention-based architectures such as transformers 51. Ideally, we will work towards devising generic methodologies spaning a wide variety of temporal models. As already mentioned, we started to investigate this problem in the framework of our work on speech enhancement 68, which may be viewed either as a recurrent VAE or, more generally, as a non-linear DNN-based formulation of SSMs. We will apply this kind of deep generative/recurrent architectures to other problems that are encountered in audio-visual perception and we will propose case-by-case tractable and efficient solvers.
3.2 Human behavior understanding
Interactions between a robot and a group of people require human behavior understanding (HBU) methods. Consider for example the tasks of detecting eye-gaze and head-gaze and of tracking the gaze directions associated with a group of participants. This means that, in addition to gaze detection and gaze tracking, it is important to detect persons and to track them as well. Additionally, it is important to extract segments of speech, to associate these segments with persons and hence to be able to determine over time who looks to whom and who is the speaker and who are the listeners. The temporal and spatial fusion of visual and audio cues stands at the basis of understanding social roles and of building a multimodal conversational model.
Performing HBU tasks in complex, cluttered and noisy environments is challenging for several reasons: participants come in an out of the camera field of view, their photometric features, e.g. facial texture, clothing, orientation with respect to the camera, etc., vary drastically, even over short periods of time, people look at an object of interest (a person entering the room, a speaking person, a TV/computer screen, a wall painting, etc.) by turning their heads away from the camera, hence facial image analysis is difficult, small head movements are often associated with speech which perturbs both lip reading and head-gaze tracking, etc. Clearly, understanding multi-person human-robot interaction is complex because the person-to-person and person-to-object, in addition to person-to-robot, interactions must explicitly be taken into account.
We propose to perform audio-visual HBU by taking explicitly into account the complementary nature of these two modalities. Differently from one current trend in AV learning 30, 42, 54, we opt for unsupervised probabilitic methods that can (i) assign observations to persons without supervision, (ii) be combined with various probabilistic noise models and (iii) and fuse various cues depending on their availability in time (i.e. handle missing data). Indeed, in face-to-face communication, the robot must choose with who it should engage dialog, e.g. based on proximity, eye gaze, head movements, lip movements, facial expressions, etc., in addition to speech. Unlike in the single-user human-robot interaction case, it is crucial to associate temporal segments of speech to participants, referred to as speech diarization. Under such scenarios, speech signals are perturbed by noise, reverberation and competing audio sources, hence speech localization and speech enhancement methods must be used in conjunction with speech recognition. The relationship with natural language understanding and spoken dialog, while very relevant, falls outside the team's expertise. This relationship will be investigated in collaboration with the Interaction Lab at Heriot-Watt University (lead by Prof. Oliver Lemon), a partner of H2020 SPRING project and with the Laboratoire d'Intelligence Artificielle at Université d'Avignon (professor Fabrice Lefèvre), partner of ANR Dialbot project.
As already explained (see Section 3.1) we have recently investigated various aspects of dynamic HBU, namely multiple-person tracking based on visual 35, audio 72, 37, or audio-visual information 15, head-pose estimation 49, eye-gaze tracking 83, e.g. Fig. 2, and audio-visual diarization 56. Our recent work has relied on Gaussian mixture regression 45, on dynamic Bayesian networks 85 and on their variational approximations, e.g. 15. Such probabilistic and statistical formulations provide robust, powerful and flexible unsupervised learning techniques for HBU. In parallel, there has been strong interest in using deep learning techniques for HBU, e.g. person detection, person tracking, facial expression recognition, etc. Nevertheless, deep neural networks still have difficulties in capturing motion information directly from image sequences. For example, human activity detection, tracking and recognition use pre-computed optical flow to compute motion information. Most of the work has focused on HBU at a single person level and less effort has been devoted into developing deep learning methods for studying group activities and behavior, in particular in the context of interaction.
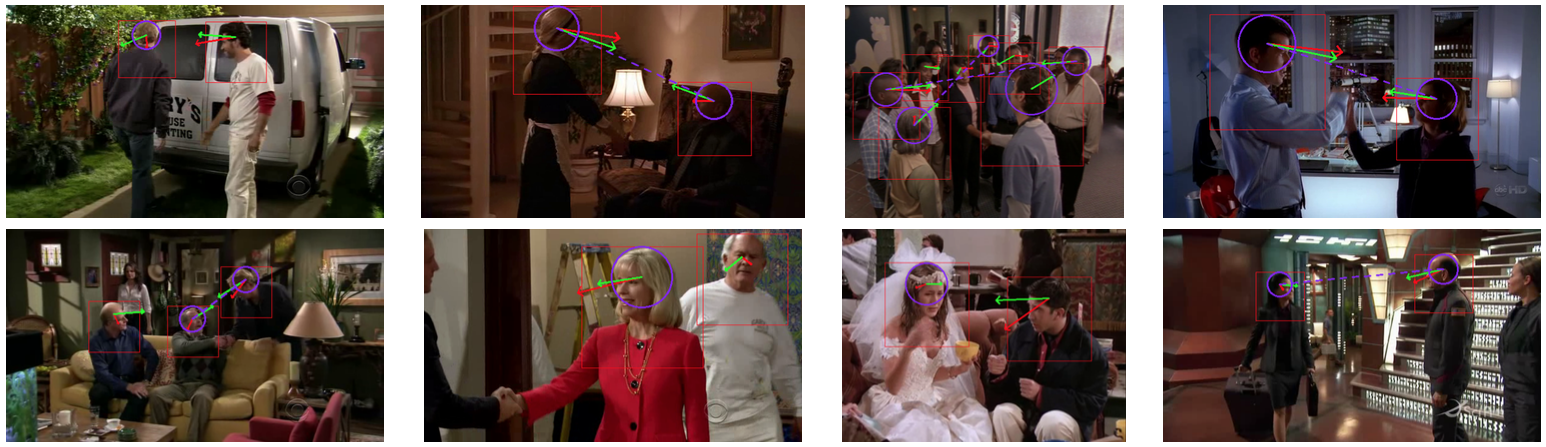
This figure shows some examples of eye-gaze detection and tracking obtained with the method proposed in . The algorithm infers both eye-gaze (green arrows) and visual focus of attention (blue circles) from head-gaze (red arrows). A side-effect of this inference is the detection of people looking at each other (dashed blue line).
This figure shows some examples of eye-gaze detection and tracking obtained with the method proposed in 83. The algorithm infers both eye-gaze (green arrows) and visual focus of attention (blue circles) from head-gaze 49 (red arrows). A side-effect of this inference is the detection of people looking at each other (dashed blue line).
A comprehensive analysis of groups of people should rely on combining Bayesian modeling with deep neural networks. Indeed, this enables us to sum up the flexibility of the former with the representative and discriminative power of the latter. We plan to combine deep generative networks (see Section 3.1) trained for person tracking with person descriptors based on deep discriminative learning. Fully generative strategies will also be investigated, possibly exploiting features pre-trained in discriminative settings, thus exploiting large-scale annotated datasets available for certain tasks. Indeed, current state of the art provides DNN architectures well suited for learning embeddings of images and of image primitives. However, these embeddings are learned off-line using very large training datasets to guarantee data variability and generality. It is however necessary to perform some kind of adaptation to the distribution of the particular data at hand, e.g. collected with robot sensors. If these data are available in advance, off-line adaptation can be done, otherwise the adaptation needs to be performed on-line or at run time. Such strategies will be useful given the particular experimental conditions of practical human-robot interaction scenarios.
On-line learning based on deep neural networks is far from being well understood. We plan to thoroughly study the incorporation of on-line learning into both Bayesian and discriminative deep networks. In the practical case of interaction, real-time processing is crucial. Therefore, a compromise must be found between the size of the network, its discriminative power and the computational cost of the learning and prediction algorithms. Clearly, there is no single solution given the large variety of problems and scenarios that are encountered in practice.
Exemplar application: multi-person facial landmark tracking
The problem of facial landmark tracking of mulitple persons can be formulated as a two-stage problem, namely, first we track each face, and second we extract facial landmarks from each tracked face, e.g. 92, and this in a robust manner. We recently proposed solutions to perform on-line multi-person tracking and started to explore how to robustly extract landmarks.
We proposed an on-line method to concurrently track a variable number of people and update the appearance model of each person 47, in order to make it more robust to changes in illumination, pose, etc. Such appearance models must yield extremely discriminative descriptors, such that two observed faces are unambiguously assigned to two different persons being tracked. This means that observation-to-person assignments, computed during the tracking itself, must be used to fine tune the (possibly deep) representation. This fine tuning needs to be carefully investigated and properly incorporated into the probabilistic tracker. Indeed, if enough data is available, the network could be fine-tuned, as in 18. Otherwise, the representation could be updated without fine-tuning the network 47.
