2023Activity reportTeamAYANA
Inria teams are typically groups of researchers working on the definition of a common project, and objectives, with the goal to arrive at the creation of a project-team. Such project-teams may include other partners (universities or research institutions).
RNSR: 202023523L- Research center Inria Centre at Université Côte d'Azur
- Team name: AI and Remote Sensing on board for the New Space
- Domain:Perception, Cognition and Interaction
- Theme:Vision, perception and multimedia interpretation
Keywords
Computer Science and Digital Science
- A2.3.1. Embedded systems
- A3.4.1. Supervised learning
- A3.4.2. Unsupervised learning
- A3.4.5. Bayesian methods
- A3.4.6. Neural networks
- A3.4.8. Deep learning
- A5.4.1. Object recognition
- A5.4.5. Object tracking and motion analysis
- A5.4.6. Object localization
- A5.9.2. Estimation, modeling
- A5.9.4. Signal processing over graphs
- A5.9.6. Optimization tools
- A9.3. Signal analysis
Other Research Topics and Application Domains
- B3.3.1. Earth and subsoil
- B3.3.2. Water: sea & ocean, lake & river
- B3.3.3. Nearshore
- B3.4. Risks
- B3.5. Agronomy
- B3.6. Ecology
- B8.3. Urbanism and urban planning
- B8.4. Security and personal assistance
1 Team members, visitors, external collaborators
Research Scientist
- Josiane Zerubia [Team leader, INRIA, Senior Researcher, HDR]
PhD Students
- Louis Hauseux [INRIA, from Oct 2023]
- Jules Mabon [INRIA]
- Martina Pastorino [UniGenoa]
Technical Staff
- Louis Hauseux [INRIA, Engineer, from Feb 2023 until Sep 2023]
Interns and Apprentices
- Priscilla Indira Osa [INRIA, Intern, from Feb 2023 until Jul 2023]
Administrative Assistant
- Nathalie Nordmann [INRIA, from May 2023]
External Collaborators
- Camilo Aguilar Herrera [Digital Barriers, until Jun 2023, R&D engineer]
- Priscilla Indira Osa [UniGenoa, from Nov 2023, PhD Student]
- Zoltan Kato [University of Szeged, Hungary, full Professor, HDR]
- Tianyu Li [Purdue University, until May 2023, PhD student]
2 Overall objectives
The AYANA AEx is an interdisciplinary project using knowledge in stochastic modeling, image processing, artificial intelligence, remote sensing and embedded electronics/computing. The aerospace sector is expanding and changing ("New Space"). It is currently undergoing a great many changes both from the point of view of the sensors at the spectral level (uncooled IRT, far ultraviolet, etc.) and at the material level (the arrival of nano-technologies or the new generation of "Systems on Chips" (SoCs) for example), that from the point of view of the carriers of these sensors: high resolution geostationary satellites; Leo-type low-orbiting satellites; or mini-satellites and industrial cube-sats in constellation. AYANA will work on a large number of data, consisting of very large images, having very varied resolutions and spectral components, and forming time series at frequencies of 1 to 60 Hz. For the embedded electronics/computing part, AYANA will work in close collaboration with specialists in the field located in Europe, working at space agencies and/or for industrial contractors.
3 Research program
3.1 FAULTS R GEMS: Properties of faults, a key to realistic generic earthquake modeling and hazard simulation
Decades of research on earthquakes have yielded meager prospects for earthquake predictability: we cannot predict the time, location and magnitude of a forthcoming earthquake with sufficient accuracy for immediate societal value. Therefore, the best we can do is to mitigate their impact by anticipating the most “destructive properties” of the largest earthquakes to come: longest extent of rupture zones, largest magnitudes, amplitudes of displacements, accelerations of the ground. This topic has motivated many studies in last decades. Yet, despite these efforts, major discrepancies still remain between available model outputs and natural earthquake behaviors. An important source of discrepancy is related to the incomplete integration of actual geometrical and mechanical properties of earthquake causative faults in existing rupture models. We first aim to document the compliance of rocks in natural permanent damage zones. These data –key to earthquake modeling– are presently lacking. A second objective is to introduce the observed macroscopic fault properties –compliant permanent damage, segmentation, maturity– into 3D dynamic earthquake models. A third objective is to conduct a pilot study aiming at examining the gain of prior fault property and rupture scenario knowledge for Earthquake Early Warning (EEW). This research project is partially funded by the ANR Fault R Gems, whose PI is Prof. I. Manighetti from Geoazur. Two successive postdocs (Barham Jafrasteh and Bilel Kanoun) have worked on this research topic funded by UCA-Jedi.
3.2 Probabilistic models on graphs and machine learning in remote sensing applied to natural disaster response
We currently develop novel probabilistic graphical models combined with machine learning in order to manage natural disasters such as earthquakes, flooding and fires. The first model will introduce a semantic component to the graph at the current scale of the hierarchical graph, and will necessitate a new graph probabilistic model. The quad-tree proposed by Ihsen Hedhli in AYIN team in 2016 is no longer fit to resolve this issue. Applications from urban reconstruction or reforestation after natural disasters will be achieved on images from Pleiades optical satellites (provided by the French Space Agency, CNES) and CosmoSKyMed radar satellites (provided by the Italian Space Agency, ASI). This project is conducted in partnership with the University of Genoa (Prof. G. Moser and Prof. S. Serpico) via the co-supervision of a PhD student, financed by the Italian government. The PhD student, Martina Pastorino, has worked with Josiane Zerubia and Gabriele Moser in 2020 during her double Master degree at both University Genoa and IMT Atlantique. She has been a PhD Student in co-supervision between University of Genoa DITEN (Prof. Moser) and Inria (Prof. Zerubia) in November 2020 until December 2023.
3.3 Marked point process models for object detection and tracking in temporal series of high resolution images
The model proposed by Paula Craciun's PhD thesis in 2015 in AYIN team, supposed the speed of tracked objects of interest in a series of satellite images to be quasi-constant between two frames. However, this hypothesis is very limiting, particularly when objects of interest are subject to strong and sudden acceleration or deceleration. The model we proposed within AYIN team is then no longer viable. Two solutions will be considered within AYANA team : either a generalist model of marked point processes (MPP), supposing an unknown and variable velocity, or a multimodel of MPPs, simpler with regard to the velocity that can have a very limited amount of values (i.e., quasi-constant velocity for each MPP). The whole model will have to be redesigned, and is to be tested with data from a constellation of small satellites, where the objects of interest can be for instance "speed-boats" or "go-fast cars". Some comparisons with deep learning based methods belonging to Airbus Defense and Space (Airbus DS) are planned at Airbus DS. Then this new model should be brought to diverse platforms (ground based or on-board). The modeling and ground-based application part related to object detection has been studied within the PhD thesis of Jules Mabon. Furthermore, the object-tracking methods have been proposed by AYANA as part of a postdoctoral project (Camilo Aguilar-Herrera). Finally, the on-board version will be developed by Airbus DS, and the company Erems for the onboard hardware. This project is financed by Bpifrance within the LiChIE contract. This research project has started in 2020 for a duration of 5 years.
4 Application domains
Our research is applied within all Earth observation domains such as: urban planning, precision farming, natural disaster management, geological features detection, geospatial mapping, and security management.
5 Highlights of the year
- Josiane Zerubia, as IAPR Fellow, was both highlighted by IAPR Newsletter with a “Getting to Know” and another “Her Story” articles for contributions to stochastic image modeling for classification, segmentation, and object detection in remote sensing.
- Josiane Zerubia was highlighted as “Femme inspirante” by Femmes & Sciences association, see the article.
- Josiane Zerubia was interwied for IEEE Signal Processing Newsletter.
6 New software, platforms, open data
6.1 New software
6.1.1 MPP & CNN for object detection in remotely sensed images
-
Name:
Marked Point Processes and Convolutional Neural Networks for object detection in remotely sensed images
-
Keywords:
Detection, Satellite imagery
-
Functional Description:
Implementation of the work presented in: "CNN-based energy learning for MPP object detection in satellite images" Jules Mabon, Mathias Ortner, Josiane Zerubia In Proc. 2022 IEEE International Workshop on Machine Learning for Signal Processing (MLSP) and "Point process and CNN for small objects detection in satellite images" Jules Mabon, Mathias Ortner, Josiane Zerubia In Proc. 2022 SPIE Image and Signal Processing for Remote Sensing XXVIII
- URL:
-
Contact:
Jules Mabon
-
Partner:
Airbus Defense and Space
6.1.2 FCN and Fully Connected NN for Remote Sensing Image Classification
-
Name:
Fully Convolutional Network and Fully Connected Neural Network for Remote Sensing Image Classification
-
Keywords:
Satellite imagery, Classification, Image segmentation
-
Functional Description:
Code related to the paper:
M. Pastorino, G. Moser, S. B. Serpico, and J. Zerubia, "Fully convolutional and feedforward networks for the semantic segmentation of remotely sensed images," 2022 IEEE International Conference on Image Processing, 2022,
- URL:
-
Contact:
Josiane Zerubia
-
Partner:
University of Genoa, DITEN, Italy
6.1.3 Stabilizer for Satellite Videos
-
Keywords:
Satellite imagery, Video sequences
-
Functional Description:
A python-implemented stabilizer for satellite videos. This code was used to produce the object tracking results shown in: C. Aguilar, M. Ortner and J. Zerubia, "Adaptive Birth for the GLMB Filter for object tracking in satellite videos," 2022 IEEE 32st International Workshop on Machine Learning for Signal Processing (MLSP), 2022, pp. 1-6
- URL:
-
Contact:
Josiane Zerubia
-
Partner:
Airbus Defense and Space
6.1.4 GLMB filter with History-based Birth
-
Name:
Python GLMB filter with History-based Birth
-
Keywords:
Multi-Object Tracking, Object detection
-
Functional Description:
Implementation of the work presented in: C. Aguilar, M. Ortner and J. Zerubia, "Adaptive Birth for the GLMB Filter for object tracking in satellite videos," 2022 IEEE 32st International Workshop on Machine Learning for Signal Processing (MLSP), 2022, pp. 1-6
- URL:
-
Contact:
Josiane Zerubia
-
Partner:
Airbus Defense and Space
6.1.5 Automatic fault mapping using CNN
-
Keyword:
Detection
-
Functional Description:
Implementation of the work published in: Bilel Kanoun, Mohamed Abderrazak Cherif, Isabelle Manighetti, Yuliya Tarabalka, Josiane Zerubia. An enhanced deep learning approach for tectonic fault and fracture extraction in very high resolution optical images. ICASSP 2022 - IEEE International Conference on Acoustics, Speech, & Signal Processing, IEEE, May 2022, Singapore/Hybrid, Singapore.
- URL:
-
Contact:
Josiane Zerubia
-
Partner:
Géoazur
6.1.6 RFS-filters for Satellite Videos
-
Keywords:
Detection, Target tracking
-
Functional Description:
Implementation of the works published in Camilo Aguilar, Mathias Ortner, Josiane Zerubia. Enhanced GM-PHD filter for real time satellite multi-target tracking. ICASSP 2023 – IEEE International Conference on Acoustics, Speech, and Signal Processing, Jun 2023, Rhodes, Greece.
- URL:
-
Contact:
Camilo Aguilar Herrera
-
Partner:
Airbus Defense and Space
7 New results
7.1 Learning CRF potentials through fully convolutional networks for satellite image semantic segmentation
Participants: Martina Pastorino, Josiane Zerubia.
