2023Activity reportProject-TeamDYLISS
RNSR: 201221035S- Research center Inria Centre at Rennes University
- In partnership with:CNRS, Université de Rennes
- Team name: Dynamics, Logics and Inference for biological Systems and Sequences
- In collaboration with:Institut de recherche en informatique et systèmes aléatoires (IRISA)
- Domain:Digital Health, Biology and Earth
- Theme:Computational Biology
Keywords
Computer Science and Digital Science
- A3.1.1. Modeling, representation
- A3.1.2. Data management, quering and storage
- A3.1.7. Open data
- A3.1.10. Heterogeneous data
- A3.2.3. Inference
- A3.2.4. Semantic Web
- A3.2.5. Ontologies
- A3.2.6. Linked data
- A3.3.3. Big data analysis
- A7.2. Logic in Computer Science
- A8.1. Discrete mathematics, combinatorics
- A8.2. Optimization
- A9.1. Knowledge
- A9.2. Machine learning
- A9.7. AI algorithmics
- A9.8. Reasoning
Other Research Topics and Application Domains
- B1.1.2. Molecular and cellular biology
- B1.1.7. Bioinformatics
- B1.1.10. Systems and synthetic biology
- B2.2.3. Cancer
- B2.2.5. Immune system diseases
1 Team members, visitors, external collaborators
Research Scientists
- Samuel Blanquart [INRIA, Researcher]
- François Coste [INRIA, Researcher]
- Anne Siegel [CNRS, Senior Researcher, HDR]
Faculty Members
- Olivier Dameron [Team leader, UNIV RENNES, Professor, HDR]
- Emmanuelle Becker [UNIV RENNES, Professor, HDR]
- Catherine Belleannée [UNIV RENNES, Associate Professor]
- Yann Le Cunff [UNIV RENNES, Associate Professor]
Post-Doctoral Fellows
- Arnaud Belcour [CNRS, Post-Doctoral Fellow, until Feb 2023]
- Victor Mataigne [CNRS]
PhD Students
- Moana Aulagner [INRIA, from Oct 2023]
- Cecile Beust [UNIV RENNES, from Oct 2023]
- Matthieu Bougueon [INSERM, until Sep 2023]
- Nicolas Buton [UNIV RENNES, until Sep 2023]
- Pablo Espana Gutierrez [ENS RENNES, from Sep 2023]
- Camille Juigne [INRAE, until Nov 2023]
- Corentin Lucas [INRIA, from Oct 2023]
- Baptiste Ruiz [INRIA]
- Kerian Thuillier [CNRS]
- Yael Tirlet [UNIV RENNES, from May 2023]
Technical Staff
- Moana Aulagner [INRAE, from Sep 2023 until Sep 2023]
- Matthieu Bougueon [CNRS, from Oct 2023]
- Pauline Giraud [CNRS]
- Jeanne Got [CNRS, Engineer]
- Corentin Lucas [UNIV RENNES, from Sep 2023 until Sep 2023]
- Noe Robert [CNRS, Engineer, from Oct 2023]
- Yael Tirlet [INRAE, until Apr 2023]
Interns and Apprentices
- Moana Aulagner [INRAE, Intern, until Jul 2023]
- Oceane Carpentier [UNIV RENNES, Intern, from Apr 2023 until Jul 2023]
- Pablo Espana Gutierrez [ENS RENNES, Intern, until Jun 2023]
- Alexandre Lereverend [UNIV RENNES, Intern, from Apr 2023 until Jul 2023]
- Corentin Lucas [CNRS, Intern, until Jul 2023]
- Sophia Pham [UNIV RENNES, Intern, from Apr 2023 until Jul 2023]
- Alix Regnier [UNIV RENNES, Intern, from Apr 2023 until Jun 2023]
Administrative Assistant
- Marie Le Roic [INRIA]
Visiting Scientist
- Oumarou Abdou-Arbi [Univ Maradi]
External Collaborators
- François Moreews [IRISA, from Feb 2023]
- François Moreews [INRAE, until Jan 2023]
- Nathalie Theret [INSERM, HDR]
2 Overall objectives
Bioinformatics context: from life data science to functional information about biological systems and unconventional species. Sequence analysis and systems biology both consist in the interpretation of biological information at the molecular level, that concern mainly intra-cellular compounds. Analyzing genome-level information is the main issue of sequence analysis. The ultimate goal here is to build a full catalogue of bio-products together with their functions, and to provide efficient methods to characterize such bio-products in genomic sequences. In regards, contextual physiological information includes all cell events that can be observed when a perturbation is performed over a living system. Analyzing contextual physiological information is the main issue of systems biology.
For a long time, computational methods developed within sequence analysis and dynamical modeling had few interplay. However, the emergence and the democratization of new sequencing technologies (NGS, metagenomics) provides information to link systems with genomic sequences. In this research area, the Dyliss team focuses on linking genomic sequence analysis and systems biology. Our main applicative goal in biology is to characterize groups of genetic actors that control the phenotypic response of species when challenged by their environment. Our main computational goals are to develop methods for analyzing the dynamical response of a biological system, modeling and classifying families of gene products with sensitive and expressive languages, and identifying the main actors of a biological system within static interaction maps. We first formalize and integrate in a set of logical or grammatical constraints both generic knowledge information (literature-based regulatory pathways, diversity of molecular functions, DNA patterns associated with molecular mechanisms) and species-specific information (physiological response to perturbations, sequencing...). We then rely on symbolic methods (Semantic Web technologies for data integration, querying as well as for reasoning with bio-ontologies, solving combinatorial optimization problems, formal classification) to compute the main features of the space of admissible models.
Computational challenges. The main challenges we face are data incompleteness and heterogeneity, leading to non-identifiability. Indeed, we have observed that the biological systems that we consider cannot be uniquely identifiable. Indeed, "omics" technologies have allowed the number of measured compounds in a system to increase tremendously. However, it appears that the theoretical number of different experimental measurements required to integrate these compounds in a single discriminative model has increased exponentially with respect to the number of measured compounds. Therefore, according to the current state of knowledge, there is no possibility to explain the data with a single model. Our rationale is that biological systems will still remain non-identifiable for a very long time. In this context, we favor the construction and the study of a space of feasible models or hypotheses, including known constraints and facts on a living system, rather than searching for a single discriminative optimized model. We develop methods allowing a precise and exhaustive investigation of this space of hypotheses. With this strategy, we are in the position of developing experimental strategies to progressively shrink the space of hypotheses and increase the understanding of the system.
Bioinformatics challenges. Our objectives in computer sciences are developed within the team in order to fit with three main bioinformatics challenges (1) data-science and knowledge-science for life sciences (see Section 3.2); (2) understanding metabolism (see Section 3.3); (3) characterizing regulatory and signaling phenotypes (see Section 3.4).
Implementing methods in software and platforms. Seven platforms have been developed in the team during the last five years: Askomics, AuReMe, FinGoc, Caspo, Cadbiom, Logol and Protomata. They aim at guiding the user to progressively reduce the space of models (families of sequences of genes or proteins, families of keys actors involved in a system response or dynamical models) which are compatible with both the knowledge and experimental observations. Most of our platforms are developed with the support of the GenOuest resource and data center hosted in the IRISA laboratory, including their computer facilities [More info]
3 Research program
3.1 Context: Computer science perspective on symbolic artificial intelligence
We develop methods that use an explicit representation of the relationships between heterogeneous data and knowledge in order to construct a space of hypotheses. Therefore, our objective in computer science is mainly to develop accurate representations (oriented graphs, Boolean networks, automata, or expressive grammars) to iteratively capture the complexity of a biological system.
Integrating data with querying languages: Semantic web for life sciences The first level of complexity in the data integration process consists in confronting heterogeneous datasets. Both the size and the heretogeneity of life science data make their integration and analysis by domain experts impractical and prone to the streetlight effect (they will pick up the models that best match what they know or what they would like to discover). Our first objective involves the formalization and management of symbolic knowledge, that is, the explicitation of relations occurring in structured data. In this setting, our main goal is to facilitate and optimize the integration of Semantic Web resources with local users data by relying on the implicit data scheme contained in biological data and Semantic Web resources.
Reasoning over structured data with constraint-based logical paradigms Another level of complexity in life science integration is that very few paradigms exist to model the behavior of a complex biological system. This leads biologists to perform and formulate hypotheses in order to interpret their data. Our strategy is to interpret such hypotheses as combinatorial optimization problems, allowing to reduce the family of models compatible with data. To that goal, we collaborate with Potsdam University in order to use and challenge the most recent developments of Answer Set Programming (ASP) 49, a logical paradigm for solving constraint satisfiability and combinatorial optimization issues.
Our goal is therefore to provide scalable and expressive formal models of queries on biological networks with the focus of integrating dynamical information as explicit logical constraints in the modeling process.
Characterizing biological sequences with formal syntactic models Our last goal is to identify and characterize the function of expressed genes such as transcripts, enzymes or isoforms in non-model species biological networks or specific functional features of metagenomic samples. These are insufficiently precise because of the divergence of biological sequences, the complexity of molecular structures and biological processes, and the weak signals characterizing these elements.
Our goal is therefore to develop accurate formal syntactic models (automata, grammars or abstract gene models) that would enable us to represent sequence conservation, sets of short and degenerated patterns, and crossing or distant dependencies. This requires both to determine the classes of formal syntactic models adequate for handling biological complexity, and to automatically characterize the functional potential embodied in biological sequences with these models.
3.2 Scalable methods to query data heterogenity
Confronted to large and complex data sets (raw data are associated with graphs depicting explicit or implicit links and correlations) almost all scientific fields have been impacted by the big data issue, especially genomics and astronomy 61. In our opinion, life sciences cumulate several features that are very specific and prevent the direct application of big data strategies that proved successful in other domains such as experimental physics: the existence of several scales of granularity (from microscopic to macroscopic) and the associated issue of dependency propagation, datasets incompleteness and uncertainty (including highly heterogeneous responses to a perturbation from one sample to another), and highly fragmented sources of information that lacks interoperability 47. To explore this research field, we use techniques from symbolic data mining (Semantic Web technologies, symbolic clustering, constraint satisfaction, and grammatical modeling) to take into account those life science features in the analysis of biological data.
3.2.1 Research topics
Facilitating data integration and querying The quantity and inner complexity of life science data require semantically-rich analysis methods. A major challenge is then to combine data (from local project as well as from reference databases) and symbolic knowledge seamlessly. Semantic Web technologies (RDF for annotating data, OWL for representing symbolic knowledge, and SPARQL for querying) provide a relevant framework, as demonstrated by the success of Linked (Open) Data 28. However, life science end users (1) find it difficult to learn the languages for representing and querying Semantic Web data, and consequently (2) miss the possibility they had to interact with their tabulated data (even when doing so was exceedingly slow and tedious). Our first objective in this axis is to develop accurate abstractions of datasets or knowledge repositories to facilitate their exploration with RDF-based technologies.
Scalability of semantic web queries. A bottleneck in data querying is given by the performance of federated SPARQL queries, which must be improved by several orders of magnitude to allow current massive data to be analyzed. In this direction, our research program focuses on the combination of linked data fragments 67, query properties and dataset structure for decomposing federated SPARQL queries.
