2024Activity reportProject-TeamAISTROSIGHT
RNSR: 202324373X- Research center Inria Lyon Centre
- In partnership with:Université Claude Bernard (Lyon 1), Hospices Civils de Lyon - Centre Hospitalier de Lyon, Theranexus
- Team name: Viewing neuron-astrocyte pharmacology through digital sciences
- Domain:Digital Health, Biology and Earth
- Theme:Computational Neuroscience and Medicine
Keywords
Computer Science and Digital Science
- A3.1.1. Modeling, representation
- A3.2.2. Knowledge extraction, cleaning
- A3.2.4. Semantic Web
- A3.3.2. Data mining
- A3.4.1. Supervised learning
- A3.4.6. Neural networks
- A3.4.8. Deep learning
- A6.1.1. Continuous Modeling (PDE, ODE)
- A6.1.2. Stochastic Modeling
- A6.1.3. Discrete Modeling (multi-agent, people centered)
- A6.1.4. Multiscale modeling
- A9.2. Machine learning
- A9.8. Reasoning
- A9.10. Hybrid approaches for AI
Other Research Topics and Application Domains
- B1.1.2. Molecular and cellular biology
- B1.1.7. Bioinformatics
- B1.1.8. Mathematical biology
- B1.1.10. Systems and synthetic biology
- B1.2.1. Understanding and simulation of the brain and the nervous system
- B1.2.3. Computational neurosciences
- B2.2.2. Nervous system and endocrinology
- B2.6.1. Brain imaging
1 Team members, visitors, external collaborators
Research Scientists
- Hugues Berry [Team leader, INRIA, Senior Researcher, HDR]
- Audrey Denizot [INRIA, Researcher]
- Thomas Guyet [INRIA, Associate Professor Detachement, HDR]
- Leonardo Trujillo Lugo [INRIA, Starting Research Position, from May 2024]
Faculty Member
- Luc Zimmer [UNIV LYON I, Hospital Staff, PU-PH, HDR]
Post-Doctoral Fellow
- Maelle Moranges [INRIA, Post-Doctoral Fellow, from Oct 2024]
PhD Students
- Ismail Bachchar [ORANGE, from Apr 2024]
- Schayma Ben Marzougui-El Garrai [INRIA]
- Andrea Ducos [INRIA]
- Florian Dupeuble [INRIA]
- Arnaud Hubert [INRIA]
- Nathan Quiblier [INRIA]
- Hana Sebia [INRIA]
Technical Staff
- Francois-Elie Calvier [INRIA, Engineer]
- Lucas Perret [INRIA, Engineer, from Jul 2024]
- Jan-Michael Rye [INRIA, Engineer]
Interns and Apprentices
- Zoe Laffitte [INRIA, Intern, until Jul 2024]
Administrative Assistant
- Noemie Rodrigues [INRIA]
External Collaborators
- Arnaud Duvermy [Fondation AP-HP, from Jun 2024]
- Benjamin Vidal [CERMEP]
2 Overall objectives
2.1 Failures in drug development for neurological diseases
The set of available drugs for neurological diseases is both aging and lacking in effectiveness. There remains a very high unmet medical need for treatments in neurology, despite heavy historical investment in the field 21, 52. A typical drug development process includes a set of successive stages (fig. 1), sequentially evaluating the effects of the candidate drug in vitro on cell culture models, then in vivo on animal models (pre-clinical), after which the mechanistic origins of the candidate drug effect can be assessed in animals (sometimes in humans). The next stage consists of studies in humans based on clinical trials that are themselves structured in successive phases: phase I to test for safety, phase II to determine the effect of the candidate on a small set of patients and phase III that includes large cohorts of patients and control people in a randomized setting. Each and every stage of this process has significant probability to fail and break off the development of the drug under process. With the recent public health issues (HIV, Covid-19) the general public has mostly been made aware of failures between the successive phases of the clinical trial stage. However, in the field of neurology, the difficulties in developing drug candidates are mainly due to a high failure rate in the clinic: the activity of the drug candidate in in vitro cell cultures or in animal models is very often not confirmed in humans 36, 40.
In recent years, numerical approaches have been proposed in the field, either with mechanistic modeling to predict the response of the cell to the candidate molecule (quantitative systems biology/pharmacology) 47, 53 or with machine learning to identify the impacted (sub)cellular systems or the effects of the candidate drug 61, 42. However, these approaches are still inefficient to meet the above challenges because they often address a unique scale or modality of interest (e.g., molecular, cellular, preclinical) and lose their predictive power at other scales (e.g. clinical, i.e. the patient). The main methodological objective of AIstroSight is to develop quantitative systems biology and Artificial Intelligence (AI) approaches able to embrace several of these scales.
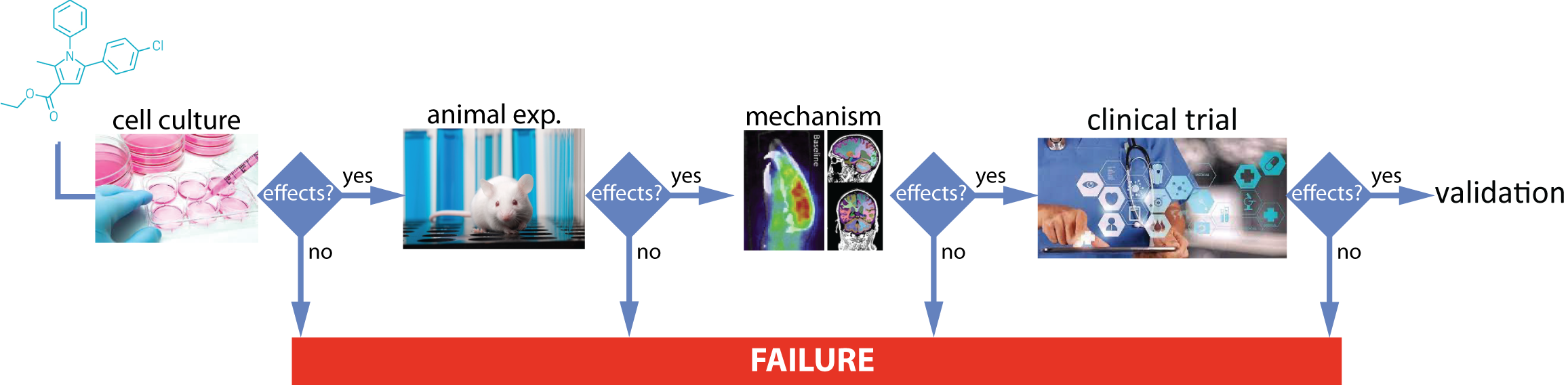
A schematic view of a drug development process
2.2 Main deliverables
Our overall goal is to develop innovative numerical methods for neuropharmacology that will provide us with levers to accelerate and derisk the early stages of drug design. As a main deliverable and proof of concept of the efficiency of these methods, our ambition for the first four years of the project is to identify a handful (2 to 4) of new candidate drugs against neurological diseases.
2.3 Overview of the AIstroSight roadmap
To improve the probability of success of drug candidates in neurology, we integrate complementary information offered by data harvested at different spatio-temporal scales (fig. 2): from the inside of the cell (molecular and cellular biology) to the whole brain (imaging) and even to a population of patients (hospital data), using numerical tools coupling mechanistic models with dedicated AI approaches. In a way, our strategy is to break down the classical stratification silo of Fig. 1, in which literature search, in vitro cell culture, in vivo preclinic studies and in vivo clinic studies are viewed as a sequential multi-stage process. Instead, we propose an integrated machine learning framework into which all those data are combined to predict the effect of a candidate drug molecule.
AIstroSight develops innovative numerical approaches to integrate these information sources into a coherent stream of data and expert knowledge, combining the analysis of experimental observations with reasoning (of different kinds). Currently, these tasks are carried out in isolation and their reconciliation is devolved to biologists/physicians. The originality of the AIstroSight contributions are approaches that automatically carry out this reconciliation to assist biologists/physicians.
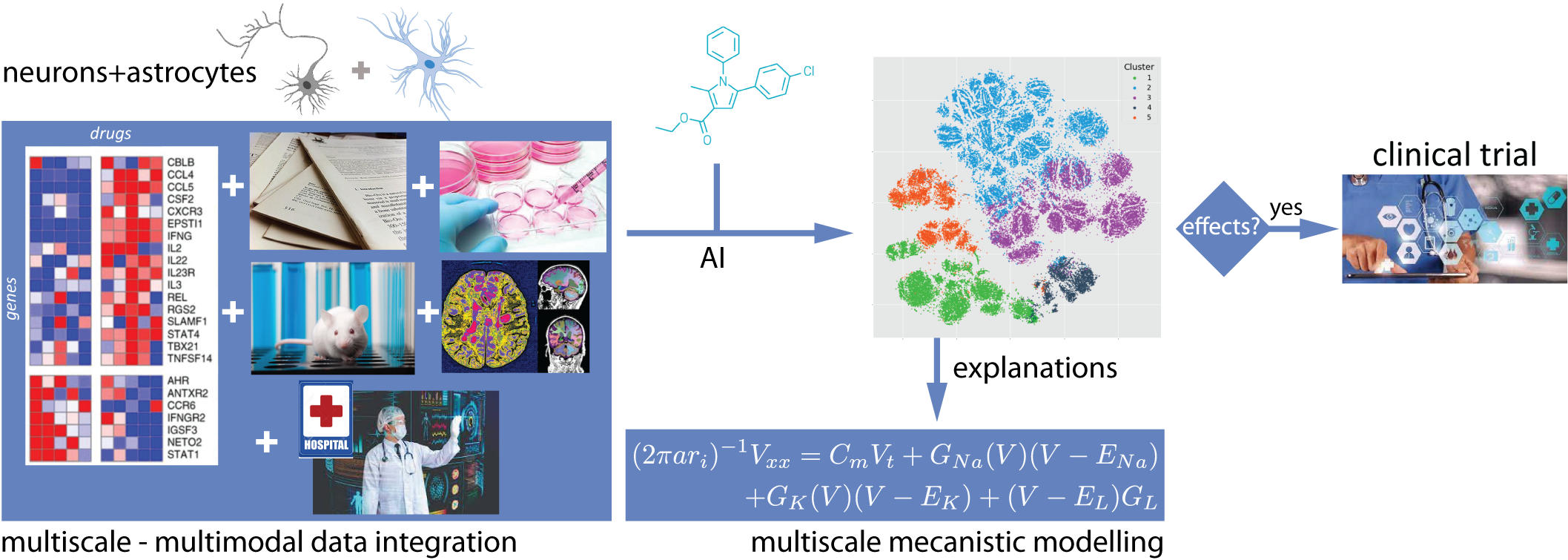
A schematic for the scientific roadmap of AIstroSight
Since AI algorithms are often black-box tools, we also develop mechanistic modeling approaches (multiscale quantitative systems biology/pharmacology) to produce explanations for the predictions of the AI algorithms, that can be rooted in neurobiology. Another important aspect of AIstroSight is to widen the focus of neuropharmacology beyond neurons, that constitute only one half of the nerve cells in the brain, and also take into account the other half, that is made up by glial cells and their interactions with neurons. In particular, we consider the pharmacology of astrocytes 63, one major subtype of glial cells, in interaction with the pharmacology of neurons.