External collaborators: Gabriele Moser [Genoa University, DITEN, Professor], Sebastiano Serpico [Genoa University, DITEN, Professor].
Keywords: image processing, stochastic models, deep learning, fully convolutional networks, multiscale analysis.
The work carried out consisted in the development of a method to automatically learn the unary and pairwise potentials of a conditional random field (CRF) from the input data in a non-parametric fashion, within the framework of the semantic segmentation of remote sensing images. The proposed model is based on fully convolutional networks (FCNs) and fully connected neural networks (FCNNs) to leverage the modeling capabilities of deep learning (DL) architectures to directly learn semantic relationships from the input data and extensively exploit the semantic and spatial information contained in the input data and in the intermediate layers of an FCN. The idea of the model is twofold: first to learn the statistics of a categorical-valued CRF via a convolutional layer, whose kernel defines the clique of interest, and, second, to favor the interpretability of the intermediate layers as posterior probabilities through the FCNNs. The multiscale information extracted by the FCN is explicitly modeled through the addition of the FCNNs at different scales.
The method was tested with the ISPRS 2D Semantic Labeling Challenge Vaihingen dataset, after modifying the ground truths to approximate the ones found in realistic remote sensing applications, characterized by scarce and spatially non-exhaustive annotations. The results (see Fig. 1) confirm the effectiveness of the proposed technique for the semantic segmentation of satellite images. This method is an extension of the method presented in 22.
The results were presented at SITIS'237 (Bangkok, Thailand) and submitted on IEEE TGRS21.
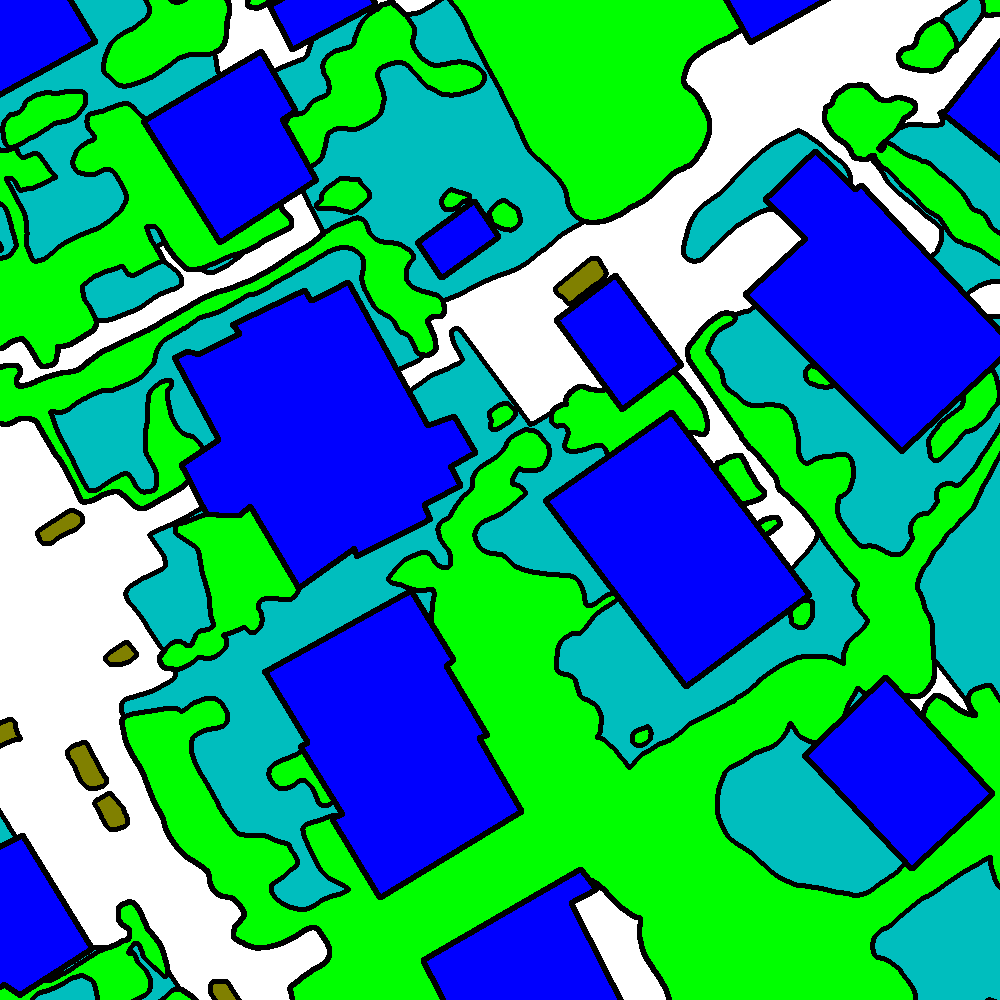
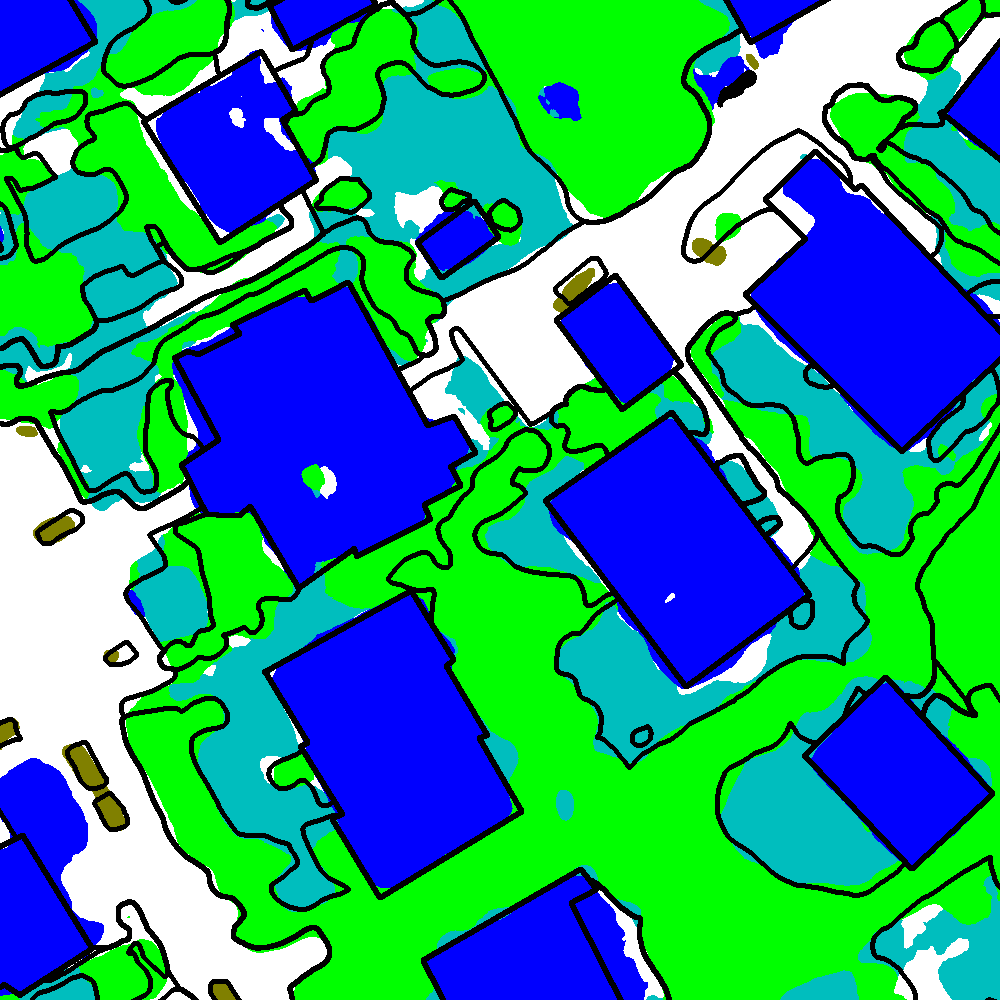
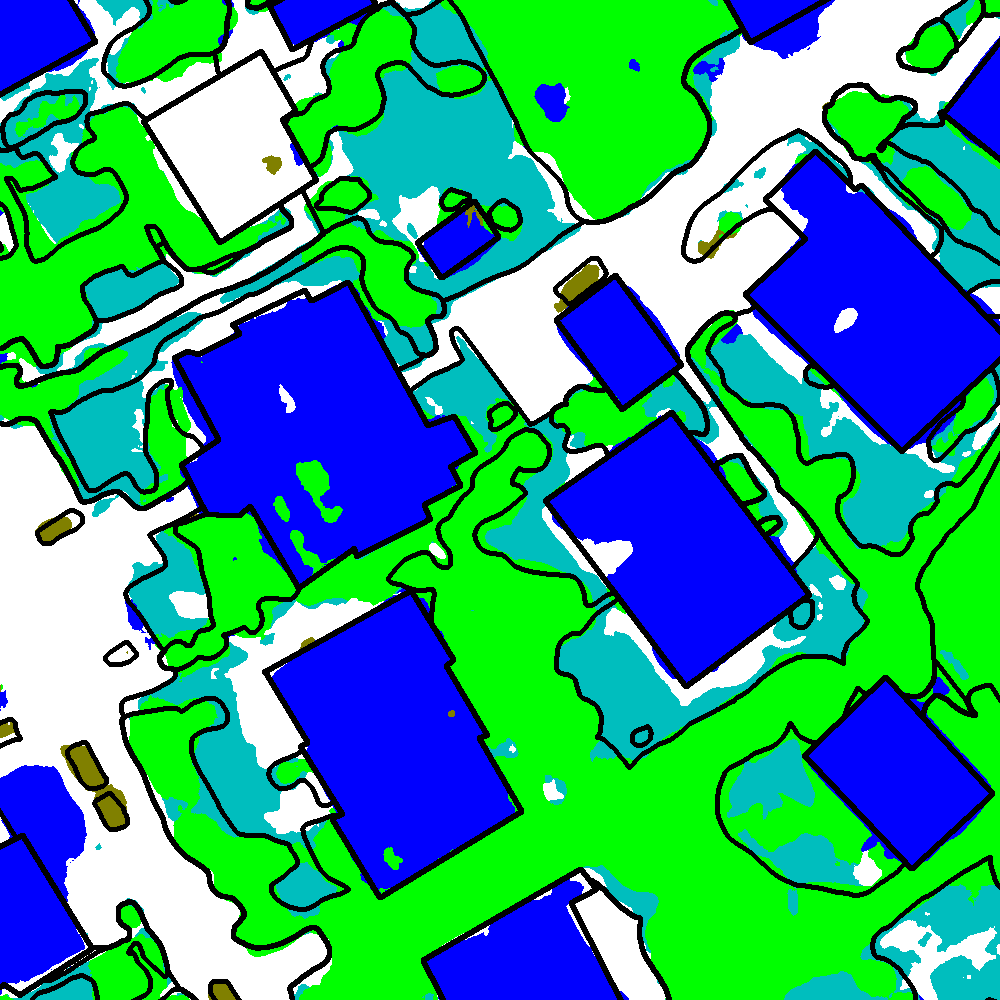
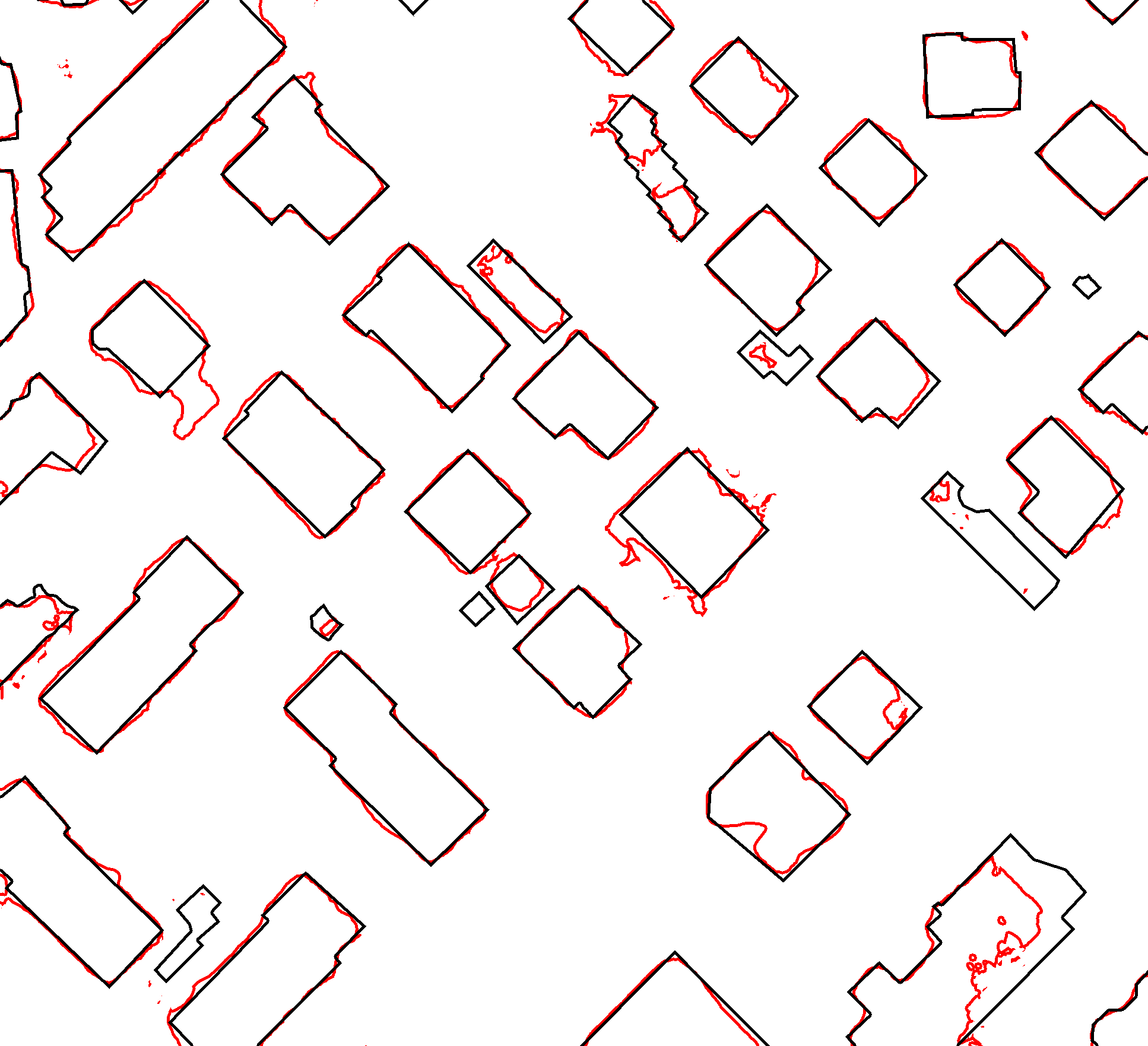
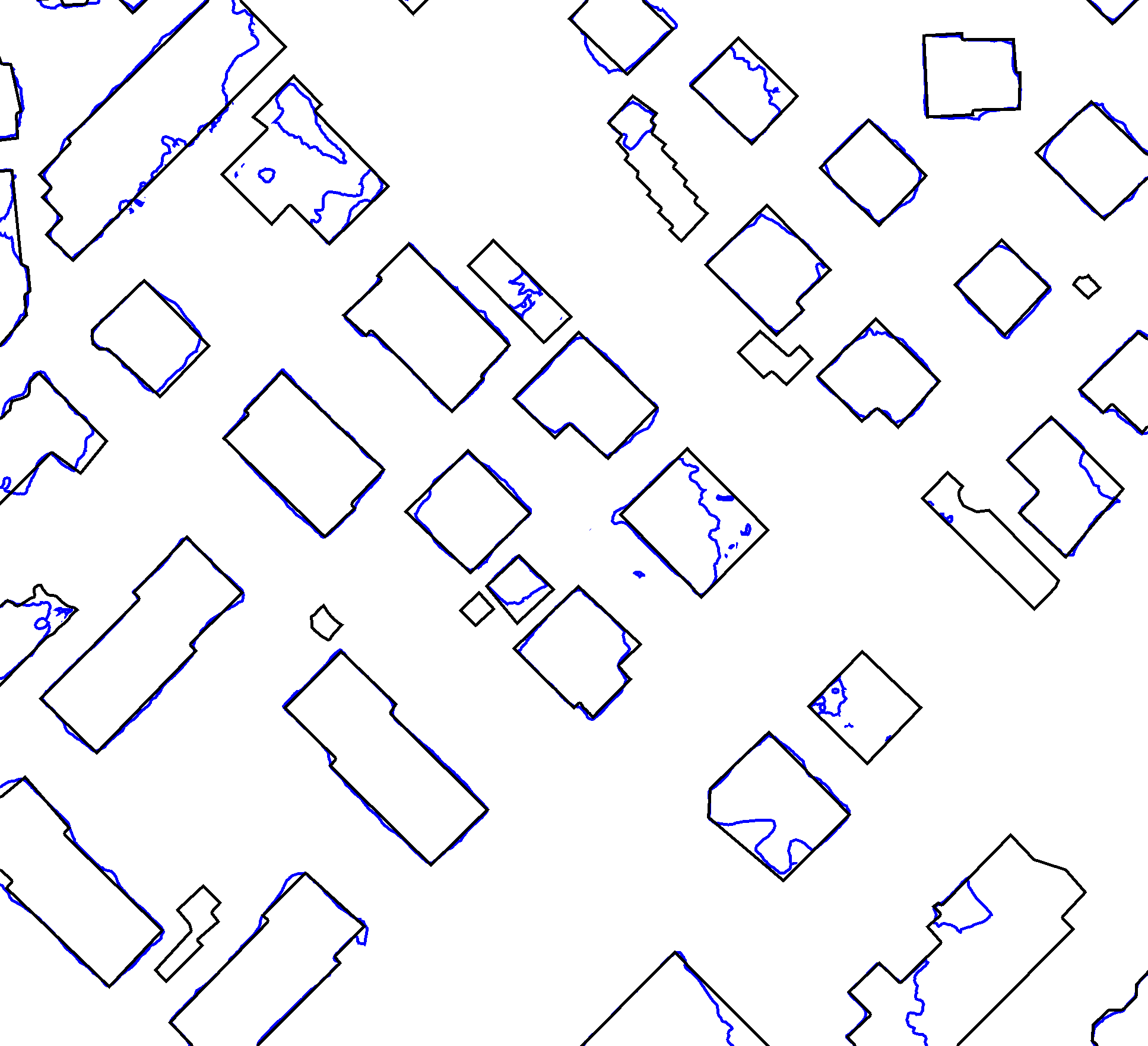
7.2 Hierarchical probabilistic graphical models and fully convolutional networks for the semantic segmentation of multimodal SAR images
Participants: Martina Pastorino, Josiane Zerubia.
External collaborators: Gabriele Moser [Genoa University, DITEN, Professor], Sebastiano Serpico [Genoa University, DITEN, Professor].
Keywords: image processing, stochastic models, deep learning, fully convolutional networks, multimodal radar images.
The method developed and tested on the optical dataset in 24 was extended to deal with synthetic aperture radar (SAR) images, in the framework of a research project funded by the Italian Space Agency (ASI). This SAR images are multimission (SAOCOM, COSMO-SkyMed, and Sentinel-1) and were collected with different modalities (TOPSAR and StripMap), bands (L, X, and C), polarizations, and several trade-offs between resolution and coverage (see Fig. 2). The possibility to deal with multimodal images offers great prospects for land cover mapping applications in the field of remote sensing. However, the development of a supervised method for the classification of SAR images – which suffer from speckle and are determined by complex scattering phenomena – presents some issues.