Building and compressing static maps of interacting compounds A final approach to handle heterogeneity is to gather multi-scale data knowledge into a functional static map of biological models that can be analyzed and/or compressed. This requires to link genomics, metabolomics, expression data and protein measurement of several phenotypes into unified frameworks. In this direction, our main goal is to develop families of constraints, inspired by symbolic dynamical systems, to link datasets together. We currently focus on health (personalized medicine) and environmental (role of non-coding regulations, graph compression) datasets.
3.2.2 Associated software tools
AskOmics platform AskOmics is an integration and interrogation software for linked biological data based on semantic web technologies1. AskOmics aims at bridging the gap between end user data and the Linked (Open) Data cloud (LOD cloud). It allows heterogeneous bioinformatics data (formatted as tabular files or directly in RDF) to be loaded into a Triple Store system using a user-friendly web interface. It helps end users (1) to take advantage of the information available in the LOD cloud for analyzing their own data, and (2) to contribute back to the linked data by representing their data and the associated metadata in the proper format, as well as by linking them to other resources. An originality is the graphical interface that allows any dataset to be integrated in a local RDF datawarehouse and SPARQL query to be built transparently and iteratively by a non-expert user.
Pax2graphml aims at easily manipulating BioPAX source files as regulated reaction graphs described in graph format. The goal is to be highly flexible and to integrate graphs of regulated reactions from a single BioPAX source or by combining and filtering BioPAX sources. The output graphs can then be analyzed with additional tools developed in the team, such as KeyRegulatorFinder.
FinGoc-tools The FinGoc tools allow filtering interaction networks with graph-based optimization criteria in order to elucidate the main regulators of an observed phenotype. The main added-value of these tools is the functionality allowing to make explicit the criteria used to highlight the role of the main regulators. (1) The KeyRegulatorFinder package searches key regulators of lists of molecules (like metabolites, enzymes or genes) by taking advantage of knowledge databases in cell metabolism and signaling2. (2) The PowerGrasp python package implements graph compression methods oriented toward visualization, and based on power graph analysis3. (3) The iggy package enables the repairing of an interaction graph with respect to expression data4.
3.3 Metabolism: from protein sequences to systems ecology
Our research in bioinformatics in relation with metabolic processes is driven by the need to understand non-model (eukaryote) species. Their metabolism have acquired specific features that we wish to identify with computational methods. To that goal, we combine sequence analysis with metabolic network analysis, with the final goal to understand better the metabolism of communities of organisms.
3.3.1 Research topics
Genomic level: characterizing functions of protein sequences Precise characterization of functional proteins, such as enzymes or transporters, is a key to better understand and predict the actors involved in a metabolic process. In order to improve the precision of functional annotations, we develop machine learning approaches that take a sample of functional sequences as input and infer a model representing their key syntactical characteristics, including dependencies between residues.
System level: enriching and comparing metabolic networks for non-model organisms
Non-model organisms often lack both complete and reliable annotated sequences, which cause the draft networks of their metabolism to largely suffer from incompleteness. In former studies, the team has developed several methods to improve the quality of eukaryotic metabolic networks, by solving several variants of the so-called Metabolic Network gap-filling problem with logical programming approaches 9, 8. The main drawback of these approaches is that they cannot scale to the reconstruction and comparison of families of metabolic networks. Our main objective is therefore to develop new tools for the comparison of species strains at the metabolic level.
Consortium level: exploring the diversity of community consortia The newly emerging field of system ecology aims at building predictive models of species interactions within an ecosystem, with the goal of deciphering cooperative and competitive relationships between species 46. This field raises two new issues: (1) uncertainty on the species present in the ecosystem and (2) uncertainty about the global objective governing an ecosystem. To address these challenges, our first research focus is the inference of metabolic exchanges and relationships for transporter identification, based on our expertise in metabolic network gap-filling. The second challenging focus is the prediction of transporters families via refined characterization of transporters, which are quite unexplored apart from specific databases 59.
3.3.2 Associated software tools
Protomata5 is a machine learning suite for the inference of automata characterizing (functional) families of proteins at the sequence level. It provides programs to build a new kind of sequence alignments (characterized as partial and local), learn automata, and search for new family members in sequence databases. By enabling to model local dependencies between positions, automata are more expressive than classical tools (PSSMs, Profile HMMs, or Prosite Patterns) and are well suited to predict new family members with a high specificity. This suite is for instance embedded in the cyanolase database 35 to automate its updade and was used for refining the classification of HAD enzymes 6 or identify shared conservations in the core proteome of extracellular vesicles produced by human and animal S. aureus strains 64.
PPSuite6 is one of the first frameworks taking into account coevolutionary dependencies between residues for the comparison of protein sequences. It proposes a complete workflow enabling to infer direct couplings between the positions of a sequence of interest by a Potts model with the help of the sequence close homologs and to score the similarity of the sequences by alignment of the inferred Potts models, as well as tools to visualize the models and their alignments 63, 62.
AuReMe and AuCoMe workspaces is designed for tractable reconstruction of metabolic networks7. The toolbox allows for the Automatic Reconstruction of Metabolic networks based on the combination of multiple heterogeneous data and knowledge sources 1. The main added values are the inclusion of graph-based tools relevant for the study of non-model organisms (Meneco and Menetools packages), the possibility to trace the reconstruction and curation procedures (Padmet package), and the exploration of reconstructed metabolic networks with wikis (wiki-export package, see: aureme.genouest.org/wiki.html) 11. It also generates outputs to explore the resulting networks with Askomics. It has been used for reconstructing metabolic networks of micro and macro-algae 57, extremophile bacteria 39 and communities of organisms 4.
Mpwt, emmapper2gbk is a Python package for running Pathway Tools8 on multiple genomes using multiprocessing. Pathway Tools is a comprehensive systems biology software system that is associated with the BioCyc database collection9. Pathway Tools is frequently used for reconstructing metabolic networks. In order to allow the output of the eggnoggmapper annotation tool to be used by Mpwt, we also developed emmaper2gbk to create relevant genome files.
Metage2metabo is a Python tool to perform graph-based metabolic analysis starting from annotated genomes (reference genomes or metagenome-assembled genomes) 26. It uses Mpwt to reconstruct metabolic networks for a large number of genomes. The obtained metabolic networks are then analyzed individually and collectively in order to get the added value of metabolic cooperation in microbiota over individual metabolism and to identify and screen interesting organisms among all.
3.4 Regulation and signaling: detecting complex and discriminant signatures of phenotypes
On the contrary to metabolic networks, regulatory and signaling processes in biological systems involve agents interacting at different granularity levels (from genes, non-coding RNAs to protein complexes) and different time-scales. Our focus is on the reconstruction of large-scale networks involving multiple scales processes, from which controllers can be extracted with symbolic dynamical systems methods. Particular attention is paid to the characterization of products of genes (such as isoform) and of perturbations to identify discriminant signature of pathologies.
3.4.1 Research topics
Genomic level: characterizing gene structure with grammatical languages and conservation information The goal here is to accurately represent gene structure, including intron/exon structure, for predicting the products of genes, such as isoform transcripts, and comparing the expression potential of a eukaryotic gene according to its context (e.g. tissue) or according to the species. Our approach consists in designing grammatical and comparative-genomics based models for gene structures able to detect heterogeneous functional sites (splicing sites, regulatory binding sites...), functional regions (exons, promotors...) and global constraints (translation into proteins) 30. Accurate gene models are defined by identifying general constraints shaping gene families and their structures conserved over evolution. Syntactic elements controlling gene expression (transcription factor binding sites controlling transcription; enhancers and silencers controlling splicing events...), i.e. short, degenerated and overlapping functional sequences, are modeled by relying on the high capability of SVG grammars to deal with structure and ambiguity 60.
System level: extracting causal signatures of complex phenotypes with systems biology frameworks Our main challenge is to set up a generic formalism to model inter-layer interactions in large-scale biological networks. To that goal, we have developed several types of abstractions: multi-experiments framework to learn and control signaling networks 10, multi-layer reactions in interaction graphs 31, and multi-layer information in large-scale Petri nets 25. Our main issues are to scale these approaches to standardized large-scale repositories by relying on the interoperable Linked Open Data (LOD) resources and to enrich them with ad-hoc regulations extracted from sequence-based analysis. This will allow us to characterize changes in system attractors induced by mutations and how they may be included in pathology signatures.
3.4.2 Associated software tools
Logol software is designed for complex pattern modeling and matching10. It is a swiss-army-knife for pattern matching on DNA/RNA/Protein sequences, based on expressive patterns which consist in a complex combination of motifs (such as degenerated strings) and structures (such as imperfect stem-loop ou repeats) 2. Logol key features are the possibilities (i) to divide a pattern description into several sub-patterns, (ii) to model long range dependencies, and (iii) to enable the use of ambiguous models or to permit the inclusion of negative conditions in a pattern definition. Therefore, Logol encompasses most of the features of specialized tools (Vmatch, Patmatch, Cutadapt, HMM) and enables interplays between several classes of patterns (motifs and structures), including stem-loop identification in CRISPR.
Caspo Cell ASP Optimizer (Caspo) software constitutes a pipeline for automated reasoning on logical signaling networks (learning, classifying, designing experimental perturbations, identifying controllers, take time-series into account)11. The software handles inherent experimental noise by enumerating all different logical networks which are compatible with a set of experimental observations 10. The main advantage is that it enables a complete study of logical network without requiring any linear constraint programs.
Cadbiom package aims at building and analyzing the asynchronous dynamics of enriched logical networks12. It is based on Guarded transition semantic and allows synchronization events to be investigated in large-scale biological networks 25. For example, it allowed to analyze controler of phenotypes in a large-scale knowledge database (PID) 5.
Recently, we have significantly refactored Cadbiom package towards a framework that allows the identification of causal regulators in large-scale models, formalized in the BioPAX language and automatically interpreted as guarded transitions. The Cadbiom framework was applied to the BioPAX version of two ressources (PID, KEGG) of the PathwayCommons database and to the Atlas of Cancer Signalling Network (ACSN). As a case-study, it was used to characterize the causal signatures of markers of the epithelial-mesenchymal transition.
4 Application domains
In terms of transfer and societal impact, we consider that our role is to develop fruitful collaborations with biology laboratories in order to consolidate their studies by a smart use of our tools and prototypes and to generate new biological hypotheses to be tested experimentally.
Marine Biology: seaweed enzymes and metabolism An important field of study is marine biology, as it is a transversal field covering challenges in integrative biology, dynamical systems and sequence analysis.
- Protein functions in seaweed metabolism Several years ago, our methods based on combinatorial optimization for the reconstruction of genome-scale metabolic networks and on classification of enzyme families based on local and partial alignments allowed the seaweed E. siliculosus metabolism to be deciphered 57, 40. The study of the HAD superfamily of proteins thanks to partial local alignments produced by Protomata tools, allowed sub-families to be deciphered and classified. Additionally, the metabolic map reconstructed with Meneco enabled the reannotation of 56 genes within the E. siliculosus genome. These approaches also shed light on evolution of metabolic processes.
