2023Activity reportProject-TeamGEOSTAT
RNSR: 200920943E- Research center Inria Centre at the University of Bordeaux
- Team name: Geometry and Statistics in acquisition data
- Domain:Applied Mathematics, Computation and Simulation
- Theme:Optimization, machine learning and statistical methods
Keywords
Computer Science and Digital Science
- A3.4.2. Unsupervised learning
- A3.4.7. Kernel methods
- A3.4.8. Deep learning
- A5.3. Image processing and analysis
- A5.3.2. Sparse modeling and image representation
- A5.3.3. Pattern recognition
- A5.3.5. Computational photography
- A5.7. Audio modeling and processing
- A5.7.3. Speech
- A5.7.4. Analysis
- A5.9. Signal processing
- A5.9.2. Estimation, modeling
- A5.9.3. Reconstruction, enhancement
- A5.9.5. Sparsity-aware processing
- A5.9.6. Optimization tools
- A6.1.2. Stochastic Modeling
- A7.3. Calculability and computability
- A8.6. Information theory
- A9.2. Machine learning
Other Research Topics and Application Domains
- B2. Health
- B2.2. Physiology and diseases
- B2.2.1. Cardiovascular and respiratory diseases
- B2.2.6. Neurodegenerative diseases
- B3. Environment and planet
- B3.3. Geosciences
- B3.3.2. Water: sea & ocean, lake & river
- B3.3.4. Atmosphere
1 Team members, visitors, external collaborators
Research Scientists
- Nicolas Brodu [Team leader, INRIA, Researcher, from Oct 2023, HDR]
- Hussein Yahia [Team leader, INRIA, Researcher, until Oct 2023, HDR]
- Nicolas Brodu [INRIA, Researcher, until Oct 2023, HDR]
- Khalid Daoudi [INRIA, Researcher, HDR]
- Hussein Yahia [INRIA, Researcher, from Oct 2023, HDR]
Post-Doctoral Fellows
- Alexandra Jurgens [INRIA, Post-Doctoral Fellow, from Feb 2023]
- Marina Saez Andreu [INRIA, Post-Doctoral Fellow, until Aug 2023]
- Farouk Yahaya [INRIA, Post-Doctoral Fellow, until Jul 2023]
- Bertrand Ygorra [INRIA, Post-Doctoral Fellow]
PhD Student
- Valentine Sollier [INRIA, from Nov 2023]
Technical Staff
- Zhe Li [INRIA, Engineer, until Jan 2023]
Administrative Assistant
- Flavie Blondel [INRIA]
External Collaborator
- Yao Liu [UNIV NEWCASTLE, from Feb 2023 until Feb 2023]
2 Overall objectives
Geostat is a research project which investigates the analysis of some classes of natural complex signals (physiological time series, turbulent universe and earth observation data sets) by determining, in acquired signals, the properties that are predicted by commonly admitted or new physical models best fitting the phenomenon. Consequently, when statistical properties discovered in the signals do not match closely enough those predicted by accepted physical models, we question the validity of existing models or propose, whenever possible, modifications or extensions of existing models. A new direction of research, based on the Concaust exploratory action and the newly accepted (in February 2021) associated team Comcausa proposed by N. Brodu with USA / UC Davis, Complexity Sciences Center, Physics Department is developped in the team.
An important aspect of the methodological approach is that we don't rely on a predetermined "universal" signal processing model to analyze natural complex signals. Instead, we take into consideration existing approaches in nonlinear signal processing (wavelets, multifractal analysis tools such as log-cumulants or micro-canonical multifractal formalism, time frequency analysis etc.) which are used to determine the micro structures or other micro features inside the acquired signals. Then, statistical analysis of these micro data are determined and compared to expected behaviour from theoretical physical models used to describe the phenomenon from which the data is acquired. From there different possibilities can be contemplated:
- The statistics match behaviour predicted by the model: complexity parameters predicted by the model are extracted from signals to analyze the dynamics of underlying phenomena. Examples: analysis of turbulent data sets in Oceanography and Astronomy.
- The signals displays statistics that cannot be attainable by the common lore of accepted models: how to extend or modify the models according to the behaviour of observed signals ? Example: audio speech signals.
Geostat is a research project in nonlinear signal processing which develops on these considerations: it considers the signals as the realizations of complex extended dynamical systems. The driving approach is to describe the relations between complexity (or information content) and the geometric organization of information in a signal. For instance, for signals which are acquisitions of turbulent fluids, the organization of information may be related to the effective presence of a multiscale hierarchy of coherent structures, of multifractal nature, which is strongly related to intermittency and multiplicative cascade phenomena ; the determination of this geometric organization unlocks key nonlinear parameters and features associated to these signals; it helps understand their dynamical properties and their analysis. We use this approach to derive novel solution methods for super-resolution and data fusion in Universe Sciences acquisitions 14. Specific advances are obtained in Geostat in using this type of statistical/geometric approach to get validated dynamical information of signals acquired in Universe Sciences, e.g. oceanography or astronomy. The research in Geostat encompasses nonlinear signal processing and the study of emergence in complex systems, with a strong emphasis on geometric approaches to complexity. Consequently, research in Geostat is oriented towards the determination, in real signals, of quantities or phenomena, usually unattainable through linear methods, that are known to play an important role both in the evolution of dynamical systems whose acquisitions are the signals under study, and in the compact representations of the signals themselves.
Signals studied in Geostat belong to two broad classes:
- Acquisitions in astronomy and earth observation.
- Physiological time series.
3 Research program
3.1 General methodology
- Fully Developed Turbulence (FDT) Turbulence at very high Reynolds numbers; systems in FDT are beyond deterministic chaos, and symmetries are restored in a statistical sense only, and multi-scale correlated structures are landmarks. Generalizing to more random uncorrelated multi-scale structured turbulent fields.
- Compact Representation Reduced representation of a complex signal (dimensionality reduction) from which the whole signal can be reconstructed. The reduced representation can correspond to points randomly chosen, such as in Compressive Sensing, or to geometric localization related to statistical information content (framework of reconstructible systems).
- Sparse representation The representation of a signal as a linear combination of elements taken in a dictionary (frame or Hilbertian basis), with the aim of finding as less as possible non-zero coefficients for a large class of signals.
- Universality class In theoretical physics, the observation of the coincidence of the critical exponents (behaviour near a second order phase transition) in different phenomena and systems is called universality. Universality is explained by the theory of the renormalization group, allowing for the determination of the changes followed by structured fluctuations under rescaling, a physical system is the stage of. The notion is applicable with caution and some differences to generalized out-of-equilibrium or disordered systems. Non-universal exponents (without definite classes) exist in some universal slowing dynamical phenomena like the glass transition and kindred. As a consequence, different macroscopic phenomena displaying multiscale structures (and their acquisition in the form of complex signals) may be grouped into different sets of generalized classes.
Geostat is a research project which investigates the analysis of some classes of natural complex signals (physiological time series, turbulent universe and earth observation data sets) by determining, in acquired signals, the properties that are predicted by commonly admitted or new physical models best fitting the phenomenon. The team makes fundamental and applied research in the analysis of complex natural signals using paradigms and methods from statistical physics such as: scale invariance, predictability, universality classes. We study the parameters related to common statistical organization in different complex signals and systems, we derive new types of sparse and compact representations, and machine learning approaches. We are also developing tools for the analysis of complex signals that better match the statistical and geometrical organisation inside these data: as a typical example, we cite the evaluation of cascading properties of physical variables inside complex signals.
When statistical properties discovered in the signals do not match closely enough those predicted by accepted physical models, we question the validity of existing models or propose, whenever possible, modifications or extensions of existing models. Also, a direction of research, based on the Concaust exploratory action and the newly accepted (in February 2021) associated team Comcausa proposed by N. Brodu with USA / UC Davis, Complexity Sciences Center, Physics Department is developped in the team.
Every signal conveys, as a measure experiment, information on the physical system whose signal is an acquisition of. As a consequence, it seems natural that signal analysis or compression should make use of physical modelling of phenomena: the goal is to find new methodologies in signal processing that goes beyond the simple problem of interpretation. Physics of disordered systems, and specifically physics of (spin) glasses is putting forward new algorithmic resolution methods in various domains such as optimization, compressive sensing etc. with significant success notably for NP hard problem heuristics. Similarly, physics of turbulence introduces phenomenological approaches involving multifractality. Energy cascades are indeed closely related to geometrical manifolds defined through random processes. At these structures’ scales, information in the process is lost by dissipation (close to the lower bound of inertial range). However, all the cascade is encoded in the geometric manifolds, through long or short distance correlations depending on cases. How do these geometrical manifold structures organize in space and time, in other words, how does the scale entropy cascades itself? To unify these two notions, a description in term of free energy of a generic physical model is sometimes possible, such as an elastic interface model in a random nonlinear energy landscape : This is for instance the correspondence between compressible stochastic Burgers equation and directed polymers in a disordered medium. Thus, trying to unlock the fingerprints of cascade-like structures in acquired natural signals becomes a fundamental problem, from both theoretical and applicative viewpoints.
