Keywords
Computer Science and Digital Science
- A3.4. Machine learning and statistics
- A3.4.1. Supervised learning
- A3.4.2. Unsupervised learning
- A3.4.4. Optimization and learning
- A3.4.6. Neural networks
- A3.4.7. Kernel methods
- A3.4.8. Deep learning
- A5.3. Image processing and analysis
- A5.3.2. Sparse modeling and image representation
- A5.3.3. Pattern recognition
- A5.3.4. Registration
- A5.4.3. Content retrieval
- A5.4.4. 3D and spatio-temporal reconstruction
- A5.4.5. Object tracking and motion analysis
- A5.4.6. Object localization
- A5.9. Signal processing
- A5.9.3. Reconstruction, enhancement
- A5.9.5. Sparsity-aware processing
- A5.9.6. Optimization tools
- A6.1. Methods in mathematical modeling
- A6.1.1. Continuous Modeling (PDE, ODE)
- A6.1.2. Stochastic Modeling
- A6.3.1. Inverse problems
Other Research Topics and Application Domains
- B1.1. Biology
- B1.1.3. Developmental biology
- B2.6. Biological and medical imaging
1 Team members, visitors, external collaborators
Research Scientists
- Xavier Descombes [Team leader, INRIA, Senior Researcher, HDR]
- Florence Besse [CNRS, Senior Researcher]
- Laure Blanc Feraud [CNRS, Senior Researcher, HDR]
- Luca Calatroni [CNRS, Researcher]
- Eric Debreuve [CNRS, Researcher, HDR]
- Grégoire Malandain [INRIA, Senior Researcher, HDR]
- Caroline Medioni [CNRS, Researcher]
PhD Students
- Asma Chalabi [UNIV COTE D'AZUR]
- Cedric Dubois [UNIV COTE D'AZUR]
- Faisal Jayousi [CNRS, from Oct 2022]
- Bastien Laville [UNIV COTE D'AZUR]
- Marta Lazzaretti [UNIV GENES et UNIV COTE D'AZUR, Co-tutelle]
- Imane Lboukili [JOHNSON]
- Alexandre Martin [INRIA]
- Clara Sanchez [UNIV COTE D'AZUR]
- Vasiliki Stergiopoulou [CNRS]
- Rudan Xiao [UNIV COTE D'AZUR, until Sep 2022]
Technical Staff
- Sébastien Schaub [CNRS, Engineer]
Interns and Apprentices
- Apolline Blachet [INRIA, Intern, from Jun 2022 until Jul 2022]
- Assia Chahidi [Université Côte d'Azur, Intern, from Mar 2022 until Aug 2022]
- Esther Jammes [INRIA, Intern, from Jun 2022 until Aug 2022]
- Fabio Merizzi [Université Côte d'Azur, Intern, from Apr 2022 until Aug 2022]
- Mohamad Mohamad [INRIA, from Sep 2022]
- Sai Muttavarapu [Université Côte d'Azur, Intern, from Apr 2022 until Aug 2022]
- Kevin Yep [INRIA, Intern, from Jun 2022 until Jul 2022]
Administrative Assistant
- Isabelle Strobant [INRIA]
2 Overall objectives
Morpheme is a joint project between INRIA, CNRS and the University of Côte d'Azur (UCA); Signals and Systems Laboratory (I3S) (UMR 6070); the Institute for Biology of Valrose (iBV) (CNRS/INSERM).
The scientific objectives of Morpheme are to characterize and model the development and the morphological properties of biological structures from the cell to the supra-cellular scale. Being at the interface between computational science and biology, we plan to understand the morphological changes that occur during development combining in vivo imaging, image processing and computational modeling.
The morphology and topology of mesoscopic structures, indeed, do have a key influence on the functional behavior of organs. Our goal is to characterize different populations or development conditions based on the shape of cellular and supra-cellular structures, including micro-vascular networks and dendrite/axon networks. Using microscopy or tomography images, we plan to extract quantitative parameters to characterize morphometry over time and in different samples. We will then statistically analyze shapes and complex structures to identify relevant markers and define classification tools. Finally, we will propose models explaining the temporal evolution of the observed samples. With this, we hope to better understand the development of normal tissues, but also characterize at the supra-cellular level different pathologies such as the Fragile X Syndrome, Alzheimer or diabetes.
3 Research program
3.1 Research program
The recent advent of an increasing number of new microscopy techniques giving access to high throughput screenings and micro or nano-metric resolutions provides a means for quantitative imaging of biological structures and phenomena. To conduct quantitative biological studies based on these new data, it is necessary to develop non-standard specific tools. This requires using a multi-disciplinary approach. We need biologists to define experiment protocols and interpret the results, but also physicists to model the sensors, computer scientists to develop algorithms and mathematicians to model the resulting information. These different expertises are combined within the Morpheme team. This generates a fecund frame for exchanging expertise, knowledge, leading to an optimal framework for the different tasks (imaging, image analysis, classification, modeling). We thus aim at providing adapted and robust tools required to describe, explain and model fundamental phenomena underlying the morphogenesis of cellular and supra-cellular biological structures. Combining experimental manipulations, in vivo imaging, image processing and computational modeling, we plan to provide methods for the quantitative analysis of the morphological changes that occur during development. This is of key importance as the morphology and topology of mesoscopic structures govern organ and cell function. Alterations in the genetic programs underlying cellular morphogenesis have been linked to a range of pathologies.
Biological questions we will focus on include:
- what are the parameters and the factors controlling the establishment of ramified structures? (Are they really organize to ensure maximal coverage? How are genetic and physical constraints limiting their morphology?),
- how are newly generated cells incorporated into reorganizing tissues during development? (is the relative position of cells governed by the lineage they belong to?)
Our goal is to characterize different populations or development conditions based on the shape of cellular and supra-cellular structures, e.g. micro-vascular networks, dendrite/axon networks, tissues from 2D, 2D+t, 3D or 3D+t images (obtained with confocal microscopy, video-microscopy, photon-microscopy or micro-tomography). We plan to extract shapes or quantitative parameters to characterize the morphometric properties of different samples. On the one hand, we will propose numerical and biological models explaining the temporal evolution of the sample, and on the other hand, we will statistically analyze shapes and complex structures to identify relevant markers for classification purposes. This should contribute to a better understanding of the development of normal tissues but also to a characterization at the supra-cellular scale of different pathologies such as Alzheimer, cancer, diabetes, or the Fragile X Syndrome. In this multidisciplinary context, several challenges have to be faced. The expertise of biologists concerning sample generation, as well as optimization of experimental protocols and imaging conditions, is of course crucial. However, the imaging protocols optimized for a qualitative analysis may be sub-optimal for quantitative biology. Second, sample imaging is only a first step, as we need to extract quantitative information. Achieving quantitative imaging remains an open issue in biology, and requires close interactions between biologists, computer scientists and applied mathematicians. On the one hand, experimental and imaging protocols should integrate constraints from the downstream computer-assisted analysis, yielding to a trade-off between qualitative optimized and quantitative optimized protocols. On the other hand, computer analysis should integrate constraints specific to the biological problem, from acquisition to quantitative information extraction. There is therefore a need of specificity for embedding precise biological information for a given task. Besides, a level of generality is also desirable for addressing data from different teams acquired with different protocols and/or sensors. The mathematical modeling of the physics of the acquisition system will yield higher performance reconstruction/restoration algorithms in terms of accuracy. Therefore, physicists and computer scientists have to work together. Quantitative information extraction also has to deal with both the complexity of the structures of interest (e.g., very dense network, small structure detection in a volume, multiscale behavior, ) and the unavoidable defects of in vivo imaging (artifacts, missing data, ). Incorporating biological expertise in model-based segmentation methods provides the required specificity while robustness gained from a methodological analysis increases the generality. Finally, beyond image processing, we aim at quantifying and then statistically analyzing shapes and complex structures (e.g., neuronal or vascular networks), static or in evolution, taking into account variability. In this context, learning methods will be developed for determining (dis)similarity measures between two samples or for determining directly a classification rule using discriminative models, generative models, or hybrid models. Besides, some metrics for comparing, classifying and characterizing objects under study are necessary. We will construct such metrics for biological structures such as neuronal or vascular networks. Attention will be paid to computational cost and scalability of the developed algorithms: biological experimentations generally yield huge data sets resulting from high throughput screenings. The research of Morpheme will be developed along the following axes:
- Imaging: this includes i) definition of the studied populations (experimental conditions) and preparation of samples, ii) definition of relevant quantitative characteristics and optimized acquisition protocol (staining, imaging, ) for the specific biological question, and iii) reconstruction/restoration of native data to improve the image readability and interpretation.
- Feature extraction: this consists in detecting and delineating the biological structures of interest from images. Embedding biological properties in the algorithms and models is a key issue. Two main challenges are the variability, both in shape and scale, of biological structures and the huge size of data sets. Following features along time will allow to address morphogenesis and structure development.
- Classification/Interpretation: considering a database of images containing different populations, we can infer the parameters associated with a given model on each dataset from which the biological structure under study has been extracted. We plan to define classification schemes for characterizing the different populations based either on the model parameters, or on some specific metric between the extracted structures.
- Modeling: two aspects will be considered. This first one consists in modeling biological phenomena such as axon growing or network topology in different contexts. One main advantage of our team is the possibility to use the image information for calibrating and/or validating the biological models. Calibration induces parameter inference as a main challenge. The second aspect consists in using a prior based on biological properties for extracting relevant information from images. Here again, combining biology and computer science expertise is a key point.
4 Application domains
Among the applications addressed by Morpheme team we can cite:
- Kidney cancer classification from histological images
- IMP-RNA granules detection and classification from confocal image
- Extra-cellular matrix detection and characterization from confocal images
- Axon growth modeling
- Glial cell detection and characterization for the study of high-fat diets
- Death time detection and type classification of cells in microscopy time-lapses
- Morphogenesis and embryogenesis
- Numerical super-resolution techniques
- Design and numerical validation of non-smooth optimization algorithms with applications to biomedical imaging
- Statistical and learning-based approaches for parameter selection in imaging inverse problems
- Interpretation of contrast- and orientation-based visual illusions
5 Highlights of the year
5.1 Awards
- V. Stergiopoulou, L. Calatroni, S. Schaub and L. Blanc-Féraud were awarded the best runner-up paper award for the ISBI 2022 proceeding 18.
- V. Stergiopoulou, L. Calatroni and L. Blanc-Féraud were awarded the prix d'excellence UCA 2022 for the best runner-up paper award.
- M. Lazzaretti was awarded with the Verizon Connect Italy grant for young female researchers, Florence, Italy.
- R. Xiao and X. Descombes were awarded the best poster award at REMIA 2022 - MICCAI Workshop on Resource-Efficient Medical Image Analysis.
6 New software and platforms
6.1 New software
6.1.1 Astec
-
Name:
Adaptative Segmentation and Tracking of Embryonic Cells
-
Keywords:
3D, 4D, Data fusion, Image segmentation, Fluorescence microscopy, Morphogenesis, Embryogenesis
-
Scientific Description:
ASTEC stands for Adaptive Segmentation and Tracking of Embryonic Cells, and was first developed during L. Guignard PhD thesis, "Quantitative analysis of animal morphogenesis: from high-throughput laser imaging to 4D virtual embryo in ascidians, Léo Guignard, 2015". It was later published in "Contact area–dependent cell communication and the morphological invariance of ascidian embryogenesis, Léo Guignard at al., Science 2020"
-
Functional Description:
This software suite aims at providing quantitative analysis of multi-angle acquisitions of SPIM images, and the segmentation of the temporal series of 3D images, together with quantitative informations.
- URL:
- Publications:
-
Contact:
Grégoire Malandain
-
Participants:
Patrick Lemaire, Leo Guignard, Emmanuel Faure, Gaël Michelin
-
Partners:
CRBM - Centre de Recherche en Biologie cellulaire de Montpellier, LIRMM
6.1.2 sparse_constraint_relax
-
Name:
sparse_constraint_relaxation
-
Keywords:
Optimization, Sparse regularization, Super-resolution
-
Functional Description:
The optimization of a L2 term under a L0 term constraint is made by relaxation of the initial functional with a continuous exact functional (with same global minimizers). Numerical algorithm is coded for super-resolution for Single Molecule localization microscopy (deconvolution and super-resolution on sparse molecules). We minimize the squared norm and the relaxation Q(x), using Nonmonotone Accelerated Proximal gradient algorithm which was presented in Accelerated proximal gradient methods for nonconvex programming by Li, Huan and Lin, Zhouchen in Advances in neural information processing systems. In order to do so, we use gradient of the square norm and the proximal of Q(x). There are two functions for the proximal operator, and reccomend the fast one for the calculations, but the normal one to better understand what the program is doing. A failsafe function is added to ensure that we always obtain a k-sparse solution. The file uses a simulated acquistion, Testimage.
- URL:
-
Authors:
Arne Henrik Bechensteen, Laure Blanc-Féraud, Gilles Aubert
-
Contact:
Arne Henrik Bechensteen
-
Partner:
I3S
6.1.3 COL0RME
-
Name:
COvariance-based l0 super-Resolution Microscopy with intensity Estimation
-
Keywords:
Super-resolution, Optimization, Fluorescence microscopy, Sparsity
-
Functional Description:
The code is dedicated to the super-resolution method COL0RME (COvariance-based l0 super-Resolution Microscopy with intensity Estimation) that improves the spatio-temporal resolution of images acquired by common fluorescent microscopes and conventional blinking or flucutating fluorophores. The method is composed of two steps: the former where both the emitters' independence and the sparse distribution of the fluorescent molecules are exploited to provide an accurate localization, the latter where real intensity values are estimated given the computed support. More information is the articles: https://hal.archives-ouvertes.fr/hal-02979332, https://hal.archives-ouvertes.fr/hal-029793
-
Release Contributions:
First version
- URL:
- Publications:
-
Authors:
Vasiliki Stergiopoulou, Laure Blanc-Féraud, Luca Calatroni, Jose Henrique De Morais Goulart
-
Contact:
Vasiliki Stergiopoulou
-
Partner:
I3S
6.1.4 FluoGAN
-
Name:
Fluorescence image deconvolution via stochastic fluctuation analysis and generative adversarial learning
-
Keywords:
Image analysis, Fluorescence microscopy, Inverse problem, Optimisation, Generative Models
-
Functional Description:
This repository contains an adaptation of CryoGAN and MSR-GAN inverse problem framework for super-resolution in fluorescence microscopy.
- URL:
-
Contact:
Luca Calatroni
6.1.5 OPS
-
Name:
Off-the-grid python made simple
-
Keywords:
Off-the-grid, Computational biology, Optimisation
-
Functional Description:
* Object-oriented, based on PyTorch, * Implements Dirac measures, curve measures and dynamic measures, * Algorithms: Sliding Frank-Wolfe, Charge Sliding Fran-Wolfe, Dynamic Frank-Wolfe, Conic Particle Gradient Descent, * Examples and tests with SMLM images stack.
- URL:
-
Contact:
Bastien Laville
7 New results
7.1 ADMM-Based Residual Whiteness Principle for Automatic Parameter Selection in Single Image Super-Resolution Problems
Participants: Luca Calatroni.
In collaboration with A. Lanza, M. Pragliola, F. Sgallari (University of Bologna).
In 13 we propose an automatic parameter selection strategy for the single image super-resolution problem for images corrupted by blur and additive white Gaussian noise with unknown standard deviation. The proposed approach exploits the structure of both the down-sampling and the blur operators in the frequency domain and computes the optimal regularisation parameter as the one optimising a suitably defined residual whiteness measure. Computationally, the proposed strategy relies on the fast solution of generalised Tikhonov - problems as proposed in 38 and 34. These problems naturally appear as substeps of the Alternating Direction Method of Multipliers used to solve single image super-resolution problems with non-quadratic, non-smooth, sparsity-promoting regularisers both in convex and in non-convex regimes. After detailing the theoretical properties allowing to express the whiteness functional in a compact way, we report an exhaustive list of numerical experiments proving the effectiveness of the proposed approach for different type of problems, in comparison with well-known parameter selection strategies such as, e.g., the discrepancy principle (see figure 1).