- Elucidating algal metabolism thanks to large-scale metabolic network reconstructions More recently, the tools developed by Dyliss (based on the AuReMe toolbox) allowed us to participate in the reconstruction of a metabolic network for the brown algae Saccharina japonica and Cladosiphon okamuranus in order to identify these species specificities on the synthesis of carotenoids biosynthesis 55. We also participated in the study of the genome of Ectocarpus subulatus, a highly stress-tolerant algal strain 45. Finally, AuReMe has been used to analyze the metabolic capacity of several strains of cyanobacteria, with results integrated in the Cyanorak database 48 and to characterize synergistic effects of the synechococcus strain WH7803 51.
- Metabolic pathway drift theory Genome annotations can contribute to understanding algal metabolism. The tool PathModel was developed to add support for biochemical reactions and metabolite structures to the theory of metabolic pathway drift with an approach combining chemoinformatics knowledge reasoning and modeling. This approach was applied to the study of the red alga Chondrus crispus, which allowed to show that even for metabolic pathways supposed to be conserved between species (sterols, mycrosporins synthesis), we can see an important turnover in the order of reactions appearing in a metabolic pathway. This work lays the foundations for the concept of "metabolic drift" analogous to the same concept in genomics. 27.
-
Algal-bacteria interactions We reconstructed the metabolic network of a symbiot bacterium Ca. P. ectocarpi 44 and used this reconstructed network to decipher interactions within the algal-bacteria holobiont, revealing several candidates metabolic pathways for algal-bacterial interactions. Similarily, our analyses suggested that the bacterium Ca. P. ectocarpi is able to provide both beta-alanine and vitamin B5 to the seaweed via the phosphopantothenate biosynthesis pathway 58.
These works paved the way to the study of host-microbial interactions, as shown in 36 where we evidenced the role of tools such as miscoto and metage2metabo to predict synthetic communities allowing to restore algal metabolic pathways. To validate these approaches experimentally, we worked with S. Dittami, researcher at the Roscoff biological station. We applied these methods on a set of about fifteen cultivable bacteria identified on the wall membrane of Ectocarpus siliculosus. Our approaches predicted that three bacteria were necessary to facilitate the growth of this alga in an axenic medium. The experiments were carried out, and indeed allowed the alga to grow in an axenic medium. This is therefore a proof of concept of the relevance of our approaches. More recently, the study of the freshwater strain of Ectocarpus subulatus evidenced the role of metabolism in adaptation, paving the way to biotechnological applications 53.
Microbiology: elucidating the functioning of extremophile consortiums of bacteria. Our main issue is the understanding of bacteria living in extreme environments. The context is mainly a collaboration with the group of bioinformatics at Universidad de Chile (co-funded by the Center of Mathematical Modeling, the Center of Regulation Genomics and Inria-Chile). In order to elucidate the main characteristics of these bacteria, our integrative methods were developed to identify the main groups of regulators for their specific response in their living environment. The integrative biology tools Meneco, Lombarde and Shogen have been designed in this context. In particular, genome-scale metabolic network been recently reconstructed and studied with the Meneco and Shogen approaches, especially on bacteria involved in biomining processes 32 and in Salmon pathogenicity 39. We have also studied the specificities of two Microbacterium strains, CGR1 and CGR2, isolated in different soils of the Atacama Desert in Chile, showing significant differences on the connectivity of metabolite production in relation to pH tolerance and CO2 production 54.
Agriculture and environmental sciences: upstream controllers of cow, pork and pea-aphid metabolism and regulation. Our goal is to propose methods to identify regulators of complex phenotypes related to environmental issues. Our work on the identification of upstream regulators within large-scale knowledge databases (tool KeyRegulatorFinder) 31 and on semantic-based analysis of metabolic networks 29 was very valuable for interpreting the differences of gene expression in pork meat 52 and figure out the main gene-regulators of the response of porks to several diets 50. Our expertise in microbiota analysis is also currently being applied to rumen microbial genomics 56.
Health: Dynamics of microenvironment in chronic liver diseases
We develop methods and models to understand the dynamics of the microenvironment in order to propose evolutionary markers and effective therapeutic targets. The matrix microenvironment is the major regulator of events related to fibrosis-cirrhosis-cancer progression and Hepatic Stellate Cells (HSC) are the main actors of microenvironment remodeling. At molecular level, the transforming growth factor TGF-
-
TGF-
signaling networks. TGF- is a multifunctional cytokine that binds to specific receptors and induce numerous signaling pathways depending on the context. Deciphering TGF- signaling networks requires to take into account a system-wide view and develop predictive models for therapeutic benefit. For that purpose we developed Cadbiom and identified gene networks associated with innate immune response to viral infection that combine TGF- and interleukin signaling pathways 25, 38. More recently we have very significantly refactored Cadbiom package towards a framework that allows the identification of causal regulators in large-scale models, formalized in the BioPAX language and automatically interpreted as guarded transitions13.The Cadbiom framework was applied to the BioPAX version of two resources (PID,KEGG) of the Pathway Commons database and to the Atlas of Cancer Signalling Network (ACSN). As a case-study, it was used to characterize the causal signatures of markers of the epithelial-mesenchymal transition. - Functional signature for ADAMTS. Hepatic Stellate Cells produce a wide variety of molecules involved in ECM remodeling, such as adamalysins 65. However, the limitations of discovering new functions of these proteins stem from the experimental approaches that are difficult to implement due to their structure and biochemical features. In that context we developed an original framework combining the identification of small modules in conserved regions independent of known domains and the concepts of phylogenomics (association of conservation and phenotype gained concurrently during evolution). The resulting evolutionary model of motif signatures and protein-protein interaction signatures of the ADAMTS family is validated by data from literature and provides biologists with many new potential functional motifs 42, 41, 43.
-
Dynamic model of hepatic stellate cells. To characterize the dynamics of HSC
activation upon TGFB1 stimulation, we developed a model using Kappa, a site graph rewriting language and its static analyzer Kasa 34. We previously demonstrated the advantages of Kappa language for modeling TGF-
signaling and extracellular matrix 66. Unlike previous model based on a population of interacting proteins, we now develop an original Kappa model based on a population of cells interacting with TGF- 33. The model recapitulates the dynamics of activation of HSC towards myofibroblast states and the reversion processes. Current work aims to identify the regulators of the repair likely to promote the resolution of fibrosis at the expense of its progression.
5 Social and environmental responsibility
5.1 Footprint of research activities
Dyliss research activities have low environmental footprints. Most of our software solution run on off-the-shelf computers and are not computationally intensive. Indirectly, the analyses and predictions we make intend to reduce the need for long, costly technically or ethically difficult biological experiments.
5.2 Impact of research results
Through our ongoing collaborations with INSERM and Rennes' Hospital, Dyliss research activities have a social impact on human health. Our collaborations with INRAe have a direct impact on vegetal and animal health, and an indirect impact in environment as these projects original motivation is to reduce fertilizers or pesticides.
6 Highlights of the year
- Emmanuelle Becker was promoted full Professor
- Catherine Belleannée was promoted Associate Professor Hors-Classe
- Yann Le Cunff was appointed as scientific director of the GenOuest bioinformatics platform
7 New software, platforms, open data
7.1 General software strategy/dissemination
In 2023, we have undertaken a major overhaul of the distribution of our softwares. This encompasses providing an architecture for their respective git repositories, and improving their visibility. We also acknowledged that we provide to the community not only individual softwares, but also more elaborate workflows, which we have started drafting (Fig. 1). This work is still in progress and we will complete this initiative with the remainder of our softwares.
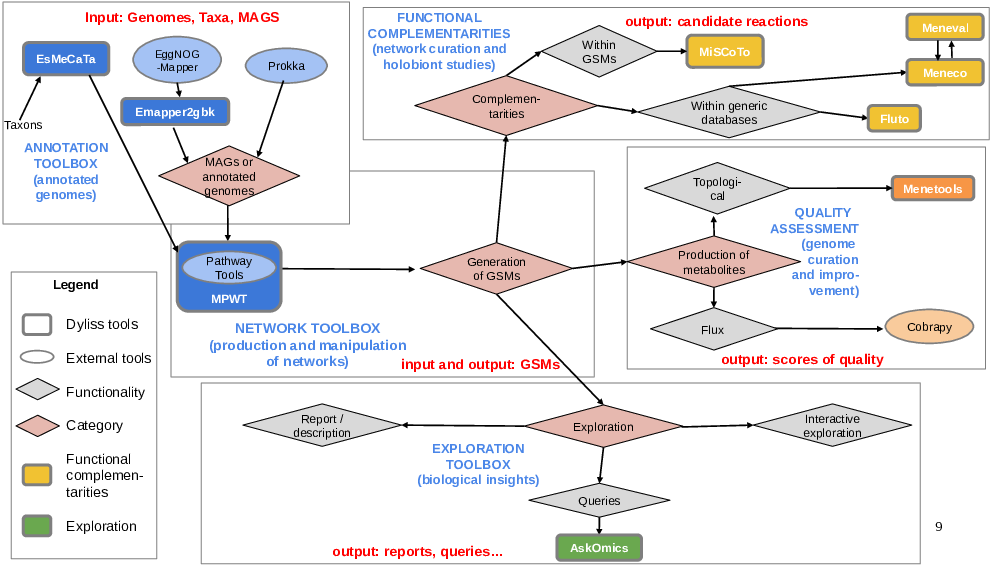
Respesentation of some of DYLISS softwares and how they can be combined (including with other third-party softwares) for studying complex biological systems.
7.2 New software
7.2.1 AskOmics
-
Name:
Convert tabulated data into RDF and create SPARQL queries intuitively and "on the fly".
-
Keywords:
RDF, SPARQL, Querying, Graph, LOD - Linked open data
-
Functional Description:
AskOmics aims at bridging the gap between end user data and the Linked (Open) Data cloud. It allows heterogeneous bioinformatics data (formatted as tabular files) to be loaded in a RDF triplestore and then be transparently and interactively queried. AskOmics is made of three software blocks: (1) a web interface for data import, allowing the creation of a local triplestore from user's datasheets and standard data, (2) an interactive web interface allowing "à la carte" query-building, (3) a server performing interactions with local and distant triplestores (queries execution, management of users parameters).
- URL:
-
Authors:
Charles Bettembourg, Xavier Garnier, Anthony Bretaudeau, Fabrice Legeai, Olivier Dameron, Olivier Filangi, Yvanne Chaussin, Mateo Boudet
-
Contact:
Olivier Dameron
-
Partners:
Université de Rennes 1, CNRS, INRA
7.2.2 Metage2Metabo
-
Keywords:
Metabolic networks, Microbiota, Metagenomics, Workflow
-
Scientific Description:
Flexible pipeline for the metabolic screening of large scale microbial communities described by reference genomes or metagenome-assembled genomes. The pipeline comprises several main steps. (1) Automatic and parallel reconstruction of metabolic networks. (2) Computation of individual metabolic potentials (3) Computation of collective metabolic potential (4) Calculation of the cooperation potential described as the set of metabolites producible by species only in a cooperative context (5) Computation of minimal-sized communities sastifying a metabolic objective (6) Extraction of key species (essential and alternative symbionts) associated to a metabolic function
-
Functional Description:
Metabolic networks are graphs which nodes are compounds and edges are biochemical reactions. To study the metabolic capabilities of microbiota, Metage2Metabo uses multiprocessing to reconstruct metabolic networks at large-scale. The individual and collective metabolic capabilities (number of compounds producible) are computed and compared. From these comparisons, a set of compounds only producible by the community is created. These newly producible compounds are used to find minimal communities that can produce them. From these communities, the keytstone species in the production of these compounds are identified.