From these tracks, one could attempt to analyse facial expressions based on, e.g. facial landmarks. Even if several facial landmark extraction algorithms exist in the literature, how to properly separate rigid head motions (such as turning the head or simply moving) from non-rigid face movements, i.e. expressions, within the current models is unclear. Indeed, the analysis of facial expressions is a difficult task by its own, even if rigid head movements have been subtracted out. We started investigating how to assess the quality of the extracted landmarks and we plan to use these assessments to design and train architectures implementing SSMs for robust facial landmark extraction and tracking. Such architectures could be easily used for other tasks such as human gesture recognition, robust body landmark estimation, facial expression recognition or speech activity estimation.
More generally, these examples are instances of the problem of on-line discriminative learning. Generally, discriminative learning uses deterministic targets/labels for learning, such as the ones produced by manual annotation of large amounts of data. In the on-line case we do not have the luxury of manual annotation. We must therefore rely on less reliable labels. In other words, we must compute and maintain over time a measure of label reliability.
Consider again the problem of tracking persons over time and let be the number of observations at each time step. Let a latent discrete variable denote the association between an observation and a person. At each time step , each observation is assigned to each person with probability . Therefore, one can replace deterministic labels with their probability distribution function. Case-by-case analysis must be carefully carried out in order to choose the proper network and learning strategy.
Research directions
Our research plan on human behavior understanding is summarized as follows:
-
Deep visual descriptors. One of the most important ingredients of HBU is to learn visual representations of humans using deep discriminative networks. This process comprises detecting people and body parts in images and then extracting 2D or 3D landmarks. We plan to combine body landmark detectors and facial landmark detectors, based on feedforward architectures, with landmark tracking based on recurrent neural networks. The advantage is twofold: to eliminate noise, outliers and artefacts, which are inherent to any imaging process, and to build spatio-temporal representations for higher-level processes such as action and gesture recognition. While the task of noise filtering can be carried out using existing techniques, the task of removing outliers and artefacts is more difficult. Based on our recent work on robust deep regression, we plan to develop robust deep learning methods to extract body and facial landmarks. In addition to the Gaussian-uniform mixture used in 67, we plan to investigate the Student t-distribution and its variants as it has interesting statistical properties, such as robustness due to their so-called heavy tail. Moreover, we plan to combine deep learning methods with robust rigid registration methods in order to distinguish between rigid and non-rigid motion and to separate them. This research will combine robust probability distributions with deep learning and hence will lead to novel algorithms for robustly detecting landmarks and tracking them over time. Simultaneously, we will address the problem of assessing the quality of the landmarks without systematic recourse to annotated datasets.
- Deep audio descriptors. We will also investigate methods for extracting descriptors from audio signals. These descriptors must be free of noise and reverberation. While there are many noise filtering and dereverberation methods available, they are not necessarily well adapted to the tasks involved in live interaction between a robot and a group of people. In particular, they often treat the case of a static acoustic scene: both the sources and the microphones remain fixed. This represents a strong limitation and the existing methods must be extended to deal with dynamic acoustic scenes, e.g. 63. Based on our recent work 73, we plan to develop deep audio descriptors that are robust against noise and reverberation. We will train these descriptors to help the tasks of speech enhancement and speech dereverberation in order to facilitate down-stream tasks such as speech-source localization and speech recognition. Moreover, we plan to develop a speaker recognition method that can operate in a complex acoustic environment. As done in computer vision for person re-identification 53, recent works adapt the embedding network to an unknown domain. Adversarial strategies to further increase the performance have also been proposed 60, and we have contributed for person re-identification 18. How to exploit these strategies with a continuous flow of observations acquired by a robotic platform remains to be investigated.
3.3 Learning and control for social robots
Traditionally, research on human-robot interaction focused on single-person scenarios also called dyadic interactions. However, over the past decade several studies were devoted to various aspects of multi-party interactions, meaning situations in which a robot interacts with a group of two or more people 94. This line of research is much more challenging because of two main reasons. First, the behavioral cues of each individual and of the group need to be faithfully extracted (and assigned to each individual). Second, the behavioral dynamics of groups of people can be pushed by the presence of the robot towards competition 41 or even bullying 40. This is why some studies restrict the experimental conditions to very controlled collaborative scenarios, often lead by the robot, such as quiz-like game playing 98 or very specific robot roles 52. Intuitively, constraining the scenario also reduces the gesture variabilty and the overall interaction dynamics, leading to methods and algorithms with questionable generalisation to free and natural social multi-party interactions.
Whenever a robot participates in such multi-party interactions, it must perform social actions. Such robot social actions are typically associated with the need to perceive a person or a group of persons in an optimal way as well as to take appropriate decisions such as to safely move towards a selected group, to pop into a conversation or to answer a question. Therefore, one can distinguish between two types of robot social actions: (i) physical actions which correspond to synthesizing appropriate motions using the robot actuators (motors), possibly within a sensorimotor loop, so as to enhance perception and maintain a natural interaction and (ii) spoken actions which correspond to synthesizing appropriate speech utterances by a spoken dialog system. In RobotLearn we will focus on the former, and integrate the latter via collaborations with research groups having with established expertise in speech technologies.
For example, robust speech communication requires clean speech signals. Nevertheless, clean speech could be retrieved by the robot in several ways and based on different strategies. The first strategy is that the robot stays still and performs audio signal processing in order to reconstruct clean speech signals from noisy ones, e.g. in the presence of reverberation and of competing audio sources. The second strategy consists of moving towards a speaking person in order to face her/him directly and to optimize the quality of the audio signals gathered with the onboard microphones. Therefore, apparently simple speech communication tasks between a robot and a person involve a complex analysis in order to take appropriate decisions: Is the room noisy? Are there many people in the robot's field of view? How far are they? Are they looking at the robot? Is speech enhancement sufficient, or should the robot move towards a person in order to reduce the effects of room reverberation and of ambient noise? Clearly, robot perception and robot action are intimately interleaved, and the robot actions should be selected on the premise that social behavior counts.
In this regard we face three problems. First, given the complexity of the environment and the inherent limitations of the robot's perception capabilities, e.g. limited camera field of view, cluttered spaces, complex acoustic conditions, etc., the robot will only have access to a partial representation of the environment, and up to a certain degree of accuracy. Second, for learning purposes, there is no easy way to annotate which are the best actions the robot must choose given a situation: supervised methods are therefore not an option. Third, since the robot cannot learn from scratch by random exploration in a new environment, standard model-free RL approaches cannot be used. Some sort of previous knowledge on the environment or a similar one should be exploited. Finally, given that the robot moves within a populated environment, it is desirable to have the capability to enforce certain constrains, thus limiting the range of possible robot actions.
Building algorithms to endow robots with autonomous decision taking is not straightforward. Two relatively distinct paradigms are available the literature. First, one can devise customized strategies based on techniques such as robot motion planning combined with sensor-based robot control. These techniques lack generalization, in particular when the robot acts in complex, dynamic and unconstrained environments. Second, one can let the robot devise its own strategies based on reinforcement learning (RL) – a machine learning paradigm in which “agents" learn by themselves by trial and error to achieve successful strategies 95. It is very difficult, however, to enforce any kind of soft- or hard-constraint within this framework. We will showcase these two scientific streams with one group of techniques for each one: model predictive control (MPC) and Q-learning, deep Q-networks (DQNs), more precisely. These two techniques are promising. Moreover, they are well documented in the robotics and machine learning. Nevertheless, combining them is extremely challenging.
MPC is a generic framework which allows the incorporation of constraints in the process of robot decision-taking. More formally MPC requires (i) a transition function , i.e. generalization of (1), (ii) a correction function and (iii) an optional constraint function . The MPC problem is formally stated as an optimisation problem 64:
where is the time horizon considered in the optimisation problem.
Often, one can devise efficient solvers to find the optimal control sequence . As discussed before, the advantage of MPC is the possibility to include constrains, modeled through . Such constraints can be used to enforce safety or other must-comply rules, the scenario at hand may require. Even if it is technically possible to learn the transition function , this has high computational cost. Therefore, one limitation of MPC is the common assumption that the transition function is completely known. In purely geometric tasks, this makes sense, since one can have a fairly accurate model of how the perception of the objects present in the evolves with the robot actions. However, it is much more complex to model how the behavior of people (from their body pose to their high-level global behavior) will change due to the robot actions. One may then rather learn the transition function.
Alternatively, an appealing framework for learning robot behavior is DQN. 84 As any RL method, DQN is based on rewards, evaluated at each time step and after taking an action at state , . The aim is to learn the optimal action policy, i.e. the one that maximises the expected accumulated reward: , where is a discount factor. To do so, DQN uses the so-called function, which is defined for a certain action policy at a state-action pair, as the expected accumulated reward when following policy :
where the expectation is taken over the future state distribution, using , therefore the latter becomes a stochastic mapping rather than a deterministic one, and the action distribution, using . Implicitly, this means that the function models jointly the effect of the transition function and of the policy action . Thus, once is learned, the effects of and cannot be disentangled.
