2023Activity reportProject-TeamERMINE
RNSR: 202224279Z- Research center Inria Centre at Rennes University
- In partnership with:Ecole Nationale Supérieure Mines-Télécom Atlantique Bretagne Pays de la Loire, Université de Rennes, CNRS
- Team name: Measuring and Managing Network operation and economics
- In collaboration with:Institut de recherche en informatique et systèmes aléatoires (IRISA)
- Domain:Networks, Systems and Services, Distributed Computing
- Theme:Networks and Telecommunications
Keywords
Computer Science and Digital Science
- A1.1.9. Fault tolerant systems
- A1.2.2. Supervision
- A1.2.4. QoS, performance evaluation
- A1.2.5. Internet of things
- A1.3.3. Blockchain
- A1.3.4. Peer to peer
- A1.3.5. Cloud
- A3.4.1. Supervised learning
- A3.4.2. Unsupervised learning
- A3.4.3. Reinforcement learning
- A3.4.6. Neural networks
- A3.4.8. Deep learning
- A6.1.2. Stochastic Modeling
- A6.2.3. Probabilistic methods
- A6.2.4. Statistical methods
- A6.2.6. Optimization
- A8.2.1. Operations research
- A8.6. Information theory
- A8.7. Graph theory
- A8.11. Game Theory
- A9.2. Machine learning
- A9.7. AI algorithmics
Other Research Topics and Application Domains
- B6.2.1. Wired technologies
- B6.2.2. Radio technology
- B6.2.4. Optic technology
- B6.3.2. Network protocols
- B6.3.3. Network Management
- B6.3.5. Search engines
- B6.4. Internet of things
- B9.6.3. Economy, Finance
1 Team members, visitors, external collaborators
Research Scientists
- Bruno Tuffin [Team leader, INRIA, Senior Researcher, HDR]
- Mael Le Treust [CNRS, Researcher, HDR]
- Gerardo Rubino [INRIA, Emeritus, HDR]
- Bruno Sericola [INRIA, Senior Researcher, HDR]
Faculty Members
- Soraya Ait Chellouche [UNIV RENNES]
- Yassine Hadjadj-Aoul [UNIV RENNES, Professor, HDR]
- Sofiene Jelassi [UNIV RENNES, Associate Professor]
- Patrick Maillé [IMT ATLANTIQUE, Professor, HDR]
- Raymond Marie [UNIV RENNES, Emeritus, HDR]
- Cesar Viho [UNIV RENNES, Professor, HDR]
Post-Doctoral Fellows
- Abdellah Kaci [UNIV RENNES, Post-Doctoral Fellow, until Feb 2023]
- Guillaume Le Gall [UNIV RENNES, Post-Doctoral Fellow, from Mar 2023 until Aug 2023]
PhD Students
- Ahcene Boumhand [ORANGE LABS, until Nov 2023]
- Abdelmounaim Bouroudi [NOKIA BELL LABS]
- Sid Hamideche [NOKIA, until May 2023]
- Ali Hodroj [UNIV RENNES and Saint-Joseph University, Beyrouth]
- Soumeya Kaada [NOKIA, until Apr 2023]
- Burak Kara [SYNAMEDIA, CIFRE, from Apr 2023]
- Wilem Lamdani [UNIV RENNES, from Oct 2023]
- Parsa Rajabzadeh [Bell Labs, Nokia Networks, CIFRE, from Sep 2023]
- Amine Rguez [EXFO SOLUTIONS, until Nov 2023]
- Orland-Medy Saizonou [INRIA, from Nov 2023]
Technical Staff
- Kaouther Boulaouinat [INRIA, Engineer, from Nov 2023]
- Ghina Dandachi [INRIA, Engineer, from Sep 2023]
- Ghina Dandachi [INRIA, Engineer, until Mar 2023]
- Federico Giarre [UNIV RENNES, Engineer, from Feb 2023 until Jul 2023]
- Wilem Lamdani [UNIV RENNES, from Jul 2023 until Jul 2023]
- Antoine Lesieur [INRIA, Engineer, until Nov 2023]
- Karthika Satheesh [UNIV RENNES, Engineer, from Mar 2023 until Jul 2023]
Interns and Apprentices
- Muhammad Al Qabbani [UNIV RENNES, Intern, from Jul 2023]
- Wilem Lamdani [UNIV RENNES, Intern, until Jun 2023]
- Amina Mokdad [UNIV RENNES, Intern, from Mar 2023 until Jul 2023]
- Ayoub Skouta [UNIV RENNES, Intern, from Jul 2023 until Jul 2023]
Administrative Assistant
- Gwenaelle Lannec [INRIA]
Visiting Scientists
- Fatima Zahra Mardi [Moulay Ismail University, Morocco, until Mar 2023]
- Edison Moreno Cardenas [UPV - VALENCIA, until Feb 2023]
2 Overall objectives
Networks are omnipresent and increasingly complex, and require an efficient management of their operations. The ERMINE team designs and analyzes procedures and policies for efficiently managing network operations, but also works on the required measurement and monitoring of performance metrics. Our specific and original management activity focuses on network economics, regulation, and automated decision making. In terms of needed measurement, we make use of standard modeling and performance analysis techniques, but also carry out direct measurements to be analyzed statistically. A cross-cutting research axis for both management and measuring is artificial intelligence. Our activity is a trade-off between methodological/mathematical developments and practical implementations.
3 Research program
3.1 Context
Telecommunication networks are ubiquitous in our daily life. The network ecosystem and its infrastructure are increasingly complex with more and more involved actors and technologies which have to interoperate. This complexity is illustrated in Figure 1, where
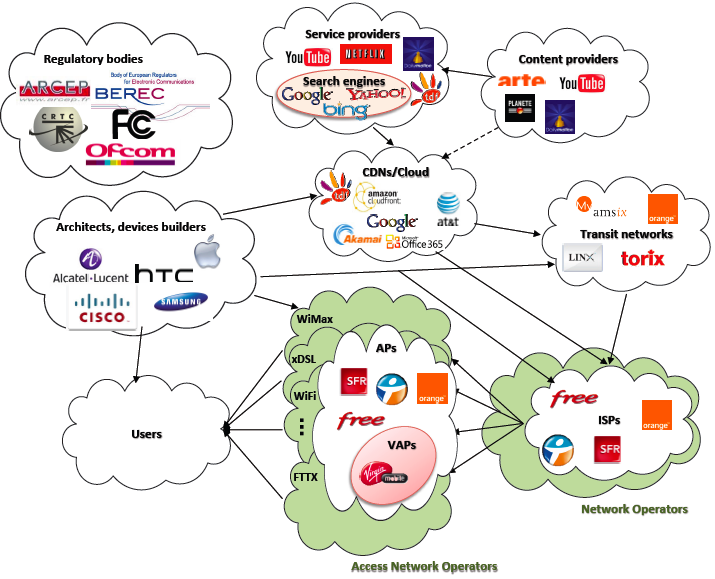
Description of the various actors on the ICT (for Information & Communications Technology) ecosystem
the basic network paradigm with network operators simply sending content from/to end users has moved to a topology with many players having different roles. A complete telecommunications system has to integrate traditional networks capabilities but also all other innovative Internet services into a single service platform. Selfishness of actors, heterogeneity of requests and technologies, interoperability of services and automation of decisions are major challenges to be tackled.
ERMINE deals with network operations management and the required associated monitoring and measurements and performance analysis. Operation network management means for us performance-based management, fault analysis, or the design of procedures and strategies for resource provisioning and quality-of-service fulfillment. On this management side, we aim at looking at the whole topic of networks economics, studying the best economic strategies of actors of the digital economy, their interactions and the potential need for regulation. We also want to address resource management through artificial intelligence for automated decision as another main research direction. On the measurement side, monitoring services of all sorts is a major challenge for regulatory bodies to ensure a fair and legal behavior of providers, and is also of primary importance for providers themselves to optimize decisions; this often requires new modeling, analysis and artificial intelligence tools. This is detailed below.
3.2 Managing network operations
The heterogeneity and complexity of the ICT (for Information & Communications Technology) world bring a number of challenges to network operations management. ERMINE addresses key issues for network management: i) the challenges of ICT economics; ii) network control and interoperability iii) automated decisions through AI.
ICT is omnipresent in our modern society, and the economy has gone beyond the industrial economy to the Internet and ICT economy. Thanks to hyper-connectivity, there are now lots of opportunities for innovation. As of May 2020, among the top-10 most valuable companies worldwide, seven are ICT companies, trusting the places from 2 to 8 (Microsoft, Apple Inc., Amazon Inc., Alphabet Inc., Facebook, Alibaba Group, Tencent). Internet Service Providers (ISPs), Content Delivery Networks (CDNs) and cloud providers, social network actors, all services and content providers are among actors needing a business model as profitable as possible. Designing and analyzing such business models and their acceptance by end users are issues to be addressed, leading to challenges in terms of outcome for all players (who act in most cases selfishly) and in terms of benefits for society. On that last point, regulators have to determine rules that actors need to follow in order to avoid harmful behaviors and maximize social welfare. The issues we address include: the design of economic rules for new services, the analysis of the impact of players decisions and interactions, and the potential design of rules or incentives from regulatory bodies leading to the most adequate (social) outcome. One of the frameworks to be used is that of game theory, and in particular of mechanism design.