- URL:
- Publication:
-
Contact:
Clemence Frioux
-
Participants:
Clemence Frioux, Arnaud Belcour, Anne Siegel
7.2.3 CADBIOM
-
Name:
Computer Aided Design of Biological Models
-
Keywords:
Health, Biology, Biotechnology, Bioinformatics, Systems Biology
-
Functional Description:
The Cadbiom software provides a formal framework to help the modeling of biological systems such as cell signaling network with Guarder Transition Semantics. It allows synchronization events to be investigated in biological networks among large-scale network in order to extract signature of controllers of a phenotype. Three modules are composing Cadbiom. 1) The Cadbiom graphical interface is useful to build and study moderate size models. It provides exploration, simulation and checking. For large-scale models, Cadbiom also allows to focus on specific nodes of interest. 2) The Cadbiom API allows a model to be loaded, performing static analysis and checking temporal properties on a finite horizon in the future or in the past. 3) Exploring large-scale knowledge repositories, since the translations of the large-scale PID repository (about 10,000 curated interactions) have been translated into the Cadbiom formalism.
-
News of the Year:
We have significantly refactored Cadbiom package towards a framework that allows the identification of causal regulators in large-scale models, formalized in the BioPAX language and automatically interpreted as guarded transitions.
- URL:
- Publications:
-
Contact:
Anne Siegel
-
Participants:
Geoffroy Andrieux, Michel Le Borgne, Nathalie Theret, Nolwenn Le Meur, Pierre Vignet, Anne Siegel
7.2.4 pax2graphml
-
Name:
pax2graphml - Large-scale Regulation Network in Python using BIOPAX and Graphml
-
Keyword:
Bioinformatics
-
Functional Description:
PAX2GRAPHML is an open source python library that allows to easily manipulate BioPAX source files as regulated reaction graphs described in .graphml format. PAX2GRAPHML is highly flexible and allows generating graphs of regulated reactions from a single BioPAX source or by combining and filtering BioPAX sources. Supporting the graph exchange format .graphml, the large-scale graphs produced from one or more data sources can be further analyzed with PAX2GRAPHML or standard python and R graph libraries.
- URL:
- Publication:
-
Contact:
Francois Moreews
-
Partner:
INRAE
7.2.5 Protomata
-
Keywords:
Proteins, Machine learning, Pattern discovery, Grammatical Inference, Bioinformatics
-
Scientific Description:
Inference of automata modelling protein sequences by partial local alignment
-
Functional Description:
This tool is a grammatical inference framework suitable for learning the specific signature of a functional protein family from unaligned sequences by partial and local multiple alignment and automata modelling. It performs a syntactic characterization of proteins by identification of conservation blocks on sequence subsets and modelling of their succession. Possible fields of application are new members discovery or study (for instance, for site-directed mutagenesis) of, possibly non-homologous, functional families and subfamilies such as enzymatic, signalling or transporting proteins.
Given a sample of sequences belonging to a structural or functional family of proteins, Protomata-Learner infers an automaton characterizing the family by partial local alignment of the sequences. Automata are graphical models representing a (potentially infinite) set of sequences. Able to express alternative local dependencies between the positions, automata offer a finer level of expressivity than classical sequence patterns (such as PSSM, Profile HMM, or Prosite Patterns) and can model more than homologous sequences. They are well suited to get new insights into a family or to search for new family members in the sequence data banks, especially when approaches based on classical multiple sequence alignments are insufficient.
The three main modules integrated in the Protomata-learner workflow are available as well as stand-alone programs: 1) paloma builds partial local multiple alignments, 2) protobuild infers automata from these alignements and 3) protomatch and protoalign scans, parses and aligns new sequences with learnt automata. The suite is completed by tools to handle or visualize data and can be used online by the biologists via a web interface on Genouest Platform.
-
News of the Year:
paloma-2 was renamed paloma-d to avoid confusion with version numbering.
- URL:
-
Contact:
François Coste
-
Participant:
François Coste
-
Partners:
Université de Rennes 1, CNRS, Inria
7.2.6 PPsuite
-
Keywords:
Proteins, Sequence alignment, Bioinformatics, Machine learning, Homology search
-
Scientific Description:
Comparison of protein sequences using coevolutionary dependencies between residues.
-
Functional Description:
This suite contains the following tools : - MakePotts infers a Potts model from a sequence or a multiple sequence alignment - PPalign aligns Potts models and corresponding sequences - VizPotts allows to visualize inferred Potts models and VizContacts allows to visualize inferred couplings with respect to actual contacts in a 3D protein structure.
- URL:
- Publications:
-
Contact:
François Coste
-
Participants:
François Coste, Hugo Talibart, Mathilde Carpentier
7.2.7 Transformer Framework for Protein Characterization
-
Keywords:
Deep learning, Transformer, Functional annotation, Proteins, Biological sequences
-
Scientific Description:
A generic framework for the specialization of a pre-trained transformer protein language model for classification or regression tasks.
-
Functional Description:
Given examples of annotated sequences, this tool allows to train and analyse resulting models with respect to evaluation metrics (accuracy, correlation) plots and the importance of the residues for the inference. The process is fully automated and the whole operation can be done by modifying a JSON configuration file and providing a JSON data set. No code skills are thus required.
-
News of the Year:
Added instructions and scripts for reproducibility of the experiments from our publication on the prediction of the enzymatic function of sequence with EnzBert.
- URL:
- Publications:
-
Contact:
Nicolas Buton
-
Participants:
Nicolas Buton, François Coste, Yann Le Cunff
7.2.8 Emapper2GBK
-
Keywords:
Bioinformatics, Metabolic networks, Functional annotation
-
Functional Description:
Starting from FASTA and Eggnog-mapper annotation files, Emapper2GBK builds a GBK file that is suitable for metabolic network reconstruction with Pathway Tools, and adds the GO terms and EC numbers annotations in the GenBank file.
- URL:
- Publication:
-
Contact:
Clemence Frioux
-
Participants:
Clemence Frioux, Arnaud Belcour, Anne Siegel
7.2.9 AuCoMe
-
Name:
Automatic Comparison of Metabolisms
-
Keywords:
Bioinformatics, Workflow, Metabolic networks, Omic data, Data analysis
-
Functional Description:
AuCoMe is a Python package that aims at reconstructing homogeneous metabolic networks and pan-metabolism starting from genomes with heterogeneous levels of annotations. Four steps are composing AuCoMe. 1) It automatically infers annotated genomes from draft metabolic networks thanks to Pathway Tools and MPWT. 2) The Gene-Protein-Reaction (GPR) associations previously obtained are propagated to protein orthogroups in using Orthofinder and, an additional robustness criteria. 3) AuCoMe checking the presence of supplementary GPR associations by finding missing annotation in all genomes. In this step, the tools BlastP, TblastN and, Exonerate are called. 4) It adding spontaneous reactions to metabolic pathways that were completed by the previous steps. AuCoMe generates several outputs to facilitate the analysis of results: tabuled files, SBML files, PADMET files, supervenn and a dendogram of reactions.
- URL:
- Publication:
-
Contact:
Anne Siegel
-
Participants:
Arnaud Belcour, Jeanne Got, Meziane Aite, Ludovic Delage, Jonas Collen, Clemence Frioux, Catherine Leblanc, Simon M. Dittami, Samuel Blanquart, Gabriel V. Markov, Anne Siegel
7.2.10 mpwt
-
Keywords:
Metabolic networks, Multi-processor
-
Functional Description:
mpwt is a Python package for running Pathway Tools on multiple genomes using multiprocessing. More precisely, it launches one PathoLogic process for each organism. This allows to increase the speed of draft metabolic network reconstruction when working on multiple organisms.
- Publication:
-
Contact:
Anne Siegel
-
Participants:
Arnaud Belcour, Anne Siegel, Clemence Frioux, Meziane Aite
7.3 Open data
All our published works provide open access to the datasets, as well as the softwares and when relevant the studies as notebooks or docker images.
- 12 dataset: zenodo DOI; software/scripts: git repository
- 14 dataset: git repository; software/scripts: git repository
- 15 dataset and scripts
- 11 dataset: zenodo DOI; software/scripts: git repositorydocker image
- 17 dataset: not applicable; software/scripts: git repository
- 13 dataset: DOI; software/scripts: not applicable
- 16 dataset: GEO GSE70838; software/scripts: git repository
- 18 dataset: dataset: GEO GSE70838; software/scripts: git repository and git repository
8 New results
————————————-
8.1 Scalable methods to query data heterogeneity
Participants: Emmanuelle Becker, Cécile Beust, Olivier Dameron, Camille Juigné, Francois Moreews, Nathalie Théret, Yael Tirlet.
Extracting robust information from BioPAX databases. [E. Becker, O. Dameron, C. Juigné, F. Moreews, A. Siegel, N. Théret] 16, 17, 21
- The utilization of ontologies and standardized formats facilitates interoperability but does not completely address all challenges associated with integrating heterogeneous data types and sources. The Biological Pathway Exchange format (BioPAX) facilitates the integration of data sources describing interactions, involving molecular complexes that play a major role in the regulation of biological pathways. However databases utilizing the BioPAX format frequently exhibit redundant molecular complexes with identical properties but distinct identifiers. Furthermore, these databases often contain invalid complexes, in which the components themselves are complexes, resulting in a recursive representation that differs from the flat representation required by the format specifications. Consequently, such non-conformity and redundancy introduce modifications within the graph, which impact the subsequent analyses. We proposed reproductible and semantically-rich SPARQL queries for identifying and fixing non-conformity and redundancy in BioPAX databases, and evaluate the consequences of fixing these non-conformities and redundancies in the Reactome database. Firstly, we show that they introduce genericity problems, as redundant representations mask implicit redundancies. Importantly, we also measure how these non-conformities lead to structures that artificially modify the topology of the graph, increasing the path length between graph nodes and compromising the analysis of the interaction network 17.
- This corrected graph can further be used as an input to link transcriptomics and metabolomics data measured in the context of understanding inter-individual variability of feed efficiency in growing pigs. We elucidate the process of identifying modules of co-expressed genes associated to feed efficiency and their connections with metabolites and fatty acids concentrations in 16. Our study establishes a link between transcriptome and metabolome data, revealing connections between immunity and fatty acid composition 18. These transcriptomic and metabolic data were further mapped on the corrected Reactome BioPAX graph, revealing that co-expressed transcripts can be connected in the corrected BioPAX graph 21.
- These different results were the support of the PhD of Camille Juigné "Integration and analysis of heterogeneous biological data through multilayer graph exploitation to gain deeper insights into feed efficiency variations in growing pigs", co-supervized by the IRISA Dyliss Team and the INRAe Pegase Team (F. Gondret), and defended on December 1st 2023 21.
