2023Activity reportProject-TeamABS
RNSR: 200818987H- Research center Inria Centre at Université Côte d'Azur
- Team name: Algorithms - Biology - Structure
- Domain:Digital Health, Biology and Earth
- Theme:Computational Biology
Keywords
Computer Science and Digital Science
- A2.5. Software engineering
- A3.3.2. Data mining
- A3.4.1. Supervised learning
- A3.4.2. Unsupervised learning
- A6.1.4. Multiscale modeling
- A6.2.4. Statistical methods
- A6.2.8. Computational geometry and meshes
- A8.1. Discrete mathematics, combinatorics
- A8.3. Geometry, Topology
- A8.7. Graph theory
- A9.2. Machine learning
Other Research Topics and Application Domains
- B1.1.1. Structural biology
- B1.1.5. Immunology
- B1.1.7. Bioinformatics
1 Team members, visitors, external collaborators
Research Scientists
- Frédéric Cazals [Team leader, INRIA, Senior Researcher, HDR]
- Dorian Mazauric [INRIA, Researcher, HDR]
- Edoardo Sarti [INRIA, Researcher]
PhD Students
- Guillaume Carriere [INRIA, from Oct 2023]
- Ercan Seckin [INRAE, from Oct 2023]
Technical Staff
- Come Le Breton [INRIA, Engineer]
Interns and Apprentices
- Tejas Anand [INRIA, Intern, from May 2023 until Jul 2023, IIT Delhi]
- Jules Herrmann [INRIA, Intern, from May 2023 until Aug 2023, Université Paris Cité]
- Parth Patel [INRIA, Intern, from May 2023 until Jul 2023, IIT Delhi]
- Manpreet Singh [INRIA, Intern, from May 2023 until Jul 2023, IIT Delhi]
- Suren Suren [INRIA, Intern, from May 2023 until Jul 2023, IIT Delhi]
Administrative Assistant
- Florence Barbara [INRIA]
External Collaborator
- David Wales [UNIV CAMBRIDGE]
2 Overall objectives
Biomolecules and their function(s).
Computational Structural Biology (CSB) is the scientific domain concerned with the development of algorithms and software to understand and predict the structure and function of biological macromolecules. This research field is inherently multi-disciplinary. On the experimental side, biology and medicine provide the objects studied, while biophysics and bioinformatics supply experimental data, which are of two main kinds. On the one hand, genome sequencing projects give supply protein sequences, and ~200 millions of sequences have been archived in UniProtKB/TrEMBL – which collects the protein sequences yielded by genome sequencing projects. On the other hand, structure determination experiments (notably X-ray crystallography, nuclear magnetic resonance, and cryo-electron microscopy) give access to geometric models of molecules – atomic coordinates. Alas, only ~150,000 structures have been solved and deposited in the Protein Data Bank (PDB), a number to be compared against the sequences found in UniProtKB/TrEMBL. With one structure for ~1000 sequences, we hardly know anything about biological functions at the atomic/structural level. Complementing experiments, physical chemistry/chemical physics supply the required models (energies, thermodynamics, etc). More specifically, let us recall that proteins with atoms has Cartesian coordinates, and fixing these (up to rigid motions) defines a conformation. As conveyed by the iconic lock-and-key metaphor for interacting molecules, Biology is based on the interactions stable conformations make with each other. Turning these intuitive notions into quantitative ones requires delving into statistical physics, as macroscopic properties are average properties computed over ensembles of conformations. Developing effective algorithms to perform accurate simulations is especially challenging for two main reasons. The first one is the high dimension of conformational spaces – see above, typically several tens of thousands, and the non linearity of the energy functionals used. The second one is the multiscale nature of the phenomena studied: with biologically relevant time scales beyond the millisecond, and atomic vibrations periods of the order of femto-seconds, simulating such phenomena typically requires conformations/frames, a (brute) tour de force rarely achieved 34.
Computational Structural Biology: three main challenges.
The first challenge, sequence-to-structure prediction, aims to infer the possible structure(s) of a protein from its amino acid sequence. While recent progress has been made recently using in particular deep learning techniques 33, the models obtained so far are static and coarse-grained.
The second one is protein function prediction. Given a protein with known structure, i.e., 3D coordinates, the goal is to predict the partners of this protein, in terms of stability and specificity. This understanding is fundamental to biology and medicine, as illustrated by the example of the SARS-CoV-2 virus responsible of the Covid19 pandemic. To infect a host, the virus first fuses its envelope with the membrane of a target cell, and then injects its genetic material into that cell. Fusion is achieved by a so-called class I fusion protein, also found in other viruses (influenza, SARS-CoV-1, HIV, etc). The fusion process is a highly dynamic process involving large amplitude conformational changes of the molecules. It is poorly understood, which hinders our ability to design therapeutics to block it.