2.4 Principles of the AIstroSight partnership
To accelerate cross-fertilization between digital science and medical research, AIstroSight will be located on the East Hospital Campus of the Lyon University Hospital, the “Hospices Civils de Lyon” (HCL), from 2024. We will also benefit from our strong association with CERMEP, the preclinical and clinical in vivo imaging platform of the HCL. In 2024, the whole team is indeed expected to move to Lyon's neurology hospital, located just across tA joint team with the HCLhe street of CERMEP.
CERMEP is also affiliated with University Claude Bernard Lyon 1, Inserm and CNRS. This provides us with an exceptional environment for the engineering of brain biochemical imaging methods that allow the study of the effect of molecules on the whole brain (fMRI, PET, fUS) and the analysis methodologies for these images. The CERMEP also hosts team BIORAN of the “Centre de Recherche en Neurosciences de Lyon” (CRNL) laboratory, that has expertise ranging from the chemistry of candidate-molecules to their biochemical assays, from radiolabelling to animal PET/MRI imaging and from preclinical models to first-in-man studies in patients. The modeling expertise on the binding between candidate molecules and receptors (structural biology, docking) is also present at the CERMEP.
As a joint team with the HCL, part of AIstroSight technology developments is intended to be integrated into the hospital information system developed during the last decade by the HCL for patient management. This is in particular the case of the development of “Multi-patient query for care pathway characterization and clinical trials”. Beyond participating in the HCL's mission as an innovation leader in digital health, AIstroSight also represents an opportunity for the HCL to reinforce its infrastructure for the organization of clinical trials, for instance in cooperation with pharma/biotech companies like Theranexus. Like other teams of Inria Lyon, AIstroSight is intensively implicated in the “AI innovation department” (“Pôle de Développement IA”) that Inria Lyon and HCL are supporting.
Finally, to ensure the impact of our works on pharmacology and provide it with potential industrial exit routes, the AIstroSight partnership also includes an industrial partner, Theranexus, a French biopharmaceutical company (an SME) that develops drugs for the treatment of nervous system diseases with an original focus on both neurons and astrocytes. Theranexus is listed on the Euronext Growth market in Paris and its social headquarters are located in Lyon. A biotech company, the expertise of its members is entirely in the experimental aspects, not in the digital. Theranexus brings to AIstroSight experimental data (experimental cell biology and brain imaging for pharmacology), and provides their expertise for the development of the digital tools needed to analyze these data. In return, the objective is that the output of these digital tools reveal novel drug targets or novel candidate molecules that Theranexus may decide to use to develop new treatments, starting with the necessary clinical trials. Importantly, the fact that these candidate drugs have been selected from an innovative numerical approach strongly consolidates the credibility of their development on the pharmaceutics market. In addition, Theranexus brings to AIstroSight its know-how and industrial expertise on the development of drug candidates up to the market and its strategic knowledge of the neuropharmacology industry. In this operating scheme, Theranexus is therefore the preferred partner for the early-phase transfer of the molecules that AIstroSight could identify.
Independenlty from AIstroSight, Theranexus and BIORAN have a longstanding collaboration together, in particular in the framework of an ANR- and AURA Region-funded joint laboratory (LabCom) called « NeuroImaging for Drug Discovery (NI2D)» that aims at the development of gliopharmacology using preclinical imaging tools (PET/MRI/brain ultrasound). This LabCom is also hosted within the CERMEP premises. NI2D aim at developing preclinical neuroimaging techniques (in animals, mainly fMRI, PET and fUS = Functional Ultra-Sound) for the evaluation of drug-candidates. AIstroSight develops numerical methods capable of integrating data at multiple scales for pharmacology, data that include imaging but also molecular data (intracellular signaling, omics data) or clinical data (biology, treatments, medico-administrative). We thus benefits from the imaging data and methodologies of NI2D. The two are therefore complementary, especially since we both share a strong interest in neuron/astrocyte interactions.
3 Research program
3.1 Characterizing the mechanisms of action of candidate drugs
Drug screening, either in vitro or in silico, generally does not provide an explanation of the mechanism by which the identified drug acts at the cellular level. However, this information is crucial (e.g., with respect to patients or health agencies), and the algorithms used for the screening must be made explicable. Our goal here is to use mathematical models and their hybridization with machine learning to provide explanations on the mechanisms of action of a candidate molecule.
We develop mechanistic models of regulatory networks or intracellular signaling pathways specific to the action of the candidate drugs identified by the screening. Those models predict the spatio-temporal evolution of the concentrations of the molecular species involved in the modelled pathways using classical reaction terms from biochemical kinetics and mass-action laws (first order reactions, bi-molecular reactions, Michaelis-Menten, Hill kinetics…). Depending on the importance of intracellular spatial gradients and biochemical noise, space and stochasticity is accounted for, thus resulting in models based on reaction-diffusion equations, stochastic or ordinary differential equations, or other related formalisms (Gillespie algorithm, flow analysis). These models allow us to simulate in time and/or space of the cell the mechanisms that govern the dynamics of the implicated molecules and how this dynamic is altered by the selected drug. The aim is to use such mechanistic modeling to produce explanations for the predictions made by the statistical learning techniques that are used in the other sections. It is unlikely that these mechanistic models in themselves allow us to decipher the totality of the molecular mechanisms involved, but they provide critical information to properly adjust the laboratory and clinical experiments.
To be efficient, this approach demands that we maintain an effective knowledge basis and expertise on the fundamental molecular mechanisms at play at these spatial scales and their modelling. To build and maintain this expertise, we rely on existing long-term collaborations between AIstroSight members and experimental neuroscientists -electrophysiologists- or neuropharmacologists on the intracellular signaling networks at play in neuron function or neuro-astrocyte interactions.
- Agonist bias in GPCR: G protein-coupled receptors (GPCR) are currently the largest family of molecular targets for potential new drugs 18. GPCR are cell-membrane receptors ubiquitously found in all mammalian cells, but in particular in brain cells (neurons and astrocytes), where they control a large repertoire of neuronal and astrocytic responses to a variety of external stimuli and molecules. Bias antagonism refers to the observation that two ligands of the same GPCR can activate very different cell responses 43. This phenomenon is still not understood, but it is one possible path towards the development of new drug discovery and has been already proposed and stated to be explored, in particular by members of AIstroSight (B. Vidal, L. Zimmer) 64, 51. Our objective here is to build realistic mechanistic models of GPCR-based cell signaling in the neuronal intracellular space. We plan then to use this/these models to propose molecular mechanisms to explain the experimentally observed biases. A first idea to explore is the hypothesis of a local subcellular compartmentalization of the signaling molecules over and close to the cell membrane (so-called nanodomains). Experimental validation of the main model predictions is then to be performed using brain imaging modalities available at CERMEP (TEP, MRI, fUS).
- Synaptic plasticity: Synaptic plasticity, the long-term adaptation of the efficacy of a synapse according to the activity of the neurons and astrocytes composing this synapse, is thought to underly learning and memory at the cellular scale 60. We have been enjoying a very fruitful collaboration with Laurent Venance's lab (INSERM U1050, CIRB, Collège de France, Paris) on the subcellular mechanisms at play in learning and memory formation by synaptic plasticity 28, 30, 31, 29, 37, 66, 41. Current work focuses on the control of synaptic plasticity mechanisms by endocannabinoids and its implication in fast learning and on metabolic regulation of synaptic plasticity by astrocytes. This collaboration is funded by ongoing ANR project EngFlea (see below).
- Calcium signaling in astrocytes: Calcium signaling in the terminal branchlets of astrocytes is thought to be crucial for astrocytic functions and neuron-astrocyte interactions 20. We are studying the local dynamics of calcium signaling in terminal branchlets of astrocytes and their interaction with synaptic activity in collaboration with U. Valentin Nägerl's lab (CNRS UMR 5297, Bordeaux) for experimental (subcellular) data with supra-resolution microscopy 32. Recently, a collaboration with Erik de Schutter's lab (Okinawa Institute of Science and Technology, Japan) has also been set up to develop new efficient modelling tools (stochastic reaction-diffusion systems) in realistic 3D geometric meshes based on the simulation framework they develop, STEPS 19, 33.
- Multiscale modelling of the effects of a candidate drug on neurons and astrocytes: To model the cellular effect of a candidate drug, the main molecular systems impacted by the drug, and thus to be accounted for in the mechanistic model, are isolated from the cellular signature data and literature exploration. Imaging data, by specifying the brain areas and structures mainly targeted by the candidate drug, helps refine these models using specific parameters. Whereas in the first models, the observation (and modelling scale) corresponds to a subcellular domain (one synapse, +/- a dendrite or the main astrocytic process in the neighborhood), we search to progressively scale up those mechanistic models from the intracellular scale of a single cell to the scale of a population of interacting brain cells, neurons and astrocytes. To do so, we explore model simplification /reduction methods, including those combining machine learning and dynamical systems modelling (see below). In the long run, this large-scale mathematical model will produce a digital twin of the pathology that will allow us to explain why the candidate drug has a positive effect on the disease. Calibration is based on fUS and fMRI imaging data in rodents obtained in the framework of the NI2D LabCom. This data provides us with quantitative measurements of the effects of microscopic perturbations by pharmacological agents or by external stimuli (e.g., visual) on the variation, correlation and spreading of cortical activity over the whole brain.