It can be shown that the optimal Q function satisfies the following Bellman equation:
In DQN, the Q function is approximated by a deep neural network, which is learned by stochastic gradient descent based on the Bellman equation. While DQN has been successfully applied to various control problems, in particular computer games, it suffers from various drawbacks. First, DQN exhibits high performance when the set of actions is discrete, as opposed to continuous actions much more suitable in robotics. Second, and this is common to the majority of RL approaches, DQN requires lots of trajectories (sequences of state-action pairs) for training. These are usually obtained through computer simulations, raising a question that remains widely open: how to efficiently simulate social interactions that follow a data distribution that the agent will face in the real world? Third, by design, RL (and hence DQN) cannot be trained in the presence of constraints. Certainly, one can discourage certain robot behaviors by designing large negative rewards of some state-action pairs, but this does not guarantee that the robot will never execute such state-action pairs.
Summarizing, on the one hand we have sensor-based robot control techniques, such as MPC, that require a faithful representation of the transition function so as to compute the optimal action trajectory, and do not allow learning. On the other hand we have learning-based techniques that allow to learn the transition function (together with the optimal policy function), but they cannot be coupled with hard-constraints. Our scenario is complex enough to require learning (part of) the transition function, and at the same time we would like to enforce constraints when controling the robot.
Exemplar application: audio-visual robot gaze control
Recently, we applied DQN to the problem of controlling the gaze of a robotic head using audio and visual information 65, 66. In summary, the robot learns by itself how to turn its head towards a group of speaking faces. The DQN-like architecture is based on a long short-time memory (LSTM) network that takes as input a sequence of states (namely motor positions, person detection and sound-source detection and localization) and which predicts a -value for each possible action (stay still, look up, look down, look left and look right).
In order to speed up training in real time, we proposed to simulate the pose of people in the scene using standard pose-estimation datasets that contain ground-truth pose. We combined the poses of different people thanks to a set of hand-crafted rules. Additionally we emulated the output of a sound localisation algorithm that would provide the direction of the most prominent active sources. The reward given to the agent would be the number of faces found in the field of view, plus an extra reward if the speaking face was within the field of view. In this way the robot learned actions that maximise the number of people within the field of view. In addition, the robot satisfactorily learned to look at a speaking person when found that person belonged to a group of people, and to look around (explore) when none of the participants were within the field of view.
While this application may seem very simple, one must understand that simulating such data in a realistic manner is not straightforward. In addition, lots of simulations were required before fine-tuning the DQN with real-world data: the pre-training phase was very intense for such a simple task. Thus, scaling up such a simulation to more complex scenarios, e.g. where one has to take into account conversational and group dynamics, remains an open question. Other strategies allowing better generalization, such as meta RL, would be highly desirable.
Research directions
-
Constrained RL. Naturally one may be tempted to combine MPC and DQN, but this is unfortunately not possible. Indeed, DQN cannot disentangle the policy from the environment , and MPC requires an explicit expression for to solve the associated optimisation problem, their direct combination is not possible. We will investigate two directions. First, to devise methodologies able to efficiently learn the transition function , to later on use it within the MPC framework. Second, to design learning methodologies that are combined with MPC, so that the actions taken within the learning process satisfy the required constraints. A few combinations of RL and MPC for robot navigation in human-free scenarios 86, 61, as well as MPC variants driven by datahave recently appeared in the literature. How to adapt this recent trend to dynamic complex environments such as a multi-party conversational situation is still to be investigated. Additionally, the use of audio-visual fusion in this context needs to be explored deeply, and this also holds for the second research line.
- Meta RL. An additional challenge, independent from the learning and control combination foreseen, is the data distribution gap between the simulations and the real-world. Meta-learning, or the ability to learn how to learn, can provide partial answers to this problem. Indeed, developing machine learning methods able to understand how the learning is achieved can be used to extend this learning to a new task and speed up the learning process on the new task. Recent developments proposed meta-learning strategies specifically conceived for reinforcement learning, leading to Meta-RL methods. One promising trend in Meta-RL is to have a probabilistic formulation involving SSMs and VAEs, i.e. hence sharing the methodology based on dynamical variational autoencoders described before. Very importantly, we are not aware of any studies able to combine Meta-RL with MPC to handle the constraints, and within a unified formulation. From a methodological perspective, this is an important challenge we face in the next few years.
4 Application domains
For the last decades, there has been an increasing interest in robots that cooperate and communicate with people. As already mentioned, we are interested Socially Assistive Robots (SARs) that can communicate with people and that are perceived as social entities.
So far, the humanoid robots developed to fill this role are mainly used as research platforms for human-robot collaboration and interaction and their prices, if at all commercially available, are in the 6-digit-euro category, e.g. 250,000
When interacting, these robots rely on a few selected modalities. The voice interface of this category of robots, e.g. Kompaï, NAO, and Pepper, is based on speech recognition similar to speech technologies used by smart phones and table-top devices, e.g. Google Home. Their audio hardware architecture and software packages are designed to handle single-user face-to-face spoken dialogue based on keyword spotting, but they can neither perform multiple sound-source analysis, fuse audio and visual information for more advanced multi-modal/multi-party interactions, nor hold a conversation that exceeds a couple of turns and that is out of very narrow predefined domain.
To the best of our knowledge, the only notable efforts to overcome some of the limitations mentioned above are the FP7 EARS and H2020 MuMMER projects. The EARS project's aim was to redesign the microphone-array architecture of the commercially available humanoid robot NAO, and to build a robot head prototype that can support software based on advanced multi-channel audio signal processing. The EARS partners were able to successfully demonstrate the usefulness of this microphone array for speech-signal noise reduction, dereverberation, and multiple-speaker localisation. Moreover, the recent IEEE-AASP Challenge on Acoustic Source Localisation and Tracking (LOCATA) comprises a dataset that uses this microphone array. The design of NAO imposed severe constraints on the physical integration of the microphones and associated hardware. Consequently and in spite of the scientific and practical promises of this design, SoftBank Robotics has not integrated this technology into their commercially available robots NAO and Pepper. In order to overcome problems arising from human-robot interaction in unconstrained environments and open-domain dialogue on the Pepper robot, the H2020 MuMMER project aimed to deploy an entertaining and helpful robot assistant to a shopping mall. While they had initial success with short deployments of the robot to the mall, they were not specifically addressing the issues arising from multi-party interaction: Pepper's audio hardware/software design cannot locate and separate several simultaneously emitting speech sources.
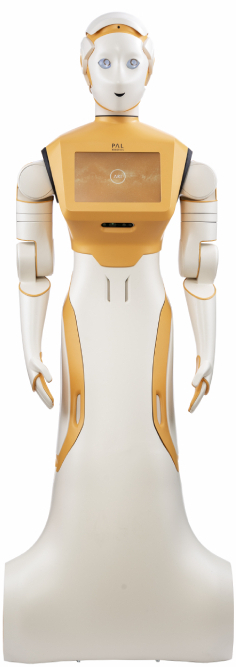
To conclude, current robotic platforms available in the consumer market, i.e. with large-scale deployment potential, are neither equipped with the adequate hardware nor endowed with the appropriate software required for multi-party social interactions in real-world environments.
In the light of the above discussion, the partners of the H2020 SPRING project decided to build a robot prototype well suited for socially assistive tasks and shared by the SPRING partners as well as by other EU projects. We participated to the specifications of the ARI robot prototype (shown on the right), designed, developed and manufactured by PAL Robotics, an industrial partner of the SPRING project. ARI is a ROS-enabled, non-holonomic, differential-drive wheeled robot, equipped with a pan and tilt head, with both color and depth cameras and with a microphone array that embeds the latest audio signal processing technologies. Seven ARI robot units were delivered to the SPRING partners in April 2021.
We are committed to implement our algorithms and associated software packages onto this advanced robotic platform, from low-level control to high-level perception, interaction and planning tasks, such that the robot has a socially-aware behaviour while it safely navigates in an ever changing environment. We will experiment in environments of increasing complexity, e.g. our robotic lab, the Amiqual4Home facility, the Inria Grenoble cafeteria and Login exhibition, as well as the Broca hospital in Paris. The expertise that the team's engineers and researchers have acquired for the last decade would be crucial for present and future robotic developments and experiments.
5 Highlights of the year
Over the past year, we have many scientific contributions that we would like to quickly summarise. More details will be provided later on.
We have developed a transformer-based architecture for multiple object tracking is now under review at TPAMI Beyond tracking, we have also contributed to multi-person body pose estimation, see our WACV paper on the topic. In this line, we have collected, curated and exploited the Extreme Pose Interaction (ExPI) dataset, where we investigate the prediction of human motion in complex actions such as aerial/acrobatic dancing steps.
We have also worked towards exploiting facial landmarks to frontalise the face, i.e. remove rigid movements, while keeping the lip movements and use them for visual speech recognition (see the associated ICCV-W publication). This is naturally related to our previous work on robust 3D face alignment. Naturally related, our contributions on speech enhancement/separation include the switching VAE for AV speech enhancement (ICASSP 2021), and the mixture VAE for speech enhancement (TSP) and for separation (MLSP 2021). These models merge the VAE methodology with other probabilistic models, including some sort of temporal dependency. We published an extensive review of models including the temporal dependency within the deep generative model, or Dynamical Variational Autoencoders, in Foundations and Trends on Machine Learning and Interspeech’21. Going back to the use of facial landmarks, we have investigated how to learn to generate inter-action sequences (submitted to TAFFC).
