Keywords
Computer Science and Digital Science
- A1.1.2. Hardware accelerators (GPGPU, FPGA, etc.)
- A1.2.1. Dynamic reconfiguration
- A1.2.2. Supervision
- A1.2.3. Routing
- A1.2.4. QoS, performance evaluation
- A1.2.5. Internet of things
- A1.2.6. Sensor networks
- A1.2.7. Cyber-physical systems
- A1.2.8. Network security
- A1.3.2. Mobile distributed systems
- A1.3.3. Blockchain
- A1.3.5. Cloud
- A1.3.6. Fog, Edge
- A1.4. Ubiquitous Systems
- A1.6. Green Computing
- A2.3. Embedded and cyber-physical systems
- A2.6.1. Operating systems
- A3.1.1. Modeling, representation
- A3.1.3. Distributed data
- A3.1.8. Big data (production, storage, transfer)
- A3.1.10. Heterogeneous data
- A3.2.2. Knowledge extraction, cleaning
- A3.2.3. Inference
- A3.3.2. Data mining
- A3.3.3. Big data analysis
- A3.4.1. Supervised learning
- A3.4.2. Unsupervised learning
- A3.4.3. Reinforcement learning
- A4.4. Security of equipment and software
- A5.11.1. Human activity analysis and recognition
- A7.1. Algorithms
- A7.1.3. Graph algorithms
- A8.6. Information theory
- A8.7. Graph theory
- A8.9. Performance evaluation
- A9.2. Machine learning
- A9.9. Distributed AI, Multi-agent
Other Research Topics and Application Domains
- B4.4. Energy delivery
- B4.4.1. Smart grids
- B4.5. Energy consumption
- B5.8. Learning and training
- B6.3.2. Network protocols
- B6.3.3. Network Management
- B6.4. Internet of things
- B6.6. Embedded systems
- B7.2.1. Smart vehicles
- B8.1.2. Sensor networks for smart buildings
- B8.2. Connected city
- B8.3. Urbanism and urban planning
- B9.5.1. Computer science
1 Team members, visitors, external collaborators
Research Scientists
- Aline Carneiro Viana [Team leader, INRIA, Senior Researcher, HDR]
- Cédric Adjih [INRIA, Researcher]
- Emmanuel Baccelli [INRIA, Senior Researcher, HDR]
- Philippe Jacquet [INRIA, Senior Researcher, HDR]
Faculty Member
- Nadjib Achir [Sorbonne Paris Nord University, Associate Professor, HDR]
Post-Doctoral Fellow
- Iman Hmedoush [Inria Saclay, from May 2022 until Nov 2022]
PhD Students
- Saeed Alsabbagh [UVSQ, from Apr 2022, (co-advised with UVSQ and Algiers 1 University)]
- Najoua Benalya [CRISTAL/ENSI/Université de la Manouba (Tunisia), co-advised: ENSI, Telecom SudParis, Inria]
- Haron Calegari Fantecele [LNCC-CAPES]
- Isadora Cardoso-Pereira [Federal University of Minas Gerais - CAPES]
- Iman Hmedoush [INRIA de Paris, until May 2022, (co-advised jointly with Paul Mühlethaler)]
- Khouloud Hwerbi [CRISTAL/ENSI/Université de la Manouba (Tunisia), (co-advised: ENSI, Telecom SudParis, Inria)]
- Anne Josiane Kouam Djuigne [INRIA]
- Felix Marcoccia [Thalès, CIFRE, from Feb 2022, (co-advised with Paul Mühlethaler, AIO Team)]
- Abhishek Mishra [INRIA]
- Rosario Patane [UNIV PARIS SACLAY, from Feb 2022]
- Koen Zandberg [INRIA]
Technical Staff
- Pedro Cruz Caminha [INRIA, Engineer]
- Fernando Molano Ortiz [INRIA, Engineer, from Oct 2022]
Interns and Apprentices
- Fernando Dias De Mello Silva [Universidade Federal do Rio de Janeiro, from May 2022 until Oct 2022]
- Joao Paulo Esper Spindula [INRIA]
- Serge Makhoul [Inria, from Mar 2022 until Jun 2022, Master Student from USJ]
Administrative Assistant
- Michael Barbosa [INRIA, from May 2022]
Visiting Scientists
- Ichrak Amdouni [ENSI TUNIS, from Mar 2022 until Mar 2022, (also in June 2022)]
- Antonio Tadeu Azevedo Gomes [Laboratório Nacional de Computação Científica (LNCC), from Nov 2022]
- Chiraz Houaidia [ENSI TUNIS, from Nov 2022 until Nov 2022]
- Luca Pappalardo [ISTI-CNR, Italy, from May 2022 until Jul 2022]
- Leila Saidane [ENSI TUNIS, from Jun 2022 until Jun 2022]
External Collaborators
- Jussara Almeida [Federal University of Minas Gerais]
- Sand Correa [Federal University of Goiás]
- Antonio Ferreira Loureiro [Federal University of Minas Gerais]
- Marco Fiore [IMDE, Spain]
- Panagiota Katsikouli [University of Copenhagen = Københavns Universitet ]
- Diego Madariaga Roman [NIC Research Labs, Chile]
- Heitor Ramos [Federal University of Minas Gerais]
- Lucas Santos De Oliveira [Inria and State University of Southwestern of Bahia, Bresil, until Sep 2022]
- Kaspar Schleiser [Freie Universität Berlin]
- Alain Tchana [Laboratoire d'Informatique de Grenoble (LIG), Université Grenoble Alpes]
- Kleber Vieira Cardoso [Federal University of Goiás]
2 Overall objectives
2.1 Vision and approach
TRiBE stands for “Internet Beyond the Usual” and belongs to the Inria theme “Networks and Telecommunications” as well as contributes to the “Challenge no 11: Toward a trustworthy Internet of Everything” of the strategic plan of Inria. Building on an approach combining protocol design, data analytics, and experimental research, the research contributions of TRiBE aim at contributing to the design of smart, unified, and tactful Internet edge networks, skilled for answering applications, services, or end-users' purposes.
All the emerging IoT specificities and requirements (i.e., heterogeneity of devices and services, densification, traffic growth, ubiquitous cyber-physical context, etc) bring new demands and consequently, new scientific and technological challenges to the edge of the Internet. In this context, our conviction is that the success of the Internet of Things is rooted: in the network design's choices involving the devices, in the intelligence of the protocols and associated services as well as in the capability of reaction and adaptation of the edge-core network's communication loop.
Toward this belief, we base our approach on the combination of protocol design, data analysis, and experimental research, while meeting the requirements and challenges brought by the IoT to the edge of the Internet. Therefore, the research of TRiBE is organized around the following research directions:
- Technologies for accommodating low-end IoT devices: we tackle the optimization, simplification, and unification requirements imposed by the heterogeneity and low capabilities of low-end IoT devices. This brings the necessity to deal with limitations and to propose solutions close to hardware and software specifications.
- Technologies for leveraging high-end IoT devices’ advent: we focus on learning the behaviors of high-end IoT devices, the smart devices. More specifically, the idea is to take advantage of the “how in the spatiotemporal scale” and the “for what purpose” these devices are used. This brings the human element into play, which dynamics are shaping the way their smart devices are interacting with the edge of the Internet and, consequently, are requesting and consuming network resources and services.
-
Technologies for edge-core network interaction:This element closes the network
usability device network loop" by bringing solutions supporting functions and communication between IoT devices and the core of the Internet, while putting into practice the solutions proposed in the two previous directions.
Through these three research axes, the team places its efforts on the three main elements composing the ecosystem of IoT devices: (1) the device itself, (2) their usability, and (3) their network context. Together, these research directions will contribute to our vision toward a Smart, Unified, and Tactful Internet edge skilled for answering the application, services, or end-users’ purposes.
2.2 New challenges
The Internet has steadily evolved over the past decades from a small homogeneous to a gigantic Internet of Things (IoT) interconnecting an extremely wide variety of machines (e.g., PCs, smartphones, sensors/actuators, smart appliances, smart vehicles), and providing an extremely wide variety of services. Globally, devices and connections are growing faster than both the population and Internet users, as foreseen by Cisco. Forecasts mention an IoT market that will attain a compound annual growth rate of 28.5% from 2016 to 2020 as well as an installed base of IoT devices of over 75.4B devices by 2025. Added to these statistics is the fact that global mobile data traffic will grow nearly twice as fast as fixed IP traffic from 2017 to 2022: Smartphones account for most of this growth.
Hence, the edge of this network now consists of dense deployment of machines ranging from PCs to smartphones, from sensors/actuators to smart appliances, and from smart vehicles to diverse kinds of robots. As a consequence, humans are immersed in a highly connected and ubiquitous cyber-physical context, and as end-users of the network and its numerous services, their satisfaction has become the main focus.
In this context, the IoT is simultaneously used as a tool to gather more data, and as a means to automate more advanced control. Some businesses and institutions aim to gather more data to better understand their customers, so as to improve services. Other efforts aim to further immerse their customers into a flourishing, integrated cyber-physical environment, which can automatically and optimally adapt to their needs. All these emerging IoT-related opportunities bring new requirements and consequently, new scientific and technological challenges to the edge of the Internet.
First, the densified deployment of heterogeneous low-end IoT devices (e.g. sensors, actuators, etc.) at the edge of the Internet requires dealing with (1) the accommodation of machines with extremely limited capabilities, with a primary focus on low power requirements while (2) allowing their seamless integration in interoperable systems (often using IP as a common factor).
Second, today's pervasiveness of high-end IoT devices (e.g. smart handheld devices) distribute increasing capabilities (i.e., processing, storage, connectivity) at the edge of the network, and make our real-life and virtual activities seamlessly merge together. In this domain, we need a better understanding of: (1) when, where, and for what the high-end IoT devices are used, (2) how the uses vary among individuals, and (3) how social norms and structure dictate individuals' behavior influence the way they interact with network services and demand resources.
Related to the challenge hereabove, people's mobility and activity patterns are general in nature, and similarities emerge in different cities worldwide. The analysis of these patterns reveals many interesting properties of human mobility and activity patterns. While all these properties have been investigated at length, the COVID-19 pandemic highly perturbed our mobility patterns and use of urban spaces. This raises important questions: (1) how mobility patterns at an urban scale were affected by the pandemic; (2) can the modeling of such patterns provide a clear association with an epidemic spread, such as COVID-19 in different areas of a city?; last but not least, (3) can we still recommend safe outdoor path inside cities in order to limit the exposure to virus propagation? The 1st question answer is also closely related to the changes in “how” and “where” network resources were demanded.
The research contributions of TRiBE aim at dealing with such requirements and challenges brought to the Internet's edge. One should design adapted algorithms and communication mechanisms and network users' behavior modeling for addressing such challenges while leveraging the new technological opportunities brought by the Internet of Things.
3 Research program
3.1 Research program
Following up on the effort initiated by the team members during the last few years and building on an approach combining protocol design, data analytics, and experimental research, we propose a research program organized around three closely related objectives that are briefly described in the following.
- [Axis 1] Technologies for accommodating low-end IoT devices: The IoT is expected to gradually connect billions of low-end devices to the Internet, and thereby drastically increase communication without human source or destination. Low-end IoT devices differ starkly from high-end IoT devices in terms of resources such as energy, memory, and computational power. Projections show this divide will not fundamentally change in the future and that IoT should ultimately interconnect a dense population of devices as tiny as dust particles, feeding off ambient power sources (energy harvesting). These characteristics constrain the software and communication protocols running on low-end IoT devices: they are neither able to run a common software platform such as Linux (or its derivatives), nor the standard protocol stack based on TCP/IP. Solutions for low-end IoT devices require thus: (i)optimized communication protocols taking into account radio technology evolution and devices constrained requirements; (ii)tailored software platforms providing high-level programming, modular software updates as well as advanced support for new security and energy concentration features; (iii)unification of technologies for low-end IoT, which is too fragmented at the moment, guaranteeing integration with core or other edge networks.
- [Axis 2] Technologies for leveraging high-end IoT devices' advents: High-end IoT devices are one of the most important instances of connected devices supporting a noteworthy shift towards mobile Internet access. As our lives become more dependent on pervasive connectivity, our social patterns (as human beings in the Internet era) are nowadays being reflected from our real life onto the virtual binary world. This gives birth to two tendencies. From one side, edge networks can now be utilized as mirrors to reflect the inherent human dynamics, their context, and interests thanks to their well-organized recording and almost ubiquitous coverage. On the other side, social norms and structures dictating human behavior (e.g., interactions, mobility, interest, cultural patterns) are now directly influencing the way individuals interact with network services and demand resources or content. In particular, we observe the particularities present in human dynamics shape the way (i.e., where, when, how, or what) resources, services, and infrastructures are used at the edge of the Internet. Hence, we claim a need to digitally study high-end IoT devices' end-users behaviors and to leverage this understanding in networking solutions' design, so as to optimize network exploitation. This suggests the integration of the heterogeneity and uncertainty of behaviors in designed networking solutions. For this, useful knowledge allowing the understanding of behaviors and context of users has to be extracted and delivered out of large masses of data. Such knowledge has to be then integrated into current design practices. This brings the idea of a more tactful networking design practice where the network is assigned with the human-like capability of observation, interpretation, and reaction to daily life features and entities involving high-end IoT devices. Research activities here include: (i) the quest for meaningful data, which includes the integration of data from different sources, the need for scaling up data analysis, the usage and analysis of fine-grained datasets, or still, the completion of sparse and coarse-grained datasets; (ii) expanding edge networks' usage understanding, which concerns analysis on how and when contextual information impact network usage, fine-grained analysis of short-term mobility of individuals, or the identification of patterns of behavior and novelty-seeking of individuals; (iii) human-driven prediction models, extensible to context awareness and adapted to individuals preferences in terms of novelty, diversity, or routines. Finally, the current epidemic crises also showed a new potential impact of mobility's understanding and patterns modeling: such investigation can potentially provide a clear association with the epidemic spread (e.g., such as COVID-19) in different areas of a city.
- [Axis 3] Articulating the IoT edge with the core of the network: The edge is the interface between the IoT devices and the core network: some of the challenges encountered by IoT devices have their continuity at the edge of the network inside the gateway (i.e., interoperability, heterogeneity and mobility support). Besides, the edge should be able to support intermediary functions between devices and the rest of the core (e.g., the cloud). This includes: (i) proxying functionality, facilitating connections between devices and the Internet; (ii) machine learning enhanced IoT solutions, designed to improve the performance of advanced IoT networked systems (e.g., through methods such as supervised, unsupervised or reinforcement learning) at adapted levels of the protocol stack (e.g., for multiple access, coding, choices); (iii) IoT data contextualization, so that the collection of meaningful IoT data (i.e., right data collected at the right time) can be earlier determined closer to the data source; (iv) intermediary computation through fog or Mobile Edge Computing (MEC) models, where IoT devices can obtain computing, data storage, and communication means with lower latency in a decentralized way; or (v) security of end-to-end IoT software supply-chain, including remote management and over-the-air updates.