An important aspect of the methodological approach is that we don't rely on a predetermined "universal" signal processing model to analyze natural complex signals. Instead, we take into consideration existing approaches in nonlinear signal processing (wavelets, multifractal analysis tools such as log-cumulants or micro-canonical multifractal formalism, time frequency analysis etc.) which are used to determine the micro structures or other micro features inside the acquired signals. Then, statistical analysis of these micro data are determined and compared to expected behavior from theoretical physical models used to describe the phenomenon from which the data is acquired. From there different possibilities can be contemplated:
-
-
The statistics match behaviour predicted by the model: complexity parameters predicted by the model are extracted from signals to analyze the dynamics of underlying phenomena. Examples: analysis of turbulent data sets in Oceanography and Astronomy.
-
-
The signals displays statistics that cannot be attainable by the common lore of accepted models: how to extend or modify the models according to the behaviour of observed signals? Example: audio speech signals.
We focus on the following theoretical developments:
- Signal processing using methods from complex systems and statistical physics,
- Sparse and compact representations, signal reconstruction, machine learning,
- Predictability in complex systems,
- Analysis, classification, detection in complex signals.
The team conducts research in nonlinear signal processing on these considerations: we consider the signals as the realizations of complex extended dynamical systems. The driving approach is to describe the relations between complexity (or information content) and the geometric organization of information in a signal. For instance, for signals which are acquisitions of turbulent fluids, the organization of information may be related to the effective presence of a multiscale hierarchy of coherent structures, of multifractal nature, which is strongly related to intermittency and multiplicative cascade phenomena ; the determination of this geometric organization unlocks key nonlinear parameters and features associated to these signals; it helps understand their dynamical properties and their analysis. We use this approach to derive novel solution methods for super-resolution and data fusion in Universe Sciences acquisitions. Specific advances are obtained in using this type of statistical/geometric approach to get validated dynamical information of signals acquired in Universe Sciences, e.g. Oceanography or Astronomy. The approach encompasses nonlinear signal processing and the study of emergence in complex systems, with a strong emphasis on geometric approaches to complexity. Consequently, research is oriented towards the determination, in real signals, of quantities or phenomena, usually unattainable through linear methods, that are known to play an important role both in the evolution of dynamical systems whose acquisitions are the signals under study, and in the compact representations of the signals themselves.
Signals under study belong to two broad classes:
- Acquisitions in Astronomy and Earth Observation.
- Physiological time series.
3.2 Turbulence in insterstellar clouds and Earth observation data
The analysis and modeling of natural phenomena, specially those observed in geophysical sciences and in astronomy, are influenced by statistical and multiscale phenomenological descriptions of turbulence; indeed these descriptions are able to explain the partition of energy within a certain range of scales. A particularly important aspect of the statistical theory of turbulence lies in the discovery that the support of the energy transfer is spatially highly non uniform, in other terms it is intermittent51. Because of the absence of localization of the Fourier transform, linear methods are not successful to unlock the multiscale structures and cascading properties of variables which are of primary importance as stated by the physics of the phenomena. This is the reason why new approaches, such as DFA (Detrented Fluctuation Analysis), Time-frequency analysis, variations on curvelets 48 etc. have appeared during the last decades. Recent advances in dimensionality reduction, and notably in compressive sensing, go beyond the Nyquist rate in sampling theory using nonlinear reconstruction, but data reduction occur at random places, independently of geometric localization of information content, which can be very useful for acquisition purposes, but of lower impact in signal analysis. We are successfully making use of a microcanonical formulation of the multifractal theory, based on predictability and reconstruction, to study the turbulent nature of interstellar molecular or atomic clouds. Another important result obtained in Geostat is the effective use of multiresolution analysis associated to optimal inference along the scales of a complex system. The multiresolution analysis is performed on dimensionless quantities given by the singularity exponents which encode properly the geometrical structures associated to multiscale organization. This is applied successfully in the derivation of high resolution ocean dynamics, or the high resolution mapping of gaseous exchanges between the ocean and the atmosphere; the latter is of primary importance for a quantitative evaluation of global warming. Understanding the dynamics of complex systems is recognized as a new discipline, which makes use of theoretical and methodological foundations coming from nonlinear physics, the study of dynamical systems and many aspects of computer science. One of the challenges is related to the question of emergence in complex systems: large-scale effects measurable macroscopically from a system made of huge numbers of interactive agents 24, 45. Some quantities related to nonlinearity, such as Lyapunov exponents, Kolmogorov-Sinai entropy etc. can be computed at least in the phase space 25. Consequently, knowledge from acquisitions of complex systems (which include complex signals) could be obtained from information about the phase space. A result from F. Takens 49 about strange attractors in turbulence has motivated the theoretical determination of nonlinear characteristics associated to complex acquisitions. Emergence phenomena can also be traced inside complex signals themselves, by trying to localize information content geometrically. Fundamentally, in the nonlinear analysis of complex signals there are broadly two approaches: characterization by attractors (embedding and bifurcation) and time-frequency, multiscale/multiresolution approaches. In real situations, the phase space associated to the acquisition of a complex phenomenon is unknown. It is however possible to relate, inside the signal's domain, local predictability to local reconstruction 15 and to deduce relevant information associated to multiscale geophysical signals 16. A multiscale organization is a fundamental feature of a complex system, it can be for example related to the cascading properties in turbulent systems. We make use of this kind of description when analyzing turbulent signals: intermittency is observed within the inertial range and is related to the fact that, in the case of FDT (fully developed turbulence), symmetry is restored only in a statistical sense, a fact that has consequences on the quality of any nonlinear signal representation by frames or dictionaries.
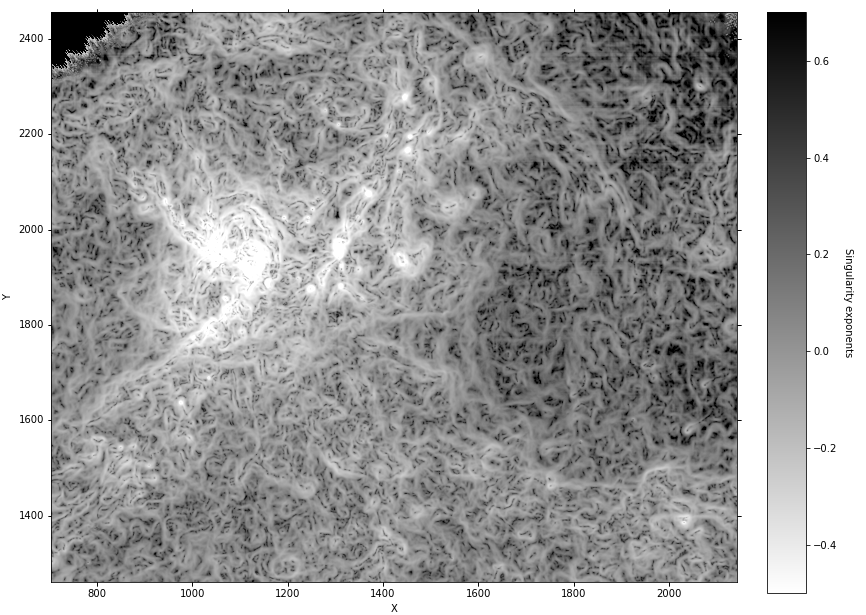
A major contribution of the collaboration with the Bordeaux Laboratory in Astrophysics and the GENESIS project (University of Cologne) is found in the display of the filamentary structure of the interstellar medium, as shown in this visualization of the singularity exponents in a Aquila sub-region Herschel observation map .