- Astrocyte roles on brain imaging signals: Although it is now widely accepted that astrocytes play a role in brain processes and pathologies, the exact perimeters of their roles remain to be delimited. For instance, variations of the signals measured by brain imaging methods (fMRI, PET, fUS) are still largely interpreted as variations of neuronal activity. Available experimental data however indicate that astrocytes also impact those signals but it not clear yet how they do it. A precise and quantitative answer to this question would allow us to use brain imaging to monitor not only the local activity of the neurons but also of the astrocytes. Such a feature would be precious in our framework of astrocyte pharmacology but it demands the development of new mathematical models. Existing models of fMRI signals, for instance, are either too crude to incorporate a separate astrocyte action (balloon models 39) or are limited to the role of astrocytes as energy suppliers of the neurons (astrocyte-neuron lactate shuttle 45). Our objective here is to start from a microscopic and mechanistic model of neuron-astrocyte-blood vessel interactions and use multi-scale modelling methodologies to obtain a large-scale model of astrocyte-neuron impact on a subset of brain imaging technics (fMRI, fUS), with explicit parametrization of local neuronal and astrocyte activities. Here again these models are calibrated using fUS and fMRI imaging data in rodents, in particular using pharmacological agents that are known to specifically silence the astrocytic population or a neuronal population in a given brain area. A crucial step is the development of a detailed, microscopic model of the astrocyte endfeet, the specialized astrocyte processes that respond to and control local vascular diameters. This model will provides us with causal mechanisms able to interlink neuronal electrical activity, astrocytic calcium activity and local blood flow. It is to be seen as a first stage towards understanding the implication of astrocytes in variations of neuroimaging signals.
Methodological challenges: The biological systems to be considered to explain drug effects on pathologies are not only very complex but also only partly understood by neurobiologists themselves. Therefore, the available biological knowledge on these systems is constantly evolving. Since we cannot know in advance what systems are affected by the candidate drug, a major difficulty for modelers is preparedness, i.e. maintain a level of expertise on the biology and modelling state-of-the-art of a wide range of those systems. This is the reason why the first three projects above are crucial to the success of our proposal.
The most important challenge we face is that of multiscale: causal data are mainly molecular but many observations are macroscopic (e.g. brain imaging). Traditionally, linking these two scales requires the development of new theories (e.g., homogenization, population boundaries, etc.), a slow and rather hazardous process. The availability of more and more important computing resources allows to consider "brute force" approaches in which all scales of time and space are numerically simulated (cf Blue Brain Project). But the results are often as difficult to interpret as the animal experiments that these simulations emulate. Instead we consider recent advances in hybrid digital-AI systems (physics-informed neural networks 56), in particular equation discovery methodologies 55. These methods usually use sparse regression techniques to select in a library of nonlinear terms and operators, those that, when composed, provide the best description of the data 24. Our idea is to generate a large number of numerical simulations at the microscopic scale of the kinetics of the biochemical reactions concerned, for example by the spatial Gillespie algorithm, and then to aggregate them at a higher spatio-temporal scale using, for example, averages at a space and time grain much higher than the spatio-temporal resolution of the initial microscopic simulations. The idea is then to use equation-discovery algorithms to infer a set of differential equations (and associated parameters) capable of describing these higher scale space-time kinetics. The resulting reduced model is then replicated in each cell of the cell population model. If successful, this model reduction process can even be reiterated at the upper scale to simulate the effect of the molecule on large brain areas. Of course, the risky and difficult nature of this objective makes it a long-term goal. If need be, alternative meta-modelling technics will also be considered when applicable (RKHS, model-order reduction).
3.2 Multi-patient query for care pathway characterization and clinical trials
Real-world data, especially the data that is routinely collected by hospitals (medical reports, hospital records…), provides rich information about possible links between patient information (demographic, pathological, life style), drug exposures and health events. In the context of drug development, this data can be useful at three stages. During the search for a new drug, they can be used to enrich cell culture data or imaging data. In this case, one can query patients that have been treated for the pathology in question and integrate their clinical data in the in-silico screening. This approach is presented below in the framework of data integration.
Electronic Health Records query algorithms:
Efficient patient query can also be used at the very initial stage of drug discovery: the assessment of the feasibility of drug development projects. Indeed, part of the pathologies we target are rare diseases. In this context, one has to make sure at the very early stages that the pathology in question is not so rare that the number of patients is too low to allow clinical trials, or that its description in terms of physiopathology is mature enough for the clinician to be able to diagnose it with good probability. We thus develop patient query algorithms on clinical data from hospitals (electronic health records, EHR), in particular of the HCL, that allow us to characterize the care pathways of the patients before and after diagnosis. They provides us with answers to many questions related to the clinical picture of the pathology, its genetic underpinnings, its prevalence rate, the typical care pathway of a patient with this pathology, the delay of diagnostic, the frequency of diagnostic errors etc. Answers to these questions are crucial to determine early on whether the drug discovery project is feasible. We aim at developping query algorithms and software pipelines for EHR that can provide us with tools able to answer these questions efficiently.
Efficient EHR query algorithms are also very useful at the final stage of the clinical trial itself (Fig. 2), where they can be used to finely select what patients should be integrated in the trial. Indeed, a major change of paradigm in medicine in recent years is the acceptation that the response of a group of patients to a drug treatment exhibits strong variability. The source of this variability is diverse 65. The definition of the pathology itself as a unique coherent class can be misleading and actually incorporate a range of different sub-classes of pathologies/disorders. The response to a drug also depends on how the patient's body affects the drug before it reaches its target organ/cells (pharmacokinetics). At the cellular level, the response can also vary because of inter-individual differences in gene sequence and receptor/protein structure (pharmacodynamics). Therefore, individual drug responses depend on the patient genes (pharmacogenetics) but also on more social factors (age, sex, anterior medical record, lifestyle, habits, exposure to pollution…). In any case, the strength of this variability is believed to be a major cause of failure for clinical trials, in particular in neurology and psychiatry 52, 46. The goal of “stratified medicine” in this perspective is to subdivide the available group of patients into a number of subgroups so that the response of each subgroup is less variable than the whole 34. Our objective is to develop computational tools and software packages able to stratify hospital data to assist in the selection of patients to be included in an evaluation protocol for a clinical trial or the building of a research cohort.
Computational phenotyping:
The task of querying patients according to a predefined criterion from a large population of EHRs is sometimes referred to as “computational phenotyping” 57. It remains a time-consuming and challenging task with complex criteria because the query is to be addressed within multiple document types and across multiple data points, in EHRs that usually comprise both structured and unstructured data. The computational challenges raised by patient query with complex criteria are therefore considerable (integration, query, analysis, privacy). Software tools (i2b2, ACE 25) have been proposed to query patients for cohorts or clinical trials based on EHRs but they can hardly be used by most of the physicians because they require advanced knowledge of the data in computer science terms (format, encoding). Moreover, our objective is to provide clinicians with tools able to manipulate these complex data together with medical concepts (e.g., exposure to a drug, treatment, or occurrence of a pathology). Data abstraction capabilities must therefore be integrated to automatically enrich the data using phenotype libraries that can be intuitively mobilized by the clinician. In analogy with bioinformatics workflows, we create workflows for computational phenotyping.
In cases where we already know how to stratify, the issue is not a learning problem but rather a query problem. On the other hand, when this is not the case, we have to develop methods to build these homogeneous subgroups, and in this case it is a question of (unsupervised) learning: the training criterion becomes a measure of cluster homogeneity. Two competing approaches can be thought of in order to create the building blocks of the workflow: 1) machine learning approaches that allow the construction of abstract patient phenotypes from massive data; 2) approaches inspired by both timed systems modeling and knowledge reasoning that rely on formal descriptions of computational phenotypes to enrich the data. The interest of formal descriptions is to be able to represent the whole data transformation in a formal way. This abstract representation of the construction of a cohort facilitates its understanding by users and its reproducibility (FAIR principle). On the other hand, they allow again to exploit intimately the formalized knowledge of the domain, but they also become objects that can be manipulated by reasoning tools. The use of semantic web technologies can therefore be an interesting tool for representing data, knowledge and their processing in order to propose query tools that guide the clinician through the knowledge.
Methodological challenge:
The challenge is to make these formal descriptions highly expressive and to ensure efficient processing of massive data. On the long run, we plan to take inspiration from the approach called “Ontology-Mediated Query Answering” which consists in using ontologies to mediate the query of a database by ontologies 23. In this context, a computational phenotype is seen as a query. The difficulties encountered with observational data is the semantic gap between the available data and the medical concepts that are interesting to manipulate. This gap may be bridged by automatic reasoning that exploits expert knowledge to relate different abstraction levels.
Since computational phenotypes are difficult to formalize, the challenge is to support clinicians in defining them. In other words, the challenge becomes to abstract phenotypes from clinical data. We plan to combine automatic reasoning methods and data analysis. The first research direction we propose is the exploration of a symbolic approach parallel to the work by Tijl de Bie 22 or by Silberschatz 59 on the notion of "subjective measure of interestingness". This approach was developed to identify user-relevant statistical analysis results by means of a statistical model to evaluate the novelty of the extracted patterns (a priori knowledge model). Symbolic approaches can be combined in a similar way by using symbolic data analysis methods such as pattern mining, and by relying on formal models of the system as a priori knowledge. Patterns that are not "explainable" by the formal model are potentially new or of particular interest to the user and will thus be extracted. This approach offers an original entry point to deeply integrate knowledge-based reasoning into pattern extraction methods. The research challenge here lies in combining formalized knowledge with experimental data. It may be implemented using the declarative pattern mining paradigm, that uses solvers to address the pattern mining task. The proofs of concept on the notion of novelty will open the way to more complex reasoning such as planning that can be used to integrate complex behaviors in biological systems, such as interaction networks. The second research direction we propose is based on recent machine learning techniques. Unsupervised ML has been applied to patient phenotyping, i.e. the discovery of phenotypes from EHR data, including temporal phenotyping 67. Our objective is to combine such kinds of algorithm with semantic knowledge to guide the discovery toward meaningful computational phenotypes. Indeed data embedding techniques can integrate ontologies to enhance data semantics 38.