4 Application domains
Hereafter, we describe the general 1) domains of research of TRiBE and 2) contexts as well their applications that our solutions are applied.
- Research domains: Computer science, Mobile wireless networks, Internet of Things, Tactile Internet, Human mobility analytics and prediction, Edge smart resource allocation, IoT software design, Social network, Energy saving.
- Tactile Internet: considered the next evolution of the Internet of Things (IoT), related applications are the ones combining ultra-low latency with extremely high availability, reliability and security. They will demand smart interaction between individuals-to-devices and devices-to-devices, enabling real-time interaction in industrial, societal and business use cases. Application examples are: Automation and robotics, education and training, 3D and educational games, x-reality applications and services. Our solutions aim 1) to bring intelligence and adaptivity (to individuals' behaviors, IoT limitations, and context of services and environment) to resource allocation, management, and usability, and 2) to contribute to Tactile Internet goals.
- IoT twins: The rise of IoT will lead to the emergence of digital twins (or copies) of complex systems, manageable via 5G or further generations of digital networks. Examples of digital twins are those copying and showing digital information (i.e., relative to working/leisure areas, traffic/road/weather conditions, air quality, state of plantations/forests, etc.) of a city, a region, or even a county. Such digital copies will be highly distributed complex systems that require a solid and reactive blockchain system. Such a blockchain system is expected to manage data flows from several hundred million sensors, also scheduled to occur at very high frequency. Our solutions related to IoT-massive edges and applications, analytic learning theory, and frugal AI aim to support the development of such applications.
- Urban planning and disaster management applications: Two are the team activities bringing insights to such types of applications. Those are related to our research topics on SafeCityMap: From spatiotemporal mobility of our society to the COVID propagation understanding. and on Geometry of virus exposure, both detailed in "New Results" section. Generally speaking, mobility analytics present on these two topics, bring an understanding of the urban space usability, which can indeed be used for epidemic prevention, but also for disaster response, and urban traffic management. These analytics also have the potential to improve mobility anticipation algorithms and quality of services offered by pervasive computing applications. Another application is the provision of energy-efficient and cost-effective network infrastructures, adapted to the modified population mobility patterns during disaster situations. As a final applicability, our results are a valid indication of activity loss, and consequently, of the imposed socio-economic impact activity-labeled areas suffered during the lockdown.
- Other applications that impacted our research are also discussed in the Social and Environmental responsibility (next section).
5 Social and environmental responsibility
5.1 Footprint of research
Our research activities are not expected to impact the environment, since we work on algorithm design and software editing. Our experiments are not going beyond extremely short-scale lab experiments. The IT activities that are most likely to impact the climate are massive data stored in data centers, bitcoin mining and heavy deep learning training and we are not practicing any of them (although we plan to do some distributed machine learning for optimizing protocols).
Furthermore, we believe our research can positively impact society and the environment. This belief is due to the following ascertainments, which naturally conduct our research and our envisaged outcomes.
Assertion: The energy efficiency in the ICT and data centers sectors is considered a key part of the energy and climate targets for 2020-2030, of the European energy policies. The high energy consumption (past and forecasted future: forecasted to consume 13% of the worldwide electricity by 2030) is due not only to the in-expansion electricity needs of technological advances (e.g., data centers, new traffic demand, and connected devices) but also due to the energy-harmful over-provisioning tendency in the ICT sector.
For example, from one side, the community agrees there is a limit on how far energy-efficient data centers could go. This limitation calls for a new architectural paradigm, where Internet intelligence should move from centralized computing facilities to distributed and in-network computation. Still, the very fast-growing trend at the Internet edge (kept by the different types and capabilities of IoT devices and consequently, by their communication needs) accelerates the unprecedented proliferation of new performance-hungry IoT applications and services. Such devices will require increasing computational power and will be more power-hungry than ever.
On the other hand, considering smart devices inherit the dynamics and the decision-making of their users, mobility and heterogeneous behavior of individuals add uncertainties on where and when network resources will be needed. The standard practice in the current Internet to tackle this instability has been the any-and-anywhere extra-supplying of resources in the network. Nevertheless, in an Internet that has become essentially mobile, such over-provisioning will make energy consumption rapidly inflate, which becomes too costly and a practice that asks for revision.
TRiBE environmental responsibilities:
- TRiBE research is naturally targetting a scenario where network intelligence is pushed much closer to end-users – and consequently, to the edge of the Internet. In this sense, edge intelligence (i.e., learning, reasoning, and decision-making) will provide distributed autonomy, replacing the classical centralized structures. TRiBE results will thus contribute to (1) using a lower amount of aggregated power in dispersed locations and (2) avoiding the energy consumption related to the transmission of information back and forth to the Internet core. This conviction is the common thread in the suitable by-design solutions of the 2nd and 3rd TRiBE's axis, which will naturally contribute to the new energy-efficient architectural evolution of the Internet.
- TRiBE research pursues the conviction that methods allowing to smartly and efficiently allocate/use resources (of devices and the network) at the Internet edge are energy-friendly and contribute to the IT sector's electricity needs. This conviction is also the common thread behind the 2nd and 3rd TRiBE's axis.
- In the 1st TRiBE's axis, TRiBE goals also relate to the provision of optimized communication protocols and software solutions designed to fit the stark specificities of low-end IoT devices while taking into account radio technology evolution. The motivation here is to efficiently use and manage the billions of low-end devices expected to (i) gradually connect to and (2) drastically increase the communication, and consequently, the energy consumption, on the Internet. TRiBE's 1st research axis pursues the conviction that the smart accommodation of low-end IoT devices' related solutions will contribute to energy efficiency at the Internet edge. In a part of our research work, we focus on constrained devices (constrained in processing power and energy) and provide efficient algorithms in computation and communication reduction, both being translated into energy savings. Moreover, by making complex computations feasible on IoT devices and at the edge, we avoid inefficiencies in transmitting information back and forth.
5.2 Impact of research results
The rise of the Internet of Things will naturally lead to an increase by a significant factor: the number of connected devices. This a priori would negatively impact the environment since it would multiply the power consumption of networks. Nevertheless, one of the main IoT applications is the control of the environment by monitoring and curing critical environmental situations. Most of them would be low-powered wireless low-end devices, which are very likely powered by solar energy sources. Our research focuses (1) on the optimization and standardization of very efficient low-end networks, (2) on the power usage contention of high-end devices, and (3) on the cost limitation of creating a sensor field's digital twin by a green blockchain design. This second goal focuses on optimizing the quantity of information device-local applications should move outside the Internet edge, such as for edge machine learning.
Besides, the understanding of the way carried high-end IoT devices move and interact with one another (i.e., related to axis 2 and 3 of TRiBE) have the potential to impact epidemiology studies, urbanization investigation, and Internet provisioning (e.g., in the successful comprehension of the spread of epidemics or of the population; in urban planning; in intelligent transportation systems in smart cities; for urban space management; or in more suitable resource allocation for devices). The SafeCityMap 5 and Ariadne Covid Inria-Covid projects carried by members of the team reinforce such assertion.
In particular, the SafeCityMap project started its 2nd phase with investigations on the impact of the 1st, 2nd, and 3rd lockdown on the regular mobility habits of Paris population. Results of such investigations are periodically posted in the interactive webpage here: SafeCityMap website. Besides, we are currently investigating if the mobility modeling performed in the 1st and 2nd phase of the project, can be used as proxies at the inference of pollution and noise indicators. Preliminary investigations show a strong correlation between such indicators and SafeCityMap mobility modeling.
A sizable part of our research activities is carried on top of open-source software that we develop, and especially the open source software platform RIOT, an OS for the Internet of Things, targeting low-power embedded devices based on microcontrollers (i.e., related to axis 1 of TRiBE). Several TRiBE members contribute actively to this platform, around which a large international community has snowballed. In this way, research and developments that improve energy efficiency are made readily available to IoT practitioners, e.g. through RIOT or other software in the ecosystem.
Last but not least, another means for our research results to have an impact is through contributions to standardization (including IETF): TRiBE members co-author standards and help to define and specify efficient protocols and their optimization.
6 Highlights of the year
- Aline C. Viana was on the organization committee of the networking prospective seminar of Inria, which gathered all the related Inria research teams for discussions, prospectives, and analysis of the Network domain.
- Inria Saclay hosted the Junior Conference on DataScience and Engineering JDSE 2022 (co-organized by Inria TRiBE, C. Adjih)
- The invited research visitor Luca Pappalardo (Digicosme Invited Professor funding) gave a course of four lessons entitled ”Introduction to Human Mobility” which was organized by Aline C. Viana jointly with TPT, in the context of the master degree of IPP
- Aline C. Viana integrated the Advisory Board of the project PrepDSpace4Mobility submitted/accepted at the EU Call ”DIGITAL-2021-CLOUD-AI-01-PREP-DS-MOB – Preparatory actions for the data space for mobility”
- Emmanuel Baccelli was promoted “Directeur de Recherche” of second degree (DR2) at Inria
- Nadjib Achir became a full research member of TRiBE in January 2022, which means he is integrally attached to the team in his research activities. He is still attached to Sorbonne Paris Nord for his teaching activities.
- Nadjib Achir was selected by Inria to coordinate the activities of 13 Inria teams involved in the project E²CO (European Edge Computing Platform and Service).
7 New software and platforms
7.1 New software
7.1.1 Gardinet
-
Keyword:
Distributed networks
-
Functional Description:
Gardinet (previously DragonNet) is a generic framework for network coding in wireless networks. It is a initially result of the GETRF project of the Hipercom2 team.
It is based on intra-flow coding where the source divides the flow in a sequence of payloads of equal size (padding may be used). The design keys of DragonNet are simplicity and universality, DragonNet does not use explicit or implicit knowledge about the topology (such as the direction or distance to the source, the loss rate of the links, ...). Hence, it is perfectly suited to the most dynamic wireless networks. The protocol is distributed and requires minimal coordination. DragonNet architecture is modular, it is based on 5 building blocks (LIB, SIG, Protocol, SEW and DRAGON). Each block is almost independent. This makes DragonNet generic and hence adaptable to many application scenarios. DragonNet derives from a prior protocol called DRAGONCAST. Indeed, DragonNet shares the same principles and theoretical overview of DRAGONCAST. It enriches DRAGONCAST by the information base and signaling required to perform broadcast in wireless networks and in wireless sensor networks in particular.
- URL:
-
Contact:
Cédric Adjih
-
Participants:
Antonia Masucci, Cédric Adjih, Hana Baccouch, Ichrak Amdouni
7.1.2 SWIF-codec
-
Name:
An open-source sliding window FEC codec
-
Keyword:
Error Correction Code
-
Functional Description:
This development is done in the context of the "Coding for Efficient Network Communications" IRTF Research Group (NWCRG, [https://datatracker.ietf.org/rg/nwcrg]) and IETF hackathon.
This work has strong relationships with the Generic API I-D [https://datatracker.ietf.org/doc/draft-roca-nwcrg-generic-fec-api/] and RFC 8681 on RLC codes [https://www.rfc-editor.org/rfc/rfc8681] as examples of sliding window codes.
- URL:
-
Authors:
Vincent Roca, Cédric Adjih, Oumaima Attia, François Michel
-
Contact:
Vincent Roca
7.1.3 SimBle
-
Name:
Simulating Privacy-Preserving Real-World BLE Traces
-
Keywords:
Privacy, Simulation, Bluetooth
-
Functional Description:
SimBle is the first BLE simulation stack capable of generating traces that preserve privacy. It introduces resolvable private addresses that are the core to BLE device and network privacy-provisions. It is capable of emulating the behavior of any real BLE device/hardware. Users have to choose the appropriate device class they want to test, based on the targeted device. It resolved the lack of ground truth for scalable scenarios after the introduction of MAC address randomization.
- URL:
- Publications:
-
Contact:
Abhishek Mishra
-
Participants:
Abhishek Mishra, Aline Carneiro Viana, Nadjib Achir
7.1.4 RIOT
-
Name:
RIOT
-
Keywords:
Internet of things, Operating system, Sensors, Iot, Wireless Sensor Networks, Internet protocols
-
Scientific Description:
While requiring as low as 1,5kB of RAM and 5kB or ROM, RIOT offers real time and energy efficiency capabilities, as well as a single API (partially POSIX compliant) across heterogeneous 8-bit, 16-bit and 32-bit low-hardware. This API is developer-friendly in that it enables multi-threading, standard C and C++ application programming and the use of standard debugging tools (which was not possible so far for embedded programming). On top of this, RIOT includes several network stacks, such as a standard IPv6/6LoWPAN stack and a information-centric network stack (based on CCN).
-
Functional Description:
RIOT is an Open Source operating system that provides standard protocols for embedded systems. RIOT allows, for example, the development of applications that collect sensor data and transmit it to a central node (e.g. a server). This data can then be used for smart energy management for instance.
RIOT is specially designed for embedded systems, which are strongly constrained in memory and energy. Further, RIOT can easily be ported to different hardware devices and follows the latest evolution of IP standards.
RIOT applications can readily be tested in the FIT IoT-Lab, which provides a large-scale infrastructure facility with 3000 nodes for testing remotely small wireless devices.
- URL:
-
Contact:
Emmanuel Baccelli
-
Participants:
Emmanuel Baccelli, Koen Zandberg, Oliver Hahm, Francois-Xavier Molina, Alexandre Abadie
-
Partners:
Freie Universität Berlin, University of Hamburg
7.1.5 SIMBox Fraud Simulator
-
Name:
SIMBox fraud strategies and detection simulator
-
Keywords:
Simulation, SIMBox fraud, Fraud detection, Data analytics
-
Scientific Description:
SIMBoxFraudSimulator is an open-source simulator of SIMBox fraud strategies and detection methods in LTE networks. It is based on the well-known and broadly used LTE-SIM tool from which we added all the required components to simulate SIMBox fraud. Besides, we inserted various traffic generators and realistic mobility modeling, providing lifelike CDR data and ground-truth for comprehensive fraud detection analysis. The aim is to deploy existing detection methods of the literature for extensive evaluations to highlight their limitations and allow to propose more accurate and rapid-evolving detection approaches.