The example of FDT as a standard "template" for developing general methods that apply to a vast class of complex systems and signals is of fundamental interest because, in FDT, the existence of a multiscale hierarchy
We show in figure 1 the result of the computation of singularity exponents on an Herschel astronomical observation map (Aquila galactic cloud, sub-region) which has been edge-aware filtered using sparse
3.3 Data analysis, causal modeling, application to earth sciences
Generally speaking, we are interested in developing new methods for inferring models of physical systems from data. The key questions are: What are the important objects of study at each description level? What are the patterns of their interactions? How to detect them in the first place? How to describe them using relevant variables? How to recover and formalize their dynamics, with analytical formula or via a computer program? Powerful mathematical tools and conceptual frameworks have been developed over years of research on complex systems and nonlinear dynamics 31, 37 ; yet the arousal of big data and regain in machine learning popularity have recently stimulated this domain of research 42, 43, 28. Our approach is to focus on how information is produced (emergence of patterns, of large-scale structures) and on how that information is maintained and transformed at different scales 30. We operate on states of similar information content, in the same way that thermodynamics operate on states of similar energy levels. Our goal is to search for the analog of Hamiltonian dynamics, but that describes information transformations instead of energy transformations. This approach would be particularly useful for modeling steady-state systems, which operate far from thermodynamic equilibrium. In these, energy dissipation is a prerequisite for maintaining the observed patterns, and thus not necessarily the most useful metric to investigate. In fact, most natural, physical and industrial systems we deal with fall in this category, while balanced quasi-static assumptions are practical approximation only for scales well below the characteristic scale of the involved processes. Open and dissipative systems are not locally constrained by the inevitable rise in entropy, thus allowing the maintaining through time of ordered structures. And, according to information theory, more order and less entropy means that these structures have a higher information content than the rest of the system, which usually gives them a high functional role. We propose to identify characteristic scales not only with energy dissipation, as usual in signal processing analysis (power spectrum analysis), but most importantly with information content. Information theory can be extended to look at which scales are most informative (e.g. multi-scale entropy 41,
3.4 Vocal biomarkers
Speech convey valuable information about the health (and emotional) state of the speaker. A disturbance in this state can affect some or all the speech production subsystems: respiratory, phonatory, articulatory, nasalic and prosodic. A vocal biomarker is thus a digital signature of a speech signal that is associated with a clinical finding/observation and can be used to assist in: diagnose a disease, monitor patients, assess the severity or stage of a disease, assess response to treatment. Vocal biomarkers could provide attractive solutions in (tele)medicine which are non- invasive, cost-effective and rapidly deployable on large scales. Consequently, during this century, there has been an ever increasing interest in the development of objective vocal biomarkers with a major peak since the Covid-19 pandemic 34, with the emergence of several start- ups in the area (www.sondehealth.com, www.evocalhealth.com,...). Traditionally, most of the research has been carried out on neurodegenerative diseases, particularly on dysarthria, a class of motor speech disorders 33. There is however now a growing contribution in mental, cognitive, respiratory, cardiovascular and other diseases. While there has been definitely a progress in understanding how some pathologies alter speech, the field is still in its early development. One reason is the domination of pure-IA research in this area, while the clinical involvement is crucial unlock the clinical, scientific and technological challenges. In GeoStat, the core strategy of research in this area is to conduct R&D with a close partnership with (top) clinician experts of the pathologies under study, in the form of clinical trials. Our main research has been on differential diagnosis of Parkinsonism. We have thus been conducting an ANR project, Voice4PD-MSA, with the neurology and ENT departments of the university hospitals of Bordeaux and Toulouse. We have been also closely collaborating in a clinical study with Czech partners in Charles university and Technical university of Prague. In these two partnerships, we address the difficult problem of early discrimination between Parkinson’s disease (PD) and atypical Parkinsonian disorders (APD) such as Progressive Supranuclear Palsy (PSP) and Multiple System Atrophy (MSA). Since the Covid-19 pandemic, we started 3 projects with different hospitals of Paris (AP-HP). The first one, VocaPnée, on the remote monitoring of patients with respiratory diseases. The second one, Respeak, on the dispatch assistance of emergency calls. The third (recent) one, AcroVox, on the diagnosis of a rare hormonal disease, acromegalia. All the projects with AP-HP are accompanied by the recently created Bernoulli lab (see). From the methodological perspective, our main goal is to develop a signal processing framework which can be more appropriate to handle the specificities of pathological voices than the classical framework. Indeed, most of the research in this area rely on methods and tools developed for healthy speech, mainly based on the linear and independent source-filter speech production model. In the presence of an impairment, many of the underlying assumptions and models can fail to capture the disease specific alteration(s). This makes it necessary to adapt existing techniques and/or to develop new ones in order to extract the useful features and cues and to achieve the classification or regression targets. Besides following this approach, our ambition is to provide a theoretical framework which can “unify” healthy and pathological speech analysis. We have been recently investigating that “Probabilistic time-frequency analysis (PTFA)” 50 under the framework of Gaussian processes 46 as a promising candidate to achieve this goal. This framework is appealing because it makes it easier and natural to provide adaptive time- frequency representations, control representation sparsity, propagate uncertainty, quantify noise, reveal non-linearity, sampling/synthesis (for data augmentation for instance). As an example, classical spectrograms, wavelets or (a class of) filter-banks become probabilistic inference in this framework. PTFA was introduced in 50 but did not attract a lot of attention from the community, mainly because of the significant computational complexity load of the approach. We are investigating low-rank covariance approximation techniques to overcome this issue. We underline that we chose not to follow (for the moment) the dominating deep learning flow (even in this area which is a small-data problem), because interpretability at the acoustico-phonetic and clinical level is crucial in our setting. We are also putting a significant effort on software development. There exists indeed no publicly available tool for the analysis of pathological speech. We have been thus developing a Python library, VocaPy, where we integrate confirmed, adapted and new algorithms, after solid validation on speech datasets altered by different pathologies. Such a software would be very beneficial to the community and would contribute considerably to the R&D progress in the field (as Praat does in healthy speech analysis).
4 Application domains
Sparse signals & optimization
This research topic involves Geostat team and is used to set up an InnovationLab with I2S company.
Sparsity can be used in many ways and there exist various sparse models in the literature; for instance minimizing the
We have shown that the two powerful concepts of sparsity and scale invariance can be exploited to design fast and efficient imaging algorithms. A general framework has been set up for using non-convex sparsity by applying a first-order approximation. When using a proximal solver to estimate a solution of a sparsity-based optimization problem, sparse terms are always separated in subproblems that take the form of a proximal operator. Estimating the proximal operator associated to a non-convex term is thus the key component to use efficient solvers for non-convex sparse optimization. Using this strategy, only the shrinkage operator changes and thus the solver has the same complexity for both the convex and non-convex cases. While few previous works have also proposed to use non-convex sparsity, their choice of the sparse penalty is rather limited to functions like the
-
Edge aware smoothing: given an input image
, one seeks a smooth image "close" to by minimizing:where
is a sparcity-inducing non-convex function and a positive parameter. Splitting and alternate minimization lead to the sub-problems:We solve sub-problem
through deconvolution and efficient estimation via separable filters and warm-start initialization for fast GPU implementation, and sub-problem through non-convex proximal form. - Structure-texture separation: design of an efficient algorithm using non-convex terms on both the data-fitting and the prior. The resulting problem is solved via a combination of Half-Quadratic (HQ) and Maximization-Minimization (MM) methods. We extract challenging texture layers outperforming existing techniques while maintaining a low computational cost. Using spectral sparsity in the framework of low-rank estimation, we propose to use robust Principal Component Analysis (RPCA) to perform robust separation on multi-channel images such as glare and artifacts removal of flash/no-flash photographs. As in this case, the matrix to decompose has much less columns than lines, we propose to use a QR decomposition trick instead of a direct singular value decomposition (SVD) which makes the decomposition faster.
-
Robust integration: in many applications, we need to reconstruct an image from corrupted gradient fields. The corruption can take the form of outliers only when the vector field is the result of transformed gradient fields (low-level vision), or mixed outliers and noise when the field is estimated from corrupted measurements (surface reconstruction, gradient camera, Magnetic Resonance Imaging (MRI) compressed sensing, etc.). We use non-convexity and multi-sparsity to build efficient integrability enforcement algorithms. We present two algorithms : 1) a local algorithm that uses sparsity in the gradient field as a prior together with a sparse data-fitting term, 2) a non-local algorithm that uses sparsity in the spectral domain of non-local patches as a prior together with a sparse data-fitting term. Both methods make use of a multi-sparse version of the Half-Quadratic solver. The proposed methods were the first in the literature to propose a sparse regularization to improve integration. Results produced with these methods significantly outperform previous works that use no regularization or simple
minimization. Exact or near-exact recovery of surfaces is possible with the proposed methods from highly corrupted gradient fields with outliers. - Learning image denoising: deep convolutional networks that consist in extracting features by repeated convolutions with high-pass filters and pooling/downsampling operators have shown to give near-human recognition rates. Training the filters of a multi-layer network is costly and requires powerful machines. However, visualizing the first layers of the filters shows that they resemble wavelet filters, leading to sparse representations in each layer. We propose to use the concept of scale invariance of multifractals to extract invariant features on each sparse representation. We build a bi-Lipschitz invariant descriptor based on the distribution of the singularities of the sparsified images in each layer. Combining the descriptors of each layer in one feature vector leads to a compact representation of a texture image that is invariant to various transformations. Using this descriptor that is efficient to calculate with learning techniques such as classifiers combination and artificially adding training data, we build a powerful texture recognition system that outperforms previous works on 3 challenging datasets. In fact, this system leads to quite close recognition rates compared to latest advanced deep nets while not requiring any filters training.