On the long run, the methods developed above may be reunified to address the problem of drug discovery at both the biological and the body scale. This justifies the coherence of the methodological approaches (Semantic Web and machine learning) that are developed in the two objectives.
4 Application domains
4.1 Targeted Pathologies
The list of pathologies that are of interest for Theranexus in the framework of AIstroSight is given in the stand-alone “convention d'équipe-projet commune” of AIstroSight. It comprises roughly 30 rare diseases of the central nervous system, including lysosomal pathologies, neurological genetic diseases, rare diseases due to
4.2 In vitro and in vivo experimental models of neurology diseases
Many of the diseases that are of interest for AIstroSight are rare diseases. This means that the volume of experimental data and the basic understanding of the pathology at the (sub-)cellular level may be too limited for the machine learning or mechanistic modeling tools that we plan to use. For example, it is known that the NPC mutation in Niemann-Pick type C induces morbid cholesterol accumulation in cells but the molecular function of NPC in cholesterol metabolism is not clearly understood 54. Similarly, MeCP2, the gene mutated in Rett syndrome, is an epigenetic regulatory factor (DNA methylation) whose mutation theoretically impacts the expression of a large number of genes but it is not clear which ones are most involved in the symptoms of the disease 44. Although molecular (omic) studies have been published for both diseases 27, 58, their molecular contexts are still unclear.
Our goal here is to generate additional preclinical molecular and imaging data to better delineate the perturbations that these diseases cause at a cellular and tissue level. We introduce into cultured cells the same deficits as those observed in patients. Transcriptomic analysis of the effect of this manipulation gives us information on the implicated molecular networks and its major molecular consequences. In parallel, we induce these same perturbations in vivo in rodents. Observing these animals using brain imaging techniques (fMRI and fUS, possibly PET) gives us a more macroscopic view of the effect of the mutation (affected brain areas, nature and amplitude of the modifications, change in response to treatments or stimuli etc, see below).
Methodological challenges: Developing experimental models of pathologies can be a very difficult task for pathologies that are due to the conjunction of multiple factors, when the molecular alterations at the origin of the pathologies have effects over a very large range of cellular processes or when comparison of the phenotype of the experimental model with its human counterpart is ill-defined (psychiatric diseases, for instance). To mitigate this risk, we develop experimental models only for pathologies that are well-defined in molecular terms, like for Rett or Niemann-Pick type C for a start. We use viral vector strategies (mostly shRNA-mediated gene silencing or possibly CRISPR-based gene editing via adeno-associated viruses, AAV) to manipulate the sequence or expression of the target gene. We start with cell lines that are easy to grow and analyze using omics approaches, and then use neurons and astrocytes differentiated from human pluripotent stem cells. This approach is also used in vivo by locally injecting the viral vector into a given brain region of an animal model, to genetically modify a particular cell type by using a specific promoter. We should therefore be able to control the area of the brain in which the genetic manipulation will be induced (e.g. visual cortex or cerebellum) as well as the type of cells targeted (neurons vs. astrocytes, for example). Of course, like all experimental models, each model taken separately has its limitations: the genes expressed by cells in culture are not necessarily those expressed by these same cells in vivo, the effects of gene silencing in a rodent are not necessarily transposable to humans, etc. However our hypothesis is that by combining these different modalities and scales of data (see above), it should be possible to better predict the effect of a potential treatment. The molecular and cellular biology technologies to be mobilized here (in vitro and in vivo mutagenesis, cell culture, proteomics) are tools routinely used by Theranexus. The expertise on the use of medical imaging to observe the effects at the brain level is provided by CERMEP and benefits from the advances of the NI2D LabCom.
4.3 Identification of multi-source multi-scale biomarkers
Recently, Theranexus changed its pharmacological strategy, from a strategy mainly based on the repositioning of pre-existing drugs to a technology based on antisense oligonucleotide drugs. These technologies rely on the ability to design on demand short RNA sequences that specifically bind the mRNA of a gene target, and knock it down after recognition by the RNase H1 enzymes present in all cells, or modulate its translation or splicing via steric hindrance . Pharmacological intervention thus consists in searching for a gene target able to correct the molecular perturbation caused by the disease and to synthesize an antisense oligonucleotide able to specifically bind this target gene. Note that the technology currently in clinical use does not (yet) provide ways to specifically target a cell type or a brain region.
Our first objective is to develop digital tools to model the molecular networks perturbated by the pathology of interest, and use this model to identify a gene or protein in the network the modulation of which would correct the perturbation caused by the pathology. These models are based on molecular data, in particular transcriptomics and metabolomics data. The set of data includes data derived from cell cultures as described above, that we augment with molecular data from the literature related to the pathology or more generic public, open access databases of transcriptomic responses to perturbating molecules, like CMap or the LINCS L1000 data repository. The latter, for instance currently includes the effect of close to 40,000 small perturbating molecules on 12,000+ genes of more than 200 cell types. We aggregate these data and use them to infer the gene interaction network, the metabolic network and/or the signaling network impacted by the pathology. Metabolic networks are important for instance for Niemann-Pick type C, to conciliate perturbations of the lipid metabolism with those of the gene expression network. This provides us with a view of the pathology at the molecular scale.
Integration of neuroimaging data:
A major objective of AIstroSight is to augment these molecular data with medical data, in particular brain imaging data and hospital data. We complement molecular data with data coming from the analysis of brain imaging (fMRI, PET, functional ultrasound brain imaging) i.e. with functional networks between brain areas targeted by the molecule or quantitative measures of radioligand binding. Most of this imaging is done in rodents (preclinic, see above) but a subset of human imaging data is also used. These imaging data are obtained by our collaborators from the CERMEP platform.
These different neuroimaging methods provide meaningful and complementary information for understanding the functional or molecular effects of drugs in the brain:
- Positron emission tomography (PET) enables to visualize and measure the concentration of a specific radiotracer, with nanomolar to picomolar sensitivity. Numerous brain PET radiotracers have been developed over the years, enabling to study various molecular processes (such as the synthesis and release of endogenous neurotransmitters, the density of receptors, transporters or proteins aggregates, neuroinflammation and cerebral metabolism) both in animals and humans in a non-invasive way. We especially focus on the measurement of cerebral glucose consumption using [18F]FDG, a radiolabeled glucose analog, while also taking advantage of the rich information that can be obtained using other brain radiotracers when needed. Indeed, the asset of [18F]FDG-PET imaging is to be relevant for virtually all drugs that are expected to be active in the brain, since the cerebral glucose uptake is related to neuron and astrocyte activities. In addition, this neuroimaging technique can be used in awake freely-moving animals, getting rid of the anesthesia or stress confound in preclinical studies.
- Functional magnetic resonance imaging (fMRI) enables to follow the dynamic changes in the BOLD signal (for “blood-oxygen level dependent”), which is also related to the brain activity, in a non-invasive way. It has a high temporal resolution (2 to 3 seconds) as compared to PET imaging (several minutes) and therefore can be used either to measure the time series of BOLD signal changes after injection of a drug (called “pharmaco-MRI”) or the changes in functional connectivity occurring after neuropharmacological stimulation. Functional connectivity is defined as the correlation in activity over time between different brain areas; this concept has largely become prominent in neurosciences over the years, and it is known to be modified in many physiological or pathological states. fMRI can be used in animals and humans, similarly to PET, but usually requires anesthesia for preclinical applications.
- Functional ultrasound imaging (fUS) is a much more recent imaging technology. It provides access to the dynamic measurement of cerebral blood volumes changes, which are more straightforwardly related to the brain activity as compared to the BOLD signal. Moreover, its spatial, temporal resolution and sensitivity are unmatched by the previous techniques, and it can be applied to freely-moving awake animals in real-time, in correlation with a particular behavior. However, it is currently limited to imaging in 2-dimensions (one brain plane at a time) and is mostly suitable for animals. Therefore, fUS imaging is a complementary way to study brain activity in small animals in the context of preclinical neuropharmacology.
Integration of clinical data:
We also plant ot integrate hospital data from the Hospices Civils de Lyon according to availability and pathologies. Hospital data provide access to rich information on possible links between patient information (demographic, pathological), drug exposures, health events or biological sample analysis (e.g., blood markers). Our goal is to integrate brain imaging and hospital data with cellular signatures to enrich them with information at the individual scale in a form that can be analyzed with machine learning (clustering, classification) or data mining (pattern matching) methods.
Methodological challenges:
A first challenge resides in the nature of action of antisense oligonucleotides, that often work by knock down/loss of function. It is not straightforward to design such a strategy in the case of a pathology that is due to a mutation that already suppressed the effect of a gene. That is precisely where numerical models of the involved gene expression and metabolic networks are important because they can be systematically assessed for the effect of gene suppression, thus providing a quick in silico screening of the potential targets. However, part of this program implies typical bioinformatics processing steps: analysis of transcriptomic networks, network reconstruction, conciliation between transcriptomic and metabolic networks… We currently do not have this expertise in the team. Therefore we leverage collaborations with local experts of the field to get the necessary operational knowledge, including experts of brain transcriptomics analysis (MeLiS lab in Lyon).
Another difficulty lies in the heterogeneity of these multiscale data, their highly categorical character, the large dimension of the corresponding variable space and often, the small number of observations. Moreover, cellular signature data are intrinsically very noisy and can have low reproducibility 48, a caveat that feature selection may improve, at least in part 35. Class imbalance can also be strong. Finally, each type of available observation (molecular networks, imaging, hospital) gives a partial, fragmented and incomplete view of an abstract complex biological system. This is a partial view because each type of observation provides data at a given spatio-temporal scale, for a certain locus. This is a fragmented view because the data will be collected from different patients, and even from very different living systems (cell cultures, animals, patients). Each patient contributes to the description of the abstract system on only few types of observations. This is incomplete because there will be many gaps to bridge the different kinds of information related to functioning of the studied biological system.
