2024Activity reportProject-TeamMORPHEME
RNSR: 201120999G- Research center Inria Centre at Université Côte d'Azur
- In partnership with:CNRS, Université Côte d'Azur
- Team name: Morphologie et Images
- In collaboration with:Laboratoire informatique, signaux systèmes de Sophia Antipolis (I3S), Institut de Biologie de Valrose
- Domain:Digital Health, Biology and Earth
- Theme:Computational Biology
Keywords
Computer Science and Digital Science
- A3.4. Machine learning and statistics
- A3.4.1. Supervised learning
- A3.4.2. Unsupervised learning
- A3.4.4. Optimization and learning
- A3.4.6. Neural networks
- A3.4.7. Kernel methods
- A3.4.8. Deep learning
- A5.3. Image processing and analysis
- A5.3.2. Sparse modeling and image representation
- A5.3.3. Pattern recognition
- A5.3.4. Registration
- A5.4.3. Content retrieval
- A5.4.4. 3D and spatio-temporal reconstruction
- A5.4.5. Object tracking and motion analysis
- A5.4.6. Object localization
- A5.9. Signal processing
- A5.9.3. Reconstruction, enhancement
- A5.9.5. Sparsity-aware processing
- A5.9.6. Optimization tools
- A6.1. Methods in mathematical modeling
- A6.1.1. Continuous Modeling (PDE, ODE)
- A6.1.2. Stochastic Modeling
- A6.3.1. Inverse problems
Other Research Topics and Application Domains
- B1.1. Biology
- B1.1.3. Developmental biology
- B2.6. Biological and medical imaging
1 Team members, visitors, external collaborators
Research Scientists
- Xavier Descombes [Team leader, INRIA, Senior Researcher, HDR]
- Florence Besse [CNRS, Senior Researcher]
- Laure Blanc Feraud [CNRS, Senior Researcher, HDR]
- Luca Calatroni [CNRS, Researcher]
- Eric Debreuve [CNRS, Researcher, HDR]
- Grégoire Malandain [INRIA, Senior Researcher, HDR]
- Caroline Medioni [CNRS, Researcher]
Faculty Member
- Thomas Boudier [Ecole Centrale Méditerranée, convention with Univ. Côte d'Azur, Associate Professor]
Post-Doctoral Fellow
- Emmanuel Bouilhol [UNIV COTE D'AZUR, Post-Doctoral Fellow]
PhD Students
- Moncef Belaskri [UNIV TLEMCEN, from Dec 2024]
- Moncef Belaskri [UNIV TLEMCEN, from Nov 2024 until Nov 2024]
- Asma Chalabi [CNRS, until Mar 2024]
- Morgane Fierville [CNRS]
- Haydar Jammoul [UNIV COTE D'AZUR]
- Faisal Jayousi [CNRS]
- Anna Kestel [INRIA, from Oct 2024]
- Imane Lboukili [JOHNSON]
- Alexandre Martin [INRIA]
- Hamza Mentagui [CNRS]
- Mohamad Mohamad [UNIV COTE D'AZUR]
- Meryem Sikouky [UNIV COTE D'AZUR, from Nov 2024]
- Larbi Touijer [UNIV GENES, from Jul 2024 until Aug 2024]
- Larbi Touijer [UNIV GENES, from May 2024 until Jul 2024]
- Aneva Doliciane Tsafack [UNIV COTE D'AZUR]
Technical Staff
- Wessim Omezzine [CNRS, Engineer, from Jul 2024 until Oct 2024]
Interns and Apprentices
- Santosh Adhikari [UNIV COTE D'AZUR, Intern, from Mar 2024 until Aug 2024]
- Christian Daniele [UNIV GENES, Intern, from Jun 2024 until Oct 2024]
- Claudio Fantasia [INRIA, Intern, from May 2024 until Oct 2024]
- Antonio Ferrigno [INRIA, Intern, from Sep 2024 until Nov 2024]
- Sarah M'Nasri [UNIV COTE D'AZUR, Intern, from May 2024 until Aug 2024]
- Ivan Magistro Contenta [INRIA, Intern, from Oct 2024]
- Alexandre Ricci [UNIV COTE D'AZUR, Intern, from Feb 2024 until Aug 2024]
- Meryem Sikouky [INRIA, Intern, from Mar 2024 until Aug 2024]
- Yu Song [INRIA, Intern, from Jun 2024 until Aug 2024]
- Anran Xu [CNRS, Intern, from May 2024 until Oct 2024]
- Zhenyu Zhu [UNIV COTE D'AZUR, Intern, from Mar 2024 until Sep 2024]
Administrative Assistants
- Nadia Belfegas [CNRS]
- Stephanie Verdonck [INRIA]
2 Overall objectives
Morpheme is a joint project between INRIA, CNRS and the University of Côte d'Azur (UniCA); Signals and Systems Laboratory (I3S) (UMR 6070); the Institute for Biology of Valrose (iBV) (CNRS/INSERM).
The scientific objectives of Morpheme are to characterize and model the development and the morphological properties of biological structures from the cell to the supra-cellular scale. Being at the interface between computational science and biology, we plan to understand the morphological changes that occur during development combining in vivo imaging, image processing and computational modeling.
The morphology and topology of mesoscopic structures, indeed, do have a key influence on the functional behavior of organs. Our goal is to characterize different populations or development conditions based on the shape of cellular and supra-cellular structures, including micro-vascular networks and dendrite/axon networks. Using microscopy or tomography images, we plan to extract quantitative parameters to characterize morphometry over time and in different samples. We will then statistically analyze shapes and complex structures to identify relevant markers and define classification tools. Finally, we will propose models explaining the temporal evolution of the observed samples. With this, we hope to better understand the development of normal tissues, but also characterize at the supra-cellular level different pathologies such as the Fragile X Syndrome, Alzheimer or diabetes.
3 Research program
3.1 Research program
The recent advent of an increasing number of new microscopy techniques giving access to high throughput screenings and micro or nano-metric resolutions provides a means for quantitative imaging of biological structures and phenomena. To conduct quantitative biological studies based on these new data, it is necessary to develop non-standard specific tools. This requires using a multi-disciplinary approach. We need biologists to define experiment protocols and interpret the results, but also physicists to model the sensors, computer scientists to develop algorithms and mathematicians to model the resulting information. These different expertises are combined within the Morpheme team. This generates a fecund frame for exchanging expertise, knowledge, leading to an optimal framework for the different tasks (imaging, image analysis, classification, modeling). We thus aim at providing adapted and robust tools required to describe, explain and model fundamental phenomena underlying the morphogenesis of cellular and supra-cellular biological structures. Combining experimental manipulations, in vivo imaging, image processing and computational modeling, we plan to provide methods for the quantitative analysis of the morphological changes that occur during development. This is of key importance as the morphology and topology of mesoscopic structures govern organ and cell function. Alterations in the genetic programs underlying cellular morphogenesis have been linked to a range of pathologies.
Biological questions we will focus on include:
- what are the parameters and the factors controlling the establishment of ramified structures? (Are they really organized to ensure maximal coverage? How are genetic and physical constraints limiting their morphology?),
- how are newly generated cells incorporated into reorganizing tissues during development? (is the relative position of cells governed by the lineage they belong to?)
Our goal is to characterize different populations or development conditions
based on the shape of cellular and supra-cellular structures, e.g. micro-vascular
networks, dendrite/axon networks, tissues from 2D, 2D+t, 3D or 3D+t images (obtained with confocal microscopy, video-microscopy, photon-microscopy or micro-tomography). We plan to extract shapes or quantitative parameters to characterize the morphometric properties of different samples. On the one hand, we will
propose numerical and biological models explaining the temporal evolution of the
sample, and on the other hand, we will statistically analyze shapes and complex
structures to identify relevant markers for classification purposes. This should
contribute to a better understanding of the development of normal tissues but
also to a characterization at the supra-cellular scale of different pathologies such
as Alzheimer, cancer, diabetes, or the Fragile X Syndrome.
In this multidisciplinary context, several challenges have to be faced. The
expertise of biologists concerning sample generation, as well as optimization of
experimental protocols and imaging conditions, is of course crucial. However,
the imaging protocols optimized for a qualitative analysis may be sub-optimal
for quantitative biology. Second, sample imaging is only a first step, as we need
to extract quantitative information. Achieving quantitative imaging remains an
open issue in biology, and requires close interactions between biologists, computer
scientists and applied mathematicians. On the one hand, experimental and imaging protocols should integrate constraints from the downstream computer-assisted
analysis, yielding to a trade-off between qualitative optimized and quantitative optimized protocols. On the other hand, computer analysis should integrate constraints specific to the biological problem, from acquisition to quantitative information extraction. There is therefore a need of specificity for embedding precise
biological information for a given task. Besides, a level of generality is also desirable for addressing data from different teams acquired with different protocols
and/or sensors.
The mathematical modeling of the physics of the acquisition system will yield
higher performance reconstruction/restoration algorithms in terms of accuracy.
Therefore, physicists and computer scientists have to work together. Quantitative
information extraction also has to deal with both the complexity of the structures of interest (e.g., very dense network, small structure detection in a volume,
multiscale behavior,
-
Imaging: this includes i) definition of the studied populations (experimental
conditions) and preparation of samples, ii) definition of relevant quantitative
characteristics and optimized acquisition protocol (staining, imaging,
) for the specific biological question, and iii) reconstruction/restoration of native data to improve the image readability and interpretation. - Feature extraction: this consists in detecting and delineating the biological structures of interest from images. Embedding biological properties in the algorithms and models is a key issue. Two main challenges are the variability, both in shape and scale, of biological structures and the huge size of data sets. Following features along time will allow to address morphogenesis and structure development.
- Classification/Interpretation: considering a database of images containing different populations, we can infer the parameters associated with a given model on each dataset from which the biological structure under study has been extracted. We plan to define classification schemes for characterizing the different populations based either on the model parameters, or on some specific metric between the extracted structures.
- Modeling: two aspects will be considered. This first one consists in modeling biological phenomena such as axon growing or network topology in different contexts. One main advantage of our team is the possibility to use the image information for calibrating and/or validating the biological models. Calibration induces parameter inference as a main challenge. The second aspect consists in using a prior based on biological properties for extracting relevant information from images. Here again, combining biology and computer science expertise is a key point.
4 Application domains
Among the applications addressed by Morpheme team we can cite:
- Kidney cancer classification from histological images
- IMP-RNA (ribonucleicc acid) granules detection and classification from confocal image
- Extra-cellular matrix detection and characterization from confocal images
- Axon growth modeling
- Glial cell detection and characterization for the study of high-fat diets
- Death and division time detection and type classification of cells in microscopy time-lapses
- Plankton images analysis and classification
- Morphogenesis and embryogenesis
- Numerical super-resolution techniques
- Convex and non-convex sparse optimization with applications to biomedical imaging
- Statistical and learning-based approaches for parameter selection in imaging inverse problems
- Physics-inspired machine learning for fluorescence microscopy
5 Highlights of the year
5.1 Video
A presentation video has been published on the CNRS Youtube channel concerning the work of Laure Blanc-Féraud.
6 New software, platforms, open data
6.1 New software
6.1.1 Obj.MPP
-
Keywords:
Object detection, Marked Point Process, Parametric model
-
Functional Description:
Obj.MPP implements the detection of parametric objects using a Marked Point Process (MPP). A parametric object is an n-dimensional piece of signal defined by a finite set of parameters. Detecting an object in a signal amounts to finding a position at which the signal can be described well enough by a specific set of parameters (unknowns of the detection problem). The detection task amounts to finding all such objects. Typically, the signal is a 2-dimensional grayscale image and the parametric objects are bright disks on a dark background. In this case, each object is defined by a single parameter: the disk radius. Note however that the core function of Obj.MPP is not tied to a particular context (2-dimensional imaging is just an example).
- URL:
- Publications:
-
Contact:
Eric Debreuve
6.1.2 RCC-VascularMorphClassify
-
Name:
Renal Cell Carcinoma Classification from Vascular Morphology
-
Keywords:
Machine learning, Cancer, Biomedical imaging
-
Functional Description:
Our proposed two sets of hand-crafted features, skeleton, and lattice features, which are extracted from the vascular network segmentation images, can classify RCC subtypes robustly.
- URL:
-
Contact:
Xavier Descombes
6.1.3 Ascidian
-
Name:
Ascidian package
-
Keywords:
Embryogenesis, Morphogenesis
-
Scientific Description:
This suite exploits the results issued from the Astec suite (https://astec.gitlabpages.inria.fr/astec/) for image series of developing ascidian embryos. It allows to name individual cells (with the Conklin nomenclature), to assess the segmentation issued from Astec, and to perform population based studies.