The results obtained by the proposed technique were also compared with those of HRNet 27, a network consisting of multiresolution subnetworks connected in parallel, and of the light-weight attention network (LWN-Attention) 19, a multiscale feature fusion method making use of multiscale information through the concatenation of feature maps associated with different scales. The proposed method, leveraging both hierarchical and long-range information attained generally higher average classification results, thus suggesting that the proposed integration of FCN and hierarchical PGM can be advantageous.
The work validated on COSMO-SkyMed and SAOCOM data was presented at IEEE IGARSS'23 in Pasadena (CA), United States 6, and the work validated on the three satellites was submitted to IEEE GRSL23.
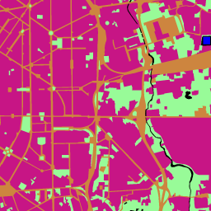
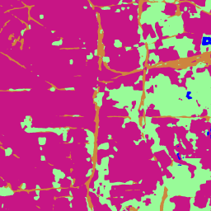
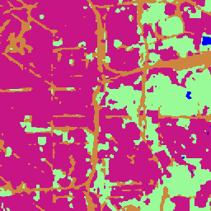
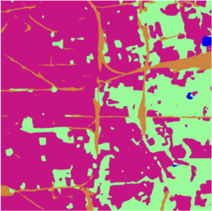
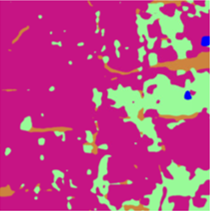
7.3 A probabilistic fusion framework for burnt zones mapping from multimodal satellite and UAV imagery
Participants: Martina Pastorino, Josiane Zerubia.
External collaborators: Gabriele Moser [Genoa University, DITEN, Professor], Sebastiano Serpico [Genoa University, DITEN, Professor].
Keywords: image processing, stochastic models, deep learning, satellite images, UAV imagery.
This work tackles the semantic segmentation of zones affected by fires by the introduction of methods incorporating multimodal UAV and spaceborne imagery. The multiresolution fusion task is especially challenging in this case because the difference between the involved spatial resolutions is very large – a situation that is normally not addressed by traditional multiresolution schemes.
The increasing occurrence of wildfires, amplified by the changing climate conditions and drought, has significant impacts on natural resources, ecosystem responses, and climate change. In this framework, deriving the extent of areas affected by wildfires is crucial not only to prevent further damage but also to manage the area itself. The joint availability of satellite and UAV acquisitions of zones affected by wildfires, with their complementary features, presents a huge potential for the mapping and monitoring of burnt areas and, at the same time, a big challenge for the development of a classifier capable to fully take advantage of this multimodal information.
To address the huge contrast between the resolutions of the available input image sources, two different methods combining stochastic modeling, decision fusion, and aspects related to machine learning and deep learning were developed. The first method proposes a pixelwise probabilistic fusion of the multiscale information after being analyzed separately. The second considers multiresolution fusion in a partially regular quadtree graph topology through the marginal posterior mode (MPM) criterion, an extension of the approach described in 24 to the case of extremely large resolution ratio. The application is a real case of fire zone mapping and management in the area of Marseille, France (see Figure 3). The data available for the segmentation of burnt zones was provided by the INRAE (Institut National de Recherche pour l'Agriculture, l'Alimentation et l'Environnement) Provence-Alpes-Côte d'Azur research centre, Aix-en-Provence.