5 New software, platforms, open data
5.1 New software
5.1.1 Fluex
-
Keywords:
Signal, Signal processing
-
Scientific Description:
Fluex is a package consisting of the Microcanonical Multiscale Formalism for 1D, 2D 3D and 3D+t general signals.
-
Functional Description:
Fluex is a C++ library developed under Gforge. Fluex is a library in nonlinear signal processing. Fluex is able to analyze turbulent and natural complex signals, Fluex is able to determine low level features in these signals that cannot be determined using standard linear techniques.
-
Contact:
Hussein Yahia
-
Participants:
Hussein Yahia, Rémi Paties
5.1.2 FluidExponents
-
Keywords:
Signal processing, Wavelets, Fractal, Spectral method, Complexity
-
Functional Description:
FluidExponents is a signal processing software dedicated to the analysis of complex signals displaying multiscale properties. It analyzes complex natural signals by use of nonlinear methods. It implements the multifractal formalism and allows various kinds of signal decomposition and reconstruction. One key aspect of the software lies in its ability to evaluate key concepts such as the degree of impredictability around a point in a signal, and provides different kinds of applications. The software can be used for times series or multidimensional signals.
-
Contact:
Hussein Yahia
-
Participants:
Antonio Turiel, Hussein Yahia
5.1.3 ProximalDenoising
-
Name:
ProximalDenoising
-
Keywords:
2D, Image filter, Filtering, Minimizing overall energy, Noise, Signal processing, Image reconstruction, Image processing
-
Scientific Description:
Image filtering is contemplated in the form of a sparse minimization problem in a non-convex setting. Given an input image I, one seeks to compute a denoised output image u such that u is close to I in the L2 norm. To do so, a minimization term is added which favors sparse gradients for output image u. Imposing sparse gradients lead to a non-convex minimization term: for instance a pseudo-norm Lp with 0 < p < 1 or a Cauchy or Welsh function. Half-quadratic algorithm is used by adding a new variable in the minimization functionnal which leads to two sub-problems, the first sub-problem is non-convex and solved by use of proximal operators. The second sub-problem can be written in variational form, and is best solved in Fourier space: it takes the form of a deconvolution operator whose kernel can be approximated by a finite sum of separable filters. This solution method produces excellent computation times even on big images.
-
Functional Description:
Use of proximal and non quadratic minimization. GPU implementation. If f is an input image, one seeks an output g such that the following functional is minimized:
l/2*(norme2(f-g) + psi(grad(g))) with : l positive constant, norme2 = L2 norm, psi is a Cauchy function used for parcimony.
This functional is also applied for debayerization.
-
Release Contributions:
This software implements H. Badri PhD thesis results.
- URL:
-
Authors:
Marie Martin, Chiheb Sakka, Hussein Yahia, Nicolas Brodu, Gabriel Zebadua Garcia, Khalid Daoudi
-
Contact:
Hussein Yahia
-
Partner:
Innovative Imaging Solutions I2S
5.1.4 Manzana
-
Name:
Manzana
-
Keywords:
2D, Image processing, Filtering
-
Scientific Description:
Software library developed in the framework of I2S-GEOSTAT innovationlab and made of high-level image processing functionalities based on sparsity and non-convex optimization.
-
Functional Description:
Library of software in image processing: filtering, hdr, inpainting etc.
-
Contact:
Hussein Yahia
-
Partner:
Innovative Imaging Solutions I2S
5.1.5 superres
-
Name:
Super-Resolution of multi-spectral and multi-resolution images
-
Keyword:
Multiscale
-
Scientific Description:
This resolution enhancement method is designed for multispectral and multiresolution images, such as these provided by the Sentinel-2 satellites (but not only). Starting from the highest resolution bands, band-dependent information (reflectance) is separated from information that is common to all bands (geometry of scene elements). This model is then applied to unmix low-resolution bands, preserving their reflectance, while propagating band-independent information to preserve the sub-pixel details.
-
Functional Description:
This super-resolution software for multi-spectral images consists of: - A core C++ library, which can be used directly - A Python module interface to this library - A Java JNI interface to the library - An end-user Python script for super-resolving Sentinel-2 images - An end-user plugin for the widely used SNAP software of the ESA.
- URL:
-
Contact:
Nicolas Brodu
-
Participant:
Nicolas Brodu
5.1.6 ProximalImageUtils
-
Name:
ProximalImageUtils
-
Keywords:
3D, Image analysis, Image processing, Signal processing, Minimizing overall energy
-
Scientific Description:
This library extends to other image processing tasks the convex/minimization approach used in the ProximalDenoising library. Please consult the BIL file of ProximalDenoising.
-
Functional Description:
Proximal algorithms in Image Processing for: - denoising, - inpainting, - reflection management, - enhancing depth maps in 3D vision - HDR (High Dynamic Range)
-
Release Contributions:
This software implements and extends H. Badri PhD thesis results.
- URL:
-
Authors:
Marie Martin, Chiheb Sakka, Hussein Yahia, Nicolas Brodu, Gabriel Zebadua Garcia, Khalid Daoudi
-
Contact:
Hussein Yahia
-
Partner:
Innovative Imaging Solutions I2S
5.1.7 ECG-PPG Denoising beat2bits
-
Name:
ECG noise reduction 6dB
-
Keywords:
Noise, Denoising, Edge aware smoothing, ECG
-
Functional Description:
The algorithm is a denoising module without post-processing that takes as input a single-channel ECG recording, and gives as output a beat interval signal.
-
Authors:
Guillaume Attuel, Hicham Badri
-
Contact:
Guillaume Attuel
5.2 New platforms
Participants: N. Brodu, K. Daoudi.
- Concaust
-
Web site:
team.inria.fr/comcausa/continuous-causal-states/.
-
Software Family:research
-
Audience:team
-
Evolution and maintenance:proto
-
Duration of the Development:2 years
-
Scientific description:This software implements the algorithm resulting from the Concaust Exploratory Action, published in 26. Despite considerable time being spent on its development, the code itself is not that large. Indeed, the difficulty lies in the theoretical and algorithmic work. Once we know what to compute and how the implementation follows, but there were many rounds of developement with different theoretical approaches. Some of these points are still not stabilized, which reflects in the API and the code performances. These are regularly tested and updated as the code is run against concrete data sets. For these reasons, the code is constantly evolving and is actively being developped within the team, but it not ready yet for diffusion to the community at large. The code is open source (MIT licence) and shared with close partners from the Comcausa Associate Team.
-
Software Family:
-
VocaPy
-
Software family:
vehicle
-
Audience:
community
-
Evolution and maintenance:
basic (still under development)
-
Duration of the Development:
1 year (still 1 year left)
-
Scientific description:
VocaPy is an interface and a Python library implementing pathological speech analysis algorithms and vocal biomarkers for one clinical applications at the moment, differential diagnosis of Parkinsonism, but it will evolve to include other clinical targets, particularly the monitoring of respiratory diseases. VocaPy works in synergy with the ADT VocaPnée-Infra dedicated to the development of an infrastructure to interact with protected health data.
6 New results
6.1 Description of turbulent dynamics in the interstellar medium: multifractal/microcanonical analysis: II. Sparse filtering of Herschel observation maps and visualization of filamentary structures at different length scales.
Participants: A. Rashidi, A. Yahia, S. Bontemps, N. Schneider, L. Bonne, P. Hennebelle, J. Scholtys, G. Attuel, I2S et al.
We introduce a sparse filtering/deconvolution approach of type l2-lp, with p variable and apply it to a large set of molecular clouds using Herschel 250 μm data in order to demonstrate their wide range of application. We thus highlight, in the Herschel data, extremely fine filamentary structures and distinguish between different types of turbulent properties in regions containing stellar cores. The results obtained on the Herschel data tend to show a significantly less log-normal behavior and a filamentary nature in the less dense regions, which, compared to the results obtained on MHD simulation outputs in which we control a magnetic field, leads us to discuss the more or less hydrodynamic character of the turbulence in the studied regions. We also use high-resolution adaptive magneto-hydrodynamic simulation data to assess the quality of de-beaming in such a simulated beaming framework.
Associated Publication in Astronomy and Astrophysics (under review)
6.2 Image Denoising in Fluorescence Microscopy using Feature based Gradient Reconstruction
Participants: S. K. Maji, H. Yahia.