To reach our objective, we explore the use of Semantic Web (SW) formalism which attracts a lot of interest in bioinformatics, to formalize knowledge and data. Data are observations of biological systems acquired within controlled experiments or in real life. Formalized knowledge is a representation of facts and rules acquired in a scientific domain, here medicine or life sciences. Applying machine learning techniques on data supports knowledge discovery, but it is only one particular source of knowledge. The methodological challenge is first to formalize the different types of available data within an abstract model of the biological system, and to integrate formalized knowledge in the model coming from medical literature and our medical expertise, including imaging or hospital data. By gathering a wide range of formalized data and knowledge within the same tool, we aim at creating a kind of abstract numerical twin that may be queried to infer new knowledge to assist drug design or drug repositioning.
On the longer run, the second challenge is to develop query answering at the abstract level but based on fragmented data. The objective is to answer queries about the numerical twin by exploiting the data coming from multiple patients. One of the difficulties is to detect groups of patients whose numerical twins are “similar to each other” (in a sense that remains to be defined). Semantic Web offers a natural framework for querying formalized data with multiple facets but may be limited by the time-efficiency of the query engines on a large number of patients. In such a context, numerical approaches (embedding) is more time-efficient but may lack accuracy. The challenge is to construct numerical representations in order to embed the data in a space in which the distances are both efficient to compute and semantically consistent with the applied notion of “similarity”. Numerical machine learning techniques turn out to be an interesting perspective to address this challenge 50. Recent research on advanced machine learning, such as representation learning, offers new perspectives to address our challenge. Our objective is to initiate collaborations with teams having strong backgrounds in machine learning (e.g. Ockham Inria Team) to propose innovative solutions. Another important point is the need for logic programming methodologies able to express complex queries, especially on heterogeneous or multimodal data. For neuroimaging, the availability of Neurolang for logic programming with heterogeneous data or NeuroQuery for query result consolidation based on automatic literature meta-analysis, for instance, should be very useful.
In most of the cases, the methodologies that we use to reach the above objectives are related to knowledge management/mining, formal reasoning, data mining or learning. Machine learning or deep learning approaches are probably less useful here. The main reason is related to the volume of available data. For rare disease like Niemann-Pick type C, for instance, the low prevalence means that 5 to 10 new patients are diagnosed in France each year, a number too low for deep neural networks. However, advances in transfer learning might be helpful here. For instance, a large number of brain pathologies come with dysfunction of intracellular cholesterol metabolism and storage. This is for instance the case of multiple sclerosis 49, for which large cohorts and databases are available worldwide. As a long term project, an interesting idea will be to leverage the large volume of data on multiple sclerosis to identify biomarkers of cholesterol dysfunction, e.g., in neuroimaging, and use transfer learning to adapt the network to Niemann-Pick type C patients.
5 Highlights of the year
Our work on the extraction of temporal phenotypes from clinical data (electronic health records) using tensor decomposition has been published in Machine Learning, featuring a usecase from Paris University Hospitals (AP-HP) 6. The corresponding open-source software, SWoTTeD, is available (see Section 6).
6 New software, platforms, open data
6.1 New software
6.1.1 SWoTTeD
-
Name:
Sliding Window Tempral Tensor Decomposition
-
Keywords:
Tensor decomposition, Temporal information, Machine learning
-
Functional Description:
SWoTTeD is a tensor decomposition framework to extract temporal phenotypes from structured data. Most recent decomposition models allow extracting phenotypes that only describe snapshots of typical profiles, also called daily phenotypes. However, SWoTTeD extends the notion of daily phenotype into temporal phenotype describing an arrangement of features over a time window.
- URL:
- Publications:
-
Contact:
Thomas Guyet
-
Participants:
Hana Sebia, Thomas Guyet
6.1.2 Metabograph
-
Name:
Metabograph
-
Keywords:
Python, Metabolic networks, Graph
-
Functional Description:
Metabograph is a Python library for generating metabolic networks from BioPAX data. The generated NetworkX graphs can be used in various application such as training graph neural networks (GNNs) or saved to files for use with viewers such as Gephi.
- URL:
-
Contact:
Jan-Michael Rye
6.1.3 jnp
-
Name:
jnp
-
Keywords:
Jupyter, Python, Presentation
-
Functional Description:
A Python library and command-line tool to automatically export presentations from Jupyter notebooks along with custom CSS and other user-configurable website content.
- URL:
-
Contact:
Jan-Michael Rye
6.1.4 PyPTU
-
Keywords:
Python, PTU
-
Functional Description:
Parse PicoQuant PTU files in Python. It includes a Python package and a command-line utility.
- URL:
-
Contact:
Jan-Michael Rye
6.1.5 VCNet
-
Name:
Variational Counter Net
-
Keywords:
Generating Explanations, Variational Autoencoder
-
Scientific Description:
Counterfactual explanation is a common class of methods to make local explanations of machine learning decisions. For a given instance, these methods aim to find the smallest modification of feature values that changes the predicted decision made by a machine learning model. One of the challenges of counterfactual explanation is the efficient generation of realistic counterfactuals. To address this challenge, we propose VCNet – Variational Counter Net – a model architecture that combines a predictor and a counterfactual generator that are jointly trained, for regression or classification tasks. Once fitted, VCNet is able to both generate predictions, and to generate counterfactual explanations without having to solve another minimization problem. Our contribution is the generation of counterfactuals that are close to the distribution of the predicted class. This is done by learning a variational autoencoder conditionally to the output of the predictor in a join-training fashion. The empirical evaluation on tabular datasets and across several interpretability metrics demonstrate that VCNet is competitive with the state-of-the-art methods.
-
Functional Description:
VCNet is a counterfactual generator for tabular datasets. Counterfactual generation is an explainable artificial intelligence technique that helps users understand the decisions of predictive models. VCNet handles both categorical and numerical variables and can also manage immutable variables (i.e., variables that cannot be changed while generating counterfactuals). Currently, it is implemented for binary classification only. VCNet is based on conditional variational encoders and offers an easy and fast training architecture that generates realistic counterfactuals (i.e., counterfactuals that likely exist in the data distribution). This library provides two versions of VCNet: * Joint-learning architecture: This version includes both the classification model and the counterfactual generator. * Post-hoc architecture: This version includes only the counterfactual generator, which can be fitted on any prediction model (Torch module).
Once the VCNet model is fitted, it can be used to generate efficiently valid counterfactuals of examples.
- URL:
- Publications:
-
Contact:
Thomas Guyet
-
Participants:
Victor Guyomard, Thomas Guyet, Françoise Fessant, Tassadit Bouadi
-
Partner:
Orange Labs
7 New results
7.1 Analysis of care pathways in hospitals
Participants: Hana Sebia, Thomas Guyet, Francois-Elie Calvier, Arnaud Duvermy.
Improving the future of healthcare starts by better understanding the current actual practices in hospital settings. This motivates the objective of discovering typical care pathways from patient data.
We have proposed to leverage tensor decomposition to extract care pathways from hospital data. Tensor decomposition has recently been gaining attention in the machine learning community for the analysis of individual traces, such as Electronic Health Records (EHR). However, this task becomes significantly more difficult when the data follows complex temporal patterns. Our paper 6 introduces the notion of a temporal phenotype as an arrangement of features over time and it proposes SWoTTeD (Sliding Window for Temporal Tensor Decomposition), a novel method to discover hidden temporal patterns. SWoTTeD integrates several constraints and regularizations to enhance the interpretability of the extracted phenotypes. We validated our proposal using both synthetic and real-world datasets, and presented an original usecase using data from the Greater Paris University Hospita (AP-HP). The results showed that SWoTTeD achieves at least as accurate reconstruction as recent state-of-the-art tensor decomposition models, and extracts temporal phenotypes that are meaningful for clinicians. The implementation of SWoTTeD has been released (see Section 6).
An alternative approach is to consider care pathways as time series and develop appropriate clustering approaches to reveal typical care pathways. The difficulty in clustering care pathways, represented by sequences of timestamped events, lies in defining a semantically appropriate metric and clustering algorithms. In ref 5, we have adapted two methods developed for time series to the clustering of timed sequences: the drop-DTW metric and the DBA approach for the construction of averaged time sequences. We applied these methods in clustering algorithms to propose original and sound clustering algorithms for timed sequences. The approach was experimented with and evaluated on synthetic and real-world data from the Greater Paris University Hospital (AP-HP). This approach leads us to start the development of a library to represent, manipulate and cluster temporal sequences such as care pathway. The first version of this library has been presented and discuss within a workshop dedicated to the analysis of trajectories.
Finally, we conducted studies on how Artificial Intelligence may change the definition of care pathways and it may impact care systems.
7.2 A new model for the formation of long-term memories in the cortex
Participants: Hugues Berry.
The standard consolidation theory states that short-term memories located in the hippocampus enable the consolidation of long-term memories in the neocortex. In other words, the neocortex slowly learns long-term memories with a transient support of the hippocampus that quickly learns unstable memories. However, it is not clear yet what could be the neurobiological mechanisms underlying these differences in learning rates and memory time-scales. With Lisa Blum Moyse, who defended here PhD in Sept. 2023, we proposed a novel modelling approach of the standard consolidation theory, that focuses on its potential neurobiological mechanisms. In addition to synaptic plasticity and spike frequency adaptation, our model incorporates adult neurogenesis in the dentate gyrus as well as the difference in size between the neocortex and the hippocampus, that we associate with distance-dependent synaptic plasticity. We also take into account the interconnected spatial structure of the involved brain areas, by incorporating the above neurobiological mechanisms in a coupled neural field framework, where each area is represented by a separate neural field with intra- and inter-area connections. To our knowledge, this is the first attempt to apply neural fields to this process. Using numerical simulations and mathematical analysis, we explore the short-term and long-term dynamics of the model upon alternance of phases of hippocampal replay and retrieval cue of an external input. This external input is encodable as a memory pattern in the form of a multiple bump attractor pattern in the individual neural fields. In the model, hippocampal memory patterns become encoded first, before neocortical ones, because of the smaller distances between the bumps of the hippocampal memory patterns. As a result, retrieval of the input pattern in the neocortex at short time-scales necessitates the additional input delivered by the memory pattern of the hippocampus. Neocortical memory patterns progressively consolidate at longer times, up to a point where their retrieval does not need the support of the hippocampus anymore. At longer times, perturbation of the hippocampal neural fields by neurogenesis erases the hippocampus pattern, leading to a final state where the memory pattern is exclusively evoked in the neocortex. Therefore, the dynamics of our model successfully reproduces the main features of the standard consolidation theory. This suggests that neurogenesis in the hippocampus and distance-dependent synaptic plasticity coupled to synaptic depression and spike frequency adaptation, are indeed critical neurobiological processes in memory consolidation. This work has been publised in the Journal of Theoretical Biology 4.