A conference paper related to this work has been submitted to IEEE IGARSS'24.
Martina Pastorino PhD thesis on Probabilistic graphical models and deep learning methods for remote sensing image analysis was successfully defended on December 12, 2023, and is in process of being published.
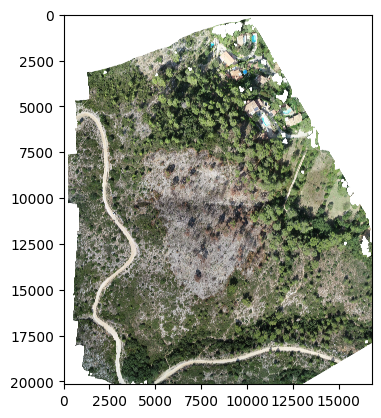
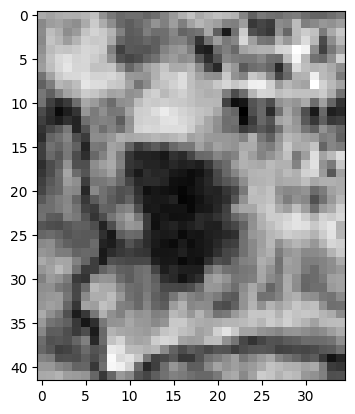
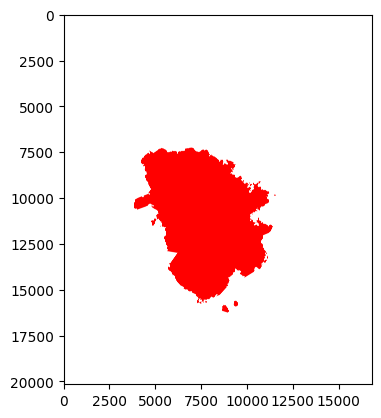
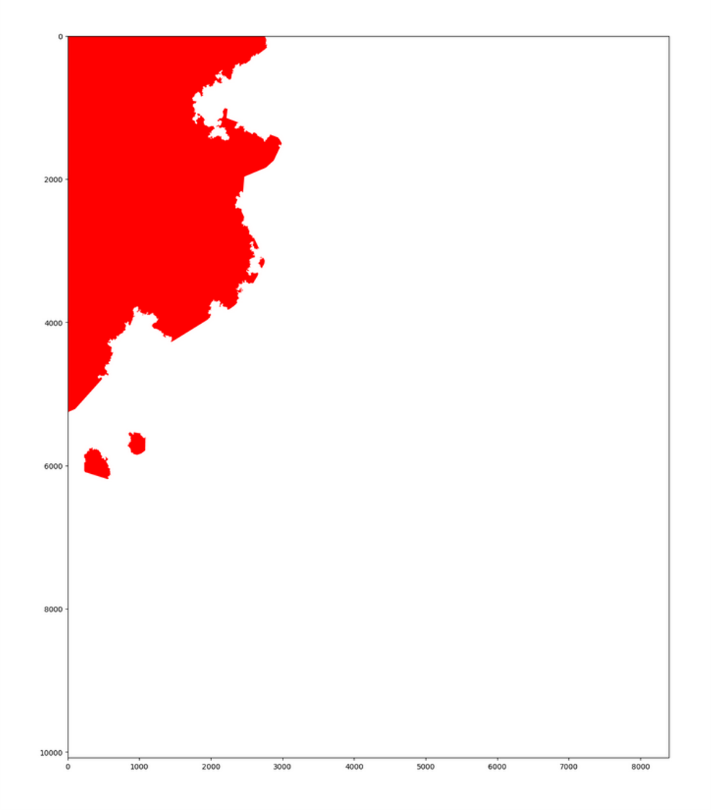
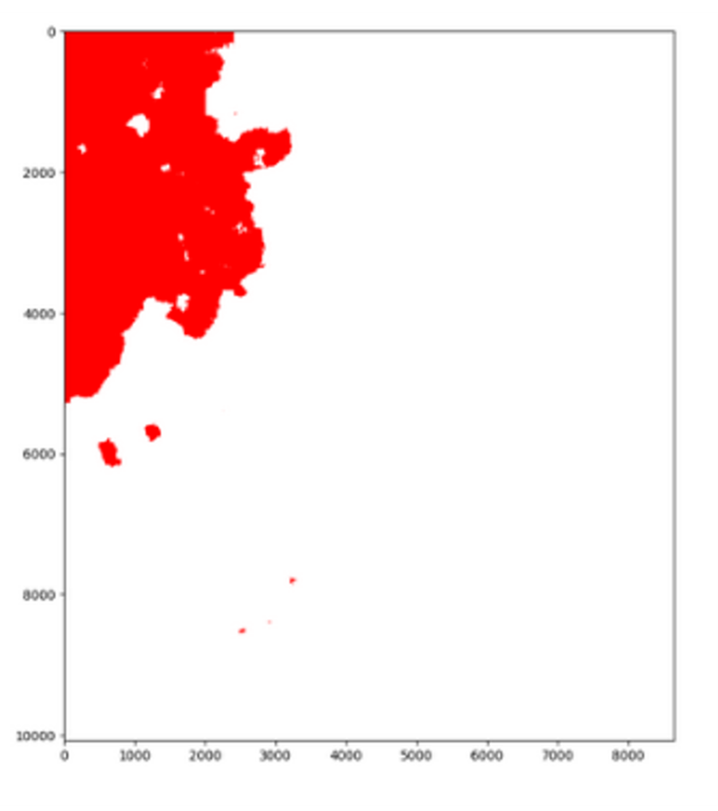
7.4 Gabor feature network for CNN-based and transformer-based bitemporal building change detection models
Participants: Priscilla Indira Osa, Josiane Zerubia.
External collaborators: Zoltan Kato [University of Szeged, Professor].
Keywords: Gabor feature, CNN, transformer, building change detection, remote sensing.
Bitemporal building change detection (BCD) in remote sensing (RS) imagery requires distinct features of the buildings to assist the model highlighting the changes of buildings and ignoring the irrelevant ones from other objects or dynamic sensing conditions. Buildings can be characterized by their shapes that form consistent textures when being observed from bird's eye view, compared to other common objects found on RS images. Moreover, satellite and aerial imagery usually cover a wide range of area which makes buildings appear as repetitive visual patterns. Based on these characteristics, Gabor Feature Network (GFN) was proposed to extract the discriminative texture features of buildings. GFN is based on Gabor filter 15, a traditional image processing filter that is excellent in extracting repetitive texture patterns on an image. GFN aims not only to maximize the network ability to capture the textures of buildings, but also to reduce the noise caused by insignificant changes such as those by color or weather conditions. Complementarily, Feature Fusion Module (FFM) was designed to merge the extracted multi-scale features from GFN with the features from the encoder of a deep learning model to preserve the extracted building textures in the deep intermediate layers of the network.
Using GFN and FFM, we built two BCD models: Transformer-based GabFormer and CNN-based AYANet. Fig.4 shows the overall architecture of the models. Both proposed models were compared with the State-of-the-Art (SOTA) models: STANet-PAM 14, BIT 13, and ChangeFormer 12 which represent a pure CNN model, a hybrid CNN-Transformer model, and a pure Transformer model, respectively. The experimental results on the LEVIR-CD 14 and WHU-CD 16 datasets indicated that both of proposed models outperform other State-of-the-Art (SOTA) models (Fig.5 shows the visualization of the ground truth and predictions of all models on some samples of images). Moreover, the cross-dataset evaluation suggested a better generalization capability of the proposed models compared to the SOTAs considered in the experiment.
A conference paper related to this work has been submitted to ICIP'24.
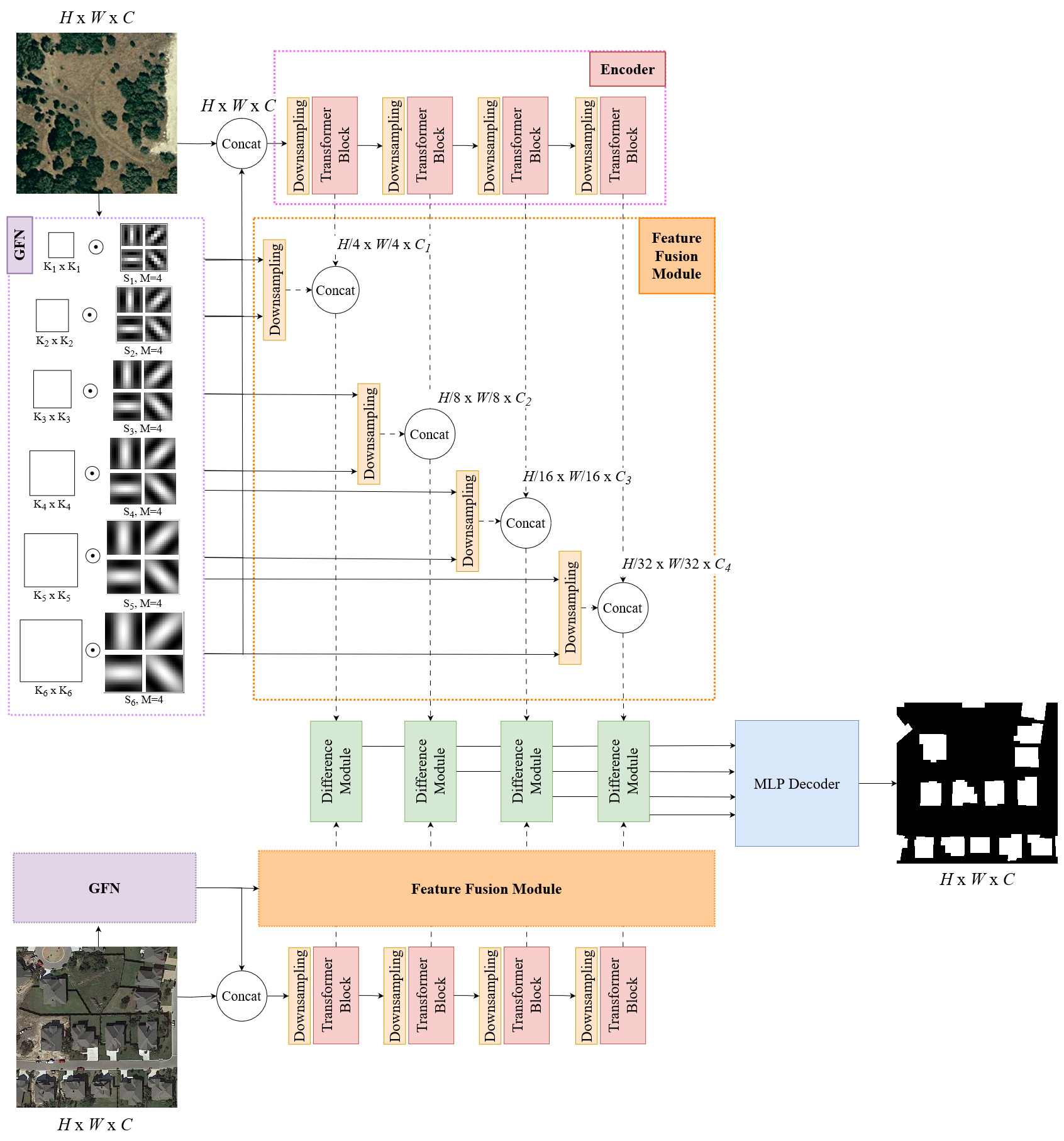
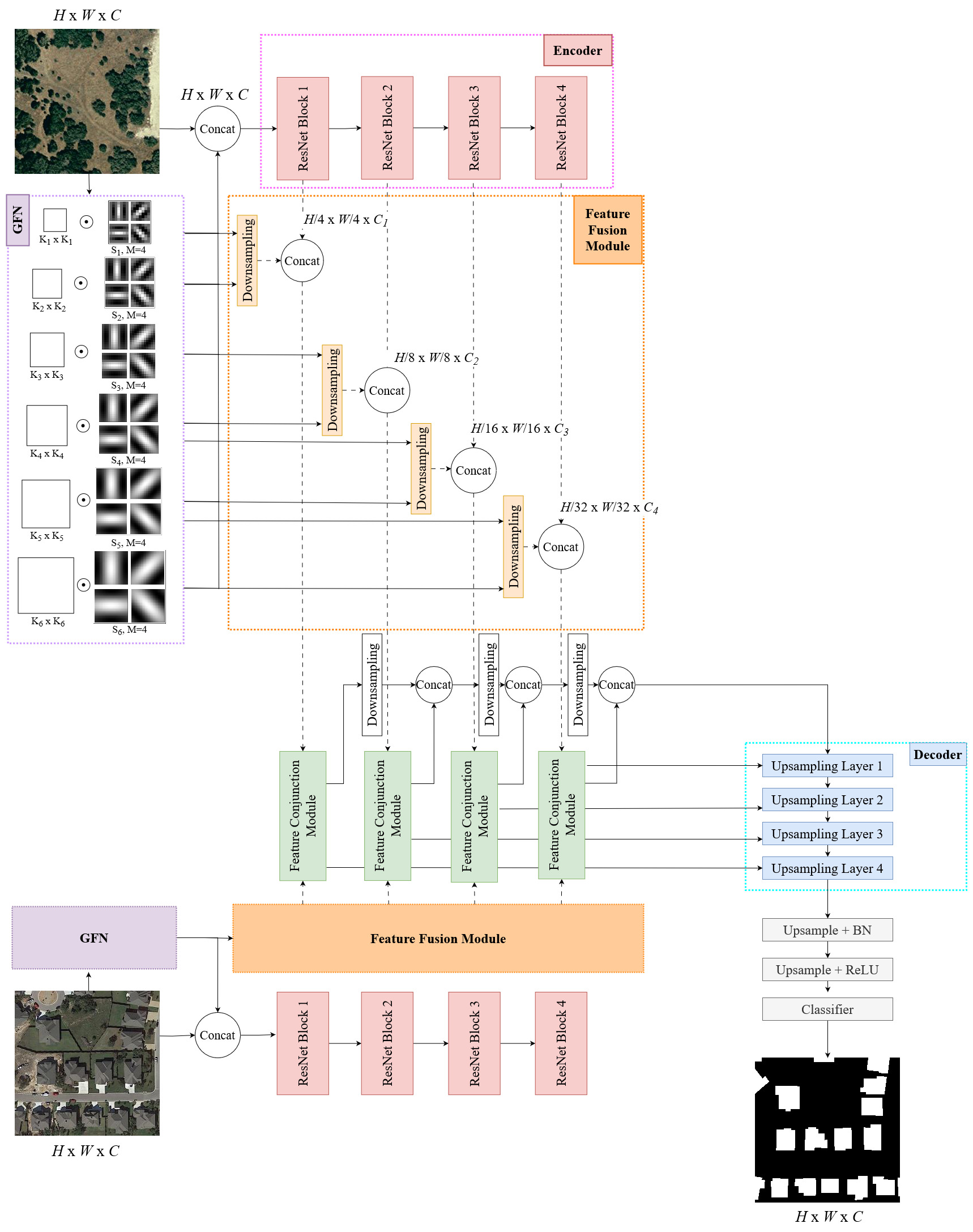
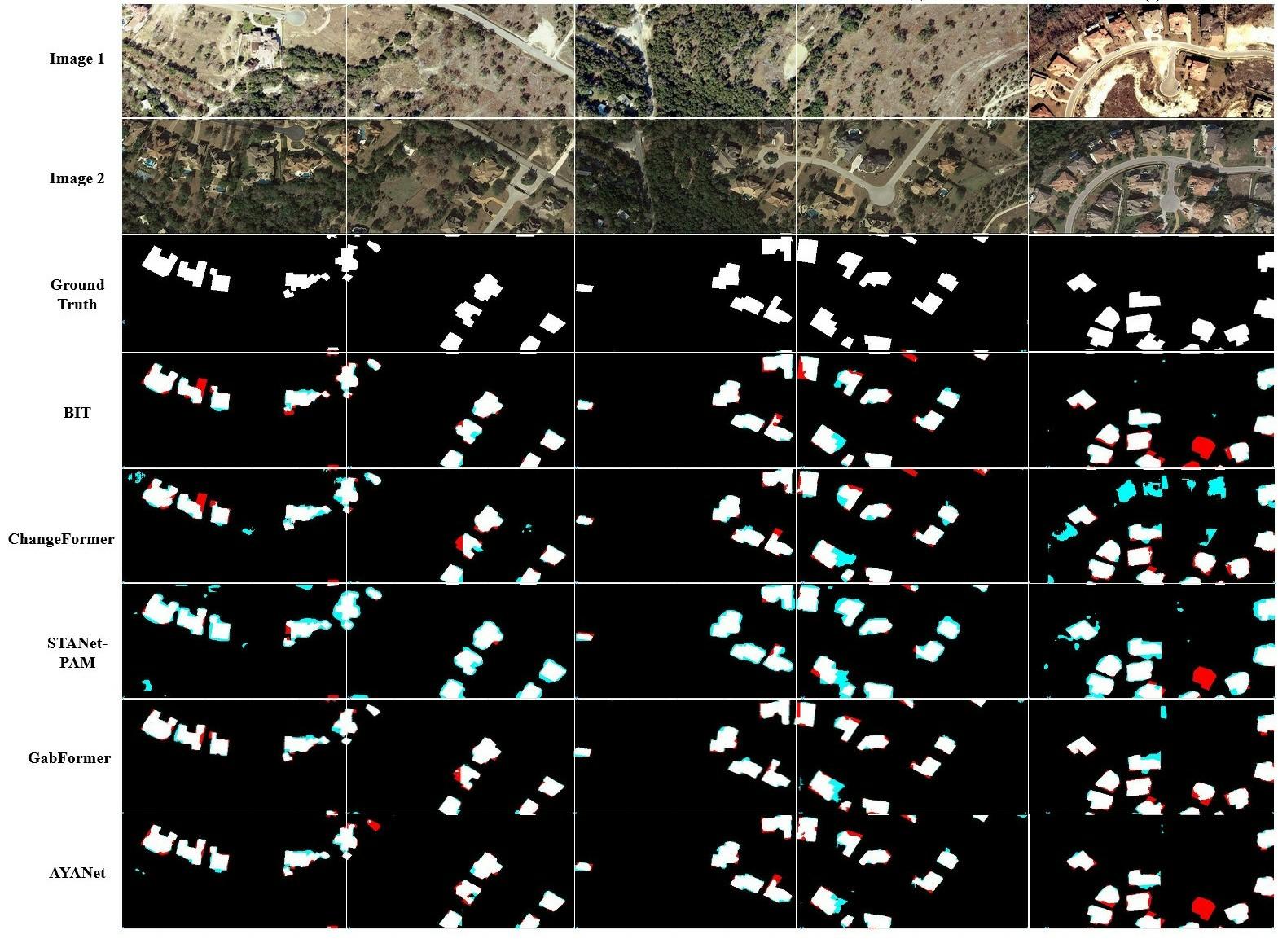
7.5 Learning stochastic geometry models and convolutional neural networks. Application to multiple object detection in aerospatial data sets.
Participants: Jules Mabon, Josiane Zerubia.