-
Functional Description:
SIMBoxFraudSimulator is an open-source simulator of SIMBox fraud strategies and detection methods in LTE networks. It is based on the well-known and broadly used LTE-SIM tool from which we added all the required components to simulate SIMBox fraud. Besides, we inserted various traffic generators and realistic mobility modeling, providing lifelike CDR data and ground-truth for comprehensive fraud detection analysis. The aim is to deploy existing detection methods of the literature for extensive evaluations to highlight their limitations and allow to propose more accurate and rapid-evolving detection approaches.
-
Release Contributions:
Deployment and configuration of 02 of the 03 most popular SIMBox architectures
- URL:
- Publications:
-
Contact:
Anne Josiane Kouam Djuigne
-
Partner:
ENS Lyon
7.2 New platforms
Open Experimental IoT Platforms
Participants: Cedric Adjih, Francois-Xavier Molina, Alexandre Abadie, Koen Zandberg, Emmanuel Baccelli.
One necessity for research in the domain of IoT is to establish and improve IoT hardware platforms and testbeds, that integrate representative scenarios (such as Smart Energy, Home Automation etc.) and follow the evolution of technology, including radio technologies, and associated experimentation tools. For that, we plan to build upon the FIT IoT-LAB federated testbeds, that we have participated in designing and deploying recently. We plan to further develop FIT IoT-LAB with more heterogeneous, up-to-date IoT hardware and radios that will provide a usable and realistic experimentation environment. The goal is to provide a tool that enables testing a validation of upcoming software platforms and network stacks targeting concrete IoT deployments.
In parallel, on the software side, IoT hardware available so far has made it uneasy for developers to build apps that run across heterogeneous hardware platforms. For instance, Linux does not scale down to small, energy- constrained devices, while microcontroller-based OS alternatives were so far rudimentary and yield a steep learning curve and lengthy development life-cycles because they do not support standard programming and debugging tools. As a result, another necessity for research in this domain is to allow the emergence of it more powerful, unifying IoT software platforms, to bridge this gap. For that, we plan to build upon RIOT, a new open source software platform that provides a portable, Linux-like API for heterogeneous IoT hardware. We plan to continue to develop the systems and network stacks aspects of RIOT, within the open source developer community currently emerging around RIOT, which we co-founded together with Freie Universitaet Berlin. The key challenge is to improve usability and add functionalities while maintaining architectural consistency and a small enough memory footprint. The goal is to provide an IoT software platform that can be used like Linux is used for less constrained machines, both (i) in the context of research and/or teaching, as well as (ii) in industrial contexts. Of course, we plan to use it ourselves for our own experimental research activities in the domain of IoT e.g., as an API to implement novel network protocols running on IoT hardware, to be tested and validated on IoT-LAB testbeds.
8 New results
8.1 Lightweight virtualization and fault isolation for small software functions on low-power IoT microcontrollers [Axis 1]
Participants: Koen Zandberg, Emmanuel Baccelli, Shenghao Yuan, Frederic Besson, JP Talpin.
Low-power operating system runtimes used on IoT microcontrollers typically provide rudimentary APIs, basic connectivity and, sometimes, a (secure) firmware update mechanism. In contrast, on less constrained hardware, networked software has entered the age of serverless, microservices and agility. With a view to bridging this gap, in the paper, we design Femto-Containers, a new middleware runtime that can be embedded on heterogeneous low-power IoT devices. Femto-Containers enable the secure deployment, execution and isolation of small virtual software functions on low-power IoT devices, over the network. We implement FemtoContainers, and provide integration in RIOT, a popular open-source IoT operating system. We then evaluate the performance of our implementation, which was formally verified for fault isolation, guaranteeing that RIOT is shielded from logic loaded and executed in a Femto-Container. Our experiments on various popular microcontroller architectures (Arm Cortex-M, ESP32 and RISC-V) show that Femto-Containers offer an attractive trade-off in terms of memory footprint overhead, energy consumption, and security
This work was published at A and A* conferences ACM MIDDLEWARE'22 31 and at CAV'22 30.
8.2 Standardization of General-Purpose Secure Software Updates for IoT Devices [Axis 1]
Participants: Koen Zandberg, Emmanuel Baccelli.
TRiBE co-authors the new IETF standard (work-in-progress) providing low-end IoT devices with secure software updates. The Internet Draft draft-ietf-suit-manifest-21 specifies a Concise Binary Object Representation (CBOR)-based Serialization Format for the Software Updates for Internet of Things (SUIT) Manifest. This specification describes the format of a manifest. A manifest is a bundle of metadata about the firmware for an IoT device, where to find the firmware, the devices to which it applies, and cryptographic information protecting the manifest. Firmware updates and secure boot both tend to use sequences of common operations, so the manifest encodes those sequences of operations, rather than declaring the metadata. The manifest also serves as a building block for secure boot.
This work is published in the IETF Internet Draft available online at draft-ietf-suit-manifest-21.
8.3 Post-Quantum Cybersecurity for Low-power IoT [Axis 1]
Participants: Koen Zandberg, Emmanuel Baccelli, Benjamin Smith, Gustavo Banegas, Adrian Herrmann.
As the Internet of Things (IoT) rolls out today to devices whose lifetime may well exceed a decade, conservative threat models should consider adversaries with access to quantum computing power. The IETF-specified SUIT standard defines a security architecture for IoT software updates, standardizing metadata and cryptographic tools – digital signatures and hash functions – to guarantee the update's legitimacy. SUIT performance has been evaluated in the pre-quantum context, but not yet in a post-quantum context. Taking the open-source implementation of SUIT available in RIOT as a case study, we survey post-quantum considerations, and quantum-resistant digital signatures in particular, focusing on low-power, microcontroller-based IoT devices with stringent memory, CPU, and energy consumption constraints. We benchmark a range of pre- and post-quantum signature schemes on a range of IoT hardware including ARM Cortex-M, RISC-V, and Espressif (ESP32), which form the bulk of modern 32-bit microcontroller architectures. Interpreting our benchmarks in the context of SUIT, we estimate the real-world impact of the transition from pre- to post-quantum signatures.
This work is published in ACNS'22 33.
8.4 Modern Random Access: Irregular Repetition Slotted Aloha (IRSA) [Axis 1]
Participants: Iman Hmedoush, Cédric Adjih, Paul Mühlethaler [Inria, EVA], Chung Shue Chen [Nokia Bell Labs], Charles Dumas [Nokia Bell Labs], Lou Salaun [Nokia Bell Labs], Kinda Khawam [UVSQ].
Wireless communications play an important part in the systems of the Internet of Things (IoT). Recently, there has been a trend towards long-range communications systems for the IoT, including cellular networks. For many use cases, such as massive machine-type communications (mMTC), performance can be gained by moving away from the classical model of connection establishment and adopting random access methods. Associated with physical layer techniques such as Successive Interference Cancellation (SIC), or Non-Orthogonal Multiple Access (NOMA), the performance of random access can be dramatically improved, giving rise to novel random access protocol designs.
In this line of work, we are studying a modern method of random access for packet networks, named “Irregular Repetition Slotted Aloha (IRSA)”, that had been recently proposed: it is based on repeating transmitted packets and on the use of successive interference cancellation at the receiver. In classical idealized settings of slotted random access protocols (where slotted ALOHA achieves 1/e), it has been shown that IRSA could asymptotically achieve the maximal throughput of 1 packet per slot.
This year, we considered that the IRSA random access, as others, can be formalized as competition and can be analyzed from a Game Theory point of view; finally, we applied more complex machine-learning techniques to IRSA (in Section 8.4.2). Most of the work had been in the context of Iman Hmedoush's Ph.D. thesis which was defended in May 2022.
8.4.1 A Game Theoretic Approach to Irregular Repetition Slotted Aloha
Participants: Iman Hmedoush, Cédric Adjih, Paul Mühlethaler, Kinda Khawam [UVSQ].
IoT networks largely rely on distributed access of billions of devices but are still lagging in terms of combined reliability and low latency. To mend that shortcoming, it is paramount to adapt existing random access methods for the IoT setting. In this line of work, we shed light on one of the modern candidates for random access protocols fitted for IoT: the “Irregular Repetition Slotted ALOHA” (IRSA). As self-managing solutions are needed to overcome the challenges of IoT, we study the IRSA random access scheme in a distributed setting where groups of users, with fixed traffic loads, are competing for ALOHA-type channel access. To that aim, we adopt a distributed game-theoretic approach where two classes of IoT devices learn autonomously their optimal IRSA protocol parameters to optimize selfishly their own effective throughput. Through extensive simulations, we assess the notable efficiency of the game-based distributed approach. We also show that our IRSA game attains the Nash equilibrium (NE) via the ”better reply” strategy, and we quantify the price of anarchy in comparison with a centralized approach. Our results imply that user competition does not fundamentally impact the performance of the IRSA protocol.
This work was published in the journal IEEE Access 16.
8.4.2 Deep Learning, Sensing-based IRSA (DS-IRSA): Learning a Sensing Protocol with Deep Reinforcement Learning
Participants: Iman Hmedoush, Cédric Adjih, Paul Mühlethaler.
Irregular Repetition Slotted Aloha (IRSA) is one candidate member of a family of random access-based protocols to solve massive connectivity problemsfor Internet of Things (IoT) networks. The key feature of this protocol is to allow users to repeat their packets multiple times in the same frame and use Successive Interference Cancellation (SIC) to decode collided packets at the receiver. Although, the plain IRSA scheme can asymptotically reach the optimal 1 [packet/slot]. But there are still many obstacles to achieving this performance, especially when considering short frame lengths. In this report, we study two new variants of IRSA with short frame length, and we optimize their performance using a Deep Reinforcement Learning approach. In our first variant, Random Codeword Selection-IRSA (RC-IRSA), we consider an IRSA approach with random codeword selection, where each codeword represents the transmission strategy of a user on the slots. We apply Deep Reinforcement Learning to optimize RC-IRSA: we train a Deep Neural Network model that chooses the slots on which the user sends its packets. Our DRL approach for RC-IRSA is a new optimization method for IRSA using a DRL approach and it works as a base for our second proposed IRSA variant DS-IRSA. Our second variant is a sensing protocol based on IRSA and trained with machine learning to synchronize the nodes during the transmission and avoid collisions. For that aim, we proposed DS-IRSA, a Deep Learning Sensing-based IRSA protocol that is composed of two phases: a sensing phase, where the nodes can sense the channel and send short jamming signals, followed by a classical IRSA transmission phase. We use a DRL algorithm to optimize its performance. Our proposed protocol has shown an excellent performance to achieve an optimal performance of almost 1 [decoded user/slot] for small frame sizes (<= 5) slots and with enough sensing duration.
This report 38 is an extension of part of the Ph.D. Thesis of Iman Hmedoush 36.
8.4.3 Connectionless Transmission in Wireless Networks (IoT) - Ph.D. Thesis
Participants: Iman Hmedoush, Cédric Adjih, Paul Mühlethaler.
The origin of the idea of adding intelligence to basic objects and making them communicate has been lost to history. But in recent times, the emergence of the Internet as a global communication network has also motivated the use of its architecture and protocols to connect objects (such as the soda vending machine famously connected to the ARPANET in the 1980s). In the past two decades, many technological enhancements have been developed to enable the “Internet of Things” (IoT). A scenario of a typical IoT network is to connect embedded devices composed of environmental sensors, microcontrollers, and communication hardware, to a central collection node. The set of data gathered by these nodes will increasingly help in analyzing and precisely understanding the phenomena and behaviors occurring in this environment. The applications of IoT technologies are endless because they are adaptable to almost any system that can provide information about its status, operation, and the environment and that one needs to monitor and control at a distance. Smart cities, healthcare, industrial automation, and wearable technology are some IoT applications that promise to make our life safer and easier. Some research and technology challenges need to be addressed for the implementation and full popularization of IoT applications including deployment, networking, security, resilience, and power control. This massive demand for connection in IoT networks will introduce new challenges in terms of connectivity, reliability, and technology. At the radio network level, IoT networks represent a huge inflow of various devices that communicate through the same shared radio medium. However, many of these devices are difficult to secure and handle. One major challenge to deploying IoT networks is the lack of efficient solutions that allow for a massive number of connections while meeting the low-latency and low-cost demands at the same time. In addition, recently, there has been a trend towards long-range communications systems for the IoT, including cellular networks. For many use cases, such as massive machine-type communications (mMTC), performance can be gained by moving away from the classical model of connection establishment and adopting grant-free, random access methods. Associated with physical layer techniques such as Successive Interference Cancellation (SIC), or Non-Orthogonal Multiple Access (NOMA), the performance of random access can be dramatically improved, giving rise to novel random access protocol designs.
In this thesis, we focus on one of the modern candidates for random access protocols “well-fitted” to the IoT: Irregular Repetition Slotted ALOHA (IRSA). As solutions are needed to overcome the challenges of IoT, we study the IRSA random access scheme from new points of view and we start with an analysis of the performance of different variations through the density evolution tool. Precisely, we start by revisiting the scenario of the IRSA protocol in the case of Multiple Packet Reception (MPR) capability at the receiver. Then, we study IRSA in different scenarios where more realistic assumptions are considered, such as IRSA with multiple powers, with capture effect, and with decoding errors. In the second part of the thesis, we concentrate on learning and dynamically adjusting IRSA protocol parameters. First, we analyze the protocol performance in a centralized approach through a variant of Reinforcement Learning and in a distributed approach through Game Theory. We also optimize short frame length IRSA through a Deep Reinforcement Learning approach. Finally, we introduce a sensing capability to IRSA, in line with carrier sense principles, and we tentatively explore how one can learn part of sensing protocols with the help of Deep Learning tools.
This thesis was defended in May 2022, and is available here: 36.
8.4.4 Breaking the Unit Throughput Barrier in Random Access Protocol-Based Distributed Systems
Participants: Parikshit Hegde, Akshit Kumar [Columbia Business School, USA], Rahul Vaze [TIFR Mumbai, India], Amira Alloum [Qualcomm, France], Cédric Adjih.
A multi-level random power transmit strategy that is used in conjunction with a random access protocol (RAP) (e.g. ALOHA) is proposed to fundamentally increase the throughput in a distributed communication network. A SIR model is considered, where a packet is decodable as long as its SIR is above a certain threshold. In a slot chosen for transmission by a RAP, a packet is transmitted with a power level chosen according to a distribution, such that multiple packets sent by different nodes can be decoded at the receiver in a single slot, by ensuring that their SIRs are above the threshold with successive interference cancelation. The achievable throughput and the upper bounds are shown to be close with the help of comprehensive simulations. The main takeaway is that the throughput of more than 1 is possible in a distributed network, by using a judicious choice of power level distribution in conjunction with a RAP.
This paper was accepted at the conference NCC 2023, Guwahati, India.