Identifying meaningful query modules from a collection of SPARQL queries. [O. Dameron, A. Regnier] 24
- Creating SPARQL queries requires users to acquire a precise undestanding of the SPARQL endpoint data schema, which is typically tedious. This task can be facilitated by relying on a collection of previous queries that can be adapted or combined to create new ones. We developped a method to identify query modules, i.e. portions of SPARQL queries that are shared among multiple queries, and investigated whether these modules correspond to biologically-relevant notions, and how they can be combined to create new queries 24.
8.2 Metabolism: from protein sequences to systems ecology
Participants: Arnaud Belcour, Catherine Belleannée, Samuel Blanquart, Matthieu Bouguéon, Nicolas Buton, François Coste, Olivier Dennler, Pablo Espana-Gutierrez, Jeanne Got, Pauline Hamon-Giraud, Yann Le Cunff, Victor Mataigne, Baptiste Ruiz, Anne Siegel, Nathalie Théret, Yael Tirlet.
Phylogenetic inference of functional sequence modules in ADAMTS-TSL proteins [C. Belleannée, S. Blanquart, F. Coste, O. Dennler, N. Théret] 14.
- The vast majority of proteins have a modular multidomain organization. Domains are conserved building blocks of proteins that are widely used to characterize and predict protein functions. However, the organization of multidomain proteins underlies a great complexity and their biological role is generally not the sum of the functions attributed to each domain. To address this problem, it is necessary to develop new methods to better identify functional signatures. Here, we developed a framework based on partial local multiple alignments (to find conserved sequence modules) and phylogenetic reconciliation methods (to integrate the evolution of species, genes, sequence modules, and Protein-Protein Interactions). Application of our framework to the search for functional sequence modules in extracellular matrix proteins from the ADAMTS (A Disintegrin-like and Metalloproteinase with ThromboSpondin motif) and ADAMTSL (ADAMTS-like) family, enabled to highlight sequence signatures potentially involved in Protein-Protein Interactions 14.
Modeling proteins with crossing dependencies [F. Coste] 23
- The PP suite 63 enables to align pairs of Potts model for a comparison of protein sequences that takes into account the coevolution of residues in the sequences. Yet, because of their intrinsic overparametrization, and because of sampling biases that could not be easily handled, the inferred Potts models to be aligned are often not comparable. We studied those issues, first by searching for a relevant canonical form of Potts models to get rid of unwanted parameters divergence, and then by exploring how explicit covariance-based methods that are able to overcome these sampling issues could be adapted to directly infer comparable models 23.
Deep attention networks for enzyme class predictions [N. Buton, F. Coste, Y. Le Cunff] 12 20
- We studied the interest of Transformer deep neural networks for the functional annotation of sequences by focusing on the prediction of enzymatic classes. Our EnzBert transformer models, trained to predict enzyme commission (EC) numbers by specialization of a protein language model, were able to significantly outperform state-of-the-art tools for monofunctional enzyme class prediction based on sequences only. We also showed that the attention of Transformers provides an interesting built-in mechanism for the interpretabitlity of these predictions by proposing a simple aggregation of the attention maps which was on par with, or better than, other classical interpretability methods on predicting the enzymatic sites of enzymes 12. This work was part of Nicolas Buton's Ph.D., defended in October 2023 20 and has been presented by Nicolas in JOBIM 2022 37.
Comparison of metabolic networks based on heterogeneous annotation sets [A. Belcour, S. Blanquart, J. Got, P. Hamon-Giraud, V. Mataigne, A. Siegel] 11
- Comparative analysis of genome-scale metabolic networks (GSMNs) may yield important information on the biology, evolution, and adaptation of species. However, it is impeded by the high heterogeneity of the quality and completeness of structural and functional genome annotations, which may bias the results of such comparisons. To address this issue, we developed AuCoMe, a pipeline to automatically reconstruct homogeneous GSMNs from a heterogeneous set of annotated genomes without discarding available manual annotations. We tested AuCoMe with three data sets, one bacterial, one fungal, and one algal, and showed that it successfully reduces technical biases while capturing the metabolic specificities of each organism. Our results also point out shared and divergent metabolic traits among evolutionarily distant algae, underlining the potential of AuCoMe to accelerate the broad exploration of metabolic evolution across the tree of life.
Dynamic genome-based metabolic modeling of the predominant cellulolytic rumen bacterium Fibrobacter succinogenes S85 [J. Got, A. Siegel] 15
- Fibrobacter succinogenes is a cellulolytic bacterium that plays an essential role in the degradation of plant fibers in the rumen ecosystem. It converts cellulose polymers into intracellular glycogen and the fermentation metabolites succinate, acetate, and formate. We developed dynamic models of F. succinogenes S85 metabolism on glucose, cellobiose, and cellulose on the basis of a network reconstruction done with the automatic reconstruction of metabolic model workspace. The accuracy of the models was acceptable in simulating F. succinogenes carbohydrate metabolism with an average coefficient of variation of the root mean squared error of 19%. The resulting models are useful resources for investigating the metabolic capabilities of F. succinogenes S85, including the dynamics of metabolite production. Such an approach is a key step toward the integration of omics microbial information into predictive models of rumen metabolism.
8.3 Regulation and signaling: detecting complex and discriminant signatures of phenotypes
Participants: Emmanuelle Becker, Catherine Belleannée, Samuel Blanquart, Mathieu Bougueon, Olivier Dameron, Anne Siegel, Nathalie Théret, Kérian Thuillier.
Creation of predictive models for liver fibrosis [M. Bougueon, N. Théret] 19. 33.
- A rule-based multiscale model of hepatic stellate cell plasticity 19 Hepatic stellate cells (HSC) are the source of extracellular matrix (ECM) whose overproduction leads to fibrosis, a condition that impairs liver functions in all chronic liver diseases. Understanding the dynamics of HSCs is required to develop new therapeutic approaches. In this work, we used the Kappa graph rewriting language to develop the first rule-based model describing the dynamics of HSCs during liver fibrosis and its reversion. Kappa offers a rule-centric approach where interaction rules locally modify the state of a system that is defined as a graph of components, connected or not. HSCs are modeled as agents presenting seven cell physiological states and interacting with TGFB1 molecules that regulate HSC activation and the secretion of type I collagen, the main component of the ECM. We introduced counters to scale the intermediate steps between cell states, and tokens to describe TGFB1 and type I collagen quantities thereby highly reducing the computational cost. Simulation studies revealed the critical role of the HSC inactivation process during fibrosis progression and reversion. We further have demonstrated the model’s sensitivity to TGFB1 parameters, suggesting its adaptability to a variety of pathophysiological conditions in which TGFB1 release associated with the inflammatory response differs. Using new experimental data from a mouse model of CCl4-induced-liver fibrosis, we validated the predicted ECM dynamics. Our model further predicts the accumulation of inactivated HSCs during chronic liver disease. By analyzing RNA sequencing data from patients with non-alcoholic steatohepatitis (NASH) associated with liver fibrosis, we confirmed this accumulation, identifying iHSCs as novel markers of fibrosis progression. Overall, our study provides the first model of HSC dynamics in chronic liver disease that can be used to explore the regulatory role of iHSCs in liver homeostasis, but our model can also be generalized to fibroblasts during repair and fibrosis in other tissues.
9 Partnerships and cooperations
9.1 International initiatives
9.1.1 Associate Teams in the framework of an Inria International Lab or in the framework of an Inria International Program
Participants: Anne Siegel.
SymBioDiversity
Title: Symbolic and numerical mining and exploration of functional biodiversity
Duration: 2020–2024
Coordinator: Alejandro MAASS (amaass@dim.uchile.cl)
Partners
- Universidad de Chile (Chili)
Inria contact: Anne Siegel
Summary: The project aims at developing methods combining data-mining, reasoning and mathematical modeling to efficiently analyze massive data about microbial biodiversity in extreme environment and identify families of species which characterize environmental niches. The partnership combines Inria Team Dyliss (systems biology, reasoning), Pléiade (systems biology, biodiversity), the chilean Center of Mathematical Modeling (modeling of ecosystems), Inria Chile (data mining, transfer) and chilean biologist partners experts in biodiversity (universidad catholica). As the team started in 2020 during the pandemic crisis, Inria decided to extend it for three additional years.
9.2 International research visitors
9.2.1 Visits to international teams
Research stays abroad
Olivier Dameron
- Visited institution: University of Sherbrooke
-
Country:
Canada
-
Dates:
10th–14th July 2023
-
Context of the visit:
École d'été interdisciplinaire en numérique de la santé
-
Mobility program/type of mobility:
Summer school, chaire en MEI en numérique de la santé du ministère de l’Économie et de l’Innovation du Québec
9.3 European initiatives
9.3.1 Other european programs/initiatives
ERC HoloE2Plant, Exploring the Holobiont concept through a Plant Evolutionary Experiment study
Participants: Moana Aulagner, Samuel Blanquart, Anne Siegel.
Exploring the Holobiont concept through a Plant Experimental Evolution study. In her ERC project, Claudia Bartoli aims at validating the holobiont concept, highlighting how the interactions with its microbiota influence a species evolution. The study will apply to a host/pathogen system, Brassica rapa / Rhizoctonia solani, associated with bacterial and fungal synthetic communities. Examining nine plant generations in an experimental-evolution apparatus should reveal the molecular outcomes of the applied selective pressures. 2022-2027, total of the grant 1500k€.
9.4 National initiatives
DeepImpact : Deciphering plant-microbiome interactions to enhance crop defense to bioagressors
Participants: Samuel Blanquart, Olivier Dameron, Jeanne Got, Victor Mataigne, Pauline Hamon-Giraud, Anne Siegel.
DEEP IMPACT is a multidisciplinary consortium-based project that aims at combining ecology, biology, plant genetics and mathematics to identify, characterize and validate the microbial communities, plant communities and abiotic factors (including agricultural managements) explaining variation in Brassica napus and Triticum aestivum resistance to several pests. For this, we will start from an in situ approach by characterizing 100 fields (50 for each crop species) for both habitat (climatic and edaphic variables) and biotic (microbiota, virome, weed communities, pest attacks and pathobiota prevalence) features. Information from this broad characterization will be integrated into sparse and correlative statistical models to describe the relative part of the variance explained by both habitat and biotic features and correlated with a reduction of pest's attacks. This analysis will allow us to identify a combination of microbial species and soils, correlated with an increase of crop's resistance to pests. These microbial consortia will be isolated by taking advantages of newly developed culturomics methods and characterized by both whole genome sequencing and biochemical assays. Synthetic Consortia (SynComs) will be reconstructed to test their efficacy on a broad range of pests attacking both crops. 2021–2026. Dyliss grant: 176k€.
SEABIOZ : Potential microbial origins of the biostimulant properties of extracts from a brown algae holobinte
Participants: Samuel Blanquart, Olivier Dameron, Jeanne Got, Anne Siegel.
