2023Activity reportProject-TeamMORPHEME
RNSR: 201120999G- Research center Inria Centre at Université Côte d'Azur
- In partnership with:CNRS, Université Côte d'Azur
- Team name: Morphologie et Images
- In collaboration with:Laboratoire informatique, signaux systèmes de Sophia Antipolis (I3S), Institut de Biologie de Valrose
- Domain:Digital Health, Biology and Earth
- Theme:Computational Biology
Keywords
Computer Science and Digital Science
- A3.4. Machine learning and statistics
- A3.4.1. Supervised learning
- A3.4.2. Unsupervised learning
- A3.4.4. Optimization and learning
- A3.4.6. Neural networks
- A3.4.7. Kernel methods
- A3.4.8. Deep learning
- A5.3. Image processing and analysis
- A5.3.2. Sparse modeling and image representation
- A5.3.3. Pattern recognition
- A5.3.4. Registration
- A5.4.3. Content retrieval
- A5.4.4. 3D and spatio-temporal reconstruction
- A5.4.5. Object tracking and motion analysis
- A5.4.6. Object localization
- A5.9. Signal processing
- A5.9.3. Reconstruction, enhancement
- A5.9.5. Sparsity-aware processing
- A5.9.6. Optimization tools
- A6.1. Methods in mathematical modeling
- A6.1.1. Continuous Modeling (PDE, ODE)
- A6.1.2. Stochastic Modeling
- A6.3.1. Inverse problems
Other Research Topics and Application Domains
- B1.1. Biology
- B1.1.3. Developmental biology
- B2.6. Biological and medical imaging
1 Team members, visitors, external collaborators
Research Scientists
- Xavier Descombes [Team leader, INRIA, Senior Researcher, HDR]
- Florence Besse [CNRS, Senior Researcher, HDR]
- Laure Blanc-Féraud [CNRS, Senior Researcher, HDR]
- Luca Calatroni [CNRS, Researcher]
- Eric Debreuve [CNRS, Researcher, HDR]
- Grégoire Malandain [INRIA, Senior Researcher, HDR]
- Caroline Medioni [CNRS, Researcher, HDR]
Faculty Member
- Fabienne De Graeve [UNIV COTE D'AZUR, CNRS, INSERM, IBV, Associate Professor]
Post-Doctoral Fellow
- Emmanuel Bouilhol [UNIV COTE D'AZUR, Post-Doctoral Fellow, from Sep 2023]
PhD Students
- Asma Chalabi [CNRS, from Oct 2023]
- Asma Chalabi [UNIV COTE D'AZUR, until Sep 2023]
- Cedric Dubois [UNIV COTE D'AZUR, until Mar 2023]
- Morgane Fierville [CNRS, from Nov 2023]
- Haydar Jammoul [UNIV COTE D'AZUR, from Oct 2023]
- Faisal Jayousi [CNRS]
- Bastien Laville [UNIV COTE D'AZUR, until Sep 2023]
- Marta Lazzaretti [UNIV GENES, until Oct 2023]
- Imane Lboukili [Johnson & Johnson]
- Alexandre Martin [INRIA]
- Hamza Mentagui [CNRS, from Dec 2023]
- Mohamad Mohamad [UNIV COTE D'AZUR, from Oct 2023]
- Clara Sanchez [UNIV COTE D'AZUR, until Sep 2023]
- Aneva Doliciane Tsafack [UNIV COTE D'AZUR, from Nov 2023]
Technical Staff
- Marta Lazzaretti [CNRS, Engineer, from Nov 2023, IE ]
Interns and Apprentices
- Chloe Benbekrite [INRIA, Intern, from Jun 2023 until Sep 2023]
- Sonia Ciuffreda [INRIA, Intern, from May 2023 until Oct 2023]
- M'hamed Essafri [Université Paul Sabatier, from Feb 2023 until Jul 2023, Co-supervision with E. Soubies]
- Rouaa Hoblos [INRIA, Intern, from Mar 2023 until Aug 2023]
- Wassila Khatir [UNIV COTE D'AZUR, Intern, until Jun 2023]
- Hamza Mentagui [CNRS, Intern, from Apr 2023 until Oct 2023]
- Mohamad Mohamad [INRIA, Intern, until Feb 2023]
- Carlo Santambrogio [CNRS, Intern, from Mar 2023 until Aug 2023]
- Aneva Doliciane Tsafack [CNRS, Intern, from Feb 2023 until Jul 2023]
Administrative Assistants
- Isabelle Strobant [INRIA]
- Stéphanie Verdonck [INRIA, from Sep 2023]
Visiting Scientists
- Stefano Aleotti [Università degli studi dell'Insubria, from Mar 2023 until Aug 2023]
- Mohamad Mohamad [Politecnico Torino, from Oct 2023 until Oct 2023]
- Mohamad Mohamad [Politecnico Torino, from Mar 2023 until Apr 2023]
- Danilo Pezzi [Università degli studi di Modena e Reggio Emilia, from Feb 2023 until Mar 2023, PhD visit]
External Collaborators
- Thomas Boudier [Centrale Méditerranée, Associate Professor, from Sep 2023, HDR]
- Ellen Van Obberghen [Inserm, DR1, HDR]
2 Overall objectives
Morpheme is a joint project between INRIA, CNRS and the University of Côte d'Azur (UniCA); Signals and Systems Laboratory (I3S) (UMR 6070); the Institute for Biology of Valrose (iBV) (CNRS/INSERM).
The scientific objectives of Morpheme are to characterize and model the development and the morphological properties of biological structures from the cell to the supra-cellular scale. Being at the interface between computational science and biology, we plan to understand the morphological changes that occur during development combining in vivo imaging, image processing and computational modeling.
The morphology and topology of mesoscopic structures, indeed, do have a key influence on the functional behavior of organs. Our goal is to characterize different populations or development conditions based on the shape of cellular and supra-cellular structures, including micro-vascular networks and dendrite/axon networks. Using microscopy or tomography images, we plan to extract quantitative parameters to characterize morphometry over time and in different samples. We will then statistically analyze shapes and complex structures to identify relevant markers and define classification tools. Finally, we will propose models explaining the temporal evolution of the observed samples. With this, we hope to better understand the development of normal tissues, but also characterize at the supra-cellular level different pathologies such as the Fragile X Syndrome, Alzheimer or diabetes.
3 Research program
3.1 Research program
The recent advent of an increasing number of new microscopy techniques giving access to high throughput screenings and micro or nano-metric resolutions provides a means for quantitative imaging of biological structures and phenomena. To conduct quantitative biological studies based on these new data, it is necessary to develop non-standard specific tools. This requires using a multi-disciplinary approach. We need biologists to define experiment protocols and interpret the results, but also physicists to model the sensors, computer scientists to develop algorithms and mathematicians to model the resulting information. These different expertises are combined within the Morpheme team. This generates a fecund frame for exchanging expertise, knowledge, leading to an optimal framework for the different tasks (imaging, image analysis, classification, modeling). We thus aim at providing adapted and robust tools required to describe, explain and model fundamental phenomena underlying the morphogenesis of cellular and supra-cellular biological structures. Combining experimental manipulations, in vivo imaging, image processing and computational modeling, we plan to provide methods for the quantitative analysis of the morphological changes that occur during development. This is of key importance as the morphology and topology of mesoscopic structures govern organ and cell function. Alterations in the genetic programs underlying cellular morphogenesis have been linked to a range of pathologies.
Biological questions we will focus on include:
- what are the parameters and the factors controlling the establishment of ramified structures? (Are they really organized to ensure maximal coverage? How are genetic and physical constraints limiting their morphology?),
- how are newly generated cells incorporated into reorganizing tissues during development? (is the relative position of cells governed by the lineage they belong to?)
Our goal is to characterize different populations or development conditions
based on the shape of cellular and supra-cellular structures, e.g. micro-vascular
networks, dendrite/axon networks, tissues from 2D, 2D+t, 3D or 3D+t images (obtained with confocal microscopy, video-microscopy, photon-microscopy or micro-tomography). We plan to extract shapes or quantitative parameters to characterize the morphometric properties of different samples. On the one hand, we will
propose numerical and biological models explaining the temporal evolution of the
sample, and on the other hand, we will statistically analyze shapes and complex
structures to identify relevant markers for classification purposes. This should
contribute to a better understanding of the development of normal tissues but
also to a characterization at the supra-cellular scale of different pathologies such
as Alzheimer, cancer, diabetes, or the Fragile X Syndrome.
In this multidisciplinary context, several challenges have to be faced. The
expertise of biologists concerning sample generation, as well as optimization of
experimental protocols and imaging conditions, is of course crucial. However,
the imaging protocols optimized for a qualitative analysis may be sub-optimal
for quantitative biology. Second, sample imaging is only a first step, as we need
to extract quantitative information. Achieving quantitative imaging remains an
open issue in biology, and requires close interactions between biologists, computer
scientists and applied mathematicians. On the one hand, experimental and imaging protocols should integrate constraints from the downstream computer-assisted
analysis, yielding to a trade-off between qualitative optimized and quantitative optimized protocols. On the other hand, computer analysis should integrate constraints specific to the biological problem, from acquisition to quantitative information extraction. There is therefore a need of specificity for embedding precise
biological information for a given task. Besides, a level of generality is also desirable for addressing data from different teams acquired with different protocols
and/or sensors.
The mathematical modeling of the physics of the acquisition system will yield
higher performance reconstruction/restoration algorithms in terms of accuracy.
Therefore, physicists and computer scientists have to work together. Quantitative
information extraction also has to deal with both the complexity of the structures of interest (e.g., very dense network, small structure detection in a volume,
multiscale behavior,
-
Imaging: this includes i) definition of the studied populations (experimental
conditions) and preparation of samples, ii) definition of relevant quantitative
characteristics and optimized acquisition protocol (staining, imaging,
) for the specific biological question, and iii) reconstruction/restoration of native data to improve the image readability and interpretation. - Feature extraction: this consists in detecting and delineating the biological structures of interest from images. Embedding biological properties in the algorithms and models is a key issue. Two main challenges are the variability, both in shape and scale, of biological structures and the huge size of data sets. Following features along time will allow to address morphogenesis and structure development.
- Classification/Interpretation: considering a database of images containing different populations, we can infer the parameters associated with a given model on each dataset from which the biological structure under study has been extracted. We plan to define classification schemes for characterizing the different populations based either on the model parameters, or on some specific metric between the extracted structures.
- Modeling: two aspects will be considered. This first one consists in modeling biological phenomena such as axon growing or network topology in different contexts. One main advantage of our team is the possibility to use the image information for calibrating and/or validating the biological models. Calibration induces parameter inference as a main challenge. The second aspect consists in using a prior based on biological properties for extracting relevant information from images. Here again, combining biology and computer science expertise is a key point.
4 Application domains
Among the applications addressed by Morpheme team we can cite:
- Kidney cancer classification from histological images
- IMP-RNA (ribonucleicc acid) granules detection and classification from confocal image
- Extra-cellular matrix detection and characterization from confocal images
- Axon growth modeling
- Glial cell detection and characterization for the study of high-fat diets
- Death and division time detection and type classification of cells in microscopy time-lapses
- Plankton images analysis and classification
- Morphogenesis and embryogenesis
- Numerical super-resolution techniques
- Convex and non-convex sparse optimization with applications to biomedical imaging
- Statistical and learning-based approaches for parameter selection in imaging inverse problems
- Physics-inspired machine learning for fluorescence microscopy
5 Highlights of the year
5.1 Awards
- Laure Blanc-Féraud has been awarded the CNRS silver medal (CNRS communication).
- Luca Calatroni has been awarded an ERC Starting Grant.
6 New software, platforms, open data
6.1 New software
6.1.1 Astec
-
Name:
Adaptative Segmentation and Tracking of Embryonic Cells
-
Keywords:
3D, 4D, Data fusion, Image segmentation, Fluorescence microscopy, Morphogenesis, Embryogenesis
-
Scientific Description:
ASTEC stands for Adaptive Segmentation and Tracking of Embryonic Cells, and was first developed during L. Guignard PhD thesis, "Quantitative analysis of animal morphogenesis: from high-throughput laser imaging to 4D virtual embryo in ascidians, Léo Guignard, 2015". It was later published in "Contact area–dependent cell communication and the morphological invariance of ascidian embryogenesis, Léo Guignard at al., Science 2020"
-
Functional Description:
This software suite aims at providing quantitative analysis of multi-angle acquisitions of SPIM images, and the segmentation of the temporal series of 3D images, together with quantitative informations.
- URL:
- Publications:
-
Contact:
Grégoire Malandain
-
Participants:
Patrick Lemaire, Leo Guignard, Emmanuel Faure, Gaël Michelin
-
Partners:
CRBM - Centre de Recherche en Biologie cellulaire de Montpellier, LIRMM
6.1.2 sparse_constraint_relax
-
Name:
sparse_constraint_relaxation
-
Keywords:
Optimization, Sparse regularization, Super-resolution
-
Functional Description:
The optimization of a L2 term under a L0 term constraint is made by relaxation of the initial functional with a continuous exact functional (with same global minimizers). Numerical algorithm is coded for super-resolution for Single Molecule localization microscopy (deconvolution and super-resolution on sparse molecules). We minimize the squared norm and the relaxation Q(x), using Nonmonotone Accelerated Proximal gradient algorithm which was presented in Accelerated proximal gradient methods for nonconvex programming by Li, Huan and Lin, Zhouchen in Advances in neural information processing systems. In order to do so, we use gradient of the square norm and the proximal of Q(x). There are two functions for the proximal operator, and recommend the fast one for the calculations, but the normal one to better understand what the program is doing. A failsafe function is added to ensure that we always obtain a k-sparse solution. The file uses a simulated acquistion, Testimage.
- URL:
-
Authors:
Arne Henrik Bechensteen, Laure Blanc-Féraud, Gilles Aubert
-
Contact:
Arne Henrik Bechensteen
-
Partner:
I3S
6.1.3 COL0RME
-
Name:
COvariance-based l0 super-Resolution Microscopy with intensity Estimation
-
Keywords:
Super-resolution, Optimization, Fluorescence microscopy, Sparsity
-
Functional Description:
The code is dedicated to the super-resolution method COL0RME (COvariance-based l0 super-Resolution Microscopy with intensity Estimation) that improves the spatio-temporal resolution of images acquired by common fluorescent microscopes and conventional blinking or flucutating fluorophores. The method is composed of two steps: the former where both the emitters' independence and the sparse distribution of the fluorescent molecules are exploited to provide an accurate localization, the latter where real intensity values are estimated given the computed support. More information in the articles: https://hal.science/hal-02979332, https://hal.science/hal-029793
-
Release Contributions:
First version
- URL:
- Publications:
-
Authors:
Vasiliki Stergiopoulou, Laure Blanc-Féraud, Luca Calatroni, Jose Henrique De Morais Goulart
-
Contact:
Vasiliki Stergiopoulou
-
Partner:
I3S
6.1.4 FluoGAN
-
Name:
Fluorescence image deconvolution via stochastic fluctuation analysis and generative adversarial learning
-
Keywords:
Image analysis, Fluorescence microscopy, Inverse problem, Optimisation, Generative Models
-
Functional Description:
This repository contains an adaptation of CryoGAN and MSR-GAN inverse problem framework for super-resolution in fluorescence microscopy.