8.5 UAV Communications
8.5.1 Flying 5G Backhaul in Urban Network
Participants: Philippe Jacquet, Bernard Mans, Dalia Popescu.
We have investigated mobile networks of Unmanned Aerial Vehicles (UAVs) to extend connectivity and guarantee data rates in the 5G by analyzing possible hovering locations based on limitations such as flight time and coverage. We provide analytic bounds on the requirements in terms of connectivity extension for vehicular networks served by fixed Enhanced Mobile BroadBand (eMBB) infrastructure, where both vehicular networks and infrastructures are modeled using stochastic and fractal geometry as a model for urban environments.We prove that assuming
This work has been published in 35.
8.5.2 UAV Search Path Planning For Livestock Monitoring
Participants: Najoua Benalaya, Cédric Adjih, Anis Laouiti [IPP/Telecom SudParis], Ichrak Amdouni [ENSI Tunis/Manouba University, Tunisia], Leila Saidane [ENSI Tunis/Manouba University, Tunisia].
Unmanned Aerial Vehicles (UAVs) are being extensively deployed in numerous Livestock Management applications such as cattle disease diagnosis, counting and behavioral monitoring from videos and images captured by drones. The paper focuses on one increasingly important family of applications, UAV-assisted cattle monitoring applications where the objective is to remotely acquire some health state information from IoT nodes attached to the herding cattle. Such livestock data acquisition applications have many challenges. One of these challenges, which is the focus of this paper, is the problem that the target cattle position may not be known precisely, and might be defined with a large area. To address this issue, we design a formulation of this UAV-cattle search path problem as a mathematical optimization problem and show how it can be derived from other well-known formulations and related literature. A Mixed-Integer Linear Programming (MILP) formulation is introduced to minimize the expected search time while covering all the search area to efficiently locate the animal. This formulation exploits a cattle position probability distribution map. The results show that the suggested approach yields excellent results using the existing MILP solvers.
This article 22 was presented at PEMWN'2022.
8.5.3 A Survey on the Opportunities of Blockchain and UAVs in Agriculture
Participants: Khouloud Hwerbi [ENSI Tunis/Manouba University, Tunisia], Najoua Benalaya [ENSI Tunis/Manouba University, Tunisia], Ichrak Amdouni [ENSI Tunis/Manouba University, Tunisia], Anis Laouiti [IPP/Telecom SudParis], Cédric Adjih, Leila Saidane [ENSI Tunis/Manouba University, Tunisia].
Unmanned Aerial Vehicles (UAVs) and blockchain Technologies are relevant systems that have a significant performance in numerous sectors. In particular, applying these emerging technologies will affect positively the agricultural ecosystem. In this paper, we investigate the opportunities offered by UAVs and blockchain (BC) in the agricultural sector. We review recent research efforts on the subject with a synthesis illustrated by a classification table. Finally, open challenges and future directions for IoT-based agriculture applications are discussed
This work has been published in 24.
8.5.4 Online Drone-based Data Gathering Strategies for Ground Sensor Networks
Participants: Celia Yasmine Tazibt [University Sorbonne Paris Nord], Nadjib Achir, Tounsia Djamah [University Mouloud Mammeri, Algeria].
In recent years, we are witnessing the development of new flying equipment (also called drones or UAVs) used in various applications such as data gathering and dissemination. Indeed, drones can be used as mobile data collectors or sinks to traverse an area where wireless sensors (WSN) are deployed. The drone's objective is, then, to collect information efficiently. This work proposes two path-planning schemes for data collection in WSN using a drone flying over the sensor nodes to collect the sensed data. We assign a weight to each sensor node corresponding to its priority in the collection process. When the drone selects its destination node, it will choose the one having the highest weight. We have defined utility functions based on the sensor nodes' information disseminated in the WSN using the OLSR routing protocol. The information required to compute the nodes' weight is added to the exchanged packets during the execution of OLSR. The first proposed strategy is Data-driven Data Gathering Strategy (DDG) that uses the amount of data stored in each sensor node buffer. A priority is given to the nodes having the most significant data amount to collect. The second strategy is called Time-driven Data Gathering Strategy (TDG) where the age of the data is considered. The simulation results show that our two proposed methods provide good performances in terms of the amount of collected data based on the first strategy and help the drone collect more valid data using the second strategy.
This has been published in the International Journal of Sensor Networks 20.
8.6 5G Communications
8.6.1 A Flexible Numerology Configuration for Efficient Resource Allocation in 3GPP V2X 5G New Radio
Participants: Lam Thanh-Son Nguyen [Paris Saclay University & Sorbonne University, France], Sondes Kallel [University of Paris Saclay, France], Nadjib Aitsaadi [UVSQ Paris-Saclay & DAVID Lab, France], Cédric Adjih, Ilhem Fajjari [Orange Labs, France].
Low latency and high-reliability communications for applications' flows is one of the main 5G cellular network objectives, which is especially relevant for connected autonomous vehicles. However, efficient wireless resource allocation is complex. To address this problem in this work, we propose to adapt the physical (PHY) layer numerology configuration by fine-tuning it with Adaptive PHY Layer Configuration (APC) algorithm with the aim to maximize the Effective Transmitted Packet (ETP). Besides, we propose an adaptive scheme to maximize the expected packet serving rate while avoiding the starvation phenomenon of low-priority Logical Channels (LC). Based on extensive simulations, results show that our proposal achieves good performance in terms of ETP maximization and starvation minimization of low-priority LCs.
This article was accepted and presented at GLOBECOM 2022.
8.6.2 A Novel Radio-Aware and Adaptive Numerology Configuration in V2X 5G NR Communications
Participants: Lam Thanh-Son Nguyen [Paris Saclay University & Sorbonne University, France], Sondes Kallel [University of Paris Saclay, France], Nadjib Aitsaadi [UVSQ Paris-Saclay & DAVID Lab, France], Cédric Adjih, Ilhem Fajjari [Orange Labs, France].
As the main goal of connected autonomous vehicles' communications is to improve traffic safety and save lives, any design of a resource allocation scheme must consider the stringent requirements of these applications in terms of latency and reliability. For this, 5G cellular networks suitably address these challenges. This paper proposes a new mechanism for the telco operator to adapt the physical (PHY) layer configuration for efficient radio resource management in a 5G New Radio (NR) based system. To tackle this issue, we propose to adjust the PHY layer numerology configuration by fine-tuning it with a Radio-Aware Adaptive PHY Layer Configuration (RA-APC) algorithm in order to maximize the Effectively Transmitted Packet (ETP). Extensive simulations show that our proposal RA-APC achieves strong improvements in terms of ETP, reliability and latency while considering safety and non-safety traffic scenarios.
This article was accepted at ICC 2023.
8.6.3 A Novel AI Security Application Function of 5G Core Network for V2X C-ITS Facilities Layer
Participants: Hadi Yakan [VSQ Paris-Saclay & DAVID Lab, France], Ilhem Fajjari [Orange Labs, France], Nadjib Aitsaadi [UVSQ Paris-Saclay & DAVID Lab, France], Cédric Adjih.
5G Cellular Vehicle-to-Everything (C-V2X) is expected to become the dominant technology to enable Cooperative Intelligent Transport System (C-ITS) applications. In this paper we address the problem of detecting falsified vehicle positions sent by misbehaving vehicles targeting C-ITS application servers over 5G networks. We propose a novel security system as 5G application function. It is based on machine learning and integrated with the 5G core network to monitor, detect and prevent potential misbehavior. Based on extensive network simulations utilizing 5G network emulator, our proposal achieves very good performances, accurately reported 98
This article was accepted at ICC 2023.
8.7 On the Edge of the Deployment: A Conceptual and Practical Survey on Multi-Access Edge Computing Implementations [Axis 3]
Participants: Pedro Cruz, Aline Carneiro Viana, Nadjib Achir.
Multi-Access Edge Computing (MEC) attracts much attention from the scientific community due to its scientific, technical, and commercial implications. Still, MEC remains unfinished. In their majority, the existing MEC implementations are incomplete, which hardens or invalidates their practical deployment. As an effort to the future solutions aiming to fill this gap, it is essential to study and understand a series of experimental implementations and deployments. In this context, this work first brings a discussion on existing MEC implementations regarding the applications they target and their vision (i.e., whether they are more network-related or more distributed systems). Second, we list literature on MEC implementations according to their strategies and their consequences for the overall implementation project. We then discuss the deployment effort for each implementation. We also compare the tools developers used to make their MEC systems a reality. Finally, we discuss the issues that MEC implementations are yet to address. By bringing a better comprehension of MEC implementations, we hope this work will help developers develop their own or use MEC implementations.
This work was accepted in ACM Computing Surveys Journal 14 and published online in December 2022.
8.8 Impact of User Privacy and Mobility on Edge Offloading [Axis 3]
Participants: Esper João Paulo [Universidade Federal de Minas Gerais, Brazil], Nadjib Achir, Kleber Vieira Cardoso [Universidade Federal de Goiás, Brazil], Jussara M. Almeida [Universidade Federal de Minas Gerais, Brazil].
Offloading high-demanding applications to the edge provides better quality of experience (QoE) for users with limited hardware devices. However, to maintain a competitive QoE, infrastructure and service providers must adapt to users' different mobility patterns, which can be challenging, especially for location-based applications. Another issue that needs to be tackled is the increasing demand for user privacy protection. With less (accurate) information regarding user location, preferences, and usage patterns, forecasting the performance of offloading mechanisms becomes even more challenging. This work discusses the impacts of users' privacy and mobility when offloading to the edge. Different privacy and mobility scenarios were simulated and discussed to shed light on the trade-offs among privacy protection, mobility, and offloading performance.
This article was submitted to ICC 2023.
8.9 Energy Efficient adaptive sampling frequency of human mobility [Axis 2]
Participants: Panagiota Katsikouli, Aline Carneiro Viana, Marco Fiore, Diego Madariaga.
In this work, we aim at answering the question “at what frequency should one sample individual human movements so that they can be reconstructed from the collected samples with minimum loss of information?”.
Our analyses on fine-grained GPS trajectories from users around the world unveil (i) seemingly universal spectral properties of human mobility, and (ii) a linear scaling law of the localization error with respect to the sampling interval. Our analysis of fine-grained trajectories unveils a novel linear scaling law of the localization error with respect to the sampling interval. Such results were published at IEEE Globecom 2017 44.
Building on these results, we challenge the idea of a fixed sampling frequency and present a lightweight, energy-efficient, mobility aware adaptive location sampling mechanism. We thus present DUCTILOC, a location sampling mechanism that takes advantage of the law above to profile users and then adapt the position tracking frequency to their mobility. Our design is energy efficient, as DUCTILOC does not rely on power-hungry sensors or expensive computations; moreover, it provides a handy knob to control energy usage, by configuring the target positioning accuracy. Real-world experiments with an Android implementation of DUCTILOC show that it can effectively adjust the sampling frequency to the mobility habits of each individual and target accuracy level, reducing the energy consumption by 60% to 98% with respect to a baseline periodic sampling.
This work is under resubmission to the IEEE ACCESS journal and also registered at Hal Inria as the Research Report 8. It represents the final contribution of the collaboration with Marco Fiore, Panagiota Katsikouli, who spent 5 months in our team working as an internship in 2017, and Diego Madariaga who spent 3 months in 2018 in our team working as an internship).
8.10 Data offloading decision via mobile crowdsensing [Axis 3]
Participants: Emanuel Lima [U. of Porto], Aline Carneiro Viana, Ana Aguiar [U. of Porto], Paulo Carvalho [Univ. Do Minho].
Several studies on the analysis of human mobility patterns have been carried out focusing on the identification and characterization of important locations in users' life in general. We extended these works by studying human mobility from the perspective of mobile data offloading. In our first study, We define Offloading Regions (ORs) as areas where a commuter's mobility would enable offloading, and propose an unsupervised learning method to extract ORs from mobility traces.
Next, we leverage human mobility to inform offloading tasks, taking a data based approach leveraging granular mobility datasets from two cities: Porto and Beijing. We evaluate the offloading opportunities (ORs) provided to users while they are travelling in terms of availability, time window to offload, and offloading delay. Results show that in 50% of the trips, users spend more than 48% of the travel time inside ORs extracted according to the proposed method. Moreover, results also show that (i) attending to users mobility, ten seconds is the minimum offloading time window that can be considered; (ii) offloading predictive methods can have variable performance according to the period of the day; and (iii) per-user opportunistic decision models can determine offloading system design and performance. This work was published at ACM CHANTS 2018 (jointly with ACM MobiCom) 46. Next we extended the above work as following.
We then assess the mobility predictability in an offloading scenario using theoretical and algorithmic evaluation of several mobility predictors. The results show that mobility predictability for offloading purposes is far more challenging than mobility between PoIs. Here, machine learning (ML) predictors outperform common Markov Chain (MC) predictors used in the literature by at least 15%, revealing the importance of context information in an offloading scenario. The conclusions and findings on offloading mobility properties are likely to generalise for varied urban scenarios given the high degree of similarity between the results obtained for the two different and independently collected mobility datasets.
This last extended work is published at the IEEE Transactions on Network and Service Management 18.
This is an on-going work with the PhD Emanuel Lima, a collaboration started when he spent 4 months in 2018 as an intern in our previous team, and his advisors.
8.11 POPAyI: Muscling Ordinal Patterns for low-complex and usability-aware transportation mode detection [Axis 2]
Participants: Isadora Cardoso, Joao B. Borges, Aline Carneiro Viana, Antonio F. Loureiro, Heitor Ramos.
The comprehension of preferences related to mobility decisions of an urban population opens new perspectives to tackle the consequences of urbanization. Detecting transportation modes' usability in spatiotemporal urban trajectories enriches such mobility comprehension. With this goal, we introduce Popayi, a transportation mode detection strategy that bases its design on the Ordinal Pattern (OP) transformation applied to mobility-related time series.
Popayi can quantify time-series dynamics in linear time, muscling time series' characteristics that straightforward classification strategies can use. This new strength comes with a low-complex cost, avoiding the need for high computational and methodological complexities in the current Machine Learning (ML) and Deep Learning (DL) literature. Popayi uses polar geodesic representation and amplitude information in time series, bringing the multivariate capability to the standard uni-dimensional OP transformation.
Our experiments show that Popayi: (i) perfectly adapts to multi-dimensional mobility time series and individuals' natural non-linear mobility behavior. (ii) presents consistent detection results in any considered number of transportation mode's classes with efficiency in terms of storage and computation complexity, using fewer features than ML approaches and computational resources than DL methods. Indeed, Popayi presents classification results equivalent to DL approaches, requiring 10 to 1000 times fewer parameters. For instance, we can increase the F1-score by 2% using 1000 fewer parameters than a lightweight DL approach.