-
Functional Description:
The Ascidian package aims at exploiting results issued from the processing of 3D+t sequences of developing embryos imaged by a light-sheet microscope. The processing, done by the ASTEC suite (https://astec.gitlabpages.inria.fr/astec/), results in a so-called property file, which is the input of Ascidian package procedure.
- URL:
- Publications:
-
Contact:
Grégoire Malandain
-
Participants:
Grégoire Malandain, Haydar Jammoul
-
Partner:
CRBM - Centre de Recherche en Biologie cellulaire de Montpellier
6.1.4 Mufasa
-
Name:
Fluorescence Fluctuations Simulation: MUFASA Simulator
-
Keywords:
Blinking simulation, SMLM, Super-resolution
-
Functional Description:
The simulation software takes into account : - different laser powers, - different camera types, blur and noise levels - different fluorophores, - Multi-protocol support: Includes blinking and fluorescence fluctuation protocols: Fluorescence Fluctuations (FF), SMLM (STORM, PALM), Blinking. The software models molecule transitions using continuous-time Markov chains (CTMC)
- URL:
-
Contact:
Wessim Omezzine
6.1.5 FibreSight
-
Keywords:
Biomedical imaging, Biostatistics
-
Functional Description:
The Python module aims to facilitate the study of fibrillar proteins in the tumour extracellular matrix using fluorescence microscopy images. It includes a preprocessing step to remove non-fibrillar aggregates, detects and characterises fibres using Gabor filters, and partitions the image into locally homogeneous regions using graphs and Voronoi diagrams. The module computes statistics within these regions and proposes an alignment index to quantify fibre organisation.
-
Contact:
Faisal Jayousi
7 New results
7.1 Fluorescence image deconvolution microscopy via generative adversarial learning (FluoGAN)
Participants: Hamza Mentagui, Laure Blanc-Féraud, Luca Calatroni, Sébastien Schaub.
Image super-resolution techniques exploiting the stochastic fluctuations of image intensities have become a powerful tool in fluorescence microscopy. Compared to other approaches, these techniques can be applied under standard acquisition settings and do not require special microscopes nor fluorophores. Most of these approaches can be mathematically modeled making use of second-order statistics possibly combined with a priori regularization on the desired solution. In 15, we consider a different paradigm and formulate a physical-inspired data-driven approach based on generative learning. By simulating fluorescence and noise fluctuations by means of a suitable double Poisson-type process, the unknown distribution of the fluctuating sequence of low- resolution and noisy images is approximated via a GAN-type approach (Generative Adversial Network) where both physical and network parameters are optimized, see 2. We also provide theoretical insights on the choice of the corresponding cost functionals and gradient computations, and assess practical performance on simulated Argolight (see Figure 1).

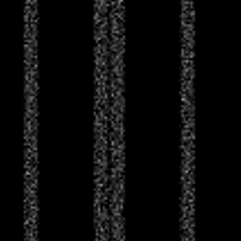

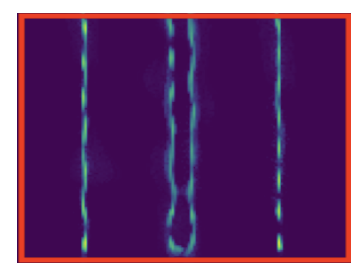
Reconstruction of data generated using the SOFI (Super-resolution Optical Fluctuation Imaging) tool software using Arolight slide-type patterns.
7.2 From Photon Emission to Super-Resolution: The MUFASA Simulator
Participants: Wessim Omezzine, Hamza Mentagui, Laure Blanc-Féraud, Luca Calatroni, Sébastien Schaub.
Advances in Super-resolution techniques, like Single Molecule Localization Microscopy (SMLM) and Fluorescence Fluctuation-based SRM (FF-SRM) allowed to go beyond the diffraction limit. These breakthroughts rely on the photophysical processes, including the stochastic nature of photon emission and transitions between quantum states. Despite this progress, replicating these protocols in experimental setups remains challenging due to the complex behavior of fluorophores, which depends on environmental factors like laser power and molecular properties. Existing simulators often offer only one type of protocol and focus primarily on ”on” and ”off” regimes, failing to capture the fluctuation characteristics of fluorescence. We introduce MUFASA (Muitl-protocol Unified Fluorescence-based Advanced Simula), a comprehensive simulator that unifies multiple protocols within a physically grounded framework. Using Continuous Time Markov Chains, MUFASA captures detailed quantum state transitions and stochastic photon emission dynamics. Unlike existing simulators, MUFASA incorporates environment-dependent transition rates and continuous emission dynamics, providing enhanced realism and flexibility. MUFASA supports key protocols, including STORM, PALM, Blinking and FFSRM, offering insights into molecular dynamics and optimizing experimental conditions. Using Wasserstein distances, we validate the distribution of MUFASA simulations against experimental data, demonstrating superior accuracy compared to existing tools. Its graphical interface enables accessible customization, empowering researchers to simulate both single-molecule behavior and complex biological structures. By bridging theoretical modeling with practical experimentation, MUFASA advances fluorescence microscopy simulation, facilitating the development and optimization of imaging techniques and reconstruction algorithms. Moreover, it serves as a valuable tool for generating realistic data to train and evaluate learning models, further enhancing research capabilities.
7.3 Off-the-grid dynamic super-resolution for fluorescence microscopy
Participants: Aneva Tsafack, Laure Blanc-Féraud, Gilles Aubert.
Recently, the Curves Represented On Charges (CROC) functional
was introduced for curve reconstruction in microscopy images, optimizing within the space of finite vector-valued Radon measures with finite divergence, denoted
We define a space of Lebesgue measures
and prove its density in the previous space
For numerical implementation, a key challenge was modeling the data term due to the mismatch between the vector-valued optimization framework and the scalar nature of the observed images. Initial attempts to derive observed vector fields from image gradients proved unsatisfactory. To address this, a scalar-based acquisition model was developed to generate scalar images from velocity vector fields along curves:
where
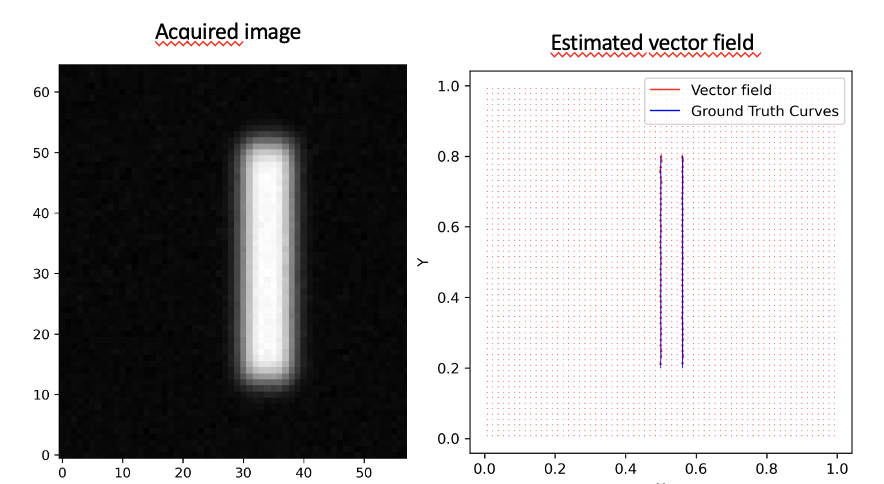
Observed blurred and noisy image of two parallel curves (left), estimated vector field recovering the two curves (right)
7.4 Off-the-grid super-resolution for Poisson data
Participants: Luca Calatroni.
This work was made in collaboration with M. Lazzaretti and C. Estatico (University of Genova, Italy).
To model acquisitions where the observed noise is due to low photon-counting processes (which is often the case in microscopy applications), we consider a variational model where off-the-grid Total Variation regularization is coupled with a Kullback-Leibler data term under a non-negativity constraint. Analytically, we study the optimality conditions of the composite functional and analyze its dual problem. Then, we consider an homotopy strategy to select an optimal regularization parameter and use it within a Sliding Frank-Wolfe algorithm. Several numerical experiments on both 1D/2D/3D simulated and real 3D fluorescent microscopy data are reported on Figure 3
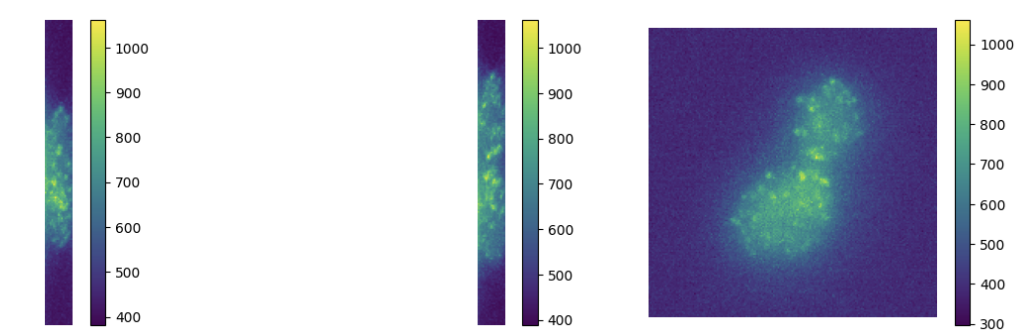
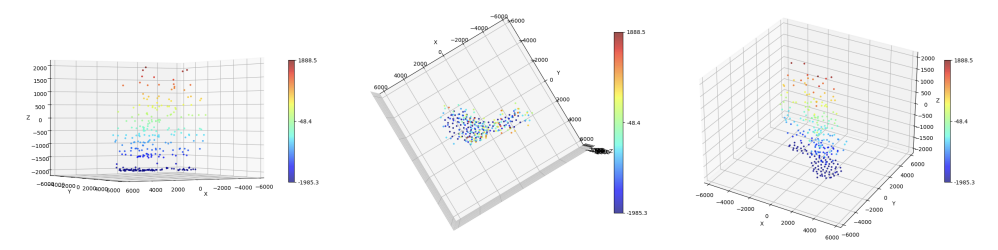
Deconvolution of 3D TIRF acquisitions. Top: 2D projections. Bottom: different views of 3D reconstruction
This work is reported in the preprint 21 and part of the PhD thesis 18.
7.5 Patch-based learning of adaptive parameter maps for image reconstruction
Participants: Luca Calatroni, Xavier Descombes, Claudio Fantasia.
In collaboration with Rim Rekik Dit Nekhili (Inria).
In this project, we consider a variational reconstruction model based on a Total Variation regularization combined with a mixed data-fidelity term adapted to the presence of either Gaussian or Poisson noise in the observed data. To adjust the effect of TV (Total Variation)regularization against data fidelity, a non-stationary (i.e., pixel-adaptive) parameter map is considered and learned patch-wise by means of a light neural network taking as input a noisy patch and providing as output a locally optimal parameter. Training is thus performed by providing as labels pairs
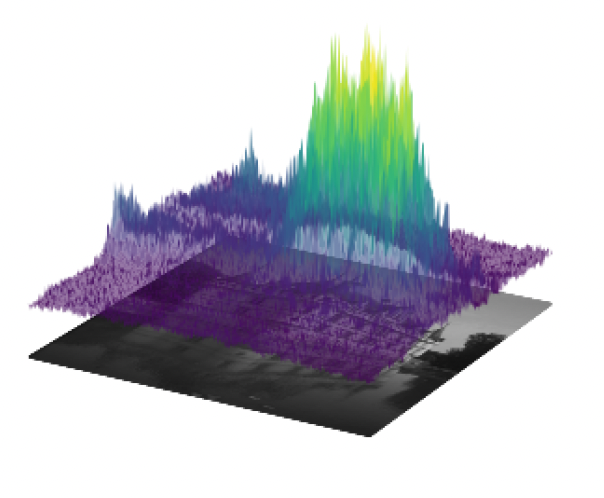
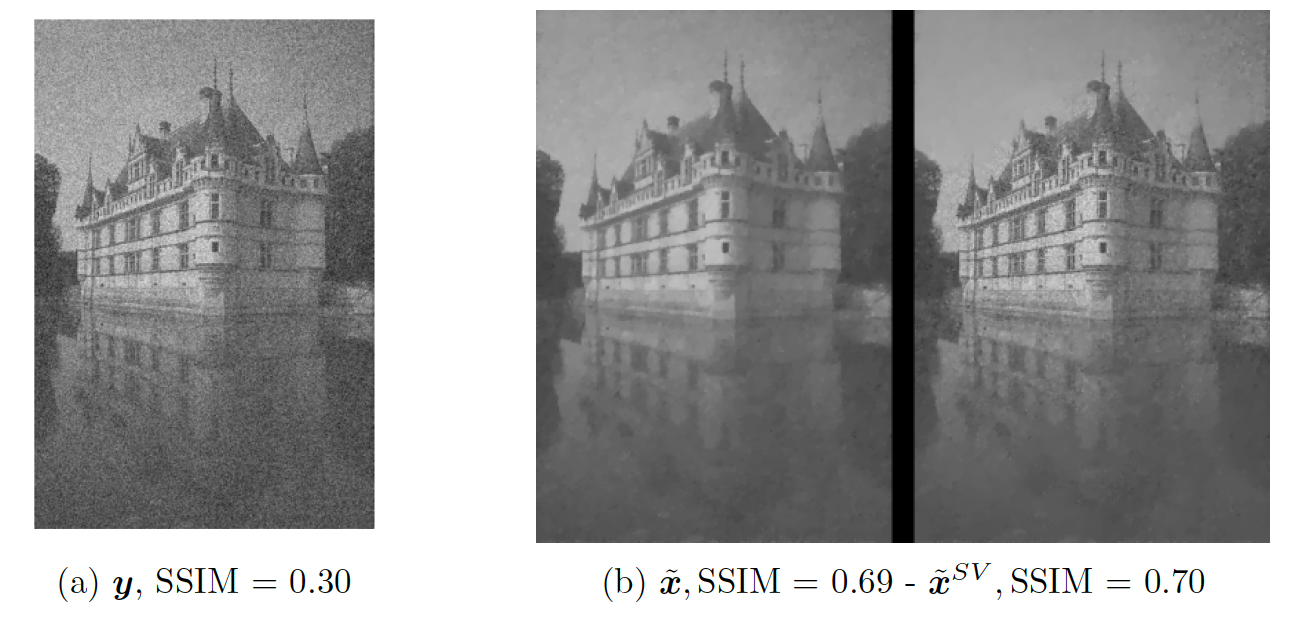
Left: parameter map computed by a deep learning model. Pixel-wise, the computed parameter weigths the data fidelity so that higher values correspond to more preservation of textured areas while lower values induce more smoothing. Right: given noisy image and comparison between scalar and non-stationary approach
7.6 Deep-equilibrium approaches for learning regularization in Poisson inverse problems
Participants: Luca Calatroni, Christian Daniele.