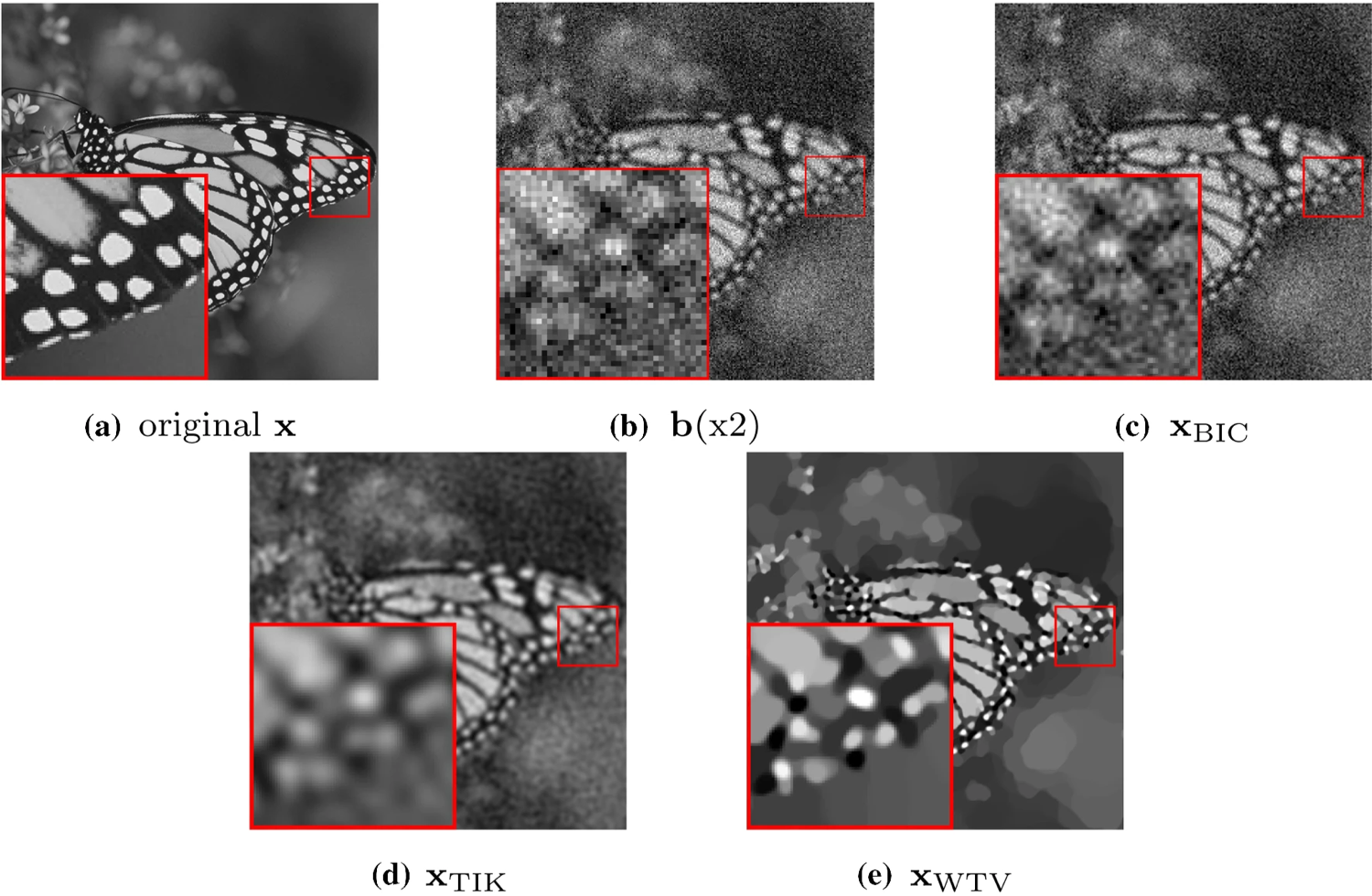
Test image (a), observed image (b), reconstruction via bicubic interpolation (c) and reconstructions obtained by applying the residual whiteness principle for automatic parameter selection to Tikhonov-ℓ2\ell _2 reconstruction model (d), and the WTV-ℓ2\ell _2 (e) variational models.
7.2 A Unified Surface Geometric Framework for Feature-Aware Denoising, Hole Filling and Context-Aware Completion
Participants: Luca Calatroni.
In collaboration with G. Reecupero, M. Huska and S. Morigi (University of Bologna).
Technologies for 3D data acquisition and 3D printing have enormously developed in the past few years, and, consequently, the demand for 3D virtual twins of the original scanned objects has increased. In this context, feature-aware denoising, hole filling and context-aware completion are three essential (but far from trivial) tasks. In 7, they are integrated within a geometric framework and realized through a unified variational model aiming at recovering triangulated surfaces from scanned, damaged and possibly incomplete noisy observations. The underlying non-convex optimization problem incorporates two regularization terms: a discrete approximation of the Willmore energy forcing local sphericity and suited for the recovery of rounded features, and an approximation of the pseudo-norm penalty favoring sparsity in the normal variation. The proposed numerical method solving the model is parameterization-free, avoids expensive implicit volume-based computations and based on the efficient use of the Alternating Direction Method of Multipliers. Experiments show how the proposed framework can provide a robust and elegant solution suited for accurate restorations even in the presence of severe random noise and large damaged areas (see figure 2).
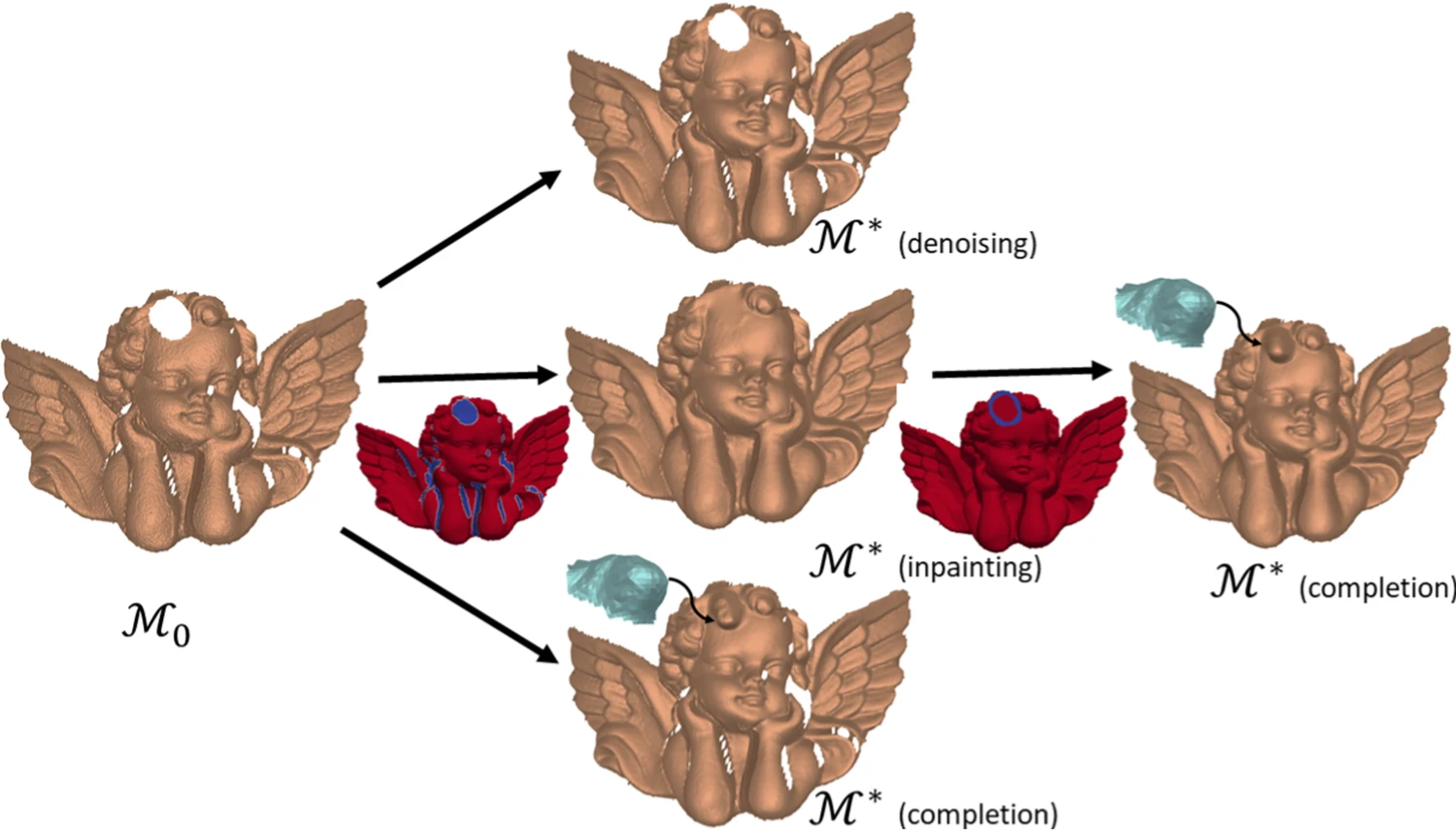
Applications of the proposed surface geometry framework: incomplete and noisy surface input ℳ0\mathcal {M}_0 (left); denoised surface ℳ*\mathcal {M}^* (center, first row), inpainting mask MVM_V and inpainting result ℳ*\mathcal {M}^* (center, second row), context-aware completion of the curly hair detail without inpainting (center, third row); context-aware completion result ℳ*\mathcal {M}^* with preliminary inpainting (right).
The project has been funded by the project Arch-AI-story at UCA and the CNRS PRIME project Imag'In.
7.3 2D & 3D covariance-based super-Resolution Microscopy with intensity Estimation and automatic parameter estimation
Participants: Luca Calatroni, Laure Blanc-Féraud, Vasiliki Stergiopoulou, Sébastien Schaub.
This work has been carried out in collaboration with J. H de Goulart (INP Toulouse/IRIT).
Super-resolution light microscopy overcomes the physical barriers due to light diffraction. However, state-of-the-art approaches require specific and often demanding acquisition conditions to achieve adequate levels of both spatial and temporal resolution. Analyzing the stochastic fluctuations of the fluorescent molecules provides a solution to the aforementioned limitations. Based on this idea, we propose COL0RME 15, a method for COvariance-based super-Resolution Microscopy with intensity Estimation, which achieves good spatio-temporal resolution by solving a sparse optimization problem in the covariance domain, discuss automatic parameter selection strategies, estimates background and noise statistics and includes a final estimation step where intensity information is retrieved. Several numerical results both on synthetic and real fluorescent microscopy images and several comparisons with state-of-the art approaches have been done. Our results show that COL0RME outperforms competing methods exploiting analogously temporal fluctuations; in particular, it achieves better localization, reduces background artifacts and avoids fine parameter tuning. In 18 a 3D COL0RME strategy is used to improve spatial resolution in Total Internal Reflectance Fluorescence (TIRF) imaging applications. The approach improves both lateral and axial resolution by combining sparsity-based modelling for precise molecule localisation and intensity estimation in the lateral plane with a 3D reconstruction procedure in the axial one using Multi-Angle TIRF (MA-TIRF). The proposed method has been validated on simulated MA-TIRF blinking-type data and on challenging real MA-TIRF acquisitions, showing significant resolution improvements (see figure 3). The paper was awarded the best runner-up paper award at the ISBI 2022 conference
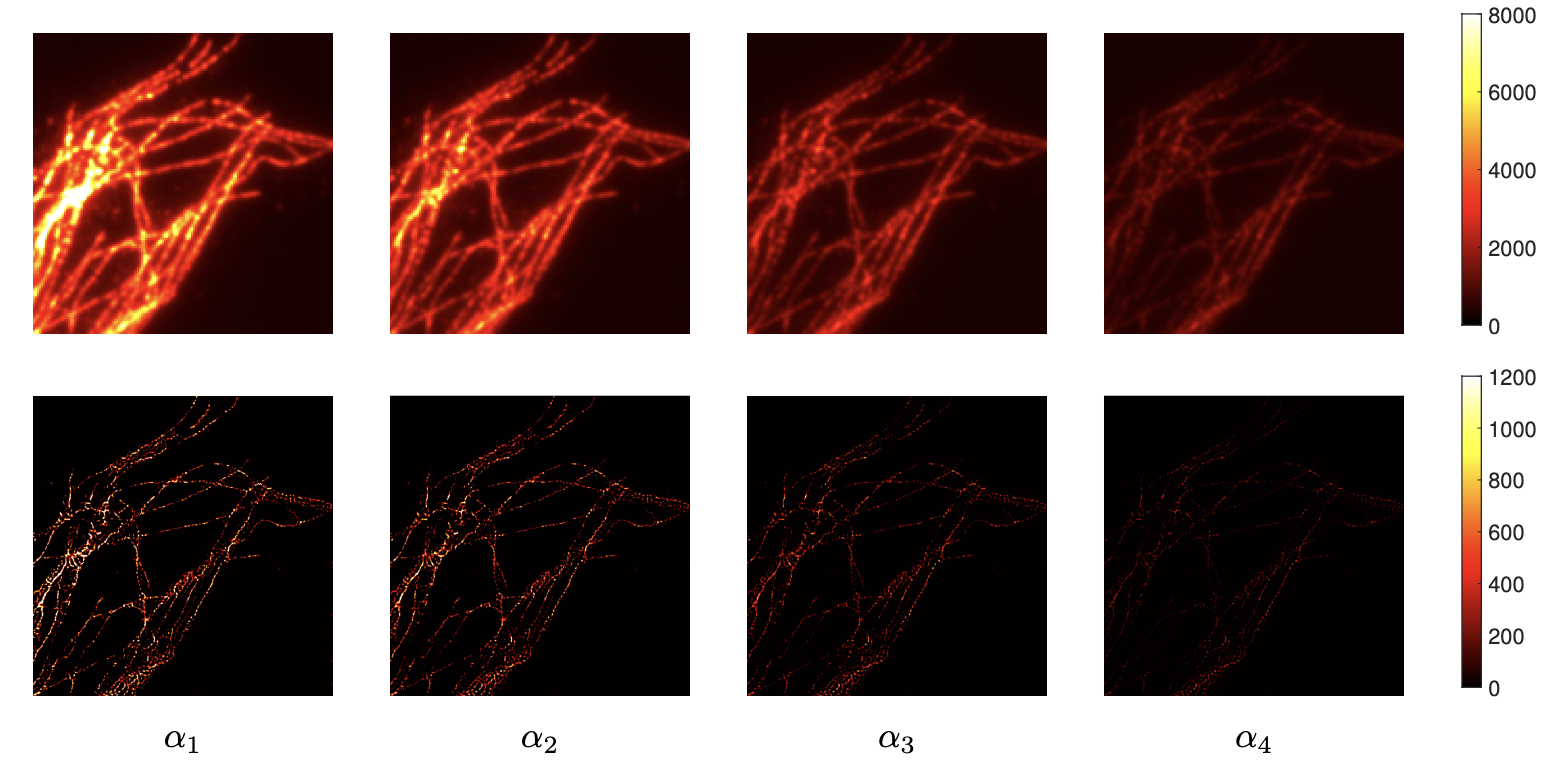
Vasiliki Stergiopoulou's work is supported by the French Government through the 3IA Côte d’Azur Investments in the Future project managed by the National Research Agency (ANR) with the reference number ANR-19-P3IA-0002.
7.4 Fluorescence image deconvolution microscopy via generative adversarial learning (FluoGAN)
Participants: Vasiliki Stergiopoulou, Laure Blanc-Féraud, Luca Calatroni, Sébastien Schaub.
In 26 we propose FluoGAN, an unsupervised hybrid approach combining the physical modelling of fluorescence microscopy timelapse acquisitions with a Generative Adversarial Network (GAN) for the problem of image deconvolution. Differently from standard approaches combining a least-square data fitting term based on one (longtime exposure) image with sparsity-promoting regularisation terms, FluoGAN relies on a data fitting term defined as a distribution distance between the fluctuating observed timelapse (short-time exposure images) and the generative model. The distance between these two distributions is computed using adversarial training of two competing architectures: a physics-inspired generator simulating the fluctuation behaviour as a Poisson process of the observed images combined with blur and undersampling, and a standard convolutional discriminator. FluoGAN is a fully unsupervised approach requiring only a fluctuating sequence of blurred, undersampled and noisy images of the sample of interest as input and it can be complemented with prior knowledge on the desired solution such as sparsity, non-negativity etc. After having described in depth the main ideas behind FluoGAN, we formulate the corresponding optimisation problem and report several results on a simulated and real phantoms used by microscopy engineers to quantitatively assess spatial resolution. The comparison of FluoGAN with state-of-the-art methodologies shows unprecedented resolution and allows for high-precision reconstruction of very fine structures in challenging real Ostreopsis cf Ovata data (see figure 4).
![]() |
![]() |
7.5 Off-the-grid dynamic super-resolution for fluorescence microscopy
Participants: Bastien Laville, Laure Blanc-Féraud, Gilles Aubert.
The last few years have seen the development in super-resolution of variational optimization in measurement spaces. These so-called "gridless" approaches offer both theoretical (uniqueness, reconstruction guarantees) and numerical results, with very convincing results in biomedical imaging. However, gridless variational optimization is formulated for the reconstruction of point sources, which is not always suitable in biomedical imaging applications: one would also need to reconstruct more realistic biological structures such as curves, to represent blood vessels or filaments.
We developed in 2022 a new approach allowing gridless reconstruction of curves, understood as the reconstruction of measurements carried by curves from a degraded acquisition. By introducing a new space of measures with finite divergence, as well as a new CROC functional, we give a result on the extremal points of the unit ball of the norm; this allows us to reconstruct with a variational gridless approach curves, in an inverse problem setting:
This also opens up promising prospects for the digital implementation, shown on the Figure 5, that we began in 2022 and continue in 2023.