This work is currently under submission at the IEEE Transactions on Intelligent Transportation Systems.
8.12 Predicting Mobility with Small Data and Physical Principles[Axis 2]
Participants: Haron Calegari Fanticelli, Antonio Tadeu A. Gomes, Aline Carneiro Viana.
The study of human mobility is fundamental because of its impact on urban planning, the spread of diseases, the well-being of the population, and the mitigation of pollution, among other applications. Among the open challenges in the area, we have as one of the most important the interpretability and generality of the generated models, and the unbalanced volume of available data; several areas have little data available, making it impossible to use existing models.
We intend to face these challenges in a way not addressed before in the literature, which is with the use of mathematical models inspired by natural phenomena—normally modeled as differential equations—combined with established ML techniques to develop prediction models in the area of mobility. We intend with this combination to bring more interpretability to the models and reduce the need for large volumes of data.
This work is focused on the area of aggregate mobility prediction because of the data that we have to carry out this work. The available data describe the flow of people between administrative regions of Paris, France, with a sampling frequency of one hour and during fourteen days. More specifically, we intend to model the visitation routine of the people to predict the population density of areas in an instant of time, thus considering mobility and people's routine as a phenomenon to be modeled.
Our work mainly seeks to answer the following problem: Is it possible, with a good level of assertiveness, to model people's visitation routine through mathematical models combined with machine learning? Several works available in the literature show that the movements of people are typically characterized by routine behavior: daily cyclical movements (home to work), few places visited in their routine, and displacements that reveal preferred trajectories. The use of mathematical models to add domain knowledge of mobility as a phenomenon in ML techniques is new in the area and to advance this study, bringing more applicability to models, is a valuable knowledge gain for applications in urban planning or epidemiology.
We believe that, in addition to producing a generic interpretable model and requiring less training data to predict the number of people present in each of the study regions at an instant of time, our thesis will open up new opportunities for the development of mobility prediction models that consider other aspects, such as the trajectories that are expected to be taken by individuals or groups of individuals.
8.13 Revealing an inherently limiting factor in human mobility prediction: novelty-seeking mobility behavior [Axis 2]
Participants: Licia Amichi, Aline Carneiro Viana, Mark Corvella [Boston Univ.], Antonio F. Loureiro [Federal U. of Minas Gerais].
Predicting how humans move within space and time is a central topic in many scientific domains such as epidemic propagation, urban planning, and ride-sharing. However, current studies neglect individuals' preferences to explore and discover new areas. Yet, neglecting novelty-seeking activities at first glance appears to be inconsequential on the ability to understand and predict individuals' trajectories.
We claim and show the opposite in this work: exploration-like visits strongly impact mobility understanding and anticipation. Hence, we focus on such phenomenon, which has rarely been tackled in the literature but indeed, represents a real issue. We first work on the understanding of the exploration phenomenon and answer the following question: What type of visits characterize the mobility of individuals?. We show that a two-dimensional modeling of human mobility, which explicitly captures both regular and exploratory behaviors, yields a powerful characterization of users. Using such model, we identify the existence of three distinct mobility profiles with regard to the exploration phenomenon – Scouters (i.e., extreme explorers), Routiners (i.e., extreme returners), and Regulars (i.e., without extreme behavior). Our results unveil important novelty preferences of people, which are ignored by literature prediction models. We then discuss how our profiling methodology could be leveraged to improve prediction.
This first work was published at the Student workshop of ACM CONEXT 2019 and in the French Algotel conference 2020 4. An extended version was then published at the ACM SigSpatial 2020 3. A journal under submission to the IEEE Transactions on Emerging Topics in Computing, where we evaluate the impacts of novelty-seeking and the prediction task formulation on the theoretical and practical predictability extents. A technical report on this last work is available at Hal Inria 41, and 42.
Next, we claim the tackling of such limitation first requires identifying the purpose of the next probable movement. In this context, we propose a novel framework for adjusting prediction resolution when probable explorations are going to happen. In fact, the geographical occurrences of explorations are far from being random in a coarser-grained spatial resolution. Exploiting these properties, instead of directly predicting a user's next location, we design a two-step predictive framework. First, we predict the mobility purpose of an individual: (i) a return, i.e., a visit to a previously known location, or (ii) an exploration, i.e., a discovery of a new place. Next, we predict the next location or the next coarse-grained zone depending on the inferred type of movement. The results demonstrate substantial improvements in the accuracy of prediction by dint of fruitfully forecasting coarse-grained zones used for exploration activities.
This work was published at the ACM SigSpatial 2021 1.
Finally, we evaluate the impacts of novelty-seeking, quality of the data, and the prediction task formulation on the theoretical and practical predictability extents. The results show the validity of our profiling and highlight the obstructive impacts of novelty-seeking activities on the predictability of human trajectories. In particular, in the next-place prediction task, from 40% to 90% of predicted locations are wrong, notably with Scouters.
This work was accepted for publication at the IEEE Transactions on Network Science and Engineering in Dec. 2022 13.
Licia Amichi did a PhD under the supervision of Aline C. Viana. She defended in November 2021 and the final version of her manuscript is on-line since Feb. 2022.
8.14 SafeCityMap: From spatiotemporal mobility of our society to the COVID propagation understanding. [Axis 2]
Participants: Aline Carneiro Viana, Artur Ziviani [LNCC], Haron Calegari Fanteceli [LNCC,Inria], Lucas Santos De Oliveira, Razvan Stanica [Inria], Solohaja Rabenjamina [Inria].
SafeCitymap is a data-driven project investigating how individuals' mobility patterns at a metropolitan scale were affected by the Covid-19 pandemic, and especially by the harsh French lockdown conditions enforced from March 17, 2020 to May 11, 2020 (i.e., two weeks before and during the first, second, and third lockdown). For this, we used spatiotemporal aggregated mobile phone data provided by SFR, a major SFR French telecom operator, covering a geographical region focused on the city of Paris. An essential property of this data is its fine-grained spatial resolution, which, to the best of our knowledge, is unique in the COVID-related mobility literature.
We perform a data-driven mobility investigation and modeling to quantify (in space and time) the population attendance and visiting flows in different urban areas. Second, when looking at periods both before and during the lockdown periods, we quantify the consequences of mobility restrictions and decisions on an urban scale. For this, per zone, we define a so-called signature, which captures behaviors in terms of population attendance in the corresponding geographical region (i.e., their land use) and allows us automatically detect activity, residential, and outlier areas. We then study three different types of graph centrality, quantifying the importance of each zone in a time-dependent weighted graph according to the habits in the mobility of the population. Combining the three centrality measures, we compute per zone of the city, its impact-factor, and employ it to quantify the global importance of zones according to the population mobility.
Our results firstly reveal the population's daily zone preferences in terms of attendance and mobility, with a high concentration on business and touristic zones. Second, results show that the lockdown mobility restrictions significantly reduced visitation and attendance patterns on zones, mainly in central Paris, and considerably changed the mobility habits of the population. As a side effect, most zones identified as mainly having activity-related population attendance in typical periods became residential-related zones during the lockdown, turning the entire city into a residential-like area. Shorter distance displacement restrictions imposed by the lockdown increased visitation to more “local” zones, i.e., close to the population's primary residence. Decentralization was also favored by the paths preferences of the still-moving population. On the other side, “jogging activities” allowing people to be outside their residences impacted parks visitation, increasing their visitation during the lockdown. By combining the impact factor and the signatures of the zones, we notice that areas with a higher impact factor are more likely to maintain regular land use during the lockdown.
Results of such investigations are periodically posted in the interactive webpage here: SafeCityMap website. This work is published at the ACM Transactions on Spatial Algorithms and Systems 15 and a extended report is available at 43.
Currently, we are investigating if the previously described mobility modeling can be used as proxies for the inference of pollution and noise indicators in a metropolitan city. While the polution and noise depend on physical deployed sensors, mobility datasets provide more precise spatiotemporal information of larger geographical areas. Preliminary investigations show a strong correlation between such indicators and SafeCityMap mobility modeling.
8.15 Edge Learning as a Hedonic Game in LoRaWAN
Participants: Kinda Khawam, Samer Lahoud, Cedric Adjih, Serge Makhoul, Rosy Al Tawil, Steven Martin.
Federated learning provides access to more data which is paramount for constrained LoRaWAN devices with limited memory storage. Learning on a larger data set will reduce the variance of the learned model, hence reducing its error. However, federating the learning process incurs a communication cost among learning devices that must be taken into account. In this paper, we formulate a Cooperative Hedonic game and introduce a new cost function that captures both the learning error and communication cost. LoRaWAN devices engage in the devised game by identifying if they should keep their learning local or federate with other devices in order to reduce both their learning error and communication cost. We compute the optimal size of formed coalitions and assess their stability. Then, we show through extensive simulations that devices have anincentive to form learning coalitions depending on the data characteristics at hand and the communication cost of LoRaWAN.
This article was accepted at the conference ICC 2023.
8.16 Privacy and security issues in collected wireless data [Axis 2]
When performing analytics from collected data related to smart devices, privacy issues come into play that can not be ignored. Dealing with such issues is essential to allow the leveraging of any extracted data knowledge in networking solutions. In this line of work, we investigate solutions allowing the design of privacy-compliant networking solutions that take profit from individuals' wireless data.
8.16.1 Do WiFi Probe-Requests Reveal Your Trajectory?
Participants: Abhishek Kumar Mishra, Aline Carneiro Viana, Nadjib Achir.
Human mobility is challenging to infer, reconstruct, or predict precisely and even further through a more privacy-preserving and scalable manner. Domains and applications are: targeted advertising, epidemic prevention, urban, transportation, or touristic planning, to cite a few. Current GPS-based localization methods are considered sparse in space and time, and RSSI-based passive sniffing methods are challenging due to miscellaneous error sources. Recent literature has shown the large and highly volatile errors in human-location estimation when using observed RSSI from passive sniffing over Wireless packets.
In this work, we propose the first framework that introduces the concept of the user's bounded trajectory. We propose to leverage the signal strength of users' public WiFi probe requests collected from measurements of multiple deployed WiFi sniffers. First, we investigate and characterize errors in RSSI-based radial distance (between the user and each sniffer) estimation. Then, we approximate such radial distances leverage and deduce bounds associated with a user's position. Finally, we infer a user's bounded trajectory using the spatiotemporal bounds of users' locations over time. We guarantee the bounds to enclose a user in space and time, with
This work is related to the ANR MITIK project (2020-), and was accepted at WCNC 2023 29.
8.16.2 Performance Analysis of a Privacy-Preserving Frame Sniffer on a Raspberry Pi
Participants: Fernando Dias de Mello Silva [Universidade Federal do Rio de Janeiro, Brazil], Abhishek Kumar Mishra, Aline Carneiro Viana, Nadjib Achir, Anne Fladenmuller [LIP6, Sorbonne Université, Paris, France], Luís Henrique M. K. Costa [Universidade Federal do Rio de Janeiro, Brazil].
Public Wi-Fi (IEEE 802.11) networks are an abundant data source that may serve different applications such as epidemic tracking and prevention, disaster response, crowdsensing, or ubiquitous urban services. Nevertheless, collecting and exploiting such data brings many privacy liabilities, considering that each transmitted frame has the MAC address (a unique device identifier) of the corresponding personal device, also considered sensitive information. Literature has shown that the MAC randomization performed by manufacturers of phones is not enough to protect devices' identification. Data obfuscation is a promising solution to avoid storing advertised identifiers of devices and prevent attackers from acquiring sensitive data. Obfuscating such identifiers while also being able to differentiate frames sent by different devices poses a significant challenge for frame capturing by low-resource IoT devices in real time. This work illustrates the impact of on-the-fly hashing as an obfuscating measure to protect people's privacy. Since no popular off-the-shelf sniffer (using wireshark or tcpdump) allows for on-the-fly hashing, we build upon the scapy library a custom-made sniffer capable of hashing the required data needed to protect user privacy. We demonstrate the viability of this privacy-preserving IoT sniffer on a Raspberry Pi platform.
This work is related to the ANR MITIK project (2020-), and was accepted and presented at CSNET 2022 23.
8.16.3 Detecting and simulating bypass SIMBox frauds in cellular networks
Participants: Anne Josiane Kouam, Aline Carneiro Viana, Alain Tchana.
Due to their complexity and opaqueness, cellular networks have been subject to numerous attacks over the past few decades. These attacks are a real problem to telecom operators and cost them about USD 28.3 Billion annually, as reported by the Communications Fraud Control Association. SIMBox fraud, which is one of the most prevalent of these telephone frauds, is the main focus of this work. SIMBox fraud consists of diverting international calls on the VoIP network and terminating them as local calls using an off-the-shelf device, referred to as SIMBox.
In this work, we first survey both the existing literature and the major SIMBox manufacturers to provide comprehensive and analytical knowledge on SIMBox fraud, fraud strategies, fraud evolution, and fraud detection methods. We provide the necessary background on the telephone ecosystem while extensively exploring the SIMBox architecture required to understand fraud strategies. We provide a complete introductory guide for research on SIMBox fraud and stimulate interest for SIMBox fraud detection, which remains little investigated. We also present insights into tomorrow's SIMBox fraud detection challenges. This survey is published in the IEEE Communication and Tutorial Surveys journal 9 and a technical report can be found in 45.
SIMBox fraud involves diverting international cellular voice traffic from regulated routes and rerouting it as local calls in the destination country.
It has significantly affected cellular networks worldwide, generating
The FraudZen simulator is mentioned in the Software section and can be found at Inria GitLab. Related publications were published at IEEE WCNC 2023 27, at the ACM Conext Student Workshop 2022 28, and at the French Cores 2022 conference 26.
8.17 Quantum Learning Theory
Participants: Philippe Jacquet.
We have studied the theory of machine learning on the quantum physical system. One of the key issues in machine learning is the characterization of the learnability of a problem. Regret is a way to quantify learnability. Quantum tomography is a special case of machine learning where the training set is a set of quantum measurements and the ground truth is the result of these measurements, but nothing is known about the hidden quantum system. We show that in some case quantum tomography is a hard problem to learn.We consider a problem related to optical fiber communication where information is encoded in photon polarizations. We will show that the learning regret cannot decay faster than
This work has been presented in Maxnet 2022 and published in 25.
8.18 Energy Saving in Mobile Multi-Hop Sensor Networks
Participants: Bartlomiej Blaszczyszyn, Philippe Jacquet, Bernard Mans, Dalia Popescu.