- URL:
-
Contact:
Luca Calatroni
6.1.5 OPS
-
Name:
Off-the-grid python made simple
-
Keywords:
Off-the-grid, Computational biology, Optimisation
-
Functional Description:
* Object-oriented, based on PyTorch, * Implements Dirac measures, curve measures and dynamic measures, * Algorithms: Sliding Frank-Wolfe, Charge Sliding Fran-Wolfe, Dynamic Frank-Wolfe, Conic Particle Gradient Descent, * Examples and tests with SMLM images stack.
- URL:
-
Contact:
Bastien Laville
7 New results
7.1 Fluorescence image deconvolution microscopy via generative adversarial learning (FluoGAN)
Participants: Hamza Mentagui, Laure Blanc-Féraud, Luca Calatroni, Sébastien Schaub.
Image super-resolution techniques exploiting the stochastic fluctuations of image intensities have become a powerful tool in fluorescence microscopy. Compared to other approaches, these techniques can be applied under standard acquisition settings and do not require special microscopes nor fluorophores. Most of these approaches can be mathematically modeled making use of second-order statistics possibly combined with a priori regularization on the desired solution. In this work, we consider a different paradigm and formulate a physical-inspired data-driven approach based on generative learning. By simulating fluorescence and noise fluctuations by means of a suitable double Poisson-type process, the unknown distribution of the fluctuating sequence of low- resolution and noisy images is approximated via a GAN-type approach (Generative Adversial Network)where both physical and network parameters are optimized, see 2. Compared to this previous work, we use a double Poisson process to simulate fluctuations of fluorophores on one side and noise due to ambiant fluorophores on the other side. We also provide theoretical insights on the choice of the corresponding cost functionals and gradient computations, and assess practical performance on simulated Argolight (see figure 1).

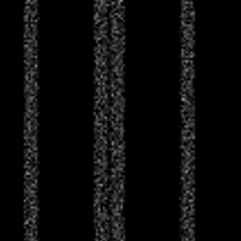

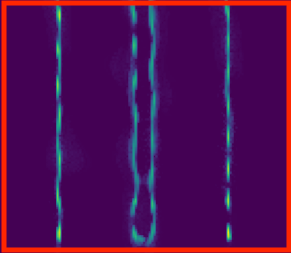
7.2 Stochastic Gradient Descent in Lebesgue spaces with variable exponents
Participants: Luca Calatroni, Marta Lazzaretti, Claudio Estatico [University of Genoa], Zeljko Kereta [University College London].
We studied stochastic gradient descent (SGD) algorithm for solving linear inverse problems (e.g., CT image reconstruction) in the Banach space framework of variable exponent Lebesgue spaces
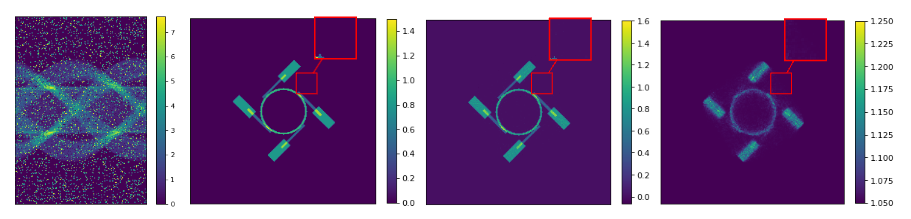
The work is published in 22.
7.3 Fluctuation-based deconvolution in fluorescence microscopy using plug-and-play denoisers
Participants: Laure Blanc-Féraud, Luca Calatroni, Vasiliki Stergiopoulou, Sach Mukherjee [University of Cambridge].
Within the framework of inverse problems, modern approaches in fluorescence microscopy reconstruct a super-resolved image from a temporal stack of frames by carefully designing suitable hand-crafted sparsity-promoting regularizers. Numerically, such approaches are solved by proximal gradient-based iterative schemes. Aiming at obtaining a reconstruction more adapted to sample geometries (e.g. thin filaments), we adopt a plug-and-play denoising approach with convergence guarantees and replace the proximity operator associated with the explicit image regularizer with an image denoiser (i.e. a pre-trained denoising network) which, upon appropriate training, mimics the action of an implicit prior. To account for the independence of the fluctuations between molecules, the model relies on second-order statistics. The denoiser is then trained on covariance images coming from data representing sequences of fluctuating fluorescent molecules with filament structure. The method is evaluated on both simulated and real fluorescence microscopy images, showing its ability to correctly reconstruct filament structures with high values of peak signal-to-noise ratio (see Figure 3).
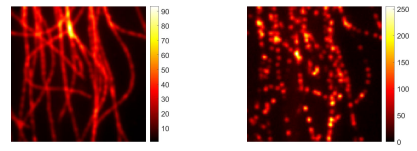
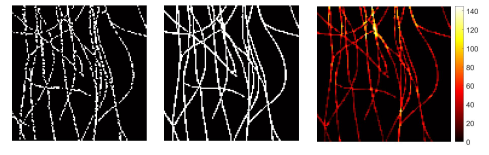
The work is published in 23.
7.4 Off-the-grid dynamic super-resolution for fluorescence microscopy
Participants: Bastien Laville, Aneva Tsafack, Laure Blanc-Féraud, Gilles Aubert.
Recent years have seen the development of super-resolution variational optimization in measurement spaces. These so-called "gridless" approaches offer both theoretical (uniqueness, reconstruction guarantees) and numerical results, with very convincing results in biomedical imaging. However, gridless variational optimization is formulated for the reconstruction of point sources, which is not always suitable for biomedical imaging applications: more realistic biological structures such as curves, to represent blood vessels or filaments, would also need to be reconstructed.
In this work, we developed a new approach for gridless reconstruction of curves, understood as the reconstruction of a vector measure
First numerical implementation is illustrated in Figure 4. A new data term for CROC has been proposed linking scalar observed data
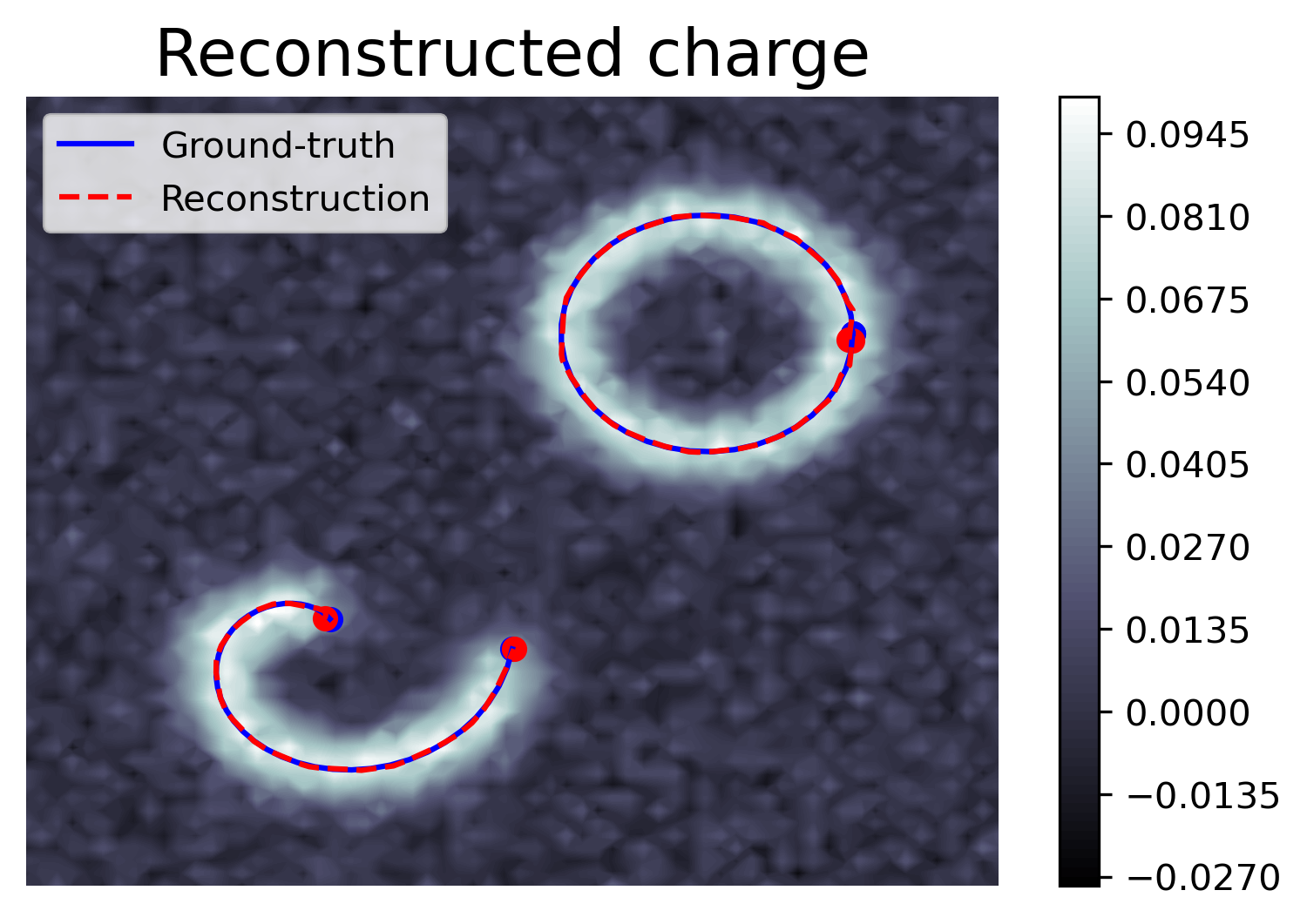
Results on synthetic examples of grid-free reconstruction. The source is in blue, the reconstruction in red.
This work is published in 20, 21.
A code is available at 6.1.5
7.5 Beyond sparse coding in V1
Participants: Luca Calatroni persIliasRentzeperis[Consejo Superior de Investigaciones Cientificas], Dario Prandi [CNRS], Laurent Perrinet [CNRS].
Growing evidence indicates that only a sparse subset from a pool of sensory neurons is active for the encoding of visual stimuli at any instant in time. Traditionally, to replicate such biological sparsity, generative models have been using the
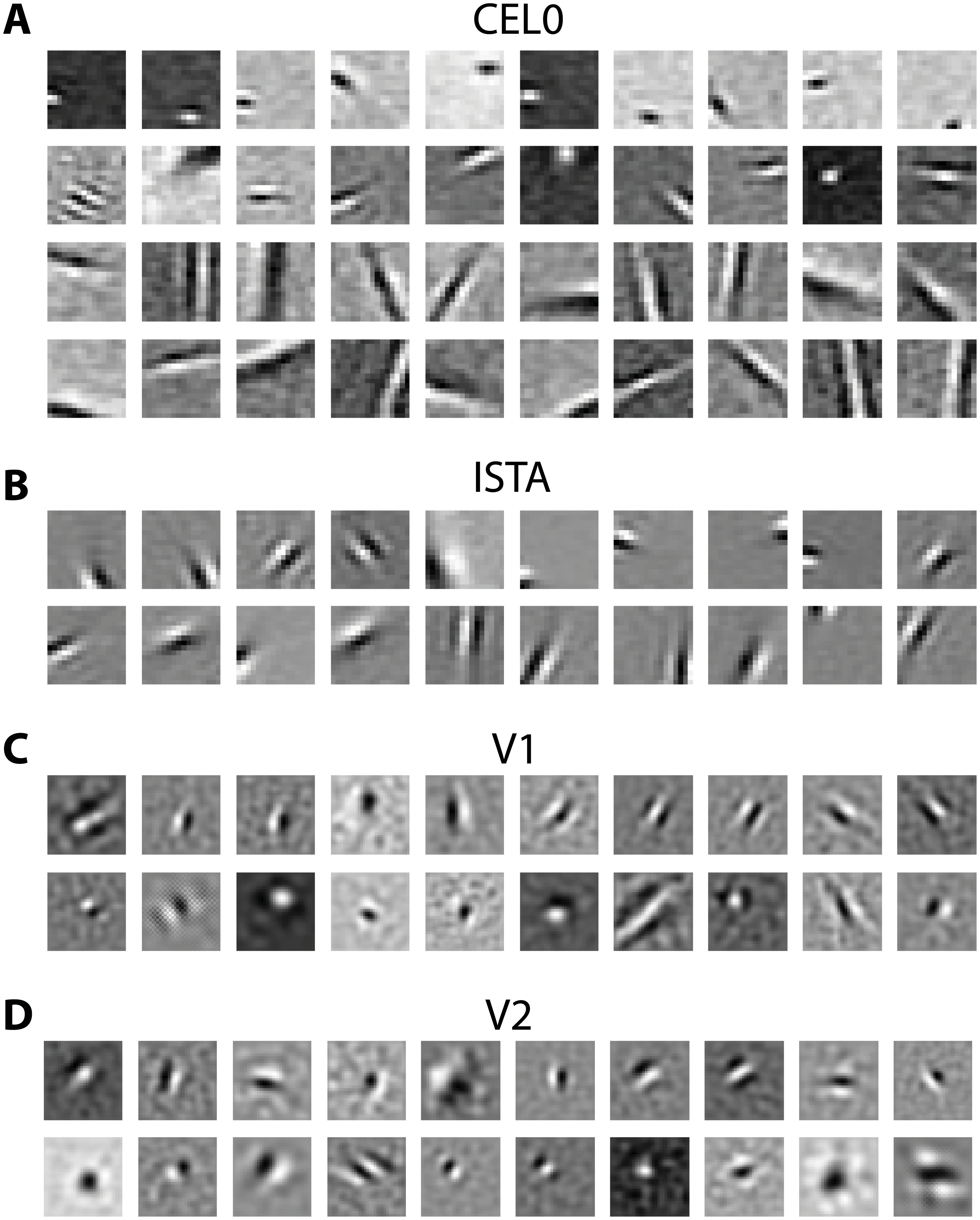
The results are published in 19.
The code is available at github.com/rentzi.
7.6 Nearest-Neighbor Kernel for the Classification of Plankton Images
Participants: Cédric Dubois, Eric Debreuve, Jean-Olivier Irisson [LOV, Villefranche-sur-mer].
Plankton organisms are a key component of the Earth bio-sphere. The phytoplankton captures the CO2 of the atmosphere, and the zooplankton, as a phytoplankton predator, aggregates it and stores it on the ocean floor when it dies. This so-called carbon pump is studied by ecologists in order to predict its future efficiency in a climate change era. A modern approach consists in studying the relation between the living environment of the organisms and their functional traits, among which the size stands out. Indeed, a high correlation has been observed between zooplankton size and carbon sequestration. Scientists have developed in situ imaging instruments and built large databases. Taxonomic identification from images is required to estimate the individual volumes of the organisms based on their morphology. The development of automated classification methods has been essential to aid operators in their identification task. The breakthrough of Artificial Neural Network (ANN) made it possible to come up with efficient classifiers. However, the decisions of such classifiers are hard to interpret or explain. We put forward the idea that following the transform-then-classify approach of ANNs using a simple, explicit transform can result in a classifier whose predictions are both interpretable (thus, trustable) and accurate. The proposed transform is defined as a linear combination of per-class target vectors, and the classification is performed, like with ANNs, by a nearest-target decision (see Figure 6). Furthermore, as a main theoretical result, we establish that the proposed transform defines a kernel associated with the Weigthed-Nearest-Neighbors (wNN) classifier. Incidentally, this allows to interpret the wNN classifier as a member of the transform-then-classify family of classifiers.