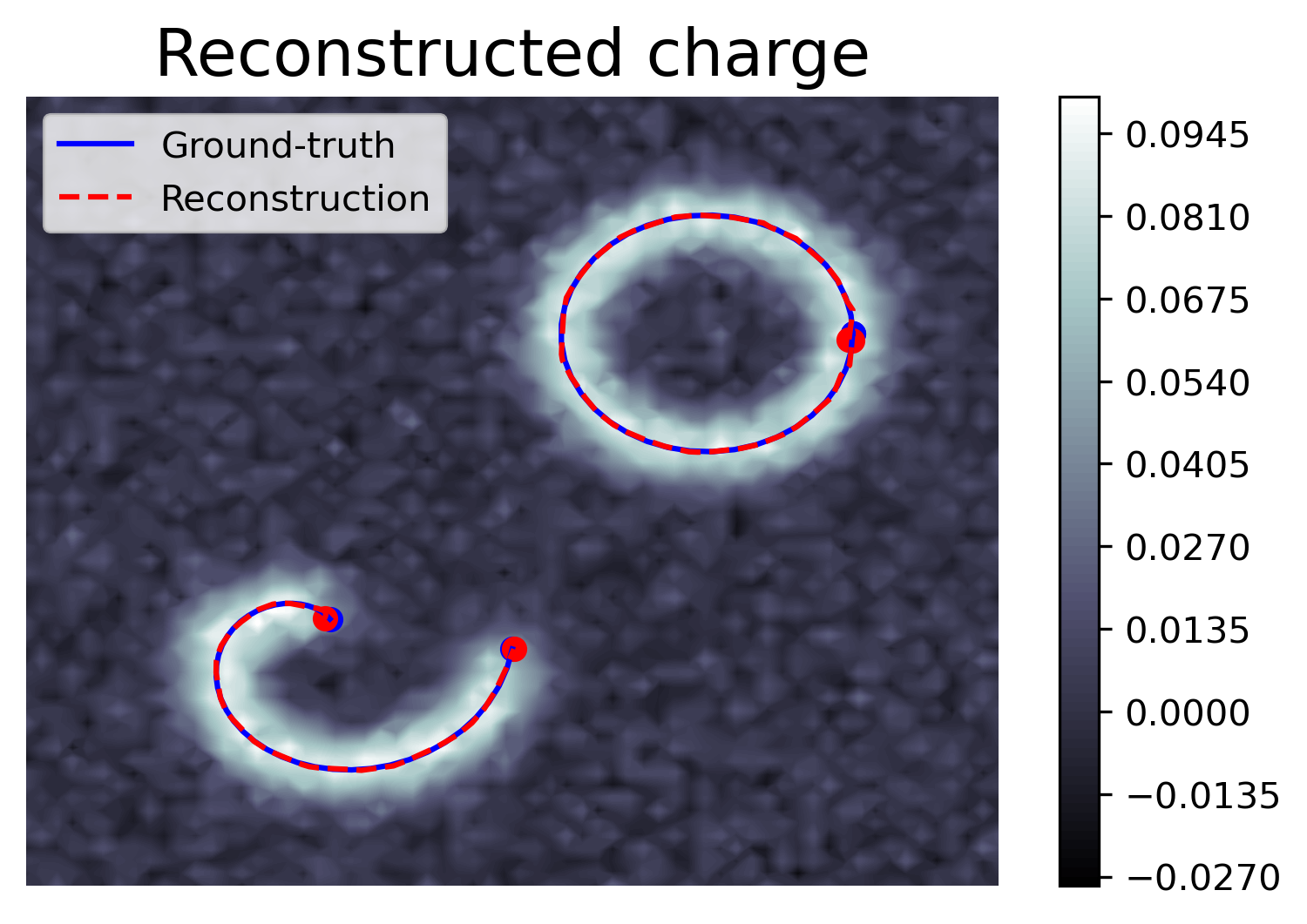
This work is published in 16, 22 and the report 28 appear in SIIMS journal, Janvier 2023.
A code is available at 6.1.5
7.6 Nearest-Neighbor Kernel for the Classification of Plankton Images
Participants: Cédric Dubois, Eric Debreuve.
This work was carried out in collaboration with Jean-Olivier Irisson (LOV, Villefranche-sur-mer, France).
Plankton organisms are a key component of the Earth bio-sphere. Most notably, the phytoplankton captures carbon from the atmosphere, and the zooplankton aggregates it and stores it on the ocean floor. This so-called carbon pump process is studied by ecologists in order to predict its future efficiency in a climate change era. A modern approach consists in studying the relation between the living environment of the organisms and their functional traits, among which the size stands out. Indeed, a high correlation has been observed between zooplankton size and carbon sequestration. Scientists have developed in situ imaging instruments and built large databases. Taxonomic identification from images is required to estimate the individual volumes of the organisms based on their morphology. The development of automated classification methods has been essential to aid operators in their identification task. Specifically, the breakthrough of Artificial Neural Network (ANN) made it possible to come up with efficient classifiers. However, the decisions of such classifiers are hard to interpret or explain. We put forward the idea that following the transform-then-classify-simply approach of ANNs using a simple, explicit transform can result in a classifier whose predictions are both interpretable (thus, trustable) and accurate. The proposed transform is defined as a linear combination of optimal, per-class targets, and the classification is performed, like with ANNs, by a nearest-target decision (see Fig. 6). Furthermore, as a main theoretical result, we establish that the proposed transform defines a kernel associated with the Weigthed-k-Nearest-Neighbor (W-kNN) classifier, and allows interpreting the W-kNN classifier as a member of a larger family of target-based classifiers which satisfies an optimality criterion. We propose a modern W-kNN implementation that allows to deal with large scale databases. We were then able to perform a Leave-One-Out Cross-Validation on a large in situ plankton images dataset from the Underwater Vision Profiler (UVP).
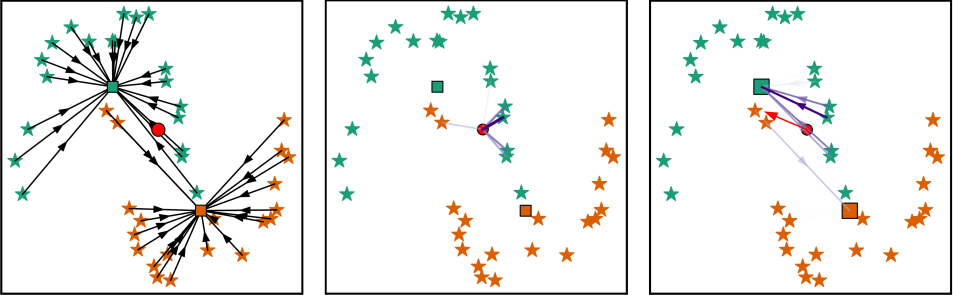
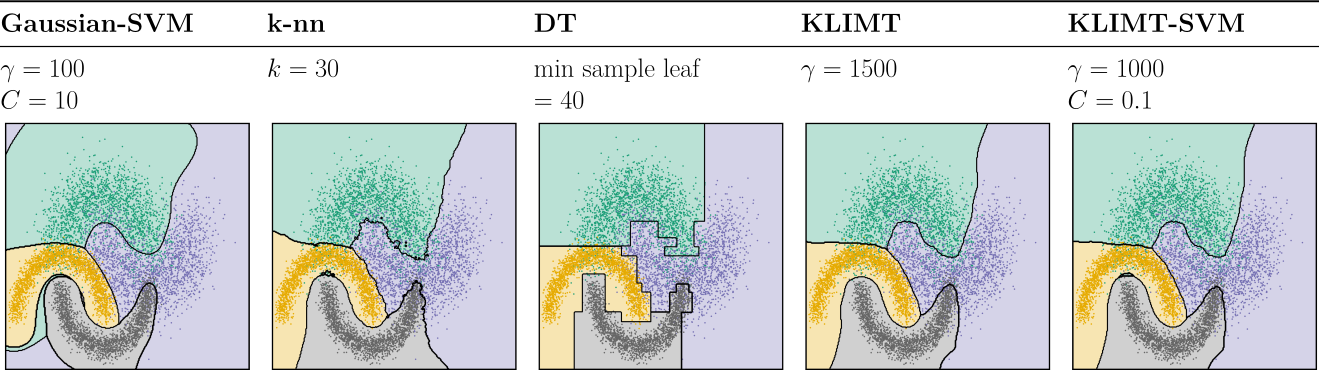
7.7 Dynamic analysis framework to detect cell division and cell death in live-cell imaging, using signal processing and machine learning
Participants: Asma Chalabi, Eric Debreuve.
This work was made in collaboration with Jérémie Roux, Ircan, Nice, France.
The detection of cell division and cell death events in live-cell assays has the potential to produce robust metrics of drug pharmacodynamics and return a more comprehensive understanding of tumor cells responses to cancer therapeutic combinations. As cancer drugs may have complex and mixed effects on the biology of the cell, knowing precisely when cellular events occur in a live-cell experiment allows to study the relative contribution of different drug effects (such as cytotoxic or cytostatic) on a cell population. Yet, classical methods require dyes to measure cell viability as an end-point assay, where the proliferation rates can only be estimated when both viable and dead cells are labeled simultaneously, not to mention that the actual cell division events are often discarded due to analytical limitations. Live-cell imaging is a promising cell-based assay to determine drug efficacies. However, its main limitation remains the accuracy and depth of the analyses, to acquire automatic measures of the cellular response phenotype, making the understanding of drug action on cell populations difficult. We propose a new algorithmic architecture integrating machine learning, image and signal processing methods to perform dynamic image analyses of single cell events in time-lapse microscopy experiments of drug pharmacological profiling. Our event detection method is based on a pattern detection approach on the polarized light entropy, making it free of any labeling step and exhibiting two distinct patterns for cell division and death events. Our analysis framework is an open source and adaptable workflow that automatically predicts cellular events (and their times) from each single cell trajectory, along with other classic cellular features of cell image analyses, as a promising solution in pharmacodynamics. (see Figure 7).
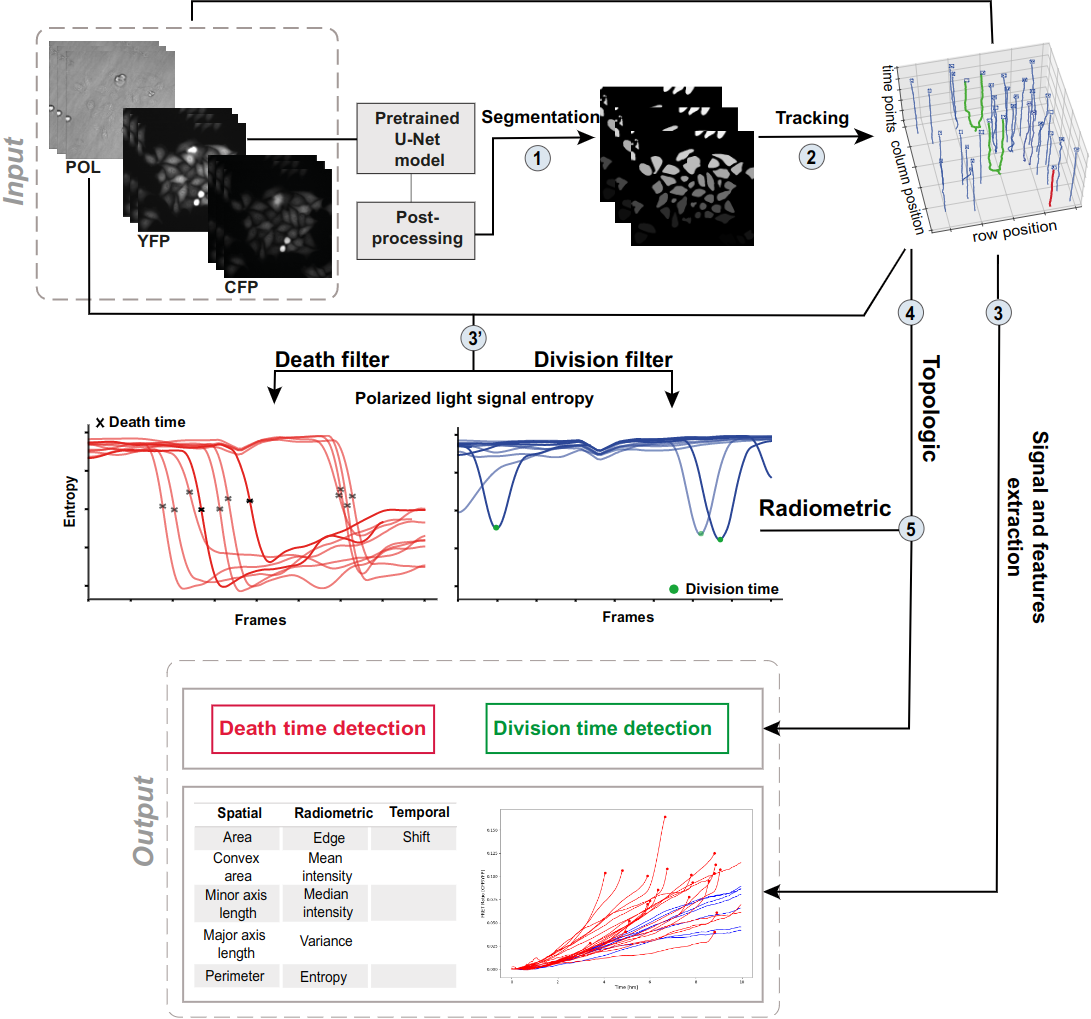
7.8 Developing methodologies to quantitatively analyze RNP granule dynamic properties upon aging
Participants: Assia Chahidi, Eric Debreuve, Xavier Descombes, Florence Besse.
This work was made in collaboration with Fabienne de Graeve, iBV, Nice, France.
Recent technological advances in bioimaging have enabled biologists to investigate dynamic processes in living cells at high spatial and temporal resolutions. Quantitative analysis of these dynamic processes relies on the development of appropriate tracking methods associated with a spatio-temporal analysis. We developed particle tracking and event detection methods to analyze the movements and interactions of Imp-RNP granules in the cell bodies of mushroom neurons in Drosophila brain explants. The individual granule trajectories were considered globally as a graph embedded in a space-time domain. The base granule fusion and splitting events can occur in specific sequences which build particular graph patterns. The proposed analysis consisted in defining the relevant patterns, and then searching them in actual graphs. (see Figure 8).

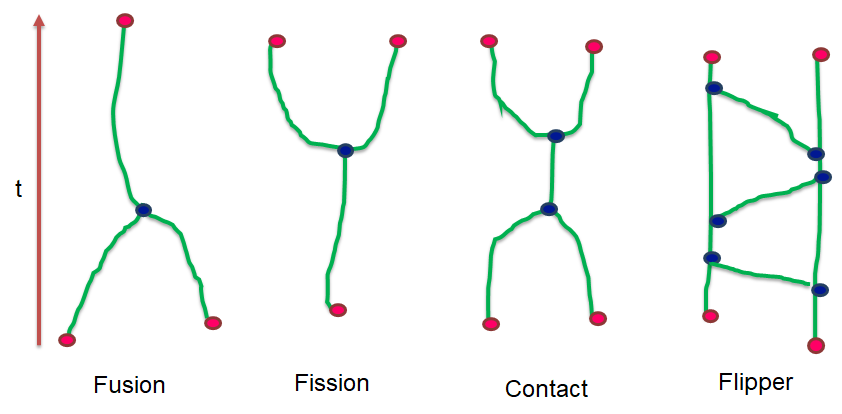
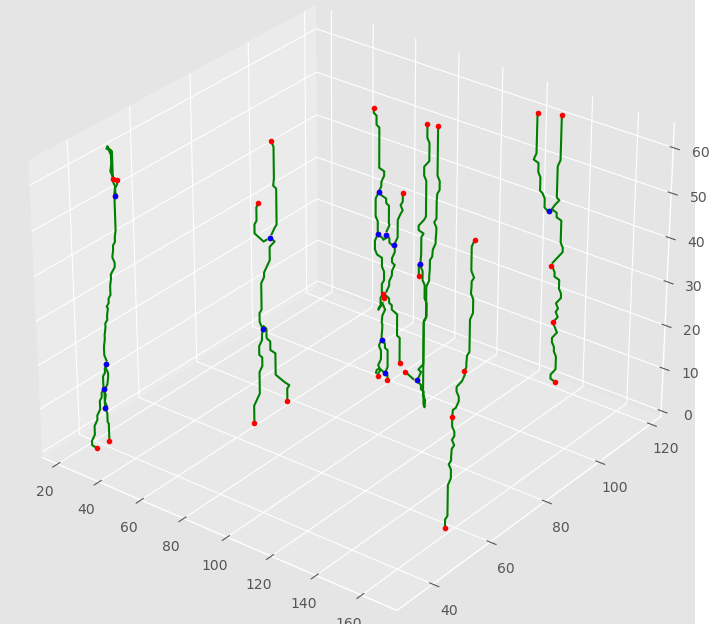
7.9 Histopathological image classification from nuclei
Participants: Yueqiao Huang, Xavier Descombes.
This work has been carried out in collaboration with Dr Damien Ambrosetti from Nice CHU.