For sustainable agriculture, new bio-based solutions include biocontrol and the use of plant biostimulants such as aqueous seaweed extracts. The most widely exploited biomass for biostimulant production is the brown seaweed Ascophyllum nodosum and its commercial extracts, including products from the Roullier Group, have demonstrated their ability to improve plant growth and mitigate certain abiotic and biotic stresses. A unique feature of the alga is its mutualistic association with the fungal endophyte Mycophycias ascophylli and other microbes constituting an holobiont. Many questions remain as to the nature and origin of the active compounds in algal extracts. Are these bioactive metabolites produced by the host or by its microbiota? The main objective of SEABIOZ is to answer these questions by combining a multi-omics approach and systems biology. 2021–2024. Dyliss grant: 120k€.
IDEALG (ANR/PIA-Biotechnology and Bioresource)
Participants: Arnaud Belcour, François Coste, Jeanne Got, Anne Siegel.
The project gathers 18 partners from Station Biologique de Roscoff (coordinator), CNRS, IFREMER, UEB, UBO, UBS, ENSCR, University of Nantes, INRA, AgroCampus, and the industrial field in order to foster biotechnology applications within the seaweed field. Dyliss is co-leader of the WP related to the establishment of a virtual platform for integrating omics studies on seaweed and the integrative analysis of seaweed metabolism. Major objectives are the building of brown algae metabolic maps, metabolic flux analysis and the selection of symbiotic bacteria for brown algae. We will also contribute to the prediction of specific enzymes (sulfatases and haloacid dehalogenase)14. 2012–2021. Total grant: 11M€. Dyliss grant: 534k€.
ENDOVIRE (ANR)
Participants: Emmanuelle Becker, Olivier Dameron, Yael Tirlet-Greiner.
The whole ANR project gathers 4 partners : the BIPAA platform (INRAe), the DGIMI laboratory, the BF2I laboratory and the Dyliss team of IRISA. The project is focused about the understanding of how genes of a endogeneized viral genome in a parasitoid wasp are the activated and regulated. The available data produced by the consortium will cover genomics, epigenomics, pathways, regulation and orthology. We will contribute to identify the key actors involved in the activation of parasitoids genes, to propose a data and knowledge integration framework for the data of the global project, and to develop integrative data analysis methods for elucidating the mechanism involving the key actors identified in the first point. It will consist in proposing a library of queries (which contains a reasoning part), and further to propose regulation mechanisms based on heterogeneous -omics data across interacting organisms. To tackle the different challenges, our appoach will be based on (1) adequate statistical analysis workflows or methods, (2) Semantic Web technologies and AskOmics developed within the team, (3) knowledge-guided traversal strategies across multiplex graphs.
2023–2025. Total grant: 630k€. Dyliss grant: 176k€.
9.4.1 Programs funded by Inria
Défi Inria OmicFinder
Participants: Olivier Dameron.
- Coordinator: Pierre Peterlongo
- Duration: 48 months (May 2023 - May 2027)
-
Partners: Inria teams: Dyliss, Zenith, Taran.
External partners are CEA-GenoScope, Elixir, Pasteur Institute, Inria Challenge OceanIA, CEA-CNRGH, and Mediterranean Institute of Oceanography.
- Description: The project aims to provide a novel global search engine making it possible to query nucleotidic sequences against the vast amount of publicly available genomic data. The central algorithmic idea of a genomic search engine is to index and query small exact words (hundreds of billions over millions of datasets), as well as the associated metadata.
- website: project.inria.fr/omicfinder
- We anticipate the recruitment of a PhD student in 2024 for working on datasets semantic annotation, a querying mechanism, and the automatic generation of descriptions characterizing query results.
10 Dissemination
10.1 Promoting scientific activities
Participants: Emmanuelle Becker, Catherine Belleannée, Samuel Blanquart, François Coste, Olivier Dameron, Jeanne Got, Yann Le Cunff, Anne Siegel, Nathalie Théret.
10.1.1 Scientific events: organisation
General chair, scientific chair
- Chair of 16th edition of the International Conference on Grammatical Inference (ICGI 2023), Rabat, Morocco [F. Coste]
Member of the organizing committees
- Colloque "limites planétaires et enjeux de durabilité", Juillet 2023, Paris, CNRS [A. Siegel]
- Colloque " reproductibilité et réplicabilité", Octobre 2023, Paris, CNRS [A. Siegel]
- Colloque "Santé environnement travail : des données à la preuve", Juin 2023, Lyon, CNRS [A. Siegel]
- Competition "Transformers+RNN: Algorithms to Yield Simple and Interpretable Representations" (TAYSIR) [F. Coste]
10.1.2 Scientific events: selection
Member of the conference program committees
- ISMB-2023 (International Symposium on Molecular Biology) [A. Siegel]
- ICFP workshop Declarative Programming in Biology and Medicine [A. Siegel]
- Jobim 2023 (Journées Ouvertes Biologie Informatique Mathématiques), France [O. Dameron, A. Siegel]
- SWAT4HCLS 2023, Basel Switzerland (international conference Semantic Web Applications and Tools for Health Care and Life Science) [O. Dameron]
- Journée Santé et IA (plateforme IA, Strasbourg 03-07 juillet 2023) [O. Dameron]
Reviewer
- SWAT4HCLS 2023 [O. Dameron]
- Jobim 2023 [O. Dameron]
- IA et santé [O. Dameron]
10.1.3 Journal
Member of the editorial boards
- Journal of Biomedical Semantics [O. Dameron]
Reviewer - reviewing activities
- Briefings in Bioinformatics [O. Dameron]
- Journal of Biomedical Semantics [O. Dameron]
- ISME Journal, Genome Biology [A. Siegel]
- Bioinformatics [F. Coste]
10.1.4 Invited talks
- Seminar of the CNB-CSIC lab, In silico screening of metabolic functions of large-scale genomes and/or OTU : towards comparative metabolism?, Madrid, December 2023 [A. Siegel]
- École d’été interdisciplinaire en numérique de la santé (EINS 2023), 10–14th July Univ. Sherbrooke Canada. « Intégration et interrogation avancées de données et de connaissances grâce au Web Sémantique » [O. Dameron]
- Journée de lancement du PEPR Atlasea Des génomes marins à la synthèse in silico et in vivo de molécules, Paris, Janvier 2023 [A. Siegel]
- Colloque Ferment IA. Étude Et Comparaison De Cartes Métaboliques De Centaines De Souches Bactériennes. Vers La Prédiction De Cocktails Produisant Des Composés Cibles ?, Saclay, Septembre 2023 [A. Siegel]
- Journée du GDR BIOSS, Étude Et Comparaison De Cartes Métaboliques : s’adapter au triptyque connaissances – données – passage à l’échelle, Lille, Novembre 2023 [A. Siegel]
- Institut Français de Bioinformatique : École thématique sur la Bioinformatique Intégrative (ETBII, 16th–20th January, Fréjus) : animateur du thème « Web Sémantique » [O. Dameron]
- Journées du Réseau Inrae PEPI IBIS (15 septembre 2023, Paris): Predicting enzymatic function of protein sequences with attention [F. Coste]
10.1.5 Leadership within the scientific community
National responsibilities
- Deputy Scientific Directory (CNRS, INS2I), in charge of interdisciplinarity between numerical sciences and other disciplines, gender equality in computer sciences, groupements de recherches (GDR) [A. Siegel]
Local responsibilities
- Organisation of the bioinformatics teams (Dyliss, GenOuest and GenScale as well as members of other bioinformatics teams in Rennes) weekly seminars [S. Blanquart]
- Chargé de mission "Numérique et Environnement" for Inria centre at Rennes University [S. Blanquart]
- Chargé de mission "Biologie et Santé Numériques" for Inria centre at Rennes University [F. Coste]
- Scientific Director of the GenOuest platform [Y. Le Cunff]
- Scientific Advisory Board of the GenOuest platform [O. Dameron]
- Responsability of the IRISA laboratory "Health-biology" cross-cutting axis [Y. Le Cunff]
- Delegate to research integrity at the University of Rennes 1 [N. Théret]
- Scientific Advisory Board of the BioGenOuest network (37 platforms) [E. Becker]
10.1.6 Scientific expertise
Evaluation of European project
- COST action, 2023 [A. Siegel]
- ERC Consolidator program, 2023 [A. Siegel]
Evaluation of National project
- ANR project, 2023 [E. Becker]
10.1.7 Research administration
Institutional boards for the recruitment and evaluation of researchers
- National Council of Universities (Conseil National des Universités - CNU), section 27 [F. Coste]
- Associate professor selection committee Université de Rennes SVE–IRSET [E. Becker president, O. Dameron]
- Commission de validation de CDIsation Univ Rennes SVE [O. Dameron]
Scientific councils
- Scientific referent (for CNRS) of the PEPR exploratoire Molecularxiv [A. Siegel]
- Comité de pilotage of the Mission for Interdisciplinarity (MITI) at CNRS [A. Siegel]
- Scientific council of the PPR Autonomy [A. Siegel]
Local responsibilities
- Member of the Inria Rennes center council [J. Got]
- Member of the Biology department council [Y. Le Cunff]
- CUMI (Commission des utilisateurs des moyens informatiques) of Inria Rennes [F. Coste]
- Member of the social committee of Univ. Rennes [C. Belleannée]
- Member of the emergency aid commission of Univ. Rennes and Rennes 2 [C. Belleannée]
10.2 Teaching - Supervision - Juries
Participants: Emmanuelle Becker, Catherine Belleannée, Samuel Blanquart, François Coste, Olivier Dameron, Jeanne Got, Camille Juigné, Yann Le Cunff, Anne Siegel, Nathalie Théret, Kerian Thuillier, Yael Tirlet.
10.2.1 Teaching
- Master : E. Becker, "Rappels de Statistiques", 15h, Master 1 in Bioinformatics, Univ. Rennes, France
- Master : E. Becker, "Short Introduction to R", 31h, Master 1 in Bioinformatics, Master 1 in Ecology and Envirronment, Univ. Rennes, France
- Master : E. Becker, "Data Visualisation and Manipulation", 12h, Master 1 in Bioinformatics, Univ. Rennes, France
- Master : E. Becker, "Object oriented programming", 30h, Master in Bioinformatics, Univ. Rennes 1, France
- Master : E. Becker, "Method", 15h, Master 2 in Computer Sciences, Univ. Rennes, France
- Master : E. Becker, "Advanced Python Programming", 38h, Master 2 in Bioinformatics, Univ. Rennes, France
- Master : E. Becker, "Biological netorks", 28h, Master 2 in Bioinformatics, Univ. Rennes, France
- Master : E. Becker, "Introduction to Bioinformatics", 3h, Master MEEF Biology, Univ. Rennes, France.
- Master : E. Becker, "Python for Life Sciences 1", 21h, Master minor in Bioinformatics, Univ. Rennes, France
- Master : E. Becker, "Insertion Professionnelle et tables rondes", 4h, Master 1 and Master 2 in Bioinformatics, Univ. Rennes, France
- Master: C. Belleannée, 18h, responsable des stages, Master 1 Ingenierie logicielle et Master 1 Cloud et Réseaux, Univ. Rennes, France
- Licence: C. Belleannée, responsable de la 2eme année du parcours Oui-si du portail ISTN, Univ. Rennes, France
- Licence: C. Belleannée, responsable de la Journée Portes Ouvertes pour l'Istic, Univ. Rennes, France
- Licence: C. Belleannée, Enseignante référente, 10h, L1 informatique, Univ. Rennes, France
- Licence: C. Belleannée, Langages formels, 17h, L3 informatique, Univ. Rennes, France.