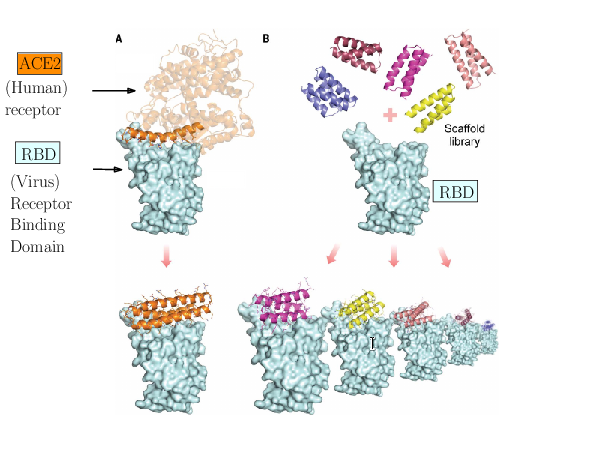
The spike of SARS-CoV-2 is responsible for the infection. Molecules can be engineered to compete with the receptor of the spike, and block infection.
Finally, the third one, large assembly reconstruction, aims at solving (coarse-grain) structures of molecular machines involving tens or even hundreds of subunits. This research vein was promoted about 15 years back by the work on the nuclear pore complex 22. It is often referred to as reconstruction by data integration, as it necessitates to combine coarse-grain models (notably from cryo-electron microscopy (cryo-EM) and native mass spectrometry) with atomic models of subunits obtained from X ray crystallography. Fitting the latter into the former requires exploring the conformation space of subunits, whence the importance of protein dynamics.
As an illustration of these three challenges, consider the problem of designing proteins blocking the entry of SARS-CoV-2 into our cells (Fig. 1). The first challenge is illustrated by the problem of predicting the structure of a blocker protein from its sequence of amino-acids – a tractable problem here since the mini proteins used only comprise of the order of 50 amino-acids (Fig. 1(A), 25). The second challenge is illustrated by the calculation of the binding modes and the binding affinity of the designed proteins for the RBD of SARS-CoV-2 (Fig. 1(B)). Finally, the last challenge is illustrated by the problem of solving structures of the virus with a cell, to understand how many spikes are involved in the fusion mechanism leading to infection. In 25, the promising designs suggested by modeling have been assessed by an array of wet lab experiments (affinity measurements, circular dichroism for thermal stability assessment, structure resolution by cryo-EM). The hyperstable minibinders identified provide starting points for SARS-CoV-2 therapeutics 25. We note in passing that this is truly remarkable work, yet, the designed proteins stem from a template (the bottom helix from ACE2), and are rather small.
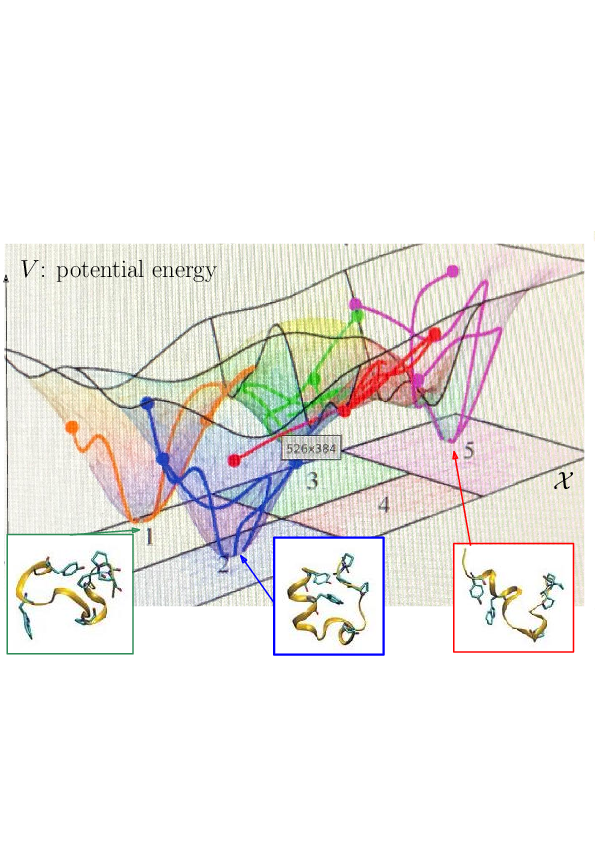
Prosaically, the energy landscape of a molecular system is the set of valleys and passes connecting them, corresponding to stable states and transitions between them.
Protein dynamics: core CS - maths challenges.
To present challenges in structural modeling, let us recall the following ingredients (Fig. 2). First, a molecular model with atoms is parameterized over a conformational space of dimension in Cartesian coordinates, or in internal coordinate–upon removing rigid motions, also called degree of freedom (d.o.f.). Second, recall that the potential energy landscape (PEL) is the mapping from to providing a potential energy for each conformation 35, 32. Example potential energies (PE) are CHARMM, AMBER, MARTINI, etc. Such PE belong to the realm of molecular mechanics, and implement atomic or coarse-grain models. They may embark a solvent model, either explicit or implicit. Their definition requires a significant number of parameters (up to ), fitted to reproduce physico-chemical properties of (bio-)molecules 36.