There has been a metamorphosis in the last few years on the management of network operations, driven by the virtualization of networks and services. This evolution allows to meet the needs in terms of the dynamic scaling of infrastructures and the agility of the decision-making, namely, a necessary prerequisite for reducing operating costs as well as improving return on investment. These developments are radically changing the way services are managed, as they become more complex (i.e., services in the form of graphs, micro-services, etc.), their expectations can be very diverse and strategies for their placement could consider a single domain or spanning across several domains, whether cooperative or not. Thus, the operation of the network becomes extremely difficult and requires not only optimization of resources but also economic considerations, especially when management involves several domains. We believe that automated management and control is the key direction for an efficient solution. We deal with the automation of the network by contributing to ongoing standardization efforts, notably by the “Zero touch network & Service Management” (ZSM) group of the ETSI (the European Telecommunications Standards Institute), and through the elaboration of solutions based on the most recent advances in machine learning techniques, and in particular in deep reinforcement learning. Some of the challenges we tackle include dynamic placement of complex and constrained – QoS/QoE – services (i.e., network slicing), automatic service scaling, congestion control in the context of new generation networks, including IoT networks (e.g., massive Machine-Type Communications (mMTC)). Different aspects are taken into account when developing the various solutions, including reliability, resilience, guaranteed performance (i.e., deterministic networks), but also the energy efficiency on which the viability of the latter depends. Ensuring the performance of the solutions when using machine learning techniques is, however, a major problem that is poorly addressed in the literature. We address it by proposing techniques that offer at least similar performances to heuristics. Indeed, even if heuristics can be very efficient, they often have, however, some limitations. One of these is the blocking at the level of local minima. AI techniques can address this issue encountered in combinatorial problems (of the NP-hard type, such as the travelling salesman one). They sometimes yield near-optimal results. AI techniques have the additional advantage of being able to learn by interacting with the environment, and can therefore find solutions that heuristics could not.
3.3 Measuring
A proper management of network services and operations cannot be effective without measurement/monitoring and without the analysis of relevant metrics. Indeed, decision making cannot be realized without knowledge and data on the past, present and even future status of the activity. This is typically the case for agile capacity planning and resource sharing for which usage needs to be computed and even predicted. This is a challenge for which tools coming from AI are very promising. Another relevant example of challenge is measurements for regulation purposes, in relation with our activity on network economics: defining regulation rules means designing measurement procedures to verify that the rules are followed. It is for example required to monitor a neutral behavior of ISPs as expected from the Net neutrality principles. While there exist a few tools towards that goal, they are actually all devoted to very specific hindrances to neutrality (blocking, degradation) for specific types of flows or traffic; a major challenge is to detect any type of non-authorized behavior. Many other actors of the ICT economy, if not all, are barely monitored while an unfair behavior could be seen as harming end users and society. To name some noticeable examples, we can cite CDNs which cache some content at the edge of the network and could unfairly propose a better quality to selected customers, or search engines who can prioritize the web. In all those cases, different and specific techniques have to be designed to analyze and detect unexpected behaviors.
Similarly, managing resources and operations calls for evaluating (measuring) the impact of decisions to determine the most appropriate ones. Modeling and performance evaluation techniques are appropriate and useful solutions at a low cost, without the need to build and run the real system. While we have in the group a long experience on performance evaluation, new challenges keep popping up due to new types of services translated into new problems to be modeled and analyzed through performance evaluation tools, and adaptations or extensions of existing techniques for better decision making. They usually require to develop new appropriate network metrics to assess network service operations effectiveness. Illustrative examples are blockchains. The popularity of blockchain lies in the introduction of the concept of a public distributed ledger shared by all participants of the system without relying on a centralized authority. This distributed ledger records all the transactions between parties efficiently and in a verifiable and permanent way. In order to enjoy higher throughput and self-adaptivity to transactions demand in ICT, it requires the development of a new architecture and thus specific modeling and analysis techniques.
3.4 Research axes
Our activity is organized in five subtopics (or axes). The first two axes are on network management, and the next two are orthogonal on the associated and required measurements. Axis 5 on AI is transverse. The axes and their intersections are described in Figure 2.
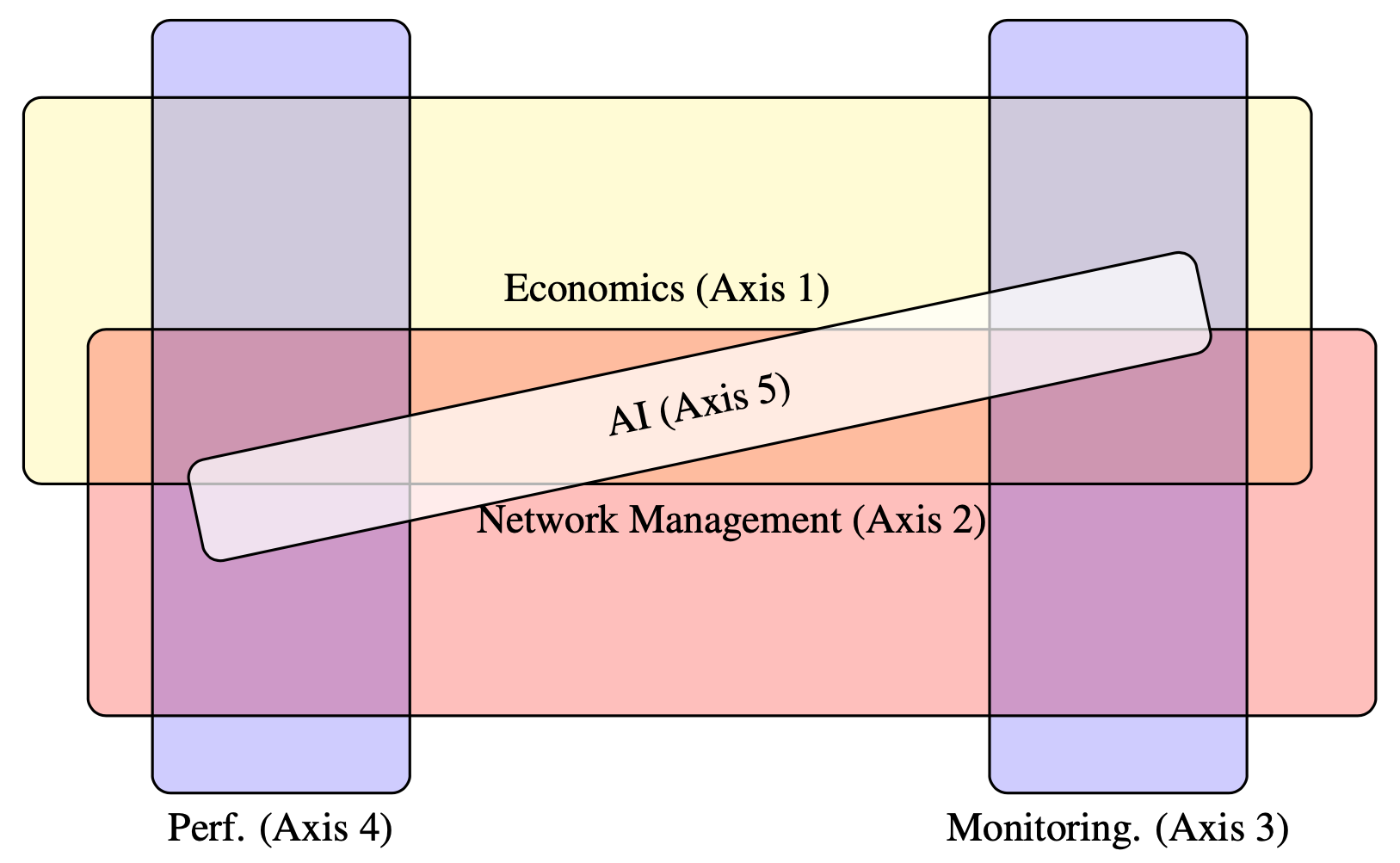
The five research axes: two on network management, two orthogonal on measurements and transverse on AI, with intersections
As a brief description:
- Network Economics. The digital economy has gained and keeps gaining in scale, scope, and significance. The ecosystem is quickly evolving and one of our main goals is to answer all questions related to the Internet & digital economy that pop up in line with what we started to do, and to be reactive to the news in that domain. We want to address issues concerning Internet resource allocation and pricing models, the economics of services, issues with vertical integration, the economics of structuring platforms, as well as economics and regulation (including network neutrality).
- Managing next generation networks & massive IoT communication. One of the fundamental challenges in the traffic management of new and emerging networking activities is to describe, analyze and control heterogeneous resources and complex services, under energy constraints. While AI is a main research direction to address this problem, there exist many other possibilities. The main questions of interest include the automation of infrastructure management (a typical example being network slicing) or the massive access in IoT networks.
- Measuring, monitoring & regulation. With the rapid evolution of networks, many needs are appearing for the design of measurement techniques associated with new services, but also for regulators to monitor network's activity. Measurements are of two possible categories: passive, monitoring existing traffic, or active, i.e., based on injecting traffic to investigate the network behavior. The goal of this research direction is to develop practical network operations measurement techniques, from the two different points of view: operators (for a better management) and regulators (for monitoring).
- Measuring performance metrics based on models. Measuring performances has to be done not only to observe and monitor directly a service like in previous subsection, but also at different phases such as at conception or to propose enhancements. Indeed, network services management and decision making cannot be applied efficiently without a valid and accurate evaluation of performance metrics through the construction and analysis of models. We are carrying out such evaluations.
- Artificial Intelligence (AI) in networking. AI is a transverse research axis, since it is used for both management and measuring. We are not only users of the technology but also interested in the methodology itself, that is, we also develop new techniques, based on our networking problems but usable outside. This concerns mainly supervised learning, in particular time series forecasting using this type of learning, and reinforcement learning. From the point of view of the tools, our skills and work are essentially related to Random Neural Networks, Reservoir Computing and deep neural architectures in Reinforcement Learning.
4 Application domains
Our global research effort concerns networking problems.
The need to support services requiring increasingly high throughput and extremely short latencies in 5G and Beyond 5G (B5G) networks is causing a major transformation of operators' infrastructure. The latter are increasingly being virtualized and expanded to be as close as possible to service consumers, in particular through the development of edge computing. Operators should increasingly collaborate to respond optimally to demands (i.e., cross-domain networks), and eventually converge their networks (i.e., networks' federation). The ultimate solution to this growing complexity is to move towards a fully automated network (i.e., zero-touch network). Our efforts will be dedicated, not on architectural improvements, but on the development of algorithms to manage these infrastructures in an automated way. The efficient and automatic placement of dynamic network services (i.e., network slicing), accommodating simultaneously a wide range of services, is certainly one of the most important concept to move towards zero-touch networks. Similarly, the limited radio resources, the random access to the medium and the spectrum sharing between different applications are critical issues in the IoT context. The focus is not only on 5G and beyond-5G networks, but also on unlicensed networks spectrum such as LoRaWan (for Long Range Wide Area Network; to enable IoT devices to communicate over long distances with minimal battery usage). In LoRaWan networks, the management challenges are even more important given their inherent characteristics, where access is not regulated at all, as it is the case in cellular networks like NB-IoT (for Narrowband Internet of Things).