This past year we have also investigated how to learn robot action policies in various contexts. First, by developeding a navigation module based on the model predictive control (MPC) methodology. This is now working on ARI. We have worked on meta/transfer reinforcement learning (RL), generalising successor features to non-linear reward functions or xi-learning. We have also contributed to the use of neural episodic control in combination with linear successor features (presented at NeurIPS-W).
6 New software and platforms
6.1 New software
6.1.1 TransCenter
-
Name:
TransCenter: Transformers with Dense Queries for Multiple-Object Tracking
-
Keywords:
Python, Multi-Object Tracking, Deep learning, Computer vision
-
Scientific Description:
Transformer networks have proven extremely powerful for a wide variety of tasks since they were introduced. Computer vision is not an exception, as the use of transformers has become very popular in the vision community in recent years. Despite this wave, multiple-object tracking (MOT) exhibits for now some sort of incompatibility with transformers. We argue that the standard representation — bounding boxes with insufficient sparse queries — is not optimal to learning transformers for MOT. Inspired by recent research, we propose TransCenter, the first transformer-based MOT architecture for dense heatmap predictions. Methodologically, we propose the use of dense pixel-level multi-scale queries in a transformer dual-decoder network, to be able to globally and robustly infer the heatmap of targets’ centers and associate them through time. TransCenter outperforms the current state-of-the-art in standard benchmarks both in MOT17 [2] and MOT20 [1]. Our ablation study demonstrates the advantage in the proposed architecture compared to more naive alternatives.
-
Functional Description:
TransCenter is a software for multiple-object tracking using deep neural networks. It allows tracking multiple people in a very crowded scenes.
- URL:
- Publication:
-
Contact:
Soraya Arias
-
Participants:
Yihong Xu, Guillaume Delorme, Xavier Alameda Pineda, Daniela Rus, Yutong Ban, Chuang Gan
6.1.2 xi_learning
-
Name:
Xi Learning
-
Keywords:
Reinforcement learning, Transfer Learning
-
Functional Description:
Transfer in Reinforcement Learning aims to improve learning performance on target tasks using knowledge from experienced source tasks. Successor features (SF) are a prominent transfer mechanism in domains where the reward function changes between tasks. They reevaluate the expected return of previously learned policies in a new target task and to transfer their knowledge. A limiting factor of the SF framework is its assumption that rewards linearly decompose into successor features and a reward weight vector. We propose a novel SF mechanism, ξ-learning, based on learning the cumulative discounted probability of successor features. Crucially, ξ-learning allows to reevaluate the expected return of policies for general reward functions. We introduce two ξ-learning variations, prove its convergence, and provide a guarantee on its transfer performance. Experimental evaluations based on ξ-learning with function approximation demonstrate the prominent advantage of ξ-learning over available mechanisms not only for general reward functions, but also in the case of linearly decomposable reward functions.
- URL:
-
Authors:
Chris Reinke, Xavier Alameda Pineda
-
Contact:
Chris Reinke
6.1.3 Social MPC
-
Keyword:
Robotics
-
Functional Description:
A library for controlling a social robot. This library allows a non-holonomic robot to navigate in a crowded environment using model predictive control and social force models. This library has been developed for the SPRING project that has received funding from the European Union’s Horizon 2020 research and innovation programme under grant agreement No 871245.
The main components of this library are: - A module to determine optimal positioning of a robot in a group, using methods from the litterature. - A navigation component to compute optimal paths - The main module, implementing a model predictive controller using the Jax library to determine optimal commands to steer the robot
-
Authors:
Alex Auternaud, Timothee Wintz, Chris Reinke
-
Contact:
Alex Auternaud
6.1.4 2D Social Simulator
-
Keywords:
Simulator, Robotics
-
Functional Description:
A python based simulator using Box2D allowing a robot to interact with people. This software enables: - The configuration of a scene with physical obstacles and people populating a room - The simulation of the motion of a robot in this space - Social force models for the behaviour of people, groups between themselves and in reaction to the motion of the robot
Rendering is done using PyGame and is optional (headless mode is possible).
A gym environment is provided for reinforcement learning.
- URL:
-
Authors:
Alex Auternaud, Timothee Wintz, Chris Reinke
-
Contact:
Alex Auternaud
6.1.5 PI-NET
-
Name:
Pose Interacting Network for Multi-Person Monocular 3D Pose Estimation
-
Keywords:
Pytorch, Pose estimation, Deep learning, -
-
Scientific Description:
Monocular 3D multi-person human pose estimation aims at estimating the 3D joints of several people from a single RGB image. PI-Net, inputs the initial pose estimates of a variable number of interactees into a recurrent architecture used to refine the pose of the person-of-interest. We demonstrate the effectiveness of our method in the MuPoTS dataset, setting the new state-of-the-art on it. Qualitative results on other multi-person datasets (for which 3D pose ground-truth is not available) showcase the proposed PI-Net. PI-Net is implemented in PyTorch.
-
Functional Description:
Monocular 3D multi-person human pose estimation aims at estimating the 3D joints of several people from a single RGB image. PI-Net, inputs the initial pose estimates of a variable number of interactees into a recurrent architecture used to refine the pose of the person-of-interest. We demonstrate the effectiveness of our method in the MuPoTS dataset, setting the new state-of-the-art on it. Qualitative results on other multi-person datasets (for which 3D pose ground-truth is not available) showcase the proposed PI-Net. PI-Net is implemented in PyTorch.
- URL:
- Publication:
-
Contact:
Xavier Alameda Pineda
-
Participants:
Wen Guo, Xavier Alameda Pineda
6.1.6 dvae-speech
-
Name:
dynamic variational auto-encoder for speech re-synthesis
-
Keywords:
Variational Autoencoder, Deep learning, Pytorch, Speech Synthesis
-
Functional Description:
It can be considered a library for speech community, to use different dynamic VAE models for speech re-synthesis (potentially for other speech application)
- URL:
- Publication:
-
Authors:
Xiaoyu Bie, Xavier Alameda Pineda, Laurent Girin
-
Contact:
Xavier Alameda Pineda
6.2 New platforms
Participants: Alex Auternaud, Timothée Wintz, Chris Reinke, Luis Camara, Nicolas Turro, Soraya Arias, Radu Horaud, Xavier Alameda-Pineda.
This year we have received the ARI robot (see Figure 3) from PAL Robotics, in the framework of the H2020 SPRING project. ARI is a high-performance robotic platform designed for a wide range of multimodal expressive gestures and behaviors, making it the ideal social robot and suitable for Human-Robot-Interaction. We have customised the platrofms to the needs of the H2020 SPRING project, adding microphones and cameras to adapt its sensing capabilities to the needs of the project. Since a few months now, we are operating ARI and obtaining the first results with it.7 New results
7.1 Transformed-based multiple object tracking
Participants: Yihong Xu, Radu Horaud, Xavier Alameda-Pineda.
Transformer networks have proven extremely powerful for a wide variety of tasks since they were introduced. Computer vision is not an exception, as the use of transformers has become very popular in the vision community in recent years. Despite this wave, multiple-object tracking (MOT) exhibits for now some sort of incompatibility with transformers. We argue that the standard representation - bounding boxes with insufficient sparse queries - is not optimal to learning transformers for MOT. Inspired by recent research, we propose TransCenter, the first transformer-based MOT architecture for dense heatmap predictions. Methodologically, we propose the use of dense pixel-level multi-scale queries in a transformer dual-decoder network, to be able to globally and robustly infer the heatmap of targets' centers and associate them through time. TransCenter outperforms the current state-of-the-art in standard benchmarks both in MOT17 and MOT20. Our ablation study demonstrates the advantage in the proposed architecture compared to more naive alternatives. See 6.1.1.7.2 Multiperson body pose estimation in interactive environments
Participants: Wen Guo, Xavier Alameda-Pineda.
Recent literature addressed the monocular 3D pose estimation task very satisfactorily. In these studies, different persons are usually treated as independent pose instances to estimate. However, in many every-day situations, people are interacting, and the pose of an individual depends on the pose of his/her interactees. In this work, we investigate how to exploit this dependency to enhance current - and possibly future - deep networks for 3D monocular pose estimation. Our pose interacting network, or PI-Net, inputs the initial pose estimates of a variable number of interactees into a recurrent architecture used to refine the pose of the person-of-interest. Evaluating such a method is challenging due to the limited availability of public annotated multi-person 3D human pose datasets. We demonstrate the effectiveness of our method in the MuPoTS dataset, setting the new state-of-the-art on it. Qualitative results on other multi-person datasets (for which 3D pose ground-truth is not available) showcase the proposed PI-Net.7.3 Extreme Pose Interaction (ExPI) Dataset
Participants: Wen Guo, Xavier Alameda-Pineda.