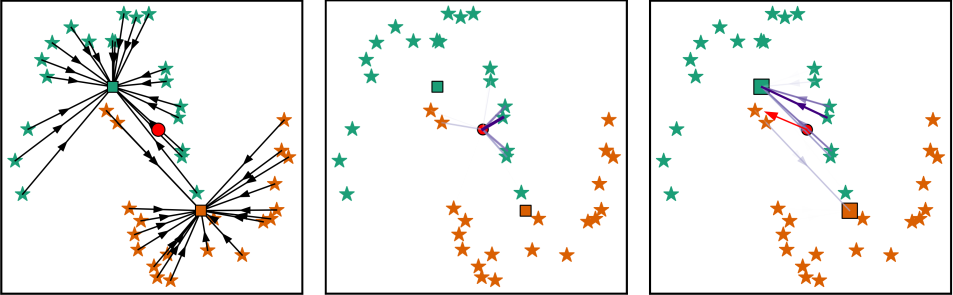
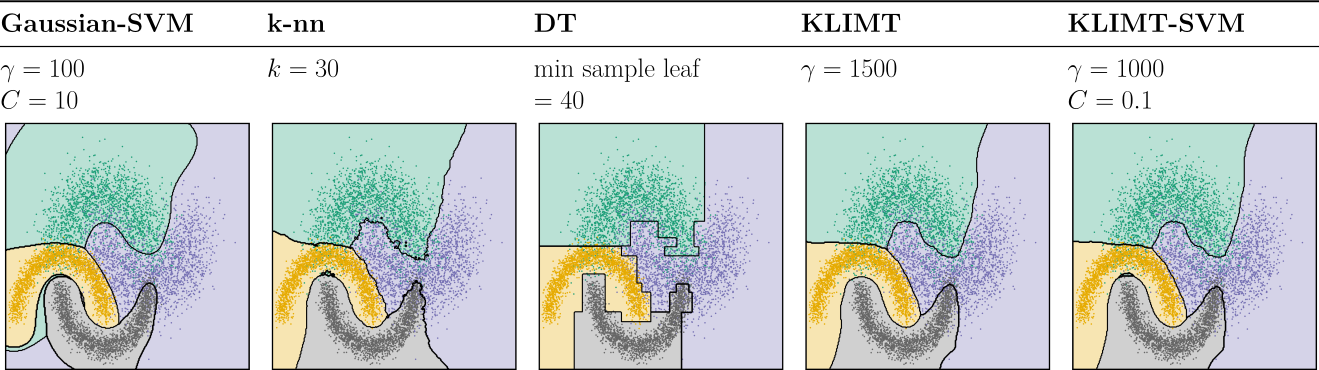
7.7 Dynamic analysis framework to detect cell division and cell death in live-cell imaging, using signal processing and machine learning
Participants: Asma Chalabi, Eric Debreuve, Jérémie Roux [Ircan, Nice].
The detection of cell division and cell death events in live-cell assays has the potential to produce robust metrics of drug pharmacodynamics and return a more comprehensive understanding of tumor cells responses to cancer therapeutic combinations. As cancer drugs may have complex and mixed effects on the biology of the cell, knowing precisely when cellular events occur in a live-cell experiment allows to study the relative contribution of different drug effects (cytotoxic/cell death or cytostatic/cell division) on a cell population. Yet, classical methods require dyes (fluorescent molecules) to measure cell viability as an end-point assay, where the proliferation rates can only be estimated when both viable and dead cells are labeled simultaneously, not to mention that the cell division events are often discarded due to analytical limitations. Live-cell imaging is a promising cell-based assay to determine drug efficacies. However, its main limitation remains the accuracy and depth of the analyses, to acquire automatic measures of the cellular response phenotype, making the understanding of drug action on cell populations difficult. We propose a new algorithmic architecture integrating machine learning, image and signal processing methods to perform dynamic image analyses of single cell events in time-lapse microscopy experiments of drug pharmacological profiling. Our event detection method is based on a pattern detection approach on the local entropy in polarized light frames, making it free of any labeling step and exhibiting two distinct patterns for cell division and death events. Our analysis framework is an open source and adaptable workflow that automatically predicts cellular events (and their times) from each single cell trajectory, along with other classic cellular features of cell image analyses, as a promising solution in pharmacodynamics (see Figure 7). This project is currently supported by two actions studying the possibility of launching a startup company (Young Entrepreneur Program, Labex SignaLife and Emergence et Accompagnement, Canceropôle PACA).
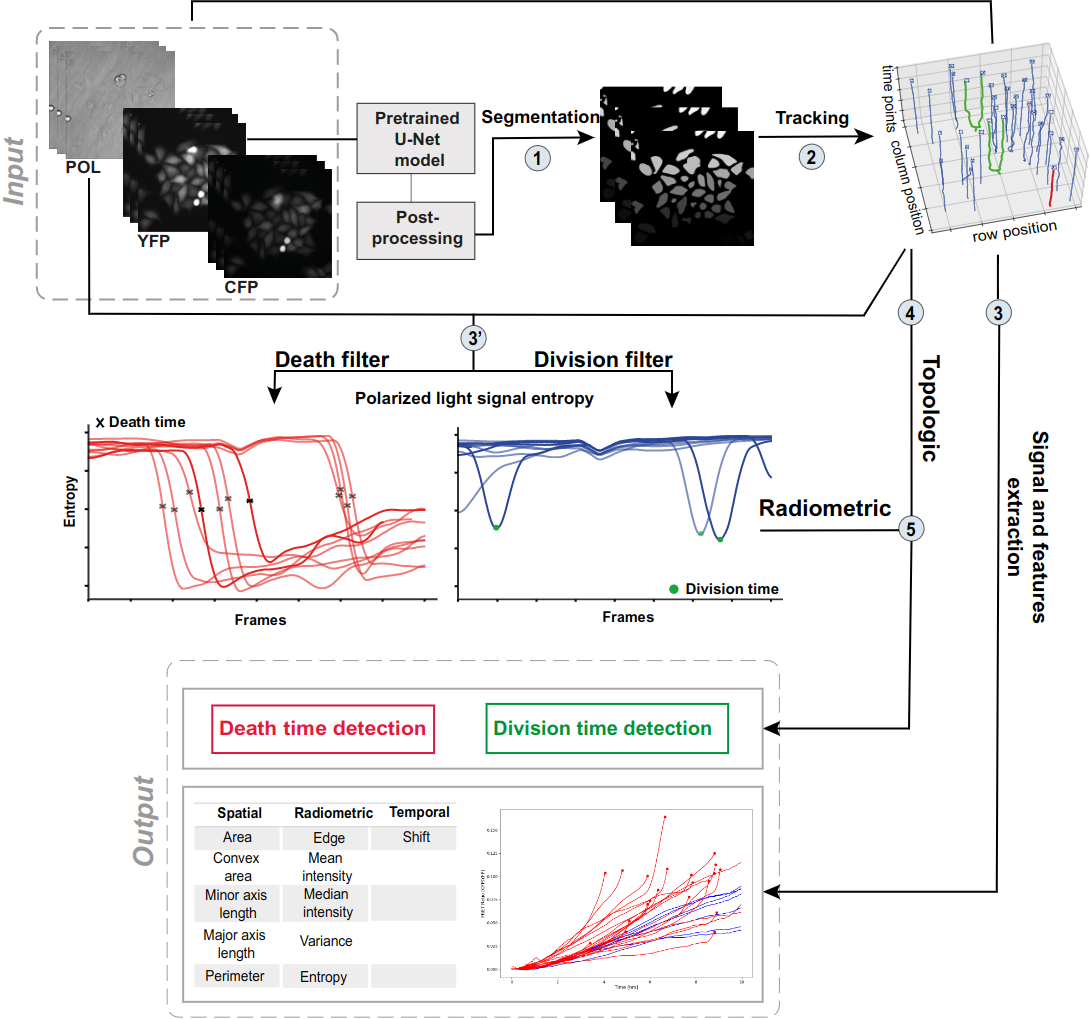
7.8 Developing methodologies to quantitatively analyze RNP granule dynamic properties upon aging
Participants: Gabriel Michaux, Eric Debreuve, Xavier Descombes, Fabienne De Graeve.
This work was made in collaboration with Fabienne de Graeve, iBV, Nice, France.
Recent technological advances in bioimaging have enabled biologists to investigate dynamic processes in living cells at high spatial and temporal resolutions. Quantitative analysis of these dynamic processes relies on the development of appropriate tracking methods associated with a spatio-temporal analysis. We developed particle tracking and event detection methods to analyze the movements and interactions of Imp-RNP (Ribonucleoprotein) granules in the cell bodies of mushroom neurons in Drosophila brain explants. The individual granule trajectories are considered globally as a graph embedded in a space-time domain. The base granule fusion and splitting events can occur in specific sequences which build particular graph patterns. The proposed analysis consists in defining the relevant patterns, and then searching them in actual graphs (see Figure 8).
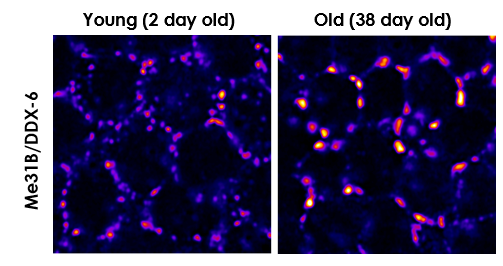
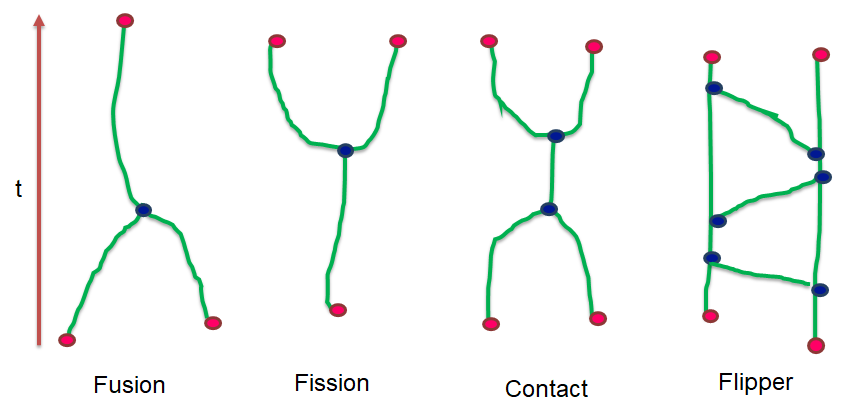
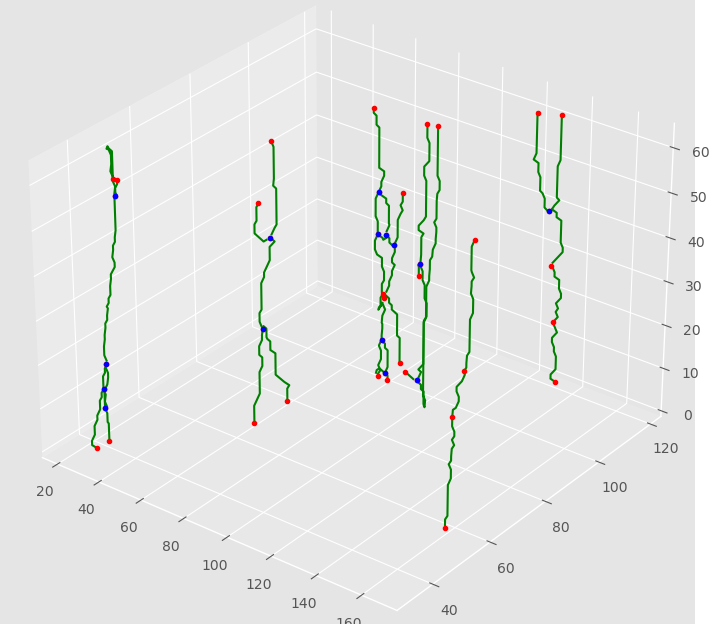
7.9 Tumoral environment characterization of epidermoid carcinoma
Participants: Wassila Khatir, Xavier Descombes, Fabienne Anjuère [IPMC, Sophia Antipolis].
This study aimed to identify biomarkers of relapse following surgical intervention by analyzing 17 primary squamous cell carcinoma tumors (9 relapsing, 8 non-relapsing) using multiplex (11 markers) mass spectrometry imaging to investigate the interactions between different cell types and structures. We first have detected cells in the different channels using local thresholding. A k-means clustering has then exihibited different populations and in particular several macrophage subpopulations. An analysis of the cells distances with respect to the tumor and of their density in specific structures (vessels, nerves) has shown some specific localization of these populations (see Figure 9).
These findings have revealed insights into the complex links between immune cells, tumor structures and relapse. The identified B cell influx, as well as the differential behavior of macrophage subpopulations, may have implications for understanding tumor progression and designing targeted therapies to prevent relapse following surgery.
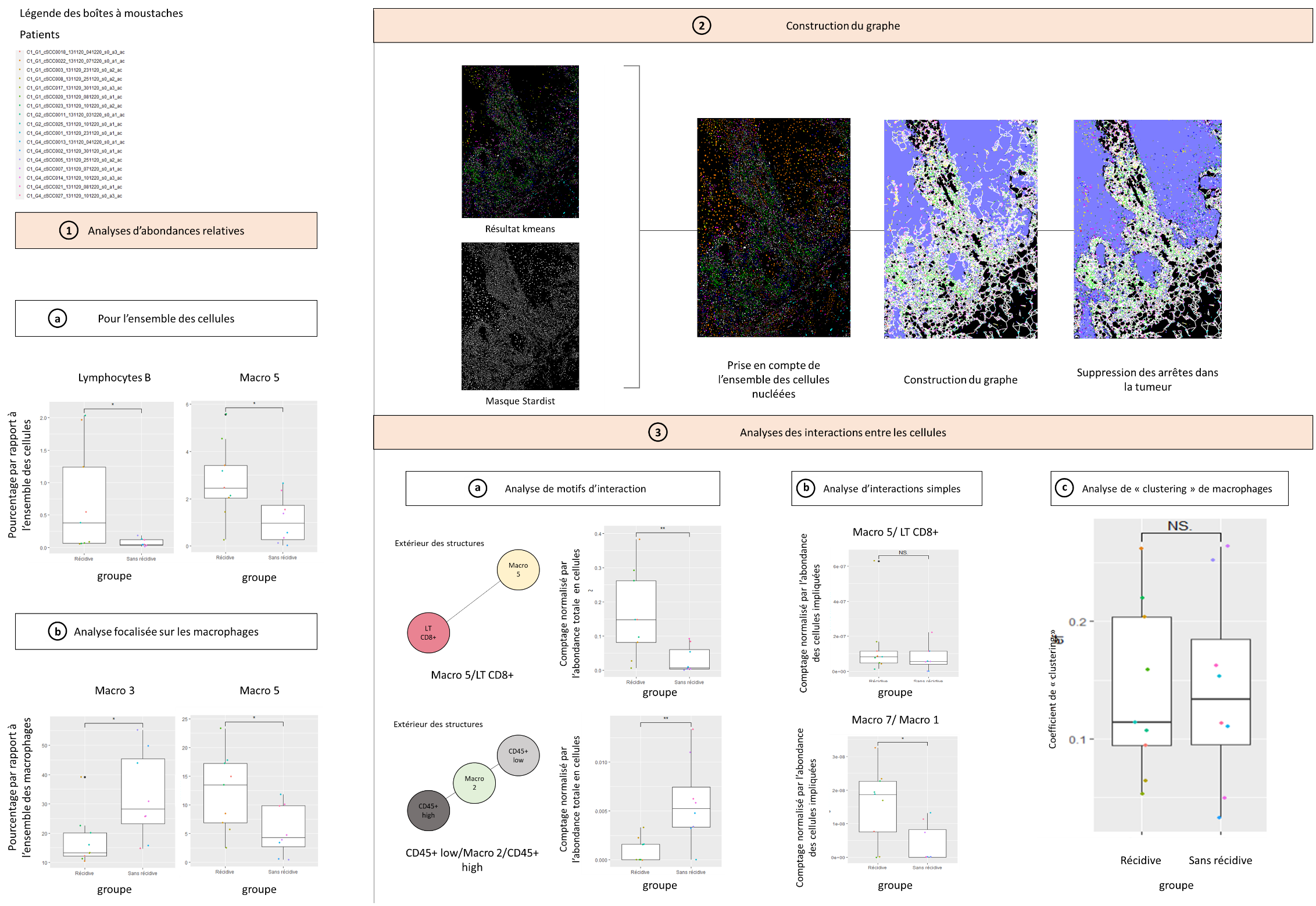
7.10 Nuclei classification from histopathological data
Participants: Chloé Benbekrite, Xavier Descombes, Damien Ambrosetti [CHU, Nice], Paul Hannetel [CHU, Nice].