External collaborators: Mathias Ortner [Airbus Defense and Space, Senior Data Scientist].
Keywords: object detection, deep learning, fully convolutional networks, stochastic models, energy based models.
Unmanned aerial vehicles and low-orbit satellites, including cubesats, are increasingly used for wide area surveillance, which results in large amounts of multi-source data that have to be processed and analyzed. These sensor platforms capture vast ground areas at frequencies varying from 1 to 60 Hz depending on the sensor. The number of moving objects in such data is typically very high, accounting for up to thousands of objects.
In this work 9, we propose the incorporation of interaction models into object detection methods, while taking advantage of the capabilities of deep convolutional neural networks. Satellite images are inherently imperfect due to atmospheric disturbances, partial occlusions and limited spatial resolution. To compensate for this lack of visual information, it becomes essential to incorporate prior knowledge about the layout of objects of interest.
On the one hand, methods based on CNN are excellent for extracting patterns in images, but struggle to learn object-to-object interaction models without having to introduce attention mechanisms such as Transformers that considerably increase the complexity of the model. For example, some approaches propose to include prior information in the form of descriptive text about objects and their relationships 20, while others use cascades of attention modules 32.
On the other hand, Point Processes propose to jointly solve the likelihood relative to the image (data term), and consistency of the object configuration itself (prior term). Firstly, Point Process approaches model configurations as vector geometry, constraining the state space by construction. In addition, these models allow explicit priors relative to configurations to be specified as energy functions. However, in most of the literature 28, 25, data terms rely on contrast measures between objects of interest and background.
Instead of increasing the complexity of the model by adding, for example, Transformers, we propose to combine CNN pattern extraction with the Point Process approach. The starting point of this approach is to use the output of a CNN as the data term for a Point Process model. From the latter we derive more efficient sampling methods for the Point Process that do not rely on application specific heuristics. Finally, we propose to bridge the gap in terms of parameters estimation using modern learning techniques inspired from Energy Based Models (EBM); namely contrastive divergence. In 8 and 5 we formalize how to use pretrained CNN to build potentials for the point process model while developing the parameter estimation method. In Figure 7 and 6 we show results of our method on both Airbus Defense and Space data, as well as the DOTA benchmark 29.
This work has been presented at SITIS'235 (Bangkok, Thailand) and GRETSI'238 (Grenoble, France).
Jules Mabon PhD thesis on Learning stochastic geometry models and convolutional neural networks. Application to multiple object detection in aerospatial data sets was successfully defended on December 20, 2023, and has been published 9.