Still on the management side, with the telecommunications ecosystem quickly evolving, one of our main goals is to answer all questions related to the Internet & digital economy that pop up in line with what we started to do, and to be reactive to the news in that domain to help economic actors and society. Typical and illustrative current questions requiring reactivity are: Is USA repealing neutrality good for some actors in the world, what reactions would be beneficial? Is free roaming in Europe bad for network providers? Is the 2019 Orange-TF1 argument part of the neutrality debate? Should search engines be regulated? Etc. Our goal is mainly to help regulators define proper rules, but we also aim at helping economic actors in decision making (economic choices of CDNs, search engines, etc.) and study their interactions.
On the measurement side, when developing practical network operations measurement techniques, we are interested in two different points of view: operators (for a better management) and regulators (for monitoring). Many of the techniques developed at Ermine are related to the analysis of complex systems in general, not only in telecommunications. For instance, our Monte Carlo methods for analyzing rare events have been used by different industrial partners, some of them in networking but recently also by companies building transportation systems.
5 Social and environmental responsibility
5.1 Impact of research results
Our research on network economics and particularly neutrality issues aims to view how, and if, regulation can help to improve social welfare, among other goals. Typical examples are how tools SNIDE and WeheDemo described later explaining how potential deviations from rules by respectively search engines and Internet service providers can be detected. Our modeling works on neutrality also allow to investigate the appropriate rules toward a benefice to society.
We have also started to work on so-called green streaming to analyze the interactions and strategies of various vendors in the market and provide valuable insights that can contribute to the sustainable growth of the Content Delivery Network (CDN) industry. By understanding the implications of energy pricing and its impact on the market dynamics, this research endeavors to offer potential solutions and recommendations to address the challenges and foster a more sustainable and efficient CDN ecosystem.
6 Highlights of the year
6.1 Awards
- Paper 22 won the best paper award at the 31st International Symposium on the Modeling, Analysis, and Simulation of Computer and Telecommunication Systems (MASCOTS 2023).
- Paper 14 won the best paper award at the 19th International Conference on Multimedia Information Technology and Applications, at Ostrava, Czech Republic (MITA 2023).
7 New software, platforms, open data
7.1 New software
7.1.1 SNIDE
-
Name:
Search Non neutratlIty DEtection
-
Keywords:
Search Engine, Statistic analysis
-
Scientific Description:
The goal of this tool is, for a search, to collect the ordered lists from the main search engines, to compare them, to perform statistical tests to point out potential outliers, and to propose two meta search engines reducing biases.
-
Functional Description:
Different search engines provide different outputs for the same keyword. This may be due to different definitions of relevance, to different ranking aggregation methods, and/or to different knowledge/anticipation of users’ preferences, but rankings are also suspected to be biased towards own content, which may be prejudicial to other content providers.
-
Contact:
Bruno Tuffin
-
Participants:
Patrick Maillé, Bruno Tuffin, Guillermo Andrade Barroso
-
Partner:
IMT Atlantique
7.1.2 DemoWehe
-
Name:
Demontrator of Wehe Net Non-Neutrality Detection Tool on Video Streaming
-
Keywords:
Network monitoring, Network neutrality
-
Functional Description:
DemoWehe creates a topology made of three virtual machines. One is acting as the Wehe server and one is acting as the client device (or end user). Communications between client and server are done thanks to the third virtual machine representing the network on which service can be differentiated. The same video is played and displayed simultaneously non-differentiated and with the chosen performance degradation. Wehe test is applied and the user can visually evaluate the difference between degradation and possible detection.
- URL:
-
Contact:
Bruno Tuffin
-
Participants:
Patrick Maillé, Bruno Tuffin, Antoine Lesieur
—————————–
8 New results
8.1 Network Economics
Participants: Patrick Maillé, Bruno Tuffin, Maël Le Treust, Yassine Hadjadj-Aoul.
Economics and regulation: network neutrality. The non-negligible part of our activity on network economics was focusing in 2023 on network neutrality and its consequences.
Paper 29 designs a model simultaneously encompassing several scenarios regarding the content-providing market structure: a monopolistic content provider, competitive ones, and a vertically-integrated one facing a non vertically-integrated competitor. We each time compare the outputs when non-neutral, weak neutral or strong neutral policies are applied, in order to get insights regarding the possible consequences of each type of neutrality regulation.
We also have dealt with network slicing is a key component of 5G-and beyond networks, requiring to define a business model for resource allocation. In 22, we consider a model with a Service Provider (SP) that may purchase a slice in a wireless network, in order to offer a "premium" service where the improved quality stems from higher prices leading to less demand and less congestion than the basic service offered by the network owner, a scheme known as Paris Metro Pricing. One optimization problem for the SP is the choice of how much resource to allocate to that slice. We also compare with the case of a unique “pipe" (no premium service) and with the case of vertical integration to evaluate the impact of slicing on all actors and identify the “best" economic scenario.
Paper 27 highlights the current trends and the necessary research directions for achieving carbon-effective streaming services. Cooperation between the vendors of the end-to-end delivery pipeline is encouraged to design standards and technologies. We discuss there the economic and regulation implications.
Search neutrality. Search engines play a significant role in shaping the Internet, but there are concerns about the motivation behind their rankings: some content and service providers have complained about being ranked abusively low to favor engines' commercially-close content, initiating the so-called search neutrality debate, in line with the network neutrality debate described above. There are similar worries about possible orientations towards some ideologies or political points of view. Whatever the opinion on this sensitive issue of the definition and need for search engine neutrality, it is important to have at our disposal tools to monitor search engines behavior and understand deviations from “expected” results. The goal of 45 is to review existing statistical tests of potential bias by search engines, compare and combine them both formally and numerically. We end up with a battery of ANOVA and Dixon Q tests for which we characterize the bias detection probability and false positive probability, the latter requiring to be minimal if tests have to be used officially and publicly. We also run the tests on a campaign of searches on real-life search engines and discuss the outcome.
Information and decision making. Information is the cornerstone of most decision-making problems. Information asymmetries in game theory raise new scenarios and new open questions, also in information theory. In particular, information reliability is crucial in decision-making problems, as well as for video coding problems, where the decoding error of internal processing must be exactly zero. In 37, 17 and 18, we develop graph theoretical tools in order to investigate the zero-error source coding problem with side information at the decoder. We characterize the solution of a class of problems of this long-standing open question in information theory and in graph theory.
8.2 Managing next generation networks & massive IoT communication
Participants: Soraya Ait Chellouche, Yassine Hadjadj-Aoul, Patrick Maillé, Fatima Zahra Mardi.
Optimization of Massive-IoT emission strategies. The proliferation of Internet of Things (IoT) devices is constantly increasing, reaching a point where the imminent risk of saturation requires a major optimisation effort. Low-power Wide Area Networks (LPWANs), in particular LoRaWan networks, are playing a key role in this domain, facilitating the deployment of large numbers of wireless devices that rely on battery power to monitor the surroundings and transmit data to gateways. In such a context, a critical trade-off for transmission performance (packet delivery ratio) versus energy conservation (and hence, the device lifespan) appears when deciding the transmission parameters, in particular, the Spreading Factor (SF) to be used by each node. In 34, we use lightweight reinforcement learning techniques, namely multi-armed bandits, for each node to select an appropriate SF, based on preferences regarding that trade-off. Unlike previous works on that topic, we relax some assumptions to aim at a realistic implementation: our solution does not assume immediate rewards, or that each device communicates with only one gateway. Additionally, we build explicit MAC commands for the method to work in practice and implement it in the ns-3 simulator using a state-of-the-art LoRaWAN module. Simulations show that when energy conservation is critical for IoT nodes, such lightweight learning algorithms outperform LoRaWAN's legacy Adaptive Data Rate algorithm, both in single- and multi-gateway scenarios.
Another challenge in massive IoT is for a large number of nodes to discover which transmission parameters to use and share that information efficiently among them to avoid excessive channel probing. In 4, we propose an approach to optimize the performance of IoT networks by making the IoT devices intelligent using machine learning techniques. We formulate the optimization problem as a massive multi-player multi-armed bandit and introduce two novel policies, one focused on the number of successful transmissions and one including fairness guarantees. We then present an efficient way to manage the trade-off between transmission energy consumption and packet losses in Long-Range (LoRa) networks, by which LoRa nodes adjust their emission parameters (Spreading Factor and transmitting power). We implement our algorithms on a LoRa network simulator and show that such learning techniques largely outperform the Adaptive Data Rate (ADR) algorithm currently implemented in LoRa devices, in terms of both energy consumption and packet losses.
Due to the increase in the number of IoT devices in recent years, managing and supporting the diversity of services is becoming more difficult. Network Slicing will be the solution, in which the network slices are tailored to the requirements of the services. In 31, network slicing is investigated in LoRaWAN networks using the Heuristic-Deep Q-Network (H-DQN) solution that manages the network resource allocation. We propose an intra-service allocation based on the deep Q-Network (DQN) algorithm by allocating virtual resource blocks to services. In addition, the intra-service allocation is based on a heuristic algorithm that assigns the transmission probability to the LoRa nodes of each service for each block in a way to maximizes the Packet Delivery Rate (PDR) of the network while ensuring that the priority of services is maintained.
Setting up IoT monitoring solutions requires a significant workforce for deployment and maintenance. This becomes particularly costly when a massive number of sensors are deployed. In 47, 38, we propose a generic solution based on energy-autonomous LPWAN sensors deployed on the fly. On one side, we propose an efficient management strategy that adapts the transmission period of sensors in order to receive a chosen amount of information on each sensor cluster that emits similar information. Moreover, we develop a method that generates realtime estimates based on the sensor message history.