These PE are usually considered good enough to study non covalent interactions – our focus, even tough they do not cover the modification of chemical bonds. In any case, we take such a function for granted 1.
The PEL codes all structural, thermodynamic, and kinetic properties, which can be obtained by averaging properties of conformations over so-called thermodynamic ensembles. The structure of a macromolecular system requires the characterization of active conformations and important intermediates in functional pathways involving significant basins. In assigning occupation probabilities to these conformations by integrating Boltzmann's distribution, one treats thermodynamics. Finally, transitions between the states, modeled, say, by a master equation (a continuous-time Markov process), correspond to kinetics. Classical simulation methods based on molecular dynamics (MD) and Monte Carlo sampling (MC) are developed in the lineage of the seminal work by the 2013 recipients of the Nobel prize in chemistry (Karplus, Levitt, Warshel), which was awarded “for the development of multiscale models for complex chemical systems”. However, except for highly specialized cases where massive calculations have been used 34, neither MD nor MC give access to the aforementioned time scales. In fact, the main limitation of such methods is that they treat structural, thermodynamic and kinetic aspects at once 28. The absence of specific insights on these three complementary pieces of the puzzle makes it impossible to optimize simulation methods, and results in general in the inability to obtain converged simulations on biologically relevant time-scales.
The hardness of structural modeling owes to three intertwined reasons.
First, PELs of biomolecules usually exhibit a number of critical points exponential in the dimension 23; fortunately, they enjoy a multi-scale structure 26. Intuitively, the significant local minima/basins are those which are deep or isolated/wide, two notions which are mathematically qualified by the concepts of persistence and prominence. Mathematically, problems are plagued with the curse of dimensionality and measure concentration phenomena. Second, biomolecular processes are inherently multi-scale, with motions spanning 15 and 4 orders of magnitude in time and amplitude respectively 21. Developing methods able to exploit this multi-scale structure has remained elusive. Third, macroscopic properties of biomolecules, i.e., observables, are average properties computed over ensembles of conformations, which calls for a multi-scale statistical treatment both of thermodynamics and kinetics.
Validating models.
A natural and critical question naturally concerns the validation of models proposed in structural bioinformatics. For all three types of questions of interest (structures, thermodynamics, kinetics), there exist experiments to which the models must be confronted – when the experiments can be conducted.
For structures, the models proposed can readily be compared against experimental results stemming from X ray crystallography, NMR, or cryo electron microscopy. For thermodynamics, which we illustrate here with binding affinities, predictions can be compared against measurements provided by calorimetry or surface plasmon resonance. Lastly, kinetic predictions can also be assessed by various experiments such as binding affinity measurements (for the prediction of and ), or fluorescence based methods (for kinetics of folding).
3 Research program
Our research program ambitions to develop a comprehensive set of novel concepts and algorithms to study protein dynamics, based on the modular framework of PEL.
3.1 Modeling the dynamics of proteins
Keywords: Molecular conformations, conformational exploration, energy landscapes, thermodynamics, kinetics.
As noticed while discussing Protein dynamics: core CS - maths challenges, the integrated nature of simulation methods such as MD or MC is such that these methods do not in general give access to biologically relevant time scales. The framework of energy landscapes 35, 32 (Fig. 2) is much more modular, yet, large biomolecular systems remain out of reach.
To make a definitive step towards solving the prediction of protein dynamics, we will serialize the discovery and the exploitation of a PEL 4, 15, 3. Ideas and concepts from computational geometry/geometric motion planning, machine learning, probabilistic algorithms, and numerical probability will be used to develop two classes of probabilistic algorithms. The first deals with algorithms to discover/sketch PELs, i.e., enumerate all significant (persistent or prominent) local minima and their connections across saddles, a difficult task since the number of all local minima/critical points is generally exponential in the dimension. To this end, we will develop a hierarchical data structure coding PELs as well as multi-scale proposals to explore molecular conformations. (NB: in Monte Carlo methods, a proposal generates a new conformation from an existing one.) The second focuses on methods to exploit/sample PELs, i.e., compute so-called densities of states, from which all thermodynamic quantities are given by standard relations 2431. This is a hard problem akin to high-dimensional numerical integration. To solve this problem, we will develop a learning based strategy for the Wang-Landau algorithm 30–an adaptive Monte Carlo Markov Chain (MCMC) algorithm, as well as a generalization of multi-phase Monte Carlo methods for convex/polytope volume calculations 29, 27, for non convex strata of PELs.
3.2 Algorithmic foundations: geometry, optimization, machine learning
Keywords: Geometry, optimization, machine learning, randomized algorithms, sampling, optimization.
As discussed in the previous Section, the study of PEL and protein dynamics raises difficult algorithmic / mathematical questions. As an illustration, one may consider our recent work on the comparison of high dimensional distribution 6, statistical tests / two-sample tests 7, 12, the comparison of clustering 8, the complexity study of graph inference problems for low-resolution reconstruction of assemblies 11, the analysis of partition (or clustering) stability in large networks, the complexity of the representation of simplicial complexes 2. Making progress on such questions is fundamental to advance the state-of-the art on protein dynamics.