We have developed a features-based supervised algorithm for nuclei classification in histopathological images. Features are composed of color and shape properties of detected nuclei as well as the color inside a crown surrounding the nuclei. We have considered five classes of cells : sane, cancerous, fibroblast, epithelial, lymphocyte. Usign a SVM classifier we have obtained a weighted F1 score of 0.89. We then consider a labelled graph where the node are defined by the nuclei and the edges by adjacent cells in the associated Voronoi tesselation. A regularization step is applied using a local majority vote. However lymphocyte and epithelial cells are excluded from this regularization step due their low prevalence. After regularization, the Voronoi tesselation provides a segmentation of the tissue in seral regions composed of tumour, sane areas and fibrous tissues (see Figure 10). These first results will be improved by considering a regularization at a coarser scale, that is considering the different obtained regions.
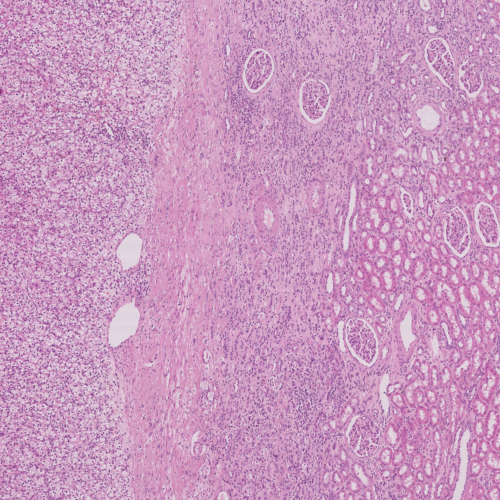
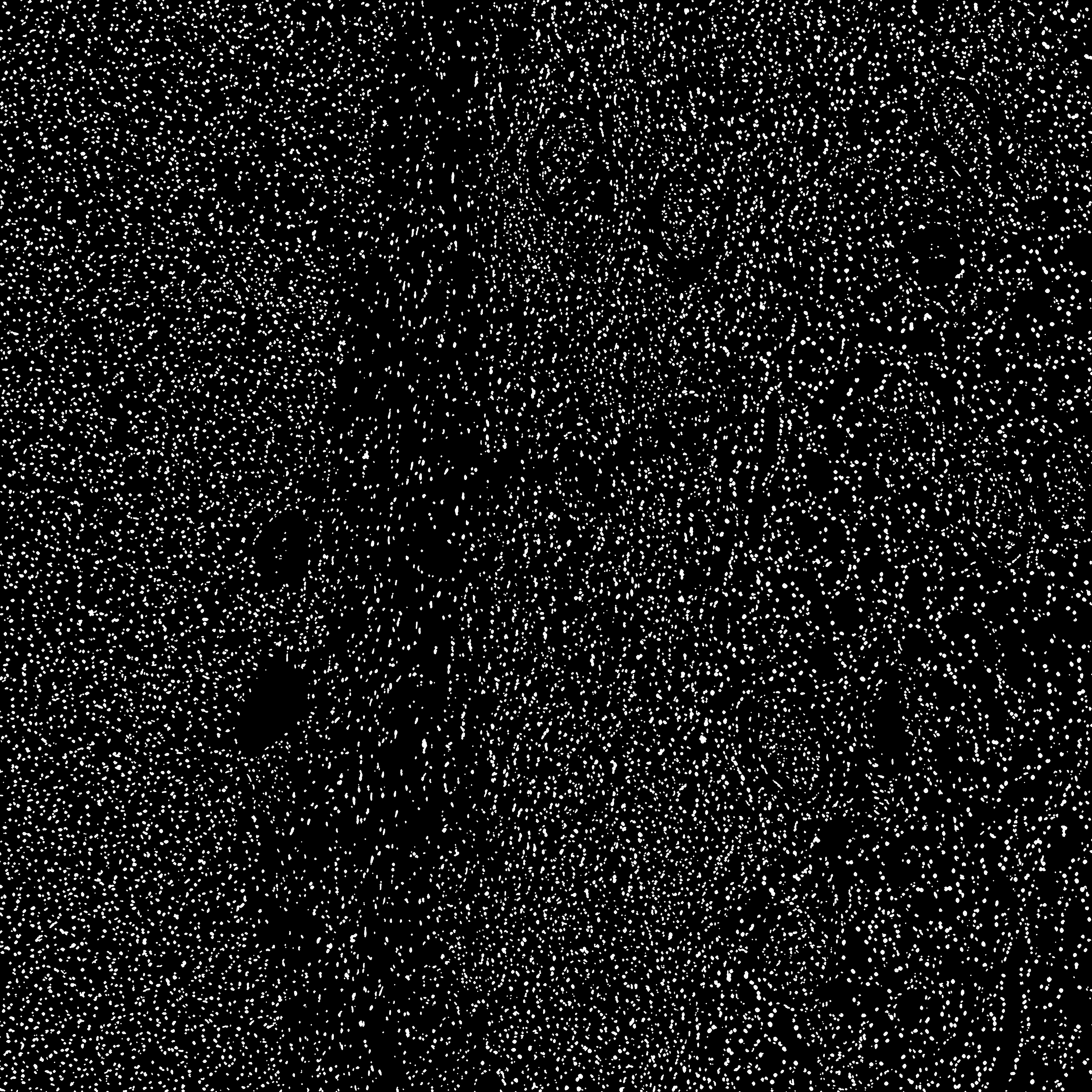
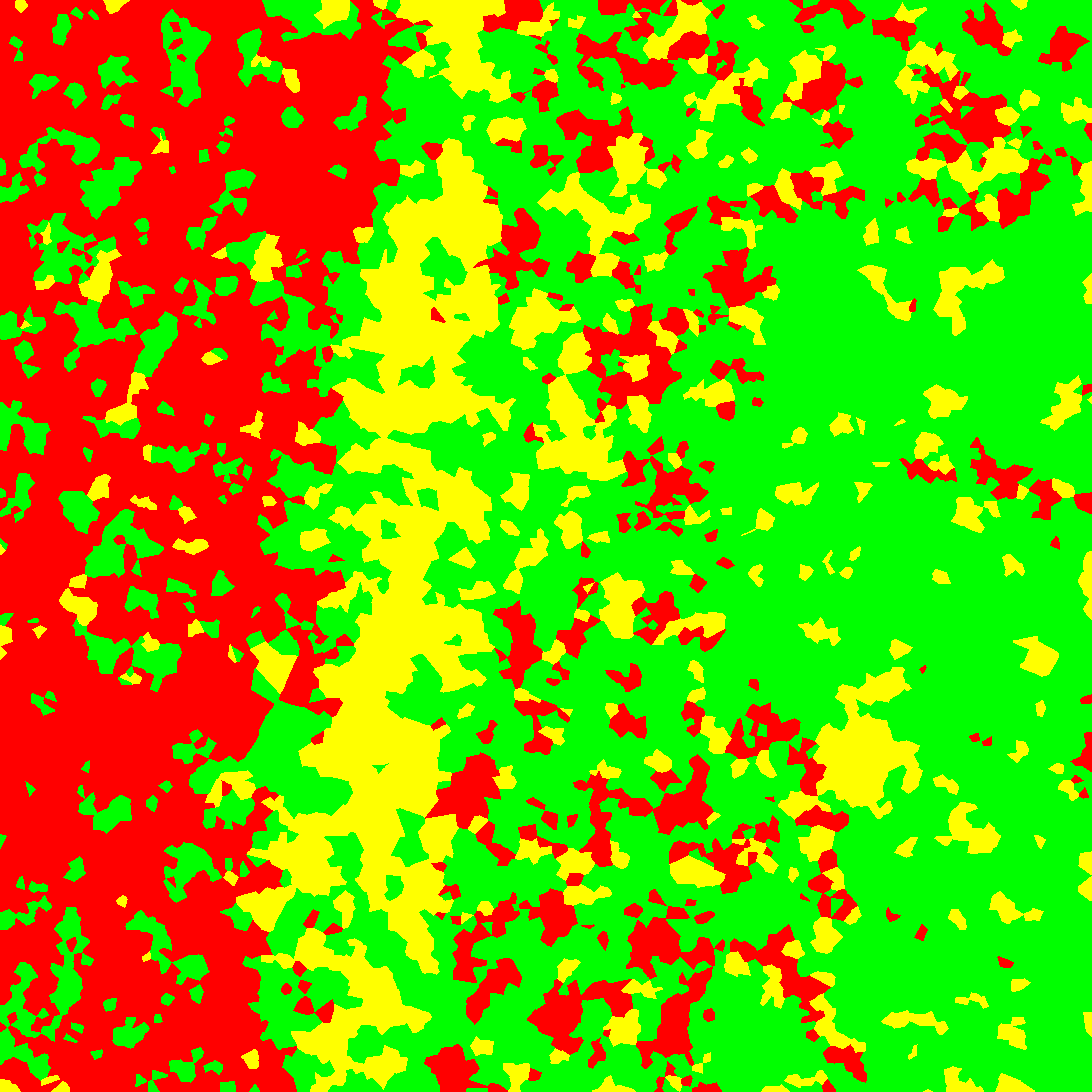
7.11 Toward a numerical BANFF
Participants: Sonia Ciuffreda, Xavier Descombes.
This work was carried out in collaboration with Francesco Ponzio from Politechnico di Torino (Italy), Damien Ambrosetti and Giorgio Toni from Nice CHU.
The Banff classification is used by pathologists for the evaluation and monitoring of kidney implants. This classification, in association with other biological and clinical elements, makes it possible to diagnose rejection and estimate the prognosis, through the analysis of different lesions in the 4 compartments (glomeruli, tubules, interstitium and vessels). However, the numerous classification criteria and their quantification, in addition to the very time-consuming nature of their evaluation, can present problems of inter- and intra-individual reproducibility, hence the relevance of automating this evaluation. We propose an analysis methodology for this automatic evaluation. The first step is to identify and separate the different compartments. Secondly, each compartment will be analyzed individually. In this first work, the lumen areas are identified by thresholding followed by mathematical morphology operators. These areas correspond either to tubules or to vessels. To classify them we use nuclear characteristics in the neighborhood of each lumen. We therefore detect the nuclei using a shape dictionary. Each nucleus is associated with a lumen by minimizing the distance. The lumens are then classified as tubule or vessel by an SVM algorithm based on geometric parameters of area, number and shape of nuclei (see Figure 11).
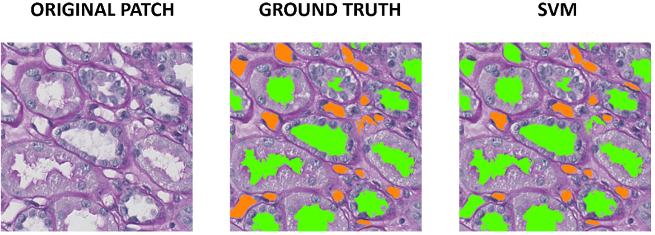
Lumen classification in tubule (green) vs vessel (orange)
7.12 Glomeruli detection and grading
Participants: Mohamad Mohamad, Xavier Descombes.
This work was carried out in collaboration with Francesco Ponzio from Politechnico di Torino (Italy), Damien Ambrosetti and Giorgio Toni from Nice CHU.
Type I diabet may lead to kydney pathology consisting of a degradation of glomeruli. In this project we applied two CNN models to detect glomeruli from histopathological images (Faster R-CNN) and grade them into sane, mild, severe or necrosis stages (see Figure 12). We tested the model on two separate datasets: one from Paris and another assembled using data from Nice. The model performed well, with the majority of errors falling within adjacent classes. Although it exhibited comparatively less proficiency on the samples from Paris (as it had not encountered any data from this center during training), it still demonstrated reasonable performance. Notably, most errors were confined to adjacent classes, typically associated with ambiguous samples. We also performed an extensive hyperparameter optimization. This involved experimenting with various architectures, adjusting parameters, and implementing advanced augmentations inspired by the circular shape of glomeruli. These attempts were undertaken in an effort to balance our training dataset and mitigate overfitting.
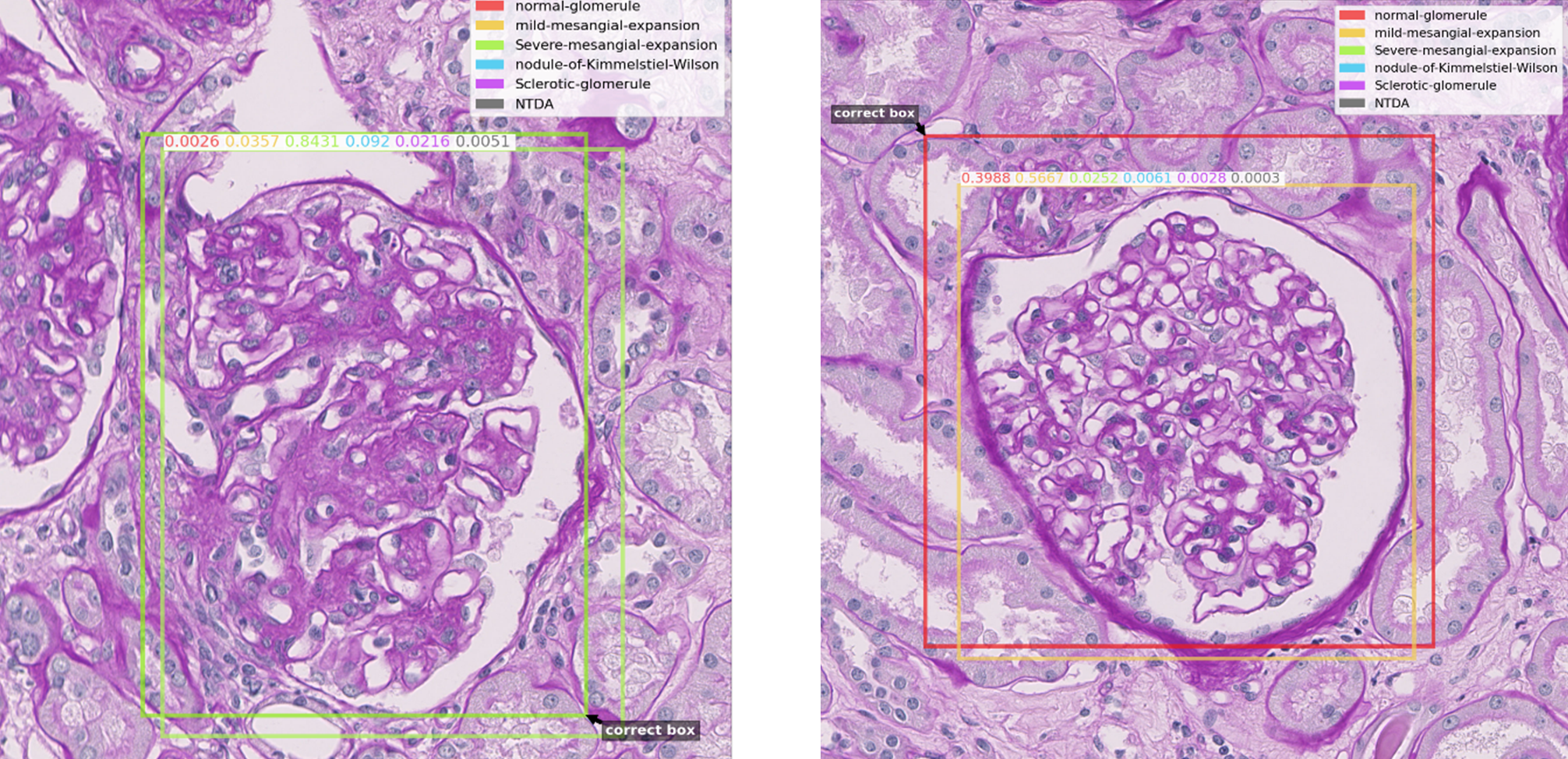
Detection label and probabilities compared to the ground truth: correct grading (left) and adjacent class error (right)
7.13 Reinforcement learning for analysis histopathological images
Participants: Mohamad Mohamad, Xavier Descombes.