Service management in the cloud-edge continuum. In the context of network slicing, the efficient placement of network services represents one of the most important and complex combinatorial task. In 36, we model the problem using an Integer Linear Program (ILP), and resolve it to obtain the global optimum (which can only be achieved in an offline scenario). Then, we propose a Column Generation (CG) formulation to deal with large instances of the problem. and we propose an efficient approach based on Branch-and-Bound (BnB), applying AI search strategies to address the problem of network service placement. Extensive evaluations confirm significant improvements when we consider a fair distribution of the resources on the edge. In 7 we extend the previous work in several aspects. Indeed, we realized that, along with obtaining optimal results to maximize service acceptance, network fragmentation is a critical issue hardly addressed in the literature. Trying to mitigate this problem, we modify our approach with the fair placement of network load to avoid fragmentation. The experiments not only confirm the effectiveness of the used tweaks on the network defragmentation but also show a significant improvement in terms of service acceptance. In addition, we notice that the completeness of our node-based BnB formulation was at stake. Accordingly, we propose a mixture of node-based and link-based formulations as a solution. From the early stages, we compare our approach with the offline optimal placement to see to what extent our approach was close to the general optimal solution. Consequently, we define the mathematical model of our problem as an ILP.
Traditional approaches of network slicing often adopt static network topologies, in which services resources consumption are not evolving, an approach increasingly inadequate in today's rapidly changing network environments 5, 19. The shortcomings of static methods become evident in the face of link failures, network congestion, topology modifications, and other unforeseen changes, emphasizing the necessity for a more robust network capable of service migration to adapt to dynamic networks. Service migration serves as a critical technique for reconfiguring and relocating network functions, thereby ensuring the resilience and availability of the slices. In 21, we propose a service migration strategy specifically designed for resilient multi-orchestrator multi-domain networks. Our approach considers multi-domain services composed of components deployed across different network domains using Kubernetes clusters. The abstract nature of this migration strategy allows for its adaptation to different orchestrators within a cluster, facilitating the integration of more diverse and heterogeneous systems.
8.3 Measuring, monitoring & regulation
Participants: Yassine Hadjadj-Aoul, Sofiene Jelassi, Antoine Lesieur, Patrick Maillé, Gerardo Rubino, Bruno Tuffin, Soumeya Kaada, Zahraa El Attar.
Our activity on monitoring has focused in 2023 on specific contexts but notice that we still work on network neutrality monitoring, starting to develop a demonstrator of the efficiency of existing tools which will help to suggest improvements.
Search neutrality monitoring.
With network neutrality regulation imposed worldwide at different extents, there is a need for monitoring tools to verify if Internet service providers (among other actors) comply with the existing rules. Among the very few maintained tools, Wehe has been developed by Northeastern University and has been publicized by the French regulator, ARCEP. The tool runs traces and produces a positive or negative response about service differentiation, but users have to trust that result without any visual perception of the degradation. In 44 we describe our demonstrator of Wehe for video streaming (DemoWehe), from which we can simultaneously see a baseline video and its manually-differentiated counterpart, as well as run Wehe to evaluate its response with respect to the perceived quality degradation. The demonstrator allows to study the efficiency of Wehe in order to propose potential improvements, if needed.
Slicing. In the context of network monitoring, the task of supervising network slices performance is becoming increasingly difficult. This complexity stems from the significant network overload associated with direct measurements. To overcome this problem, we suggest in 20 using network tomography to estimate slices' delays in the network. In particular, we investigate the problem of finding the minimal combination of end-to-end simple paths monitoring needed to minimize the estimation error of the slices' delays in a network. We propose a new genetic algorithm to identify the optimal monitoring paths required to achieve network tomography and minimize their number. To improve the search for the optimal solution, we investigate both a fixed mutation approach and our proposed adaptive mutation approach. Our evaluations show the effectiveness of both approaches; however, the adaptive mutation method outperforms the fixed method by exploring new solutions and avoiding local minima, leading to faster convergence and better results.
Resilience in 5G Radio Access networks
Resilience is defined as the ability of a network to resist, adapt to and quickly bounce back from disruptions, to continue keeping an acceptable level of service from users’ perspective. To characterize and analyze the resilience of a 5G Radio Access Network, it is vital to be able to measure current and prospective resiliency levels using relevant metrics. In 24, we perform an analysis and a quantification of 5G-RAN resilience based on radio coverage indicator and one of the Quality of Service indicators, namely the service integrity. They are considered as the main performance indicators for network planners and operators. We model network states using the performance indicators, namely Reference Signal Received Power and Received User Equipment Throughput. Then, we use them to build Markovian models and to define resilience metrics. By means of simulations, we evaluate the ability of our analytical model to accurately capture network resiliency levels.
In sensing-enabled mobile infrastructure, the network itself acts as a whole sensor by leveraging radio data or signals collected within Base Stations (BSs). This data is exploited for the development of data-driven machine learning solutions to augment network’s capabilities. Nevertheless, large-scale qualitative data is required for achieving high accuracy learning. However, their training phase leads to prohibitive cost and heavy constraints on data collection and storage that are not desirable for network. To overcome this problem, we propose to use synthetic data instead of real data for training machine learning models to avoid high cost data sharing/storage. In 25, we are interested in real-life Environment Sensing Network in a context of limited data amount sharing. We focus on Indoor-Outdoor Detection (IOD) using unsupervised machine learning classification models. For this purpose, experiments are conducted following the paradigm of Training on Synthetic data and Testing on Real Data (TSTR). We conduct a comparative study of four well-known generative models, that are able to generate synthetic 3GPP radio data with similar distribution than the source data. We investigate the quality of these synthetic generated radio data according to three dimensions: distribution similarity, data variability and detection capability. The classification models trained with synthetic generated data are tested in real-life context to infer whether a user connected to the network is inside or outside a building. The study shows convincing results with an Indoor/Outdoor unsupervised classification performance up to 80% of F1 score as in real-life data training scenarios.
8.4 Measuring performance metrics based on models
Participants: Bruno Tuffin, Gerardo Rubino, Raymond Marie, Sofiene Jelassi, Bruno Sericola.
Our work on performance analysis in 2023 can be decomposed in simulation, with application to resilience, transient analysis, with application to protocols, and distributed systems.
Monte Carlo simulation. We have continued our work on rare event simulation. In 33, when estimating a function of means, where not necessarily all of them correspond to rare events, we provide conditions under which having efficient estimators of each individual mean leads to an efficient estimator of the function of the means. We illustrate this setting through several examples, and numerical results complement the theory. We also give bounds in 39 on the approximation error of geometric sums by an exponential random variable when the number of summands is large, simplifying estimations in many rare contexts. It is typically the case when estimating the distribution of the waiting time in an M/G/1 queue or the hitting time to a rare set for a regenerative process. One of the main advantages of the approximation is to reduce the estimation of the whole distribution to the estimation of its mean, for which efficient (rare event) simulation are usually available in the literature.
We continued to work on flow reliability problems, where the goal is to evaluate the probability that a given transportation network is able to transport at least a specific amount of flow, under possible failures of its components. We describe in 2 a methodology that can efficiently estimate this reliability metric when components’ failures are not necessarily independent. In that paper we propose a Splitting–based Monte Carlo method that uses the Marshall–Olkin copula for the case of non independent components. This proposal operates on the so-called Destruction Process because the Marshall–Olkin copula model over networks is quite related - and somehow similar - to that scheme, which is unusual but here necessary.
In 12, we consider static models in network reliability, that cover a huge family of applications. The analysis of these models is in general #P-complete, and Monte Carlo remains the only effective approach. We underline the interest in moving from the typical binary world where components and systems are either up or down, to a multi-variate one, where the up state is decomposed into several performance levels. The chapter focuses on the resilience, which is the expected number of pairs of nodes that are connected by at least one path in the model. We estimate this metric, together with variations of it. We also discuss another side effect of the sampling technique proposed in the text, the possibility of easily computing the sensitivities of these metrics with respect to the individual reliabilities of the components. We show that this can be done without a significant overhead of the procedure that estimates the resilience metric alone. A preliminary version of this work was presented in 13.
Another domain in simulation concerns Randomized Quasi-Monte Carlo (RQMC) methods. RQMC methods provide unbiased estimators whose variance often converges at a faster rate than standard Monte Carlo as a function of the sample size. However, computing valid confidence intervals is challenging because the observations from a single randomization are dependent and the central limit theorem does not ordinarily apply. The natural solution is to replicate the RQMC process independently a small number of times to estimate the variance and use a standard confidence interval based on the normal or Student distribution. In 30 we investigate bootstrap methods for getting nonparametic confidence intervals for the mean using a modest number of replicates. Our main conclusion is that intervals based on the Student t distribution are more reliable than even the bootstrap t method on the integration problems arising from RQMC. In our examples, the individual RQMC estimates often had extreme kurtosis but always had mild skewness. That situation is very favorable for the standard confidence intervals.
Transient analysis. Network protocols sometimes make use of random walks (i.e., token circulation) on networks, to explore networks to collect information from them and to make systems reliable. Important performance measures of these protocols such as the search time and the time to visit all sites obviously depend on some quality measures of random walks such as the hitting and the cover times. The cover time of a discrete-time homogenous Markov chain or of a random walk the time needed to visit all the states of the process. We analyze in 6 both the distribution and the moments of the cover time and we are interested in exact results instead of asymptotic values of the mean cover time which are generally considered in the literature. These results are applied to particular graphs namely the generalized cycle graph, the complete graph and the generalized path graph.
We analyze in detail in 43 the moments and the distribution of the hitting and cover times of a random walk in the specific case of a complete graph. We study both the time needed to reach any subset of states and the time needed to visit all the states of a subset at least once. We obtain recurrence relations for the moments of all orders and we use these relations to analyze the asymptotic behavior of the hitting and cover times distributions when the number of states tends to infinity. We perform a similar analysis in 48 on the lollipop graph which is the graph exhibiting the maximum expected hitting time among all the graphs having the same number of nodes.
In 9, we describe our last results on two transformations of an arbitrary square matrix into two new matrices, its power-dual pd() and its exponential-dual ed(). Their interest is that the powers of (resp. the matrix exp() – or the function exp() with scalar) can be obtained by evaluating the powers of its power-dual (resp. the exponential of its exponential-dual). We describe the main properties of these transforms, and provide some examples of their applications.