The aim of this project is to segment histopathological images into tumor, non tumor and fibrous areas based on nuclei information. The first step, consisting of nuclei detection, is performed by applying successively color deconvolution, local normalization, Otsu thresholding and spatial smoothing using mathematical morphology. We then extract several parameters describing the nuclei shape and the grey level statistics within the nuclei and in its neighborhood. A statistical analysis of the parameters histograms provides a features selection. The most discriminating parameters are then classified using the unsupervised k-means algorithm. The last step consists in regularizing this first classification. We consider as graph description of the nuclei population provided by the Delaunay tessellation. The nuclei label are finally regularized using a set of rules derived from the majority vote principle.
First results have shown that our approach provide a description of the different tissues based on their nuclei population. On figure 9 can notably distinguish the tumor area on left from a fibrous area. Currently the nuclei classes are defined based on an unsupervised classification. We currently investigate a supervised classification based on a predefined dictionary of cells.
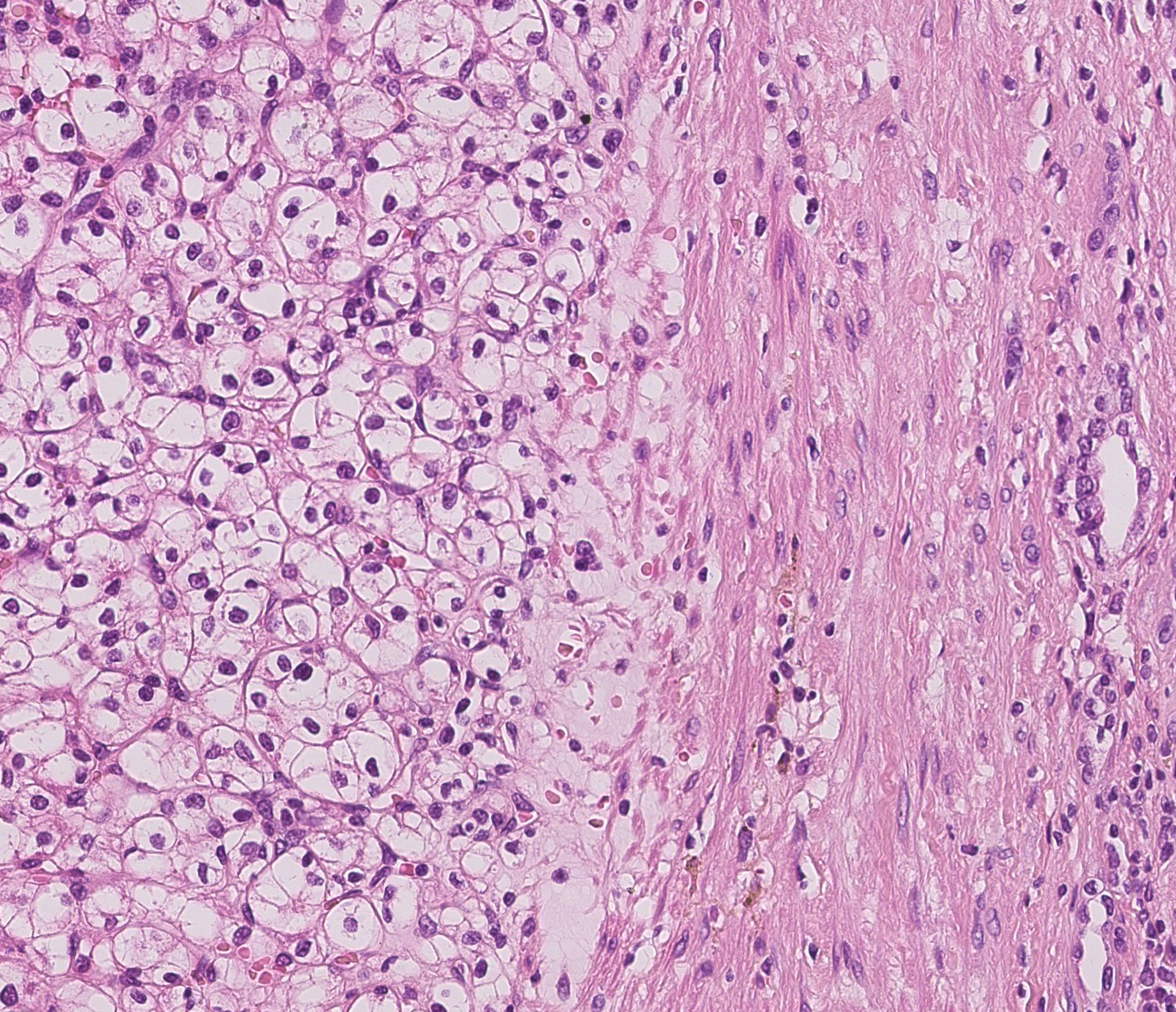
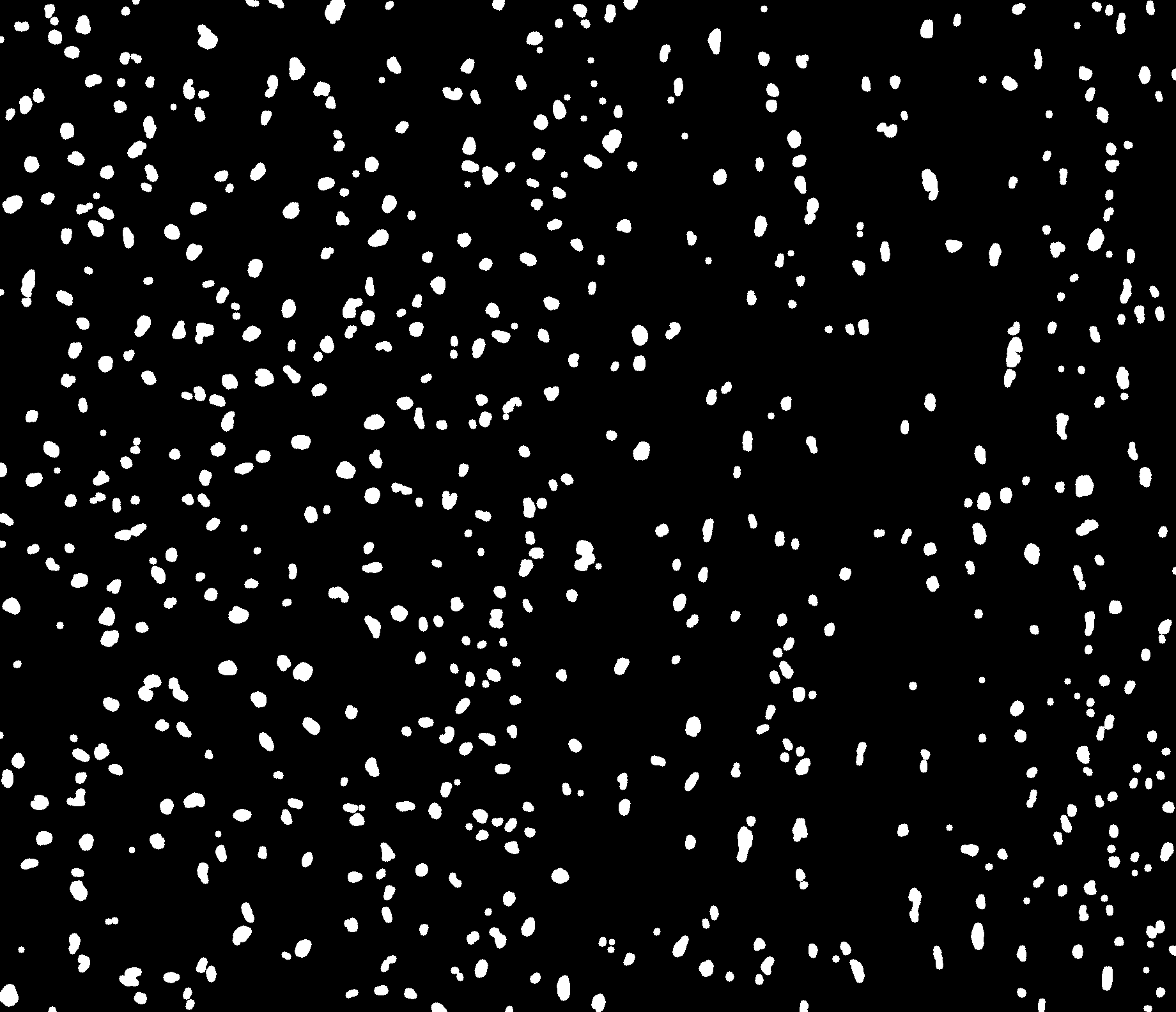
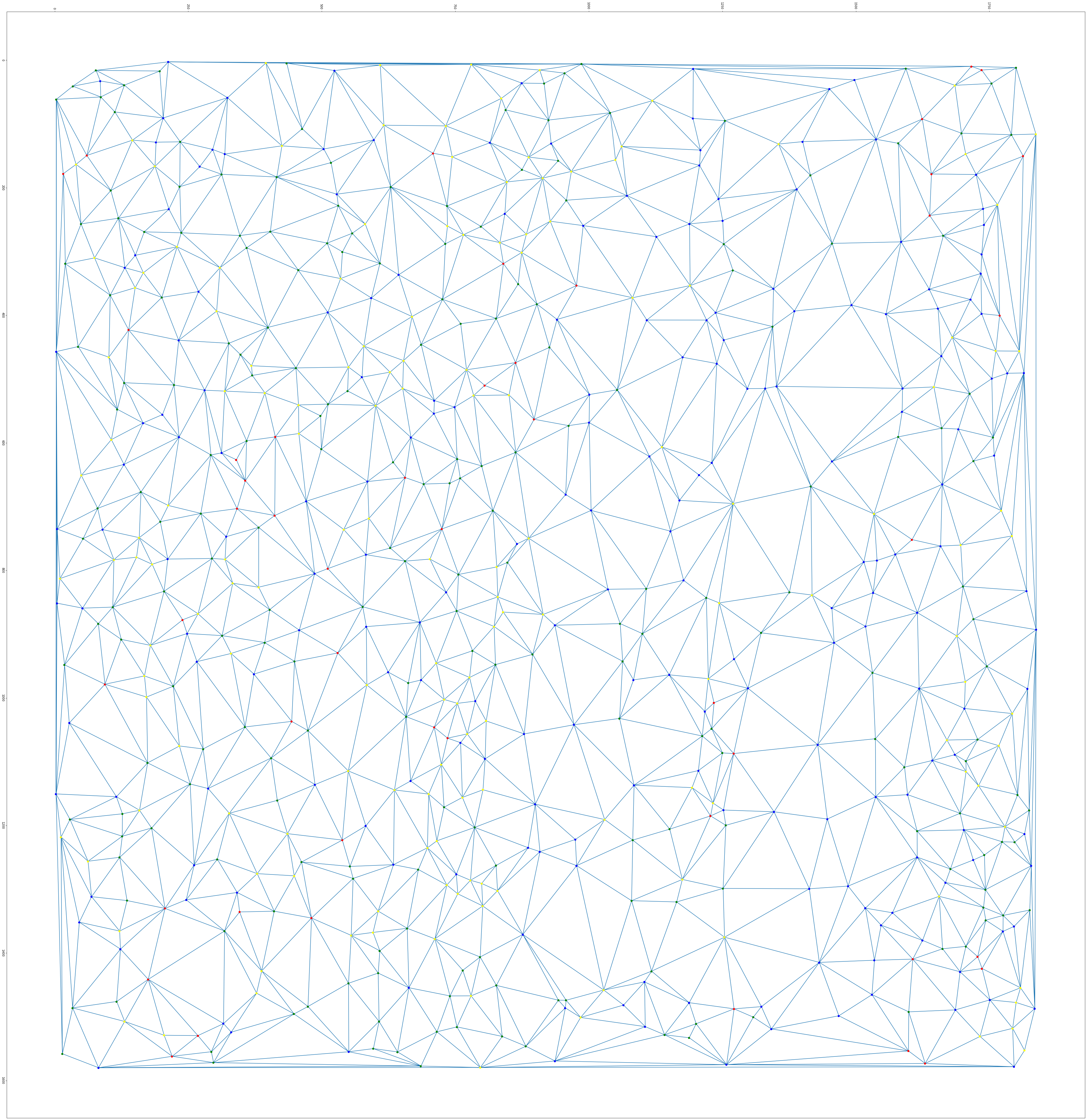
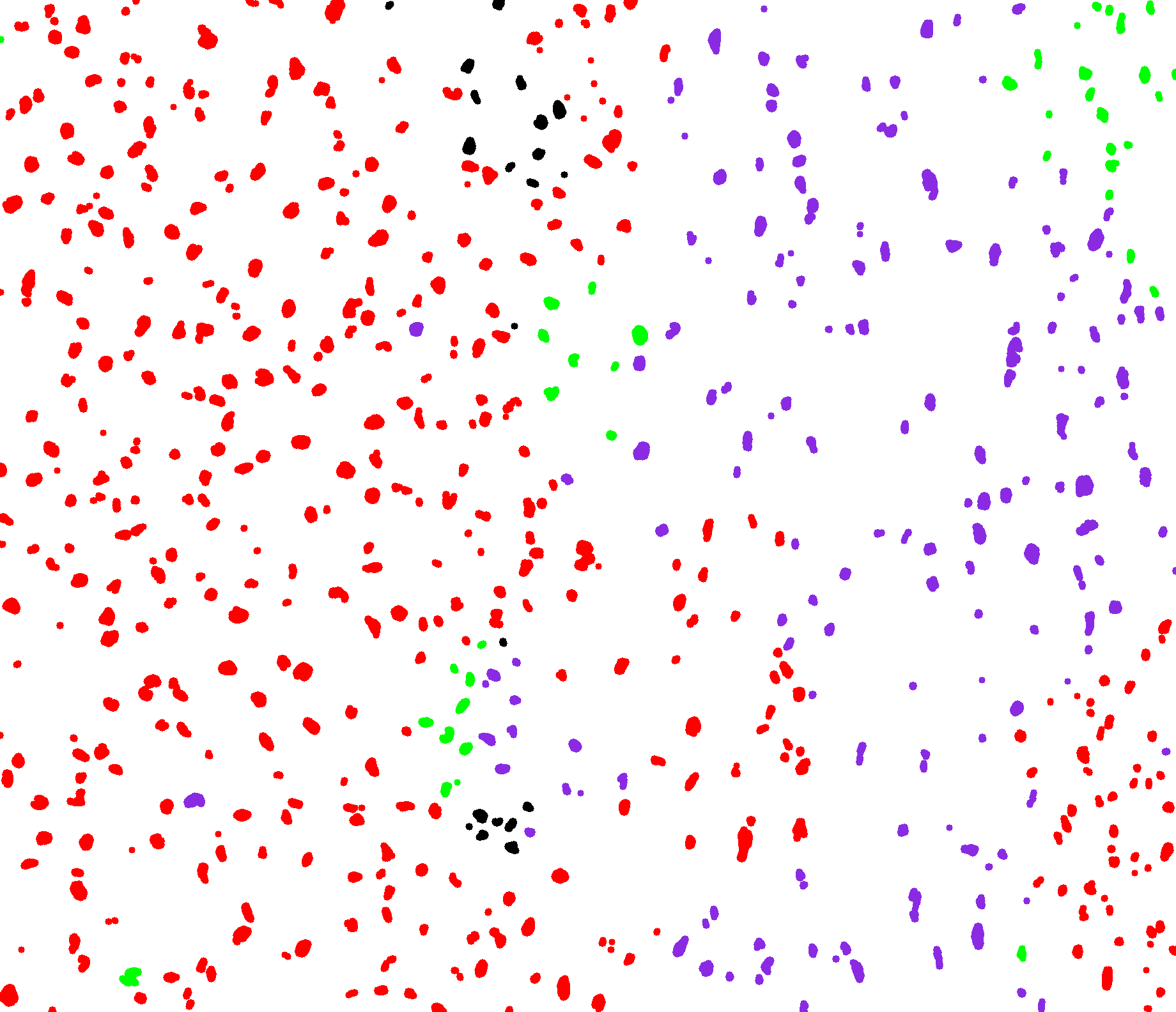
7.10 Simultaneous vascular network segmentation and classification using a multi-tasks semi-supervised neural network
Participants: Rudan Xiao, Xavier Descombes.
This work was carried out in collaboration with Damien Ambrosetti, Nice CHU. It was supported by a PACA PhD grant
Vascular network analysis is crucial to define the tumoral architecture and then diagnose the Renal Cell Carcinoma (RCC) subtypes. However, automatic vascular network segmentation from histopathological images is still a challenge due to the background complexity. Last year, we have proposed a method that reduces reliance on labeled data through semi-supervised learning (SSL). Additionally, considering the correlation between tumor classification and vascular segmentation, we have proposed a multi-task learning (MTL) model, which can simultaneously segment the vascular network using SSL and predict the tumor class in a supervised context. To train the network we have considered weakly labeled data consisting of constant width bands vessels. We used this annotation approach to speed up the process. Besides, we aim to classify RCC subtypes based on the skeleton of the vascular network that reflect the network connectivity. Therefore, the vessel width can be ignored. In this situation the Dice loss function, based on the objects area, is not adapted. We have proposed a new loss function robust with respect to width errors. We dilate (with a disk of radius 3), the segmentation result from S to obtain DS and the ground truth GT to obtain DGT. We computed the ratio of miss-detected vessels as:
and the ratio of falsely detected vessels as:
Finally, we defined the following global performance index:
Figure 10 illustrates the errors between the real mask and the manual detection (so called ground truth) and the errors of the obtained segmentation.