This work was made in collaboration with S. Villa (University of Genoa) and S. Vaiter (LJAD, CNRS).
We consider the framework of deep equilibrium models to learn image regularizers in the context of imaging data corrupted by Poisson noise. In this framework, the Kullback-Leibler divergence is usually considered as a data term, which poses some difficulties due to its lack of Lipschitz-smoothness around zero. By using a mirror descent algorithm and enforcing a Lipschitz-like condition to guarantee convergence of the scheme even in non
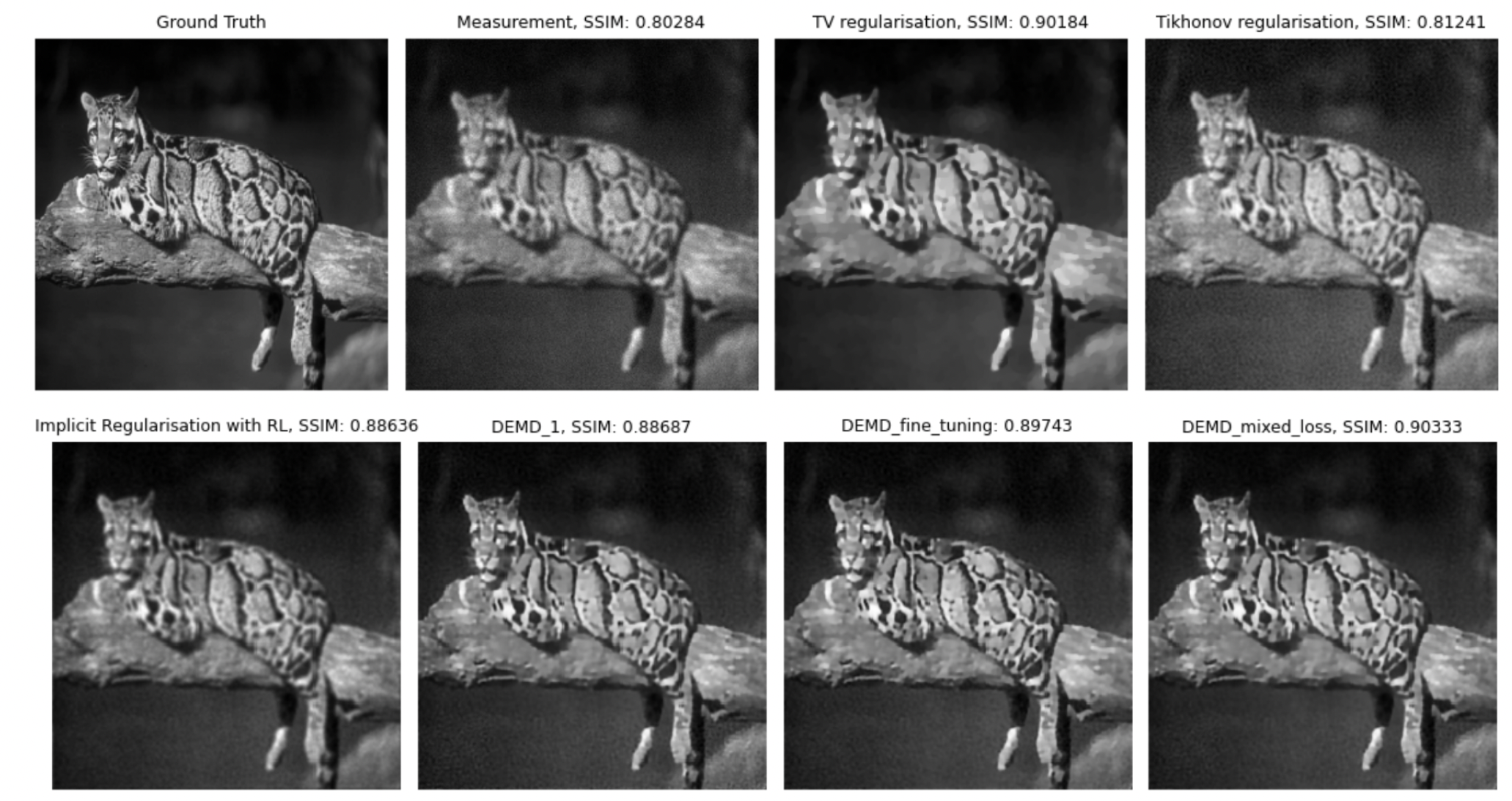
Ground truth, blurred and noisy data and model-based reconstructions (with TV, Tikhonov, iterative regularization obtained by minimizing the Kullback-Leibler) and two Deep-Equilibrium models trained in the project with low/high level of noise and with/without further TV smoothing.
7.7 continuous Bregman-relaxations for sparse problems with non quadratic data terms
Participants: Luca Calatroni, M'hamed Essafri.
This work was made in collaboration with E. Soubies (IRIT, CNRS).
We consider the minimization of
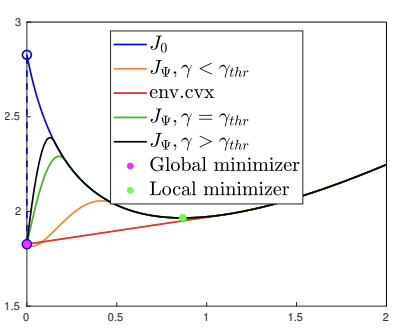
Illustration of the obtained relaxations for a 1D example in the case of minimization of a functional
A preprint of this work is online at 20 and the study on the KL case is published in 12.
7.8 Parameter Free FISTA
Participants: Luca Calatroni.
This work was made in collaboration with A. Rondepierre, C. Dossal (IMT et INSA, Toulouse), J.F. Aujol (IMB, Bordeaux) and H. Labarriere (University of Genoa).
We consider a parameter-free version of the famous FISTA algorithm to solve composite non-smooth optimization problems ubiquitous in the field of regularized inverse problems and machine learning. The technique relies on the use of a combined restarting and adaptive backtracking strategy. Several variants of FISTA enjoy a provable linear convergence rate for the function values of the form under the prior knowledge of problem conditioning. These parameters are nonetheless hard to estimate in many practical cases. Recent works address the problem by estimating parameters in two steps via suitable adaptive strategies. In our work, both parameters can be estimated at the same time by means of an algorithmic restarting scheme where, at each restart, a nonmonotone estimation is performed. For this scheme, theoretical convergence results are proved, showing that linear convergence can be achieved along with quantitative estimates of the conditioning. The resulting free-FISTA is therefore parameter-free. Several numerical results are reported to confirm the practical interest of its use in many example problems (see Figure 7).
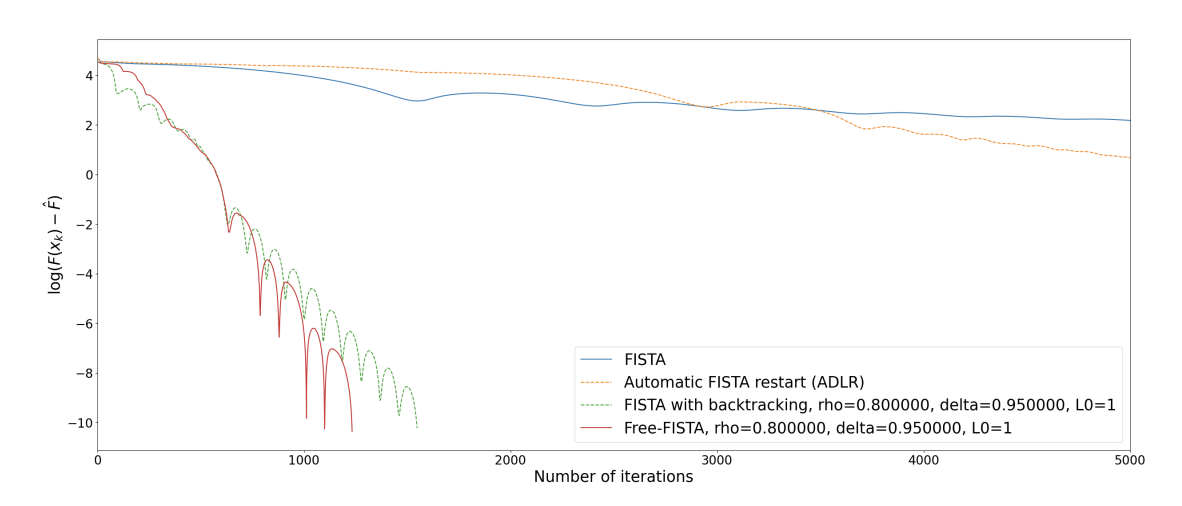
Convergence in function values for a logistic regression problem for plain FISTA (no estimation of parameters), ADLR (automatic restart FISTA, only the growth parameter is estimated with now step-size), FISTA with backtracking (only step-size is estimated), FISTA with restarting (only growth parameter is estimated) and parameter Free-FISTA.
This project has been published in 7.
7.9 Dynamic analysis framework for live-cell imaging to detect cell responses to anticancer drugs using image and signal processing, and deep learning
Participants: Asma Chalabi, Eric Debreuve.
This work was conducted in collaboration with Jérémie Roux, IPMC, Sophia Antipolis, France.
Cell division and cell death are the main indicators to evaluate cancer drug action, and only their accurate measures can reveal the actual potency and efficacy of a compound. The detection of cell division and cell death events in live-cell assays has the potential to produce robust metrics of drug pharmacodynamics and return a more comprehensive understanding of tumor cells responses to cancer therapeutic combinations. Knowing precisely when a cell death or a cell division occurs in a live-cell experiment allows to study the relative contribution of different drug effects, such as cytotoxic or cytostatic effects, on a cell population. Yet, classical methods require dyes to measure cell viability as an end-point assay with whole population counts, where the proliferation rates can only be estimated when both viable and dead cells are labeled simultaneously.
Live-cell imaging is a promising cell-based assay to determine drug efficacies, with the main limitation being the accuracy and depth of the analyses to detect and predict automatically cellular response phenotypes (cell death and division, which share some morphological features). This work proposes a method integrating deep learning using neural networks, and image and signal processing to perform dynamic image analyses of single-cell events in time-lapse microscopy experiments of drug pharmacological profiling. The method works by automatically tracking the cells, extracting radiometric and morphologic cell features, and analyzing the temporal evolution of these features for each cell to detect cellular events such as division and cell death, as well as acquiring signaling pathway dynamics (see Figure 8). A case of study comprising the analyses of caspase-8 single-cell dynamics and other cell responses to cancer drugs has been achieved. The aim was to perform automatically, at a large scale, the necessary analyses to augment a previous phenotype prediction method and to apply it to various cancer cell lines of a human cancer cell line panel to improve our live-cell OMICS profiling approaches, and, in a longer term, to scale up pharmacological screening of new cancer drugs.