We will continue to work on such questions, motivated by CSB / theoretical biophysics, both in the continuous (geometric) and discrete settings. The developments will be based on a combination of ideas and concepts from computational geometry, machine learning (notably on non linear dimensionality reduction, the reconstruction of cell complexes, and sampling methods), graph algorithms, probabilistic algorithms, optimization, numerical probability, and also biophysics.
3.3 Software: the Structural Bioinformatics Library
Keywords: Scientific software, generic programming, molecular modeling.
While our main ambition is to advance the algorithmic foundations of molecular simulation, a major challenge will be to ensure that the theoretical and algorithmic developments will change the fate of applications, as illustrated by our case studies. To foster such a symbiotic relationship between theory, algorithms and simulation, we will pursue high quality software development and integration within the SBL, and will also take the appropriate measures for the software to be widely adopted.
Software in structural bioinformatics.
Software development for structural bioinformatics is especially challenging, combining advanced geometric, numerical and combinatorial algorithms, with complex biophysical models for PEL and related thermodynamic/kinetic properties. Specific features of the proteins studied must also be accommodated. About 50 years after the development of force fields and simulation methods (see the 2013 Nobel prize in chemistry), the software implementing such methods has a profound impact on molecular science at large. One can indeed cite packages such as CHARMM, AMBER, gromacs, gmin, MODELLER, Rosetta, VMD, PyMol, .... On the other hand, these packages are goal oriented, each tackling a (small set of) specific goal(s). In fact, no real modular software design and integration has taken place. As a result, despite the high quality software packages available, inter-operability between algorithmic building blocks has remained very limited.
The SBL.
Predicting the dynamics of large molecular systems requires the integration of advanced algorithmic building blocks / complex software components. To achieve a sufficient level of integration, we undertook the development of the Structural Bioinformatics Library (SBL, SB) 5, a generic C++/python cross-platform library providing software to solve complex problems in structural bioinformatics. For end-users, the SBL provides ready to use, state-of-the-art applications to model macro-molecules and their complexes at various resolutions, and also to store results in perennial and easy to use data formats (SBL Applications). For developers, the SBL provides a broad C++/python toolbox with modular design (SBL Doc). This hybrid status targeting both end-users and developers stems from an advanced software design involving four software components, namely applications, core algorithms, biophysical models, and modules (SBL Modules). This modular design makes it possible to optimize robustness and the performance of individual components, which can then be assembled within a goal oriented application.
3.4 Applications: modeling interfaces, contacts, and interactions
Keywords: Protein interactions, protein complexes, structure/thermodynamics/kinetics prediction.
Our methods will be validated on various systems for which flexibility operates at various scales. Examples of such systems are antibody-antigen complexes, (viral) polymerases, (membrane) transporters.
Even very complex biomolecular systems are deterministic in prescribed conditions (temperature, pH, etc), demonstrating that despite their high dimensionality, all d.o.f. are not at play at the same time. This insight suggests three classes of systems of particular interest. The first class consists of systems defined from (essentially) rigid blocks whose relative positions change thanks to conformational changes of linkers; a Newton cradle provides an interesting way to envision such as system. We have recently worked on one such system, a membrane proteins involve in antibiotic resistance (AcrB, see 16). The second class consists of cases where relative positions of subdomains do not significantly change, yet, their intrinsic dynamics are significantly altered. A classical illustration is provided by antibodies, whose binding affinity owes to dynamics localized in six specific loops 13, 14. The third class, consisting of composite cases, will greatly benefit from insights on the first two classes. As an example, we may consider the spikes of the SARS-CoV-2 virus, whose function (performing infection) involves both large amplitude conformational changes and subtle dynamics of the so-called receptor binding domain. We have started to investigate this system, in collaboration with B. Delmas (INRAE).
In ABS, we will investigate systems in these three tiers, in collaboration with expert collaborators, to hopefully open new perspectives in biology and medicine. Along the way, we will also collaborate on selected questions at the interface between CSB and systems biology, as it is now clear that the structural level and the systems level (pathways of interacting molecules) can benefit from one another.
4 Application domains
The main application domain is Computational Structural Biology, as underlined in the Research Program.
5 Social and environmental responsibility
5.1 Footprint of research activities
A tenet of ABS is to carefully analyze the performances of the algorithms designed–either formally or experimentally, so as to avoid massive calculations. Therefore, the footprint of our research activities has remained limited.
5.2 Impact of research results
The scientific agenda of ABS is geared towards a fine understanding of complex phenomena at the atomic/molecular level. While the current focus is rather fundamental, as explained in Research program, an overarching goal for the current period (i.e. 12 years) is to make significant contributions to important problems in biology and medicine.