- Licence: C. Belleannée, Projet professionnel et communication, 16h, L1 informatique, Univ. Rennes, France
- Licence: C. Belleannée, Spécialité informatique : Functional and immutable programming , 44h, L1 informatique, Univ. Rennes, France
- Master: C. Belleannée, Algorithmique du texte et bioinformatique, 10h, M1 informatique, Univ. Rennes, France
- Master: C. Belleannée, Programmation logique et contraintes, 32h, M1 informatique, Univ. Rennes, France
- Licence: C. Belleannée, Données structurées et bases de données, 20h, L2 informatique, Univ. Rennes, France
- Licence: C. Belleannée, Outils formels pour l'informatique, 24h, L2 informatique, Univ. Rennes, France
- Licence: C. Belleannée, Fondements mathématiques, 49h, L1 informatique, Univ. Rennes, France
- Master: F. Coste, Supervised machine learning, 10h, M2 Science Informatique, Univ. Rennes, France
- Licence: O. Dameron, "Programmation 1", 98h, Licence 1 informatique, Univ. Rennes, France
- Licence: O. Dameron, "Complément informatique", 24h, Licence 1 informatique, Univ. Rennes, France
- Licence: O. Dameron, "Data analysis and statistics", 24h, Licence 2 informatique, Univ. Rennes, France
- Licence: O. Dameron, "Graph Modeling and Algorithms", 21h, Licence 2 informatique, Univ. Rennes, France
- Licence: O. Dameron, "Programmation", 36h, Licence 3 miage, Univ. Rennes, France
- Master: O. Dameron, "Semantic Web", 10h, Master 1 miage, Univ. Rennes 1, France
- Master: O. Dameron, 2h, "Internship", Master 1 in bioinformatics, Univ. Rennes 1, France
- Master: O. Dameron, 20h, "Data Engineering in Life Science", Master 2 in bioinformatics, Univ. Rennes 1, France
- Master: O. Dameron, 28h, "Internship", Master 2 in bioinformatics, Univ. Rennes 1, France
- Doctoral level: A. Siegel, Ecole jeunes chercheurs "Modélisation des systèmes biologiques", Porquerolles, France.
- Licence : Y. Le Cunff "Modélisation des phénomènes du vivant", 30h, L2 Biologie, Univ. Rennes 1, France
- Master: Y. Le Cunff, "Apprentissage statistique", 110h, Master 1 in Bioinfortmatics Univ. Rennes 1, France
- Master: Y. Le Cunff, "Biologie aux interfaces", 25h, Master 1 in Biology, Univ. Rennes 1, France
- Master: Y. Le Cunff,"Simulating dynamic systems in biology", Master 2 in bioinformatics, 20h, Univ. Rennes 1, France
- Master: Y. Le Cunff, "Applied Interdisciplinarity", 20h, Master 2 in biology, Univ. Rennes 1, France
- PhD program: Y. Le Cunff, "Introduction to Machine Learning", 20h, FdV PhD Program, Sorbonne Paris Université, Paris, France
10.2.2 Supervision
PhD thesis
- PhD in progress: Moana Aulagner, Modeling microbiota interactions in plants to build synthetic microbial communities for enhanced biocontrol and biostimulation, started in Oct 2023, supervized by S. Blanquart, A. Siegel and C. Bartoli-Kautski (INRAe IGEPP)
- PhD in progress: Moussa Baddour, Extraction de phénotypes à partir de comptes-rendus médicaux textuels et mise en relation avec le génotype, started in May 2023, supervized by O. Dameron, M. De Tayrac (Rennes Hospital), S. Paquelet (b<>com) and T. Labbé (Orange)
- PhD in progress: Cécile Beust, Knowledge-guided rules for generating context-specific views on a knowledge graph: application to biological networks, started in Oct 2023, supervized by E. Becker, O. Dameron and N. Théret
- PhD: Matthieu Bouguéon, Modélisation prédictive pour le ciblage thérapeutique du TGF-beta dans les pathologies chroniques hépatiques, started in Oct 2020, defended in Dec 2023 supervized by N. Théret and A. Siegel
- PhD: Nicolas Buton, Deep learning for proteins functional annotation : novel architectures and interpretability methods, started in Oct 2020, defended in Oct 2023 supervized by F. Coste, O. Dameron and Y. Le Cunff
- PhD in progress: Pablo Espana Gutierrez, Learning models with explicit dependencies between residues to predict protein functions, started in Oct 2023, supervized by F. Coste and O. Dameron
- PhD: Camille Juigné, Analyse des données biologiques hétérogènes par exploitation de graphes multicouches pour comprendre et prédire les variations d’efficacité alimentaire chez le porc, started in Dec 2020, defended in Dec 2023, supervized by E. Becker and F. Gondret (INRAe Pegase)
- PhD in progress: Corentin Lucas, Integration of multi-modal data for longitudinal follow-up of Crohn’s disease patients, started in Oct 2023, supervized by E. Becker, Y. Le Cunff
- PhD in progress: Baptiste Ruiz, Algorithmes d’apprentissage automatique appliqués au microbioteIntégration de connaissances a priori pour de meilleures prédictions de phénotype, started in Oct 2021, supervized by Y. Le Cunff, A. Siegel
- PhD in progress: Kerian Thuillier, Inférence de règles booléennes contrôlant des modèles hybrides de systèmes biologiques multi-échelles, started in Oct 2021, supervized by A. Siegel and L. Paulevé (LABRI)
- PhD in progress: Yael Tirlet-Greiner, Integrative method for multi-omics data analysis with application to the activation and regulation of an endogeneized viral genome in a parasitoid wasp, started in Oct 2023, supervized by E. Becker, O. Dameron and F. Legeai (INRAe)
Internship
- M2 internship: Moana Aulagner, Reconstruction of metabolic networks from PacBio assembly genomes for identifying representative Synthetic Communities of the Brassica rapa microbiota, Jan.–Jul. 2023, supervized by S. Blanquart and C. Bartoli-Kautski (INRAe IGEPP)
- M2 internship: Pablo Espana Gutierrez, Learning canonical Potts models, Jan.–Jun. 2023, supervized by F. Coste
- M2 internship: Corentin Lucas, Étude des interactions métaboliques entre les algues brunes et leur microbiote, Apr.–Jul. 2023, supervized by S. Blanquart, J. Got and A. Siegel
- M1 internship: Alexandre Lerévérend, Identification de règles de régulation du métabolisme sur des réseaux biologiques à grande échelle, Apr.–Jul. 2023, supervized by K. Thuillier and A. Siegel
- Engineering internship: Sophia Pham, Analyse de l'expression des transcrits dans l'ovaire chez Hyposoter didymator pour identifier les transcrits différentiellement exprimés lors de la morphogénèse de l'ovaire, Apr.–Jul. 2023, supervized E. Becker and Y. Tirlet
- M1 internship: Alix Régnier, Identification systématique de modules dans une collection de requêtes SPARQL, Apr.–Jun. 2023, supervized by O. Dameron
- M1 internship: Oceane Carpentier, Analyse conjointe de données transcriptomiques et métabolomiques dans les réseaux métaboliques au format BioPax, Apr.–Jun. 2023, supervized by E. Becker and C. Juigné
10.2.3 Doctoral advisory committees (CSID)
- Yvon Awuklu, Université de Bordeaux [O. Dameron]
- Tiphaine Casy, Université de Rennes [O. Dameron]
- Guénolé Dande, Université de Rennes [O. Dameron]
- Simon Brocard, Nantes Université [F. Coste]
- Guillaume Doré, Université de Rennes [E. Becker]
10.2.4 Juries
Referee of PhD thesis
- Nicolas Levy, ENS Lyon [A. Siegel]
- Timothée Jean-Rémi Bigot, Aix-Marseille University [E. Becker]
- Marine Djaffardjy, Paris-Saclay University [O. Dameron]
Member of PhD thesis juries
- Camille Juigné, Univ Rennes [E. Becker]
- Antoine Salomon, Univ Rennes [O. Dameron, president]
Member of habilitation thesis juries
- Nolwenn Le Meur, Univ Rennes [E. Becker]
10.3 Popularization
Participants: Emmanuelle Becker, Yann Le Cunff.
10.3.1 Articles and contents
- Trousse à projet, Territoire du numérique éducatif "le numérique, c’est que pour les garçons?" tne.trousseaprojets.fr/professionnel-education-nationale/idees-recues-sur-le-numerique
10.3.2 Interventions
- Trousse à projet, Territoire du numérique éducatif "le numérique, c’est que pour les garçons?"