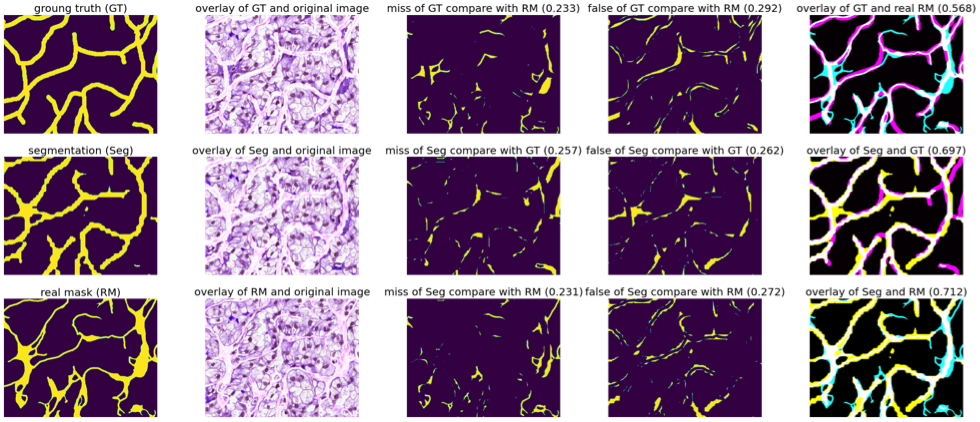
Missing part and false part between the generated segmentations, weakly labels, and real vascular masks (on the overlay images in the last column: cyan represents the real mask, magenta represents the ground truth, yellow represents the segmentation by our proposed MTL-SSL and white represents the overlap part).
Missing part and false part between the generated segmentations, weakly labels, and real vascular masks (on the overlay images in the last column: cyan represents the real mask, magenta represents the ground truth, yellow represents the segmentation by our proposed MTL-SSL and white represents the overlap part).
7.11 Self Supervised Neural Network for kidney cancer classification from histopathological images
Participants: Mohamad Mohamad, Xavier Descombes.
This work was carried out in collaboration with Francesco Ponzio from Politechnico di Torino (Italy) and Damien Ambrosetti from Nice CHU.
Annotation scarcity is a major obstacle for the effective deployment of deep networks in the medical field. Based on that, our goal was to investigate the uprising Self-Supervision methodology to help us leverage models performance even with few annotations. We investigate a new self supervision pretext task with RCC WSI dataset. The pretext task proceeds as patch allocation/localization in an image by exploiting different level of magnification/resolution benefiting from both object-level (cell, nuclei) and tissue-level features. We consider 2 images from 2 different magnification levels, the image of higher resolution (patch) lies inside the one with lower resolution. The task consists in assigning a label to the patch corresponding to one of the 16 possible locations in the low resolution image. To complexify the task we also consider rotated and flipped patches. This pretext task does not require any annotations, its goal is to learn the features extraction part (or encoder) of the network.
We then consider a classification task with few annotated data by learning only the decoder part. The classification at the patient level is obtained by a majority vote among the different patches. We have compared the proposed approached using a ResNet four times smaller than the network used with transfer learning from ImageNet database (see figure 11)
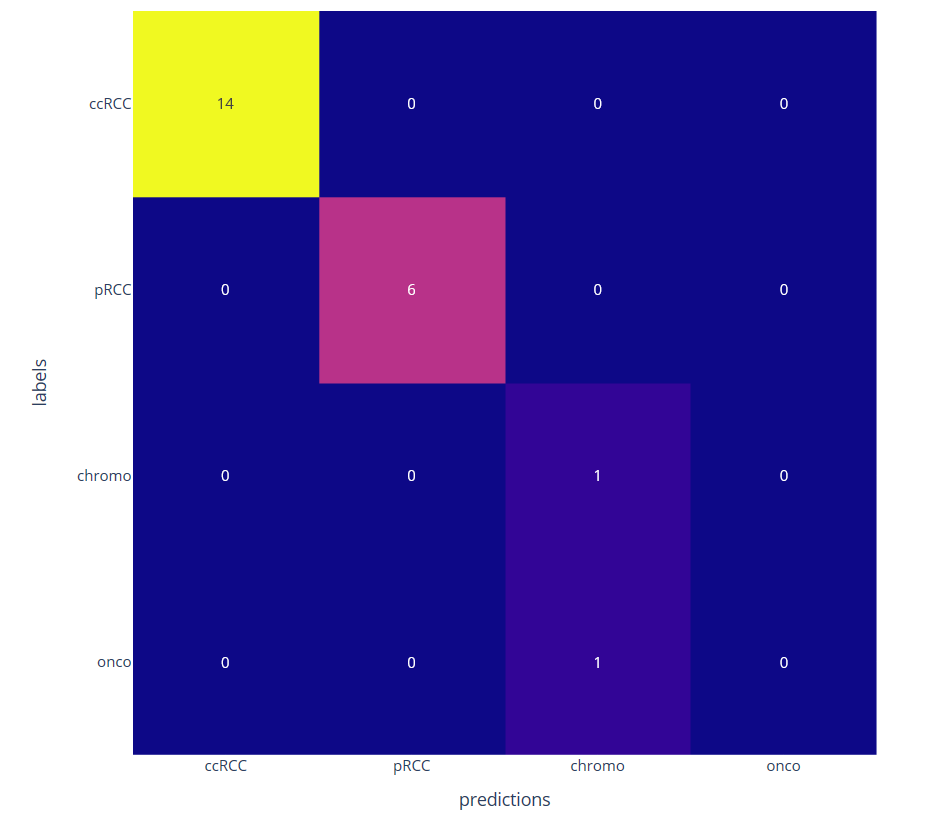
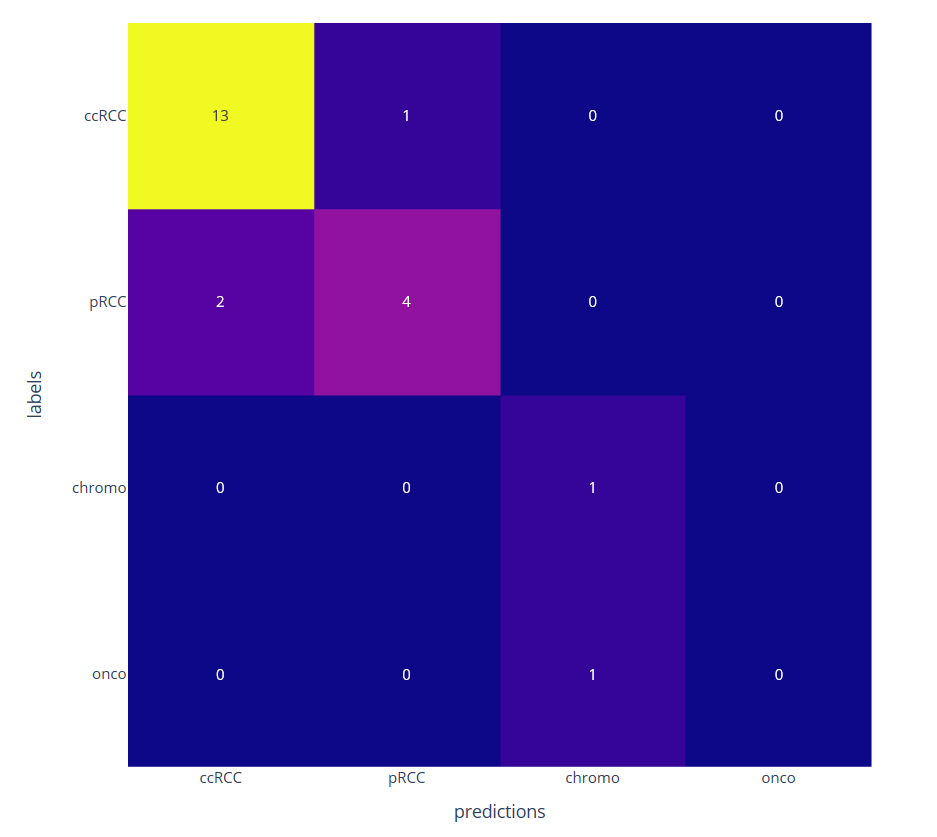
Confusion matrix on four kidney subtypes classification obtained with the proposed self-supervised (left) and the transfer learning (right) approaches on 22 patients leading to respectively 1 and 4 misclassifications
7.12 Automatic cell identification and analysis on in vivo reflectance confocal microscopy images of the human epidermis
Participants: Imane Lboukili, Xavier Descombes.
This work was carried out in collaboration with Georgios Stamatas (Johnson & Johnson Consumer Health R&D) as part of a collaboration contract with Johnson & Johnson.
The study of Reflectance Confocal Microscopy (RCM) images provides information on epidermal structure, a key to skin health and barrier which changes in each epidermal layer, with age and with certain skin conditions. RCM can also reveal dynamic changes to the epidermis in response to stimuli, as it enables repetitive sampling of the skin without damaging the tissue. Studying RCM images requires manual identification of each cell to derive its geometrical and topological characteristics, which is time-consuming and subject to expert interpretation. More insights could be derived from these data if not for the tediousness of their manual segmentation, highlighting the need for an automated cell identification method.
We have previously proposed a method for automated cell detection in RCM images of the epidermis based on the morphological features of the cells 23. This year we have validated this approach on the study of skin maturation and ageing in people aged 0 to 80 years old on the cheek and volar forearm (paper under review, first large-scale study using RCM images 36).
To overcome manual parametrization and reduce computational time we have proposed a new unsupervised approach based on multi-task learning. Our approach consists of a dual-task Cycle Generative Adversarial Networks model. The architecture is composed on two Cycle GAN to respectively learn RCM images noise and/or texture model from the initial image and the epidermis structure from a Gabor filtering (see figure 12). To our knowledge, this is the first time a Cycle GAN has been embedded in a multi-task learning model.
An international patent has been submitted for this work.
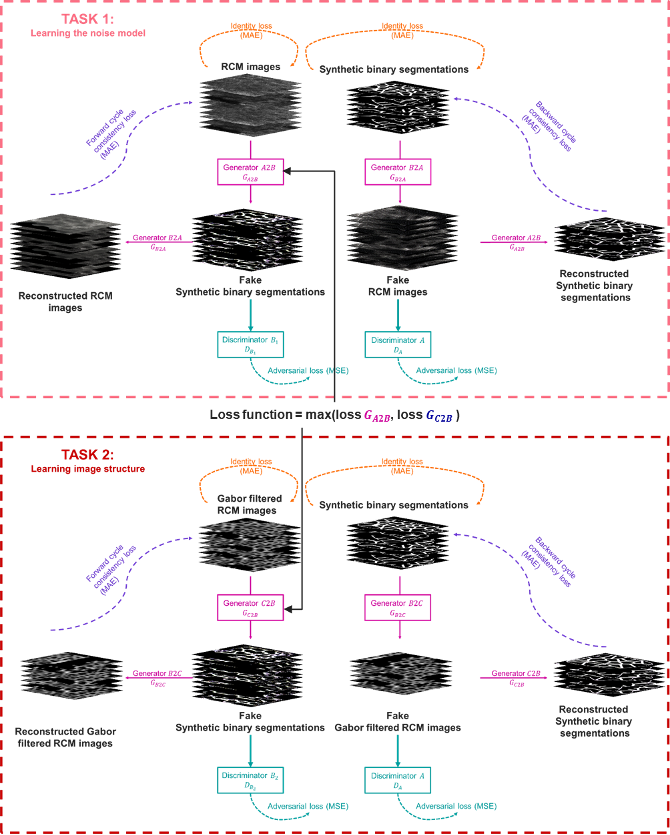
Multi-task Cycle Generative Adversarial Networks architecture. The first task maps domain A (RCM images) to the unpaired domain B (synthetic binary segmentation) while the second task learns the structure RCM images of the epidermis by translating domain C (Gabor filtered RCM images) into domain B.
Multi-task Cycle Generative Adversarial Networks architecture. The first task maps domain A (RCM images) to the unpaired domain B (synthetic binary segmentation) while the second task learns the structure RCM images of the epidermis by translating domain C (Gabor filtered RCM images) into domain B.
7.13 Machine learning for organoid phenotyping
Participants: Alexandre Martin, Xavier Descombes.
This work is part of the ANR project Morpheus.The aim of this work is to built a phenotypic map of organoid. We model the organoid as a 3D shape containing several layers of cells composed of 2D point patterns on the sphere. Our goal is to classify organoids with respect to these three components.
We first focus on the classification of point patterns on the sphere. We have create a synthetic database of 2D point patterns composed of simulations of different models such as Poisson and Mattern processes (see figure 13 top). We have proposed a new neural network architecture to estimate both the model and its parameters value from a set of points on the sphere, interpreted as a graph using the Delaunay tesselation. This deep learning approach has been compared with the estimation obtained from statistical tests.
Beside, we have proposed a pipeline to analyze 3D confocal images as shown on figure 13 (middle and bottom lines). Nuclei are detected using a template matching approach. We estimate the organoid hull by computing an alpha shape from the set of detected nuclei centers. We then classify the nuclei with respect to their distance from the organoid hull. The obtained classes correspond to the different layers. Finally we approximate the organoid shape by a sphere using a matching to the first layers centers and project nuclei centers on this sphere to obtained the 2D point patterns. This process provides the three components of our organoid model that are a 3D shape, a number of cell layers and 2D point patterns on the sphere corresponding to the different layers.