Purpose The utility of fluorescence microscopy imaging comes with the challenge of low resolution acquisitions, which severely limits information extraction and quantitative analysis. Image denoising is a technique that aims to remove noise from microscopy acquisitions by taking into account prior statistics of the corrupting noise. In this work, we propose an image denoising technique for fluorescence microscopy imaging. Approach The proposed technique is based on the principle of multifractal feature extraction from a noisy sample followed by a reconstruction technique from these features. It is observed that by following a proper hierarchical classification procedure, meaningful features can be extracted from a noisy image. A denoised image is then estimated from this sparse feature set through proper formulation of an optimization problem. Results Experiments are performed on both synthetic image databases as well as on real fluorescence microscopy data. Superior denoising results, in comparison to multiple comparing techniques, validate the potential of the proposed approach. Conclusion The proposed method gives superior denoising results for low resolution fluorescence microscopy image acquisitions and can be used for post processing of data by biologists.
Associated Publication HAL
6.3 A Review on Despeckling of the Earth's Surface Visuals Captured by Synthetic Aperture Radar
Participants: A. Saha, S. K. Maji, H. Yahia.
Today, processing pictures of the Earth’s surface is frequently used for a variety of purposes. Given the increased need for this data, several sensors are presently being deployed for the accurate recording of remote sensing images. These sensors may be divided into two main categories: optical sensors and radar-based sensors. Radar-based sensors are widely used to acquire data for remote sensing because they have an advantage over optical sensors in that they can capture high-quality data even in the dark portions of the Earth’s surface.
Associated Publication HAL
6.4 Improved mapping of flood affected villages in India: a novel three-stage approach using PolSAR polarization signatures and ensembled dilated CNNs
Participants: G. S. Phartiyal, D. Singh, H. Yahia.
During floods, updated and accurate information on affected human settlements helps save lives and reduces time to rescue. Therefore, approaches that can provide reliable information during floods via the use of all-weather and real-time functional technology is highly needful. The study presented here attempts to efficiently and precisely map villages in the Indian sub-continent during floods via a three-stage approach which uses PolSAR data. However, an accurate segregation of villages in India even with PolSAR data is challenging because the built-up structures in the villages of rural India are closely placed and are randomly oriented w.r.t. each other. This condition either hinders their segregation or otherwise induces false alarms during extraction. More descriptive land cover characterization features and powerful feature classifiers may address this challenge. The study in this paper proposes a novel approach to efficiently detect and map flood affected villages which utilize polarization signatures from PolSAR imagery, ensemble-of -dilated-convolutions based CNNs, apriori knowledge and image morphology. The approach broadly involves three stages: first, built-up area extraction from a PolSAR image: second, detection of villages in a built-up area image and third, identification and mapping of villages that are affected by the flood. In the first stage, an ensemble of varying dilated-convolutions based novel CNN classifier which directly utilizes PolSAR-2 polarization signatures (PSs) in window-mode as features are developed to extract built-up areas. The second stage provides a novel village detection filter based on apriori knowledge and image morphology to detect actual villages and mask out the false objects. Finally, in the third stage, flood affected villages are mapped via a series of morphological operations based degree-of-intersection measure. Experiments are conducted on both simulated and natural flooded area datasets. Experimental results show 81% detection accuracy and 100% mapping performance of the proposed approach which indicates its potential as an effective flood affected village mapping system.
Associated Publication HAL
6.5 Synergistic exploitation of localized spectral-spatial and temporal information with DNNs for multisensor-multitemporal image-based crop classification
Participants: G. S. Phartiyal, D. Singh, H. Yahia.
The challenge of performing efficient and reliable crop classification with multisensor multitemporal (MSMT) images in mixed land cover scenarios i.e. presence of small land parcels (area < 20,000-meter square) of crops and other land covers such as built-up or grasslands, is significant. Specially in countries (ex. India) where diverse crops are practiced in small land parcels. This challenge can be addressed if deep neural network (DNN) based models can exploit all three i.e. spatial, spectral, and temporal information of a crop, present in the MSMT images, efficiently and effectively. Therefore, this study presents a novel DNN based model that exploits all three information in a synergistic fashion to achieve improved crop classification. At first, the model increases the significance of local spectral information via a strategy that creates versions of spectral band set wherein neighbourhood of spectral bands is permuted. Then, the model utilizes three-dimensional convolutions, in a time-distributed fashion, to extract local spectral-spatial features. Finally, the model utilizes bidirectional long short-term memory or LSTM-RNNs to extract the temporal information embedded in the time-distributed feature-space created after the convolutions. The developed model is trained and evaluated on Sentinel-1 and Sentinel-2 MSMT data to achieve a 6-class classification including two major crops grown in the region. One of the proposed models namely Perm-3D-CRNN-v1 showed a 97.77% overall accuracy on test samples and reflected satisfactory on quantitative analysis. The localized spectral-spatial convolutions created prominent class-specific features whereas the bidirectional information flow in the recurrent layer improved the exploitation of crop-phenology type features making the model perform efficiently.
Associated Publication HAL
6.6 Analysis of speech glottal flow for early differential diagnosis between Parkinson’s disease and multiple systems atrophy
Participants: K. Daoudi, F. Yahaya, S. Milhé de Saint Victor, A. Foubert Samier, M. Fabbri, A. Pavy-Le Traon, O. Rascol, V. Woisard, W. G. Meissner.
Speech disorder represents an early and prominent clinical feature of atypical parkinsonian syndromes such as multiple system atrophy (MSA). Phonation has been the most widely studied subsystem of Parkinsonian speech production. The latter have been essentially analyzed by perturbation measures or/and cepstral measures. Whilst these measures have become baseline features, they suffer from some limitations. In particular, their computation is based on the analysis of the time/frequency representation of the recorded signal which is formed by a contribution of all the production subsystems. Therefore, the measurements are not guarantied to reflect the only performance of the phonation subsystem. The study of the glottal flow, which is the airflow excitation signal generated by the vocal folds, represents thus an appealing alternative in order to restrict the analysis to the pure phonatory component generated by the vocal folds. The glottal flow can be estimated from the speech signal using glottal source inverse filtering. We studied the utility of glottal flow features as a discriminative cue between PD and MSA-P, the parkinsonian variant of MSA. We used the traditional sustained phonation task and introduced a novel analysis using diadochokinetic (DDK) speech tasks. We found that early PD speech does not exhibit glottal flow impairment in sustained phonation and in DDK tasks. On the contrary, we found that early MSA-P speech manifest glottal flow impairment in DDK tasks, but not in sustained phonation. This indicated, first, that the latter is not a suitable task to reflect potential impairment in the glottal flow production of Parkinsonian speech. More importantly, this indicated that DDK is a more suited task for the evaluation of glottal flow performance and can be a useful cue to discriminate between PD and MSA-P, at early disease stage.
Associated Preprint: Biomedical Signal Processing and Control (Elsevier), 2024.
6.7 Reduced-rank spectral mixtures Gaussian processes for probabilistic time-frequency representations
Participants: A. Fradi, K. Daoudi.
Deterministic time-frequency representations are commonly used in signal processing, particularly in audio processing. Whilst presenting many potential advantages, their probabilistic counterparts are not widely used, essentially because of the computational load and the lack of clear interpretability of the different underlying models. However, using state space models, they have been shown recently to be equivalent to Spectral Mixtures Gaussian processes (SM-GP). This pioneer work unlocks this problem and opens the path for the development of tractable and interpretable probabilistic time-frequency analysis. We proposed a relatively simple yet a significant improvement of that work in terms of computational complexity, flexibility and practical application. To do so, we used a recent approach for covariance approximation to develop an algorithm for faster inference of SM-GP, while opting for a frequency-domain approach to hyperparameter learning. We illustrated the practical potential of our method using voiced speech data. We first showed that key speech features can be accurately learned from the data. Second, we showed that our method can yield better performances in denoising.
Associated Publication HAL
6.8 Whales in Space: Experiencing Aquatic Animals in Their Natural Place with the Hydroambiphone
Participants: J. P. Crutchfield, D. D. Dunn, A. Jurgens.
Recording the undersea three-dimensional bioacoustic sound field in real-time promises major benefits to marine behavior studies. We describe a novel hydrophone array – the hydroambiphone (HAP) – that adapts ambisonic spatial-audio theory to sound propagation in ocean waters to realize many of these benefits through spatial localization and acoustic immersion. Deploying it to monitor the humpback whales (Megaptera novaeangliae) of southeast Alaska demonstrates that HAP recording provides a qualitatively-improved experience of their undersea behaviors; revealing, for example, new aspects of social coordination during bubble-net feeding. On the practical side, spatialized hydrophone recording greatly reduces post-field analytical and computational challenges – such as the "cocktail party problem" of distinguishing single sources in a complicated and crowded auditory environment – that are common to field recordings. On the scientific side, comparing the HAP's capabilities to single-hydrophone and nonspatialized recordings yields new insights into the spatial information that allows animals to thrive in complex acoustic environments. Spatialized bioacoustics markedly improves access to the humpbacks' undersea acoustic environment and expands our appreciation of their rich vocal lives.