Human motion prediction aims to forecast future poses given a sequence of past 3D skeletons. While this problem has recently received increasing attention, it has mostly been tackled for single humans in isolation. In this work, we explore this problem when dealing with humans performing collaborative tasks, we seek to predict the future motion of two interacted persons given two sequences of their past skeletons. We propose a novel cross interaction attention mechanism that exploits historical information of both persons, and learns to predict cross dependencies between the two pose sequences. Since no dataset to train such interactive situations is available, we collected ExPI (Extreme Pose Interaction), a new lab-based person interaction dataset of professional dancers performing Lindy-hop dancing actions, which contains 115 sequences with 30K frames annotated with 3D body poses and shapes. We thoroughly evaluate our cross interaction network on ExPI and show that both in short- and long-term predictions, it consistently outperforms state-of-the-art methods for single-person motion prediction. See the dedicated webpage.7.4 Robust Face Frontalization For Visual Speech Recognition
Participants: Zhiqi Kang, Mostafa Sadeghi, Radu Horaud, Xavier Alameda-Pineda.
Face frontalization consists of synthesizing a frontally-viewed face from an arbitrarily-viewed one. The main contribution is a robust method that preserves non-rigid facial deformations, i.e. expressions. The method iteratively estimates the rigid transformation and the non-rigid deformation between 3D landmarks extracted from an arbitrarily-viewed face, and 3D vertices parameterized by a deformable shape model. The one merit of the method is its ability to deal with large Gaussian and non-Gaussian errors in the data. For that purpose, we use the generalized Student-t distribution. The associated EM algorithm assigns a weight to each observed landmark, the higher the weight the more important the landmark, thus favouring landmarks that are only affected by rigid head movements. We propose to use the zero-mean normalized cross-correlation score to evaluate the ability to preserve facial expressions. We show that the method, when incorporated into a deep lip-reading pipeline, considerably improves the word classification score on an in-the-wild benchmark. See the decicated webpage.7.5 Switching Variational Autoencoders
Participants: Mostafa Sadeghi, Xavier Alameda-Pineda.
Recently, audio-visual speech enhancement has been tackled in the unsupervised settings based on variational auto-encoders (VAEs), where during training only clean data is used to train a generative model for speech, which at test time is combined with a noise model, e.g. nonnegative matrix factorization (NMF), whose parameters are learned without supervision. Consequently, the proposed model is agnostic to the noise type. When visual data is clean, audio-visual VAE-based architectures usually outperform the audio-only counterpart. The opposite happens when the visual data is corrupted by clutter, e.g. the speaker not facing the camera. In this work, we propose to find the optimal combination of these two architectures through time. More precisely, we introduce the use of a latent sequential variable with Markovian dependencies to switch between different VAE architectures through time in an unsupervised manner: leading to switching variational auto-encoder (SwVAE). We propose a variational factorization to approximate the computationally intractable posterior distribution. We also derive the corresponding variational expectation-maximization algorithm to estimate the parameters of the model and enhance the speech signal. Our experiments exhibit the performance of SwVAE.7.6 Mixture of Inference Networks for VAE-based Audio-visual Speech Enhancement
Participants: Mostafa Sadeghi, Xavier Alameda-Pineda.
In this work, we are interested in unsupervised (unknown noise) speech enhancement, where the probability distribution of clean speech spectrogram is simulated via a latent variable generative model, also called the decoder. Recently, variational autoencoders (VAEs) have gained much popularity as probabilistic generative models. In VAEs, the posterior of the latent variables is computationally intractable, and it is approximated by a so-called encoder network. Motivated by the fact that visual data, i.e. lip images of the speaker, provide helpful and complementary information about speech, some audio-visual architectures have been recently proposed. The initialization of the latent variables at test time is crucial as the overall inference problem is non-convex. This is usually done by using the output of the encoder where the noisy audio and clean video data are given as input. Current audio-visual models do not provide an effective initialization because the two modalities are tightly coupled (concatenated) in the associated architectures. To overcome this issue, we inspire from mixture models, and introduce the mixture of inference networks variational autoencoder (MIN-VAE). Two encoder networks input, respectively, audio and visual data, and the posterior of the latent variables is modeled as a mixture of two Gaussian distributions output from each encoder network. The mixture variable is also latent, and therefore the inference of learning the optimal balance between the audio and visual inference network is unsupervised as well. By training a shared decoder, the overall network learns to adaptively fuse the two modalities. Moreover, at test time, the video encoder, which takes (clean) visual data, is used for initialization. A variational inference approach is derived to train the proposed generative model. Thanks to the novel inference procedure and the robust initialization, the proposed audio-visual VAE exhibits superior performance on speech enhancement than using the standard audio-only as well as audio-visual counterparts.7.7 Deep Variational Generative Models for Audio-visual Speech Separation
Participants: Mostafa Sadeghi, Xavier Alameda-Pineda.
In this work, we are interested in audio-visual speech separation given a single-channel audio recording as well as visual information (lips movements) associated with each speaker. We propose an unsupervised technique based on audio-visual generative modeling of clean speech. More specifically, during training, a latent variable generative model is learned from clean speech spectrograms using a variational auto-encoder (VAE). To better utilize the visual information, the posteriors of the latent variables are inferred from mixed speech (instead of clean speech) as well as the visual data. The visual modality also serves as a prior for latent variables, through a visual network. At test time, the learned generative model (both for speaker-independent and speaker-dependent scenarios) is combined with an unsupervised non-negative matrix factorization (NMF) variance model for background noise. All the latent variables and noise parameters are then estimated by a Monte Carlo expectation-maximization algorithm. Our experiments show that the proposed unsupervised VAE-based method yields better separation performance than NMF-based approaches as well as a supervised deep learning-based technique.7.8 Dynamical Variational Autoencoders
Participants: Xiaoyu Bie, Laurent Girin, Xavier Alameda-Pineda.
In this work, we are interested in audio-visual speech separation given a single-channel audio recording as well as visual information (lips movements) associated with each speaker. We propose an unsupervised technique based on audio-visual generative modeling of clean speech. More specifically, during training, a latent variable generative model is learned from clean speech spectrograms using a variational auto-encoder (VAE). To better utilize the visual information, the posteriors of the latent variables are inferred from mixed speech (instead of clean speech) as well as the visual data. The visual modality also serves as a prior for latent variables, through a visual network. At test time, the learned generative model (both for speaker-independent and speaker-dependent scenarios) is combined with an unsupervised non-negative matrix factorization (NMF) variance model for background noise. All the latent variables and noise parameters are then estimated by a Monte Carlo expectation-maximization algorithm. Our experiments show that the proposed unsupervised VAE-based method yields better separation performance than NMF-based approaches as well as a supervised deep learning-based technique.7.9 A Benchmark of Dynamical Variational Autoencoders applied to Speech Spectrogram Modeling
Participants: Xiaoyu Bie, Laurent Girin, Xavier Alameda-Pineda.
The Variational Autoencoder (VAE) is a powerful deep generative model that is now extensively used to represent high-dimensional complex data via a low-dimensional latent space learned in an unsupervised manner. In the original VAE model, input data vectors are processed independently. In recent years, a series of papers have presented different extensions of the VAE to process sequential data, that not only model the latent space, but also model the temporal dependencies within a sequence of data vectors and corresponding latent vectors, relying on recurrent neural networks. We recently performed a comprehensive review of those models and unified them into a general class called Dynamical Variational Autoencoders (DVAEs). In the present work, we present the results of an experimental benchmark comparing six of those DVAE models on the speech analysis-resynthesis task, as an illustration of the high potential of DVAEs for speech modeling.7.10 Variational Inference and Learning of Piecewise-linear Dynamical Systems
Participants: Xavier Alameda-Pineda, Radu Horaud.
Modeling the temporal behavior of data is of primordial importance in many scientific and engineering fields. Baseline methods assume that both the dynamic and observation equations follow linear-Gaussian models. However, there are many real-world processes that cannot be characterized by a single linear behavior. Alternatively, it is possible to consider a piecewise-linear model which, combined with a switching mechanism, is well suited when several modes of behavior are needed. Nevertheless, switching dynamical systems are intractable because their computational complexity increases exponentially with time. In this work, we propose a variational approximation of piecewise linear dynamical systems. We provide full details of the derivation of two variational expectation-maximization algorithms, a filter and a smoother. We show that the model parameters can be split into two sets, static and dynamic parameters, and that the former parameters can be estimated off-line together with the number of linear modes, or the number of states of the switching variable. We apply the proposed method to the head-pose tracking, and we thoroughly compare our algorithms with several state of the art trackers.7.11 SocialInteractionGAN: Multi-person Interaction Sequence Generation
Participants: Louis Airale, Dominique Vaufreydaz, Xavier Alameda-Pineda.
Prediction of human actions in social interactions has important applications in the design of social robots or artificial avatars. In this work, we model human interaction generation as a discrete multi-sequence generation problem and present SocialInteractionGAN, a novel adversarial architecture for conditional interaction generation. Our model builds on a recurrent encoder-decoder generator network and a dual-stream discriminator. This architecture allows the discriminator to jointly assess the realism of interactions and that of individual action sequences. Within each stream a recurrent network operating on short subsequences endows the output signal with local assessments, better guiding the forthcoming generation. Crucially, contextual information on interacting participants is shared among agents and reinjected in both the generation and the discriminator evaluation processes. We show that the proposed SocialInteractionGAN succeeds in producing high realism action sequences of interacting people, comparing favorably to a diversity of recurrent and convolutional discriminator baselines. Evaluations are conducted using modified Inception Score and Fréchet Inception Distance metrics, that we specifically design for discrete sequential generated data. The distribution of generated sequences is shown to approach closely that of real data. In particular our model properly learns the dynamics of interaction sequences, while exploiting the full range of actions.7.12 -Learning: Successor Feature Transfer Learning for General Reward Functions
Participants: Chris Reinke, Xavier Alameda-Pineda.