7.3 Modeling compartmentalization within intracellular signaling pathway
Participants: Nathan Quiblier.
A signalling pathway, or biochemical cascade, is a chain of biochemical events activated by a receptor upon ligand binding, that ultimately leads to a cell response, e.g. changes in gene expression, cell growth, metabolism. However, several receptors, including in the pleiotropic familiy of G-protein coupled receptors (GPCR) do not permanently stay at the outer cell membrane but become internalied inside the cell, via a vesicle surrounded by an area of cell membrane which contains receptor (and other) molecules. These vesicles, called endosomes, and their molecular content, can further be degraded or be partly recycled back to the outer cell membrane. These spatial movements induce, upon ligand binding, an heterogeneous population of receptors spread out between the outer membrane and a dynamic population of endosomes with distinct micro-environment. Key evidence have shown that the intra-cellular traffic of internalized receptors has a major impact in cell response to a given stimuli. However, the mathematical models of cell signalling usually do not take endosomal trafficking into account.
In the article 3, we have presented a novel approach to modeling receptor-activated signaling pathways that take into account the compartmentalization of receptors and their effectors, both on the cell surface and in dynamic intracellular vesicles called endosomes. The first building block of the model concerns compartment dynamics. It takes into account creation of de novo endosomes, i.e. endocytosis, and further recycling of endosomes to the cell surface or degradation, as well as fusion of endosomes via coagulation dynamics. The second building block concerns biochemical reactions on the cell surface and within intra-cellular compartments. Both building blocks are coupled by the transfer of molecules that occurs at each event that modifies the compartments. The model is formulated as a integro-partial differential equation, with transport and coagulation operators, and source terms, coupled to an integro-differential equation. In this work, we proved sufficient conditions to obtain exponential ergodicity for the size distribution of intracellular compartments. We further designed a finite volume scheme to simulate our model. Finally, we showed two application cases that show qualitative agreement with recently published data, proving that our model can help capture the spatio-temporal complexity of receptor-activated signaling pathway.
8 Bilateral contracts and grants with industry
8.1 Bilateral contracts with industry
Participants: Hugues Berry, Audrey Denizot, Thomas Guyet, Jan-Michael Rye, Benjamin Vidal, Luc Zimmer, Andrea Ducos, Hana Sebia, Zoe Laffitte, Florian Dupeuble, Lucas Perret.
AIstroSight is a joint project-team with the biotech company Theranexus. A plain “tutelle” of the team, Theranexus brings its research expertise in in vitro cell culture, disease modelling and imaging, both in terms of research workforce and data. The stand-alone “convention d'équipe-projet commune” of AIstroSight lists a group of 30 rare diseases of the central nervous system, that are of direct interest to Theranexus and thhat are associated with a specific regimen in terms of IP and legal affairs. However, AIstroSight members are allowed to work on pathologies outside this list without any restriction but with a different legal regimen vis-à-vis Theranexus.
9 Partnerships and cooperations
9.1 International initiatives
9.1.1 Visits of international scientists
Christopher K Salmon
-
Status:
Postdoctoral scholar
-
Institution of origin:
McGill University
-
Country:
Canada
-
Dates:
26/09/2024-07/10/2024
-
Context of the visit:
Invited talk and collaborative work. Dr. Chris Salmon visited our team from September 26th to October 4th. During his stay, the team gained knowledge in the analysis of 3D electron microscopy stacks. Moreover, we launched a collaborative project based on 3D reconstructions of astrocytes from Pr. Keith Murai lab, McGill University, Canada. The goal of the project is to simulate astrocyte activity in these realistic shapes to better understand the functional implications of the morphological alterations they observe in astrocytes in Alzheimer Disease.
-
Mobility program/type of mobility:
Guest Researcher funding by the Lyon Inria center
Erik De Schutter
-
Status:
Professor
-
Institution of origin:
Okinawa Institute of Science and Technology
-
Country:
Japan
-
Dates:
17/09/2024
-
Context of the visit:
Invited talk at the workshop "Linking brain structure & function at the nanoscale: an interdisciplinary workshop" organized by our team and collaborative work.
-
Mobility program/type of mobility:
Research visit
Other international visits to the team
Marc Theveneau
-
Status:
PhD student
-
Institution of origin:
McGill University
-
Country:
Canada
-
Dates:
26/09/2024-07/10/2024
-
Context of the visit:
Collaboration with the Shape Analysis Group, led by Pr. Kaleem Siddiqi, McGill University, Canada and attendance to the "Linking brain structure & function at the nanoscale: an interdisciplinary workshop"
-
Mobility program/type of mobility:
Research stay funded by McGill University
9.2 National initiatives
ABC4M
Participants: Nathan Quiblier, Hugues Berry.
-
Title:
Approximate Bayesian computation-driven multimodal microscopy to explore the nuclear mobility of transcription factor
-
Partner Institution(s):
- Inria, Lyon (supervision)
- Institut Langevin, ESPCI, Paris
- Phlam laboratory, Lille
-
Date/Duration:
2020-2025
-
Additionnal info/keywords:
Funded by the French National Agency for Research (ANR), Call “AAP2020" (grant ANR-20-CE45-0023-01). We combine computer simulations and Approximate Bayesian computation with simultaneous multiple microscopy methods (FCS and spt-PALM) to quantify the way transcription factors explore the nucleus to find their binding sites
EngFlea
Participants: Arnaud Hubert, Leonardo Trujillo Lugo, Hugues Berry.
-
Title:
Engram of fast learning in the striatum
-
Partner Institution(s):
- CIRB, Collège de France, Paris (supervision)
- Inria, Lyon (supervision)
-
Date/Duration:
2022-2026
-
Additionnal info/keywords:
Funded by the French National Agency for Research (ANR), Call “AAP2021" (grant ANR-21-CE16-0022-02). We study the link between endocannabinoid-mediated synaptic plasticity and fast learning of rodents thanks to a multidisciplinary approach combining in vitro and in vivo experimental neurophysiology with detailed subcellular biophysical models and large-scale neural network models.
SecNet
Participants: Schayma Ben Marzougui-El Garrai, Hugues Berry, Audrey Denizot.
-
Title:
Spatio-temporal dynamics of second messenger networks
-
Partner Institution(s):
- Institut de la Vision, Paris (supervision)
- Inria, Lyon
-
Date/Duration:
2023-2026
-
Additionnal info/keywords:
Funded by the French National Agency for Research (ANR), Call “AAP2022" (grant 2023-ANR-22-CE16-0034-02). We combine cell biology approaches and mathematical modeling to provide a description of compartmentalized networks of second messengers that specifically regulate axon guidance and cell migration in response to repellent molecules.
AIRACLES
Participants: Thomas Guyet, Hana Sebia, Arnaud Duvermy.
-
Title:
Chair AIRACLES (Fondation APHP)
-
Partner Institution(s):
- APHP, Paris
- CentralSupelec, Saclay
- Inria, Lyon
-
Date/Duration:
2020-2024
-
Additionnal info/keywords:
The AI-RACLES Chair in Artificial Intelligence, created in 2020 and co-directed by Etienne Audureau (AP-HP), Thomas Guyet (Inria), Laurent Le Brusquet and Arthur Tenenhaus (CentraleSupélec), aims at exploiting the massive data of the AP-HP's Health Data Warehouse (HDW) in order to carry out research focused on the exploration of the concept of vulnerability in health, whether related to ageing or to pathologies such as cancer or COVID-19.
SAFEPaw
Participants: Thomas Guyet, Francois-Elie Calvier.
-
Title:
SAFEPaw (PEPR Santé Numérique)
-
Partner Institution(s):
- CNRS, Paris
- Université de Tours
- Ecole Normale Supérieure Paris-Saclay
- Aix-Marseille Université
- Ecole des Hautes Etudes En Santé Publique
- CHU Grenoble - Université Grenoble Alpes
- CHU Bordeaux - Université de Bordeaux
- Mines Saint-étienne
- Inria, Lyon
-
Date/Duration:
2023-2027
-
Additionnal info/keywords:
The SAFEPaw project is a multidisciplinary project to question the improvement or optimization of care organization by distinguishing three points of view: Regulators / Patients / Healthcare professionals that includes doctors, health care institutions and ambulatory care. Our contribution to this project is to develop tools that would support decision making about the organization of care. It requires dually to be able to describe what is actually the current organization of care and to identify changes that may be improved or optimized. For that, we develop innovative visualization, data mining and operational research tools for care pathways analysis, management and planning. Their originality lays in their ability to consider three views of the care pathways: the patient, the regulator and the provider.
9.3 Regional initiatives
QuickRare
Participants: Thomas Guyet.
-
Title:
QuickRare
-
Partner Institution(s):
- HCL, Lyon
- Univ Lyon 3
- Inria, Lyon
-
Date/Duration:
sept 2024-2026
-
Additionnal info/keywords:
QuickRare is a multidisciplinary project that aims to develop and analyse AI tools to reduce the lenght of patients' journey before obtaining a diagnosis for a rare disease. The technical challenge is to conceive a decision support tool that could be used by general practioners simply by providing past medical reports of patients. The project is focused on the decision to address a patient to a rare disease reference center dedicated to the pediatric nephrology diseases. The team is complemented with social science researchers and philosophers who are investigating questions raised by the use of AI tools in this context. QuickRare is funded by a “Projet d'amorçage” grant of the SHAPE-Med@Lyon funding program.
BrainChat
Participants: Hugues Berry.