- A la découverte de la recherche, "Informatique pour la biologie et la santé" (Lycée Assomption Rennes, 5 avril 2023) [E. Becker]
- "How will we learn with next-gen AI ?" (Learning Planete Institute, Paris, France) [Y. Le Cunff]
- Table ronde "Intelligence artificielle" (CSIESR, Toulon, France) [Y. Le Cunff]
- "Impact de l'IA sur l'enseignement", Interview in Journal du Dimanche [Y. Le Cunff]
- Conference "Les nouvelles écritures et l'IA" (Journées nationales de l'ANSTIA, Rennes, France) [Y. Le Cunff]
- "enjeux sociétaux de l'IA" (SciencesPo Rennes et élus du département) [Y. Le Cunff]
11 Scientific production
11.1 Major publications
- 1 articleTraceability, reproducibility and wiki-exploration for "à-la-carte" reconstructions of genome-scale metabolic models.PLoS Computational Biology145e1006146May 2018HALDOIback to text
- 2 inproceedingsLogol: Expressive Pattern Matching in sequences. Application to Ribosomal Frameshift Modeling.PRIB2014 - Pattern Recognition in Bioinformatics, 9th IAPR International Conference8626Lukas KALLStockholm, SwedenSpringer International PublishingAugust 2014, 34-47HALDOIback to text
- 3 articleOptimal Threshold Determination for Interpreting Semantic Similarity and Particularity: Application to the Comparison of Gene Sets and Metabolic Pathways Using GO and ChEBI.PLoS ONE2015, 30HALDOI
- 4 articlePutative bacterial interactions from metagenomic knowledge with an integrative systems ecology approach.MicrobiologyOpen512015, 106-117HALDOIback to text
-
5
inproceedingsIdentifying Functional Families of Trajectories in Biological Pathways by Soft Clustering: Application to TGF-
Signaling.CMSB 2017 - 15th International Conference on Computational Methods in Systems BiologyLecture Notes in Computer SciencesDarmstadtSeptember 2017, 17HALback to text - 6 inproceedingsAutomated Enzyme classification by Formal Concept Analysis.ICFCA - 12th International Conference on Formal Concept AnalysisCluj-Napoca, RomaniaSpringerJune 2014HALback to text
- 7 articleScalable and exhaustive screening of metabolic functions carried out by microbial consortia.Bioinformatics3417September 2018, i934 - i943HALDOI
- 8 articleHybrid Metitebolic Network Completion.Theory and Practice of Logic ProgrammingNovember 2018, 1-23HALback to text
- 9 articleMeneco, a Topology-Based Gap-Filling Tool Applicable to Degraded Genome-Wide Metabolic Networks.PLoS Computational Biology131January 2017, 32HALDOIback to text
- 10 articlecaspo: a toolbox for automated reasoning on the response of logical signaling networks families.Bioinformatics2017HALDOIback to textback to text
11.2 Publications of the year
International journals
- 11 articleInferring and comparing metabolism across heterogeneous sets of annotated genomes using AuCoMe.Genome Research33June 2023, 972 - 987HALDOIback to textback to textback to text
- 12 articlePredicting enzymatic function of protein sequences with attention.Bioinformatics3910October 2023, 1-10HALDOIback to textback to textback to text
- 13 articleBayesTICS: Local temporal image correlation spectroscopy and Bayesian simulation technique for sparse estimation of diffusion in fluorescence imaging.Biological Imaging32023, 1-12HALDOIback to text
- 14 articlePhylogenetic inference of the emergence of sequence modules and protein-protein interactions in the ADAMTS-TSL family.PLoS Computational Biology198August 2023, e1011404HALDOIback to textback to textback to text
- 15 articleDynamic genome-based metabolic modeling of the predominant cellulolytic rumen bacterium Fibrobacter succinogenes S85.mSystems83June 2023, 1-23HALDOIback to textback to text
- 16 articleSmall networks of expressed genes in the whole blood and relationships to profiles in circulating metabolites provide insights in inter-individual variability of feed efficiency in growing pigs.BMC Genomics232023, 1-20HALDOIback to textback to textback to text
- 17 articleFixing molecular complexes in BioPAX standards to enrich interactions and detect redundancies using Semantic Web Technologies.Bioinformatics3952023, btad257HALDOIback to textback to textback to text
International peer-reviewed conferences
- 18 inproceedingsCombined transcriptomics and metabolomics in the whole blood to depict feed efficiency in pigs.Book of abstracts74. Annual meeting of the european federation of animal science (EAAP)29Book of abstracts of the 74st annual meeting of the european federation of animal scienceLyon, FranceWageningen Academic Publishers2023, 516HALback to textback to text
Doctoral dissertations and habilitation theses
- 19 thesisKappa modeling of hepatic stellate cell dynamics during fibrosis development and reversion.Université de Rennes (2023-....)December 2023HALback to textback to text
- 20 thesisModèle Transformer pour l’interprétabilité et les prédictions multi-niveaux des fonctions des protéines à partir de leurs séquences.Université de RennesOctober 2023HALback to textback to text
- 21 thesisIntegration and analysis of heterogeneous biological data through multilayer graph exploitation to gain deeper insights into feed efficiency variations in growing pigs.Institut Agro - Rennes AngersDecember 2023HALback to textback to textback to text
Reports & preprints
- 22 miscSmall networks of expressed genes in the whole blood and relationships to profiles in circulating metabolites provide insights in inter-individual variability of feed efficiency in growing pigs.March 2023HAL
Other scientific publications
- 23 thesisLearning canonical Potts models.Université de rennesJune 2023HALback to textback to text
- 24 thesisIdentification systématique de modules dans une collection de requêtes SPARQL.Université de RennesJune 2023HALback to textback to text
11.3 Cited publications
- 25 articleAn integrative modeling framework reveals plasticity of TGF-Beta signaling.BMC Systems Biology812014, 30HALDOIback to textback to textback to text
- 26 articleMetage2Metabo, microbiota-scale metabolic complementarity for the identification of key species.eLife9December 2020HALDOIback to text
- 27 articleInferring Biochemical Reactions and Metabolite Structures to Understand Metabolic Pathway Drift.iScience232February 2020, 100849HALDOIback to text
- 28 articleA Framework for Web Science.Foundations and Trends in Web Science112007, 1--130back to text
- 29 articleSemantic particularity measure for functional characterization of gene sets using gene ontology.PLoS ONE91e865252014HALDOIback to text
- 30 articleAssisted transcriptome reconstruction and splicing orthology.BMC Genomics1710Nov 2016, 786URL: https://doi.org/10.1186/s12864-016-3103-6DOIback to text
- 31 articleUsing a large-scale knowledge database on reactions and regulations to propose key upstream regulators of various sets of molecules participating in cell metabolism.BMC Systems Biology812014, 32HALDOIback to textback to text
- 32 articlePutative bacterial interactions from metagenomic knowledge with an integrative systems ecology approach.MicrobiologyOpen512015, 106-117HALDOIback to text
- 33 incollectionThe rule-based model approach. A Kappa model for hepatic stellate cells activation by TGFB1.Systems Biology Modelling and Analysis: Formal Bioinformatics Methods and ToolsWileyNovember 2022, 1-76HALback to textback to text
- 34 inproceedingsKaSa: A Static Analyzer for Kappa.CMSB 2018 - 16th International Conference on Computational Methods in Systems Biology11095LNCSBrno, Czech RepublicSpringer VerlagSeptember 2018, 285-291HALDOIback to text
- 35 articleCyanoLyase: a database of phycobilin lyase sequences, motifs and functions.Nucleic Acids ResearchNovember 2012, 6HALDOIback to text
- 36 articleMetabolic Complementarity Between a Brown Alga and Associated Cultivable Bacteria Provide Indications of Beneficial Interactions.Frontiers in Marine Science7February 2020, 1-11HALDOIback to text
- 37 miscEnzBert: Deep attention network for enzyme class predictions.PosterJuly 2022, 1-1HALback to text
-
38
inproceedingsIdentifying Functional Families of Trajectories in Biological Pathways by Soft Clustering: Application to TGF-
Signaling.CMSB 2017 - 15th International Conference on Computational Methods in Systems BiologyLecture Notes in Computer SciencesDarmstadt, FranceSeptember 2017, 17HALback to text - 39 articleAnalysis of Piscirickettsia salmonis Metabolism Using Genome-Scale Reconstruction, Modeling, and Testing.Frontiers in Microbiology8December 2017, 15HALDOIback to textback to text
- 40 inproceedingsAutomated Enzyme classification by Formal Concept Analysis.ICFCA - 12th International Conference on Formal Concept AnalysisCluj-Napoca, RomaniaSpringerJune 2014HALback to text
- 41 miscPhylogenetic Functional Module Characterization of the ADAMTS / ADAMTS like Protein Family.PosterAugust 2021HALback to text
- 42 mastersthesisCaractérisation en modules fonctionnels de la famille de protéines ADAMTS / ADAMTSL.MA ThesisUniv RennesJune 2019HALback to text
- 43 phdthesisCaractérisation en modules fonctionnels des protéines ADAMTS-TSL, par approches de phylogénies.Université Rennes 1December 2022HALback to text
- 44 articleGenome and metabolic network of "Candidatus Phaeomarinobacter ectocarpi" Ec32, a new candidate genus of Alphaproteobacteria frequently associated with brown algae.Frontiers in Genetics52014, 241HALDOIback to text
- 45 articleThe genome of Ectocarpus subulatus -- A highly stress-tolerant brown alga.Marine Genomics52January 2020, 100740HALDOIback to text
- 46 articleMicrobial interactions: from networks to models.Nat. Rev. Microbiol.108Jul 2012, 538--550back to text
- 47 articleThe 2015 Nucleic Acids Research Database Issue and molecular biology database collection.Nucleic acids research43Database issue2015, D1--D5back to text
- 48 articleCyanorak v2.1: a scalable information system dedicated to the visualization and expert curation of marine and brackish picocyanobacteria genomes.Nucleic Acids Research49D1October 2020, D667--D676HALDOIback to text
- 49 bookAnswer Set Solving in Practice.Synthesis Lectures on Artificial Intelligence and Machine LearningMorgan and Claypool Publishers2012back to text
- 50 inproceedingsData integration.Meeting INRA-ISUAmes, United StatesMarch 2015, 11HALback to text
- 51 articleSynergic Effects of Temperature and Irradiance on the Physiology of the Marine Synechococcus Strain WH7803.Frontiers in Microbiology11July 2020HALDOIback to text
- 52 articleThe longissimus and semimembranosus muscles display marked differences in their gene expression profiles in pig.PLoS ONE95e964912014HALDOIback to text
- 53 articleInsights into the potential for mutualistic and harmful host-microbe interactions affecting brown alga freshwater acclimation.Molecular Ecology3232022, 703-723HALDOIback to text
- 54 articleGenome-scale metabolic models of Microbacterium species isolated from a high altitude desert environment.Scientific Reports101December 2020, 1-12HALDOIback to text
- 55 articleGenome--Scale Metabolic Networks Shed Light on the Carotenoid Biosynthesis Pathway in the Brown Algae Saccharina japonica and Cladosiphon okamuranus.Antioxidants 811November 2019, 564HALDOIback to text
- 56 articleRumen microbial genomics: from cells to genes (and back to cells).CAB Reviews Perspectives in Agriculture Veterinary Science Nutrition and Natural Resources2022August 2022HALDOIback to text
- 57 articleThe genome-scale metabolic network of Ectocarpus siliculosus (EctoGEM): a resource to study brown algal physiology and beyond.Plant JournalSeptember 2014, 367-81HALDOIback to textback to text
- 58 articleMeneco, a Topology-Based Gap-Filling Tool Applicable to Degraded Genome-Wide Metabolic Networks.PLoS Computational Biology131January 2017, 32HALDOIback to text
- 59 articleThe Transporter Classification Database (TCDB): recent advances.Nucleic Acids Res.44D1Jan 2016, D372--379back to text
- 60 articleString variable grammar: A logic grammar formalism for the biological language of DNA.The Journal of Logic Programming241Computational Linguistics and Logic Programming1995, 73 - 102URL: http://www.sciencedirect.com/science/article/pii/074310669500034HDOIback to text
- 61 articleBig Data: Astronomical or Genomical?PLoS biology1372015, e1002195back to text
- 62 phdthesisComparison of homologous protein sequences using direct coupling information by pairwise Potts model alignments.Université Rennes 1February 2021HALback to text
- 63 articlePPalign: optimal alignment of Potts models representing proteins with direct coupling information.BMC Bioinformatics22317December 2021, 1-22HALDOIback to textback to text
- 64 articleExtracellular vesicles produced by human and animal Staphylococcus aureus strains share a highly conserved core proteome.Scientific Reports101April 2020, 1-13HALDOIback to text
- 65 articleADAM and ADAMTS Proteins, New Players in the Regulation of Hepatocellular Carcinoma Microenvironment.Cancers1372021, 1563HALDOIback to text
- 66 incollectionIntegrative models for TGF-beta signaling and extracellular matrix.Extracellular Matrix Omics7Biology of Extracellular MatrixSpringerDecember 2020, 17HALDOIback to text
- 67 articleTriple Pattern Fragments: a Low-cost Knowledge Graph Interface for the Web.Journal of Web Semantics37--38March 2016, 184--206URL: http://linkeddatafragments.org/publications/jws2016.pdfDOIback to text