Transfer in Reinforcement Learning aims to improve learning performance on target tasks using knowledge from experienced source tasks. Successor features (SF) are a prominent transfer mechanism in domains where the reward function changes between tasks. They reevaluate the expected return of previously learned policies in a new target task and to transfer their knowledge. A limiting factor of the SF framework is its assumption that rewards linearly decompose into successor features and a reward weight vector. We propose a novel SF mechanism, ξ-learning, based on learning the cumulative discounted probability of successor features. Crucially, ξ-learning allows to reevaluate the expected return of policies for general reward functions. We introduce two ξ-learning variations, prove its convergence, and provide a guarantee on its transfer performance. Experimental evaluations based on ξ-learning with function approximation demonstrate the prominent advantage of ξ-learning over available mechanisms not only for general reward functions but also in the case of linearly decomposable reward functions.7.13 Successor Feature Neural Episodic Control
Participants: David Emukpere, Xavier Alameda-Pineda, Chris Reinke.
A longstanding goal in reinforcement learning is to build intelligent agents that show fast learning and a flexible transfer of skills akin to humans and animals. We investigate the integration of two frameworks for tackling those goals: episodic control and successor features. Episodic control is a cognitively inspired approach relying on episodic memory, an instance-based memory model of an agent’s experiences. Meanwhile, successor features and generalized policy improvement (SF&GPI) is a meta and transfer learning framework allowing to learn policies for tasks that can be efficiently reused for later tasks which have a different reward function. Individually, these two techniques have shown impressive results in vastly improving sample efficiency and the elegant reuse of previously learned policies. Thus, we outline a combination of both approaches in a single reinforcement learning framework and empirically illustrate its benefits.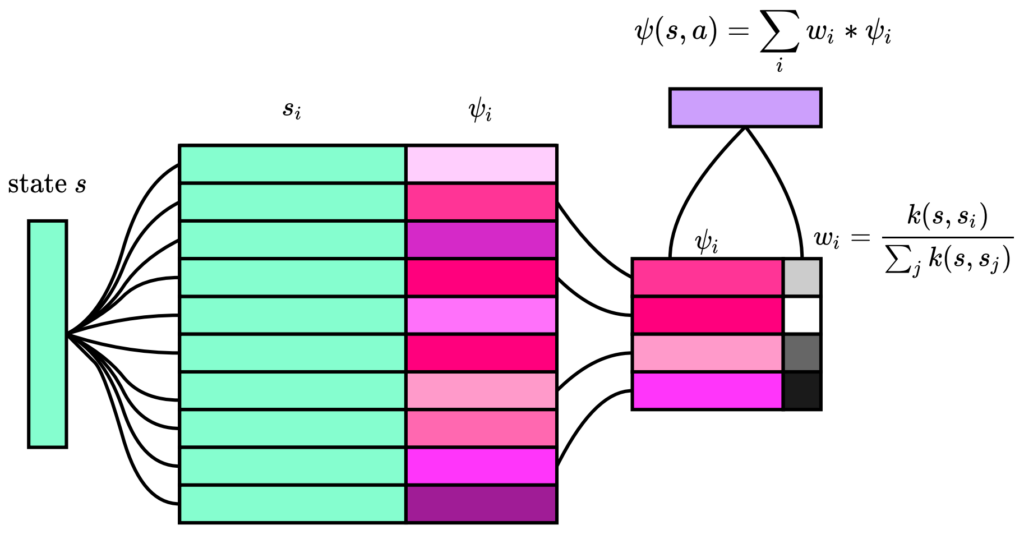
8 Bilateral contracts and grants with industry
8.1 Bilateral Grants with Industry
8.1.1 VASP
Participants: Zhiqi Kang, Mostafa Sadeghi, Xavier Alameda-Pineda, Radu Horaud.
-
Title:
Visually-assisted speech processing
-
Duration:
1 October 2020 - 30 September 2021
-
Principal investigator:
Radu Horaud
-
Partner:
Facebook Reality Labs Research, Redmond WA, USA
-
Summary:
We investigate audio-visual speech processing. In particular we plan to go beyond the current paradigm that systematically combines a noisy speech signal with clean lip images and which delivers a clean speech signal. The rationale of this paradigm is based on the fact that lip images are free of any type of noise. This hypothesis is merely verified in practice. Indeed, speech production is often accompanied by head motions that considerably modify the patterns of the observed lip movements. As a consequence, currently available audio-visual speech processing technologies are not usable in practice. In this project we develop a methodology that separates non-rigid face- and lip movements from rigid head movements, and we build a deep generative architecture that combines audio and visual features based on their relative merits, rather than making systematic recourse to their concatenation. It is also planned to record and annotate an audio-visual dataset that contains realistic face-to-face and multiparty conversations. The core methodology is based on robust mixture modeling and on variational auto-encoders.
9 Partnerships and cooperations
9.1 European initiatives
9.1.1 H2020 Project SPRING
Participants: Alex Auternaud, Timothée Wintz, Chris Reinke, Luis Camara, Gaetan Lepage, Nicolas Turro, Soraya Arias, Radu Horaud, Xavier Alameda-Pineda.
Started on Januray 1st, 2020 and finalising on May 31st, 2024, SPRING is a research and innovation action (RIA) with eight partners: Inria Grenoble (coordinator), Università degli Studi di Trento, Czech Technical University Prague, Heriot-Watt University Edinburgh, Bar-Ilan University Tel Aviv, ERM Automatismes Industriels Carpentras, PAL Robotics Barcelona, and Hôpital Broca Paris. The main objective of SPRING (Socially Pertinent Robots in Gerontological Healthcare) is the development of socially assistive robots with the capacity of performing multimodal multiple-person interaction and open-domain dialogue. In more detail:
- The scientific objective of SPRING is to develop a novel paradigm and novel concept of socially-aware robots, and to conceive innovative methods and algorithms for computer vision, audio processing, sensor-based control, and spoken dialog systems based on modern statistical- and deep-learning to ground the required social robot skills.
- The technological objective of SPRING is to create and launch a brand new generation of robots that are flexible enough to adapt to the needs of the users, and not the other way around.
- The experimental objective of SPRING is twofold: to validate the technology based on HRI experiments in a gerontology hospital, and to assess its acceptability by patients and medical staff.
9.2 National initiatives
9.2.1 ANR JCJC Project ML3RI
Participants: Chris Reinke, Xiaoyu Lin, Radu Horaud, Xavier Alameda-Pineda.
Starting on March 1st 2020 and finalising on February 28th 2024, ML3RI is an ANR JCJC that has been awarded to Xavier Alameda-Pineda. Multi-person robot interactionin the wild (i.e. unconstrained and using only the robot's resources) is nowadays unachievable because of the lack of suitable machine perception and decision-taking models. Multi-Modal Multi-person Low-Level Learning models for Robot Interaction (ML3RI) has the ambition to develop the capacity to understand and react to low-level behavioral cues, which is crucial for autonomous robot communication. The main scientific impact of ML3RI is to develop new learning methods and algorithms, thus opening the door to study multi-party conversations with robots. In addition, the project supports open and reproducible research.
9.2.2 ANR MIAI Chair
Participants: Xiaoyu Bie, Anand Ballou, Radu Horaud, Xavier Alameda-Pineda.
The overall goal of the MIAI chair “Audio-visual machine perception & interaction for robots” it to enable socially-aware robot behavior for interactions with humans. Emphasis on unsupervised and weakly supervised learning with audio-visual data, Bayesian inference, deep learning, and reinforcement learning. Challenging proof-of-concept demonstrators. We aim to develop robots that explore populated spaces, understand human behavior, engage multimodal dialog with several users, etc. These tasks require audio and visual cues (e.g. clean speech signals, eye-gaze, head-gaze, facial expressions, lip movements, head movements, hand and body gestures) to be robustly retrieved from the raw sensor data. These features cannot be reliably extracted with a static robot that listens, looks and communicates with people from a distance, because of acoustic reverberation and noise, overlapping audio sources, bad lighting, limited image resolution, narrow camera field of view, visual occlusions, etc. We will investigate audio and visual perception and communication, e.g. face-to-face dialog: the robot should learn how to collect clean data (e.g. frontal faces, signals with high speech-to-noise ratios) and how to react appropriately to human verbal and non-verbal solicitations. We plan to demonstrate these skills with a companion robot that assists and entertains the elderly in healthcare facilities.
Website: Website
10 Dissemination
Participants: Radu Horaud, Xavier Alameda-Pineda.