This work was carried out in collaboration with Francesco Ponzio from Politechnico di Torino (Italy), Damien Ambrosetti from Nice CHU and Nicolas Pote from Bichat hospital.
Reinforcement learning (RL) is one of the machine learning paradigms, alongside supervised and unsupervised learning, where an agent learns from its direct interaction and manipulation of its environment through a set of actions. The agent acts based on observations known as states, and it learns using a reward signal received from the environment. We aimed to derive a RL framework to address histopathological image analysis tasks. This will be exemplified on kidney and lung cancer datasets.
The main challenges present in RL, unlike other paradigms, include the concept of delayed rewards and the necessity for exploration (where the data observed depends on the agent's trajectory) ...Our objective is to train an agent to perform diagnosis on whole slide images, similar to how pathologists do it: by zooming in and out, moving around and select patches/patterns that characterize specific tumor. Throughout the first three months of this project, we conducted an in-depth literature review in the following categories:
- RL algorithms,
- deep RL algorithms, inspired by both Q-value and Policy-gradient approaches,
- Intrinsic motivation and exploration techniques, and
- RL for medical images and computer vision.
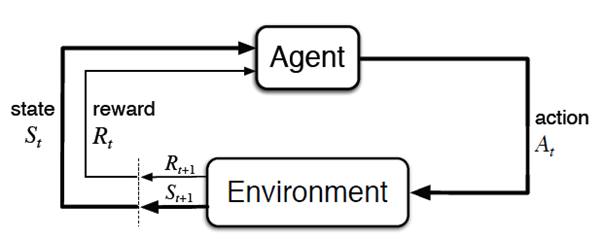
RL paradigm.
7.14 A multi-tasks cycle-GANs architecture for cells segmentation
Participants: Imane Lboukili, Xavier Descombes, Georgios Stamatas [Johnson & Johnson Consumer Health R&D].
The study of Reflectance Confocal Microscopy (RCM) images provides information on epidermal structure, a key to skin health and barrier which changes in each epidermal layer, with age and with certain skin conditions. RCM can also reveal dynamic changes to the epidermis in response to stimuli, as it enables repetitive sampling of the skin without damaging the tissue. Studying RCM images requires manual identification of each cell to derive its geometrical and topological characteristics, which is time-consuming and subject to expert interpretation. More insights could be derived from these data if not for the tediousness of their manual segmentation, highlighting the need for an automated cell identification method. We have previously developed a new unsupervised approach based on multi-task learning to identify cells on RCM images. This approach consists of a dual-task Cycle Generative Adversarial Networks model, where the first task learns RCM images noise and/or texture model from the initial image, while the second task learns the epidermis structure from a Gabor filtering, thus allowing us to denoise RCM images while keeping the position and integrity of the membranes. To our knowledge, this is the first time a Cycle GAN has been embedded in a multi-task learning model. We have started exploring the use of this unsupervised method on images acquired using different imaging modalities and representing different tissues without retraining (histology images, cell culture images, mass spectroscopy images, and fluorescence microscopy images). Indeed, multi-task approaches tend to be less data dependent, and therefore more easily transposable to other types of data not included in the training of the approach. We developed 3 iterations of DermoGAN depending on the type of cell organization (confluent cells, non-confluent cells, and histology images), considering alternatives to the Gabor filter and to the simulated expected results, for example using Canny filter and a Marked Point Process (see Figure 14). We theorize that the performance of DermoGAN is dependent on the cell organization visible in the images used in the training, not on the imaging modality or tissue.
An international patent has been submitted for this work.
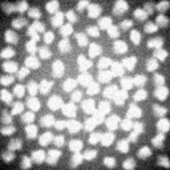
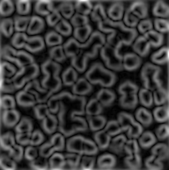
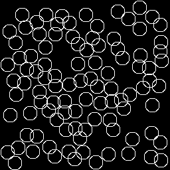
7.15 Machine learning for organoid phenotyping
Participants: Alexandre Martin, Xavier Descombes, Stephan Clavel [IPMC, Sophia Antipolis].
The aim of this work is to built a phenotypic map of organoids. We model an organoid as a 3D shape containing several layers of cells composed of 2D point patterns on the sphere. Our goal is to classify organoids with respect to these three components.
We propose an architecture for classifying organoids (clusters of stem cells, cf Figure 15) developed in the presence of endocrine disruptors. First, we proposed a simplified organoid model, from which we generated synthetic datasets composed of point patterns on the sphere defining a graph using the Voronoi representation. We carried out a comparative study between a classification method based on Deep Learning for graphs, and a method based on spatial statistics. We thus showed that the Deep Learning approach was at least as effective and more robust to noise than the other. We then developed an algorithm allowing the nuclei detection of organoids that have been cultured in the laboratory. In order to enable the use of Deep Learning architectures from a limited number of organoids, we used Data Augmentation techniques. We are currently developing two types of architecture (one based on convolution networks, the other on layers dedicated to graphs), to obtain a baseline. With the aim of ultimately proposing a robust and interpretable model, we seek to enrich it with a part based on the 3D analysis of the shape of the organoid, in particular via the decomposition of these shapes into spherical harmonics.

Examples of prostatic organoid phenotypes
7.16 Detection and Segmentation of Fibronectin Structures in the Tumour Extracellular Matrix
Participants: Faisal Jayousi, Xavier Descombes, Laure Blanc-Féraud, Ellen Van Obberghen-Schilling, Emmanuel Bouilhol, Anne Sudaka [CAL, Nice].
This work is on the detection and characterization of Fibronectin (FN) structures found in the tumour extracellular matrix (ECM). Three structures were identified in our multiplex fluorescent images: aggregates, representing accumulations of insoluble, non-fibrillar FN; FN fibres; and tumour cells expressing cytokeratin (Figure 16).
.png)
Fluorescence Microscopy Image of FN distribution in an invasive squamous cell carcinoma of the tongue. (
A greedy algorithm, leveraging the geometric assumptions we have about the aggregates — specifically, that they exhibit an elliptical resemblance — has been developed and tested. This algorithm is grounded in a marked point process model proposed by X. Descombes 4. Additionally, a modified Gabor kernel 31 has been introduced to enhance fibre detection, minimizing the blurring effect. Classical image processing techniques were applied to achieve an accurate segmentation of tumour cells (Figure 17). Ongoing efforts are directed towards characterizing the diverse components of the ECM using this segmentation.
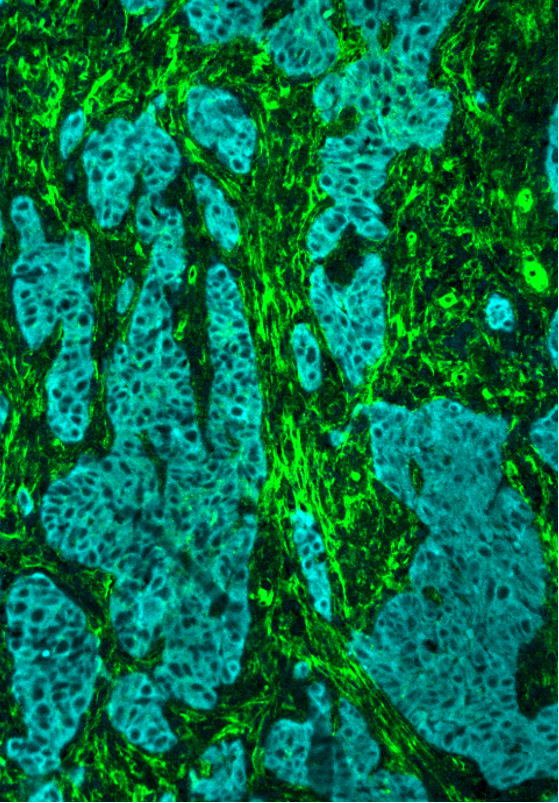
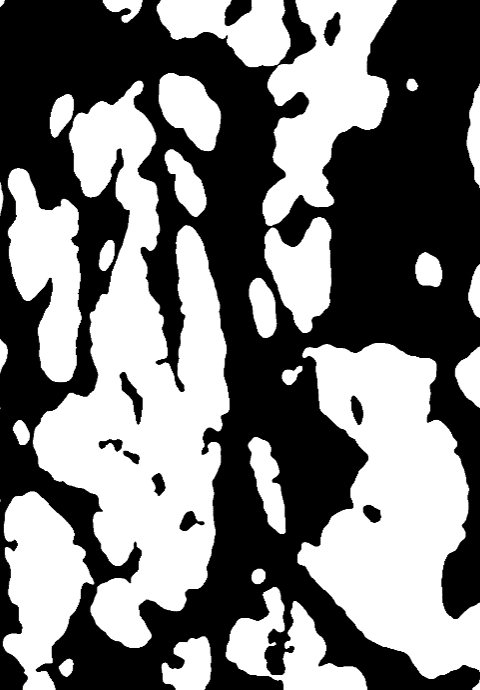
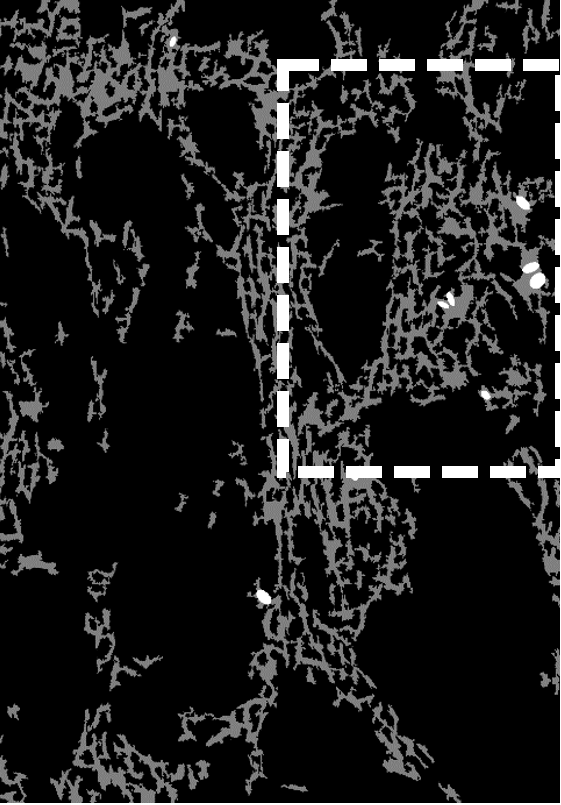
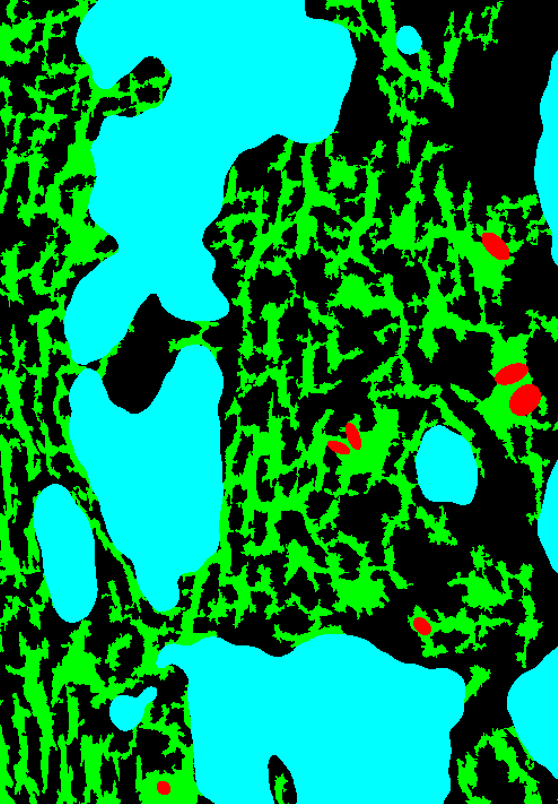
Output of the image processing pipeline. (a) Input image. (b) Tumour mask obtained through Otsu's method and connected components analysis. (c) Aggregates detected using our algorithm, with enhanced fibres using the modified Gabor kernel. (d) Final output with a zoomed view on the dotted rectangle of (c).
7.17 Quantitative analysis of the tumor extracellular matrix
Participants: Emmanuel Bouilhol, Ellen Van Obberghen-Schilling, Xavier Descombes, Laure Blanc-Féraud, Anne Sudaka [CAL, Nice].
The research activities of this project focus on the analysis of microscopy images of the extracellular matrix (ECM) in the microenvironment of head and neck cancer tumors. This project builds on previous works carried out within the team, through collaborations with clinical partners (CAL, Unicancer, Head and Neck Group) and with Fabienne Anjuère, immunologist at IPMC. This research, carried out as part of the TOPNIVO and CHECK-UP clinical trials, has led to the development of a pipeline for acquiring multiplexed immunofluorescence and HES-stained histological images. In this context, some 6,000 multispectral images and 350 HES (Whole slide images) have been acquired.
The current work focuses on analyzing the topological and geometrical changes in fiber networks of the tumor ECM (Extra-Cellular Matrix) using the developed image analysis pipelines and algorithms. We are also working on characterizing the organization of ECM proteins and finding structural biomarkers to develop predictive models for patient response to immunotherapy, This can be summarized in two main parts (see figure 18):
1- The development of deep learning algorithms for image processing (signal extraction, segmentation, and classification of biological structures of interest), which requires both expert annotation and software development so that these images, which can weigh several gigabytes, can be processed by Deep Learning.
2 - The implementation of quantitative statistics to search for spatial signatures or biomarkers in the images, and more specifically in the extracellular matrix, in order to predict patient response to immunotherapy, as well as to provide insights on the functioning of immunosuppressive functions.