In 8 we define and develop an eigenvalue characterization of when for a stochastic matrix converges to a steady state distribution , as converges to . This result generalizes previously known linear algebraic properties about stochastic matrices to somewhat stochastic matrices. As an application, an alternative method to calculate infinite-time gambler’s ruin on a finite state space using algebraic duality is described. When the eigenvalues of certain classes of matrices are known, then the or less steps gambler’s ruin probabilities can often be determined in terms of these eigenvalues using Siegmund duality.
Uniformization (also called Randomization, or Jensen’s method) is one of the most used techniques to compute the transient distribution of a Continuous Time Markov Chain. In 42 we present a new variant of this method, which we call Mass Controlled Uniformization (MCU, for short). A central characteristic of MCU is that in many usually encountered situations it allows computing the transient distribution faster (sometimes orders of magnitude faster) than the commonly used Uniformization versions. This performance gain is mainly due to the selection of “the most relevant” state probabilities at each iteration of the algorithm, ignoring the very small ones, where the total accumulated error introduced by our selection approach is specified beforehand and can be as low as desired. MCU allows analyzing large Markovian models (say, with millions of states) in an efficient way. In some cases, however, MCU can perform slower than other current versions of Uniformization. In our work we identify these situations and propose a second approach, called Hybrid MCU (HMCU) that, in each iteration, adaptively behaves as MCU or as a selected standard Uniformization procedure.
Markov chains. Duality ideas connect irreducible Markov chains with absorbing ones, but there are other ways of building relations between these two classes of processes. In 11 and 10 we discuss two other connections between these models when time goes to infinity. In the first one, the focus of 11, we make a correspondence between a specific irreducible model associated with a given absorbing one, allowing to derive results for which we will show applications. One of them leads to the second type of connection, the focus of 10, where we show relations in absorbing chains corresponding to balance relations of the probabilistic flow across cuts in related irreducible stationary models. We show how using these relations leads to simplifications in computations on absorbing models.
Another study concerned certain systems that can be seen as proactive, anticipating future service demands. In 46, we present some basic pro-active systems from the perspective of the performance criteria used for classical queues. The resources may anticipate services up to a given limit. This limit is a key factor with respect to the efficiency of the system under study. An application of these results, in which these proactive queues model the stocks of exchangeable spares on the operational site of a fleet of complex systems, was the subject of a publication at ICIL'2023 32.
Measuring the performance of distributed systems. Randomized rumor spreading or gossiping is an important communication mechanism that allows the dissemination of information in large-scale and open networks. A large-scale and open network comprises a collection of sequential computing entities (e.g., processes, processors, nodes, agents, peers) that join and leave the system at any time, and communicate with one another by exchanging messages. In contrast to reliable communication broadcasts which must provide agreement on the broadcast value with possibly additional ordering guarantees on the delivery of updates from different sources, a randomized rumor spreading procedure provides reliability only with some probability. A randomized spreading rumor protocol describes the rules required for one or more pieces of information known by an arbitrary node in the network to be spread to all the nodes of the network. We analyze in 3 a new asynchronous rumor spreading protocol to deliver a rumor to all the nodes of a large-scale distributed network. This protocol relies on successive pull operations involving different nodes, with , and called k-pull operations. Specifically during a -pull operation, an uninformed node a contacts other nodes at random in the network, and if at least one of them knows the rumor, then node a learns it. We perform a detailed study in continuous-time of the total time needed for all the nodes to learn the rumor. These results extend those obtained in a previous paper which dealt with the discrete-time case. We obtain the mean value, the variance and the distribution of together with their asymptotic behavior when the number of nodes n tends to infinity.
In 28 we present and study the performance of the -pull operation in the presence of a certain fraction of non-cooperative nodes. The goal is to understand the impact of on the propagation of the rumor despite the presence of a fraction of non-collaborative nodes.
8.5 Artificial Intelligence (AI) in networking
Participants: Yassine Hadjadj-Aoul, Gerardo Rubino, César Viho.
On Time Series Forecasting using Machine Learning. From one side, Evolutionary Algorithms have enabled enormous progress over the last years in the optimization field. They have been applied to a variety of problems, including optimization of Neural Networks’ architectures. On the other side, the Echo State Network (ESN) model has become increasingly popular in time series prediction, for instance when modeling chaotic sequences. The network has numerous hidden neurons forming a recurrent topology, so-called reservoir, which is fixed during the learning process. Initial reservoir design has mostly been made by human experts; as a consequence, it is prone to errors and bias, and it is a time-consuming task. In 1, we introduce an automatic general neuroevolutionary framework for ESNs, on which we develop a computational tool for evolving reservoirs, called EVOlutionary Echo State Network (EvoESN). To increase efficiency, we represent the large matrix of reservoir weights in the Fourier space, where we perform the evolutionary search strategy. After updating the Fourier coefficients, we go back to the weight space and perform a conventional training phase for full setting the reservoir architecture. We analyze the evolutionary search employing genetic algorithms and particle swarm optimization, obtaining promising results with the latter over three well-known chaotic time series. The proposed framework leads fast to very good results compared with modern ESN models. Hence, this contribution positions an important family of recurrent systems in the promising neuroevolutionary domain.
Forecasting the temperature at a given place in a given future epoch is of evident huge interest. In 40, we focus on the problem of forecasting if a given future period (say, next summer) will be hotter than usually. For this task, most of the production tools are of the dynamic type (that is, physical, usually huge systems of partial differential equations) or statistical, or a combination of both. We explore the use of modern Machine Learning tools and show that they can compete with those dedicated and always heavy and complex procedures, in spite of the fact that our results come from standard tools and hardware. We illustrate the results with data coming from a project where these questions are studied, concerning a large area in the Southern part of South America. In 41, we consider the time series of the daily maximal temperatures collected at many meteorological stations in a wide area, and we try to forecast the maximal temperatures in the next few days at all those points. These are pretty irregular series, and we chose a particularly powerful algorithm belonging to the GNN class called Graph WaveNet for this task. The algorithm hasn't been applied before to this type of target. We explor its behavior with no need for any feature engineering, and we also compared it with that of XGBoost tool for which we should invest a significant effort to exploit the spatial aspects of data. We considered the forecasting up to 10 days, the meteorological range. Basically, Graph WaveNet behaves better for very short prediction horizons and becomes less accurate when the prediction horizon increases. XGBoost stays closer to the mean of the series when the horizon gets large (close to 10 days).
Network Traffic Classification for Multi-Activity Situations Detection. Network traffic classification is an active research field that acts as an enabler for various applications in network management and cybersecurity. Numerous studies from this field have targeted the case of classifying network traffic into a set of single-activities (e.g., chatting, streaming). However, the proliferation of internet services and devices has led to the emergence of new consuming patterns such as multi-tasking that consists in performing several activities simultaneously. Recognizing the occurrence of such multi-activity situations may help service providers to design quality of service (QoS) solutions that fit users' requirements in a better way. In 15, we propose a framework that is able to recognize multi-activity situations based on network traces. The aim of this framework is twofold: (i) generating synthetic multi-activity network traces from single-activity network traces, (ii) preprocessing and then affecting a multi-activity network trace to the corresponding class. To recognize the occurrence of multi-activity situations, we conceive a classifier that pre-processes a multi-activity network trace and then affects it into a predicted multi-activity class. Additionally, as we base our classifier on machine learning techniques, we conduct a comparison between the performance of some recent models (e.g., LSTM with attention mechanisms, Transformer). Our experiments show that our solution is able to achieve promising results despite the complexity of the task that we target.
Federated Representation Learning for Indoor-Outdoor Detection in Future Mobile Networks. Scarcity of labelled datasets makes it challenging to train robust mobile user environment detection models. Labelling and centralizing large data amounts for training is expensive. To address these issues, semi-supervised learning techniques aim to reduce data labelling, while Federated Learning (FL) avoids centralizing the data. In 23 , we propose a novel approach for Indoor/Outdoor detection by combining the strengths of federated and semi-supervised learning. It consists of 2 steps: (1) Unsupervised Federated representation Learning to learn representations using large unlabelled data. We leverage unlabelled data from diverse sources situated across various geographical locations. Through FL, we develop high-quality representations by jointly learning from this distributed unlabelled data. (2) We capitalize on the acquired representations and further employ transfer learning to achieve accurate detection using a reduced amount of labelled data. We also add an optimization module referred as User Behavioural Optimizer that corrects environment detection errors by tracking behavioural anomalies. We obtain an F1-score of 95.06% using only 30% of the entire amount of labelled data available.
ML-based Network Slicing. The advent of virtualization, combined with the power of AI, has brought new opportunities in network management. To effectively address the challenges that come with this paradigm shift, we continue our activities in exploring the transformative potential of ML methods, and in particular AI, in enabling smart network management.
To deal with capacity-limited resources while insuring an extreme availability of services, several approaches have been proposed in the literature, some of them converge quickly to a local minimum, while others are not explainable and therefore do not provide the necessary guarantees for their deployment in a real network. In this context, we propose, in 35, a new approach for Virtual Network Embedding (VNE) based on the Greedy Adaptive Search Procedure (GRASP). Using the GRASP meta-heuristic ensures the robustness of the solution to changing constraints and environments. Moreover, the proposed realistic approach allows a more efficient and directed exploration of the solution space, in opposition to existing techniques. The simulation results show the potential of the proposed method for solving services' placement problems and its superiority over existing approaches.
To ensure low latency, service providers are increasingly turning to edge computing, pushing services and resources from the Cloud to the Edge of the network, as close as possible to users. However, since video and image processing applications are particularly computationally intensive, their deployment is typically based on distributed provisioning between the Edge and the Cloud, which can increase the risk of failure when relying on unreliable networks. In 26, we propose the algorithm RAP-G (Reliability-Aware service Placement with Genetics), which considers the reliability of network links and distributes services between the Cloud and the Edge using a genetic algorithm (GA). We also develop a new variant of the first-fit algorithm called RF2 (Reliability-Aware First-Fit) that considers reliability within a reasonable time. The experimental results show the importance of considering reliability in service delivery and the superiority of RAP-G. In 16, we propose utilizing the Algorithm Selection (AS) paradigm. This selects the most optimal Deep Reinforcement Learning (DRL) algorithm from a portfolio of agents, in an offline manner, based on past performance. To evaluate our solution, we develop a simulation platform using the OMNeT++ framework, with an orchestration module containerized using Docker. The proposed solution shows good performance and outperforms standalone algorithms.