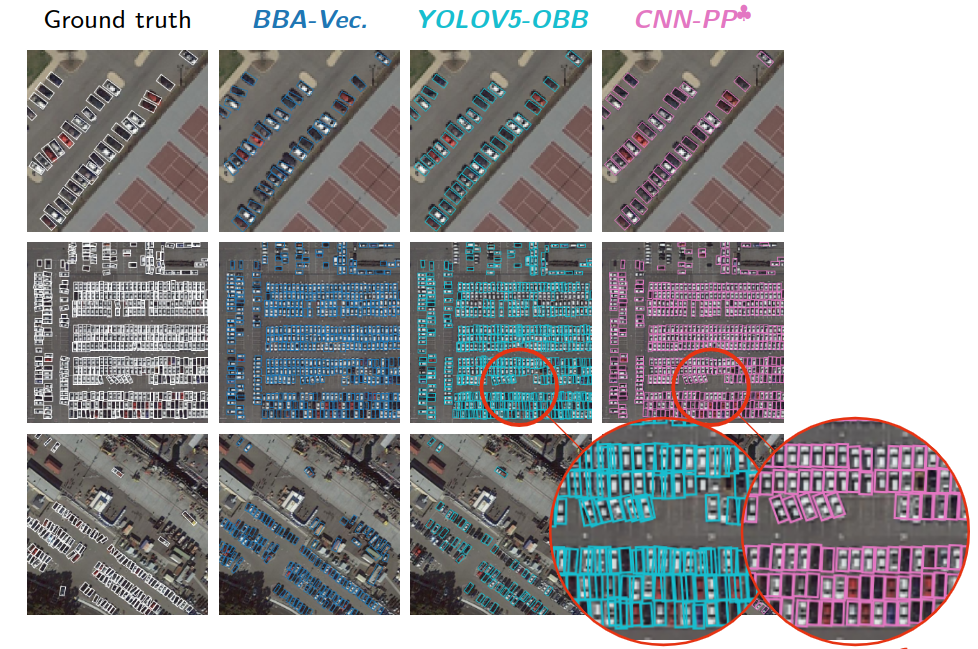
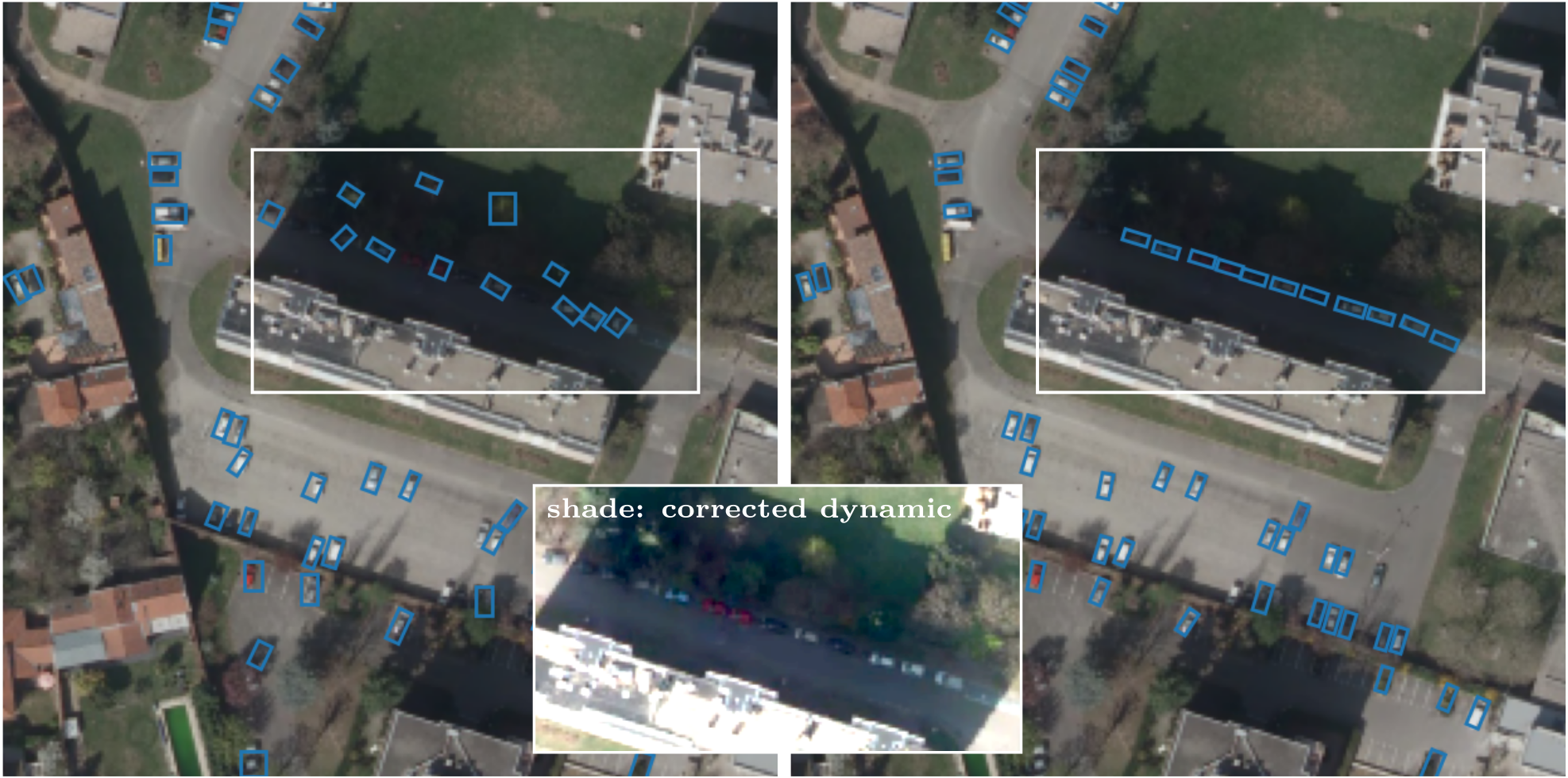
7.6 Small object detection and tracking in satellite videos
Participants: Camilo Aguilar, Josiane Zerubia.
External collaborators: Mathias Ortner [Airbus Defense and Space, Senior Data Scientist].
Keywords: image processing, object tracking, multi-target tracking, convolutional neural networks, Gaussian mixture probability hypothesis density.
Multi-target tracking (MTT) estimates the number of objects and their states from observations. Applications include the areas of security surveillance, autonomous driving, and remote sensing videos.
Despite significant advancements in computer vision, detecting and tracking objects in large ground areas presents several challenges. Targets span between 5 to 15 pixels in width and often lack discriminative features. Furthermore, objects move at varying directions and speeds; a target in a high-speed road reduces its velocity drastically when approaching intersections. Additionally, satellite videos present sources of artifacts such as clutter detections and misdetections. For example trees, roofs, containers, or small structures could become false positive detections.
We present a real-time multi-object tracker using an enhanced version of the Gaussian mixture probability hypothesis density (GM-PHD) filter to track detections of a state-of-the-art convolutional neural network (CNN). This approach adapts the GM-PHD filter to a real-world scenario to recover target trajectories in remote sensing videos. Our GM-PHD filter uses a measurement-driven birth, considers past tracked objects, and uses CNN information to propose better hypotheses initialization. Additionally, we present a label tracking solution for the GM-PHD filter to improve identity propagation given target path uncertainties. Our results show competitive scores against other trackers while obtaining real-time performance.
In this work 3, we propose three improvements to original GM-PHD filter in order to better track noisy CNN-based detections. First, we improve the traditional label tracking for the GM-PHD filter. Second, we adapt hypothesis birth distribution by using a measurement-driven and a history-based birth. Finally, we improve the birth initialization using deep learning-based information. We show that our approach obtains better tracking scores than competing tracking or joint tracking-detection methods for satellite images while preserving real-time performance (See Fig. 8).
This work 3 was presented at the ICASSP'23 conference in Rhodes, Greece.
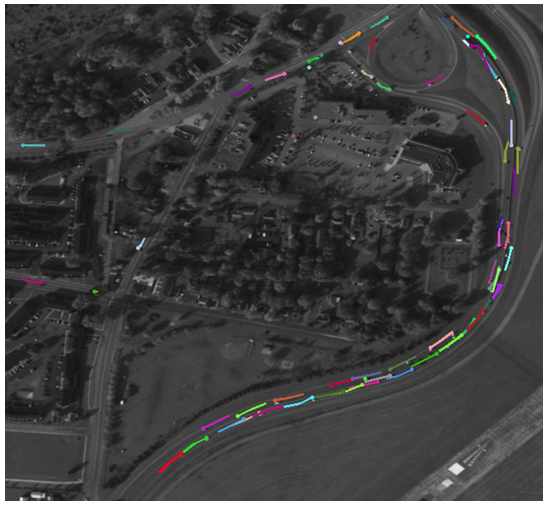
7.7 Graph based approach for galaxy filament extraction
Participants: Louis Hauseux, Josiane Zerubia.
External collaborators: Konstantin Avrachenkov [Centre Inria d'Université Côte d'Azur, NEO Team].
Keywords: geometric graphs, continuum percolation, Fréchet mean, galaxy filaments.
We propose 4 an original density estimator built from a cloud of points . To do this, we consider geometric graphs on the cloud. These graphs depend on a radius . By varying the radius, we see the emergence of large components around certain critical radii, which is the phenomenon of continuum percolation. Percolation allows us to have both a local view of the data (through local constraints on the radius ) and a global one (the emergence of macrostructures).
Since continuum percolation is a very fast phenomenon, our estimator is able to identify two neighboring levels of close density.
With this tool, we address the problem of galaxy filament extraction. The density estimator gives us a relevant graph on galaxies. With an algorithm sharing the ideas of the Fréchet mean, we extract a subgraph from this graph, the galaxy filaments.
Compared with conventional density estimators for this type of problem, it is already showing very good results, especially for high-density clusters.
In the future, we will try to further improve these results focusing on three principal research directions. First, we mainly looked at one type of clusters, `filaments' 4. Having an estimator of density levels allows us to look at other types, such as `super-clusters', `walls' and `voids'. Second, a galaxy was represented only by a point 4. Its mass (represented by its luminosity) could be taken into account using different radii, depending on galaxies.
Third, we worked with graphs 4. We could look at other notions of connectivity (e.g. connectivity of simplicial complexes, also known as hypergraphs).
We show an example of such filament extraction on a synthetic 2D-image of galaxies. At a glance, we compare our results with a stochastic method 26 (see Fig. 9).
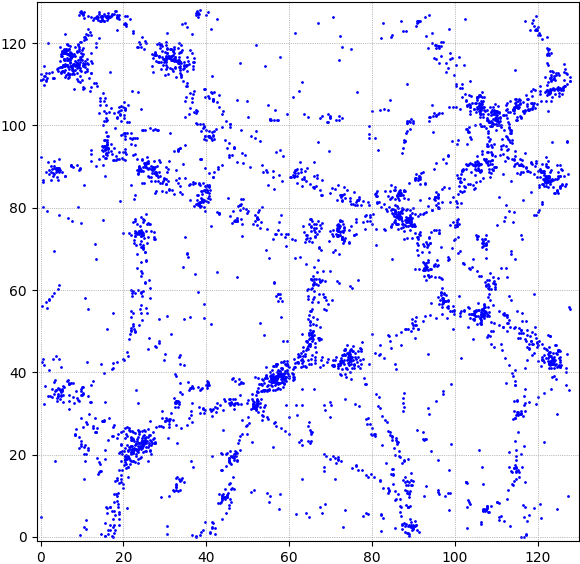
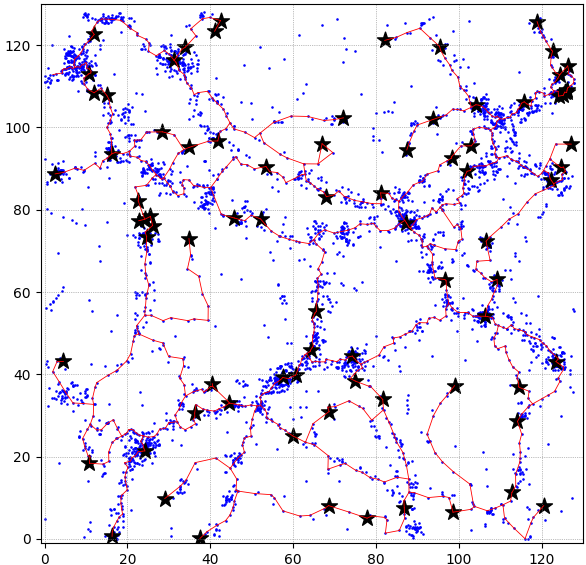
This work has been presented at the Complex Networks 2023 conference in Menton, France 4.