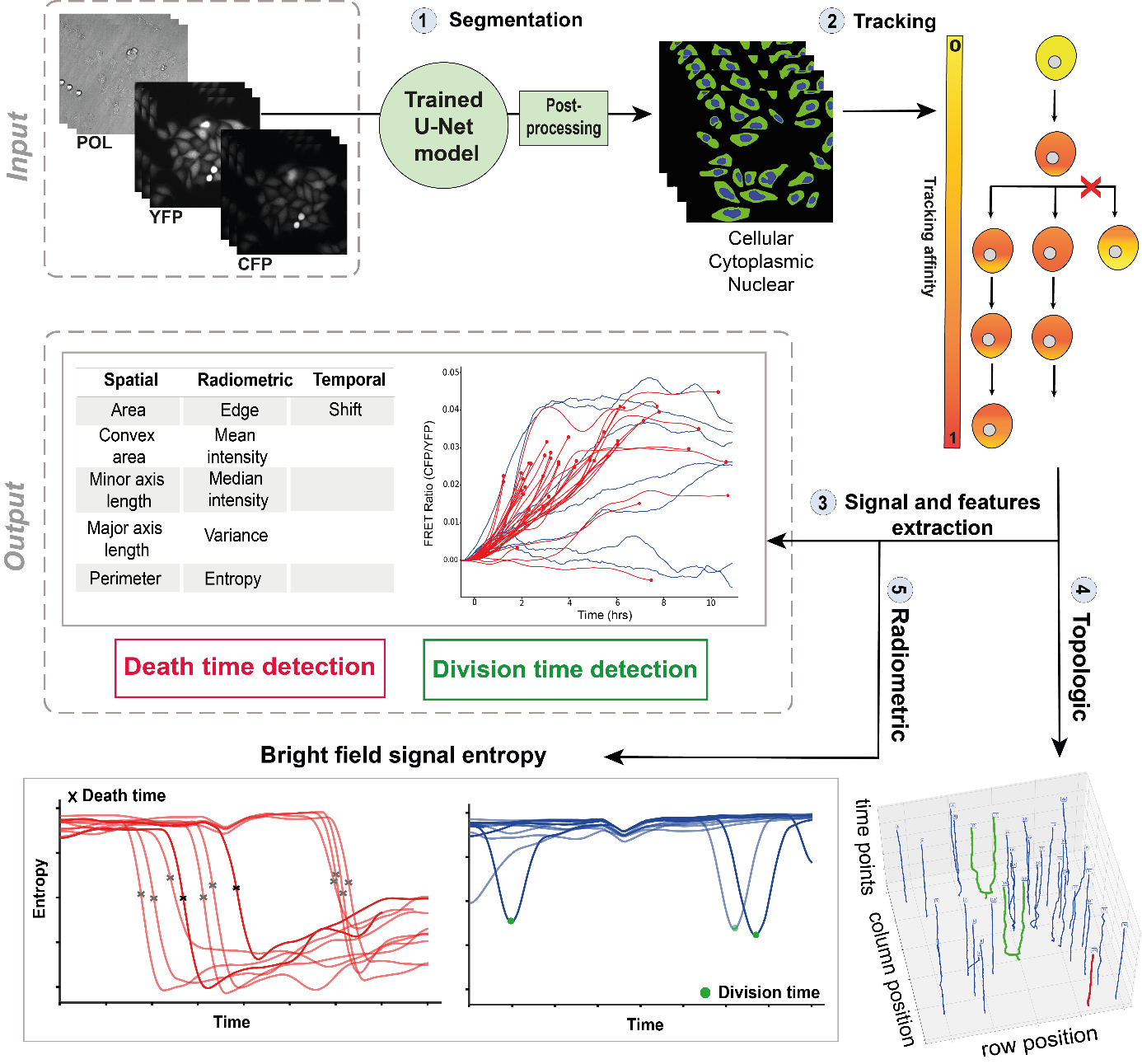
Automated framework to detect cell division and cell death times in live-cell microscopy data. (1) The Yellow Fluorescent Protein channel is used to perform cell segmentation using U-Net model. (2) Cells are tracked over time to create single trajectories. (3) Spatial, radiometric, and temporal features are extracted for each single-cell (4) and (5) Cell division and cell death are detected with both topologic tracking-based approach and radiometric pattern matching based approach.
7.10 Identifying autofluorescence in biological samples using hyperspectral imaging
Participants: Eric Debreuve.
This work was made in collaboration with Sébastien Schaub and Jenifer Croce, IMEV, Villefranche-sur-mer, France.
Fluorescence imaging of marine samples (animals or plants) remains a challenge due to the inevitable endogenous fluorescence (or autofluorescence) common in these samples, for example due to an animal ingesting algae exhibiting endogenous fluorescence. The autofluoresence superimposes with the fluorescence of the probes which are the target of a specific study. The aim of this work is to take advantage of recent improvements in fluorescence imaging to identify and subtract sample autofluorescence from probe fluorescence using hyperspectral imaging, i.e. using so-called
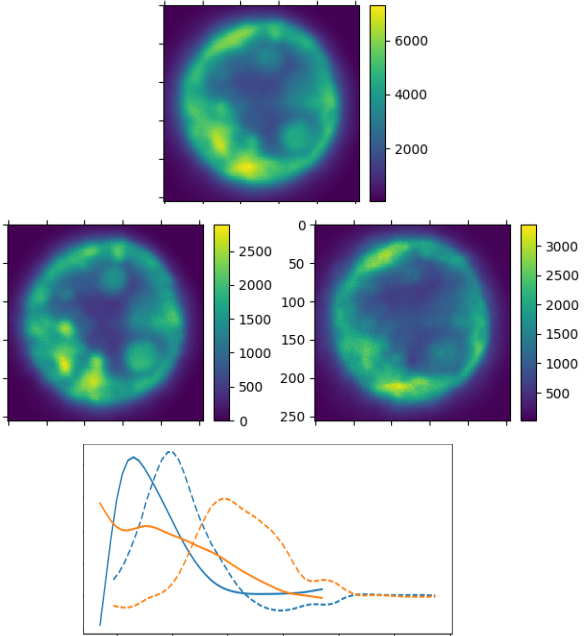
7.11 Machine learning for organoid phenotyping
Participants: Alexandre Martin, Xavier Descombes, Ivan Magistro Contenta, Stephan Clavel [IPMC, Sophia Antipolis], Xavier Coumoul [Metatox, Paris].
The aim of this work is to built a phenotypic map of organoids. We model an organoid as a 3D shape containing several layers of cells composed of 2D point patterns on the sphere. Our goal is to classify organoids with respect to these three components.
The main work during this year concerns machine learning for graphs in order to combine discriminative capacity and interpretability. We advanced on organoid classification by integrating more complex neural network architectures. We first considered Convolutional Neural Networks (CNNs) to extract spatial features from organoid images. Although effective, CNNs struggled to capture the relational and structural complexity of cellular arrangements. We therefore moved to Graph Neural Networks (GNNs), representing the data as graphs with nodes for cells and edges for spatial or functional relationships. Early experiments with Graph Convolutional Networks (GCNs) showed promise but lacked the capacity to handle the geometric properties of the data. To address this bottleneck, we employed geometric GNNs, including Equivariant Neural Networks (ENNs), which respect rotational and translational symmetries. These models significantly improved the classification accuracy by taking advantage of the invariances inherent to biological structures. This progression in architecture has improved both the performance and biological relevance of the results. Furthermore, data augmentation techniques were applied to enhance model robustness and performance.
The organoid modeling is based on nuclei detection, each nuclei representing a graph node. In previous year, we have compared different approaches to detect nuclei. Cellpose, a state of the art deep learning model, has provided the best performances in terms of accuracy on 2D slices (see Figure 10). The performances on 3D datasets are similar but the computation time is heavy (about 20 min for one volume). Therefore, we have studied different frugal approaches to reduce the model size, including pruned and dilated models. Currently we investigate the distillation process by considering a teacher-student framework. The first results are encouraging.
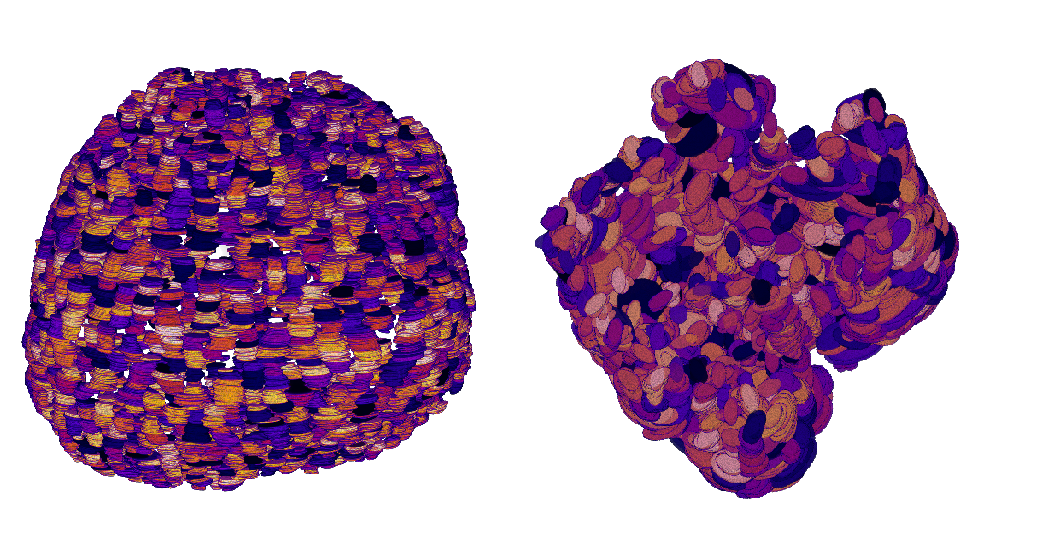
Reconstruction of two different organoid phenotypes.
7.12 Detection and analysis of pores in the basal lamina of breast cancer spheroids
Participants: Zhenyu Zhu, Xavier Descombes, Frédéric Luton [IPMC, Sophia Antipolis].
The pores are detected on a 2D image of Collagen IV. By comparing on six annoted samples a geometric method based on marked point process and the neuronal networks, Cellpose and Stardist, the most efficient method and the appropriate settings have been selected. After fine-tuning the model, Cellpose appears to be better suited to detecting pores (see Figure 11). Based on the detection results, we can establish statistics on the size and number of pores. In a next step, we considered the structure of Collagen in 3D. The pore detection is still performed in 2D and the pores in 3D are obtained by aggregating the 2D slices. To recover the local geometry in order to have a precise estimation of the pore size, we compute an alpha shape of the spheroid and project the detected 3D pores on the local tangent plane.
A work in progress consists then to detect filipodes representing cell extensions through the pores.
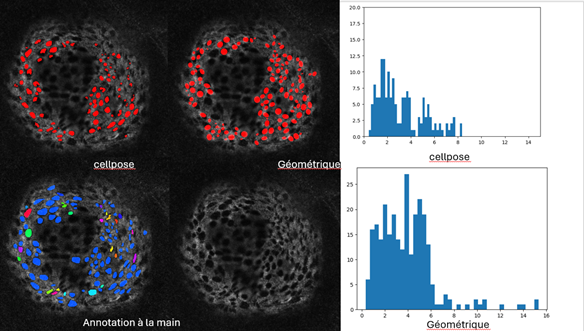
7.13 An automatic BANFF classification
Participants: Meryem Sikouky, Mohammad Mohammad, Xavier Descombes, Damien Ambrosetti [CHU, Nice], Giorgio Toni [CHU, Nice], Paul Hannetel [CHU, Nice].
The Banff classification is a standardized system used to assess transplant rejection risks from kidney biopsies. It involves the examination of whole-slide images (WSIs) by pathologists to identify and score various histological features. Automating this process through image analysis and machine learning could potentially improve the efficiency and objectivity of the evaluation. As a first step towards this goal, this project focuses on the segmentation of kidney histology images. The aim is to accurately identify and delineate different anatomical structures within the kidney, such as glomeruli, PTC, vessels, and tubules. The initial phase of the research involved exploring various neural network architectures for this segmentation task, with the Attention Unet model proving to be the most effective as shown for the example of glomeruli segmentation in Figure 12 (top panel). Building upon this, the project is now investigating a multi-architecture approach for segmentation. Instead of using a single model to segment all kidney structures, the idea is to employ specialized architectures, each dedicated to segmenting a specific structure. This approach aims to leverage the strengths of individual architectures and enhance the overall accuracy of the segmentation process. The next challenge lies in effectively combining the outputs from these individual architectures to generate a comprehensive segmentation map encompassing all kidney structures. To achieve this, the project is exploring various fusion mechanisms, including both neural network-based architectures (mainly the self-supervised learning paradigm) and traditional statistical methods. While the initial work focused on segmenting image patches, the ultimate goal is to extend this capability to whole-slide images (WSIs) (example in Figure 12, bottom panel). WSIs are the standard format used by pathologists for diagnosis, and the ability to segment these images directly will significantly enhance the clinical applicability of the research.