6 Highlights of the year
The main scientific achievement of the year has been the finalization of sampling techniques to explore large amplitude conformation changes of flexible loops, see 17, 18, based on Monte Carlo Markov chain techniques we introduced for the calculation of the volume of polytopes 9.
7 New software, platforms, open data
7.1 New software
7.1.1 SBL
-
Name:
Structural Bioinformatics Library
-
Keywords:
Structural Biology, Biophysics, Software architecture
-
Functional Description:
The SBL is a generic C++/python cross-platform software library targeting complex problems in structural bioinformatics. Its tenet is based on a modular design offering a rich and versatile framework allowing the development of novel applications requiring well specified complex operations, without compromising robustness and performances.
More specifically, the SBL involves four software components (1-4 thereafter). For end-users, the SBL provides ready to use, state-of-the-art (1) applications to handle molecular models defined by unions of balls, to deal with molecular flexibility, to model macro-molecular assemblies. These applications can also be combined to tackle integrated analysis problems. For developers, the SBL provides a broad C++ toolbox with modular design, involving core (2) algorithms, (3) biophysical models, and (4) modules, the latter being especially suited to develop novel applications. The SBL comes with a thorough documentation consisting of user and reference manuals, and a bugzilla platform to handle community feedback.
-
Release Contributions:
The achievements in 2023 are twofold. First, a structure file reader handling the PDB and mmCIF formats was integrated, based on the libcifpp library (voir https://github.com/PDB-REDO/libcifpp). Second, the development of three packages of broad interest for users was finalized: Loopsampler (sampler for flexible loops, Kpax (structural alignments), and Spectrus (decomposition of proteins into quasi-rigid domains). These packages will be integrated to the public release early 2024.
- URL:
- Publication:
-
Contact:
Frédéric Cazals
8 New results
Participants: F. Cazals, D. Mazauric, E. Sarti.
8.1 Modeling the dynamics of proteins
Keywords: Protein flexibility, protein conformations, collective coordinates, conformational sampling, loop closure, kinematics, dimensionality reduction.
8.1.1 Enhanced conformational exploration of protein loops using a global parameterization of the backbone geometry
Participants: F. Cazals, T. O'Donnell.
Flexible loops are paramount to protein functions, with action modes ranging from localized dynamics contributing to the free energy of the system, to large amplitude conformational changes accounting for the repositioning whole secondary structure elements or protein domains. However, generating diverse and low energy loops remains a difficult problem.
This work 18 introduces a novel paradigm to sample loop conformations, in the spirit of the Hit-and-Run (HAR) Markov chain Monte Carlo technique. The algorithm uses a decomposition of the loop into tripeptides, and a novel characterization of necessary conditions for Tripeptide Loop Closure to admit solutions. Denoting the number of tripeptides, the algorithm works in an angular space of dimension . In this space, the hyper-surfaces associated with the aforementioned necessary conditions are used to run a HAR-like sampling technique. On classical loop cases up to 15 amino acids, our parameter free method compares favorably to previous work, generating more diverse conformational ensembles. We also report experiments on a 30 amino acids long loop, a size not processed in any previous work.
8.2 Algorithmic foundations
Keywords: Computational geometry, computational topology, optimization, graph theory, data analysis, statistical physics.
8.2.1 Geometric constraints within tripeptides and the existence of tripeptide reconstructions
Participants: F. Cazals, T. O'Donnell, V. Agashe, IIT Delhi, India.
Designing movesets providing high quality protein conformations remains a hard problem, especially when it comes to deform a long protein backbone segment, and a key building block to do so is the so-called tripeptide loop closure (TLC) 17. Consider a tripeptide whose first and last bonds ( and ) are fixed, and so are all internal coordinates except the six dihedral angles associated to the three carbons. Under these conditions, the TLC algorithm provides all possible values for these six dihedral angles–there exists at most 16 solutions. TLC moves atoms up to in one step and retains low energy conformations, whence its pivotal role to design move sets sampling protein loop conformations.
In this work 17, we relax the previous constraints, allowing the last bond () to freely move in 3D space–or equivalently in a 5D configuration space. We exhibit necessary geometric constraints in this 5D space for TLC to admit solutions. Our analysis provides key insights on the geometry of solutions for TLC. Most importantly, when using TLC to sample loop conformations based on consecutive tripeptides along a protein backbone, we obtain an exponential gain in the volume of the -dimensional configuration space to be explored.
8.3 Applications in structural bioinformatics and beyond
Keywords: Docking, scoring, interfaces, protein complexes, phylogeny, evolution.
8.3.1 Discriminating physiological from non-physiological interfaces in structures of protein complexes: A community-wide study
Participant: F. Cazals.
Community contribution in the scope of the Elixir / 3D Bioinfo project, see the paper and benchmark.