7.8 Curvilinear structure detection in images by connected-tube marked point process
Participants: Josiane Zerubia.
External collaborators: Tianyu Li [Purdue University, US, PhD student], Mary Comer [Purdue University, US, Associate professor].
Keywords: remote sensing, road segmentation, convolutional neural network (CNN), two stage learning.
This work consisted of the development of a two-stage learning strategy for road segmentation in remote sensing images. With the development of new technology in satellites, cameras and communications, massive amounts of high-resolution remotely-sensed images of geographical surfaces have become accessible to researchers. To help in analyzing these massive image datasets, automatic road segmentation has become more and more important.
Unsupervised stochastic approaches, using a Marked Point Process (MPP), have been proposed for curvilinear structure detection. 17 proposed the Quality Candy model for line network extraction. Then, 25 presented a forest point process for the automatic extraction of networks. These methods address the two tasks of curvilinear structure detection at the same time and present good results on tasks such as road detection and river detection in satellite images.
However, they describe the relationship between different line segments in a complex way that results in calculation burden on their optimization algorithm.
To make the MPP based unsupervised model simpler and more efficient, we propose a 2D connected-tube MPP model, which incorporates a connection prior that favors certain connections between tubes. We test the connected-tube MPP model on fiber detection in material images by combining it with an ellipse MPP model. Also, we test it on satellite images to show its potential to detect road networks.
Moreover, we find that the binary segmentation by the connected-tube MPP model alone may not be very accurate as the segmentation is object based rather than pixel based. To improve the segmentation accuracy, we propose a binary segmentation algorithm based on the detected tube networks. We test this method on STARE and DRIVE databases for vessel network detection in retina images (see Fig. 10).
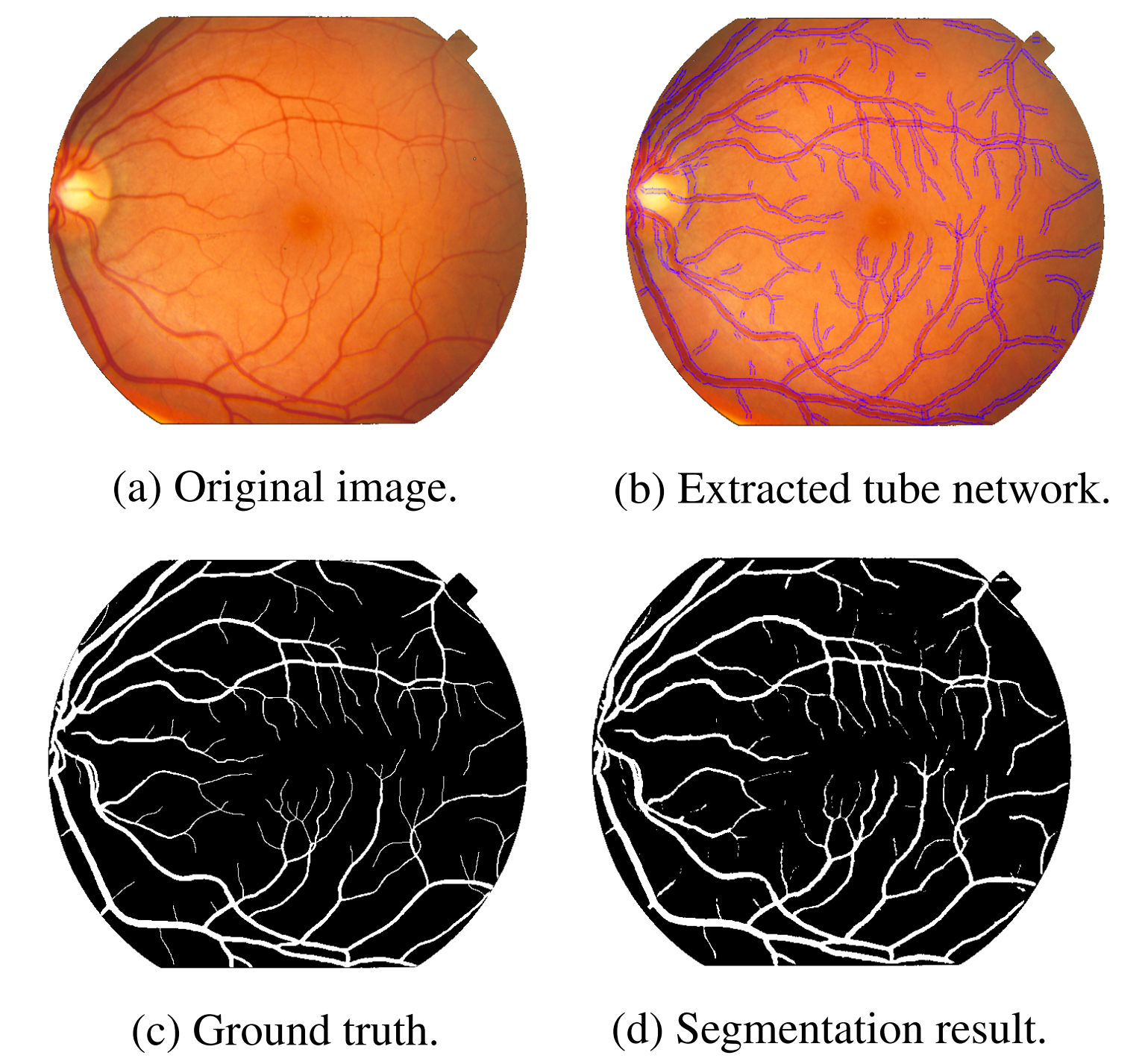
To investigate the supervised curvilinear structure detection method, we focus on the application of road detection in satellite images and propose a two-stage learning strategy for road segmentation. A probability map is generated in the first stage by a selected neural network, then we attach the probability map image to the original RGB images and feed the resulting four images to a U-Net-like network in the second stage to get a refined result. Our experiments on the Massachusetts road dataset show the average Intersection over Union (IoU) can increase up to 3% from stage one to stage two, which achieves state-of-the-art results on this dataset. Moreover, from the qualitative results, obvious improvements from stage one to stage two can be seen: fewer false positives and better connection of road lines (see Fig. 11).
Furthermore, we extend the 2D tube model to 3D tube model, with each tube modeled as a cylinder. The 3D tube MPP model can be directly applied to fiber detection in microscopy images without post processing to connect the 2D results. We achieve promising result on synthesized data.
Tianyu Li obtained his PhD degree from Purdue University in May 2023 on Curvilinear structure detection in images by connected-tube Marked Point Process and anomaly detection in time series18.
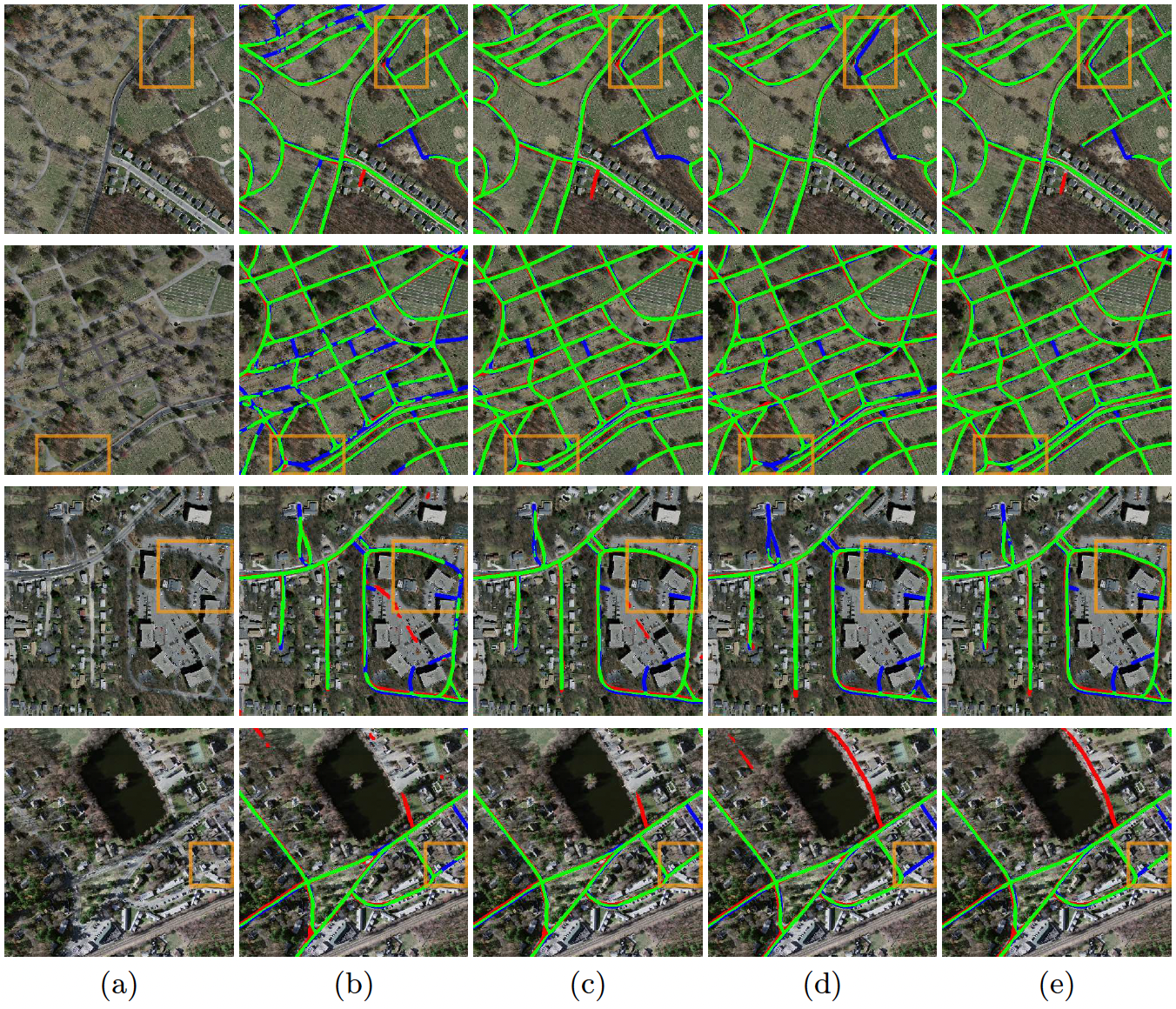
8 Bilateral contracts and grants with industry
8.1 Bilateral contracts with industry
8.1.1 LiChIE contract with Airbus Defense and Space funded by Bpifrance
Participants: Louis Hauseux, Jules Mabon, Josiane Zerubia.
External collaborators: Mathias Ortner [Airbus Defense and Space, Senior Data Scientist].
Automatic object detection and tracking on sequences of images taken from various constellations of satellites. This contract covered one PhD (Jules Mabon from October 2020 until December 2023), one postdoc (Camilo Aguilar Herrera from January 2021 until September 2022), and one research engineer (Louis Hauseux from February until April 2023).