We have characterized some trade-offs between the end-to-end communication delay and the energy in urban mobile communications with infrastructure assistance. Our study exploits the self-similarity of the location of communication entities in cities by modeling them with a hyperfractal model which characterizes the distribution of mobile nodes and relay nodes by a fractal dimension8.19 Information-Theoretic Study of Covid Genome
Participants: Philippe Jacquet.
The outbreak of the pandemic SARS-2 Covid 19 disease has been the major event of these two last years. There have been many speculations on the origin of the virus and about its future and pasts mutations. Therefore the genome of SARS-2 has drawn much attention. The foundation of Information Theory is to extract patterns and similarities between structures without necessarily relying on the functional significance of the common fragments, e.g. the meaning of words in texts, or the proteins translated in genomes. Nevertheless we will show that the information-theoretic tools, such as the joint complexity, are powerful enough to draw some conclusions about the recent speculations on the origin of the virus even in absence of medical specific background.We have analyzed the genome sequence of covid 19 from an information point of view and we compare it with past and present genomes. We use the powerful tool of joint complexity in order to quantify the various potential parent genomes. Our finding does not find any significant match between the Covid genome and the genome of VIH. The apparent matches which gave rise to significant turmoil in the medical community seem to be merely accidental and unavoidable due to the large database of the sequenced variant of the VIH genomes.
The working paper has been published in 39.
8.20 Precise Minimax Regret in Logistic Regression
Participants: Philippe Jacquet, Gil Shamir, Wojciech Szpankowski.
We study online logistic regression with binary labels and general feature values in which a learner sequentially tries to predict an outcome/ label based on data/ features received in rounds. Our goal is to evaluate precisely the (maximal) minimax regret which we analyze using a unique and novel combination of information-theoretic and analytic combinatorics tools such as the Fourier transform, saddle point method, and the Mellin transform in multi-dimensional settings. To be more precise, the pointwise regret of an online algorithm is defined as the (excess) loss it incurs over a constant comparator (weight vector) that is used for prediction. It depends on the feature values, label sequence, and the learning algorithm. In the maximal minimax scenario, we seek the best weights for the worst label sequence over all label distributions. For dimensionThis work has been presented at ISIT 2022 and published in 32.
8.21 Theoretical Study of Depth First Search in Random Digraph
Participants: Philippe Jacquet, Svante Janson.
The depth-first search is one of the most used algorithms in computer science. We present the analysis of the depth-first search algorithm in a random digraph model with geometric degree distributions. This problem posed by Don Knuth in his next to appear volume of The Art of Computer Programming gives an interesting insight into one of the most elegant and efficient algorithms for graph analysis due to Tarjan. The depth-first search algorithm may be useful to model the propagation of disease or information in a dynamic graph.This work has been presented in AofA 2022 and is published in 34.
8.22 TinyML and Edge AI
Participants: Cédric Adjih, Nadjib Achir, Emmanuel Baccelli, Anis Laouiti [IPP/Telecom SudParis], Wassim Seifeddine.
This year, work continued on the topic of TinyML and EdgeAI. In particular, we continued to work on the novel technique for embedded IoT systems that uses support from edge or cloud servers, and we propose a hierarchical execution model as shown in the following figure, and published in proposed in 11.
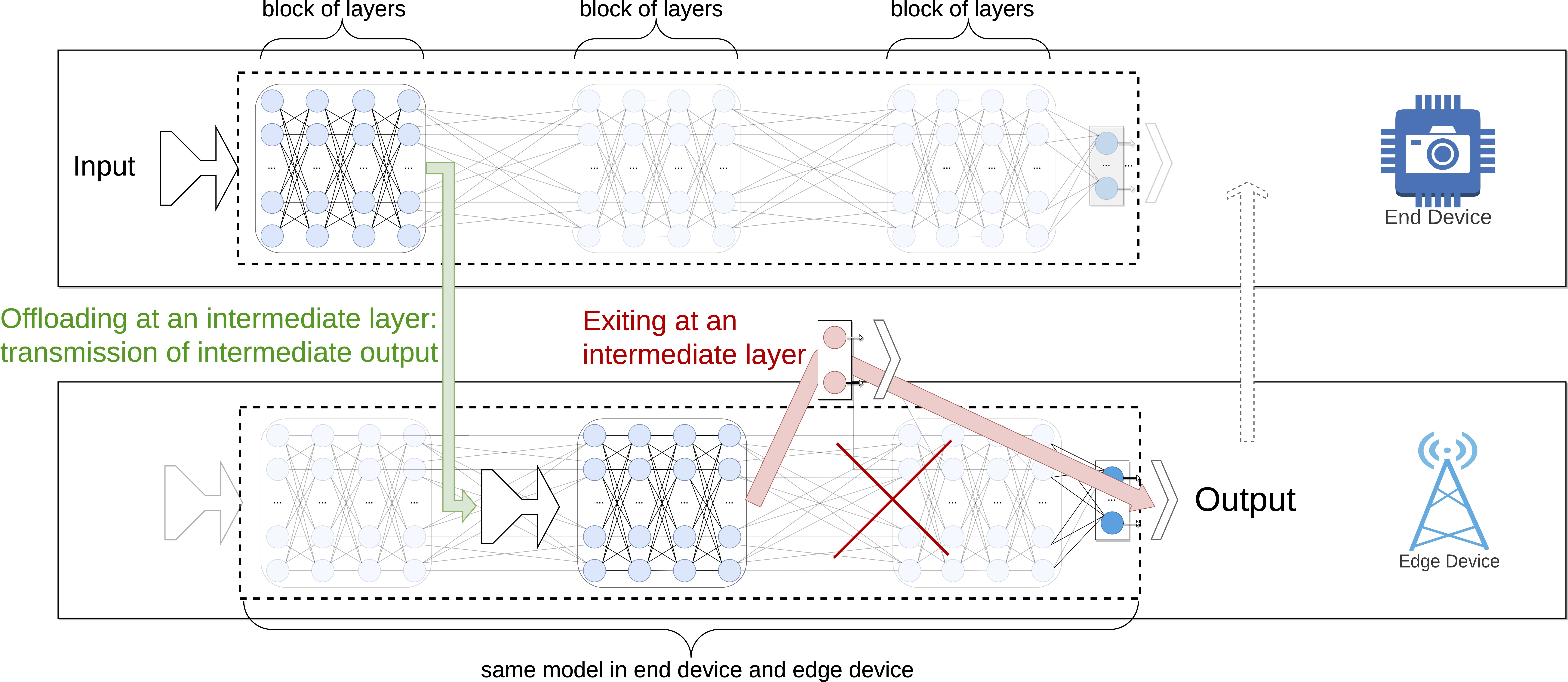
- "Embedded AI for Empty Places Recognition in Parking Lots", internship, Hong Jowell (with UTP and Telecom Sud-Paris)
- "Embedded AI for Drone Swarm Identification", internship, Nik Ahmad Syakir Nik Adib (with UTP and Telecom Sud-Paris)
Finally, a keynote was given on Machine Learning for IoT in Oct. 2022.
9 Bilateral contracts and grants with industry
9.1 Bilateral grants with industry
Nokia (ADR): Network Information Theory
Participants: Cedric Adjih, Iman Hmedoush.
Through the common Inria-Nokia laboratory, the team was involved in the action "Network Information Theory" (ADR, "Action De Recherche") that concluded with Iman Hmedoush's defense. In collaboration with Nokia, Inria EPI MARACAS, and EPI EVA, we had been working on the subject of optimization and evaluating communications for IoT networks. This includes 5G and beyond, medium-access level/random access techniques protocols, modern random access, and applying machine learning techniques to wireless communications.
Thalès - CIFRE Thesis
Participants: Cedric Adjih, Paul Muhlethaler, Felix Marcoccia.
Felix Marcoccia is a CIFRE student at Thalès, co-advised at Inria by P. Mühlethaler and C.Adjih, on the subject of: "Study of MANET Solutions for a Radio Communication System Based on Artificial Intelligence Algorithms"10 Partnerships and cooperations
10.1 International initiatives
10.1.1 Inria associate team not involved in an IIL or an international program
MLNS2
-
Title:
Machine Learning, Network, System and Security
-
Duration:
2021 ->
-
Coordinators:
David Bromberg, (French side: University of Rennes 1, Inria WIDE-IRISA) and Thomas B. Bouetou (Cameroonian side: ENSPY)
-
Partners:
- University of Rennes 1, France
- WIDE-IRISA, TRiBE (INRIA), France
- ENS-Lyon, France
- ENSPY (Cameroonian)
-
Inria contact:
David Bromberg
- website:
-
Summary:
The MLNS2 (Machine Learning, Network, System and Security) is an Inria International Team involving three Inria teams (AVALON, WIDE, and TRiBE), the ENS Lyon (LIP), and the Université of Yaoundé I (Cameroon). Smartphones become the most natural tool for people to communicate, and as such, it involves more than five billion people around the globe. As a consequence, cellular networks are an infrastructure of prime importance, so does the software ecosystem related to smartphones. In this context, the aim of this collaboration is to adequately design and investigate efficient approaches to fight against simbox frauds and malware proliferation. Addressing such challenges require multidisciplinary knowledge such as Machine Learning, Network, System, and Security (MLNS2). In this direction, we focus on two major challenges: Attacks at the infrastructure level and Attacks at the software ecosystem level.
MAGICO
-
Title:
Machine-Learning Enabled Next-Generation IoT Communications
-
Duration:
2022 ->
-
Coordinator:
Sanjeev Sharma (IIT BHU Varanasi) and Kuntal Deka (IIT Guwahati)
-
Partners:
- Indian Institute of Technology, Varanasi ITT (BHU) and Indian Institute of Technology, Guwahati (India)
-
Inria contact:
Cédric Adjih
- Website:
-
Summary:
The proposed Associated Team is focusing on modern communications for the Internet of Things (IoT), for 5G and beyond. Traditionally the wireless network systems have been designed in "layers" (e.g. OSI Layers). This is especially true for cellular communications, the main focus of this proposition. Designing next-generation communications for IoT requires revisiting this separation of layers, for some important use-cases, such as Industrial IoT and/or massive Machine Type Communications, and this will be the key of this project. We rely on the expertise of both teams. In addition, Machine Learning techniques are methods of prime interest to improve the performance of these communication methods. Specifically, we will devise an architecture for IoT communications that is based on a mix of the principles of the methods "Irregular Repetition Slotted ALOHA" (IRSA), of the methods of type "Non-Orthogonal Multiple Access" (NOMA), in particular, Sparse-Code Multiple Access (SCMA) - their principles are similar, and each partner of the Associated Team has expertise in one, or several of these. We will provide novel, efficient, variants of these protocols. Another important contribution of the project will be the addition of "sensing" of the active devices, which is extremely important in IoT scenarios; it is well-known in non-cellular networks, but it is much less explored in cellular networks, as it was unnecessary for non-IoT communications. We intend to develop machine learning-based, compressive-sensing-based, and group-testing-based methods for sensing in SA. Furthermore, the pattern of transmission of the devices (the codewords of SCMA) can be designed and assigned to the users dynamically using deep-learning-based methods. Finally, the proposed algorithms and methods will be implemented and demonstrated in an IoT testbed.
PSAR
-
Title:
Physical Security Applied to (R)IOT
-
Duration:
2020 ->
-
Coordinator:
Schiller Jochen (Jochen.Schiller@fu-berlin.de)
-
Partners:
- Freie Universität Berlin Berlin (Allemagne)
-
Inria contact:
Emmanuel Baccelli
-
Summary:
The PSAR associate team supports and develops a collaboration between Freie Universität Berlin and Inria Saclay (team TRiBE) in the domain of cybersecurity for the Internet of Things. The work aims to complement the security mechanisms tackled in the Inria Project lab RIOT-fp. RIOT-fp focuses on enhancing the security of IoT devices running RIOT via (1) lower-memory, high-speed, high-security IoT crypto primitives, (2) guarantees for software execution on low-end IoT devices, and (3) enabling secure IoT software updates, over low-power IP protocols. PSAR focuses on complementary schemes exploiting physical characteristics to secure IoT devices running RIOT, namely (i) Physically Unclonable Functions for IoT device authentication, (ii) exploiting the physical network layer for secure communication bootstrapping, and (iii) holistic approaches and mechanisms enabling, remote, periodic "health checks" for IoT deployments that are up and running.
10.1.2 STIC/MATH/CLIMAT AmSud projects
LINT
-
Title:
Leveraging federated mobility learning for tactile Internet services
-
Duration:
2022 ->
-
Coordinator:
Nadjib Achir (INRIA)
-
Partners:
- Universidad del Desarrollo, Santiago de Chile, Chile
- Universidade Federal do Rio de Janeiro, Rio de Janeiro, Brazil
- Universidad de Buenos Aires, Buenos Aires, Argentina
- INRIA Saclay, France
- Universidade Federal de Minas Gerais, Brazil
- Universidade Federal de Goiás, Brazil
- University Sorbonne Paris Nord, France
-
Inria contact:
Nadjib Achir
-
Summary:
The Tactile Internet requires ultra-low latency and high availability of cloud-like resources. Multi-Access Edge Computing (MEC) addresses this requirement, serving users with MEC hosts nearby. Nevertheless, user mobility hinders this strategy, increasing the host-user distance over time. LINT (Leveraging federated mobility learning for Tactile Internet's services) aims to predict user trajectories with federated learning, using them to optimally allocate MEC resources, while preserving user privacy.
10.1.3 Participation in other International Programs
PHC-Utique 2021 - PARADICE (21G1116)
-
Title:
Precision Agriculture in the Era of Drones and Artificial Intelligence
-
Partner Institution(s):
- Laboratoire CRISTAL, ENSI (Ecole Nationale des Sciences de l'Informatique), Tunisia
- Telecom SudParis, IPP, France
- Inria Saclay, France
-
Date/Duration:
Started in 2021
10.2 International research visitors
10.2.1 Visits of international scientists
Other international visits to the team
- Chiraz Houaidia
-
Status:Researcher
-
Institution of origin:ENSI
-
Country:Tunisia
-
Dates:31 oct.-5 nov. 2022
-
Context of the visit:progress on the Project "Precision Agriculture in the era of Drones and Artificial Intelligence"
-
Mobility program/type of mobility:PHC Utique PARADICE (21G1116)
-
Status:
- Leila Saidane and Ichrak Amdouni
-
Status:Researcher
-
Institution of origin:ENSI
-
Country:Tunisia
-
Dates:22-26 jun. 2022 and 22 jun.-6 jul 2022 (resp.)