Reliably scoring and ranking candidate models of protein complexes and assigning their oligomeric state from the structure of the crystal lattice represent outstanding challenges. A community-wide effort was launched to tackle these challenges 19. The latest resources on protein complexes and interfaces were exploited to derive a benchmark dataset consisting of 1677 homodimer protein crystal structures, including a balanced mix of physiological and non-physiological complexes. The non-physiological complexes in the benchmark were selected to bury a similar or larger interface area than their physiological counterparts, making it more difficult for scoring functions to differentiate between them. Next, 252 functions for scoring protein-protein interfaces previously developed by 13 groups were collected and evaluated for their ability to discriminate between physiological and non-physiological complexes. A simple consensus score generated using the best performing score of each of the 13 groups, and a cross-validated Random Forest (RF) classifier were created. Both approaches showed excellent performance, with an area under the Receiver Operating Characteristic (ROC) curve of 0.93 and 0.94, respectively, outperforming individual scores developed by different groups. Additionally, AlphaFold2 engines recalled the physiological dimers with significantly higher accuracy than the non-physiological set, lending support to the reliability of our benchmark dataset annotations. Optimizing the combined power of interface scoring functions and evaluating it on challenging benchmark datasets appears to be a promising strategy.
9 Partnerships and cooperations
Participants: Frédéric Cazals, Edoardo Sarti.
9.1 International research visitors
9.1.1 Visits of international scientists
Inria International Chair
- David Wales, Cambridge University, is endowed chair within 3IA Côte d'Azur / ABS.
9.2 National initiatives
Action Exploratoire Inria.
The AEx DEFINE, involving Inria ABS and Laboratory of Computational and Quantitative Biology (LCQB) from Sorbonne University started in Septembre 2023, for a period of four years.
ABS develops novel methods to study protein structure and dynamics, using computational geom- etry/topology and machine learning. LCQB is a leading lab addressing core questions at the heart of modern biology, with a unique synergy between quantitative models and experiments. The goal of DEFINE is to provide a synergy between ABS and LCQB, with a focus on the prediction of protein functions, at the genome scale and for two specific applications (photosynthesis, DNA repair).
Co-supervised PhD thesis Inria-INRAE.
The PhD thesis of Ercan Seckin started in october 2023 is co-supervised by Etienne Danchin (supervisor) and Dominique Colinet at the INRAE GAME team and Edoardo Sarti at ABS.
The thesis title is: Détection, histoire évolutive et relations structure - fonction des gènes orphelins chez les bioagresseurs des plantes. The two teams are closely collaborating for advancing current knowledge on the emergence of orphan genes/proteins in the Meloidogyne genus as well as their structural and functional characterization. Notably, the ABS team will focus on the structural and functional inference, and the interplay between structure and function in the process of gene formation.
10 Dissemination
Participants: Frédéric Cazals, Dorian Mazauric, Edoardo Sarti.
10.1 Promoting scientific activities
10.1.1 Scientific events: organisation
Frédéric Cazals was involved in the organization of:
- Winter School Algorithms in Structural Bioinformatics: From structure resolution to dynamical modeling in cryo-electron microscopy, Institute for Scientific Study of Cargese (IESC), November 20-24th. Web: AlgoSB.
General chair, scientific chair
- Energy Landscapes, 2023. F. Cazals was the general chair of the workshop Energy Landscapes (Eland), the premier meeting for scientists (physicists, chemical physicists, bio-physicists, biologists, computer scientists) working on the problem of computing (potential, free) energies for bio-molecular systems.
Member of the organizing committees
Edoardo Sarti participated to the following organizing committee:
- Journées Ouvertes en Biologie, Informatique et Mathématiques (JOBIM), June 27-30th. Web: JOBIM2023
Member of the conference program committees
Frédéric Cazals participated to the following program committees:
- Symposium on Solid and Physical Modeling
- Intelligent Systems for Molecular Biology (ISMB)
- Journées Ouvertes en Biologie, Informatique et Mathématiques (JOBIM)
Dorian Mazauric participated to the following program committee:
- Rencontres Francophones sur les Aspects Algorithmiques des Télécommunications (AlgoTel 2023)
10.1.2 Invited talks
Frédéric Cazals gave the following invited talks:
- Studying complex molecular mechanisms via rigid domains detection and conformational sampling of flexible linkers, Integrative Structural Biology congress, Marseille, November 2023.
- Sampling protein conformations, Thematic meeting Probabilistic sampling for physics, Institut Blaise Pascal, Paris-Saclay, September 2023.
- Subspace-Embedded Spherical Clusters: a novel cluster model for compact clusters of arbitrary dimension, DataShape workshop, Porquerolles, May 2023.
10.1.3 Leadership within the scientific community
Frédéric Cazals:
- 2010-...: Member of the steering committee of the GDR Bioinformatique Moléculaire, for the Structure and macro-molecular interactions theme.
- 2017-...: Co-chair, with Yann Ponty, of the working group / groupe de travail (GT MASIM - Méthodes Algorithmiques pour les Structures et Interactions Macromoléculaires), within the GDR de BIoinfor- matique Moléculaire (GDR BIM, GDR BIM).
10.1.4 Research administration
Frédéric Cazals
- 2020-...: Member of the bureau of the EUR Life, Université Côte d’Azur.