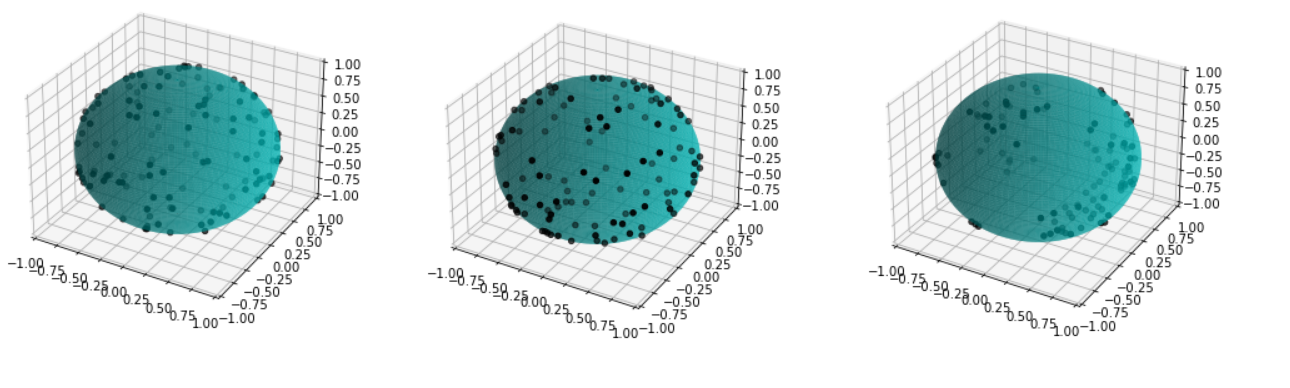
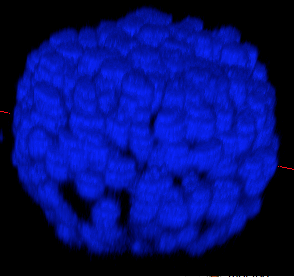
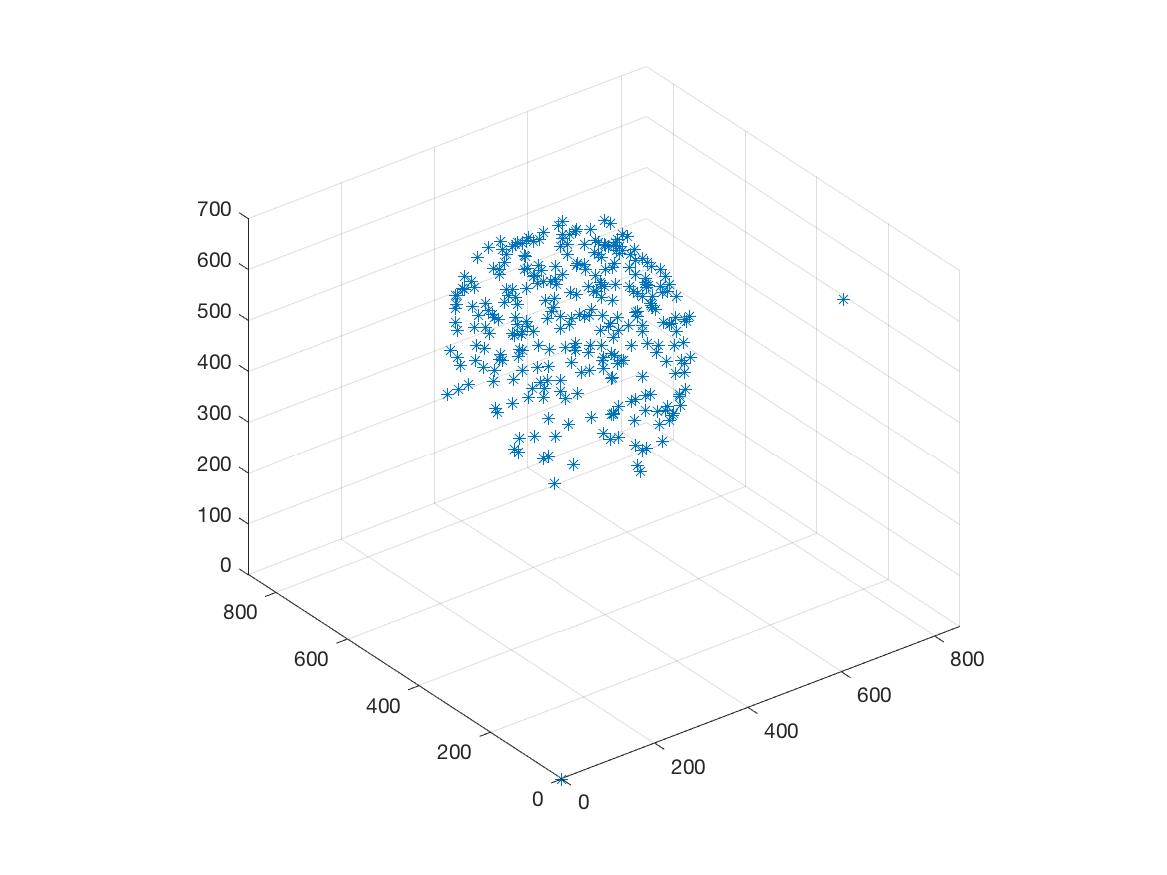
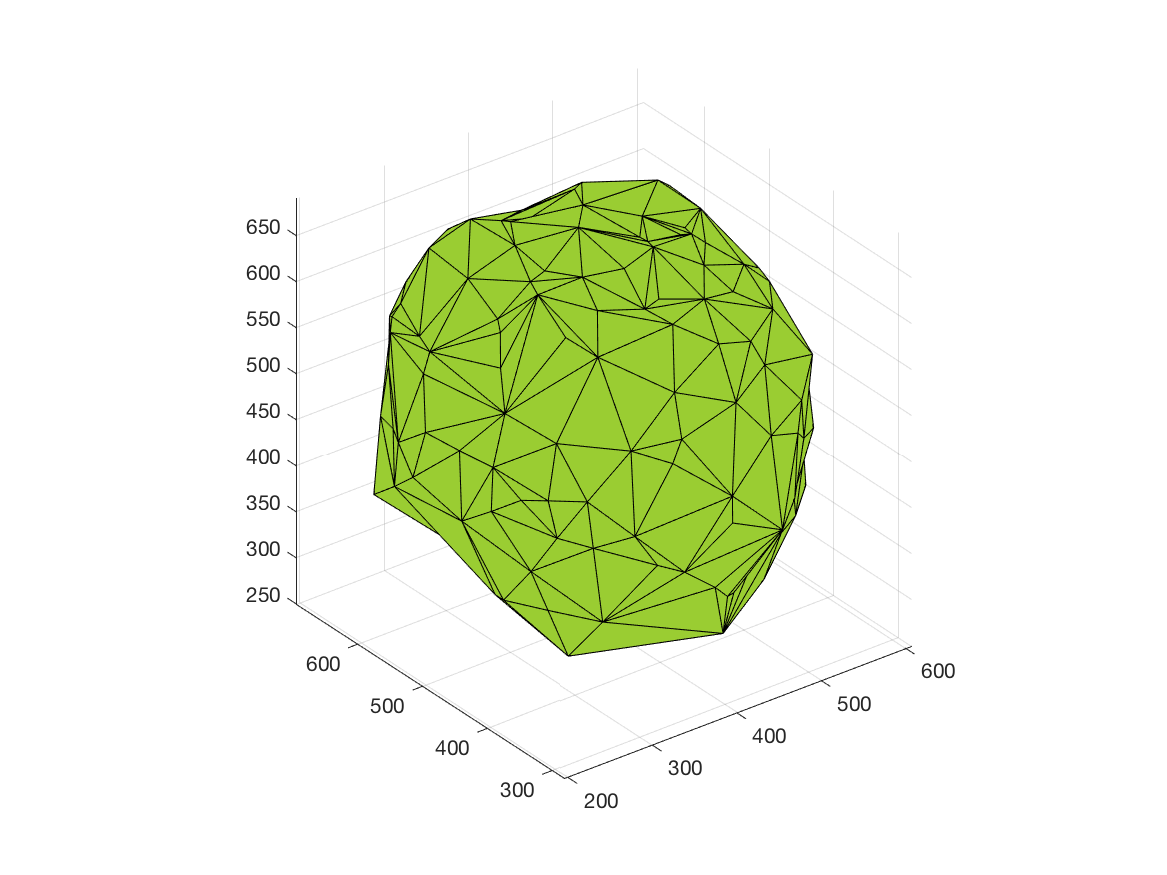
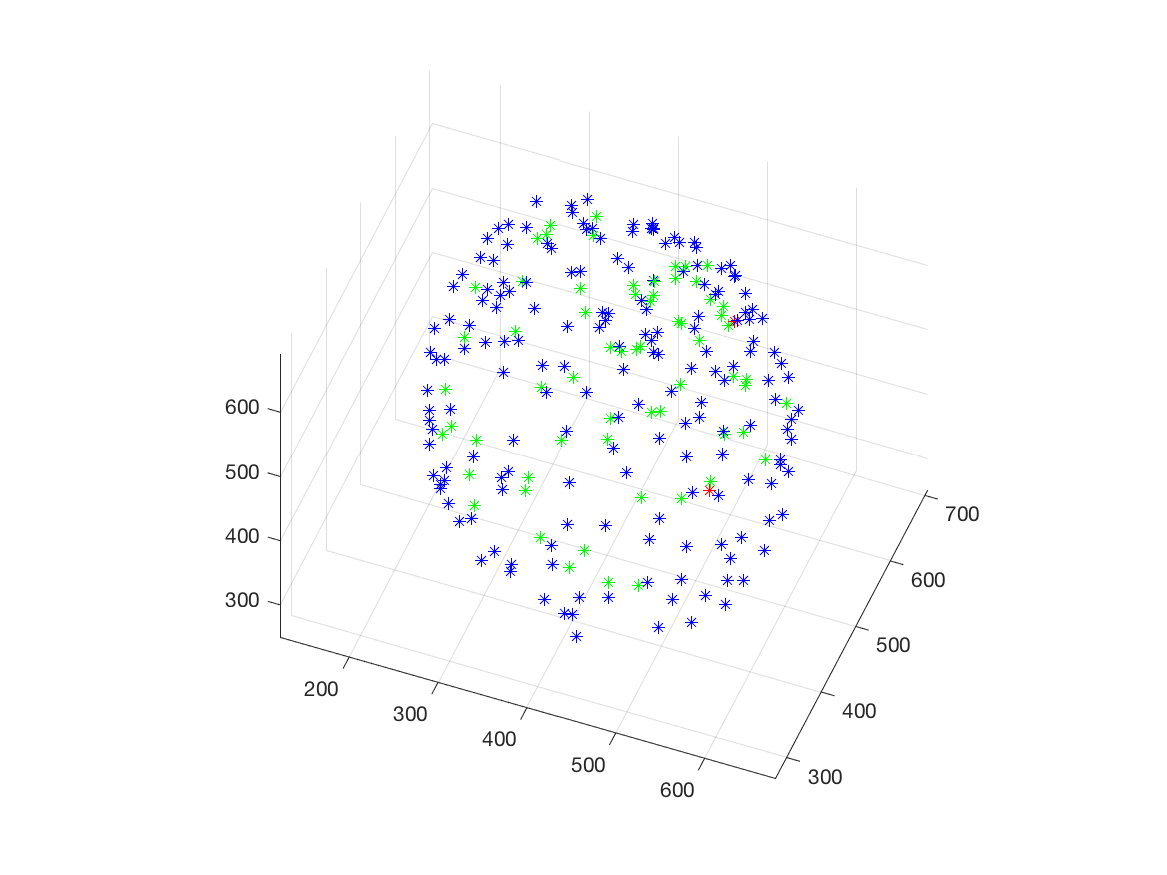
7.14 Geometrical and statistical analysis of the extra-cellular matrix
Participants: Faisal Jayousi, Esther Jammes, Xavier Descombes, Laure Blanc-Féraud.
This work was carried out in collaboration with Ellen Van Obberghen-Schilling and Morgane Rogissard, iBV, Nice, France.
We studied in this work the protein named fibronectine that is a main component of the extra-cellular matrix. We have imaged a cohort of several hundred tissue samples on a fluorescence multiplex scanner. We then have defined six different classes to characterize the fibronectin patterns as for example aggregates, intracellular, aligned, net shaped. Our goal is to recognize these patterns on images to obtained a segmentation map. We have considered the PanCK channel to segment the tumor, the DAPI channel to detect nuclei. Adding these information to the fibronectine channel we have derived a decision tree to classify the different fibronectine areas. To translate this decision tree into an algorithm, the main challenge was to differentiate aligned and net-shaped structures. To do so, we considered a graph representation of the fibronectine network (see figure 14). The graph has been extracting by first filtering the image with modified Gabor functions, binarizing and skeletonizing the filtered image.
We are currently finalizing the approach and expect to differentiate the classes from the topological information of the graphs (for example from the ratios of the nodes order). Finally, we expect to link these classes to the patient response to immunotherapy.
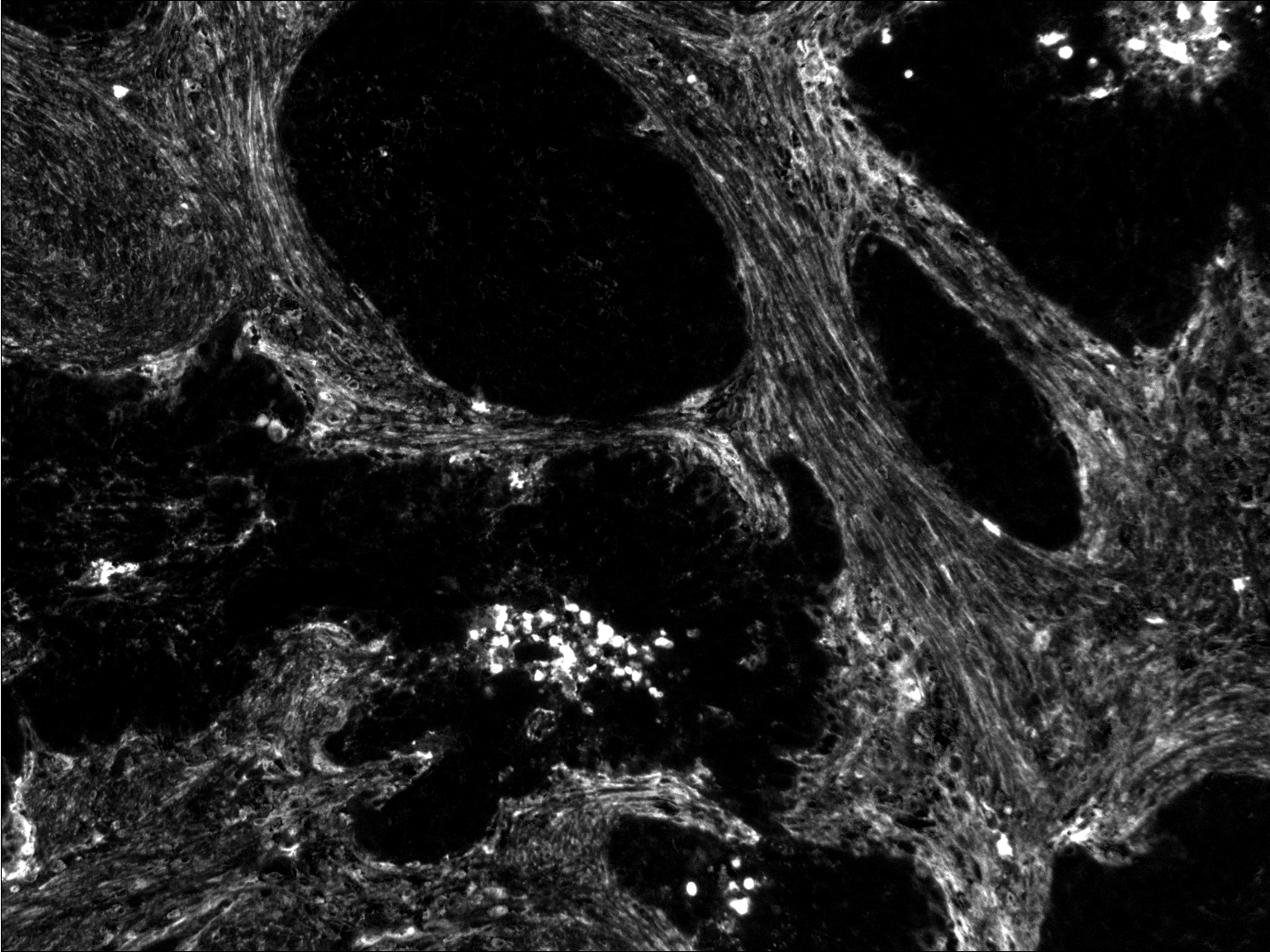
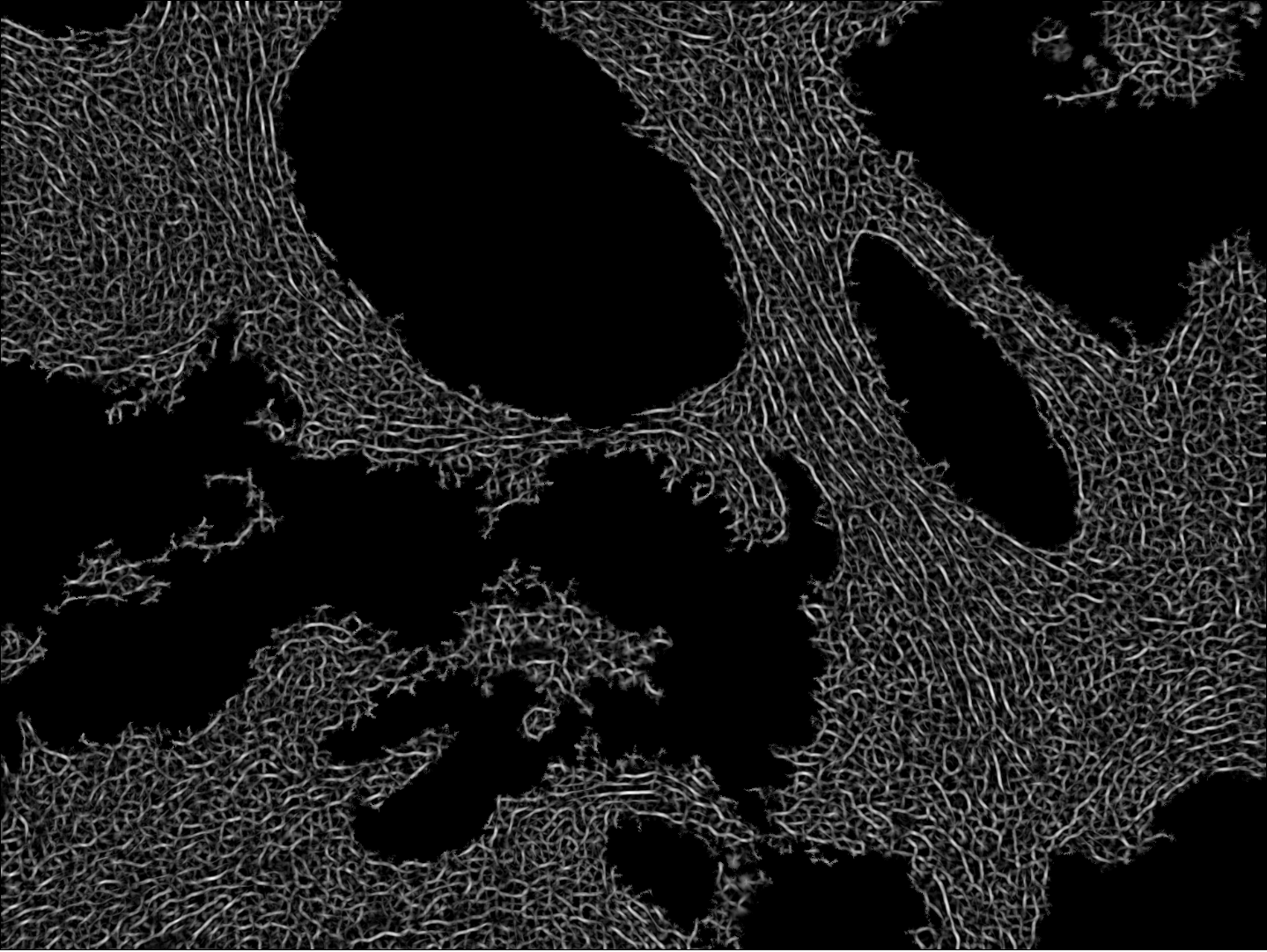
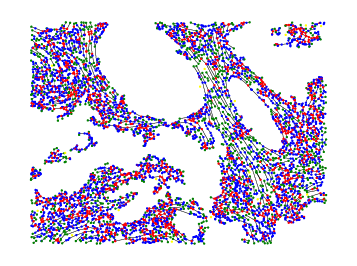
7.15 Ascidian embryo registration
Participants: Grégoire Malandain, Kevin Yep.