Using Self Organizing Maps to model cognitive deseases. In 1982 Teuvo Kohonen proposed an algorithm called Self Organizing Map (SOM) that is basically a stochastic model of the establishment of topology preserving connections between the retina and the cortex in our brain. That model has proved an efficient classification tool, a source of inspiration for other biological models and also for the understanding of the principles of self-organization. In 14, we present a modification of the initial SOM to address an important problem in semantic cognition: the representation of human concepts in the brain. The paper discusses the model and describes some preliminary results that show its interest as the basis of possible new tools to help to understand the considered type of diseases.
9 Bilateral contracts and grants with industry
9.1 Bilateral contracts with industry
9.1.1 Common Lab INRIA - Nokia
Participants: Yassine Hadjadh-Aoul, Gerardo Rubino.
-
Title:
SmartNet “Analytics and machine learning”
-
Partner Institution(s):
- Nokia Bell Labs
- Inria
-
Date/Duration:
2019-2023
-
Summary:
The objective was to carry out common research on an integrated framework for 5G, programmable networks, IoT and clouds that aims at statically and dynamically managing and optimizing the 5G infrastructure using, in particular, Machine Learning techniques. The project involves several teams in INRIA and the work of several PhD students. It ended its life-time in 2023. At Ermine, Yassine Hadjadj-Aoul is an active member of the action.
9.1.2 ANR Intelligentsia
Participants: Soraya Ait Chellouche, Ghina Dandachi, Yassine Hadjadj-Aoul, Patrick Maillé, Gerardo Rubino.
-
Title:
INTelligent Edge using Learning Loops & Information GEneration for NeTwork State Inferencebased Automation.
-
Partner Institution(s):
- Orange Labs
- Inria Rennes
- CNAM
- Acklio
- Aguila
-
Date/Duration:
Nov. 2020-Nov. 2024 (4 years)
-
Summary:
The Intelligentsia project aims at (1) specifying novel media access protocols and resources sharing policies to be able to support network slicing for IoT access networks in general, and LoRa networks in particular; (2) designing of a network automation framework that incorporates novel learning algorithms to configure the IoT access network.
9.1.3 ANR EDEN4SG
Participants: Patrick Maillé.
-
Title:
Efficient and Dynamic ENergy Management for Large-Scale Smart Grids
-
Partner Institution(s):
- Orange Labs
- Université Toulouse 3 - Paul Sabatier
- CNRS
- IRISA
- EDF
- SRD
-
Date/Duration:
January 2023-January 2027 (4 years)
-
Summary:
The wide-scale deployment of electrical vehicles (EVs) represents a challenge as well as an opportunity to render more efficient and affordable the transformation of the current power system into a smarter grid. The goal of the project is to develop more efficient, decentralised, and dynamic energy management methods able to ensure coordination of large-scale fleets of EVs with conflicting objectives/constraints. In addition, the energy management of power systems closer-and-closer to real-time will require the intensive use of pervasive information and communication technologies (ICT). These technologies may suffer from an imperfect quality of service (QoS) (e.g. delays) which may greatly decrease the performance of smart grids.
9.1.4 BPI 5G JO
Participants: Yassine Hadjadj-Aoul, Gerardo Rubino, Kaouther Boulaouinat.
-
Title:
5G JO
-
Partner Institution(s):
- Orange
- Inria Rennes
- CEA Saclay
- Ericsson
-
Date/Duration:
Nov. 2020-Nov. 2023 (3 years)
-
Summary:
The objective of the project is to develop a platform for the placement of constrained services on the fly, with environmental consideration.
9.1.5 AAP PME NaviDEC
Participants: Guillaume Le Gall, Abdellah Kaci, Soraya Ait Chellouche, Yassine Hadjadj-Aoul.
- Title:
-
Partner Institution(s):
- Sodira-Connect
- Université de Rennes
- Inpixal
-
Date/Duration:
Sep. 2021 - Apr. 2024
-
Summary:
The NaviDEC project aims to research, develop, test, and validate an “IoT and Image Processing” platform that brings processing capabilities to the micro-edge of the network. This platform will enable easy deployment of intelligent services, even in the context of intermittent connectivity.
9.1.6 Common lab IMT Atlantique - b<>com with AI@IMT project
Participants: Patrick Maillé.
The objective is to carry out common research on the usage of AI in Cybersecurity for Networking context.
9.2 Bilateral Grants with Industry
9.2.1 Cifre on Resiliency as a Service for 5G, with Nokia
Participants: Sofiene Jelassi, Gerardo Rubino.
This is a Cifre contract 2020-2023 including a PhD thesis supervision (PhD of Soumaya Kaada), done with Nokia (Paris-Saclay), about Resiliency as a Service for 5G networks using Machine Learning. It concerns providing on demand and evolving resiliency schemes over 5G network using advanced Machine Learning algorithms. It relies on a highly flexible network infrastructure supporting both wired and wireless programmable data planes through a highly-efficient distributed network operating system.
9.2.2 Cifre on availability-aware NFVs placements, with Exfo
Participants: Yassine Hadjadh-Aoul, Gerardo Rubino.
This is a Cifre contract 2020–2023 including a PhD thesis supervision (PhD of Amine Rguez), done with Exfo (Rennes), about availability-aware NFVs placements of network services, using Deep Reinforcement Learning. The objective of the thesis is to exploit the potential of Deep Learning, and more particularly, of Deep Reinforcement Learning, in order to guarantee the high availability of placed network services.
9.2.3 Cifre on Automation beyond 5G, with Nokia Bell Lab
Participants: César Viho.
This is a Cifre contract 2020–2023 including a PhD thesis supervision (PhD of Sid Ali Hamideche), done with Nokia Bell Labs (Nozay) and IRISA-Université Rennes. We are searching for solutions to improve the decision process in SONs (Self Organized Networks), in a 5G environment and beyond, by introducing external knowledge through personalization. The objective is to build a system for profiling mobile network user behavior using artificial intelligence methods. In other words, it is to provide mobile networks with the ability to understand the habits of these users. This knowledge is then used to configure and optimize the mobile network according to the needs of each user while better managing resources.
9.2.4 Cifre on Multi-task learning to discover the context of the Home, with Orange
Participants: César Viho, Yassine Hadjadj-Aoul.
This is a Cifre contract 2020-2023 including a PhD thesis supervision (PhD of Ahcen Boumhand), done with Orange(Rennes) and IRISA-Université Rennes. The objective is to design a multi-task learning model capable of recognising the different elements involved in users' digital activities (i.e. digital context) from network traces. We also aim to designing a solution capable of recognising (detecting) the appearance of a Media multitasking situation and describing it in a detailed manner (identifying the activities, their temporal framework, their semantic relations) from the network traces.
9.2.5 Cifre on network slicing, with Nokia
Participants: Yassine Hadjadh-Aoul, Gerardo Rubino.
This is a Cifre contract 2021-2024 including a PhD thesis supervision (PhD of Abdelmounaim Bouroudi), done with Nokia Bell Labs (Nozay) and INRIA, on multi-agent reinforcement learning for resource allocation and scheduling in post-5G networks. The objective of the thesis is to propose a distributed resource placement and control system allowing automating network operations in their whole life-cycle from the creation of an end-to-end network slice to its deletion, while continuously optimally self-tuning the resource allocation to match the targeted performances.
9.2.6 Cifre on green video streaming: modeling, evaluation and regulation
Participants: Bruno Tuffin.
This is a Cifre contract 2023-2025 including a PhD thesis supervision (PhD of Burak Kara), done with Synamedia, on the modeling of end-to-end streaming for a greener distribution. The goal is to determine which strategies could allow to reduce the energy impact of streaming. Thoses strategy can be implemented via a regulator. We can think of economic regulation, through taxes and/or incentives, or a technicval regulation by imposing constraints.
9.2.7 Cifre on safe resource management in 6G with distributed decision making
Participants: Yassine Hadjadh-Aoul, Soraya Aït Chellouche.
This is a Cifre contract 2023-2026 including a PhD thesis supervision (PhD of Parsa Rajabzadeh), done with Nokia Bell Labs (Nozay) and INRIA, on IA/ML driven safe resource management in 6G with distributed decision making. The objective of the thesis is to propose a trusted and scalable scheduling strategies for managing network resources in a multi-level distributed context with a distributed decision-making using artificial intelligence (e.g.,multi-agent deep reinforcement learning).
10 Partnerships and cooperations
10.1 International initiatives
10.1.1 Informal international partners
Participants: Yassine Hadjadh-Aoul, Mael Le Treust, Gerardo Rubino, Bruno Tuffin.
- B. Tuffin works with M.K. Nakayama (NJIT, USA) and P.W. Glynn (Stanford University, USA) on rare event simulation.
- B. Tuffin works with P. L'Ecuyer (Université de Montréal, Canada), M.K.Nakayama (NJIT, USA) and A. Owen (Stanford University, USA) on randomized quasi-Monte Carlo methods.
- M. Le Treust works with M. Bloch, Georgia Tech Atlanta, on coordination and channel state masking.
- M. Le Treust works with L. Briceño Arias, Universidad Técnica Federico Santa María, Santiago de Chile, on convex optimization algorithms.
- M. Le Treust works with T. Oechtering, KTH Stockholm, on coding for decentralized control problems.
- M. Le Treust works with T. Tomala, HEC Paris, on sender-receiver games, persuasion and mechanism design.
- G. Rubino works with Alan Krinik from CalPoly, California, on stochastic processes analysis, and with S. Basterrech from the Technical University of Denmark, on Machine Learning topics.
10.1.2 STIC/MATH/CLIMAT AmSud projects
Climate AmSud program: project “ClimateDL”
Participants: Yassine Hadjadh-Aoul, Gerardo Rubino.
- Title: ClimateDL
-
Partner Institution(s):
- Universidad de la República (Uruguay) / Faculty of Engineering: Pablo Rodríguez-Bocca (coord.), Diego Kiedanski, Guillermo Pereira / Faculty of Sciences: Madeleine Renom, Yilian Montesino
- Universidad de Buenos Aires, Argentina: Matilde Rusticucci (coord.), Mariana Barrucand, Soledad Collazo, Solange Suli Universidad Adolfo Ibáñez(Chile)/Faculty of Engineering and Sciences: RodrigoCarrasco (coord.), Javiera Barrera, Eduardo Moreno
- INRIA Rennes: Yassine Hadjadh-Aoul(coord.), Gerardo Rubino, Sebastián Basterrech(collab. ext.)