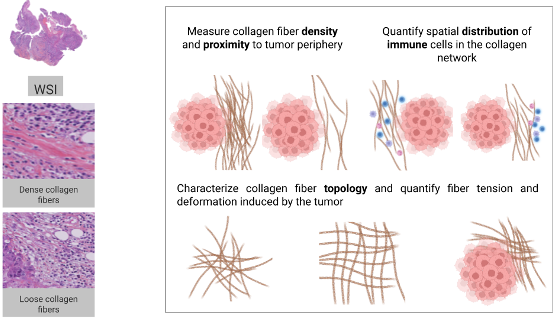
7.18 Heterochrony of cell division in ascidian
Participants: Grégoire Malandain, Rouaa Hoblos, Killian Biasuz [CRBM, Montpellier], Patrick Lemaire [CRBM, Montpellier].
It is admitted that ascidian exhibited a stereotyped development in the first stages of their embryogenesis 30. Moreover, we demonstrated that the developmental speed (characterized as the derivative of the count of cells) is identical from one embryo to the other (up to a scaling factor, the normal developmental speed being linearly correlated to the medium temperature), suggesting that the cell division rate is identical in a population of normally developing embryos (see Figure 19 left and middle).
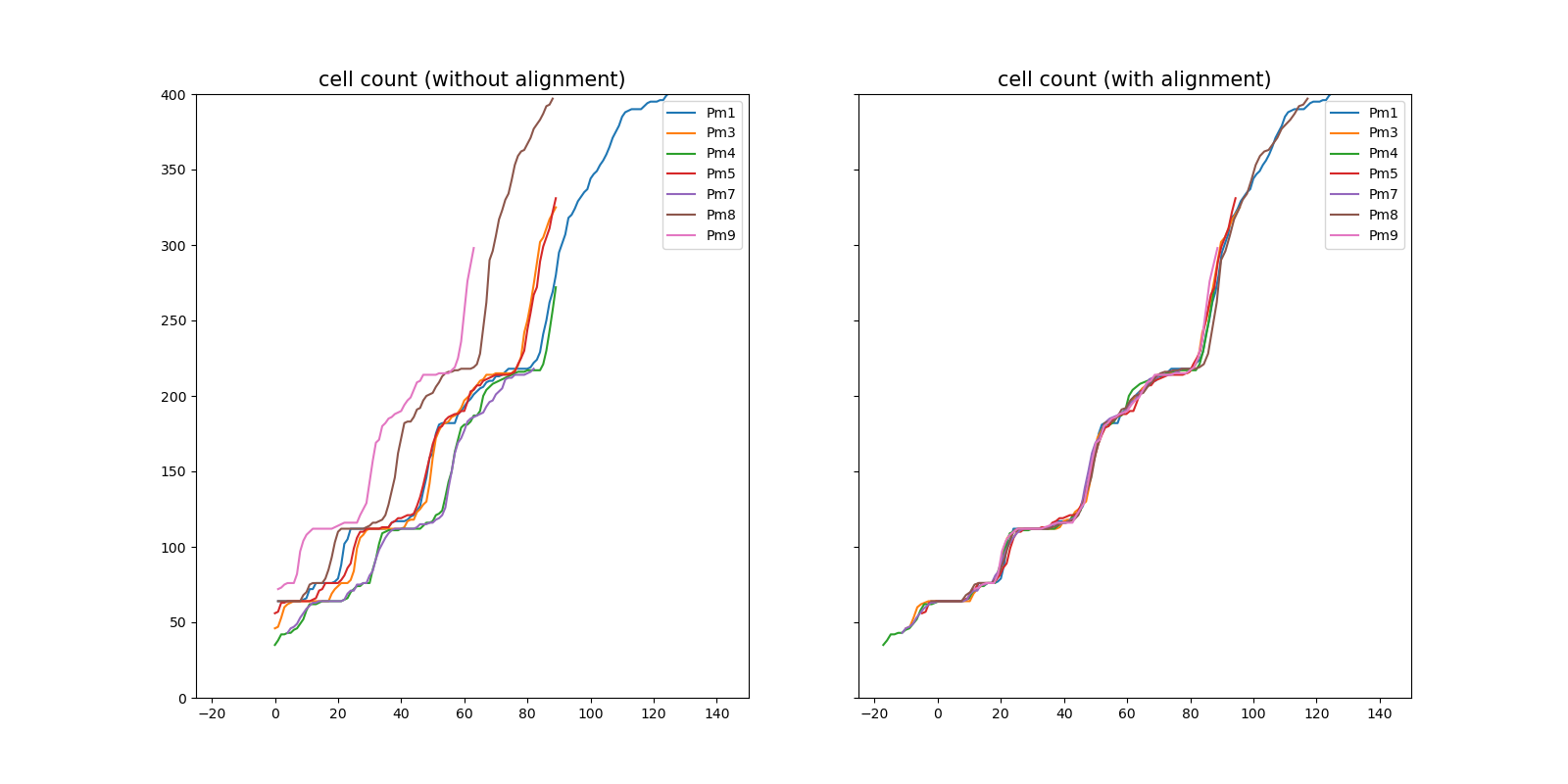
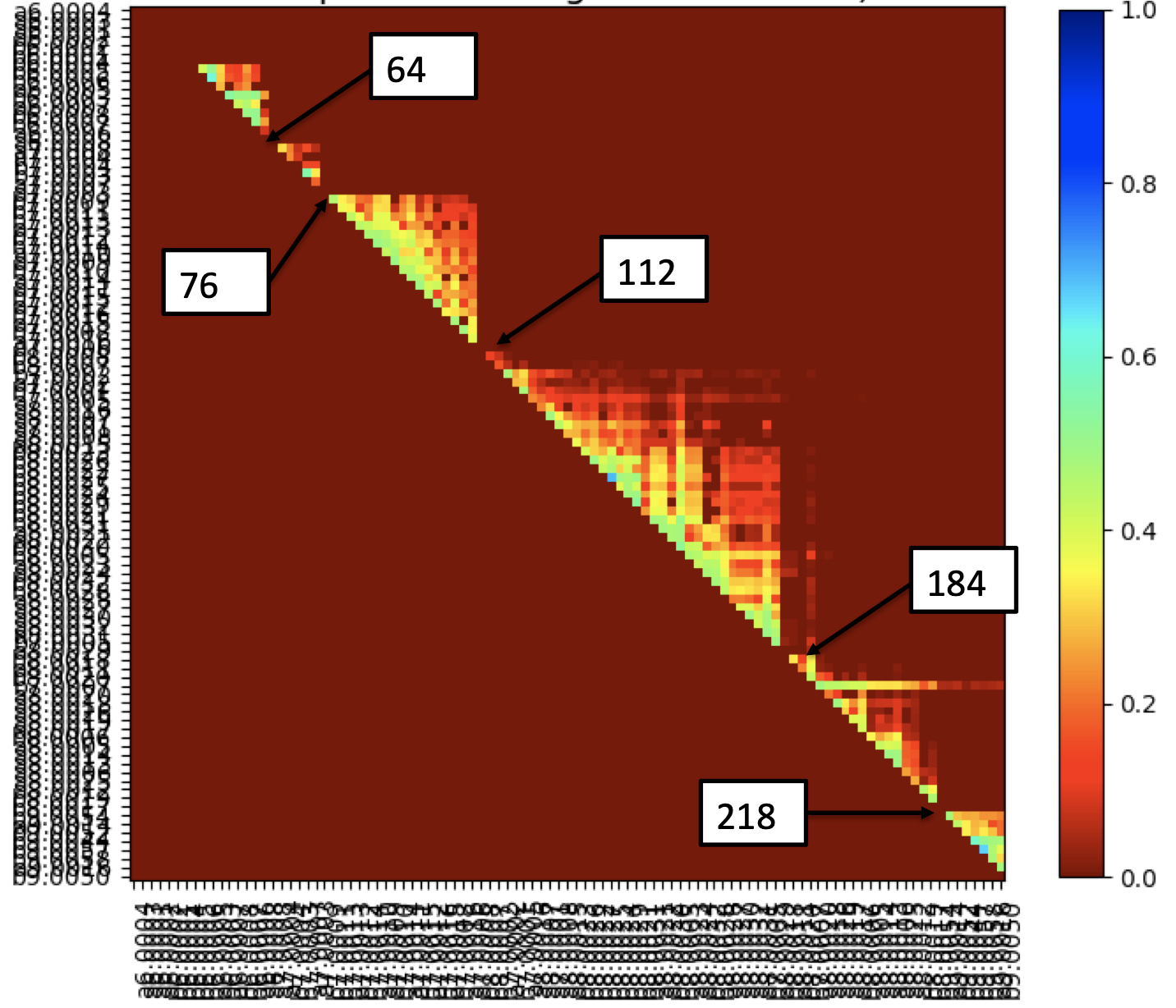
Left: embryo cell counts along acquisition time. Middle: embryo cell counts along acquisition time after linear warping. Left: confusion matrix of division occurrences.
Thanks to this consistent cell division rate amongst embryos, we can study whether the order of cell divisions is identical across embryos. For this purpose, we ordered the cells with respect to their average division time and estimate the probability that a cell with a late average division divides before a cell with an earlier division (Figure 19 right). This emphasizes the different rounds of divisions that succeed during the embryo development, with still phases at the stages of 64, 76, 112, 184 and 218 cells. It also reveals that the closer the average division times are, the higher the probability that the late one occurs before the early one.
7.19 Geometric variability of cell division in ascidian
Participants: Haydar Jammoul, Grégoire Malandain, Killian Biasuz [CRBM, Montpellier], Patrick Lemaire [CRBM, Montpellier].
Ascidian exhibited a stereotyped development in the first stages of their embryogenesis 30. Unpublished results demonstrate a strong reproducibility of cell naming with respect to geometrical (cell position), and topological (cell neighborhood) considerations, but have also revealed that some cells exhibit different division orientation patterns.
In this work, we aim at studying the variability of cell division, namely whether a cell division has different orientation patterns across embryos.
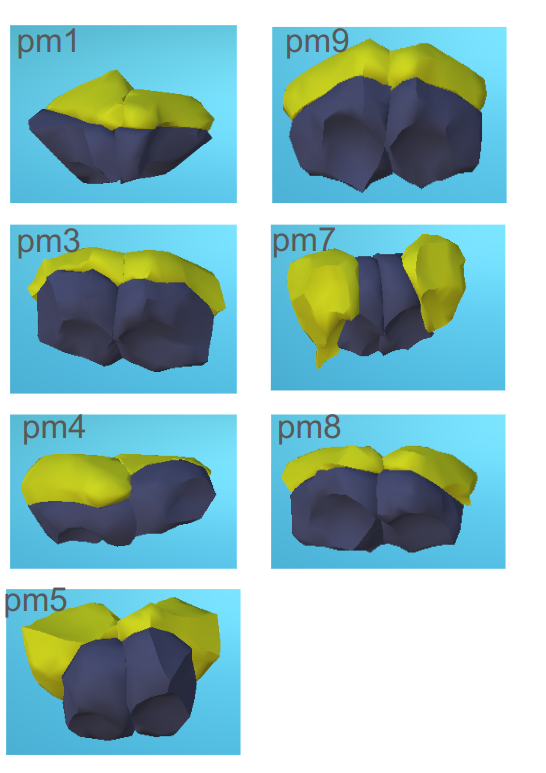
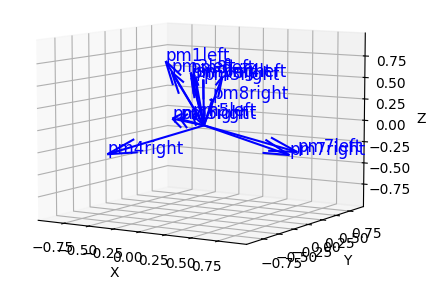
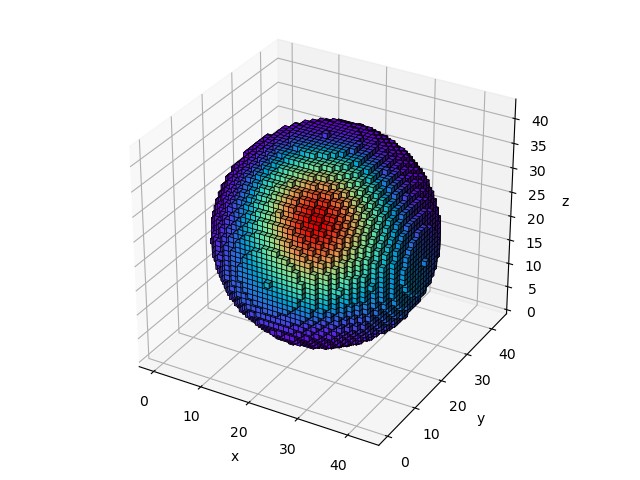
Left: snapshots of b7.2 cell divisions in differents embryos. Middle: cell division directions in a common referential. Right: division direction distribution.
We study each cell division individually. First, all embryos are registered against one common reference (one of the embryo at hand), so they share a common geometric referential. Having defined the division orientation as the direction between the centers of mass of the two daughter cells, we collect the direction and compute a kernel-based direction density function (on the 3D sphere), see Figure 20. Extracting the number of modes of this density function together with the number of samples participating to each mode is a powerful means to point out cells whose division is more likely to exhibit different orientation patterns, see Figure 21.
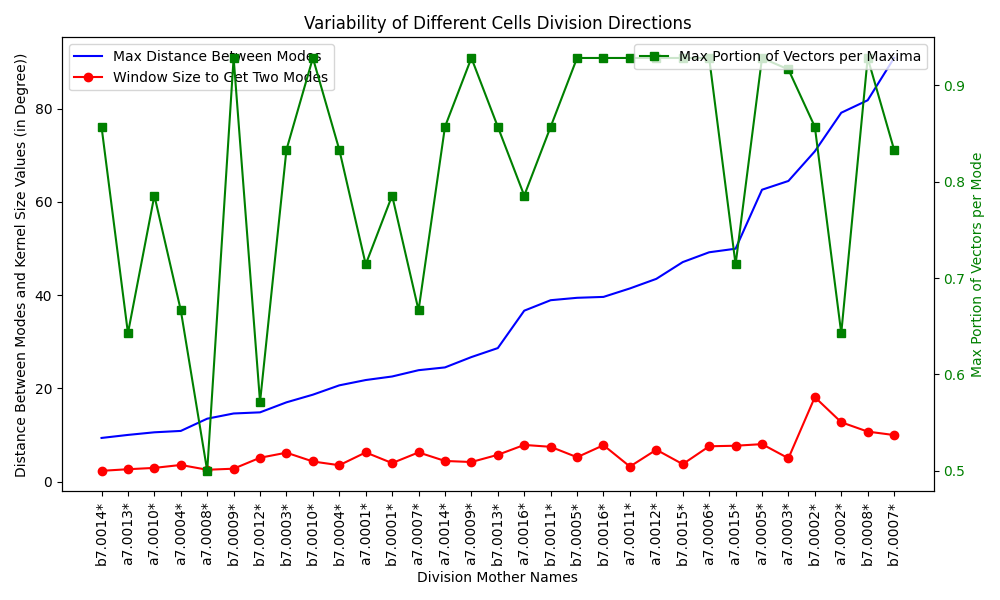
Assessment of cell division variability for the cells of the 7th generation. Blue: distance between the two detected modes. Red: kernel size to get a bimodal distribution. Green: balance of samples between the two detected modes.