This work has been carried out in collaboration with Killian Biasuz and Patrick Lemaire from CRBM, Montpellier, within the ANR Cell Whisper (section 9.3.6).
It is admitted that ascidian exhibited a stereotyped development in the first stages of their embryogenesis 35. As a consequence, ascidian embryo cells can be unambiguously canonically named after their fate: this provides a common reference space into which population of individuals can be projected and compared. Moreover, this stereotypy also provides a means to name a new sequence from already named ones.
In 5 (see also Astec 6.1.1), we proposed a pipeline to segment and track the embryo cells over several generations from a temporal sequence of light-sheet microscopy 3D images. This pipeline allows to extract a number of quantitative data (lineage, cell volume, cell barycenter, cell contact surfaces, etc.) from a 3D+t image series depicting a developing embryo.
We demonstrated (data not yet published) that the development stereotypy induces cell neighborhood invariance. This allows to entirely name, from a set of atlases (ie already named series) the cells of a developing embryo, according that one time point has been named.
In 37, we have proposed a registration method between segmented embryo 3D images, that offers a first means to transfer names from an atlas to an unnamed embryo, thus to name a time point of an unnamed series. We have investigated whether this approach can be adapted to register two embryos (having the same number of cells) knowing only the quantitative data.
7.16 Segmentation of prostate organoids
Participants: Grégoire Malandain, Apolline Blachet.
This work is made in collaboration with S. Clavel and L. Bastistic (C3M, Nice) and M. Rauzi and B. Delorme (IBV, Nice), within the ANR Morpheus
The ANR Morpheus project aims at using organoids to test the toxicity of endocrine disruptors (EDCs). Within this project, we aimed at studying and quantifying the organoid growth in both normal and perturbed conditions. This requires imaging 3D+t series of developing organoids, and to extract quantitative information at the cell levels.
We have first adapted and test several imaging protocols, with the IBV's light sheet microscope. To assess imaging protocols, resulting 3D+t series were segmented using the Astec pipeline (see section 6.1.1) and segmentation results were visually evaluated (see figure 15). In addition, some Astec parameters were also assessed.
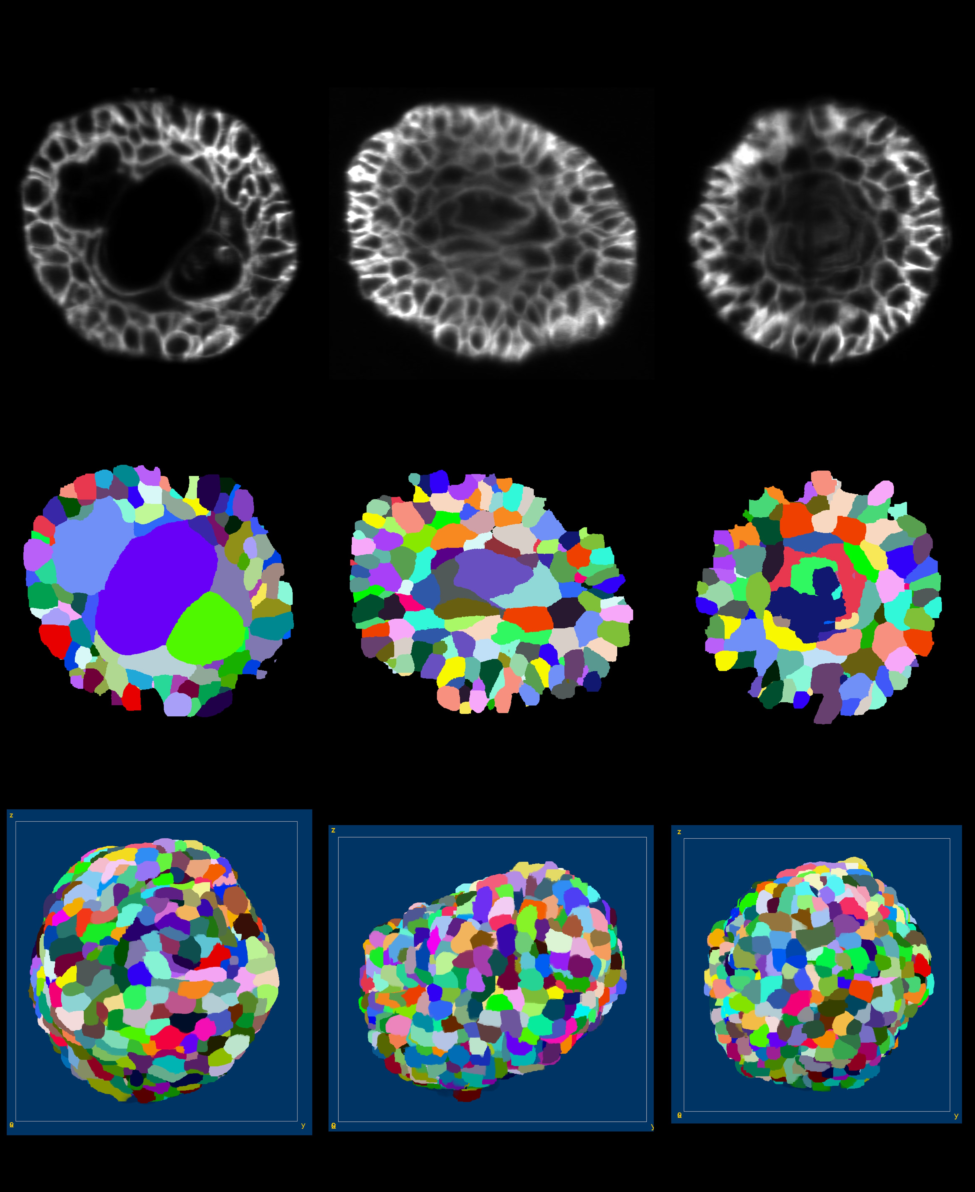
8 Bilateral contracts and grants with industry
8.1 Bilateral contracts with industry
Participants: Imane Lboukili, Xavier Descombes.
- Johnson & Johnson, Santé Beauté France, Paris: 36-month companion contract (Jan 2021 – Dec 2023) for the PhD thesis of Imane Lboukili.
9 Partnerships and cooperations
9.1 International initiatives
9.1.1 Participation in other International Programs
IEA VaMOS “Variable-Metric & inexact sparse Optimisation for Super-resolution microscopy” (VaMOS)
Participants: Luca Calatroni.
This project is in collaboration with S. Rebegoldi (University of Florence, Italy).
The purpose of this project is the design novel sparse optimisation approaches for image microscopy inverse problems. To do that, the following challenges will be addressed.
The design of new models defined in terms of tailor-made continuous and non-convex sparsity-promoting regularisations as well as non-Gaussian data terms (Poisson, Gauss + Poisson...); The design and theoretical analysis of new inexact and variable metric algorithms solving the models above and their MATLAB open-source implementation; The validation of the models and algorithms above on synthetic and real fluorescence microscopy data. The use of inexact and variable-metric approaches for imaging inverse problems has gained a lot of attention in the recent years at an international level but has not been sufficiently explored by the French imaging community. S. Bonettini (UniMoRe, Italy) and S. Rebegoldi who are experts on the topic could boost the French research activity on the topic with new ideas by establishing long-lasting collaborations.
Projet WWW-PIC (World Wide Web of Plankton Image Curation) du Belmont Forum
Participants: Cédric Dubois, Eric Debreuve.
This project is in collaboration with Laboratoire d'Océanographie de Villefranche-sur-mer, Oregon State University (USA), Universidade de São Paolo (Brésil), Japan Agency for Marine-Earth Science and Technology.
The goal is to develop a platform to collect plankton images (in the range of hundreds of millions), to enable them to be named in a consistent manner (using an automatic classifier and a manual correction interface), to store the associated ecological information (time, position...), and to make this data easily accessible. Studies on the health of ecosystems, worldwide planktonic diversity, and the contribution of plankton to carbon storage, for example, will thus be facilitated or even made possible. Funding is dedicated to equipment and missions.
9.1.2 Visits to international teams
- L. Calatroni, L. Blanc-Féraud, V. Stergiopoulou and M. Lazzaretti visited the Cambridge Image Analysis group at the University of Cambridge (UK) as part of the H2020 NoMADS RISE project.
- L. Calatroni visited the Machine Learning Genoa (Genoa, IT) as part of the H2020 NoMADS RISE project.
9.2 European initiatives
9.2.1 H2020 projects
Luca Calatroni is local PI and WP coordinator of the H2020 RISE project NoMADS on Nonlocal methods for arbitrary data sources. The project includes approximately 20 academic and industrial partners and funds research visits of minimum one month for fostering collaborations.
9.3 National initiatives
9.3.1 Labex SIGNALIFE
The Morpheme team belongs to the Labex (Laboratory of Excellence) Signalife. This Labex embeds four biology institutes in Nice, Sophia Antipolis and two Inria teams.
9.3.2 ANR JCJC TASKABILE
Participants: Luca Calatroni.
This project is in collaboration with Alessandro Lanza (University of Bologna, IT) and Thomas Moreau (Inria Saclay, FR).
In this project we propose to improve upon the intrinsic specificity and rigidity of standard bilevel learning approaches by means of a novel task-adapted highly-flexible modelling. To mitigate the computational efforts required for solving bilevel problems numerically, tailored inexact and stochastic optimisation schemes will be studied. The following questions will be addressed:
- The definition of novel, possibly non-smooth and non-convex task-adapted (not only reconstruction-based) quality measures inspired by empirical evaluation metrics and visual perception;
- The use of flexible statistical distributions as a more plausible prior model describing image statistics and their comparison with highly-generalisable deep architectures;
- The use of inexact and stochastic first-order optimisation algorithms computing solutions of bilevel schemes with reduced computational efforts and controlled accuracy.
The project features an international consolidated skilled team of researchers with strong expertise in applied imaging inverse problems, non-smooth & non-convex optimisation and statistical image processing. A PhD student, a one-year postdoc and several master students will be recruited during the project (48 months).
9.3.3 ANR PRC MICROBLIND
Participants: Luca Calatroni, Laure Blanc-Féraud.
This project is a collaborative project led by Pierre Weiss (IMT, Toulouse)[PI].
Several recent revolutions in imaging rely on numerical computations. One can think of single molecule localization microscopy (Nobel Prize 2014) or cryo-electron microscopy (Nobel Prize 2017). What they have in common is the need to perform prior mathematical modeling and calibration of the system. Although they have made it possible to observe phenomena that were previously out of reach, their expansion is currently limited by an important problem: it is difficult to precisely control the imaging conditions (e.g. temperatures, wavelengths, refractive indices). This results in modeling errors that can have disastrous repercussions on the quality of the images produced. Thus, these technologies are currently reserved for a handful of research centers possessing state-of-the-art equipment and considerable interdisciplinary experience. The objective of this project is to bring new theoretical and numerical solutions to overcome these difficulties, and then to apply them to different optical microscopes. This should allow to democratize their use, to reduce their cost and the preparation time of the experiments.
The central idea is to characterize a measurement device, not by a single operator (e.g. a convolution), but by a small dimensional family allowing to model all possible states of the system. To our knowledge, this idea has been very little explored so far and opens many difficult questions: how to evaluate this family experimentally and numerically? How to identify the state of the system from indirect noisy observations? How to exploit this information to reconstruct images in short computing times? We have begun to explore these questions in recent works and wish to continue this effort using tools from optimization, harmonic analysis, probability and statistics, algebraic geometry, machine learning and massively parallel computing. We hope to make significant advances in the field of blind inverse problems. We will validate them on photonic microscopy problems in collaboration with opticians, responsible for two microscopy platforms. This will allow us to obtain direct feedback for real problems in biology. We will particularly study the problems of super-resolution by single molecule, multi-focal localization and blind structured illumination. Moreover, several companies in the Toulouse area (INNOPSYS, IMACTIV-3D, AGENIUM), will provide us with data from their microscopes (line scanning microscope, light sheet fluorescence microscope), which will ensure direct transfers to industry.
9.3.4 ANR JCJC RUBIN-VASE
Participants: Luca Calatroni.
This project is in collaboration with Dario Prandi (L2S)[PI], Valentina Franceschi (UniPD), Laurent Perrinet (INT) and Giuseppina Turco (LLF, Paris).
In this project we will design novel variational models defined in suitable functional spaces to translate the neurobiological principle of sensory efficient representation into mathematical terms. This will be done by addressing the following three different problems:
- The modelling of neuronal processes in V1, especially through the study of hallucinatory patterns;
- The design of a neuro-inspired framework for sound processing and speech reconstruction;
- The comparison between neurobiological models and (deep) data-driven ones, notably relying on ideas borrowed from the fields of sparse sensing and predictive coding.
Our goal is to formulate new effective reference models adapted to describe both visual and auditory perceptual phenomena, overcoming the limitations associated to WC equations and L+NL models.
This project will be carried out by coupling in parallel the development of rigorous analytical theories with their numerical and experimental validation. It features a focused team of young researchers with a synergy of combined expertise in variational methods, signal processing, optimisation, control theory, sparse coding, computational neurosciences, and speech analysis. The project will last till October 2023.
9.3.5 ANR MORPHEUS
Participants: Xavier Descombes [PI], Grégoire Malandain.
In this project we propose to use the cutting-edge organoid technology to test the toxicity of endocrine disruptors (EDCs) on human organs. The aim is to develop computational tools and models to allow the use of organoid technology for EDC toxicity testing. The project is thus divided in two main objectives: to build up and analyze a phenotypic landscape of EDC effect on organoid and to develop explicative or predictive models for their growth. The first goal is to define and construct a phenotypic map of organoids, modelled as graphs (the nodes representing the cells and edges adjacency between them) for classifying EDCs families. The second is to classify organoid growth trajectories on this map. We will consider two organoid models, gastruloids and prostate organoids. To derive the phenotypic map we will combine a graph representation and a deep learning approach. The deep learning approach will be considered for its discriminating properties whereas a correspondence between the bottleneck layer of the chosen neural network and the stratified graph space will bring some explicability to the derived classification.This 4-years project started in november 2021 and is leaded by X. Descombes. It involves 3 groups : C3M (S. Clavel, Nice), Metatox, Inserm (X. Coumoul, Paris) and Morpheme.
9.3.6 ANR Cell Whisper
Participants: Grégoire Malandain.
Successful embryogenesis requires the differentiation of the correct cell types, in defined numbers and in appropriate positions. In most cases, decisions taken by individual cells are instructed by signals emitted by their neighbours. A surprisingly small set of signalling pathways is used for this purpose. The FGF/Ras/ERK pathway is one of these and mutations in some of its individual components cause a class of human developmental syndromes, the RASopathies. Our current knowledge of this pathway is, however, mostly static. We lack an integrated understanding of its spatio-temporal dynamics and we can imperfectly explain its highly non-linear response to a graded increase in input stimulus.
This systems biology project combines advanced quantitative live imaging, pharmacological/optogenetics perturbations and computational modelling to address 3 major unanswered questions, each corresponding to a specific aim:
- Aim 1: What is the spatio-temporal dynamic of intracellular signal transduction in response to FGF (Fibroblast Growth Factor) ?
- Aim 2: What is the molecular basis of the switch-like response to graded extracellular signals?
- Aim 3: Can the results be integrated into a predictive computational model of the pathway?
Through this approach, in a simplified model system, we hope to gain an integrated view of the spatio-temporal dynamics of this pathway and of its robustness to parameter variations. Participants are CRBM (Montpellier), LIRMM (Montpellier), MOSAIC (INRIA Lyon) and Morpheme.
9.3.7 Inria Large-scale initiative Naviscope
Participants: Grégoire Malandain.
This action gathers the expertise of seven Inria research teams (Aviz, Beagle, Hybrid, Morpheme, Parietal, Serpico and Mosaic) and other groups (MaIAGE, INRAE, Jouy-en-Josas and UMR 144, Institut Curie Paris) and aimed at developing original and cutting-edge visualization and navigation methods to assist scientists, enabling semi-automatic analysis, manipulation, and investigation of temporal series of multi-valued volumetric images, with a strong focus on live cell imaging and microscopy application domains. More precisely, the three following challenges will be addressed:
- Novel machine learning methods able to detect the main regions of interest, and automatic quantification of sparse sets of molecular interactions and cell processes during navigation to save memory and computational resources.
- Novel visualization methods able to encode 3D motion/deformation vectors and dynamics features with color/texture-based and non-sub-resolved representations, abstractions, and discretization, as used to show 2D motion and deformation vectors and patterns.
- Effective machine learning-driven navigation and interaction techniques for complex functional 3D+Time data enabling the analysis of sparse sets of localized intra-cellular events and cell processes (migration, division, etc.).