Dorian Mazauric
- 2019-...: Member of the comité Plateformes.
Edoardo Sarti
- 2020-...: Member of the Commission de Développement Technologique at Inria Université Côte d’Azur
10.2 Teaching - Supervision - Juries
10.2.1 Teaching
- 2014–...: Master Data Sciences Program (M2), Department of Applied Mathematics, Ecole Centrale-Supélec; Foundations of Geometric Methods in Data Analysis; F. Cazals and M. Carrière, Inria Sophia / (ABS, DataShape). Web: FGMDA.
- 2021–...: Master Data Sciences & Artificial Intelligence (M1), Université Côte d’Azur; Introduction to machine learning (course leader); E. Sarti; Web: IntroML
- 2021–...: Master Data Sciences & Artificial Intelligence (M2), Université Côte d’Azur; Geometric and topological methods in machine learning; F. Cazals, J-D. Boissonnat and M. Carrière, Inria Sophia / (ABS, DataShape, DataShape); Web: GTML.
- 2021–...: Master Cancérologie et Recherche Translationnelle (M2), Université Côte d’Azur; Binding affinity maturation and protein interaction network analysis: two examples of bioinformatics applications in medicine; F. Cazals.
- 2020–...: Master Sciences du Vivant (M2), parcours Biologie, Informatique, Mathématiques, Université Côte d’Azur; Introduction to statistical physics of biomolecules; F. Cazals.
- 2022–2023...: Master : Algorithmique et Complexité, 42h Cours et TD, niveau M1, Polytech Nice Sophia, Université Côte d'Azur, filière Sciences Informatiques, France; D. Mazauric.
- 2022–2023...: Master : Algorithmique avancée, 24h Cours et TD, niveau M1, Polytech Nice Sophia, Université Côte d'Azur, filière Sciences Informatiques, France; D. Mazauric (avec Éric Pascual)
- 2022–...: Bachelor Sciences de la Vie (L2), Université Côte d'Azur; Introduction à la programmation (course leader), E. Sarti; Web: IntroInfo
- 2021–2023: Bachelor Informatique (L1), Université Côte d'Azur; Introduction aux Systemes Unix (practicals), E. Sarti
- Dizaine de formations (pour les enseignantes et enseignants, personnels de médiathèque, d'associations, etc.)
10.2.2 Supervision
PhD thesis:
- Ongoing, October 2023-...: Guillaume Carrière. Attention mechanisms for graphical models, with applications to protein structure analysis. Advisor: F. Cazals.
- Ongoing, October 2023-...: Ercan Seckin. Détection, histoire évolutive et relations structure – fonction des gènes orphelins chez les bioagresseurs des plantes. Advisor: Etienne Danchin (INRAE), Co-advisors: Dominique Colinet (INRAE), Edoardo Sarti.
- Ongoing, May 2023-...: Sebastián Gallardo Diaz. Optimizing newspaper aesthetics preserving style under visual constraints: a computational approach of layouting.. Advisor: P. Kornprobst, D. Mazauric.
10.2.3 Juries
Frédéric Cazals participated to the following committees:
- Conor Thomas Cafolla, Cambridge University, November 2023. Rapporteur for the PhD thesis On Critical Care Data and Machine Learning Loss Function Landscapes. Advisor: David Wales.
- Jeanne Trinquier, Sorbonne Université. September 2023. Rapporteur for the thesis Data-driven generative modeling of protein sequence landscapes and beyond. Advisors: Martin Weigt, Francesco Zamponi.
10.3 Popularization
10.3.1 Internal or external Inria responsibilities
Dorian Mazauric
- 2019-...: Coordinator of Terra Numerica – vers une Cité du Numérique, an ambitious scientific popularisation project. Its main goal is to create a "Dedicated Digital space" in the south of France, (in the spirit of the "Cité des Sciences" or "Palais de la découverte" in Paris). To do so, Terra Numerica is developing and structuring popularisation activities, supports which are spread in different antennas throughout the territory (e.g., Espace Terra Numerica - Valbonne Sophia Antipolis, Maison de l'Intelligence Artificielle (MIA), in schools, exhibition extensions...). This large-scale project involves (brings together) all the actors of research, education, industry, associations and collectivities... It is actually composed of more than one hundred people.
- Supervision of a bachelor student (apprenti) and two Master internships, in the scope of Terra Numerica.
- 2018-...: Member of the Conseil d'Administration de l'association les Petits Débrouillards.
- 2017-...: Member of projet de médiation Galéjade : Graphes et ALgorithmes : Ensemble de Jeux À Destination des Ecoliers... (mais pas que).
10.3.2 Interventions
Dorian Mazauric participated and/or organized 379 popularization events in 2023 (including 407 classes and 11 000 young students). See Terra Numerica website.