8 Bilateral contracts and grants with industry
8.1 Bilateral contracts with industry
Participants: Imane Lboukili, Xavier Descombes.
- Johnson & Johnson, Santé Beauté France, Paris: 36-month companion contract (Jan 2021 – Dec 2023) for the PhD thesis of Imane Lboukili.
9 Partnerships and cooperations
9.1 International initiatives
9.1.1 Participation in other International Programs
CAPES COFECUB ROAPI
Participants: Laure Blanc-Féraud.
This project is in collaboration with University of Campinas, Brasil with Joao Romano and Adilson Chinatto and with Satie Lab of University Paris-Saclay with Pascal Larzabal. The aim of this project is the application of sparse methods in antenna processing which has been proving to be a promising alternative to standard methods. It needs to be studied in greater depth to improve its implementation and quantify its benefits. A difficult step in this approach is the tuning of the regularizing parameter which compounds the weight between the data term and the sparse regularizing term. We have worked on a process adapted to the problem of direction of arrival (DOA) in antenna communications which gives an estimation of the regularizing parameter suitable for the DOA problem.
9.1.2 Visits of international scientists
Vishal Monga
-
Status
senior researcher
-
Institution of origin:
PennState College of Engineering
-
Country:
USA
-
Dates:
April 6-7th, 2023
- Context of the visit:
-
Mobility program/type of mobility:
seminar, visit of the Morpheme project
Romain Petit
-
Status
Post-doctoral researcher
-
Institution of origin:
University of Genoa
-
Country:
USA
-
Dates:
April 5-6th, 2023
-
Mobility program/type of mobility:
seminar, visit of the Morpheme project
9.1.3 Visits to international teams
Research stays abroad
- Within the H2020 RISE NoMADS project, Luca Calatroni visited the MaLGa research center (Genoa, Italy) for 2 weeks (February, May) and the Mathematics Department at the University of Cambridge (UK) for two weeks (April).
9.2 European initiatives
9.2.1 H2020 RISE project NoMADS
Participants: Luca Calatroni.
The project NoMADS focuses on data processing and analysis techniques which can feature potentially very complex, nonlocal, relationships within the data. In this context, methodologies such as spectral clustering, graph partitioning, and convolutional neural networks have gained increasing attention in computer science and engineering within the last years, mainly from a combinatorial point of view. However, the use of nonlocal methods is often still restricted to academic pet projects. There is a large gap between the academic theories for nonlocal methods and their practical application to real-world problems. The reason these methods work so well in practice is far from fully understood. Our aim is to bring together a strong international group of researchers from mathematics (applied and computational analysis, statistics, and optimization), computer vision, biomedical imaging, and remote sensing, to fill the current gaps between theory and applications of nonlocal methods. We will study discrete and continuous limits of nonlocal models by means of mathematical analysis and optimization techniques, resulting in investigations on scale-independent properties of such methods, such as imposed smoothness of these models and their stability to noisy input data, as well as the development of resolution-independent, efficient and reliable computational techniques which scale well with the size of the input data. As an overarching applied theme we focus in particular on image data arising in biology and medicine, which offers a rich playground for structured data processing and has direct impact on society, as well as discrete point clouds, which represent an ambitious target for unstructured data processing. Our long-term vision is to discover fundamental mathematical principles for the characterization of nonlocal operators, the development of new robust and efficient algorithms, and the implementation of those in high quality software products for real-world application.
9.3 National initiatives
9.3.1 Labex SIGNALIFE
The Morpheme team belongs to the Labex (Laboratory of Excellence) Signalife. This Labex embeds four biology institutes in Nice, Sophia Antipolis and two Inria teams.
9.3.2 ANR JCJC TASKABILE
Participants: Luca Calatroni [PI].
This project is in collaboration with Alessandro Lanza (University of Bologna, IT) and Thomas Moreau (Inria Saclay, FR). In this project we propose to improve upon the intrinsic specificity and rigidity of standard bilevel learning approaches by means of a novel task-adapted highly-flexible modeling. To mitigate the computational efforts required for solving bilevel problems numerically, tailored inexact and stochastic optimization schemes will be studied. The following questions will be addressed:
- The definition of novel, possibly non-smooth and non-convex task-adapted (not only reconstruction-based) quality measures inspired by empirical evaluation metrics and visual perception;
- The use of flexible statistical distributions as a more plausible prior model describing image statistics and their comparison with highly-generalizable deep architectures;
- The use of inexact and stochastic first-order optimization algorithms computing solutions of bilevel schemes with reduced computational efforts and controlled accuracy.
The project features an international consolidated skilled team of researchers with strong expertise in applied imaging inverse problems, non-smooth & non-convex optimization and statistical image processing.
9.3.3 ANR PRC MICROBLIND
Participants: Luca Calatroni, Laure Blanc-Féraud.
This project is a collaborative project led by Pierre Weiss (IMT, Toulouse)[PI].
Several recent revolutions in imaging rely on numerical computations. One can think of single molecule localization microscopy (Nobel Prize 2014) or cryo-electron microscopy (Nobel Prize 2017). What they have in common is the need to perform prior mathematical modeling and calibration of the system. Although they have made it possible to observe phenomena that were previously out of reach, their expansion is currently limited by an important problem: it is difficult to precisely control the imaging conditions (e.g. temperatures, wavelengths, refractive indices). This results in modeling errors that can have disastrous repercussions on the quality of the images produced. Thus, these technologies are currently reserved for a handful of research centers possessing state-of-the-art equipment and considerable interdisciplinary experience. The objective of this project is to bring new theoretical and numerical solutions to overcome these difficulties, and then to apply them to different optical microscopes. This should allow to democratize their use, to reduce their cost and the preparation time of the experiments.
The central idea is to characterize a measurement device, not by a single operator (e.g. a convolution), but by a small dimensional family allowing to model all possible states of the system. To our knowledge, this idea has been very little explored so far and opens many difficult questions: how to evaluate this family experimentally and numerically? How to identify the state of the system from indirect noisy observations? How to exploit this information to reconstruct images in short computing times? We have begun to explore these questions in recent works and wish to continue this effort using tools from optimization, harmonic analysis, probability and statistics, algebraic geometry, machine learning and massively parallel computing. We hope to make significant advances in the field of blind inverse problems. We will validate them on photonic microscopy problems in collaboration with opticians, responsible for two microscopy platforms in Nice and Toulouse. This will allow us to obtain direct feedback for real problems in biology. We will particularly study the problems of super-resolution by single molecule, multi-focal localization and blind structured illumination. Moreover, several companies in the Toulouse area (INNOPSYS, IMACTIV-3D, AGENIUM), will provide us with data from their microscopes (line scanning microscope, light sheet fluorescence microscope), which will ensure direct transfers to industry.
9.3.4 ANR MORPHEUS
Participants: Xavier Descombes [PI], Grégoire Malandain, Alexandre Martin.
In this project we propose to use the cutting-edge organoid technology to test the toxicity of endocrine disruptors (EDCs) on human organs. The aim is to develop computational tools and models to allow the use of organoid technology for EDC toxicity testing. The project is thus divided in two main objectives: to build up and analyze a phenotypic landscape of EDC effect on organoid and to develop explicative or predictive models for their growth. The first goal is to define and construct a phenotypic map of organoids, modeled as graphs (the nodes representing the cells and edges adjacency between them) for classifying EDCs families. The second is to classify organoid growth trajectories on this map. We will consider two organoid models, gastruloids and prostate organoids. To derive the phenotypic map we will combine a graph representation and a deep learning approach. The deep learning approach will be considered for its discriminating properties whereas a correspondence between the bottleneck layer of the chosen neural network and the stratified graph space will bring some explicability to the derived classification.
This 4-years project started in november 2021 and is leaded by X. Descombes. It involves 3 groups : C3M (S. Clavel, Nice), Metatox, Inserm (X. Coumoul, Paris) and Morpheme.
9.3.5 ANR Cell Whisper
Participants: Grégoire Malandain.
Successful embryogenesis requires the differentiation of the correct cell types, in defined numbers and in appropriate positions. In most cases, decisions taken by individual cells are instructed by signals emitted by their neighbors. A surprisingly small set of signalling pathways is used for this purpose. The FGF/Ras/ERK pathway is one of these and mutations in some of its individual components cause a class of human developmental syndromes, the RASopathies. Our current knowledge of this pathway is, however, mostly static. We lack an integrated understanding of its spatio-temporal dynamics and we can imperfectly explain its highly non-linear response to a graded increase in input stimulus.
This systems biology project combines advanced quantitative live imaging, pharmacological/optogenetics perturbations and computational modeling to address 3 major unanswered questions, each corresponding to a specific aim:
- Aim 1: What is the spatio-temporal dynamic of intracellular signal transduction in response to FGF (Fibroblast Growth Factor) ?
- Aim 2: What is the molecular basis of the switch-like response to graded extracellular signals?
- Aim 3: Can the results be integrated into a predictive computational model of the pathway?
Through this approach, in a simplified model system, we hope to gain an integrated view of the spatio-temporal dynamics of this pathway and of its robustness to parameter variations. Participants are CRBM (Montpellier), LIRMM (Montpellier), MOSAIC (INRIA Lyon) and Morpheme.
9.3.6 Targeted Project Filling Gaps
Participants: Grégoire Malandain.
This targeted project, "Filling the gaps between scales to understand biomass properties", is issued from the PEPR B-Best.
The architecture of biomass is highly complex and can be defined as a continuum of length-scales from molecules to particles, including polymers, nano-structures, assemblies, cells, and/or tissues. These scales are strongly interconnected and reflect not only chemical and structural properties of biomass but most importantly their reactivity to transformation processes such as chemical, physical, mechanical or biological reactions.
The goal of this project is to identify and quantify markers at different scales in order to be able to propose a generic model (at least for each biomass type considered) that describes and predict their properties and possibly their reactivity (at the chemical, biological, physical levels), with a focus on lignocellulosic and algal biomass. Morpheme team will address the image analysis issues.
9.3.7 Inria Large-scale initiative Naviscope
Participants: Grégoire Malandain.
This action gathers the expertise of seven Inria research teams (Aviz, Beagle, Hybrid, Morpheme, Parietal, Serpico and Mosaic) and other groups (MaIAGE, INRAE, Jouy-en-Josas and UMR 144, Institut Curie Paris) and aims at developing original and cutting-edge visualization and navigation methods to assist scientists, enabling semi-automatic analysis, manipulation, and investigation of temporal series of multi-valued volumetric images, with a strong focus on live cell imaging and microscopy application domains. More precisely, the three following challenges will be addressed:
- Novel machine learning methods able to detect the main regions of interest, and automatic quantification of sparse sets of molecular interactions and cell processes during navigation to save memory and computational resources.
- Novel visualization methods able to encode 3D motion/deformation vectors and dynamics features with color/texture-based and non-sub-resolved representations, abstractions, and discretization, as used to show 2D motion and deformation vectors and patterns.
- Effective machine learning-driven navigation and interaction techniques for complex functional 3D+Time data enabling the analysis of sparse sets of localized intra-cellular events and cell processes (migration, division, etc.).
9.3.8 3IA Senior chair, "Imaging for Biology"
Participants: Laure Blanc-Féraud.
Recent advances in microscope technology provide outstanding images that allow biologists to address fundamental questions. This project aims at developing new AI methods and algorithms for (i) novel acquisition setups for super resolution imaging, and (ii) extraction of valuable quantitative information from these large heterogeneous datasets. More precisely we search for biomarkers in multispectral fluorescence images of tumor tissues to predict the response of immunotherapy in head and neck cancers.
9.4 Regional initiatives
9.4.1 Bi-master IDEX JEDI project : NMAC in TiME
Participants: Xavier Descombes, Wassila Khatir.
This project is a collaboration with F. Anjuère from IPMC.
In the context of this proposal, our aim is to quantitatively characterize the neurons and macrophage subsets infiltrating CSCC (Cutaneous Squamous Cell Carcinoma) and their reciprocal interactions. To that end, we will combine an innovative high-dimensional imaging approach, the Imaging Mass Cytometry (IMC) technology enabling the simultaneous detection of 39 protein targets on one tissue section, with the development of a computational pipeline for the quantitative analysis of macrophage, neuron and tumor cell subsets and their reciprocal interactions. This pipeline is a key step for the automatic cell and structure segmentation of all IMC images, for the subsequent quantification of cell-cell and cell-structure interactions. By comparing quantitatively and statistically the features of relapsing and non-relapsing CSCC tumors, using a high-dimensional imaging technology combined with an optimized computational pipeline of analysis, we will be able to identify unappreciated spatial features associated to tumor relapse on a unique tumor tissue section.
10 Dissemination
10.1 Promoting scientific activities
10.1.1 Scientific events: organization
Member of the organizing committees
- Luca Calatroni was co-organizer of the IX conference on Scale Space & Variational Methods in computer vision (May 22-25, 2023, S. Margherita di Pula, Italy), the conference 30 years of mathematics for optical imaging (September 25-27, Marseille, France) and the worskhop Artificial intelligence & applied mathematics for history & archaeology (November 27-28, Nice, France).
- Luca Calatroni was co-organizer of the Erasmus+ BIP PhD school “Advanced methods for mathematical image analysis” (Bologna, January 2023).
- Luca Calatroni is co-organizer of the monthly seminar series on optimization (OptAzur) starting from September 2023.
10.1.2 Scientific events: selection
Member of the conference program committees
- Luca Calatroni was program committee member of the XI conference on Mathematics and Image Analysis (MIA), Berlin, DE.
- Xavier Descombes was member of the scientific committee of IABM 2023 (Colloque Français d'Intelligence Artificielle en Imagerie Biomédicale)
Reviewer
- Grégoire Malandain has been reviewer for the IEEE ISBI conference (International Symposium on Biomedical Imaging).
- Laure Blanc-Féraud has been reviewer for IEEE ICASSP, IEEE ISBI, IEEE ICIP conferences.
- Eric Debvreuve has been reviewer for IEEE ICIP Conference.
10.1.3 Journal
Member of the editorial boards
- Associate Editor, Frontiers in biomedical imaging, Thomas Boudier.
- Associate Editor, Journal of Mathematical Imaging and Vision, Inverse Problems, Luca Calatroni.
- Series Editor of the Cambridge University Press series Elements in Non-Local Data Interactions: Foundations and Applications, Luca Calatroni
- Area Chair, IEEE Signal Processing Magazine, Laure Blanc-Féraud.
- Associate Editor encyclopedia SCIENCES ISTE/WILEY for the image domain, Laure Blanc-Féraud.
Reviewer - reviewing activities
- SIAM Journal on Imaging Sciences, Inverse Problems, COAP, SIAM Journal on Optimization, IEEE TCI, IEEE TSP, Journal of Machine Learning Research, IEEE Signal Processing Magazine, IEEE ICASSP, GRETSI, SSVM, Luca Calatroni.
- Nature Communications, BMC bioinformatics, Thomas Boudier.
- Biological Imaging, IEEE Open Journal of Signal Processing, Medical Image Analysis (Media), Journal of Machine Learning Research, Eric Debreuve.
10.1.4 Invited talks
- IPMC, "Tools for Automation in Image Analysis", Thomas Boudier.
- ICMS Workshop, Interfacing Bayesian Statistics, Machine Learning, Applied Analysis, and Blind and Semi-Blind Imaging Inverse Problems, Edinburgh, UK, Janvier 24-26, 2023, Laure Blanc-Féraud.