-
Context of the visit:progress on the Project "Precision Agriculture in the era of Drones and Artificial Intelligence"
-
Mobility program/type of mobility:PHC Utique PARADICE (21G1116)
-
Status:
- Lucca Pappalardo
-
Status:Researcher
-
Institution of origin:ISTI-CNR (Institute of Information Science and Technologies - Italian National Research Council)
-
Country:Tunisia
-
Dates:May 2022 to Jul 2022
-
Context of the visit:progress on collaboration realted to ”Generalized predictability measure for human mobility accounting for spatial, temporal, and sequential dimensions – GenPM-STD”
-
Mobility program/type of mobility:Digicosme Invited Professors
-
Status:
- Antonio Tadeu A. Gomes
-
Status:Researcher
-
Institution of origin:Laboratório Nacional de Computação Científica (LNCC)
-
Country:Tunisia
-
Dates:2 weeks (Nov-Dec 2022)
-
Context of the visit:progress on collaboration realted to ”On the Interplay of Mathematical Models and Machine Learning in Mobility Prediction” also concerning the advising of Haron C. F.
-
Mobility program/type of mobility:Inria Saclay funding for invited researchers
-
Status:
10.3 European initiatives
10.3.1 H2020 projects
SPARTA
SPARTA project on cordis.europa.eu
-
Title:
Strategic programs for advanced research and technology in Europe
-
Duration:
From February 1, 2019 to June 30, 2022
-
Partners:
- Institut National de Recherche EN Informatique et Automatique (Inria), France
- Cesnet Zajmove Sdruzeni Pravnickych Osob (CESNET), Czechia
- Joanneum Research Forschungsgesellschaft Mbh (Joanneum Research), Austria
- Naukowa I Akademicka Siec Komputerowa - Panstwowy Instytut Badawczy (NASK), Poland
- Tartu Ulikool (University of Tartu), Estonia
- Mykolo Romerio Universitetas (Mykolaromeris University), Lithuania
- Latvijas Mobilais Telefons SIA, Latvia
- Security Made in Letzebuerg (SMILE), Luxembourg
- Fraunhofer Gesellschaft Zur Forderung Der Angewandten Forschung EV (FHG), Germany
- Fundacion Tecnalia Research & Innovation (TECNALIA), Spain
- Technische Universitaet Muenchen (TUM), Germany
- Thales SIX GTS France SAS (THALES SIX GTS France), France
- Commissariat a l'Energie Atomique et aux energies alternatives (CEA), France
- Stowarzyszenie Polska Platforma Bezpieczenstwa Wewnetrznego (PPBW), Poland
- Institut National Des Sciences Appliquees De Lyon (INSA LYON), France
- SAP SE, Germany
- FORTISS GMBH, Germany
- Luxembourg Institute Of Science And Technology (LIST), Luxembourg
- Vysoke Uceni Technicke V Brne (Brno University Of Technology), Czechia
- Fundacion Centro De Tecnologias De Interaccion Visual Y Comunicaciones VICOMTECH (VICOM), Spain
- Indra Sistemas SA (INDRA), Spain
- Institut Mines-TELECOM, France
- Rheinische Friedrich-Wilhelms-Universitat Bonn, Germany
- Universite Du Luxembourg (uni.lu), Luxembourg
- Consiglio Nazionale Delle Ricerche (CNR), Italy
- National Center For Scientific Research "DEMOKRITOS" (NCSR D), Greece
- Lietuvos Kibernetiniu Nusikaltimu Kompetenciju Ir Tyrimu Centras (Lithuanian Cybercrime Center Of Excellence For Training Research & educatio), Lithuania
- Kentro Meleton Asfaleias (center For Security Studies Centre D'etudes De Securite), Greece
- Indra Factoria Tecnologica SL, Spain
- Universitat Konstanz (UKON), Germany
- Leonardo - societa Per Azioni (LEONARDO), Italy
- Kauno Technologijos Universitetas (university Of Technology, KAUNAS), Lithuania
- Technikon Forschungs- Und Planungsgesellschaft MBH (TECHNIKON), Austria
- ITTI SP ZOO (ITTI), Poland
- Direzione Generale Per Le Tecnologie Delle Comunicazioni E la Sicurezza Informatica - istituto Superiore Delle Comunicazioni E delle Tecnologie Dell'Informazione (DG TCSI-ISCOM), Italy
- Generolo Jono Zemaicio Lietuvos Karo Akademija (general Jonas Zemaitismilitary Academy Of Lithuania), Lithuania
- Fundacio Eurecat (EURECAT), Spain
- Consorzio Nazionale Interuniversitario Per Le Telecomunicazioni (CNIT), Italy
- CentraleSupelec (CentraleSupélec), France
- YES WE HACK (YWH), France
- Instituto Superior Tecnico (IST), Portugal
- Secretariat General De La Defense Et De La Securite Nationale (SGDSN), France
- Universite De Namur Asbl (UNamur), Belgium
- Inov Instituto De Engenharia De Sistemas E Computadores Inovacao (INOV), Portugal
- Centre d'Excellence en Technologies de l'Information et de la Communication (CETIC), Belgium
- CZ.NIC, ZSPO (CZ.NIC), Czechia
- Consorzio Interuniversitario Nazionale per L'Informatica (CINI), Italy
-
Inria contact:
Thomas Jensen
-
Coordinator:
Florent Kirchner, CEA
-
Summary:
In the domain of Cybersecurity Research and innovation, European scientists hold pioneering positions in fields such as cryptography, formal methods, or secure components. Yet this excellence on focused domains does not translate into larger-scale, system-level advantages. Too often, scattered and small teams fall short of critical mass capabilities, despite demonstrating world-class talent and results. Europe’s strength is in its diversity, but that strength is only materialised if we cooperate, combine, and develop common lines of research. Given today’s societal challenges, this has become more than an advantage – an urgent necessity. Various approaches are being developed to enhance collaboration at many levels. Europe’s framework programs have sprung projects in cybersecurity over the past thirty years, encouraging international cooperation and funding support actions. More recently, the Cybersecurity PPP has brought together public institutions and industrial actors around common roadmaps and projects. While encouraging, these efforts have highlighted the need to break the mould, to step up investments and intensify coordination. The SPARTA proposal brings together a unique set of actors at the intersection of scientific excellence, technological innovation, and societal sciences in cybersecurity. Strongly guided by concrete and risky challenges, it will setup unique collaboration means, leading the way in building transformative capabilities and forming world-leading expertise centres. Through innovative governance, ambitious demonstration cases, and active community engagement, SPARTA aims at re-thinking the way cybersecurity research is performed in Europe across domains and expertise, from foundations to applications, in academia and industry.
10.4 National initiatives
Equipex FIT:
Participants: Cedric Adjih, Alexandre Abadie [Inria, SED], Emmanuel Baccelli.
- Partners: Sorbonne Université, Inria (Lille, Sophia-Antipolis, Grenoble), INSA, Institut Telecom Paris, Institut Télécom Evry, LSIIT Strasbourg.
-
Abstract: FIT (Future Internet of Things) aims to develop an experimental facility, a federated and competitive infrastructure with international visibility and a broad panel of customers. It provides this facility with a set of complementary components that enable experimentation on innovative services for academic and industrial users. The project gives french internet stakeholders a means to experiment on mobile wireless communications at the network and application layers thereby accelerating the design of advanced networking technologies for the future internet. FIT was one of 52 winning projects from the first wave of the French Ministry of Higher Education and Research's "Équipements d'Excellence" (Equipex) research grant program, in 2011.
One component of the FIT platform is the sets of IoT-LAB testbeds (see the IoT-LAB web site). These were motivated by the observation that the world is moving towards an “Internet of Things”, in which most communication over networks will be between objects rather than people.
Project 5G-mMTC:
Participants: Cedric Adjih, Alexandre Abadie [Inria, SED], Nadjib Achir, Emmanuel Baccelli.
- Funding instrument: AAP - Plan de relance « Souveraineté dans les réseaux de télécommunications afin d'accélérer les applications de la 5G » (France Relance)
- Project acronym: 5G-mMTC
- Duration: 2021–2024
- Partners: Amarisoft, EDF R&D, Fédération francaise de cyclisme, Inria Saclay, Institut Mines Telecom, IS2T, Sequans communications, Sparkling Tech, Université de Versailles (UVSQ Paris-Saclay), Webdyn
- Abstract: The 5G-mMTC project aims to provide software and hardware tools for the rapid implementation of a 5G solutions for the IoT. Two use cases will be implemented directly within the framework of this project: one developed in conjunction with the French Cycling Federation (FFC), which will enable real-time analysis of athletes' data and their performances; the other will be worked on jointly with EDF, to enable real-time management of the entire fleet of existing heterogeneous sensors
- Website: 5g-mMTC web site
Inria Challenge on Federated Learning FedMalin:
Participants: Cedric Adjih, Aline Carneiro Viana, Nadjib Achir.
- Partners: Inria Teams (ARGO, COATI, COMET, EPIONE, MAGNET, MARACAS, NEO, SPIRALS, TRIBE, WIDE).
- Abstract: FedMalin is a research project that spans 10 Inria research teams and aims to push FL research and concrete use-cases through a multidisciplinary consortium involving expertise in ML, distributed systems, privacy and security, networks, and medicine. We propose to address a number of challenges that arise when FL is deployed over the Internet, including privacy & fairness, energy consumption, personalization, and location/time dependencies. FedMalin will also contribute to the development of open-source tools for FL experimentation and real-world deployments, and use them for concrete applications in medicine and crowdsensing. The FedMalin Inria Challenge is supported by Groupe La Poste, sponsor of the Inria Foundation.
10.4.1 ANR
MITIK
Participants: Aline Carneiro Viana, Nadjib Achir, Abhishek Mishra, Catuscia Palamidessi.
- Funding instrument/scientific committee: PRC/CE25
- Project acronym: MITIK
- Project title: Mobility and contact traces from non-intrusive passive measurements
- Duration: 2020–2024
- Coordinator: Aline Carneiro Viana
- Other partners: COMETE/Inria, Universite de la Rochelle, Sorbonne Universite (UPMC).
- ANR MITIK website
- Abstract: The MITIK project is a 42-month ANR project that will start in February 2020. Mitik's primary objective is the design of an entirely new methodology to help the community obtain real wireless contact traces that are non-intrusive, representative, and independent of third parties. The secondary outcome of the project is be the public release of (1) the measurement tool designed for the easy contact gathering task; (2) contact traces which are clean, processed, and privacy-preserving, i.e., protecting both the anonymity and the location privacy of the users; and (3) their spatiotemporal statistical analysis. We expect that Miti's outcomes will support non-biased research on the modeling as well as on the leveraging of wireless contact patterns.
10.5 Regional initiatives
AI for More Accessible Cities (AI-4-MAC)
Participants: Aline Carneiro Viana, Andrea Araldo.
- Funding instrument/scientific committee: H!Paris
- Project acronym: AI-4-MAC
- Project title: AI for More Accessible Cities
- Duration: 2022–2024
- Coordinator: Andrea Araldo
- Other partners: TRiBE-Inria
- Abstract: In AI4MAC we apply Reinforcement Learning over Graphs to guide the design of future multi-modal public transit in big urban conurbations. We study the impact of such a novel design on human mobility combining data-driven analysis and agent-based simulation.
11 Dissemination
Cédric Adjih , Emmanuel Baccelli , Nadjib Achir , Philippe Jacquet , Aline Carneiro Viana .
11.1 Promoting scientific activities
11.1.1 Scientific events: organisation
- Cédric Adjih co-organized and hosted the Junior Conference on DataScience and Engineering 2022 (JDSE 2022) at Inria Saclay, Turing Building.
11.1.2 Scientific events: selection
Member of the conference program committees
- Cédric Adjih: PEMWN 2022, ICC 2023, WINCOM 2022
- Emmanuel Baccelli: PEMWN 2022, RIOT Summit 2022
- Nadjib Achir: PEMWN 2022, Globecom 2022,
- Aline C. Viana: Algotel 2022, IEEE WoWMon 2023.
11.1.3 Journal
Member of the editorial boards
- Aline C. Viana:
- (since Feb. 2018) Editorial Board member of Urban Computing Spring book series.
- (since May 2014) Area editor of ACM SIGCOMM Computer Communication Review – ACM CCR.
Reviewer - reviewing activities
- Nadjib Achir: Computer Networks, IEEE Transactions on Vehicular Technology
11.1.4 Invited talks
- Cédric Adjih gave a keynote on Machine Learning for IoT at the Workshop on IoT and Emerging Technologies IoT&ET, Sousse, Tunisia, Oct 2022.
- Aline C. Viana gave a keynote at the CS workshop at Polytechnic School of Yaounde on Habits in behaviors: human beauty and vulnerability.
11.1.5 Leadership within the scientific community
- Aline C. Viana: is the coordinator of ANR MITIk (since 2020-) and SafeCityMap Inria-Covid mission project (since 2020-);
11.1.6 Scientific expertise
- Aline C. Viana integrated the Advisory Board of the project PrepDSpace4Mobility submitted/accepted at the EU Call ”DIGITAL-2021-CLOUD-AI-01-PREP-DS-MOB – Preparatory actions for the data space for mobility”
11.1.7 Research administration
- Aline C. Viana was on the organization committee of the networking prospective seminar of Inria 2022, which gathered all the related Inria research teams for discussions, prospectives, and analysis of the Network domain.
- Aline C. Viana: (1) the leader of the TRiBE Project-Team of Inria since its creation (Jul.2019); She was the leader of the previous INFINE project-tem for one year 2018-2019; (2) the coordinator of ANR MITIk (since 2020-) and SafeCityMap Inria-Covid mission project (since 2020-); (3) Member of the CE from 2020-2023; (4) member of the BCEP from 2014-2023)
11.2 Teaching - Supervision - Juries
11.2.1 Teaching
- Master : Emmanuel Baccelli, “IoT & Security”, summer semester seminar (April 2022 - June 2022), Freie Universitaet Berlin.
- Master : Emmanuel Baccelli, “Operating Systems for Small Connected Devices in the Internet of Things”, cours magistral, Formation PESTO Corps des Mines, Telecom ParisTech, Paris France. Sept. 2022.
- Nadjib Achir is associate professor at the Sorbonne Paris Nord University and do his full service at the university (Engineering School).
- Engineering School: Cédric Adjih, “Internet of Things”, 12h lab sessions in 2022, ENSEA.
11.2.2 Supervision
- PhDs supervision
- PhD, defended on 18 May 2022: Iman Hmedoush, “Connection protocols for the 5G IoT”. Advisors: C. Adjih and Paul Mühlethaler.
- PhD in progress: Saeed Alsabbagh (UVSQ), “Security of V2X Communications in 5g networks”, since Sep. 2022. Advisors: N. Aitsaadi, C. Adjih and A. Adouane.