- Start year: 2022; length: 2 years
- Global coordinator of the project: Pablo Rodríguez-Bocca
- Description: The ClimateDL project brings together experts in climatology, data science, probability and statistical techniques, with as its main objective, the exploration of the different available methods for the forecasting of extreme climate events. Our focus was on the case of extreme temperatures on the seasonal scale, and we addressed the case of the southern South America geographical area, which corresponds to the geographical position of the South American partners. As a completely related goal, we worked on finding new forecasting technologies, centering our research around machine learning tools, but taking into account classic and more recent statistical procedures. We also looked at similar target as meteorological predictions, or at mean temperatures on a future period.
10.2 National initiatives
10.2.1 IRT B-Com
Yassine Hadjadj-Aoul was participating at 20% of his time to the IRT B-Com granted by the ANR until September 2023.
10.2.2 PEPR 5G and future networks
Participants: Yassine Hadjadj-Aoul, Patrick Maillé, Bruno Tuffin.
We participate to the project one NF-MUST of the PEPR 5G and Future Networks. It focuses mainly on transforming client requests into end-to-end service orderings and on mapping them to resources and network level services (to be) provisioned by the multiple underlying networks. There is a clear evolution of 5 and 6G networks towards the provisioning of services involving multiple players and multiple technologies. Project NF-MUST addresses the related roles and interactions between customers and multiple domains in connection to the other “PEPR 5G and Future Networks” projects, to ensure automated production and operation of multi-domain services across multiple providers. Besides ordering services, NF-MUST will drive the management of the life cycle of the infrastructures” provisioned services and partake in their dynamic and automated adaptation and operation.
10.2.3 Inria pilot-project REGALIA
Participants: Patrick Maillé, Bruno Tuffin.
The Regalia pilot project, led by Inria, aims to build a software environment for testing and regulation support to address the risks of bias and disloyalty generated by the algorithms of digital platforms. We participate to this project through the development of the demonstrator DemoWehe on the supervision and detection of differentiated behaviors for Internet service providers.
10.3 Regional initiatives
10.3.1 Informal local partners
Participants: Bruno Sericola.
We collaborate with Emmanuelle Anceaume (Inria team Cidre) and François Castella (Inria team Mingus) on the analysis of population protocols and of random walks on graphs.
11 Dissemination
11.1 Promoting scientific activities
Participants: Yassine Hadjadj-Aoul, Mael Le Treust, Patrick Maillé, Gerardo Rubino, Bruno Sericola, Bruno Tuffin.
11.1.1 Scientific events: organisation
Member of the organizing committees
- Bruno Tuffin has been the Chair of the Steering Committee of the International Conference on Monte Carlo Methods and Applications (MCM) series since August 2021.
- Gerardo Rubino and Bruno Tuffin are members of the Steering Committee of the International Workshop on Rare Event Simulation (RESIM).
- Yassine Hadjadj-Aoul is chairing the Steering Committee of the International Conference on Information and Communication Technologies for Disaster Management (ICT-DM) since 2022.
- Gerardo Rubino was one of the responsibles of the Special Session of the International Joint Conference on Neural Networks (IJCNN'23) intitled “Lifelong Learning: recent advances and challenges”, code L3-RAC, June 2023.
11.1.2 Scientific events: selection
Chair of conference program committees
- Yassine Hadjadj-Aoul served as a TPC Track chair at the IEEE International Conference on Information and Communication Technologies for Disaster Management, Cosenza, Italy (September 2023)
Member of the conference program committees
- Bruno Tuffin served as TPC member of the 35th International Teletraffic Congress (ITC 35), Turin, Italy, October 3-5, 2023, and of Globecom 2023 CSM (2023 IEEE Global Communications Conference).
- Maël Le Treust served as TPC member of the IEEE International Symposium on Information Theory (ISIT).
- Bruno Sericola served as TPC member of the 27th International Conference on Analytical & Stochastic Modelling Techniques & Applications (ASMTA 2023).
- Yassine Hadjadj-Aoul served as TPC member of many international conferences, such as IEEE Globecom, IEEE ICC, IEEE CloudNet, IEEE PIMRC, IEEE ISCC and ACM MSWIM.
- Patrick Maillé served as TPC member of IEEE Globecom and IEEE ICC
- Gerardo Rubino served as TPC member of the conference MASCOTS'23 (31th International Symposium on the Modeling, Analysis, and Simulation of Computer and Telecommunication Systems) and IJCNN'23 (International Joint Conference on Neural Networks).
- Soraya Aït Chellouche served as TPC track co-chair in the IEEE International Conference on Information and Communication Technologies for Disaster Management, Cosenza, Italy (September 2023).
11.1.3 Journal
Member of the editorial boards
- Bruno Tuffin is Area Editor for INFORMS Journal on Computing and Associate Editor for ACM Transactions on Modeling and Computer Simulation and Queueing Systems.
- Maël Le Treust is Associate Editor for IEEE Transactions on Information Theory, area Privacy and Security, since Oct. 2021.
- Bruno Sericola is associate editor for Performance Evaluation. He is also Editor in Chief of the books series “Stochastic Models in Computer Science and Telecommunications Networks”, ISTE/WILEY.
- Gerardo Rubino is associate editor for Performance Evaluation and for the Journal of Dynamics & Games.
- Patrick Maillé is Associate Editor for Electronic Commerce Research and Applications, and for the IEEE Open Journal of the Communications Society.
11.1.4 Invited talks
- Yassine Hadjadj-Aoul delivered a talk, entitled: “The Quest for Safe Deep Reinforcement Learning-driven Network & Slicing: Progress, Pitfalls and Potential” 50, in the SAFE International Workshop, which is part of the International Conference on emerging Networking EXperiments and Technologies (CoNEXT), Paris, France, 2023.
- Gerardo Rubino was invited to give a talk intitled “Time Series Forecasting with Machine Learning” at the Faculty of Sciences of UDELAR, Montevideo, Uruguay, December 2023.
- Bruno Tuffin participated to a panel discussing for young scholars in the field of simulation with valuable insights into identifying suitable publication avenues for their research endeavors 49. Along with other senior editors and panelists, he shared his own experience and suggestions on the peer review process, such as how to navigate through revisions and rejections, and ethical policies. Young scholars were then able to learn the importance of serving the community as a reviewer, and what senior editors expect from reviewers.
11.1.5 Leadership within the scientific community
- Yassine Hadjadj-Aoul is a member of the scientific committee of GT ARC (Automatique et Réseaux de Communication), since Nov. 2017
- Yassine Hadjadj-Aoul is a member of the “IEEE Sig on Big Data with Computational Intelligence” Special Interests Group under the IEEE COMSOC Big Data TC, Since June 2017.
11.1.6 Scientific expertise
- Yassine Hadjadj-Aoul was a member of the scientific evaluation panel for the CHIST-ERA European Research Funding.
- Yassine Hadjadj-Aoul was a projects' evaluator for the Japan Society for Promotion of Science (JSPS), Japan.
- Bruno Tuffin served as a reviewer for the Israel Science Foundation.
11.1.7 Research administration
- Bruno Tuffin was member of the Inria Rennes CRCN selection committee in 2023.
- Maël Le treust was secretary of the Scientific Council of CNRS INS2I in 2019-2023.
11.2 Teaching - Supervision - Juries
Participants: Soraya Ait Chellouche, Yassine Hadjadj-Aoul, Sofiene Jelassi, Mael Le Treust, Patrick Maillé, Gerardo Rubino, Bruno Sericola, Bruno Tuffin, Cesar Viho.
11.2.1 Teaching
- Master: Bruno Tuffin, MEPS (simulation and performance evaluation), 22 hours, M1 University of Rennes 1, France
- Master: Bruno Tuffin, MEPS (simulation), 20 hours, ENS Rennes, France
- Master: Patrick Maillé & Bruno Tuffin, GTA (Game Theory and Applications), 20 hours, M2, ENS Rennes, France
- Master: Patrick Maillé (Game Theory and Agent-Based Modeling), 20 hours, IMT Atlantique
- Bachelor L3: Patrick Maillé (Network performance modeling basics), 20 hours, IMT Atlantique
- Bachelor L3: Patrick Maillé (Models for semiconductor-based components), 15 hours, IMT Atlantique
- Master: Patrick Maillé (Economics of Digital Platforms), 15 hours, IMT Atlantique
- Master, 1st year: Patrick Maillé (Probability and Statistics), 50 hours, IMT Atlantique
- Master, 2nd year: Gerardo Rubino, Scalable Network Infrastructure (SNI), 10 hours, The Research in Computer Science (SIF) master and EIT Digital Master/University of Rennes 1, France
- Supelec Rennes 3rd year: Gerardo Rubino, Dependability Analysis, 15 hours.
- Master 2nd year: Gerardo Rubino, Quality of Experience, twice 4 hours (two different groups of students), Esir/University of Rennes 1, France
- Master Pro 2nd year: Gerardo Rubino, Quality of Experience, 4 hours Esir/University of Rennes 1, France
- Master: Bruno Sericola, MEPS (performance evaluation), 35 hours, M1, Univ Rennes , France
- BUT 3: Bruno Sericola, Probability and Statistics, 20 hours, IUT de Rennes - Univ Rennes, France
- Master M2-Cyber: César Viho, Dynamic Routing Algorithms, 6 hours, ISTIC/University of Rennes, France
- Master M1-Cloud and Networks: César Viho, Networks : from Services to protocols, 36 hours, ISTIC/University of Rennes, France
- Bachelor L3: César Viho, Algorithms on graphs, 40 hours, ISTIC/University of Rennes, France
- Bachelor L3: César Viho, Linux commands/scripts and C Programming, 64 hours, IsticISTIC/University of Rennes, France
- Bachelor L2: César Viho, Network basics and main protocols, 32 hours, ISTIC/University of Rennes, France
- Bachelor L3: Sofiene Jelassi, Oriented Object Programming (JAVA), 44 hours, Istic/University of Rennes 1, France
- Bachelor L3: Sofiene Jelassi, Computer Networks, 20 hours, Istic/University of Rennes 1, France
- Bachelor L3: Sofiene Jelassi, Computer Networks, 4 hours, Istic/University of Rennes 1, France
- BUT 2 : Soraya Ait Chellouche, Network security, 12 hours, IUT de Lannion - Univ Rennes 1, France
- BUT 1 : Soraya Ait Chellouche, Internet architecture, 50 hours, IUT de Lannion - Univ Rennes 1, France
- BUT 1 : Soraya Ait Chellouche, Network services, 26 hours, IUT de Lannion - Univ Rennes 1, France.