Associated Preprint arXiv
6.9 Twainspotting: Identity Revealed During a Simple, but Extended Conversation with a Humpback Whale
Participants: J. P. Crutchfield, A. Jurgens.
Extended acoustic interactions with a humpback whale (Megaptera novaeangliae) were captured via human-initiated playbacks of the purported humpback “throp” social call and hydrophone recordings of the animal’s vocalized responses during August 2021 in Frederick Sound, Southeast Alaska. Multivariate statistical analyses performed after the event, that adapted the Wasserstein metric to spectrograms, strongly imply that the played back call was from the same animal, which had been recorded the previous day and initially selected for its clarity. This suggests these interactive playback studies may have formed a probe of time-delayed self-recognition. Fluke photographs taken that previous day and during the interactions revealed that the animal had been identified 38 years earlier, now known as the female humpback named Twain 14 years ago. This exciting and to our knowledge unique series of interactive vocalization events, while hopeful, poses more questions than answers. Most basically, did the half-hour long series of acoustic exchanges constitute an interspecies conversation? We argue that analysis tools available to infer the implied causality of interaction—statistical dependency, multivariate information theory, and machine learning—leave the question open. That said, and perhaps more importantly, the extended interaction broaches questions whose answers bear directly on future interspecies communication and, more generally, the human appreciation of nonhuman intelligence. The reported human-humpback interactions will facilitate exploring these issues at new depths.
Associated Preprint bioRxiv
6.10 Whale Casting: Remote mobile streaming humpback whale vocalizations to the world
Participants: J. P. Crutchfield, A. Jurgens.
Over several days in early August 2021, while at sea in Chatham Strait, Southeast Alaska, aboard M/Y Blue Pearl, an online this http URL stream broadcast in real-time humpback whale vocalizations monitored via hydrophone. Dozens on mainland North American and around the planet listened in and chatted via the stream. The webcasts demonstrated a proof-of-concept: only relatively inexpensive commercial-off-the-shelf equipment is required for remote mobile streaming at sea. These notes document what was required and make recommendations for higher-quality and larger-scale deployments. One conclusion is that real-time, automated audio documenting whale acoustic behavior is readily accessible and, using the cloud, it can be directly integrated into behavioral databases – information sources that now often focus exclusively on nonreal-time visual-sighting narrative reports and photography.
Associated Preprint arXiv
6.11 A Whale With A History: Sighting Twain The Humpback Over Three Decades
Participants: J. P. Crutchfield, A. Jurgens.
Extended acoustic interactions with a humpback whale (Megaptera Novaeangliae) were captured via playbacks of the purported “whup/throp” social call and hydrophone recordings of the animal’s vocalized responses during August 2021 in Frederick Sound, Southeast Alaska. Fluke photographs identified the animal as a female named Twain (HappyWhale.com identity SEAK-0401) first observed some 35 years ago. We document Twain’s life history via sightings made over three decades, reporting as much as is known (and allowed for public distribution) about Twain. The observational history gives illuminating snapshots of the long history of the individual behind the acoustic interactions.
Associated Preprint bioRxiv
6.12 6-Years of SAR (Sentinel-1) and optical (Sentinel 2, Landsat-8) acquisitions over agricultural surfaces in southwestern, France
Participants: F. Baup, R. Fieuzal, B. Ygorra, A. A. Gorrab, S. Riazanoff, A. Martin-Comte, K. Gross, F. Frappart.
Agricultural surfaces monitoring from space has been recently developed. Since 2016, Sentinel-1 and Sentinel-2 satellites, carrying a synthetic aperture radar (SAR) and an optical radiometer, respectively, monitor Europe with fine spatial and temporal resolutions. Earth Observation (EO) data provide valuable information for maintaining a sustainable development of agronomic, economic and environment. It helps in the determination of the different vegetation type and state in the agricultural practices. Vegetation Indexes were found as better descriptors of the vegetation biophysics: the most commonly used is the Normalized Differenced Vegetation Index (NDVI). Several studies used SAR backscatter intensities for the estimation of crop biophysical parameters. With 5 years of continuous monitoring, the Auradé and Lamasquère experimental fields provide a long time-series of vegetation and soil biophysical parameters. With the help of remotely sensed data, a large range of climatic conditions can be covered and the dynamics of the vegetation index derived from optical sensors and backscatter intensities from SAR can be thoroughly analysed. This work focused on the effect of climatic conditions on optical, SAR signals and on the vegetation dynamics of several crop types. Differences in the crop development and growth duration were found according to yearly climatic conditions. With this long time-series analysis, results were obtained for multiple climatic conditions, including the hottest year in France. Some crops showed optical signal/backscatter intensity anomalies this year.
Article in review for the IGARSS Symposium
6.13 CuSum-NRT as a crop monitoring system: analysing 3 years of Sentinel-1 data over fields in southwestern France
Participants: B. Ygorra, F. Baup, R. Fieuzal, A. A. Gorrab, S. Riazanoff, A. Martin-Comte, K. Gross, F. Frappart, J-P. Wigneron.
Since 2016, land surfaces can be monitored from optical and synthetic aperture radar sensors onboard Sentinel-1 and Sentinel-2 satellites at high spatial and temporal resolutions. Monitoring agricultural surfaces through satellite-based estimations of biophysical parameters is a key issue for agriculture sustainability in the context of an increasing climate change. With its all-weather vision capability, it is important to develop methods based on the use of Sentinel-1 images. In order to maintain a sustainable development of environment, economic and agronomic, spatial information about agricultural practices is needed. Earth Observation (EO) data acquired through remote sensing satellite imagery has been proven important in providing and understanding the different vegetation type and state in the agricultural practices. Data acquired by Synthetic Aperture Radar (SAR) sensors provide images unaffected by clouds, compared to data acquired by optical sensors. Radar measurements are affected by soil roughness, soil moisture and vegetation due to their dielectric and structural characteristics. A common exploited method to simplify the use of remotely sensed data is the use of Vegetations Indices (VI) interpreted as descriptors of vegetation cover. In the optical domain, the most commonly used indices are NDVI, MTVI2, NDRE, etc., whereas in the active microwave domain, RVI or the cross-polarization ratio (VV/VH in the case of Sentinel-1) are more often used. The Cumulative Sum (CuSum), initially developed for financial purpose method has been recently applied to EO data to monitor forests or natural wetlands and its ability to monitor vegetation changes near-real-time is being studied. We attempted to monitor the phenotypical state of agricultural fields in the South-West of France based on the application of CuSum-NRT to Sentinel-1 SAR data. We found that the detections by the CuSum can be related to the different components of surface (soil and vegetation states). The detections by the CuSum are also influenced by the climatic variable according to the type of crop, as shown by the sorghum crop in years 2018 / 2020.
Article in review for the IGARSS Symposium
6.14 A near-real-time tropical deforestation monitoring algorithm based on CuSum change detection method
Participants: B. Ygorra, F. Frappart, J-P. Wigneron, T. Catry, B. Pillot, G. Demarchin, J. Subervie, A. Pfefer, J. Courtalon, S. Riazanoff.
Tropical forests are currently under pressure from increasing threats. These threats are related mainly to human activities. Earth observations are increasingly used for monitoring forest, especially synthetic aperture radar (SAR), that is less affected than optics by atmospheric conditions. Since the launch of the Sentinel-1 constellation, numerous methods for forest disturbance monitoring have been developed, including near real-time (NRT) operational algorithms providing early warnings such as Radar for Detecting Deforestation (RADD), Global Land Analysis and Discovery (GLAD), Real Time Deforestation Detection System (DETER), and Jica-Jaxa Forest Early Warning System (JJ-FAST). These algorithms provide online disturbance maps and are applied at continental / global scale with a Minimum Mapping Unit (MMU) ranging from 0.1 ha to 6.25 ha. For local operators, these algorithms are hard to customized to meet users’ specific needs. Recently, the Cumulative sum change detection (CuSum) method have been developed for the monitoring of forest disturbances on long time series of Sentinel-1 images. We developed an NRT version of CuSum with spatial recombination (cross-Tc) with a MMU of 0.03 ha and compared it to the Recursive CuSum called ReCuSum. This was done in an area validated through visual interpretation of changes using optical two-epoch images. The values of the different parameter were determined to optimize the detection of changes using F1-score. In the best configuration, 68% precision, 72% recall, 93% accuracy and 0.71 F1-score were obtained.
Article in review for the International Journal of Applied Earth Observation and Geoinformation
6.15 Sentinel-1 Based Cusum Capabilities As A Forest / Non-Forest Mask In Tropical Areas
Participants: B. Ygorra, F. Frappart, J-P. Wigneron, T. Catry, B. Pillot, S. Riazanoff.