- IABM, colloque français d'Intelligence Artificielle en Imagerie Biomédicale, Intitut Curie, Paris, March 30-31, 2023, Laure Blanc-Féraud.
- Assemblée Générale du GdR ISIS, Lyon, May 30th - June 1st, 2023, Laure Blanc-Féraud.
- Workshop ”Learning for Inverse Problems” (LIP), Rome, IT; workshop “Mathematical modeling and Analysis of degradation and restoration in Cultural Heritage” (MACH), Rome, IT; Workshop ”Super-resolution of fluctuation-based fluorescence data via GANs” at MiFoBio 2023, Hyeres, FR, Luca Calatroni.
- SPOT seminars (Toulouse), "Machine Learning and Signal Processing" (MLSP) seminars (ENS Lyon), Applied Mathematics Seminars (UniPv, IT), ModeMat-CIMAT webinars, IIT, Luca Calatroni. molecular microscopy and spectroscopy seminars (Italy), Luca Calatroni.
- Invited talk at symposia at UMI congress (Pisa, IT), ICIAM 2023 congress (Tokyo, Japan), Luca Calatroni.
- BioIAS Workshop, "Shaping tumors and their microenvironment: quantitative characterization of fibronectin architecture", Nice, February 2nd, 2023, Ellen Van Obberghen-Schilling and Xavier Descombes.
- BioIAS Workshop, "Dynamic analysis framework to detect diverging cell responses to cancer drugs in live-cell microscopy, using signal processing and machine learning", Nice, February 2nd, 2023, Jérémie Roux and Eric Debreuve.
- Acad. 4 day, "Caractérisation de l'architecture de la lame basale par analyses mathématiques d'images 3D", Sophia Antipolis, October 6th, 2023, Xavier Descombes (with F. Luton, IPMC).
- ReinCaRe workshop, invited talk on self-supervision for RCC subtyping, May, 10-12 2023, Mohamad Mohamad.
- BioIAS Workshop, "Dynamic analysis framework to detect diverging cell responses to cancer drugs in live-cell microscopy, using signal processing and machine learning", Nice, February 2nd, 2023, Matteo Rauzi and Grégoire Malandain.
- Emmanuel Bouilhol gave talks/posters on November 5-8, 2023 Next generation bioimage analysis workflows hackathon (Zurich), November 22-25, 2023 Sophia Summit 2023, The sixth edition of the international AI conference (Sophia Antipolis), December 1st, 2023 3IA PhD/Postdoctoral Seminar (Sophia Antipolis) and, December 19th, 2023 - 3IA Côte d'Azur Scientific Day (Sophia Antipolis).
10.1.5 Leadership within the scientific community
- IEEE BISP Technical commmittee, head of Publication and Journal Subcommittee, Laure Blanc-Féraud.
- Head of Axis 3 IA for Biology and Bio-inspired IA at 3IA Côte d'Azur, Laure Blanc-Féraud.
- Director of the Italian research group on Mathematics of Imaging and Vision (MIVA) labelled by the Italian Mathematical Union, Luca Calatroni.
- Vice President of ANR CE 45, Xavier Descombes
- IEEE Biomedical Imaging and Image Processing (BIIP) technical committee, Grégoire Malandain.
- Scientific committee of the MATHNUM department of INRAE, Grégoire Malandain
10.1.6 Scientific expertise
- Expert at MENSR for CIR ("Crédits Impôts Recherche) and JEI ("Jeunes Entreprises Innovantes"), Xavier Descombes.
10.1.7 Research administration
- Laure Blanc-Féraud was member of the jury awarding the EUR DS4H theses grants, member of the Scientific and pedagogic committee (CoSP) of EUR DS4H, member of the Scientific committee of 3IA Côte d'Azur Institute, vice-chair of Academy 1 of Idex UCA JEDI, member of "comités de sélection" université Toulouse (Pr 26), Annecy (PR 61), Nice (MCF 61), Caen (PR 61), "comité repyramidage" Toulouse (Pr 61), External member of a selection committee Professor Télécom Paris-Saclay.
- Grégoire Malandain was a member of a INRAE researcher (CRCN) selection committee.
10.2 Teaching - Supervision - Juries
10.2.1 Teaching
- Licence: Florence Besse, Subcellular RNA targeting, 4h, L3, ENS Ulm, France
- Master: Florence Besse, Neurobiologie cellulaire et moléculaire, 6h, M1/2, Université Côte d'Azur, France
- Master: Florence Besse, Outils génétiques pour l'étude des circuits neuronaux, 4h, M1/2, Université Côte d'Azur, France
- Master: Florence Besse, Transport axonal, 2h, M1/2, Université Côte d'Azur, France
- Master: Laure Blanc-Féraud, module Machine Learning for Image processing and module Applied AI of master SD, 12h Eq. TD, Niveau M2, Université Côte d'Azur, France.
- Master: Eric Debreuve, Scientific image processing and Machine learning, Master SVS, 15h EqTD, M1 + M2, Université Côte d'Azur, France
- Master: Xavier Descombes, Traitement d'images, Analyse de données, Techniques avancées de traitement d'images, 10h EqTD, Niveau M2, ISAE, France.
- Master: Xavier Descombes, Machine Learning and Image Processing,Polytech Sophia, master, 9h Eq. Université Côte d'Azur, France.
- Master: Xavier Descombes, Bio-imagerie, master IRIV, 6h EqTD, Niveau M2, Université de Strasbourg, France
- Master: Xavier Descombes, Image Analysis, master GBM, 9h EqTD, Niveau M2, Université Côte d'Azur, France.
- Master: Xavier Descombes, Image processing and Machine learning for Biology, master SVS, 15h Eq.TD, Niveau M2, Université Côte d'Azur.
- Master: Xavier Descombes, Artificial Intelligence for Histopathologist, master oncology, 3h Eq. TD, Niveau M2, Université Côte d'Azur.
- Master: Mohamad Mohamad, Introduction to Machine Learning and Deep Learning, DU AI and Health, 3h Eq. TD, Niveau M2, Université Côte d'Azur.
- Master: Alexandre Martin, Machine Learning for Image Analysis, Polytech Sophia, master, 4.5h Eq.TD, Niveau M2, Université Côte d'Azur.
- Master: Alexandre Martin, Image processing and Machine learning, master SVS, 10h Eq.TD Niveau M2, Université Côte d'Azur.
- Master: Luca Calatroni, Laure Blanc-Féraud, Faisal Jayousi, Inverse problems in image processing, niveau M2, 30h, MSc DSAI, Université Côte d'Azur, France.
- PhD: Luca Calatroni, Smooth and non-smooth optimisation for imaging, 8h, University of Bologna, Italy.
- Master: ECUE histoire de l'histoire de l'art: Luca Calatroni, Where arts & mathematics meet, niveau M1, 2h, Université Côte d'Azur, France.
- Engineer 3rd year: Cédric Dubois, Statistics and R, 26h TD, Polytech Nice Sophia, Université Côte d'Azur, France.
- Polytech L2 : Bastien Laville, Automatic, 58.5 hours hours (2nd year PeiP, equivalent to L2) Polytech Sophia Antipolis, UniCA.
- Polytech admission jury : Bastien Laville, 5 hours, Polytech Nice Sophia Antipolis, Université Côte d'Azur.
- Faisal Jayousi, Numérisation de l'information, BUT1 RT, 43.5 hours.
- Faisal Jayousi, internship defence, MSc 2 Data Science & Artificial Intelligence, 10 hours, Polytech Nice Sophia Antipolis, Université Côte d'Azur.
- Aneva Tsafack, Processus stochastique pour l'ingénieur, MAM 4, 24 heures, Polytech Nice Sophia Antipolis, Université Côte d'Azur.
- Centrale Méditerranée Bachelor1: Thomas Boudier, Computer Sciences and Programming (60h).
- Centrale Méditerranée DigitalLab: Thomas Boudier, Projects in AI (36h).
10.2.2 Supervision
- Xavier Descombes is supervising the PhD of Alexandre Martin, Mohamad Mohamad and co-supervising the PhD of Faisal Jayousi and Morgane Fierville. He was supervising the PhD of Imane Lboukili. He has supervised the internship of Mohamad Mohamad, Sonia Ciuffreda, Chloé Benbekrite and Waasila Khatir.
- Luca Calatroni is supervising the PhD of Marta Lazzaretti (co-tutelle with University of Genoa), the PhD of Perrine Saillard (co-supervision with CEPAM, Nice), from November 2023 he is co-supervising (with E. Soubies, CNRS) the PhD of M'hamed Essafri and from December 2023 he is co-supervising (with L. Blanc-Féraud) the PhD of Hamza Mentagui. He has supervised the internship of C. Santambrogio, Hamza Mentagui and M'hamed Essafri.
- Eric Debreuve is or was supervising Cédric Dubois (defense: March 2023) and co-supervising Asma Chalabi (with Jérémie Roux, Ircan/IPMC) and Clara Sanchez (with Carole Rovère, IPMC, defense: October 2023).
- Grégoire Malandain was a co-supervisor of Manuel Petit’s PhD (also co-supervised by C. Godin, Mosaic, Lyon), who defended his Phd June the 29th.
- Grégoire Malandain is supervising the PhD of Haydar Jammoul.
- Grégoire Malandain has supervised R. Hoblos (intern).
10.2.3 Juries
- Laure Blanc-Féraud was member of 4 PhD thesis juries (one as reviewer) and one HDR jury.
- Xavier Descombes was member of 4 PhD thesis juries (one as reviewer and two as president), he is member of two PhD committee (CSI).
- Luca Calatroni was member of 2 PhD thesis juries (one as reviewer).
- Eric Debreuve was member of 1 PhD thesis jury (as reviewer; University of Bergen, Norway).
- Grégoire Malandain was member of one PhD thesis committee.
- Thomas Boudier was member of one PhD thesis jury as reviewer.
10.2.4 Articles and contents
Article Nice-Matin, December 6th, 2023, Laure Blanc-Féraud.
10.2.5 Interventions
Interview BFM Côte d'Azur, October 25th, 2023, Laure Blanc-Féraud.
11 Scientific production
11.1 Major publications
-
1
articleA continuous relaxation of the constrained
problem.Journal of Mathematical Imaging and VisionJanuary 2021HAL - 2 articleFluorescence image deconvolution microscopy via generative adversarial learning (FluoGAN).Inverse Problems395April 2023, 054006HALDOIback to text
- 3 articleDetecting and quantifying stress granules in tissues of multicellular organisms with the Obj.MPP analysis tool.TrafficJuly 2019HALDOI
- 4 articleMultiple objects detection in biological images using a marked point process framework.Methods2016HALDOIback to text
- 5 articleFibronectin Extra Domains tune cellular responses and confer topographically distinct features to fibril networks.Journal of Cell ScienceFebruary 2021HAL
- 6 articleContact area-dependent cell communication and the morphological invariance of ascidian embryogenesis.ScienceJuly 2020HALDOI
11.2 Publications of the year
International journals
- 7 articleSpace-Variant Image Reconstruction via Cauchy Regularisation: Application to Optical Coherence Tomography.Signal Processing205April 2023HALDOI
- 8 articleA mobility-SAV approach for a Cahn-Hilliard equation with degenerate mobilities.Discrete and Continuous Dynamical Systems - Series SSeptember 2023HALDOI
- 9 articleFluorescence image deconvolution microscopy via generative adversarial learning (FluoGAN).Inverse Problems395April 2023, 054006HALDOI
- 10 articleFast and stable schemes for non-linear osmosis filtering.Computers & Mathematics with Applications133March 2023, 30-47HALDOI
- 11 articleCoupled mechanical mapping and interference contrast microscopy reveal viscoelastic and adhesion hallmarks of monocyte differentiation into macrophages.Nanoscale1529June 2023, 12255-12269HALDOI
- 12 articleA spatial statistical framework for the parametric study of fiber networks: application to fibronectin deposition by normal and activated fibroblasts.Biological Imaging2023HALDOI
- 13 articleOff-the-grid curve reconstruction through divergence regularisation: an extreme point result.SIAM Journal on Imaging Sciences162June 2023, 867-885HALDOI
- 14 articleAge‐dependent changes in epidermal architecture explored using an automated image analysis algorithm on in vivo reflectance confocal microscopy images.Skin Research and Technology295May 2023HALDOI
- 15 articleAutomatic granular and spinous epidermal cell identification and analysis on in vivo reflectance confocal microscopy images using cell morphological features.Journal of Biomedical Optics2804April 2023HALDOI
- 16 articleA machine learning framework for the quantification of experimental uveitis in murine OCT.Biomedical optics express1472023, 3413HALDOI
- 17 articleImproving CNNs classification with pathologist-based expertise: the renal cell carcinoma case study.Scientific Reports131December 2023, 15887HALDOI
- 18 articleOn and beyond Total Variation regularisation in imaging: the role of space variance.SIAM ReviewAugust 2023, 601-685HALDOI
-
19
articleBeyond
1 sparse coding in V1.PLoS Computational Biology199September 2023, e1011459HALDOIback to text
International peer-reviewed conferences
- 20 inproceedingsOff-the-Grid Charge Algorithm for Curve Reconstruction in Inverse Problems.LNCS. Lecture Notes in Computer ScienceSSVM 2023 -International Conference on Scale Space and Variational Methods in Computer VisionLNCS - 14009Scale Space and Variational Methods in Computer Vision : 9th International Conference, SSVM 2023, Santa Margherita di Pula, Italy, May 21–25, 2023, ProceedingsSanta Margherita di Pula, ItalySpringer International PublishingMay 2023, 393-405HALDOIback to text
- 21 inproceedingsReconstruction sans-grille de courbes : un algorithme pour la super-résolution.GRETSI'23 - XXIX ème Colloque Francophone de Traitement du Signal et des ImagesGrenoble, FranceAugust 2023HALback to text
- 22 inproceedingsStochastic gradient descent for linear inverse problems in variable exponent Lebesgue spaces.Scale Space and Variational Methods in Computer Vision. SSVM 2023. Lecture Notes in Computer Science, vol 14009IX conference on Scale Space and Variational Methods in Computer Vision 202314009Lecture Notes in Computer ScienceSanta margherita di Pula, ItalySpringer International PublishingMay 2023, 457-470HALDOIback to text
- 23 inproceedingsFluctuation-based deconvolution in fluorescence microscopy using plug-and-play denoisers.Scale Space and Variational Methods in Computer Vision14009Lecture Notes in Computer ScienceSardinia, ItalySpringer International PublishingMarch 2023, 498-510HALDOIback to text
Doctoral dissertations and habilitation theses
- 24 thesisA nearest-neighbours kernel for classification : a case study of in situ two-dimensional plankton images with correction of total volume estimates for copepods.Université Côte d'AzurMarch 2023HAL
- 25 thesisAnalysis and characterization of the tissue structure of the epidermis from confocal imaging.Université Côte d'AzurOctober 2023HAL
- 26 thesisLearning and optimization for 3D super-resolution in fluorescence microscopy.Université Côte d'AzurJanuary 2023HAL
Reports & preprints
11.3 Cited publications
- 30 bookThe organization and cell-lineage of the ascidian egg / by Edwin G. Conklin..Philadelphia :[Academy of Natural Sciences],1905, 182URL: http://www.biodiversitylibrary.org/item/24005back to textback to text
- 31 articleUncertainty relation for resolution in space, spatial frequency, and orientation optimized by two-dimensional visual cortical filters..J Opt Soc Am A27July 1985, 1160-9back to text