10.1 Promoting scientific activities
10.1.1 Scientific events: organisation
Area Chair Xavier Alameda-Pineda was Area Chair for IEEE/CVF WACV 2021, ACM Multimedia 2021, and AAAI 2022.
10.1.2 Scientific events: selection
Reviewer Xavier Alameda-Pineda was a reviewer for IEEE/CVF CVPR 2022 and for IEEE ICASSP 2022.
10.1.3 Journal
Member of the editorial boards During 2021, Xavier Alameda-Pineda was Associated Editor of three top-tier journals: Computer Vision and Image Understanding, ACM Transactions on Mutimedia Tools and Applications and IEEE Transactions on Multimedia.
10.1.4 Invited talks
During 2021, the members of the team were invited to give a series of talks:
- Deep generative modeling of sequential data with dynamical variational autoencoders (Jun'21) at IEEE ICASSP 2021.
- Unsupervised Learning for Human Robot Perception (Jun'21) at Robotics and AI Summer School 2021.
- Towards socially intelligent robots: preliminary results of the H2020 SPRING and the ANR ML3RI projects (Jun'21) at PI Stories University of Trento
- Unsupervised Audio-Visual Fusion for Upstream Human Behavior Understanding (May'21) at AI4Media Workshop on New Learning Paradigms and Distributed AI4Media
- Variational Autoencoders for Audio, Visual and Audio-Visual Learning (Feb'21) at DaSCI Webinars
- Speaker localisation and enhancement in populated environments – invited talk (Jan'21) at ICPR 2020 Workshop on Deep Learning for Human-Centric Activity Understanding
- Combining auditory and visual data to enhance the speech signal – invited talk (Jan'21) at ICPR 2020 Workshop on Multimodal pattern recognition for social signal processing in human computer interaction
10.1.5 Leadership within the scientific community
Since 2021, Xavier Alameda-Pineda is the vice-chair of the 9th Technical Committee of the International Association for Pattern Recognition with title “pattern recognition in human machine interaction.”
10.2 Teaching - Supervision - Juries
10.2.1 Teaching
In 2021, Xavier Alameda-Pineda was involved in teaching two coursers at Masters 2 level:
- Fundamentals of Probabilistic Data Mining - at Master of Science in Industrial and Applied Mathematics
- Machine Learning for Computer Vision and Audio Processing - at Master of Science in Informatics at Grenoble
10.2.2 Supervision (defences)
PhD defence: Guillaume Delorme, Adaptation de domaine non supervisée pour modèle de suivi multi-partie et identification visuelle appliquée à l'interaction homme-robot, defended on October 8th, 2021. Directors: Radu Horaud and Xavier Alameda-Pineda.
MSc defence: David Emukpere, Successor Feature Neural Episodic Control, defended on June 22nd, 2021. Directors: Xavier Alameda-Pineda and Chris Reinke.
10.2.3 Juries
In 2021, Xavier Alameda-Pineda participated to the following PhD committees as examiner:
- Julien Audibert (U. Sorbonne)
- Maria Kabtoul (University Grenoble-Alpes)
and to the following ones as a reviewer:
- Manuel Pariente (U. Lorraine)
- Marco Godi (U. Verona)
11 Scientific production
11.1 Major publications
- 1 articleTracking Multiple Audio Sources with the Von Mises Distribution and Variational EM.IEEE Signal Processing Letters266June 2019, 798 - 802
- 2 articleJoint Alignment of Multiple Point Sets with Batch and Incremental Expectation-Maximization.IEEE Transactions on Pattern Analysis and Machine Intelligence406June 2018, 1397 - 1410
- 3 articleAudio-Visual Speaker Diarization Based on Spatiotemporal Bayesian Fusion.IEEE Transactions on Pattern Analysis and Machine Intelligence405July 2018, 1086 - 1099
- 4 articleNeural Network Based Reinforcement Learning for Audio-Visual Gaze Control in Human-Robot Interaction.Pattern Recognition Letters118February 2019, 61-71
- 5 articleA Comprehensive Analysis of Deep Regression.IEEE Transactions on Pattern Analysis and Machine Intelligence429September 2020, 2065-2081
- 6 articleOnline Localization and Tracking of Multiple Moving Speakers in Reverberant Environments.IEEE Journal of Selected Topics in Signal Processing131March 2019, 88-103
- 7 articleMultichannel Identification and Nonnegative Equalization for Dereverberation and Noise Reduction based on Convolutive Transfer Function.IEEE/ACM Transactions on Audio, Speech and Language Processing2610May 2018, 1755-1768
- 8 articleMultichannel Speech Separation and Enhancement Using the Convolutive Transfer Function.IEEE/ACM Transactions on Audio, Speech and Language Processing273March 2019, 645-659
- 9 articleAudio-noise Power Spectral Density Estimation Using Long Short-term Memory.IEEE Signal Processing Letters266June 2019, 918-922
- 10 articleTracking Gaze and Visual Focus of Attention of People Involved in Social Interaction.IEEE Transactions on Pattern Analysis and Machine Intelligence4011November 2018, 2711 - 2724
- 11 articleAudio-Visual Speech Enhancement Using Conditional Variational Auto-Encoders.IEEE/ACM Transactions on Audio, Speech and Language Processing28May 2020, 1788-1800
- 12 articleIncreasing Image Memorability with Neural Style Transfer.ACM Transactions on Multimedia Computing, Communications and Applications152June 2019
- 13 articleProbabilistic Graph Attention Network with Conditional Kernels for Pixel-Wise Prediction.IEEE Transactions on Pattern Analysis and Machine IntelligenceDecember 2020
11.2 Publications of the year
International journals
International peer-reviewed conferences
Reports & preprints
11.3 Cited publications
- 29 articleScoping review on the use of socially assistive robot technology in elderly care.BMJ open822018, e018815
- 30 inproceedingsSelf-supervised learning of audio-visual objects from video.Computer Vision--ECCV 2020: 16th European Conference, Glasgow, UK, August 23--28, 2020, Proceedings, Part XVIII 16Springer2020, 208--224
- 31 inproceedingsAudio-Visual Variational Fusion for Multi-Person Tracking with Robots.ACMMM 2019 - 27th ACM International Conference on MultimediaNice, FranceACM PressOctober 2019, 1059-1061
- 32 articleA Geometric Approach to Sound Source Localization from Time-Delay Estimates.IEEE Transactions on Audio, Speech and Language Processing226June 2014, 1082-1095
- 33 articleVision-Guided Robot Hearing.The International Journal of Robotics Research344-5April 2015, 437-456
- 34 articleA tutorial on particle filters for online nonlinear/non-Gaussian Bayesian tracking.IEEE Transactions on signal processing5022002, 174--188
- 35 articleAn On-line Variational Bayesian Model for Multi-Person Tracking from Cluttered Scenes.Computer Vision and Image Understanding153December 2016, 64--76
- 36 inproceedingsTracking a Varying Number of People with a Visually-Controlled Robotic Head.IEEE/RSJ International Conference on Intelligent Robots and SystemsVancouver, CanadaIEEESeptember 2017, 4144-4151
- 37 articleTracking Multiple Audio Sources with the Von Mises Distribution and Variational EM.IEEE Signal Processing Letters266June 2019, 798 - 802
- 38 articleUsing computer agents to explain medical documents to patients with low health literacy.Patient education and counseling7532009, 315--320
- 39 articleAssistive social robots in elderly care: a review.Gerontechnology822009, 94--103
- 40 inproceedingsEscaping from children's abuse of social robots.Proceedings of the tenth annual acm/ieee international conference on human-robot interaction2015, 59--66
- 41 inproceedingsThe effect of group size on people's attitudes and cooperative behaviors toward robots in interactive gameplay.RO-MAN International Symposium on Robot and Human Interactive CommunicationIEEE2012, 845--850
- 42 inproceedingsSoundspaces: Audio-visual navigation in 3d environments.Computer Vision--ECCV 2020: 16th European Conference, Glasgow, UK, August 23--28, 2020, Proceedings, Part VI 16Springer2020, 17--36
- 43 articleHyper-Spectral Image Analysis with Partially-Latent Regression and Spatial Markov Dependencies.IEEE Journal of Selected Topics in Signal Processing96September 2015, 1037-1048
- 44 articleAcoustic Space Learning for Sound-Source Separation and Localization on Binaural Manifolds.International Journal of Neural Systems251February 2015, 21p
- 45 articleHigh-Dimensional Regression with Gaussian Mixtures and Partially-Latent Response Variables.Statistics and Computing255September 2015, 893-911
- 46 articleCo-Localization of Audio Sources in Images Using Binaural Features and Locally-Linear Regression.IEEE Transactions on Audio, Speech and Language Processing234April 2015, 718-731
- 47 inproceedingsODANet: Online Deep Appearance Network for Identity-Consistent Multi-Person Tracking.ICPR 2021 - 25th International Conference on Pattern Recognition / WorkshopsMilano / Virtual, ItalyJanuary 2021
- 48 inproceedingsHead Pose Estimation via Probabilistic High-Dimensional Regression.IEEE International Conference on Image Processing, ICIP 2015Proceedings of the IEEE International Conference on Image ProcessingQuebec City, QC, CanadaIEEESeptember 2015, 4624-4628