Concerning the work on glomeruli detection conducted last year, we have executed the experiments on a Paris cohort to test the multicentric property of our approach. The accuracy is slighlty reduced compared to that obtained on the Nice cohort used for the training. However, we were able to increase this accuracy after a domain transfer obtained with a generative adversial network (GAN).
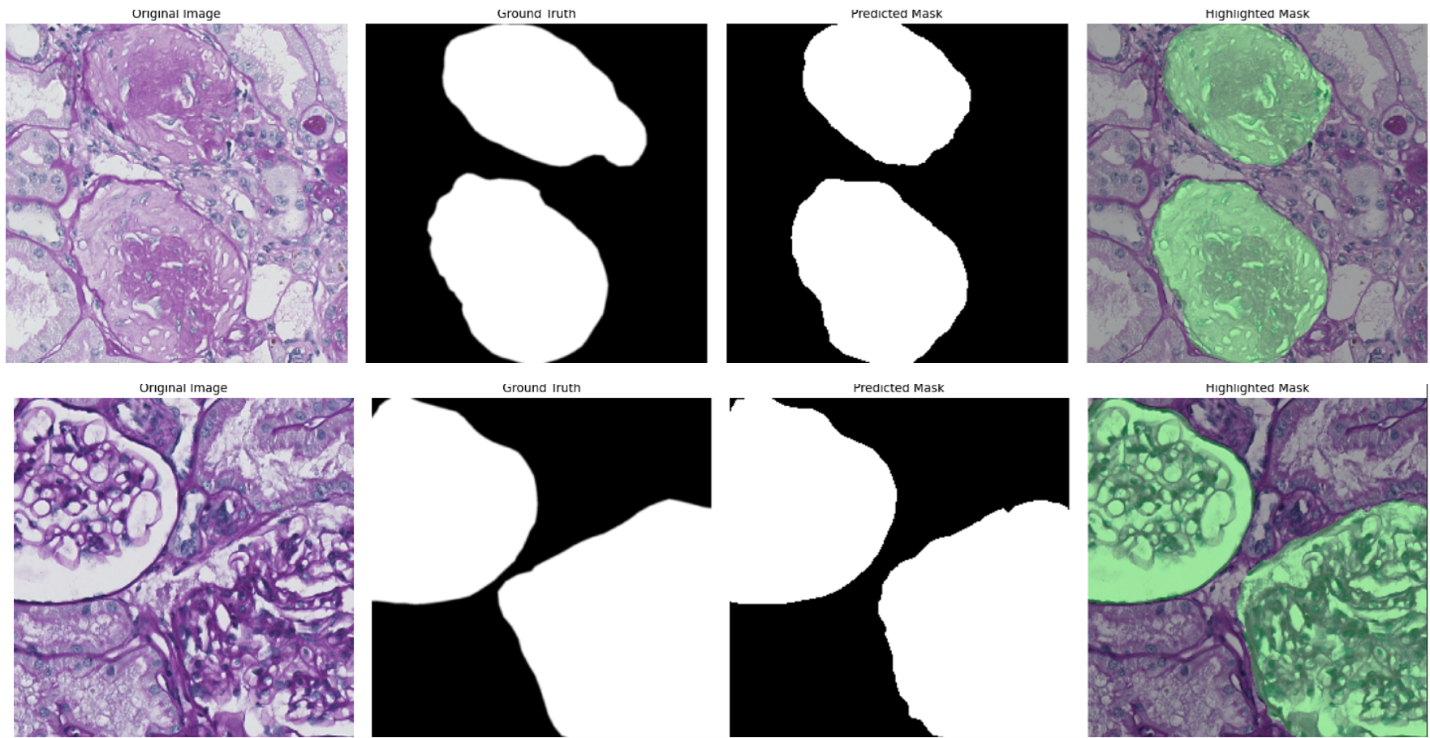
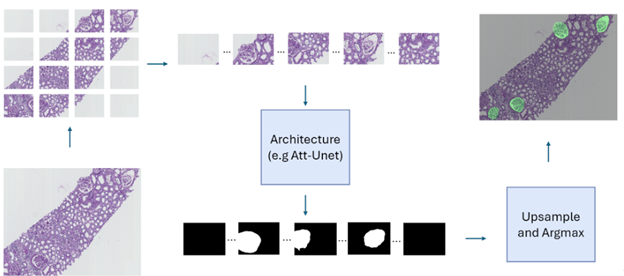
7.14 Reinforcement Learning in histopathology
Participants: Mohamad Mohamad, Xavier Descombes, Damien Ambrosetti [CHU, Nice], Nicolas Pote [Hôpital Bichat, Paris], Maxime Gassier [Hôpital Bichat, Paris].
Drawing inspiration from how pathologists perform diagnoses, we sought to model the whole-slide image (WSI) diagnostic problem as a decision-making one using reinforcement learning (RL). In a typical RL problem, two key components are involved: an environment and an agent, as illustrated in Figure 13. The agent starts in a given state performs an action causing the environment to transition dynamically to a new state, while receiving a reward. Our first step was to bring this interaction-driven dynamic to histopathology by developing a modular and general RL environment tailored to managing WSIs, termed HistoRL. This environment is designed to support a wide range of WSI diagnostic applications, making it a versatile framework for implementing and testing various applications. We created a toy environment based on our earlier self-supervised learning (SSL) task. This allowed us to begin the algorithm and pipeline development cycle. We trained an RL agent on several instances of this toy problem and are currently in the development phase, focusing on generalizing the agent to perform well across unseen environments during evaluation. Figure 13 shows some preliminary results. A paper has been accepted and will be presented at BioImagining 2025 conference (Porto Portugal, 2025 February 20-22).
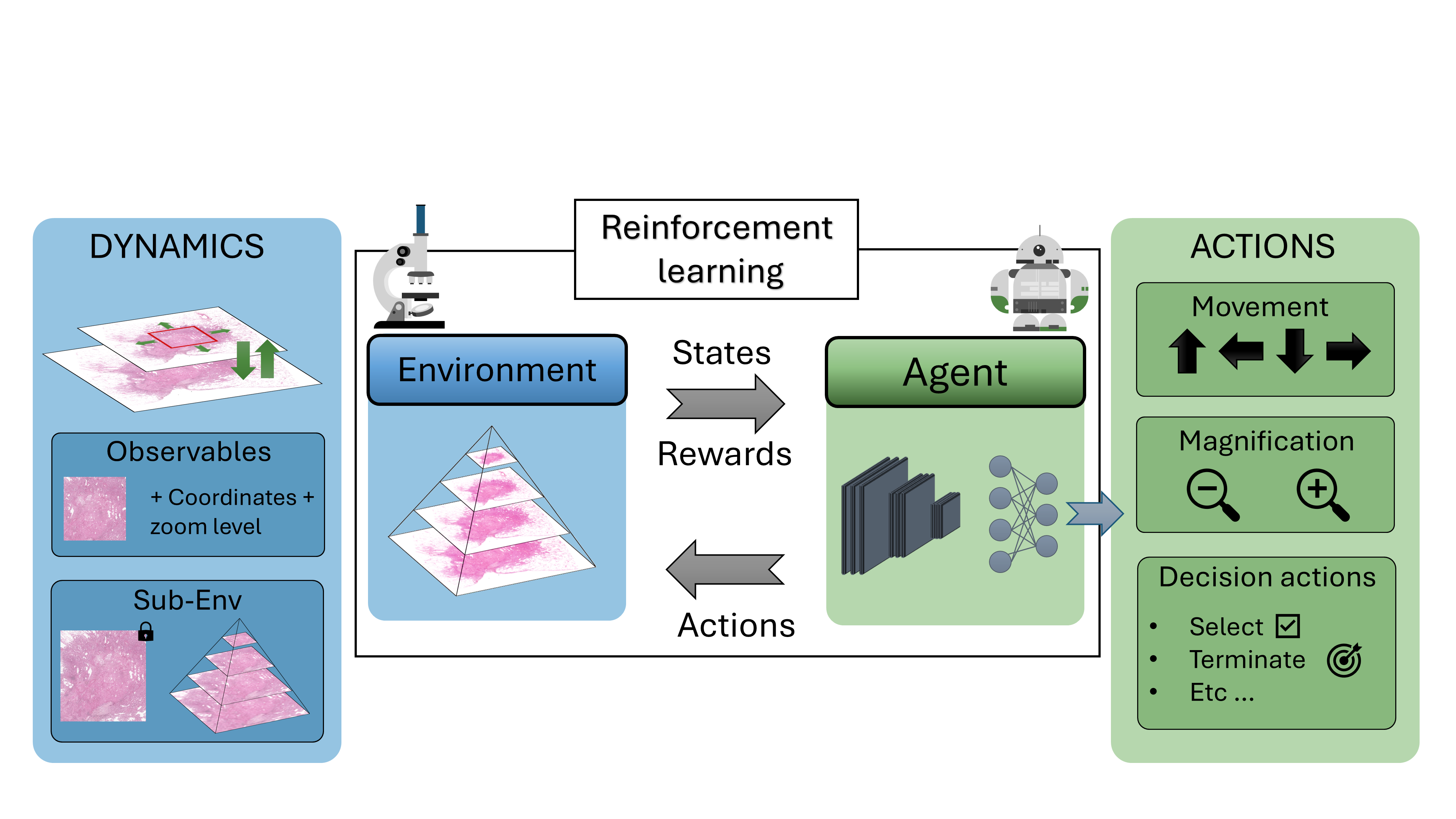
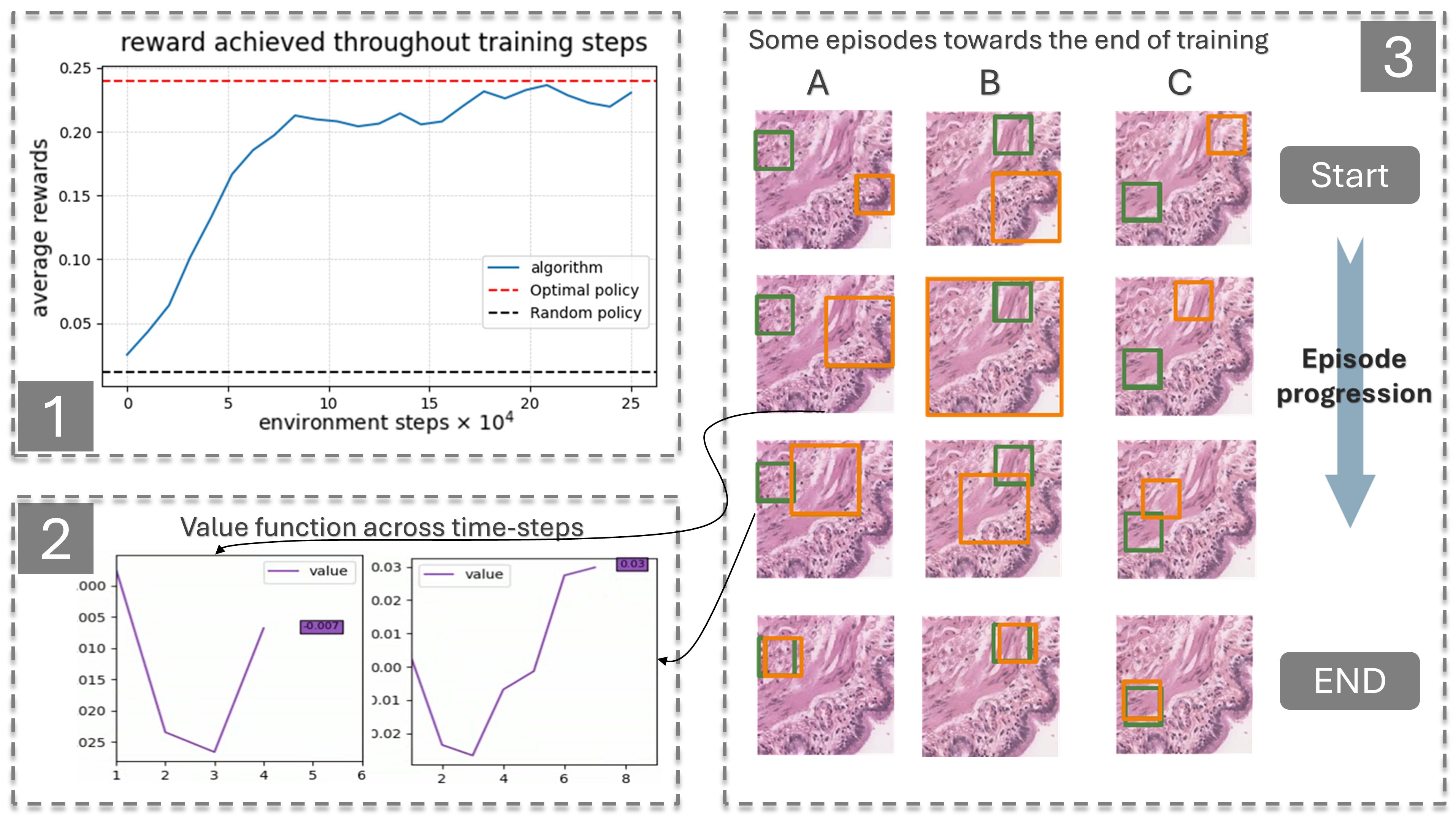
7.15 Linear structure unfolding: application to spatial transcriptomic analysis
Participants: Morgane Fierville, Xavier Descombes, Pascal Barbry [IPMC, Sophia Antipolis], Kevin Lebrigand [IPMC, Sophia Antipolis].