Tropical forests are vulnerable to deforestation. This phenomenon led to the development of a wide number of forest monitoring systems based on remotely sensed data. The number of approaches for forests monitoring using various satellite images has steadily been increasing. The resolution Forest/Non-Forest (FNF) masks has gained in spatial resolution with the launch of new generations of sensors. These masks can be used for multiple applications including forest and land cover monitoring forest carbon fluxes estimation. In recent deforestation monitoring studies, FNF masks were used as a basis to reduce the number of false positives in non-forest areas. As the radar signal is sensitive to soil roughness and to soil moisture, using a good FNF mask allows to reduce the false positives due to changes in non-forest areas. The Cumulative Sum (CuSum) algorithm is a change point detection method used to analyse timeseries. It was initially developed for financial studies and later applied to radar data to monitor temperate and tropical forests disturbances. The method developed in this study is based on a modified version CuSum we developed earlier applied to a dense Sentinel-1 C-SAR image timeseries ranging from 01/01/2018 to 01/01/2020 in the Parà State, Brazil. The modified CuSum is based on the Cross-Tc spatial recombination method with a high threshold of 100 and a low threshold of 80. This method allows for the detection of any structural change in the time series, overlooking most seasonal variability. It is sensitive to changes in soil moisture and roughness. We used this method as a mean to determine all the changes in radar backscatter which occur over non forest areas. Pixels showing a change were considered as NF at the end of the timeseries. In this study, we used Global Forest Watch data to create a FNF mask as reference data. The comparison of the CuSum results with the FNF mask from GFW (Figure 4), showed that most NF areas are detected by the CuSum. The confusion matrix statistics exhibit a high recall value (78.0%), which corresponds to the percentage of area correctly detected as NF on the total NF area. There are few False Positives, as the precision is also high (79.0%). These two statistics lead to a high F1-score (0.785).
Associated publication: HAL
6.16 CONCAUST Exploratory Action
Participants: N. Brodu, J. P. Crutchfield, L. Bourel, M. Sáez-Andreu, S. Mangiarotti.
Concaust Exploratory Action Web page. The budget was eallocated into 2 years of post-doctorate positions: - 1 year for working on the core methodology - 1 year for researching the applicability of the method on the El Niño / La Niña south pacific oscillations (ENSO phenomenon). Due to covid constraints, the postdoctorate candidate (Alexandra Jurgens) that was selected for the core methodology could not come to France in fall 2021. She finally came in February 2023 and has been very productive. The second year of post-doctorate research was offered to Marina Sáez-Andreau, who worked with Luc Bourrel and Sylvain Mangiarotti at IRD. While she was with us, she first worked on a method developped by these partners that she was familiar with, and planned to apply Concaust for comparison later. Meanwhile, Marina has found an academic position and quitted the team. We plan to pursue that work with another PhD or post-doctorate condidate on complementary funding, such as PEPR.
6.17 COMCAUSA Associated team
Participants: N. Brodu, J. P. Crutchfield, et al. (see Website).
Web page The associate team Comcausa was created as part of the Inria@SiliconValley international lab, between Inria Geostat and the Complexity Sciences Center at University of California, Davis. This team is managed by Nicolas Brodu (Inria) and Jim Crutchfield (UC Davis) and the full list of collaborators is given on the web site. Alexandra Jurgens, formerly at UC Davis, has now joined Inria as a post-doctoral researcher. This is the team that became progressively the core of Nicolas Brodu activities, which is planned to lead to the creation of a new Inria project-team of the same name.
6.18 Speech acoustic indices for differential diagnosis between Parkinson's disease, multiple system atrophy and progressive supranuclear palsy
Participants: K. Daoudi, B. Das, T. Tykalova, J. Klempir, J. Rusz.
While speech disorder represents an early and prominent clinical feature of atypical parkinsonian syndromes such as multiple system atrophy (MSA) and progressive supranuclear palsy (PSP), little is known about the sensitivity of speech assessment as a potential diagnostic tool. Speech samples were acquired from 215 subjects, including 25 MSA, 20 PSP, 20 Parkinson's disease participants, and 150 healthy controls. The accurate differential diagnosis of dysarthria subtypes was based on the quantitative acoustic analysis of 26 speech dimensions related to phonation, articulation, prosody, and timing. A semi-supervised weighting-based approach was then applied to find the best feature combinations for separation between PSP and MSA. Dysarthria was perceptible in all PSP and MSA patients and consisted of a combination of hypokinetic, spastic, and ataxic components. Speech features related to respiratory dysfunction, imprecise consonants, monopitch, slow speaking rate, and subharmonics contributed to worse performance in PSP than MSA, whereas phonatory instability, timing abnormalities, and articulatory decay were more distinctive for MSA compared to PSP. The combination of distinct speech patterns via objective acoustic evaluation was able to discriminate between PSP and MSA with very high accuracy of up to 89% as well as between PSP/MSA and PD with up to 87%. Dysarthria severity in MSA/PSP was related to overall disease severity. Speech disorders reflect the differing underlying pathophysiology of tauopathy in PSP and α-synucleinopathy in MSA. Vocal assessment may provide a low-cost alternative screening method to existing subjective clinical assessment and imaging diagnostic approaches.
Publication: HAL), Nature npj, parkinson's disease).
6.19 A comparative study on vowel articulation in Parkinson's disease and multiple system atrophy
Participants: K. Daoudi, B. Das, S. Milhé de Saint Victor, A. Foubert Samier, M. Fabbri, A. Pavy-Le Traon, O. Rascol, V. Woisard, W. G. Meissner.
Acoustic realisation of the working vowel space has been widely studied in Parkinson's disease (PD). However, it has never been studied in atypical parkinsonian disorders (APD). The latter are neurodegenerative diseases which share similar clinical features with PD, rendering the differential diagnosis very challenging in early disease stages. This paper presents the first contribution in vowel space analysis in APD, by comparing corner vowel realisation in PD and the parkinsonian variant of Multiple System Atrophy (MSA-P). Our study has the particularity of focusing exclusively on early stage PD and MSA-P patients, as our main purpose was early differential diagnosis between these two diseases. We analysed the corner vowels, extracted from a spoken sentence, using traditional vowel space metrics. We found no statistical difference between the PD group and healthy controls (HC) while MSA-P exhibited significant differences with the PD and HC groups. We also found that some metrics conveyed complementary discriminative information. Consequently, we argue that restriction in the acoustic realisation of corner vowels cannot be a viable early marker of PD, as hypothesised by some studies, but it might be a candidate as an early hypokinetic marker of MSA-P (when the clinical target is discrimination between PD and MSA-P).
Publication: HAL), Interspeech 2022).
6.20 Advanced super-resolution
Participants: A. Rashidi, H. Yahia, A. Cherif [I2S], J. L. Vallancogne [I2S], A. Cailly [I2S].
In the framework of the acquisition chain and devices built by i2S, the objective of this PhD is to provide efficient algorithms able to merge different acquired images corresponding to slight spatial translational displacements to get a wider super-resolved image. Consequently, this PhD takes place within the general subject of super-resolution. In this thesis, super-resolution is performed using different acquisitions. We propose the use of sensor displacement within the cameras of I2S. We propose a scheme to achieve an image with up to two times higher resolution using this technique. Furthermore, we also propose an additional image deconvolution algorithm that helps to improve the image quality further and to address any degradation problem that may occur through the super-resolution scheme. Our image deconvolution algorithm is based on variable splitting and takes advantage of the proximal operator and Fourier transform. We also proposed the use of new potential functions that can be used as prior information in image inverse problems for the first time used in image processing. Experimental results show promising capabilities of the proposed algorithm. The algorithm is successfully implemented within various cameras and devices of I2S. The practical experiments on real-world data prove the effectiveness and flexibility of our image-deconvolution. Experiments were conducted on Herschel observation maps, and promising results were obtained on such imaging data. In the last part of the thesis, the idea of plug-and-play priors for image denoising and deconvolution is presented. This thesis proposes the implementation of plug-and play-priors in an alternating minimisation scheme. The early result has shown potential to be adequate for image denoising/deconvolution application.
Publication: A. Rashidi PhD's thesis, HAL.
7 Partnerships and cooperations
7.1 International initiatives
7.1.1 Associate Teams in the framework of an Inria International Lab or in the framework of an Inria International Program
COMCAUSA
-
Title:
Computation of causal structures
-
Duration:
2021 ->
-
Coordinator:
James Crutchfield (chaos@cse.ucdavis.edu)
-
Partners:
- University of California Davis (États-Unis)
-
Inria contact:
Nicolas Brodu
-
Summary:
This project introduces new methods for the analysis of complex systems, based on observations. Classical signal analysis is mostly built around frequency decomposition, correlations and power spectrum analysis. This tells the scales and locations at which energy is dissipated. We aim to go beyond this, by also characterizing how energy is dissipated. Thanks to computational mechanics, we can identify whether patterns are created, at which scale, and how they interact. Our goal is to create practical algorithms that would inform on the hidden dynamics in scientific data sets, providing data interpretability, a feature often missing from concurrent machine learning and signal analysis models.