- PhD in progress: Najoua Benalya (ENSI), “Agriculture de précision dans l'ère des drones et d'intelligence artificielle”, since Nov. 2021. Advisors: I. Amdouni, A. Laouiti, L. Saidane, C. Adjih.
- PhD in progress: Khouloud Hwerbi (ENSI), “Optimized Architectures and Algorithms for Blockchain and IoT-based Applications”, since Nov. 2021. Advisors: I. Amdouni, A. Laouiti, L. Saidane, C. Adjih.
- PhD in progress: Koen Zandberg (IPP), “Cybersecure Multi-Tenant Microcontroller-based IoT”, since March 2020. Advisor: Emmanuel Baccelli.
- PhD in progress: Zhaolan Huang (FUB), “On-device Low-power Machine Learning for IoT”, since Jan. 2023. Advisor: Emmanuel Baccelli.
- PhD in progress: Rosario Patane, “VehiCloud: How can Vehicles increase Cloud intelligence?”, since Dec. 2021. Advisors: Lila Boukhatem, Andrea Araldo, Nadjib Achir.
- PhD in progress: IPP, Abhishek K. Mishra, “Detection of bypass frauds in cellular network datasets”, since Nov 2019. Advisor: Aline C. Viana and Nadjib Achir.
- PhD in progress: IPP, Anne Josiane Kouam Djuigne, “Detecting and simulating bypass SIMBox frauds in cellular networks”, since Nov 2019. Advisor: Aline C. Viana and Alain Tchana.
- PhD in progress: LNCC (Brazil), Haron C. Fantecele , “Mobility modeling and prediction through flow model”, since Feb. 2020. Advisor: Aline C. Viana and Antonio Tadeu (LNCC).
- General PhD involvment in research; monthly team meetings circulating ideas, PhD progress and talk training, and impact of the PhD works.
- Master supervision: the team regularly hosts master students and PhD interns for periods of 3 to 6 months. The list of students/interns concerned by this report year is mentioned in team members list.
- João Paulo Esper, “Impact of User Privacy and Mobility on Edge Offloading”. Advisors: Nadjib Achir and Kleber Vieira Cardoso (UFG) and Jussara M. Almeida (UFMG)
- Fernando Dias de Mello Silva, “Performance Analysis of a Privacy-Preserving Frame Sniffer on a Raspberry Pi”, from 01/02/2022 to 31/07/2022 Advisors: Aline C. Viana and Nadjib Achir and Luis Henrique M. K. Costa (UFRJ) and Anne Fladenmuller (Sorbonne University).
- Serge Makhoul, on “Federated and Edge Learning in IoT ” from 1/3/2022 to 30/6/2022. This internship contributed to the ICC 2023 publication see Section 8.15. Advisors: Kinda Khawam (UVSQ), S. Lahoud (USJ, Lebanon), C. Adjih.
- Team members continuously encourage, teach, and mentor students:
- to perform international short visits;
- to engage collaborations on related thesis subject;
- to advise master students during their thesis;
- to review articles, when possible;
- to the culture of open-source code, of ethical/privacy issues in data collection, and/or of standardization, according to their thesis subject;
- to give scientific talks (e.g., project meetings, Working Groups of associated communities, team meetings, etc);
- to participate to general activities such as Fête de la Science, popularization committee, shadow TPCs, etc
- for the ones interested in teaching, the good pointers at campus and when to start
- to find opportunities post-thesis: CV and personal website's advising; facilitating contacts with other Institutions/Researchers; recommendation letters
11.2.3 Juries
- Cédric Adjih (Reviewer): PhD defence of Aymeric de Javel, “5G RAN : physical layer implementation and network slicing”, IPP Telecom Paris, 30
- Cédric Adjih (Reviewer): PhD defence of Flavien Ronteix–Jacquet, “Reducing Latency and Jitter in the 5G Radio Access Network”, IMT Atlantique, 13 Dec. 2022.
- Cedric Adjih was an examiner for the mi-term committee of the following PhD student: Benoît-Marie Robaglia (“Application de l’IA à l’accès multiple non-coordonné”, IPP, Telecom Paris, 15 June 2022).
- Nadjib Achir (examiner): PhD defence of Sehla Khabaz, Radio Resource Allocation in C-V2X: From LTE-V2X to 5G-V2X, Sorbonne University, November 2022.
- Nadjib Achir (examiner): PhD defence of David Cordova, Blockchain Application for Mesh and Mobile Ad Hoc Networks, Sorbonne University, November 2022.
- Aline C. Viana (examiner): PhD defense of Renato C. Neto; Privacy-aware Aggregation of IoT Streams in Multi-Owner Networks; University of Strasbourg; July 2022
- Aline C. Viana (examiner): PhD defense of Victor Perrier; LEO/GEO congestion control mechanism based on the contribution of artificial intelligence; ENAC - Ecole Nationale de l'Aviation Civile; July 2023.
- Aline C. Viana (jury member): Mi-term PhD defence of Sniffer deployment in urban area for human trajectory reconstruction and contact tracing; Sniffer deployment in urban area for human trajectory reconstruction and contact tracing; University of La Rochelle; September 2012
11.3 Popularization
11.3.1 Internal or external Inria responsibilities
- For Aline C. Viana:
- At Inria:
- At the regional eco-system:
- Other committees:
11.3.2 Interventions
- Anne Josiane Kouam Djuigne, Abhishek Mishrak, and C. Adjih have welcomed and have presented some team subjects and aspects of research to a group of high-school students: insights on research careers, research topics on cellular simbox fraud, IoT concepts and programmation, etc.
12 Scientific production
12.1 Major publications
- 1 inproceedingsFrom movement purpose to perceptive spatial mobility prediction.ACM SIGSPATIAL 2021Beijing, ChinaNovember 2021
- 2 reportImpacts of novelty seeking on predictability in human mobility.InriaDecember 2021
- 3 inproceedingsUnderstanding individuals' proclivity for novelty seeking.ACM SIGSPATIAL 2020 - 28th ACM SIGSPATIAL International Conference on Advances in Geographic Information SystemsSeattle, Washington, United StatesNovember 2020
- 4 inproceedings Explorateur ou Routinier: Quel est votre profile de mobilité? ALGOTEL 2020 – 22èmes Rencontres Francophones sur les Aspects Algorithmiques des Télécommunications Lyon, France September 2020
- 5 reportSafeCityMap (1st phase) – COVID INRIA mission: Investigating population mobility habits in metropolitan zones and the lockdown impact using mobile phone data.InriaMay 2021
- 6 articleJoint String Complexity for Markov Sources: Small Data Matters *.Discrete Mathematics and Theoretical Computer Science2020
- 7 inproceedingsAssociating the Randomized Bluetooth MAC Addresses of a Device.Consumer Communications & Networking ConferenceLas Vegas, United StatesJanuary 2021
- 8 reportA Novel Sensor-Free Location Sampling Mechanism.University of Copenhagen, Faculty of Science, Denmark; Inria Saclay; NIC Chile Research Labs, University of Chile; IMDEA Software Institute; CNR - IEIITFebruary 2021
- 9 articleSIMBox bypass frauds in cellular networks: Strategies, evolution, detection, and future directions.Communications Surveys and Tutorials, IEEE Communications Society2021
- 10 miscR. E.RFC Editor (https://www.rfc-editor.org/)TinyMT32 Pseudorandom Number Generator (PRNG) (RFC 8682).RFC 8682, Standards Track, TSVWG (Transport Area) working group of IETF (Internet Engineering Task Force), https://www.rfc-editor.org/rfc/rfc8682.htmlJanuary 2020
- 11 inproceedingsDynamic Hierarchical Neural Network Offloading in IoT Edge Networks.PEMWN 2021 - 10th IFIP International Conference on Performance Evaluation and Modeling in Wireless and Wired NetworksOttawa / Virtual, CanadaIEEENovember 2021, 1-6
- 12 inproceedingsFemto-Containers: Lightweight Virtualization and Fault Isolation For Small Software Functions on Low-Power IoT Microcontrollers.Middleware 2022 - 23rd ACM/IFIP International Conference Middlewarequebec, CanadaNovember 2022, 1-12
12.2 Publications of the year
International journals
- 13 articleRevealing an inherently limiting factor in human mobility prediction.IEEE Transactions on Emerging Topics in Computing2022
- 14 articleOn the Edge of the Deployment: A Survey on Multi-Access Edge Computing.ACM Computing Surveys2022
- 15 articleData-driven Mobility Analysis and Modeling: Typical and Confined Life of a Metropolitan Population.ACM Transactions on Spatial Algorithms and Systems83September 2022, 1-33
- 16 articleA Game Theoretic Approach to Irregular Repetition Slotted Aloha.IEEE Access10January 2022, 4600-4614
- 17 articleEnergy and Delay Trade-Offs of End-to-End Vehicular Communications using a Hyperfractal Urban Modelling.Annals of Telecommunications - annales des télécommunications2023
- 18 articleHuman Mobility Support for Personalised Data Offloading.IEEE Transactions on Network and Service Management192February 2022, 1505-1520
- 19 articleHArMoNICS: High-Assurance Microgrid Network Infrastructure Case Study.IEEE Access102022, 115372-115383
- 20 articleOnline Drone-based Data Gathering Strategies for Ground Sensor Networks.International Journal of Sensor Networks2022
International peer-reviewed conferences
- 21 inproceedingsAnalyse de la qualité des liens Wi-Fi à partir de données expérimentales.CORES 2022 – 7ème Rencontres Francophones sur la Conception de Protocoles, l’Évaluation de Performance et l’Expérimentation des Réseaux de CommunicationSaint-Rémy-Lès-Chevreuse, FranceMay 2022, 1-4
- 22 inproceedingsUAV Search Path Planning For Livestock Monitoring.2022 IEEE 11th IFIP International Conference on Performance Evaluation and Modeling in Wireless and Wired Networks (PEMWN)Rome, ItalyIEEEJanuary 2023, 1-6
- 23 inproceedingsPerformance Analysis of a Privacy-Preserving Frame Sniffer on a Raspberry Pi.CSNet 2022 - 6th Cyber Security in Networking ConferenceRio de Janeiro, BrazilIEEEOctober 2022
- 24 inproceedingsA Survey on the Opportunities of Blockchain and UAVs in Agriculture.2022 IEEE 11th IFIP International Conference on Performance Evaluation and Modeling in Wireless and Wired Networks (PEMWN)Rome, ItalyIEEENovember 2022, 1-6
- 25 inproceedings Is Quantum Tomography a difficult problem for Machine Learning? Physical Sciences Forum (ISSN: 2673-9984) MAXENT'22 : 41st International Workshop on Bayesian Inference and Maximum Entropy Methods in Science and Engineering Paris, France 2023
- 26 inproceedingsGénération de traces cellulaires réalistes.CORES 2022 - 7ème Rencontres Francophones sur la Conception de Protocoles, l’Évaluation de Performance et l’Expérimentation des Réseaux de CommunicationSaint-Rémy-Lès-Chevreuse, FranceMay 2022
- 27 inproceedingsLSTM-based generation of cellular network traffic.WCNC 2023 - IEEE Wireless Communications and Networking ConferenceGlasgow, United KingdomMarch 2023
- 28 inproceedingsSimulating SIMBox frauds for detection investigation.CoNEXT Student Workshop 2022 - The 18th International Conference on emerging Networking EXperiments and TechnologiesRoma, ItalyDecember 2022
- 29 inproceedings Do WiFi Probe-Requests Reveal Your Trajectory? IEEE WCNC 2023 - IEEE Wireless Communications and Networking Conference Glasgow, United Kingdom March 2023
- 30 inproceedingsEnd-to-end Mechanized Proof of an eBPF Virtual Machine for Micro-controllers.CAV 2022 - 34th International Conference on Computer Aided VerificationHaifa, IsraelAugust 2022, 1-23
- 31 inproceedingsFemto-Containers: Lightweight Virtualization and Fault Isolation For Small Software Functions on Low-Power IoT Microcontrollers.Middleware 2022 - 23rd ACM/IFIP International Conference Middlewarequebec, CanadaNovember 2022, 1-12
National peer-reviewed Conferences
- 32 inproceedingsPrecise Minimax Regret for Logistic Regression.https://ieeexplore.ieee.org/xpl/conhome/9834325/proceedingISIT 2022Espoo, Finland2022
Edition (books, proceedings, special issue of a journal)
- 33 proceedingsG.Gustavo BanegasK.Koen ZandbergE.Emmanuel BaccelliA.Adrian HerrmannB.Benjamin SmithQuantum-Resistant Software Update Security on Low-Power Networked Embedded Devices.13269Lecture Notes in Computer ScienceSpringer International PublishingJune 2022, 872-891
- 34 proceedingsP.Philippe JacquetS.Svante JansonVariations on depth-first search performance in random digraphs.Special Issue: Probabilistic, Combinatorial and Asymptotic Methods for the Analysis of Algorithms”Springer Nature2023
- 35 periodicalConnecting flying backhauls of unmanned aerial vehicles to enhance vehicular networks with fixed 5G NR infrastructure.IET Smart Cities4September 2022, 239 - 254
Doctoral dissertations and habilitation theses
- 36 thesisConnectionless Transmission in Wireless Networks (IoT).Sorbonne UniversitéMay 2022
Reports & preprints
- 37 miscEnergy and Delay Trade-Offs of End-to-End Vehicular Communications using a Hyperfractal Urban Modelling.January 2022
- 38 reportDeep Learning, Sensing-based IRSA (DS-IRSA): Learning a Sensing Protocol with Deep Reinforcement Learning.RR-9479INRIA-SACLAYJune 2022
- 39 miscInformation Theoretic Study of Covid 19 Genome.January 2022
- 40 miscZen: LSTM-based generation of individual spatiotemporal cellular traffic with interactions.January 2023
12.3 Cited publications
- 41 techreportAn inherent limiting factor of human mobility prediction.INSTITUT POLYTECHNIQUE DE PARIS ; INRIA Saclay, équipe TribeFebruary 2021
- 42 techreportImpacts of novelty seeking on predictability in human mobility.InriaDecember 2021
- 43 techreportSafeCityMap (1st phase) -- COVID INRIA mission: Investigating population mobility habits in metropolitan zones and the lockdown impact using mobile phone data.InriaMay 2021
- 44 inproceedingsOn the Sampling Frequency of Human Mobility.IEEE GLOBECOM 2017Singapore, SingaporeDecember 2017, 1-6
- 45 unpublishedSIMBox bypass frauds in cellular networks: Strategies, evolution, and detection survey.January 2021, working paper or preprint
- 46 inproceedingsImpacts of Human Mobility in Mobile Data Offloading.ACM Chants 2018 - 13th Workshop on Challenged Networks4New Delhi, IndiaOctober 2018