Spatial transcriptomics allow serial analyses of the expression of hundreds of genes in tissue sections by a combination of molecular biology and imaging approaches. This technique allows the exploration of large tissue sections (up to 3 cm
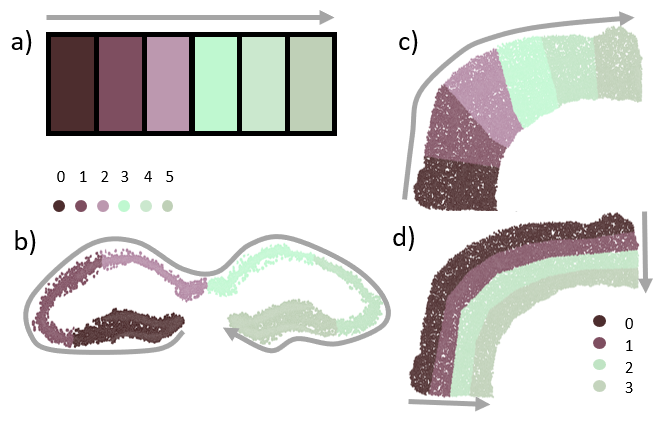
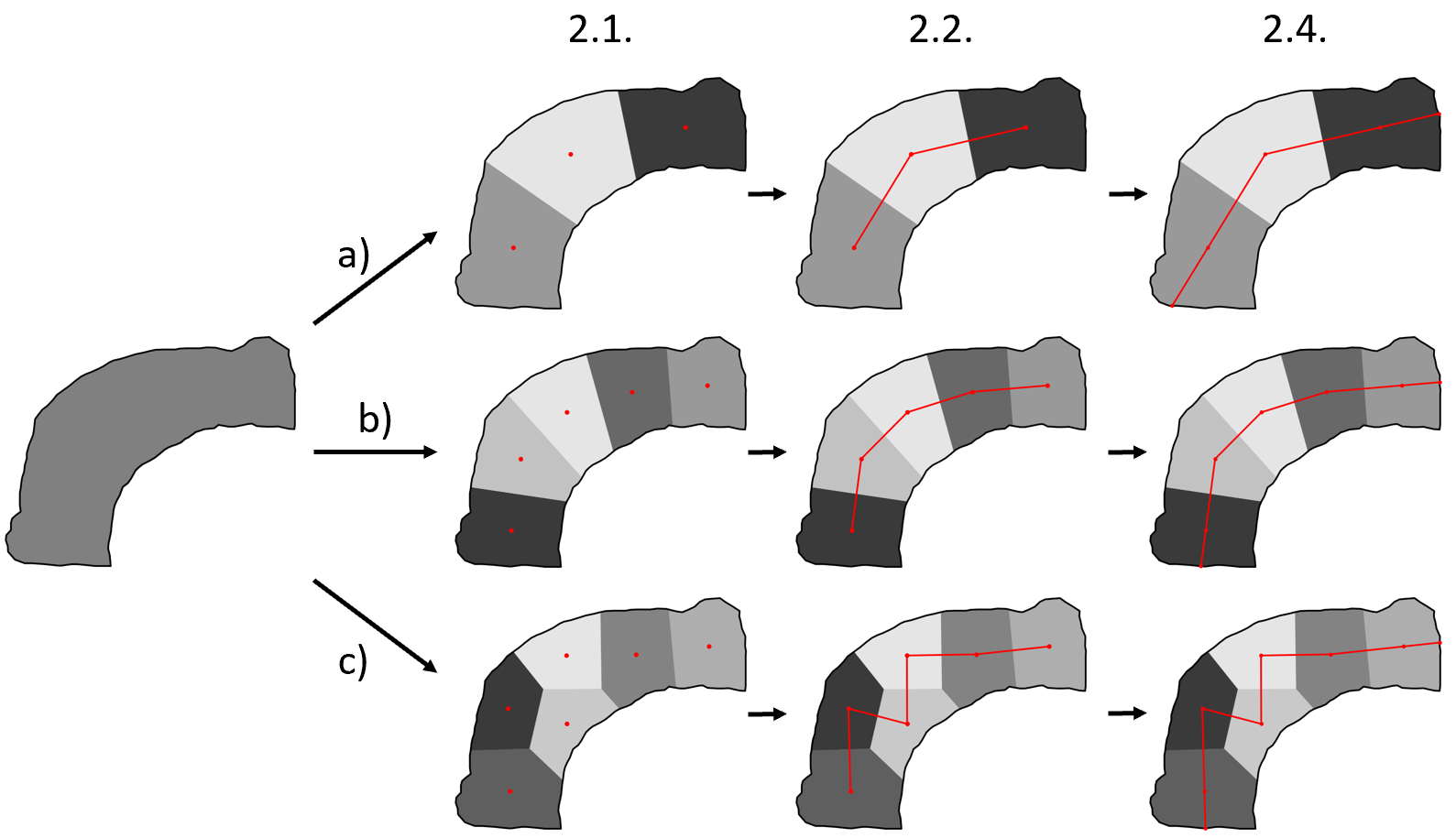
7.16 Detection and Segmentation of Fibronectin Structures in the Tumor Extracellular Matrix
Participants: Faisal Jayousi, Xavier Descombes, Laure Blanc-Féraud, Ellen Van Obberghen-Schilling, Emmanuel Bouilhol, Anne Sudaka [CAL, Nice].
This work is about the development of a computational pipeline for the automated detection and characterization of Fibronectin (FN) in tumor tissue on fluorescence images. FN plays a significant, yet under-explored role in tumor biology. Understanding its geometric and topological properties could provide valuable insights into cancer patients’ level of resistance to immunotherapy. The objective of this work is to design a computational pipeline for the automated detection of fibrillar FN and to propose discriminant statistical measures capable of characterizing its geometry and topology. We worked on FN from normal mouse embryo cultured fibroblasts (see Figure 15(a)) and cells treated with TGF-β1 to mimic the tumor-like phenotype of carcinoma-associated fibroblasts. From the fluorescent images of FN, a pipeline is developed to construct a graph representing the FN on which quantitative measures repesenting the geometry of the FN are computed. Statisitcs computed on 360 images show that the most discriminant features are alignment and branch length.
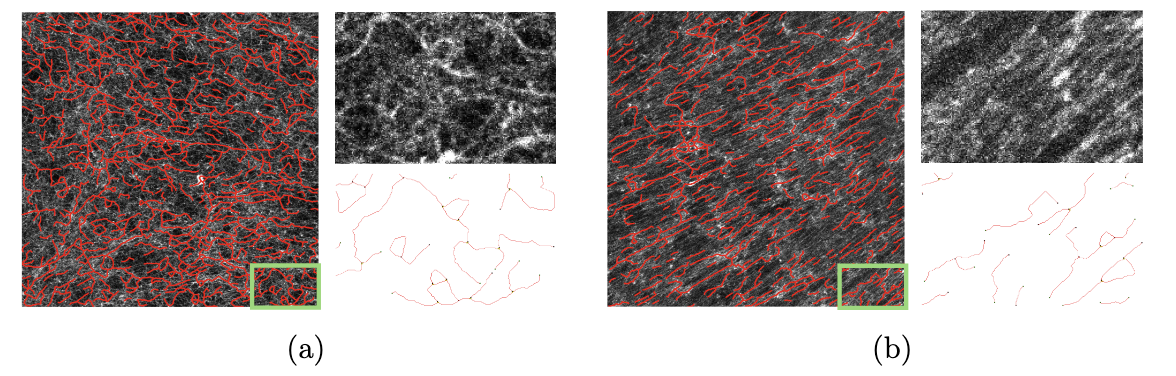
Images of FN in decellularized ECM (Extra Cellular Matrix) produced by (a) control, or (b) TGF-β1- treated, tumor-like fibroblasts and their corresponding graph representation (in red)
Future efforts will focus on adapting the pipeline for clinical immunofluorescence images, addressing challenges such as increased heterogeneity and artifacts. The open-source implementation of the pipeline (FibreSight) is available on GitHub.
7.17 Detecting symmetry in ascidian embryo
Participants: Grégoire Malandain, Patrick Lemaire [CRBM, Montpellier].
Ascidians are an appealing model to study embryogenesis since the late 19th century: they are close relative to vertebrates, some species have transparent embryos which makes them suitable for observation, and, more remarkably, embryos have a stereotyped development during the first stages, even across species. This stereotypy allowed to aggregate information from a population and compare development between individuals, thanks to a cell nomenclature 23.
For each newly acquired embryo, this requires to name its cells. This can be done by name transfer, assuming cells can be paired between two different segmented embryos. Embryos co-registration can achieve efficiently this pairing. However, the tesselated structure of embryos impairs the ability of iterative registration methods to find the sought pairing if the initial pose is out of the right convergence basin.
Exploring a (discretized) space of 3D rigid transformations is surely a way not to miss the sought transformation, but at a high computational cost. Alternatively we can take advantage of the symmetrical nature of the embryos, by aligning the symmetry planes and then only discretize the set of 2D rotations: this dramatically reduces the number of initial transformations to be tested.
In 14, we demonstrate that the cell surface contacts can define a quasi-unambiguous cell signature, and thus allows to recognize pairs of symmetrical cells, which, in turn, allow to characterize the embryo symmetry plane.
7.18 Population study of ascidian embryos
Participants: Haydar Jammoul, Grégoire Malandain, Kilian Biasuz [CRBM, Montpellier], Patrick Lemaire [CRBM, Montpellier].
During the embryogenesis, ascidian exhibit a highly stereotyped development 22. In addition, their developmental speed (rate of cell count increase) is highly consistent across embryos, up to a linear normalization (Figure 16), even if the cell division order is different between any two embryos. It suggests that the ascidian development is highly canalized, and may be simulated by a few simple rules. Eg, drawing cell lifetimes from empirical distributions allows to mimic qualitatively the number of cells of developing embryos. Our first results demonstrate that the development is governed by the cell lifetime. Additionaly, we demonstrated that, after cell division, the lifetimes of the sister cells are correlated, which was not described in the literature.
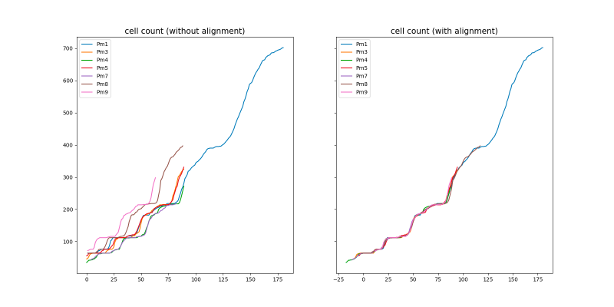
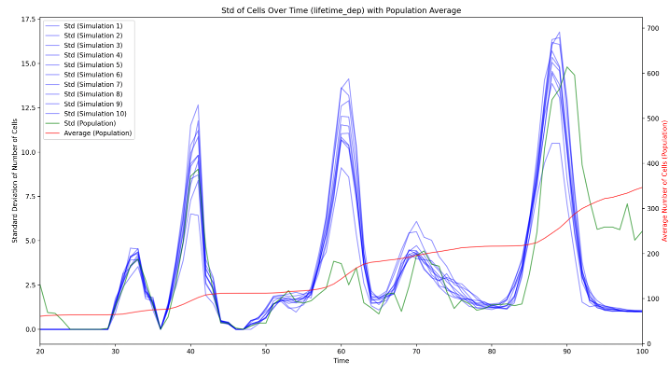
8 Partnerships and cooperations
8.1 International initiatives
8.1.1 Participation in other International Programs
Projet CAPES COFECUB ROAPI
Participants: Laure Blanc-Féraud.
-
Partner Institution(s):
- Campinas university, Brasil
- Paris-Saclay university (Satie Lab)
-
Date/Duration:
December, two weeks
-
Additional info/keywords:
sparse optimization, antenna processing, Direction of Arrival (DOA).