- 49 articleRobust Head-Pose Estimation Based on Partially-Latent Mixture of Linear Regressions.IEEE Transactions on Image Processing263March 2017, 1428 - 1440
- 50 articleJoint Alignment of Multiple Point Sets with Batch and Incremental Expectation-Maximization.IEEE Transactions on Pattern Analysis and Machine Intelligence406June 2018, 1397 - 1410
- 51 articleTransformer-based Conditional Variational Autoencoder for Controllable Story Generation.arXiv preprint arXiv:2101.008282021
- 52 articleAutomatically classifying user engagement for dynamic multi-party human--robot interaction.International Journal of Social Robotics952017, 659--674
- 53 inproceedingsSelf-similarity grouping: A simple unsupervised cross domain adaptation approach for person re-identification.Proceedings of the IEEE/CVF International Conference on Computer Vision2019, 6112--6121
- 54 inproceedingsVisualvoice: Audio-visual speech separation with cross-modal consistency.IEEE/CVF CVPR2021
- 55 articleEM Algorithms for Weighted-Data Clustering with Application to Audio-Visual Scene Analysis.IEEE Transactions on Pattern Analysis and Machine Intelligence3812December 2016, 2402 - 2415
- 56 articleAudio-Visual Speaker Diarization Based on Spatiotemporal Bayesian Fusion.IEEE Transactions on Pattern Analysis and Machine Intelligence405July 2018, 1086 - 1099
- 57 articleExtending the Cascaded Gaussian Mixture Regression Framework for Cross-Speaker Acoustic-Articulatory Mapping.IEEE/ACM Transactions on Audio, Speech and Language Processing253March 2017, 662-673
- 58 articleRobotic assistance in the coordination of patient care.The International Journal of Robotics Research37102018, 1300--1316
- 59 inproceedingsBackprop kf: Learning discriminative deterministic state estimators.Advances in neural information processing systems2016, 4376--4384
- 60 articleAugmentation adversarial training for self-supervised speaker recognition.arXiv preprint arXiv:2007.120852020
- 61 inproceedingsPractical Reinforcement Learning For MPC: Learning from sparse objectives in under an hour on a real robot.Learning for Dynamics and ControlPMLR2020, 211--224
- 62 articleAuto-encoding variational bayes.arXiv preprint arXiv:1312.61142013
- 63 articleA Variational EM Algorithm for the Separation of Time-Varying Convolutive Audio Mixtures.IEEE/ACM Transactions on Audio, Speech and Language Processing248August 2016, 1408-1423
- 64 inproceedingsModel predictive control for tilt recovery of an omnidirectional wheeled humanoid robot.International conference on robotics and automation (ICRA)IEEE2015, 5134--5139
- 65 inproceedingsDeep Reinforcement Learning for Audio-Visual Gaze Control.IROS 2018 - IEEE/RSJ International Conference on Intelligent Robots and SystemsMadrid, SpainIEEEOctober 2018, 1555-1562
- 66 articleNeural Network Based Reinforcement Learning for Audio-Visual Gaze Control in Human-Robot Interaction.Pattern Recognition Letters118February 2019, 61-71
- 67 inproceedingsDeepGUM: Learning Deep Robust Regression with a Gaussian-Uniform Mixture Model.ECCV 2018 - European Conference on Computer Vision11209Lecture Notes in Computer ScienceMunich, GermanySpringerSeptember 2018, 205-221
- 68 inproceedingsA Recurrent Variational Autoencoder for Speech Enhancement.IEEE International Conference on Acoustics, Speech and Signal ProcessingIEEEBarcelone, SpainMay 2020, 1-7
- 69 inproceedingsA variance modeling framework based on variational autoencoders for speech enhancement.EEE International Workshop on Machine Learning for Signal Processing (MLSP)Aalborg, DenmarkIEEESeptember 2018, 1-6
- 70 inproceedingsSemi-supervised multichannel speech enhancement with variational autoencoders and non-negative matrix factorization.ICASSP 2019 - IEEE International Conference on Acoustics, Speech and Signal ProcessingBrighton, United KingdomIEEEMay 2019, 101-105
- 71 inproceedingsA Cascaded Multiple-Speaker Localization and Tracking System.Proceedings of the LOCATA Challenge Workshop - a satellite event of IWAENC 2018Tokyo, JapanSeptember 2018, 1-5
- 72 articleOnline Localization and Tracking of Multiple Moving Speakers in Reverberant Environments.IEEE Journal of Selected Topics in Signal Processing131March 2019, 88-103
- 73 articleMultichannel Identification and Nonnegative Equalization for Dereverberation and Noise Reduction based on Convolutive Transfer Function.IEEE/ACM Transactions on Audio, Speech and Language Processing2610May 2018, 1755-1768
- 74 inproceedingsReverberant Sound Localization with a Robot Head Based on Direct-Path Relative Transfer Function.IEEE/RSJ International Conference on Intelligent Robots and SystemsIEEEDaejeon, South KoreaIEEEOctober 2016, 2819-2826
- 75 articleMultichannel Online Dereverberation based on Spectral Magnitude Inverse Filtering.IEEE/ACM Transactions on Audio, Speech and Language Processing279May 2019, 1365-1377
- 76 articleMultichannel Speech Separation and Enhancement Using the Convolutive Transfer Function.IEEE/ACM Transactions on Audio, Speech and Language Processing273March 2019, 645-659
- 77 articleExpectation-Maximization for Speech Source Separation using Convolutive Transfer Function.CAAI Transactions on Intelligent Technologies41March 2019, 47 - 53
- 78 articleEstimation of the Direct-Path Relative Transfer Function for Supervised Sound-Source Localization.IEEE/ACM Transactions on Audio, Speech and Language Processing2411November 2016, 2171 - 2186
- 79 articleMultiple-Speaker Localization Based on Direct-Path Features and Likelihood Maximization with Spatial Sparsity Regularization.IEEE/ACM Transactions on Audio, Speech and Language Processing251016 pages, 4 figures, 4 tablesOctober 2017, 1997 - 2012
- 80 unpublishedNarrow-band Deep Filtering for Multichannel Speech Enhancement.September 2020, working paper or preprint
- 81 articleAudio-noise Power Spectral Density Estimation Using Long Short-term Memory.IEEE Signal Processing Letters266June 2019, 918-922
- 82 articlePeople respond better to robots than computer tablets delivering healthcare instructions.Computers in Human Behavior432015, 112--117
- 83 articleTracking Gaze and Visual Focus of Attention of People Involved in Social Interaction.IEEE Transactions on Pattern Analysis and Machine Intelligence4011November 2018, 2711 - 2724
- 84 articleHuman-level control through deep reinforcement learning.nature51875402015, 529--533
- 85 bookMachine learning: a probabilistic perspective.MIT press2012
- 86 inproceedingsNeural network dynamics for model-based deep reinforcement learning with model-free fine-tuning.IEEE International Conference on Robotics and Automation (ICRA)IEEE2018, 7559--7566
- 87 article“Are we ready for robots that care for us?” Attitudes and opinions of older adults toward socially assistive robots.Frontiers in aging neuroscience72015, 141
- 88 articleLearning nonlinear dynamical systems using the expectation-maximization algorithm.Kalman filtering and neural networks62001, 175--220
- 89 articleMixture of Inference Networks for VAE-based Audio-visual Speech Enhancement.IEEE Transactions on Signal ProcessingMarch 2021
- 90 inproceedingsRobust Unsupervised Audio-visual Speech Enhancement Using a Mixture of Variational Autoencoders.IEEE International Conference on Acoustics, Speech and Signal Processing (ICASSP)Barcelona, SpainMay 2020
- 91 inproceedingsSwitching Variational Auto-Encoders for Noise-Agnostic Audio-visual Speech Enhancement.IEEE International Conference on Acoustics, Speech and Signal ProcessingToronto, CanadaIEEEJune 2021, 1-5
- 92 unpublishedUnsupervised Performance Analysis of 3D Face Alignment.October 2020, Submitted to Computer Vision and Image Understanding
- 93 articleAudio-Visual Speech Enhancement Using Conditional Variational Auto-Encoders.IEEE/ACM Transactions on Audio, Speech and Language Processing28May 2020, 1788-1800
- 94 articleRobots in groups and teams: a literature review.Proceedings of the ACM on Human-Computer Interaction4CSCW22020, 1--36
- 95 bookReinforcement learning: An introduction.MIT press2018
- 96 articleThe attitudes and perceptions of older adults with mild cognitive impairment toward an assistive robot.Journal of Applied Gerontology3512016, 3--17
- 97 articleAcceptance of an assistive robot in older adults: a mixed-method study of human--robot interaction over a 1-month period in the Living Lab setting.Clinical interventions in aging92014, 801
- 98 articleMulti-party turn-taking in repeated human--robot interactions: an interdisciplinary evaluation.International Journal of Social Robotics1152019, 693--707
- 99 articleVr-goggles for robots: Real-to-sim domain adaptation for visual control.IEEE Robotics and Automation Letters422019, 1148--1155