9 Partnerships and cooperations
9.1 International research visitors
9.1.1 Visits of international scientists
Other international visits to the team
Zoltan Kato
- Prof. Zoltan Kato from Szeged University, Hungary, visited AYANA team from July 5 to July 12, 2023.
9.2 National initiatives
ANR FAULTS R GEMS
Participants: Josiane Zerubia.
External collaborators: Isabelle Manighetti [OCA, Géoazur, Senior Physicist].
AYANA team is part of the ANR project FAULTS R GEMS (2017-2024, PI Geoazur) dedicated to realistic generic earthquake modeling and hazard simulation.
10 Dissemination
Participants: Hauseux Louis, Osa Priscilla, Pastorino Martina, Zerubia Josiane.
10.1 Promoting scientific activities
Member of the organizing committees
10.1.1 Scientific events: selection
Member of the conference program committees
- Josiane Zerubia was a member of the conference program committee of SPIE Remote Sensing'23 (Amsterdam, Netherlands).
Reviewer
- Josiane Zerubia reviewed for the international conferences IEEE ICASSP'23, IEEE ICIP'23, IEEE IGARSS'23, SPIE Remote Sensing'23 and ACVIS'23.
- Martina Pastorino reviewed an article for the international conference IEEE IGARSS'23.
10.1.2 Journal
Member of the editorial boards
- Josiane Zerubia has been a member of the editorial board of Fondation and Trend in Signal Processing (2007- ).
Reviewer - reviewing activities
- Josiane Zerubia reviewed an article for the international journal IEEE SPMag.
- Martina Pastorino reviewed an article for the international journal IEEE TGRS.
- Martina Pastorino reviewed an article for the international journal IEEE GRSL.
- Martina Pastorino reviewed an article for the international journal IEEE JSTARS.
- Martina Pastorino reviewed an article for the international journal MDPI Remote Sensing.
- Louis Hauseux reviewed an article for the international journal Probability in the Engineering and Informational Sciences.
10.1.3 Invited talks
- Josiane Zerubia presented AYANA research work at Airbus DS and CNES Data Campus, Toulouse, 3-4 Jan. 2023.
- Priscilla Osa was invited during three weeks by Professor Zoltan Kato and presented her work at RGVC team, University of Szeged, Hungary, June 2023.
- Louis Hauseux presented a poster entitled “Un nouvel estimateur des niveaux de densité utilisant les graphes et complexes simpliciaux” 11 at the XVIe Journées de Géostatistique (Mines de Paris – PSL), 7 Sept. 2023 at Fontainebleau.
- Martina Pastorino presented her work at GTTI (Group of Telecommunications and Information Technology) Annual Meeting, Rome, Italy, 11-13 Sep. 2023.
- Josiane Zerubia participated in Toulouse to both the Pléiades Neo day co-organized by SFPT, Airbus DS and the French Space Agency (CNES) on October 5 and the “Rencontres Techniques & Numériques” organized by CNES on Oct. 10 2023.
- Josiane Zerubia presented AYANA research work, as well as the activities of INRIA Defense and Security mission, during the visit of the French Space Command (CDE) at INRIA in Sophia Antipolis on Nov. 8 2023.
10.1.4 Leadership within the scientific community
- Josiane Zerubia is a Fellow of IEEE (2002- ), EURASIP (2019- ) and IAPR (2020- ).
- Josiane Zerubia is a member of the Teaching Board of the Doctoral School STIET at University of Genoa, Italy (2018- ).
- Josiane Zerubia is a member of the Scientific Council of GdR Géosto (Stochastic Geometry) from CNRS (2021-2023).
- Josiane Zerubia is a member of the Scientific Council of the IMPT-TDMA summer school (2022-2023).
10.1.5 Scientific expertise
- Josiane Zerubia did consulting for CAC (Cosmeto Azur Consulting), December 2023.
10.1.6 Research administration
- Josiane Zerubia is a member of various committees at INRIA-Centre d'Université Côte d'Azur (Comité des Equipes Projets (CEP), Comité de Centre (CC), Commission Bureaux (CB)).
- Josiane Zerubia is in charge of the aerospace research field for the Defense and Security mission at INRIA, headed by Frédérique Segond since Jan. 2023.
- Josiane Zerubia was president of the working group (INRIA, INRAE, Cirad, Bordeaux Sciences Agro) for the creation of a joint team (EVERGREEN) in Montpellier, from April to November 2023. EVERGREEN team was created in January 2024.
10.2 Teaching - Supervision - Juries
10.2.1 Teaching
- Josiane Zerubia taught master course on Remote Sensing: 13.5h eq. TD (9h of lectures), Master RISKS, Université Côte d'Azur, France (2019-2023). This course was given to Masters students
- Martina Pastorino co-taught (official teaching appointment) a Remote Sensing for Hydrography course, Master in Geomatics, Istituto Idrografico della Marina, University of Genoa, Italy (2022-2023).
- Martina Pastorino is in charge of the teaching support activities for the course of Signals and Systems for the Telecommunications (laboratories), University of Genoa (2023-2024).
- Louis Hauseux with Konstantin Avrachenkov taught the master course “Statistical Analysis of Networks”: 30H eq. TD (12h of lectures and 12h of TD), M2 Data Science and Artificial Intelligence, Université Côte d'Azur (2023).
10.2.2 Supervision
- Josiane Zerubia supervized one research engineer (Louis Hauseux), one Master student (Priscilla Osa) and three PhD students (Jules Mabon, Martina Pastorino, and Louis Hauseux) within the AYANA team.
10.2.3 Juries
- Josiane Zerubia was a member of 3 PhD committees in France (one as President) and one PhD committee in the USA (Purdue University, as external reviewer).
10.3 Popularization
- Martina Pastorino and Josiane Zerubia presented their work in front of 300 high-school students from both Lycée Simone Veil and Lycée international de Valbonne (CIV) during the International Women's Day, Valbonne, Mar. 2023. Inria and CIV Twitts.
10.3.1 Articles and contents
- During his research engineer time (paid on the LiChIE contract), Louis Hauseux worked with Josiane Zerubia and Mathias Ortner (Airbus DS), writing two dissemination papers. One in French, “Au cœur de la télédétection par satellite : l’équipe Ayana de l’Inria” published in the Newsletter of the Société Française de Photogrammétrie et de Télédétection on Jules Mabon's work (as a PhD student in AYANA team): about the detection of small objects on satellite images. The second paper “Remote Sensing: Detecting and Tracking Vehicles on Satellite Images and Videos” was published in ERCIM News and also discussed the work of Camilo Aguilar (PostDoc 2020-2022 in AYANA Team) on the tracking of small objects of interest on Remote sensing videos.
- For the IEEE Signal Processing Society 75th Anniversary special issue, Rabab Kreidieh Ward, picked Josiane Zerubia among other women researchers to illustrate their article on The Evolution of Women in Signal Processing and Science, Technology, Engineering, and Mathematics.
11 Scientific production
11.1 Major publications
- 1 articleSmall Object Detection and Tracking in Satellite Videos With Motion Informed-CNN and GM-PHD Filter.Frontiers in Signal Processing2April 2022HALDOI
- 2 articleSemantic Segmentation of Remote Sensing Images through Fully Convolutional Neural Networks and Hierarchical Probabilistic Graphical Models.IEEE Transactions on Geoscience and Remote Sensing602022, 1-16HALDOI
11.2 Publications of the year
International peer-reviewed conferences
National peer-reviewed Conferences
Doctoral dissertations and habilitation theses
Reports & preprints
Other scientific publications
11.3 Cited publications
- 12 inproceedingsA Transformer-Based Siamese Network for Change Detection.IEEE International Geoscience and Remote Sensing Symposium (IGARSS)2022, 207-210DOIback to text
- 13 articleRemote Sensing Image Change Detection With Transformers.IEEE Transactions on Geoscience and Remote Sensing602022, 1-14DOIback to text
- 14 articleA Spatial-Temporal Attention-Based Method and a New Dataset for Remote Sensing Image Change Detection.Remote Sensing122020, 1662back to textback to text
- 15 articleTheory of Communication.Journal of Institution of Electrical Engineers9331946, 429--457back to text
- 16 articleFully Convolutional Networks for Multisource Building Extraction From an Open Aerial and Satellite Imagery Data Set.IEEE Transactions on Geoscience and Remote Sensing5712019, 574-586DOIback to text
- 17 articlePoint processes for unsupervised line network extraction in remote sensing.IEEE TPAMI27102005, 1568--1579back to text
- 18 phdthesisCurvilinear Structure Detection in Images by Connected-Tube Marked Point Process and Anomaly Detection in Time Series.Purdue University2023back to text
- 19 inproceedingsLight-Weight Attention Semantic Segmentation Network for High-Resolution Remote Sensing Images.IGARSS 2020 - 2020 IEEE International Geoscience and Remote Sensing SymposiumWaikoloa, HI, USASep 2020, 2595-2598back to text
- 20 articleFew-Shot Object Detection in Aerial Imagery Guided by Text-Modal Knowledge.IEEE Transactions on Geoscience and Remote Sensing61February 2023, 1--19DOIback to text
- 21 unpublishedFully Convolutional Network to Learn the Potentials of a CRF for the Semantic Segmentation of Remote Sensing Images.2023back to text
- 22 inproceedingsFully convolutional and feedforward networks for the semantic segmentation of remotely sensed images.ICIP 2022- IEEE International Conference on Image ProcessingBordeaux, FranceOct 2022, URL: https://hal.inria.fr/hal-03720693.DOIback to text
- 23 unpublishedMultimission and Multifrequency SAR Image Classification through Hierarchical Markov Models and Convolutional Networks.2023back to text
- 24 articleSemantic Segmentation of Remote-Sensing Images Through Fully Convolutional Neural Networks and Hierarchical Probabilistic Graphical Models.IEEE Transactions on Geoscience and Remote Sensing6054071162022, 1-16DOIback to textback to text
- 25 articleForest Point Processes for the Automatic Extraction of Networks in Raster Data.ISPRS Journal of Photogrammetry and Remote Sensing126April 2017, 38--55DOIback to textback to text
- 26 articleDetection of cosmic filaments using the Candy model.Astronomy & Astrophysics43422005, 423-432DOIback to textback to text
- 27 inproceedingsDeep High-Resolution Representation Learning for Human Pose Estimation.IEEE/CVF Conference on Computer Vision and Pattern Recognition (CVPR)5686-5696, 2019back to text
- 28 articleDetecting Parametric Objects in Large Scenes by Monte Carlo Sampling.International Journal of Computer Vision1061January 2014, 57--75DOIback to text
- 29 inproceedingsDOTA: A Large-Scale Dataset for Object Detection in Aerial Images.Proceedings of the IEEE/CVF Conference on Computer Vision and Pattern Recognition (CVPR)Salt Lake City, USAJune 2018, 3974--3983DOIback to text
- 30 articleOn the Arbitrary-Oriented Object Detection: Classification Based Approaches Revisited.International Journal of Computer Vision1305May 2022, 1340--1365DOIback to text
- 31 inproceedingsOriented Object Detection in Aerial Images with Box Boundary-Aware Vectors.Proc. IEEE Winter Conf. on Applications of Computer Vision2021back to textback to text
- 32 articleDynamic Cascade Query Selection for Oriented Object Detection.IEEE Geoscience and Remote Sensing Letters20August 2023, 1--5DOIback to text
- 33 inproceedingsConditional Random Fields as Recurrent Neural Networks.2015 IEEE International Conference on Computer Vision (ICCV)2015, 1529-1537DOIback to textback to text