8.2 International research visitors
8.2.1 Visits of international scientists
Other international visits to the team
Ulugbek Kamilov
-
Status
researcher
-
Institution of origin:
Washington University in St. Louis
-
Country:
USA
-
Duration:
two days
-
Context of the visit:
visit and seminar
Francesco Ponzio
-
Status
researcher
-
Institution of origin:
Politecnico Torino
-
Country:
Italy
-
Duration:
two days
-
Context of the visit:
visit for co-supervision of students
8.2.2 Visits to international teams
Research stays abroad
- Luca Calatroni visited for a week the team GAIM (Group for Artificial Intelligence and Sparse Modelling) in Ghent, Belgium during the period June 17-21, 2024.
8.3 National initiatives
8.3.1 Labex SIGNALIFE
The Morpheme team belongs to the Labex (Laboratory of Excellence) Signalife. This Labex embeds four biology institutes in Nice, Sophia Antipolis and two Inria teams.
8.3.2 ANR JCJC TASKABILE
Participants: Luca Calatroni [PI].
This project is in collaboration with Alessandro Lanza (University of Bologna, IT) and Thomas Moreau (Inria Saclay, FR). In this project, we propose to improve upon the intrinsic specificity and rigidity of standard bilevel learning approaches by means of a novel task-adapted highly-flexible modeling. To mitigate the computational efforts required for solving bilevel problems numerically, tailored inexact and stochastic optimization schemes is studied. The following questions are addressed:
- The definition of novel, possibly non-smooth and non-convex task-adapted (not only reconstruction-based) quality measures inspired by empirical evaluation metrics and visual perception;
- The use of flexible statistical distributions as a more plausible prior model describing image statistics and their comparison with highly-generalizable deep architectures;
- The use of inexact and stochastic first-order optimization algorithms computing solutions of bilevel schemes with reduced computational efforts and controlled accuracy.
The project features an international consolidated skilled team of researchers with strong expertise in applied imaging inverse problems, non-smooth & non-convex optimization and statistical image processing.
8.3.3 ANR PRC MICROBLIND
Participants: Luca Calatroni, Laure Blanc-Féraud.
This project is a collaborative project led by Pierre Weiss (IMT, Toulouse)[PI].
Several recent revolutions in imaging rely on numerical computations. One can think of single molecule localization microscopy (Nobel Prize 2014) or cryo-electron microscopy (Nobel Prize 2017). What they have in common is the need to perform prior mathematical modeling and calibration of the system. Although they have made it possible to observe phenomena that were previously out of reach, their expansion is currently limited by an important problem: it is difficult to precisely control the imaging conditions (e.g. temperatures, wavelengths, refractive indices). This results in modeling errors that can have disastrous repercussions on the quality of the images produced. Thus, these technologies are currently reserved for a handful of research centers possessing state-of-the-art equipment and considerable interdisciplinary experience. The objective of this project is to bring new theoretical and numerical solutions to overcome these difficulties, and then to apply them to different optical microscopes. This should allow to democratize their use, to reduce their cost and the preparation time of the experiments.
The central idea is to characterize a measurement device, not by a single operator (e.g. a convolution), but by a small dimensional family allowing to model all possible states of the system. To our knowledge, this idea has been very little explored so far and opens many difficult questions: how to best evaluate this family experimentally and numerically? How to identify the state of the system from indirect noisy observations? How to exploit this information to reconstruct images in short computing times? We have begun to explore these questions in recent works and wish to continue this effort using tools from optimization, harmonic analysis, probability and statistics, algebraic geometry, machine learning and massively parallel computing. We hope to make significant advances in the field of blind inverse problems. We will validate them on photonic microscopy problems in collaboration with opticians, responsible for two microscopy platforms in Nice and Toulouse. This allows us to obtain direct feedback for real problems in biology. We particularly study the problems of super-resolution by single molecule, multi-focal localization and blind structured illumination. Moreover, several companies in the Toulouse area (INNOPSYS, IMACTIV-3D, AGENIUM), provide us with data from their microscopes (line scanning microscope, light sheet fluorescence microscope), which will ensure direct transfers to industry.
8.3.4 ANR MORPHEUS
Participants: Xavier Descombes [PI], Grégoire Malandain, Alexandre Martin, Ivan Magistro Cotenta.
In this project, we propose to use the cutting-edge organoid technology to test the toxicity of endocrine disruptors (EDCs) on human organs. The aim is to develop computational tools and models to allow the use of organoid technology for EDC toxicity testing. The project is thus divided in two main objectives: to build up and analyze a phenotypic landscape of EDC effect on organoid and to develop explicative or predictive models for their growth. The first goal is to define and construct a phenotypic map of organoids, modeled as graphs (the nodes representing the cells and edges adjacency between them) for classifying EDCs families. The second is to classify organoid growth trajectories on this map. We will consider two organoid models, gastruloids and prostate organoids. To derive the phenotypic map, we combine a graph representation and a deep learning approach. The deep learning approach is considered for its discriminating properties whereas a correspondence between the bottleneck layer of the chosen neural network and the stratified graph space brings some explicability to the derived classification.
This 4-years project started in november 2021 and is leaded by X. Descombes. It involves 3 groups: IPMC (S. Clavel, Nice), Metatox, Inserm (X. Coumoul, Paris) and Morpheme.
8.3.5 ANR Cell Whisper
Participants: Grégoire Malandain.
Successful embryogenesis requires the differentiation of the correct cell types, in defined numbers and in appropriate positions. In most cases, decisions taken by individual cells are instructed by signals emitted by their neighbors. A surprisingly small set of signalling pathways is used for this purpose. The FGF/Ras/ERK pathway is one of these and mutations in some of its individual components cause a class of human developmental syndromes, the RASopathies. Our current knowledge of this pathway is, however, mostly static. We lack an integrated understanding of its spatio-temporal dynamics and we can imperfectly explain its highly non-linear response to a graded increase in input stimulus.
This systems biology project combines advanced quantitative live imaging, pharmacological/optogenetics perturbations and computational modeling to address 3 major unanswered questions, each corresponding to a specific aim:
- Aim 1: What is the spatio-temporal dynamic of intracellular signal transduction in response to FGF (Fibroblast Growth Factor) ?
- Aim 2: What is the molecular basis of the switch-like response to graded extracellular signals?
- Aim 3: Can the results be integrated into a predictive computational model of the pathway?
Through this approach, in a simplified model system, we hope to gain an integrated view of the spatio-temporal dynamics of this pathway and of its robustness to parameter variations. Participants are CRBM (Montpellier), LIRMM (Montpellier), MOSAIC (INRIA Lyon) and Morpheme.
8.3.6 Targeted Project Filling Gaps
Participants: Grégoire Malandain, Anna Kestel.
This targeted project, "Filling the gaps between scales to understand biomass properties", is issued from the PEPR B-Best.
The architecture of biomass is highly complex and can be defined as a continuum of length-scales from molecules to particles, including polymers, nano-structures, assemblies, cells, and/or tissues. These scales are strongly interconnected and reflect not only chemical and structural properties of biomass but most importantly their reactivity to transformation processes such as chemical, physical, mechanical or biological reactions.
The goal of this project is to identify and quantify markers at different scales in order to be able to propose a generic model (at least for each biomass type considered) that describes and predict their properties and possibly their reactivity (at the chemical, biological, physical levels), with a focus on lignocellulosic and algal biomass. Morpheme team will address the image analysis issues.
8.3.7 3IA Senior chair, "Imaging for Biology"
Participants: Laure Blanc-Féraud.
Recent advances in microscope technology provide outstanding images that allow biologists to address fundamental questions. This project aims at developing new AI methods and algorithms for (i) novel acquisition setups for super-resolution imaging, and (ii) extraction of valuable quantitative information from these large heterogeneous datasets. More precisely we search for biomarkers in multispectral fluorescence images of tumor tissues to predict the response of immunotherapy in head and neck cancers.
8.4 Regional initiatives
8.4.1 MathArchi
Participants: Xavier Descombes [Co-PI], Zhenyu Zhu.
This project is in collaboration with IPMC (Frédéric Luton). It is supported by Academy 4 of IDEX JEDI (université Côte d'Azur). The goal is to characterize the basal lamina by analyzing 3D confocal images of spheroid of breast cancer. It includes detecting pores and filipodes using deep learning and image processing.
8.4.2 Characterization and quantification of cell geometry and topology changes in urchin invagination
Participants: Grégoire Malandain [Co-PI], Alexandre Ricci.
This project, supported by Academy 4 of IDEX JEDI (Université Côte d'Azur), aimed at characterizing geometric and topological changes at the cellular level that occurred during the urchin embryo invagination. It was conducted in collaboration with M. Rauzi (IBV, Nice).
8.4.3 A live imaging screen of the Zebrafish Extracellular Vesicle/Particle secretome
Participants: Santosh Adhikari, Xavier Descombes, Maximilian Fürthauer.
This project, supported by the Canceropole within the programme Emergence, aimed at analyzing and modeling endogeneous particle traffic in the zebrafish. We have developed algorithms for tracking particles from in vivo images.
9 Dissemination
9.1 Promoting scientific activities
9.1.1 Scientific events: organisation
General chair, scientific chair
- Xavier Descombes was general co-chair of EURASIP- IEEE SPS Summer School on Remote Sensing and Microscopy Image Processing, Vezprem, August 2024.
Member of the organizing committees
- Luca Calatroni was member of the organization/scientific committee of: the workshop "Mathematics for Imaging, Vision and their Applications workshop" (Naples, February 2024), Applied Mathematis
Learning Approaches for Cultural Heritage and the Arts (Cagliari, April 2024), the ERASMUS+ BIP school "Mathematics machine learning for image analysis” (Bologna, June 2024), the scientific day " -based minimization: from continuous relaxations and non-convex algorithms to global optimization ” (IHP, Paris, September 2024) and the workshop "Learning and Optimization in Côte d'Azur ” (I3S, September 2024). - Laure Blanc-Féraud was member of the organization/scientific committee of the Biological Image Processing and Analysis (BioIAS) one day colloquium organized in Nice (June 14th).
9.1.2 Scientific events: selection
Member of the conference program committees
- Luca Calatroni was co-organizer of two scientific mini-symposia in the occasion of the international conferences SIAM Imaging sciences (Atlanta, US, May 2024) and European conference on operation research and optimisation (EURO 2024, Copenhagen, July 2024).
Reviewer
- Luca Calatroni was reviewer for the conferences: ICML 2024, ICASSP 2024.
- Eric Debreuve was reviewer for the conference ICIP 2024.
- Grégoire Malandain was reviewer for the conferences ISBI 2024 and EMBC 2024.
- Xavier Descombes was reviewer for the conference IEEE ISBI 2024.
- Laure Blanc-féraud was reviewer for IEEE ISBI 2024, ICASSP ICIP 2024.
9.1.3 Journal
Member of the editorial boards
- Thomas Boudier is associate editor in the journal Frontiers in Computational BioImaging
- Luca Calatroni is associate editor in the journals: Journal of Mathematical Imaging and Vision, Inverse Problems.
- Laure Blanc-Féraud is associate editor for the encyclopedia SCIENCES edited by ISTE/WILEY for the image domain.
Reviewer - reviewing activities
- Thomas Boudier: Review for Nature Communication, F1000 Research
- Eric Debreuve: Review for IEEE Transactions on Medical Imaging
- Eric Debreuve: Review for Springer Multimedia Tools and Applications
- Xavier Descombes: Reviews for Biological Imaging.
9.1.4 Invited talks
- Thomas Boudier was invited speaker at “Biological Image Processing and Analysis (BioIAS)” in Nice on June 14.
- Luca Calatroni was invited speaker for the following seminars/workshops: "Big Data Inverse Problems" workshop ICMS, Edinburgh, May 2024), workshop "Advanced Numerical Analysis for Imaging: from theory to industry" (Naples, June 2024), Ghent University, Belgium, A. Pizurica's group (seminar, September 2024), OIP worksohp (Modena, September 2024), CNR-IMATI seminar (Genova, Italy). He was also invited speaker at mini-symposia at the conferences: SIAM conference on Imaging Sciences (Atlanta, US, May 2024), EURO (Copenhagen, July 2024), European Congress on Mathematics (EMS, Seville, July 2024).
- Laure Blanc-Féraud was invited speaker for the following workshops/summer school: Colloque IABM : Intelligence Artificielle en Imagerie Biomédicale in Grenoble; EURASIP Summer School on Remote Sensing and Microscopy Image Processing in Veszprem Hungary; one scientific day of GdR IASIS on sparse optimization; workshop on Optimization Techniques for Inverse Problems Modena, Italy, 50th birthday of L2S lab.
- Xavier Descombes was invited speaker for “Biological Image Processing and Analysis (BioIAS)” in Nice on June 14, ReinCare in Nice on June 26-28, "Rencontre Thématique Dermatologie" in Sophia Antipolis on November 8 and EURASIP- IEEE SPS "Summer School on Remote Sensing and Microscopy Image Processing", Vezprem, August 2024.