- LP ASUR : Soraya Ait Chellouche, TIC, 50 hours, IUT de Saint Malo - Univ Rennes 1, France
- Engineering school 3rd year & Master 2, Soraya Ait Chellouche, Voice Over IP, 15 hours, ESIR & ISTIC - univ Rennes 1, France
- L3 - ISTIC : Soraya Ait Chellouche, Network services, 9h.
- ISTIC/ETRS - FC : Soraya Ait Chellouche, Computer networks fundamentals, 39h.
- ISTIC/ETRS - FC : Soraya Ait Chellouche, Linux fundamentals and Network services, 40h.
- ISTIC/ETRS - FC : Soraya Ait Chellouche, Network security, 98h.
- Master, 2nd year: Yassine Hadjadj-Aoul, Scalable Network Infrastructure (SNI), 18 hours, The Research in Computer Science (SIF) master and EIT DigitalMaster/University of Rennes 1, France
- Master, pro 2nd year: Yassine Hadjadj-Aoul, Multimedia streaming over IP (MMR), 48 hours, Esir/University of Rennes 1, France
- Master, pro 2nd year: Yassine Hadjadj-Aoul,Multimedia services in IP networks (RSM), 29 hours, Esir/University of Rennes 1, France
- Master, pro 2nd year: Yassine Hadjadj-Aoul, Software Defined Networks, 10 hours, Istic/University of Rennes 1, France
- Master, 2nd year: Yassine Hadjadj-Aoul, Video streaming over IP, 8 hours, Istic/University of Rennes 1, France
- Master: YassineHadjadj-Aoul, Introduction to networking (RES), 26 hours, Esir/University of Rennes 1, France
- Master: Yassine Hadjadj-Aoul,Mobile and wireless networking (RESA), 20 hours, Esir/University of Rennes 1, France
- Master 2nd year: Yassine Hadjadj-Aoul, Overview of IoT technologies: focus on LPWAN, 2 hours, INSA, France
- C. Viho was a member of the juries for the recruitment of young Associate Professors and senior Professors at ISTIC-Université Rennes 1.
- Soraya Ait Chellouche was member of validation and certification of studies juries for military personal on network security.
- Soraya Ait Chellouche supervised 15 students on dual study program, IUT de Lannion/IUT de Saint Malo - univ-Rennes 1 & engineering school ESAIP.
- Master M1-SIC, Maël Le Treust, Information Theory
11.2.2 Supervision
Thesis defended in 2023:
- Nicolas Charpenay : “Combinatorial and strategic aspects in Information Theory", funded by CDSN ENS Paris-Saclay, co-supervised with Aline Roumy (Sirocco) at Inria/IRISA, Rennes, October 2020 - December 2023.
- Ali Hodroj : "Enhancing content delivery to multi-homed users in broadband mobile networks, co-supervised by Bruno Sericola and Yassine Hadjadj-Aoul, from Rennes and by Marc Ibrahim from St Joseph University of Beyrouth, november 2015 - December 2023.
- Gwen Maudet: "Exploiting sensor similarity to enhance data collection in massive IoT networks", funded by the ValaDoE chair, co-supervised by Patrick Maillé, Laurent Toutain (IMT Atlantique), Mireille Batton-Hubert (Mines St Etienne), October 2020 - November 2023
Thesis in progress:
- PhD in progress: Burak Kara “Towards a green video streaming: modeling, evaluation and regulation". CIFRE Thesis with Synamedia, started in 2023, supervised by Bruno Tuffin.
- PhD in progress: Orland-Medy Saizonou, “Towards an efficient economic orchestration of 5G and beyond networks". PhD funded by the PEPR 5G and furutre network, started in November 2023 and supervised by Patrick Maillé and Bruno Tuffin.
- PhD in progress: Parsa Rajabzadeh “IA/ML driven safe resource management in 6G with distributed decision making". CIFRE Thesis with Nokia Bell Labs, started in 2021, co-supervised by Yassine Hadjadj-Aoul and Soraya Aït-Chellouche.
- PhD in progress: Zahraa El Attar “Network Function Virtualization and Slice Monitoring". PhD funded by a Ministery Scholarship and started in 2021, co-directed by Yassine Hadjadj-Aoul.
- PhD in progress: Abdelmounaim Bouroudi, “Multi-Agent Reinforcement learning for resources allocation in 5G". CIFRE Thesis with Nokia Bell Labs, started in 2021, co-supervised by Yassine Hadjadj-Aoul and Gerardo Rubino.
- PhD in progress: Amine Rguez, “An availability-aware NFVs placementusing Deep Reinforcement Learning". CIFRE Thesis with EXFO, started in 2020, co-supervised by Yassine Hadjadj-Aoul and Gerardo Rubino.
- PhD in progress: Soumeya Kaada, “Resilience as a Service on Demand for 5G Networks Led by Machine Learningxxx”, CIFRE thesis with Nokia Bell Labs, started in 2020, co-supervised by Sofiene Jelassi and Gerardo Rubino.
- PhD in progress: Ahcene Boumhand, “Multi-Task Learning for Home Context Discovery”, CIFRE thesis with Orange, started in 2020, co-supervised by Yassine Hadjadj-Aoul and César Viho.
- PhD in progress: Sid Ali Hamideche, “Automation beyond 5G: Machine learning applied to profiling user behaviour in mobile services” CIFRE thesis with Nokia Bell Labs, started in 2020, supervised by César Viho.
- PhD in progress: Wilem Lamdani “User/network context-aware resource management for constrained-services in 5G and Beyond 5G networks". Doctoral Contract of university of Rennes, started in 2023, co-supervised by Soraya Aït-Chellouche and César Viho.
External thesis co-supervised by team members:
- PhD in progress: Mengyuan Zhao “Fundamental bounds on distributed information processing”, funded by VR (Swedish Research Council), co-supervised with Tobias Oechtering at KTH, Stockholm, June 2023 - May 2027.
- PhD in progress: Masoud Taghavian “VNF Placement in 5G Networks using AI/ML". Thesis funded by the IRT B-Com, started in 2020, co-supervised by Yassine Hadjadj-Aoul.
11.2.3 Juries
- Bruno Tuffin was an examiner for the PhD of Yuanlu Bai, “Topics in Stochastic Simulation : Rare-Event Estimation and Model Calibration", Columbia University, USA, March 2023.
- Maël Le Treust was external opponent (reviewer) for the PhD defense of Michail Mylonakis, supervised by Mikael Skoglund at KTH, Stockholm, 10th Feb. 2023.
- Yassine Hadjadj-Aoul was a reviewer for the PhD of Tran-Tuan Chu, “A new solution for the complex management of the Internet of Things combining software-driven networks and edge computing: use case in e-health ”, UPEC, Paris, France, December 2023.
- Yassine Hadjadj-Aoul was an invited member in the jury of for the PhD of Gwen Maudet, “Exploiting Sensor Similarity to Enhance Data Collection in MassiveIoT Networks”, IMT Atlantique, November 2023.
- Yassine Hadjadj-Aoul was an examiner for the PhD of Alessandro Aimi, “Algorithms for Reliability Insurance in Low PowerInternet of Things Communication Networks”, Le CNAM, September 2023.
- Yassine Hadjadj-Aoul was a reviewer for the PhD of Kiranpreet Kaur, “Dynamic migration and instantiation of microservices while maintaining end-to-end service chain performance”, Le CNAM, September 2023.
- Yassine Hadjadj-Aoul was a reviewer for the PhD of Farah Yassin, “Radio Resource Management in NB-IoT Networks”, Saint Joseph University of Beyrouth, Lebanon, July 2023.
- Yassine Hadjadj-Aoul was a reviewer for the PhD of El-Heithem Mohammedi, “Machine learning for detecting and localizing configuration errors in an operator network”, Toulouse University, Toulouse, July 2023.
- Gerardo Rubino was a reviewer for the HdR of Sami Souihi intitled “Contributions to Intelligent Networkig – The Next Generation Networking and Internet”, Université Paris Est Créteil, February 17, 2023.
- Gerardo Rubino was a member of the PhD of Patricia Morales Calvo intitled “Elastic Optical Networks Design for the new contexts of Resource Multiplexing”, Technical University Federico Santa María, Valparaíso, Chile, July 2023.
- Soraya Aït Chellouche was a member in the jury of Accreditation to Supervise Research (HDR in French) of Sofiane Hamrioui, “High-performance, autonomous, and secure connected objects networks: Contributions to improving communications between connected objects”, University of Haute Alsace, November 2023.
11.3 Popularization
Participants: Yassine Hadjadj-Aoul, Gerardo Rubino.
11.3.1 Education
- In the context of the CMA xG, Yassine Hadjadj-Aoul participated to the project RIS3 “Intelligent secured, sustainable and sovereign networks”. The RIS3 project aims to address challenges in the digital infrastructure sector by creating a graduate school, providing training for students and professionals, and enhancing the sector's attractiveness. The project leverages the telecommunications industry in Brittany and the expertise of renowned research laboratories to ensure excellence in training and research for future communication systems.
- G.Rubino makes regular presentations to high school students about the research work in general, and specific technical topics in particular. Current talks:
- Randomness as a tool
- Internet as a research problem
- Greatest challenge in maths:the Riemann Hypothesis
- Greatest challenge in computer science: the “P versus NP” problem
12 Scientific production
12.1 Publications of the year
International journals
Invited conferences
International peer-reviewed conferences
National peer-reviewed Conferences
Conferences without proceedings
Reports & preprints
Other scientific publications