9.3.8 PRIME CNRS, INS2I & INEE “Transformation and destruction processes of painted images” (Imag’In)
Participants: Luca Calatroni.
This project is in collaboration with Rosa Maria Dessì (CEPAM, UCA).
This project focuses on the development of novel imaging and learning approaches for the analysis and restoration of images from cultural heritage. The particular focus of the project is the development of image reconstruction and analysis approaches able to improve the understanding of the historical and cultural processes ancient images have undergone in order to make more interpretable their historial and artistic context. As case studies, the project focuses in particular on the study of visible and invisible (IR, UV) data acquired from images of ancient damaged frescoes in the Alpine arc in some old chapels of the XIV century. For that some image reconstruction (inpainting) approaches and some data-driven classification methods are being developed for a better analysis.
9.3.9 3IA Senior chair, "Imaging for Biology"
Participants: Laure Blanc-Féraud.
Recent advances in microscope technology provide outstanding images that allow biologists to address fundamental questions. This project aims at developing new AI methods and algorithms for (i) novel acquisition setups for super resolution imaging, and (ii) extraction of valuable quantitative information from these large heterogeneous datasets. More precisely we search for biomarkers in multispectral fluorescence images of tumor tissues to predict the response of immunotherapy in head and neck cancers.9.4 Regional initiatives
9.4.1 IDEX JEDEI bi-master project: RNP_IMAGE
Participants: Florence Besse [PI], Xavier Descombes [PI], Eric Debreuve.
The goal of this project is to develop methodologies to quantitatively analyze RNP (Ribonucleoprotein) granule dynamic properties upon aging. We extract granule trajectories from time sequence images and model them with graphs embedding fusion and division events. These graphs are then classified based on these features. Participants are IBV (Fabienne De Graeve, Florence Besse) and Morpheme.10 Dissemination
10.1 Promoting scientific activities
10.1.1 Scientific events: organisation
General chair, scientific chair
- Luca Calatroni co-orgnised the first French-Italian workshop on mathematical image analysis between the French RT (ex GdR) MIA and the Italian research group on imaging (MIVA), September 12-14.
- Luca Calatroni co-organised of the winter PhD school Advanced methods for mathematical image analysis which took place during January-February 2022 in 4 teaching blocks each organised in one Italian Univeristy among: Università degli studi dell'Insubria, Università degli studi di Modena e Reggio Emilia, Università degli studi di Genova e Università degli studi di Bologna.
Member of the organizing committees
- Luca Calatroni was co-organiser (with Y. Traonmilin and P. Escande) of a mini-symposium on Non-Convex Optimization Methods for Inverse Problems in Imaging: From Theory to Applications in the occaseion of the SIAM Imaging Science conference 2022.
10.1.2 Scientific events: selection
Chair of conference program committees
- Xavier Descombes was co-chair of a session in the ReinCare workshop organized in Nice.
Reviewer
- Grégoire Malandain has been reviewer for the IEEE ISBI conference.
- Laure Blanc-Féraud has been reviewer for the IEEE ISBI, ICASSP, ICIP conferences.
10.1.3 Journal
Member of the editorial boards
- Xavier Descombes is associate editor for Digital Signal Processing.
- Luca Calatroni is member of the Editorial Board of Image Processing On Line (IPOL).
- Luca Calatroni is guest editor for the Cambridge Elements series (Cambrige University Press) on Nonlocal data interactions: Foundations and Applications and of the Inverse Problems (IOP) special issue on Optimisation and learning methods for inverse problems in microscopy.
- Laure Blanc-Féraud is Aera editor for feature articles of the IEEE Signal Processing Magazine.
- Laure Blanc-Féraud is responsible of the Image Domain of the Encyclopedia SCIENCES Istey/Wiley, 15 books in preparation.
Reviewer - reviewing activities
- Luca Calatroni has been reviewer for: Handbook of Mathematical Models and Algorithms in Computer Vision and Imaging, Journal of Mathematical Imaging and Vision, Applied Mathematical Modeling, Frontiers in Applied Mathematics and Statistics, Computational Optimization and Applications, La Matematica, Inverse Prolbems, Computer and Mathematics with Applications, Journal of Machine Learning Research.
- Eric Debreuve has been reviewer for: Digital Signal Processing (Elsevier) and Medical Image Analysis (Elsevier).
10.1.4 Invited talks, Conferences, Workshops
- Luca Calatroni gave a plenary invited talk in the occasion of the 3rd IMA conference on Inverse Problems, Edinburgh, UK, May 2022.
- Luca Calatroni gave a keynote talk in the occasion of the workshop ”Advanced Techniques in Optimization for Machine learning & Imaging” (ATOMI), Roma, IT, June 2022.
- Luca Calatroni gave an invited seminar for the Machine Learning Genoa Centre seminars, November 2022.
- Luca Calatroni gave an invited talk in mini-symposia organised in the occasion of the following international conferences: SIAM conference on Imaging Sciences 2022, Inverse Problems Modelling and Simulation 2022.
- Xavier Descombes gave invited talks in "Journée des Matériaux" in Sophia Antipolis and in the Forum Teratec in Ecole Polytechnique (Saclay).
- Vasiliki Stergiopoulou has been invited to present her work at the SIAM conference on Imaging Sciences 2022 conference, Cambridge Advanced Imaging Centre seminar, MRC Laboratory of Molecular Biology seminar. She also presented her at: workshop Mathematical models for P&P restoration, conference ISBI 2022,Journées Imagerie Optique Non Conventionnelle.
- Marta Lazzaretti has been invited to present her work at the following events: conference SIAM on Mathematics of Data Science 2022, conference AIRO 2022.
- Laure Blanc-Féraud has been invited to present her work at the following events: PICOF’22, 10th Conference on Inverse Problems, Control and Shape Optimization, Caen 25-27 October 2022 and Workshop AI for the Sciences, Mines de Paris, Sophia Antipolis, 9-10 June 2022.
- Bastien Laville has been invited to present his work at the national conference SMAI CANUM (June 2022) and Laffitte prize as finalist (Oct. 22). He also prensented his work at IEEE ICASSP (April 22) and Gretsi (Sept. 22).
- Faisal Jayousi has presented a poster at the 3IA Annual Scientific Meeting; 14-15 November 2022, INRIA Sophia Antipolis, France.
- Rudan Xiao presented a poster in REMIA 2022 - MICCAI Workshop on Resource-Efficient Medical Image Analysis on september 22th 2022.
- Imane Lboukili has presented a poster at ESDR (European Society for Dermatological Research) 2022 annual meeting on august 30th 2022, has given a talk at ISBS 2022 World Congress on Biophysics and Imaging of the Skin on march 29th 2022, has presented a poster at SPIE Photonics Europe conference in Strasbourg in 2022.
10.1.5 Leadership within the scientific community
- Luca Calatroni is the co-founder and co-director of the Italian group on imaging and vision (Gruppo MIVA) labelled by the Italian Mathematical Union (UMI).
- Grégoire Malandain is member of the IEEE Biomedical Imaging and Image Processing technical committee.
- Grégoire Malandain is member of the scientific committee of the MATHNUM department of INRAE.
- Laure Blanc-Féraud is member of the IEEE Biomedical Image and Signal Processing Technical Committee.
10.1.6 Scientific expertise
- Xavier Descombes is an MENSR expert for the CIR and JEI programs.
- Grégoire Malandain was a member of a INRAE researcher (CRCN) selection committee.
- Laure Blanc-Féraud is expert for the Italian ministery of research and for research projects in Cataluna.
10.1.7 Research administration
- Laure Blanc-Féraud is member of CAP of CNRS for chargés de recherche.
- Laure Blanc-Féraud is member of the board of the Academy "Network, Information and Digital Society" of the Idex of université Côte d'Azur.
- Laure Blanc-Féraud is member of the scientific committee of the 3IA Côte d'Azur Institute and responsible of Axis on computational biology and Bio-inspired AI.
- Xavier Descombes is member of the scientific committee of the Labex SIGNALIFE.
10.2 Teaching - Supervision - Juries
10.2.1 Teaching
- Licence: Florence Besse, Subcellular RNA targeting, 4h, L3, ENS Ulm, France
- Master: Florence Besse, Neurobiologie cellulaire et moléculaire, 6h, M1/2, Université Côte d'Azur, France
- Master: Florence Besse, Outils génétiques pour l’étude des circuits neuronaux, 4h, M1/2, Université Côte d'Azur, France
- Master: Florence Besse, Transport axonal, 2h, M1/2, Université Côte d'Azur, France
- Master: Laure Blanc-Féraud, module Machine Learning for Image processing and module Applied AI of master SD, 12h Eq. TD, Niveau M2, Université Côte d’Azur, France.
- Master: Eric Debreuve, Scientific image processing and Machine learning, Master SVS, 15h EqTD, M1 + M2, Université Côte d'Azur, France
- Master: Xavier Descombes, Traitement d’images, Analyse de données, Techniques avancées de traitement d’images, 10h EqTD, Niveau M2, ISAE, France.
- Master: Xavier Descombes, Polytech Sophia, master, 9h Eq. Université Côte d’Azur, France.
- Master: Xavier Descombes, Bio-imagerie, master IRIV, 6h EqTD, Niveau M2, Université de Strasbourg, France
- Master: Xavier Descombes, Image Analysis, master GBM, 9h EqTD, Niveau M2, Université Côte d’Azur, France.
- Master: Xavier Descombes, Scientific image processing and Machine learning, master SVS, 15h Eq.TD, Niveau M2, Université Côte d'Azur.
- Master: Xavier Descombes, Artificial Intelligence for Histopathologist, master oncology, 3h Eq. TD, Niveau M2, Université Côte d'Azur.
- Master: Alexandre Martin, Image Analysis, master GBM, 4.5h Eq.TD, Niveau M2, Université Côte d'Azur.
- Master: Alexandre Martin, Scientific image processing and Machine learning, master SVS, 10h Eq.TD Niveau M2, Université Côte d'Azur.
- Master: Luca Calatroni, Laure Blanc-Féraud, Vasiliki Stergiopoulou, Marta Lazzaretti, Inverse problems in image processing, niveau M2, 30h, MSc DSAI, UCA, France.
- PhD: Luca Calatroni, Advanced mathematical methods for image analysis, 4h, University of Modena and Reggio Emilia, Italy.
- Master: ECUE histoire de l'histoire de l'art: Luca Calatroni, Where arts & mathematics meet, niveau M1, 2h, Université Côte d'Azur, France.
- Engineer 3rd year: Cédric Dubois, Statistics and R, 26h TD, Polytech Nice Sophia, Université Côte d'Azur, France.
- MSc Data Science: Vasiliki Stergiopoulou, Machine Learning in Image Analysis (2h).
- BUT RT Signaux et Systèmes pour les transmission (R205): Vasiliki Stergiopoulou, Numérisation de l'information (R206) (in total 60h).
- Polytech L2 : Bastien Laville, Automatic, 39 hours (2nd year PeiP, equivalent to L2) Polytech Sophia Antipolis, UCA.
- Polytech L3 : Bastien Laville, mathematics, 15 hours, L3 Polytech Nice Sophia Antipolis, UCA.
10.2.2 Supervision
- Luca Calatroni, Laure Blanc-Féraud and Sebastien Schaub are supervising Vasiliki Stergiopoulou (PhD student).
- Luca Calatroni is supervising Marta Lazzaretti (PhD student) in collaboration with Claudio Estatico (University of Genova).
- Luca Calatroni is co-supervising Ilias Rentzeperis (PostDoc) working at the L2S laboratory in CentraleSupelec, in collaboration with D. Prandi (CNRS) and L. Perrinet (CNRS).
- Luca Calatroni, Laure Blanc-Féraud and Vasiliki Stergiopoulou supervised Sai Muttavarapu (Master intern).
- Luca Calatroni supervised Fabio Merizzi (Master intern) in collaboration with Elena Loli Piccolomini (University of Bologna).
- Xavier Descombes is supervising Rudan Xiao, Imane Lboukili, Alexandre Martin and co-supervising Faisal Jalousi (PhD students).
- Xavier Descombes has supervised Yueqiao Huang and co-supervised Assia Chahidi (Master 2 interns).
- Grégoire Malandain is co-supervisor of Manuel Petit’s PhD (also co-supervised by C. Godin, Mosaic, Lyon).
- Grégoire Malandain has supervised A. Blachet and K. Yep (interns).
- Eric Debreuve is supervising Cédric Dubois and co-supervising Asma Chalabi and Clara Sanchez.
- Eric Debreuve has co-supervised Assia Chahidi (Master 2 intern).
- Laure Blanc-Féraud supervises Bastien Laville with Gilles Aubert, Vasilina Stregiopoulou with Luca Calatroni and Sebastien Schaub and Faisal Jayousi with Xavier Descombes.
10.2.3 Juries
- Luca Calatroni was examiner for the PhD jury of Maeva Maulin (CEA Cadarache).
- Luca Calatroni was examiner for the PhD jury of Pasquale Cascarano (University of Bologna, Italy)
- Xavier Descombes was reviewer for the PhD jury of Jelica Vasiljevic (univ. Strasbourg) and examiner for the PhD jury of Rudan Xiao (univ. Côte d'Azur).
- Xavier Descombes is a member of the PhD CSI of Laura Claude (Centrale Supelec), Jules Mabon (Univ. Côte d'Azur) and Martina Pastorino (Univ. Côte d'Azur).
- Grégoire Malandain was reviewer for the PhD committee of H. Wu (Paris-Saclay univ.).
- Grégoire Malandain was member of the PhD committee of K. Biasuz (Montpellier univ.).
- Eric Debreuve is a member of the PhD CSI of Quentin Guimard (Univ. Côte d'Azur), Sardor Israilov (Univ. Côte d'Azur) and Miguel Romero (Univ. Côte d'Azur).
- Laure Blanc-Féraud was reviewer for the HDR committee of V. Duval (Paris-Saclay univ) and C. Herzet (Rennes univ.) and was member of the PhD committee of T. Liaudat (Paris-Saclay univ), E. Bouilhol (Bordeaux univ.), D. Brault (Clermont-Ferrand univ.), F. Zidane (UCA).
- Laure Blanc-Féraud is a member of the PhD CSI of Xavier Pic (Univ. Côte d'Azur), Marta Lazzaretti (Genova Univ.), Alice Gros (Univ. d’Aix-Marseille) and Paul Tournaire (Univ. Côte d'Azur).
10.3 Popularization
10.3.1 Articles and contents
Laure Blanc-Féraud was co-writer of a tribune in journal Le Monde on "Intelligence artificielle en médecine : l’imagerie médicale au cœur des débats" appeared on 1st of June.
10.3.2 Interventions
- Xavier Descombes was interviewed for the November 23 France 3 Côte d'Azur journal on the use of AI in medical science in the context of SophI.A Summit.
- Laure Blanc-Féraud was invited at the RFI emission "Autour de la question" on 29 June for speaking about "Le corps en image".
11 Scientific production
11.1 Major publications
-
1
articleA continuous relaxation of the constrained
problem.Journal of Mathematical Imaging and VisionJanuary 2021 - 2 articleDetecting and quantifying stress granules in tissues of multicellular organisms with the Obj.MPP analysis tool.TrafficJuly 2019
- 3 articleMultiple objects detection in biological images using a marked point process framework.Methods2016
- 4 articleFibronectin Extra Domains tune cellular responses and confer topographically distinct features to fibril networks.Journal of Cell ScienceFebruary 2021
- 5 articleContact area-dependent cell communication and the morphological invariance of ascidian embryogenesis.ScienceJuly 2020
- 6 articleCOL0RME: Super-resolution microscopy based on sparse blinking/fluctuating fluorophore localization and intensity estimation.Biological Imaging2February 2022, e1
11.2 Publications of the year
International journals
International peer-reviewed conferences
National peer-reviewed Conferences
Conferences without proceedings
Scientific book chapters
Reports & preprints
Other scientific publications
11.3 Other
Scientific popularization
11.4 Cited publications
-
34
inproceedingsResidual Whiteness Principle for Automatic Parameter Selection in
2 - 2 Image Super-Resolution Problems.SSVM 2021 - 8th International Conference on Scale Space and Variational Methods in Computer Vision12679Lecture Notes in Computer ScienceVirtual Event, FranceSpringer International PublishingMay 2021, 476-488 - 35 bookThe organization and cell-lineage of the ascidian egg / by Edwin G. Conklin..Philadelphia :[Academy of Natural Sciences],1905, 182URL: http://www.biodiversitylibrary.org/item/24005
- 36 miscAge-dependent changes in epidermal architecture explored using an automated image analysis algorithm on in vivo reflectance confocal microscopy images .
- 37 inproceedingsCell Pairings for Ascidian Embryo Registration.ISBI - International Symposium on Biomedical ImagingThe 2015 IEEE International Symposium on Biomedical Imaging proceedingsInstitute of Electrical and Electronics Engineers (IEEE). USA.New York, United StatesThe Printing House, IncApril 2015
-
38
articleFast Single Image Super-Resolution Using a New Analytical Solution for
- Problems.IEEE Transactions on Image Processingvol. 25n°August 2016, pp. 3683-3697