- Morgane Fierville gave a talk at SincellTE 2024 - 5th Workshop in single cell data analyses : Transcriptomics, Spatial and Long-reads and Matching Day Spatial Transcriptomics (October 15, 2024) Cancéropole à Marseille; she gave a flash talk at REBICA 2024 (July 1, 2024, Université Côte d'Azur in Nice).
9.1.5 Leadership within the scientific community
- Laure Blanc-Féraud: member of the IEEE Bio Imaging and Signal Processing Technical Committee (BISP).
- Head of Axis 3 IA for Biology and Bio-inspired IA at 3IA Côte d'Azur, Laure Blanc-Féraud.
- Luca Calatroni is elected member of the IEEE Bio Imaging and Signal Processing Technical Committee (BISP).
- Xavier Descombes is Vice-President of the ANR committee CE45, he is member of the Scientific Committee of Labex Signalife.
- Grégoire Malandain: member of the IEEE Biomedical Imaging and Image Processing Technical Committee (BIIP); member of the scientific committee of the MathNum department of INRAE.
9.1.6 Scientific expertise
- Luca Calatroni was member of the committee for the assignment for the best paper award in the occasion of the SMAI-MODE days in Lyon (March 2024).
- Eric Debreuve reviewed two ANR projects of the AAPG 2024 in panel CE45 - Interfaces: mathematics, digital sciences - biology, health.
9.1.7 Research administration
- Laure Blanc-Féraud is member of the Scientific and pedagogic committee (CoSP) of EUR DS4H, vice-chair of Academy 1 of Idex UCA JEDI, responsible of axis on biology of the 3IA Côte d'Azur Institute, member of steering committee of IRIT lab, member of the scientific committee of GdR IASIS, member of a COS at UniCA, member of committee selection for chairs of UniCA Idex/Programme de recherche Avancées.
- Xavier Descombes is member of the PhD committee selection of the EUR DS4H.
- Haydar Jammoul contributed to the organization of the INRIA PhD seminars at INRIA Sophia Antipolis.
9.2 Teaching - Supervision - Juries
9.2.1 Teaching
- Licence: Florence Besse, Subcellular RNA targeting, 4h, L3, ENS Ulm, France
- Master: Florence Besse, Neurobiologie cellulaire et moléculaire, 6h, M1/2, Université Côte d'Azur, France
- Master: Florence Besse, Outils génétiques pour l'étude des circuits neuronaux, 4h, M1/2, Université Côte d'Azur, France
- Master: Florence Besse, Transport axonal, 2h, M1/2, Université Côte d'Azur, France
- Master: Laure Blanc-Féraud, module Machine Learning for Image processing and module Applied AI of master SD, 12h Eq. TD, Niveau M2, Université Côte d'Azur, France.
- Master: Eric Debreuve, Scientific image processing and Machine learning, Master SVS, 15h EqTD, M1 + M2, Université Côte d'Azur, France
- Master: Xavier Descombes, Traitement d'images, Analyse de données, Techniques avancées de traitement d'images, 10h EqTD, Niveau M2, ISAE, France.
- Master: Xavier Descombes, Machine Learning and Image Processing, Polytech Sophia, master, 9h Eq. Université Côte d'Azur, France.
- Master: Xavier Descombes, Bio-imagerie, master IRIV, 6h EqTD, Niveau M2, Université de Strasbourg, France
- Master: Xavier Descombes, Image Analysis, master GBM, 9h EqTD, Niveau M2, Université Côte d'Azur, France.
- Master: Xavier Descombes, Image processing and Machine learning for Biology, master SVS, 15h Eq.TD, Niveau M2, Université Côte d'Azur.
- Master: Xavier Descombes, Artificial Intelligence for Histopathologist, master oncology, 3h Eq. TD, Niveau M2, Université Côte d'Azur.
- Master: Mohamad Mohamad, Introduction to Machine Learning and Deep Learning, DU AI and Health, 3h Eq. TD, Niveau M2, Université Côte d'Azur.
- Master: Alexandre Martin, Polytech Nice, computer Science Master ; Machine Learning for Image Analysis (4.5h) and Applied AI (1.5h)
- Master: Mohamad Mohamad, Polytech Nice, computer Science Master ; Machine Learning for Image Analysis (4.5h) and Applied AI (1.5h), Unica, : Génie Biomédical (9h).
- Licence: Morgane Fierville, Introduction à l’informatique, Licence Sciences de la vie, 20h, Niveau L2, Université Côte d’Azur.
- Licence: Morgane Fierville, Bio-informatique, Licence Sciences de la vie, 14h, Niveau L3, Université Côte d’Azur.
- Master : Morgane Fierville, Programmation Python et environnement Linux, Master and Licence Sciences du Vivant, 20h, Niveau L3-M1, Université Côte d’Azur.
- Polytech GB3: Morgane Fierville, ECUE Analyses bio-informatiques de séquences biologiques, Polytech GB3, 9h, Université Côte d’Azur.
- Master: Luca Calatroni, Laure Blanc-Féraud, Faisal Jayousi, Inverse problems in image processing, niveau M2, 30h, MSc DSAI, Université Côte d'Azur, France.
- Master: ECUE histoire de l'histoire de l'art: Luca Calatroni, Where arts & mathematics meet, niveau M1, 2h, Université Côte d'Azur, France.
- Faisal Jayousi, Numérisation de l'information, BUT1 RT, (43.5h).
- Faisal Jayousi, Theory of Statistical Learning. MSc 2 Data Science & Artificial Intelligence, (12h).
- Faisal Jayousi, Statistiques & Régression Linéaire. Licence Économie et Gestion, (18h).
- Aneva Tsafack, Processus stochastique pour l'ingénieur (36h), MAM 4,Polytech Nice Sophia Antipolis, Université Côte d'Azur.
- Aneva Tsafack, MAM 5, Reinforcement learning (7h30), data valorization (20h), Polytech Nice Sophia Antipolis, Université Côte d'Azur.
- Hamza Mentagui, Centrale Méditerranée Bachelor, Python (32h)
- Haydar Jammoul, Université Côte d'Azur, L1, Python (64 hours).
- Centrale Méditerranée Bachelor: Thomas Boudier, Computer Sciences and Programming (140h).
- Centrale Méditerranée Centrale, DigitalLab (Master Level): Thomas Boudier, Programming (40h).
- Centrale Méditerranée Centrale, DigitalLab (Master Level): Thomas Boudier, direction.
9.2.2 Supervision
- Luca Calatroni is the PhD co-supervisor of: H. Mentagui (with L. Blanc-Féraud), M. Essafri (with E. Soubies), P. Saillard (with R. M. Dessì, M. Corneli). He was supervisor of the master internships of: C. Fantasia, C. Daniele, A. Xu and S. M'Nasri.
- Eric Debreuve was the PhD co-supervisor of Asma Chalabi (with J. Roux, IPMC, Sophia Antipolis); Defense: May 2024.
- Laure Blanc-Féraud is co-supervisor of the PhD thesis of Hamza Mentagui (with Luca Calatroni), Faisal Jayousi (with Xavier Descombes), Aneva Tsafack (with Gilles Aubert).
- Xavier Descombes is the PhD supervisor of Alexandre Martin, Mohamad Mohamad; he is co-supervisor of Faisal Jayousi (with Laure Blanc-Féraud), Morgane Fierville (with Pascal Barbry, IPMC) and Meryem Sikouky (with Thomas Boudier).
- Grégoire Malandain is the co-supervisor of the PhD thesis of H. Jammoul (with P. Lemaire, CRBM, Montpellier) and A. Kestel (with Y. Refahi and G. Paes, INRAE, REIMS)
9.2.3 Juries
- Luca Calatroni was part of the PhD jury of the following students: S. Willingham (MidSweden University + Inria, Rennes), mid-evalation; G. Giacchi (University of Bologna + Engineering school of Sion, CH), mid-term evaluation; J. Chirinos-Rodriguez (University of Genoa); G. Monteverde and G. Dominijanni (University La Sapienza, Rome).
- Laure Blanc-Féraud was reviewer of 2 PhD thesis and 2 HDR, and member of 2 Phd thesis.
- Xavier Descombes was reviewer of 3 PhD thesis and 1 HDR, president of 1 PhD thesis defense and member of 1 PhD thesis jury. He was member of 4 CSI (mid-term evaluation of PhD).
10 Scientific production
10.1 Major publications
-
1
articleA continuous relaxation of the constrained
problem.Journal of Mathematical Imaging and VisionJanuary 2021HAL - 2 articleFluorescence image deconvolution microscopy via generative adversarial learning (FluoGAN).Inverse Problems395April 2023, 054006HALDOIback to text
- 3 articleDetecting and quantifying stress granules in tissues of multicellular organisms with the Obj.MPP analysis tool.TrafficJuly 2019HALDOI
- 4 articleMultiple objects detection in biological images using a marked point process framework.Methods2016HALDOI
- 5 articleFibronectin Extra Domains tune cellular responses and confer topographically distinct features to fibril networks.Journal of Cell ScienceFebruary 2021HAL
- 6 articleContact area-dependent cell communication and the morphological invariance of ascidian embryogenesis.ScienceJuly 2020HALDOI
10.2 Publications of the year
International journals
- 7 articleParameter-Free FISTA by Adaptive Restart and Backtracking.SIAM Journal on Optimization344October 2024, 3259-3285HALDOIback to text
- 8 articleSpot Spine, a freely available ImageJ plugin for 3D detection and morphological analysis of dendritic spines.F1000Research13September 2024, 176HALDOI
- 9 articleAn image-based RNAi screen identifies the EGF.R signaling pathway as a regulator of Imp/ IGF2BP RNP granules.Journal of Cell ScienceOctober 2024, Online ahead of printHALDOI
- 10 articleDermoGAN: multi-task cycle generative adversarial networks for unsupervised automatic cell identification on in-vivo reflectance confocal microscopy images of the human epidermis.Journal of Biomedical Optics29082024, 086003HALDOI
- 11 articleDeep image prior inpainting of ancient frescoes in the Mediterranean Alpine arc.Heritage Science121February 2024, 41HALDOI
International peer-reviewed conferences
- 12 inproceedingsOn L0 Bregman-Relaxations for Kullback-Leibler Sparse Regression.IEEE XploreMLSP 2024 - IEEE 34th International Workshop on Machine Learning for Signal Processing2024 IEEE 34th International Workshop on Machine Learning for Signal Processing (MLSP)London, United KingdomIEEESeptember 2024, 1-6HALDOIback to text
- 13 inproceedingsDetection and Characterisation of Fibronectin Structures in the Tumour Extracellular Matrix.EUSIPCO 2024 - 32nd European signal processing conferenceLyon, FranceAugust 2024HAL
- 14 inproceedingsDetecting symmetry in ascidian embryo.ISBI 2024 - 21th International Symposium on Biomedical ImagingAthenes, Greece2024, 4HALback to text
- 15 inproceedingsPhysics-inspired generative adversarial modelling for fluctuation-based super-resolution microscopy.IEEE XploreISBI 2024 - 2024 IEEE International Symposium on Biomedical Imaging2024 IEEE International Symposium on Biomedical Imaging (ISBI)Athens, GreeceIEEEMay 2024, 1-4HALDOIback to text
Conferences without proceedings
- 16 inproceedingsA 4D quantification pipeline to characterize plant cell wall enzymatic hydrolysis in highly deconstructed samples.RFP 2024 - 14èmes journées du Réseau Français des ParoisSaint Malo, France, FranceAugust 2024HAL
- 17 inproceedingsWhiteness-based bilevel learning of regularization parameters in imaging.2024 32nd European Signal Processing Conference (EUSIPCO)Lyon, FranceOctober 2024HAL
Doctoral dissertations and habilitation theses
- 18 thesisOptimisation algorithms in non-standard Banach spaces for inverse problems in imaging.Université Côte d'Azur; Università degli studi (Gênes, Italie)April 2024HALback to text
Reports & preprints
- 19 miscAlgorithmic unfolding for image reconstruction and localization problems in fluorescence microscopy.March 2024HAL
- 20 miscExact continuous relaxations of l0-regularized criteria with non-quadratic data terms.February 2024HALback to text
- 21 miscOff-the-grid regularisation for Poisson inverse problems.January 2025HALback to text
Other scientific publications
- 22 inproceedingsDevelopmental stereotypy assessment in ascidian embryos.AI & imaging for Cell and developmental biology: Insights, limits and perspectivesParis, FranceSeptember 2024HALback to text
10.3 Cited publications
- 23 bookThe organization and cell-lineage of the ascidian egg / by Edwin G. Conklin..Philadelphia :[Academy of Natural Sciences],1905, 182URL: http://www.biodiversitylibrary.org/item/24005back to text