11 Scientific production
11.1 Major publications
- 1 articleDistributed Link Scheduling in Wireless Networks.Discrete Mathematics, Algorithms and Applications1252020, 1-38HALDOI
- 2 articleOn the complexity of the representation of simplicial complexes by trees.Theoretical Computer Science617February 2016, 17HALDOIback to text
- 3 articleEnergy landscapes and persistent minima.The Journal of Chemical Physics14452016, 4URL: https://www.repository.cam.ac.uk/handle/1810/253412DOIback to text
- 4 articleConformational Ensembles and Sampled Energy Landscapes: Analysis and Comparison.J. of Computational Chemistry36162015, 1213--1231URL: https://hal.archives-ouvertes.fr/hal-01076317DOIback to text
- 5 articleThe Structural Bioinformatics Library: modeling in biomolecular science and beyond.Bioinformatics338April 2017HALDOIback to text
- 6 inproceedingsBeyond Two-sample-tests: Localizing Data Discrepancies in High-dimensional Spaces.IEEE/ACM International Conference on Data Science and Advanced AnalyticsIEEE/ACM International Conference on Data Science and Advanced AnalyticsIEEE/ACM International Conference on Data Science and Advanced AnalyticsParis, FranceMarch 2015, 29HALback to text
- 7 inproceedingsLow-Complexity Nonparametric Bayesian Online Prediction with Universal Guarantees.NeurIPS 2019 - Thirty-third Conference on Neural Information Processing SystemsVancouver, CanadaDecember 2019HALback to text
- 8 articleComparing Two Clusterings Using Matchings between Clusters of Clusters.ACM Journal of Experimental Algorithmics241December 2019, 1-41HALDOIback to text
- 9 inproceedingsEfficient computation of the volume of a polytope in high-dimensions using Piecewise Deterministic Markov Processes.AISTATS 2022 - 25th International Conference on Artificial Intelligence and StatisticsVirtual, FranceMarch 2022HALback to text
- 10 articleWang-Landau Algorithm: an adapted random walk to boost convergence.Journal of Computational Physics4102020, 109366HALDOI
- 11 articleComplexity dichotomies for the Minimum F -Overlay problem.Journal of Discrete Algorithms52-53September 2018, 133-142HALDOIback to text
- 12 articleA Sequential Non-Parametric Multivariate Two-Sample Test.IEEE Transactions on Information Theory645May 2018, 3361-3370HALback to text
- 13 reportHigh Resolution Crystal Structures Leverage Protein Binding Affinity Predictions.RR-8733InriaMarch 2015HALback to text
- 14 articleNovel Structural Parameters of Ig–Ag Complexes Yield a Quantitative Description of Interaction Specificity and Binding Affinity.Frontiers in Immunology8February 2017, 34HALDOIback to text
- 15 articleHybridizing rapidly growing random trees and basin hopping yields an improved exploration of energy landscapes.J. Comp. Chem.3782016, 739--752URL: https://hal.inria.fr/hal-01191028DOIback to text
- 16 articleStudying dynamics without explicit dynamics: A structure‐based study of the export mechanism by AcrB.Proteins - Structure, Function and BioinformaticsSeptember 2020HALDOIback to text
11.2 Publications of the year
International journals
Reports & preprints
11.3 Cited publications
- 21 articleMolecular dynamics: survey of methods for simulating the activity of proteins.Chemical reviews10652006, 1589--1615back to text
- 22 articleThe molecular architecture of the nuclear pore complex.Nature45071702007, 695--701back to text
- 23 articleDynamics on statistical samples of potential energy surfaces.The Journal of chemical physics11151999, 2060--2070back to text
- 24 bookThermodynamics and an Introduction to Thermostatistics.Wiley1985back to text
- 25 articleDe novo design of picomolar SARS-CoV-2 miniprotein inhibitors.Science37065152020, 426--431back to textback to textback to textback to text
- 26 articleEnergy landscapes and persistent minima.The Journal of Chemical Physics14452016, 4URL: https://www.repository.cam.ac.uk/handle/1810/253412DOIback to text
- 27 articleA practical volume algorithm.Mathematical Programming Computation822016, 133--160back to text
- 28 bookUnderstanding molecular simulation.Academic Press2002back to text
-
29
articleRandom walks and an
) volume algorithm for convex bodies.Random Structures & Algorithms1111997, 1--50back to text - 30 bookA guide to Monte Carlo simulations in statistical physics.Cambridge university press2014back to text
- 31 bookFree energy computations: A mathematical perspective.World Scientific2010back to text
- 32 articlePrediction, determination and validation of phase diagrams via the global study of energy landscapes.Int. J. of Materials Research10022009, 135back to textback to text
- 33 articleImproved protein structure prediction using potentials from deep learning.Nature2020, 1--5back to text
- 34 articleAtomic-level characterization of the structural dynamics of proteins..Science33060022010, 341--346URL: http://dx.doi.org/10.1126/science.1187409back to textback to text
- 35 bookEnergy Landscapes.Cambridge University Press2003back to textback to text
- 36 articleBuilding force fields: an automatic, systematic, and reproducible approach.The journal of physical chemistry letters5112014, 1885--1891back to text