-
Title:
BrainChat
-
Partner Institution(s):
- Inria, Lyon
- SBRI, Inserm U1208, Lyon
- Univ Claud Bernard Lyon 1
-
Date/Duration:
sept 2024-2026
-
Additionnal info/keywords:
BrainChat joins forces between teams and platforms working in neurosciences, single cell dataset production and digital sciences to develop a workflow to extract transcriptional signatures related to neurological diseases, while discarding confounding information (i.e. transcriptional differences related to their maturation and/or regional location). Our goal is to produce high resolution spatial RNA-Sequencing datasets that will add important spatial information on the dysregulated processes as well as the proximity of diseased astrocytes to neurons and develop data analysis methods to predict the molecular interactions occurring between astrocytes and with neurons, both in terms of ligand-receptor interactions and in terms of metabolic interactions (cholesterol, lactate…). BrainChat is funded by a “Projet d'amorçage” grant of the SHAPE-Med@Lyon funding program.
10 Dissemination
10.1 Promoting scientific activities
10.1.1 Scientific events: organisation
General chair, scientific chair
- Scientific chair of the French conference on Knowledge management and discovery (Extraction et Gestion de Connaissances – EGC) to be held in January 2025, Thomas Guyet
- Organizer of the hybrid international workshop “Linking brain structure & function at the nanoscale: an interdisciplinary workshop”, September 2024, Lyon, Audrey Denizot
.
Invited speakers:
- Carles Bosch, The Francis Crick Institute, England
- Corrado Cali, Neuroscience Institute Cavalieri Ottolenghi, Italy
- Michael Chirillo, University of Texas, USA
- Audrey Denizot, Inria, France
- Erik De Schutter, Okinawa Institute of Science and Technology, Japan
- Christel Genoud, Université de Lausanne, Switzerland
- Daniel Keller, Ecole Polytechnique Fédérale de Lausanne, Switzerland
- Constantin Pape, University of Göttingen, Germany
- Karin Pernet-Gallay, Université Grenoble Alpes, France
- Padmini Rangamani, University of California San Diego, USA
- Chris Salmon, McGill University, Canada
Member of the organizing committees
- Member of the organizing commitee of the conference of the young scientists of the GDR Imabio, June 2024, Nathan Quiblier
10.1.2 Scientific events: selection
Chair of conference program committees
- Co-chair of the tenth AALTD workshop colocated with the conference ECML 2024, Thomas Guyet
Reviewer
- Maëlle Moranges reviewed 1 article for the French conference on Knowledge management and discovery (Extraction et Gestion de Connaissances - EGC) and 3 articles for the “EXPLAIN’AI” workshop.
- Thomas Guyet reviewed for ECAI, ECML, IDA, IJCAI, TIME, AAAI, SimBig, EGC
10.1.3 Journal
Member of the editorial boards
- Hugues Berry has been Associate Editor (since 2018) then Section Editor for Neuroscience (from June 2024) of PLoS Computational Biology.
- Thomas Guyet is member of the Editorial Committee of Revue Ouverte d'Intelligence Artificielle.
Reviewer - reviewing activities
- Thomas Guyet reviewed 1 paper for each of the following journals: Transactions on Systems, Man and Cybernetics, Transactions on Pattern Analysis and Machine Intelligence
- Audrey Denizot reviewed articles for Cell, eLife, PLOS Computational Biology, and Communications Biology.
10.1.4 Invited talks
- “Linking astrocyte nano-architecture and function: insights from computational tools”, “Linking brain structure & function at the nanoscale: an interdisciplinary workshop”, Lyon, France, 09/2024, Audrey Denizot
- “Linking astrocyte shape to function at the nanoscale: insights from computational approaches”, Astrocyte Cafe, online, 06/2024, Audrey Denizot
- “Towards elucidating astrocyte function in the brain: insights from stochastic spatially-extended models”, Groupe de Travail Maths-Bio, Lyon, France, 05/2024, Audrey Denizot
- “Linking astrocyte morphology to function: insights from computational approaches”, GliaLab, Oslo University, Norway, 02/2024, Audrey Denizot
- “Dissecting astrocyte function with computational models”, Simula, Numerical Analysis and Scientific Computing (SCAN) department meeting, Oslo, Norway, 02/2024, Audrey Denizot
- International Workshop “Shaping the Future of AI and Health”, Oxford (UK), september 2024, Thomas Guyet
- “Folles journées de l'anévrisme”, Nantes, septembre 2024, Thomas Guyet
- Worshop on “Méthodes analyse données biographiques”, INED, novembre 2024, Thomas Guyet
- Meeting “Inria x France Biotech – DonneesSynthetiques”, PariSantéCampus, octobre 2024, Thomas Guyet
- International and Interdisciplinary Summer School on Digital Health (EINS), Sherbrooke (CA), juin 2024, Thomas Guyet
- “Access to SNDS data for research in medical data science”, Yearly MADICS meeting, Blois, mai 2024, Thomas Guyet
- OPTISOINS projet, Bernoulli Lab Meeting, mars 2024, Thomas Guyet
- “Génération d'explications contrefactuelles”, invited presentation to the XAI workshop at EGC, January 2024, Thomas Guyet
- Exploring the possible effects of astrocyte signal integration scenarios on the dynamics of stochastic spiking network, 4th ”Synaptic Microenvironment” Mini-symposium and Workshop, Solden, Austria, March 2024, Hugues Berry
- Le parti pris de modélisation multi-échelle dans le PEPR Santé Numérique, Seminaire Inrae Emerg'in + LiPh4SAS, Tours, May 2024, Hugues Berry
- L’apport de l’IA dans la recherche biomédicale : état des lieux et perspectives, 48e colloque annuel de l'AFSTAL, Lille, June 2024, Hugues Berry
10.1.5 Poster presentations
- “Linking astrocyte function to cell shape: insights from computational models”, French Glial Cell Club annual meeting, Sète, France, 10/2024, Audrey Denizot
- “Exploring behavioral correlations with neuron activity through synaptic plasticity”, Bernstein Conference, Frankfurt am Main, Germany, Sept 2024, Arnaud Hubert
- “Computational modeling of neurovascular coupling at the gliovascular unit”, French Glial Cell Club annual meeting, Sète, France, 10/2024, Florian Dupeuble
10.1.6 Leadership within the scientific community
- Vice-president of the French Association for Artificial Intelligence, Thomas Guyet
- Chair of the steering commity of the French Platform of Artificial Intelligence, Thomas Guyet
- Member of the steering committee of the TIME Symposium, Thomas Guyet
- Appointed member of the INSERM 7th specialized scientific board (CSS7 “Technologies de sante”) , Hugues Berry .
- Chair of Inserm's Search Committee for Senior Research Scientists (President jury d'admissibilité DR2 2024), for CSS7 “Technologies de santé”, Hugues Berry .
- Inria's representative at the Board of Directors (Conseil d'administration) of Lyonbiopôle, the Lyon competitiveness cluster (Pôle de compétitivité), Hugues Berry .
- Scientific coordinator/Chargé de mission of the Inria Lyon Research Center for the interactions with the local Lyon biomedical ecosystem, Hugues Berry .
10.1.7 Scientific expertise
- Member of the evaluation panel AAPG24-CE45 (interface between mathematics, computer science, biology & health) of the French National Research Agency (ANR), Audrey Denizot
- Member of the scientific board of EBRAINS-FR, the french node of the EU research infrastructure EBRAINS, Hugues Berry .
- Member of the Comité d'Expertise et Scientifique pour les Recherches, les Etudes et les Evaluations dans le domaine de la Santé (CESREES), Hugues Berry .
- Member of the GTECH (Technical Assessment Group) of the local competitiveness cluster, LyonBiopôle, Hugues Berry .
- Evaluator of applications for call for proposals of ANR (French National Research Agency) AAP “Générique”, Hugues Berry .
- Member of the jury of “Prix de Thèse AFIA”, Thomas Guyet
- Member of the scientific board of BRGM/JUNON project developping a digital twin for environment and water management, Thomas Guyet .
- Member of the national evaluation committee of Inrae Engineers (section Computer Science), Thomas Guyet
- Scientific correspondent of the “Comité opérationnel d’évaluation des risques légaux et éthiques” (Coerle) for Inria Lyon, Jan-Michael Rye
10.1.8 Research administration
- Member of the Comité Stratégique de Pilotage for the INRAE "DigitBio" metaprogram, Hugues Berry
- Inria's representative at the Comité de la Recherche en Matiere Biomedicale et de Sante Publique (CRBSP), Hugues Berry
10.2 Teaching - Supervision - Juries
10.2.1 Supervision
- Master internship, Zoë Laffitte , UCBL, Lyon, France, entitled "Implementation of an open-access database of 3D cell meshes for reaction-diffusion simulations", 11/2023-07/2024, Audrey Denizot , Jan-Michael Rye
10.2.2 Juries
- Member of the PhD evaluation committee of D.-W. Garcia, Paris-Saclay, Audrey Denizot
- Reviewer for H. Le Bayer's PhD jury (Univ. de Montpellier), 2024, Thomas Guyet
- Reviewer for B. Cissoko's PhD jury (Univ. de Strasbourg), 2024, Thomas Guyet
- Reviewer for C. Bolut's PhD jury, Univ. Toulouse III, France, July 2024, Hugues Berry
- Reviewer for O. Bin Ka'b Ali's PhD jury, Concordia Univ., Montreal, Canada, March 2024, Hugues Berry
- Examiner for M. di Volo's HDR jury, Univ. Claude-Bernard Lyon 1, France, March 2024, Hugues Berry
- Examiner for C. Manfredotti's HDR jury (AgroParisTech), 2024, Thomas Guyet
10.3 Popularization
10.3.1 Specific official responsibilities in science outreach structures
- Member of the board of directors & editorial committee of the Papier Mâché association. Goal: explain the content of scientific publications in French & outline the scientific method & publication process, Audrey Denizot
10.3.2 Participation in Live events
- Participation to "Journée Filles et informatique : une équation lumineuse", ENS Lyon, France ; speed-meeting with women studying in high school, 11/2024 Audrey Denizot , Andréa Ducos
- conference “Simuler numériquement le fonctionnement de notre cerveau pour mieux le soigner”, Université Ouverte Lyon 1, “Créer des mondes informatiques pour explorer le vivant”, Villeurbanne, France, 02/2024, Audrey Denizot
11 Scientific production
11.1 Major publications
11.2 Publications of the year
International journals
- 3 articleModeling compartmentalization within intracellular signaling pathway.ESAIM: Proceedings and Surveys77November 2024, 100-122HALDOIback to text
- 4 articleA Coupled Neural Field Model for the Standard Consolidation Theory.Journal of Theoretical Biology5882024, 111818HALback to text
- 5 articleClustering of timed sequences – Application to the analysis of care pathways.Data and Knowledge Engineering156March 2025, 102401HALDOIback to text
- 6 articleSWoTTeD: an extension of tensor decomposition to temporal phenotyping.Machine LearningApril 2024HALDOIback to textback to text
Invited conferences
- 7 inproceedingsDécouvrir des trajectoires de soins typiques dans le SNDS.La folle journée de l'anévrismeNantes, FranceDecember 2024HAL
International peer-reviewed conferences
- 8 inproceedingsPlateformisation de l'apprentissage machine en épidémiologie : enjeux philosophiques.Conférence National en Intelligence ArtificielleLa Rochelle, FranceJuly 2024HAL
- 9 inproceedingsPlateformisation de l’apprentissage machine en épidémiologie: enjeux philosophiques.Conférence Nationale en Intelligence ArtificielleLa Rochelle, FranceJune 2024HAL
National peer-reviewed Conferences
- 10 inproceedingsCatégorisation de séquences temporelles - Application à l'analyse de parcours de soins.Extraction et Gestion des Connaissances (EGC)RNTI-E-40Dijon, FranceRNTIJanuary 2024, 119-130HAL
Conferences without proceedings
- 11 inproceedingsSNDS: contexte, contenu et usages de la base de l'assurance maladie.Symposium MADICSBlois, FranceMay 2024HAL
Scientific books
- 12 bookD.Dominique Longin, N.Nadia Abchiche-Mimouni, G.Ghislain Atemezing, N.Nathalie Aussenac-Gilles, J.-G.Jean-Guy Mailly, C.Catherine Roussey, F.François Schwarzentruber, A.Anaelle Wilczynski and H.Haïfa Zargayouna, eds. Conférence Nationale d’Intelligence Artificielle Année 2024.Association Française pour l'Intelligence ArtificielleOctober 2024HAL
Scientific book chapters
Reports & preprints
- 14 miscEnhancing Fluorescence Correlation Spectroscopy with machine learning to infer anomalous molecular motion.January 2025HAL
- 15 miscAutomatic analysis of negation cues and scopes for medical texts in French using language models.April 2024HAL
- 16 miscVascular Segmentation of Functional Ultrasound Images using Deep Learning.October 2024HAL
Other scientific publications
- 17 inproceedingsSingle-cell multi-omics data integration powered by PCA-like autoencoders.JOBIM 2024Toulouse, FranceJune 2024HAL
11.3 Cited publications
- 18 articleG-Protein-Coupled Receptors in CNS: A Potential Therapeutic Target for Intervention in Neurodegenerative Disorders and Associated Cognitive Deficits.Cells922020, 506back to text
- 19 inproceedingsSimulation of Astrocytic Calcium Dynamics in Lattice Light Sheet Microscopy Images.IEEE International Symposium on Biomedical Imaging, ISBI2021, 135-139back to text
- 20 articleAstrocyte calcium signaling: the third wave.Nature Neuroscience192016, 182-189back to text
- 21 articleFailed trials for central nervous system disorders do not necessarily invalidate preclinical models and drug targets.Nature Reviews Drug Discovery152016, 516back to text
- 22 inproceedingsSubjective interestingness in exploratory data mining.International Symposium on Intelligent Data Analysis2013, 19-31back to text
- 23 inproceedingsOntology-mediated query answering: harnessing knowledge to get more from data.Proceedings IJCAI'162016, 4058-4061back to text
- 24 articleDiscovering governing equations from data by sparse identification of nonlinear dynamical systems.Proc Natl Acad Sci USA1132016, 3932back to text
- 25 articleACE: the Advanced Cohort Engine for searching longitudinal patient records.J Am Med Inform Assoc2872021, 1468-1479back to text
- 26 incollectionMeCP2 Dysfunction in Rett Syndrome and Neuropsychiatric Disorders.Psychiatric Disorders: Methods and Protocols, Methods in Molecular Biology2011Humana, New York, NY2019, 573-592back to text
- 27 articleSingle Cell Transcriptome Analysis of Niemann-Pick Disease, Type C1 Cerebella.Int J Mol Sci212020, 5368back to text
- 28 articleEndocannabinoids mediate bidirectional striatal spike-timing dependent plasticity.J Physiol593132015, 2833-2849back to text
- 29 articleRobustness of STDP to spike timing jitter.Scientific Reports82018, 8139back to text
- 30 articleEndocannabinoid dynamics gate spike-timing dependent depression and potentiation.eLife52016, e13185back to text
- 31 articleAstroglial-Kir4.1 in lateral habenula drives neuronal bursts in depression.Nature5542018, 323-327back to text
- 32 articleSimulation of calcium signaling in fine astrocytic processes: effect of spatial properties on spontaneous activity.PLoS Comput Biol1582019, 1006795back to text
- 33 articleStochastic spatially-extended simulations predict the effect of ER distribution on astrocytic microdomain Ca2+ activity.ACM NanoCom202021, 1-5back to text
- 34 articleContested futures: envisioning "Personalized', Stratified," and "Precision" medicine.New Genet Soc3832019, 308-330back to text
- 35 articleRepresenting high throughput expression profiles via perturbation barcodes reveals compound targets.PLoS Comput Biol1322017, 1005335back to text
- 36 articleBridging the Valley of Death of therapeutics for neurodegeneration.Nature Medicine162010, 1227-1232back to text
- 37 articleModulation of spike-timing dependent plasticity: towards the inclusion of a third factor in computational models.Frontiers in Computational Neuroscience122018, 49back to text
- 38 articlePhe2vec: Automated disease phenotyping based on unsupervised embeddings from electronic health records.Patterns292021, 100337back to text
- 39 articleNonlinear Responses in fMRI: The Balloon Model, Volterra Kernels, and Other Hemodynamics.NeuroImage122000, 466-477back to text
- 40 articleValley of death: A proposal to build a "translational bridge" for the next generation.Neurosci Res1152017, 1-4back to text
- 41 articleBDNF controls bidirectional endocannabinoid-plasticity at corticostriatal synapses.Cerebral Cortex1302020, 197-217back to text
- 42 articleArtificial intelligence to deep learning: machine intelligence approach for drug discovery.Molecular Diversity252021, 1315-1360back to text
- 43 articleG Protein-coupled Receptor Biased Agonism.J Cardiovasc Pharmacol6732016, 193-202back to text
- 44 articleRett syndrome: insights into genetic, molecular and circuit mechanisms.Nat Rev Neurosci192018, 368-382back to textback to text
- 45 articleMulti-timescale Modeling of Activity-Dependent Metabolic Coupling in the Neuron-Glia-Vasculature Ensemble.PLoS Comput Biol112015, 1004036back to text
- 46 articleImproving clinical trial outcomes in amyotrophic lateral sclerosis.Nature Reviews Neurology1712 2020back to text
- 47 articleSystems pharmacology in drug development and therapeutic use — A forthcoming paradigm shift.Eur J Pharm Sci942016, 4-14back to text
- 48 articleEvaluation of connectivity map shows limited reproducibility in drug repositioning.Sci Rep1112013, 17624back to text
- 49 articleThe role of cholesterol metabolism in multiple sclerosis: From molecular pathophysiology to radiological and clinical disease activity.Autoimmunity Rev2162022, 103088back to text
- 50 incollectionDiscovering alignment relations with Graph Convolutional Networks: a biomedical case study.2021, 03452182back to text
- 51 articleTranslating biased agonists from molecules to medications: Serotonin 5-HT1A receptor functional selectivity for CNS disorders.Pharmacology Therapeutics1079372021back to text
- 52 articleStrategies to Address Challenges in Neuroscience Drug Discovery and Development.International Journal of Neuropsychopharmacology222019, 445 - 448back to textback to text
- 53 articleUsing quantitative systems pharmacology for novel drug discovery.Expert Opin Drug Discov10122015, 1315-1331back to text
- 54 articleNPC intracellular cholesterol transporter 1 (NPC1)-mediated cholesterol export from lysosomes.J Biol Chem29452019, 1706-1709back to textback to text
- 55 inproceedingsD-code: discovering closed-form ODEs from observed trajectories.Proceedings ICLR 20212021back to text
- 56 articlePhysics-informed neural networks : A deep-learning framework for solving forward and inverse problem involving nonlinear partial differential equations.J Comput Phys3782019, 686-707back to text
- 57 articleA review of approaches to identifying patient phenotype cohorts using electronic health records.J Am Med Inform Assoc2122014, 221-230back to text
- 58 articleTranscriptome level analysis in Rett syndrome using human samples from different tissues.Orphanet J Rare Dis1312018, 113back to text
- 59 inproceedingsOn subjective measures of interestingness in knowledge discovery.Proceedings KDD'951995, 275-281back to text
- 60 articleThe synaptic plasticity and memory hypothesis: encoding, storage and persistence.Philos Trans R Soc Lond B3692014, 20130288back to text
- 61 articleApplications of machine learning in drug discovery and development.Nature Reviews Drug Discovery182019, 463-477back to text
- 62 articleNiemann-Pick disease type C.Orphanet J Rare Dis5162010back to text
- 63 articlePhysiology of Astroglia.Physiol Rev9812018, 239-289back to text
- 64 articleIn vivo biased agonism at 5-HT1A receptors: characterisation by simultaneous PET/MR imaging.Neuropsychopharmacology2018, 2310-2319back to text
- 65 articleInheritance and Drug Response.N. Engl J Med3482003, 529-537back to text
- 66 articleDopamine- endocannabinoid interactions mediate spike-timing dependent potentiation in the striatum.Nature Communications92018, 4118back to text
- 67 inproceedingsLearning phenotypes and dynamic patient representations via rnn regularized collective non-negative tensor factorization.Proceedings of the 2019 AAAI Conference on Artificial Intelligence2019, 1246-1253back to text
- 68 articleAstrocyte-only Npc1 reduces neuronal cholesterol and triples life span of Npc1-/- mice.J Neurosci Res862008, 2848-2856back to text