7.2 International research visitors
7.2.1 Visits of international scientists
Mikhael Semaan from University of Utah (USA) came in October 2023 to visit INRIA and discuss the applications of the continuous-causal state research to ecology, as well as further discussion on the physics of information and non-equilibrium steady states.
Yao Liu from Northumbria University (Newcastle, UK) came in February 2023 and in October 2023 to visit INRIA and discuss modeling methods for memory effects in plants and the dynamics of ecosystems.
7.2.2 Visits to international teams
Nicolas Brodu stayed at Northumbria University (Newcastle, UK) in August 2023 to visit Yao Liu and discuss modeling methods for memory effects in plants and the dynamics of ecosystems.
Alexandra Jurgens visited Trinity College in June 2023 to discuss with colleagues Alec Boyd and Felix Binder on the thermodynamics of information processing
Alexandra Jurgens visited University of California, Davis in December 2023 to work with colleague James P. Crutchfield on Computational Mechanics and application to bioacoustics.
7.3 National initiatives
-
PEPR:
with AgroParisTech. Title: Modeling the global states and dynamics of ecosystems - Applications to carbon flux and stock in ecosystems modified by agricultural activities. This project is supporting research on causality presently in Geostat and in the post-Geostat being currently set up by N. Brodu. PIs: N. Brodu, E. Vaudour. Duration: 60 months. Funding: 1,5 M euros.
- CONCAUST Exploratory Action
The exploratory action « TRACME » was renamed « CONCAUST » and is going on with good progress. Collaboration with J. P. Crutchfield and its laboratory has lead to a first article, “Discovering Causal Structure with Reproducing-Kernel Hilbert Space
-Machines”, available here. That article poses the main theoretical fundations for building a new class of models, able to reconstruct a measured process « causal states » from data. - CovidVoice project: Inria Coind-19 mission. The CovidVoice project evolved into the VocaPnée project in partnership with AP-HP and co-directed by K. Daoudi and Thomas Similowski, responsible for the pulmonology and resuscitation service at La Pitié-Salpêtrière hospital and UMR-S 1158. The objective of the VocaPnée project is to bring together all the skills available at Inria to develop and validate a vocal biomarker for the remote monitoring of patients at home suffering from an acute respiratory disease (such as Covid) or chronic (such as asthma) . This biomarker will then be integrated into a telemedicine platform, ORTIF or COVIDOM for Covid, to assist the doctors in assessing the patient's respiratory status. VocaPnée is divided into 2 longitudinal pilot clinical studies, a hospital study and another in tele-medicine.
- Vocapy. The ADT (IA Plan) project VocaPy, led by K. Daoudi, started in November 2021 for a 2 years duration. The goal of VocaPy is to develop a Python library dedicated to pathological speech analysis and vocal biomarkers conception. In case of success, our R&D on vocal biomarkers would obviously have a non-negligible impact at the clinical, scientific, technological and socio-economical levels. VocaPy and VocaPnée-Infra will serve a kernel for the development of disease-specific IA solutions to achieve the clinical targets and for their technological transfer to the clinical (and/or future industrial) partners.
- ANR project Voice4PD-MSA, led by K. Daoudi, which targets the differential diagnosis between Parkinson's disease and Multiple System Atrophy. The total amount of the grant is 468555 euros, from which GeoStat has 203078 euros. Initial duration of the project was 42 months, and has been extended until January 2023. Partners: CHU Bordeaux (Bordeaux), CHU Toulouse, IRIT, IMT (Toulouse).
- GEOSTAT is a member of ISIS (Information, Image & Vision), AMF (Multifractal Analysis) GDRs.
- GEOSTAT is participating in the CNRS IMECO project Intermittence multi-échelles de champs océaniques : analyse comparative d’images satellitaires et de sorties de modèles numériques. CNRS call AO INSU 2018. PI: F. Schmitt, DR CNRS, UMR LOG 8187. Duration: 2 years.
8 Dissemination
Participants: K. Daoudi, N. Brodu, A. Jurgens, B. Ygorra.
Reviewer - reviewing activities
K. Daoudi is a regular reviewer of IEEE/ACM Transactions on Audio, Speech, and Language Processing, and the ICASSP and Interspeech conferences. He also reviewed in 2023 for Nature’ npj-Parkinson’s Disease.
B. Ygorra is a regular reviewer for the IGARSS conference and for the International Journal of Applied Earth Observation and Geoinformation.
Invited talks
K. Daoudi gave a talk at university of Massachusetts in June 2023 entitled “Speech-based differential diagnosis in Parkinsonism”.
A. Jurgens was invited speaker at the “Information Theory as a Bridge Across the Geosciences and Modeling Sciences” workshop at Umweltforschungsstation Schneefernerhaus (Septembre 2023), where she presented “Finite and Infinite Models: Optimal Prediction of Hidden Markov Processes”.
A. Jurgens was invited speaker at the “Sensory Prediction: Engineered and Evolved” workshop at Santa Fe Institute (July 2023), where she presented “Finite and Infinite Models: Optimal Prediction of Hidden Markov Processes”.
A. Jurgens was invited speaker at the “Decomposing Multivariate Information in Complex Systems” workshop (June 2023) at Max Planck Institute for the Physics of Complex Systems, where she presented “Divergent Memory: Optimal Prediction of Hidden Markov Processes”.
N. Brodu was invited speaker at the "Journées scientifiques Inria Chili" (December 2023), where he gave the talk "Inferring effective state variables and dynamics from data"
N. Brodu was invited speaker at the "Brest Workshop on Environmental Physics and Signal Processing" (June 2023), where he gave the talk "Inferring effective state variables and dynamics from data".
N. Brodu was invited speaker at the “Sensory Prediction: Engineered and Evolved” workshop at Santa Fe Institute (July 2023), where he presented “CompMech on real data: using kernel ε-machines to infer process dynamics”.
Supervision
K. Daoudi co-supervised the Master thesis of Younes El Belghiti, university of Rabat, Morocco. Subject: Inspiration detection from speech using deep learning
Juries
H. Yahia is a member of the PhD thesis of A. Saini (as reviewer), IIT Roorkee PhD title: Development of computer vision approach for railroad track health/assets monitoring with drone data To be defended in 2024.
Popularization
A. Jurgens was an invited guest for the podcast of "Physics Frontiers" to discuss her paper "Functional thermodynamics of Maxwellian ratchets: Constructing and deconstructing patterns, randomizing and derandomizing behaviors and the field of stochastic thermodynamics and physics of information".
9 Scientific production
9.1 Major publications
- 1 articleMultifractal Desynchronization of the Cardiac Excitable Cell Network During Atrial Fibrillation. II. Modeling.Frontiers in Physiology10April 2019, 480 (1-18)HALDOI
- 2 articleMultifractal desynchronization of the cardiac excitable cell network during atrial fibrillation. I. Multifractal analysis of clinical data.Frontiers in Physiology8March 2018, 1-30HALDOI
- 3 articleA Non-Local Low-Rank Approach to Enforce Integrability.IEEE Transactions on Image ProcessingJune 2016, 10HAL
- 4 inproceedingsFast and Accurate Texture Recognition with Multilayer Convolution and Multifractal Analysis.European Conference on Computer VisionECCV 2014Zürich, SwitzerlandSeptember 2014HAL
- 5 articleSpeech acoustic indices for differential diagnosis between Parkinson's disease, multiple system atrophy and progressive supranuclear palsy.npj Parkinson's DiseaseOctober 2022HALDOI
- 6 articlePhysical and Biological Satellite Observations of the Northwest African Upwelling: Spatial Extent and Dynamics.IEEE Transactions on Geoscience and Remote SensingOctober 2019, 1-13HALDOI
- 7 articleIncreasing the Resolution of Ocean pCO₂ Maps in the South Eastern Atlantic Ocean Merging Multifractal Satellite-Derived Ocean Variables.IEEE Transactions on Geoscience and Remote SensingJune 2018, 1 - 15HALDOI
- 8 articleReconstruction of super-resolution ocean pCO 2 and air-sea fluxes of CO 2 from satellite imagery in the Southeastern Atlantic.BiogeosciencesSeptember 2015, 20HAL
- 9 articleAn efficient solution to sparse linear prediction analysis of speech.EURASIP Journal on Audio, Speech, and Music Processing201312013, 3HAL
- 10 articleDetection of Glottal Closure Instants based on the Microcanonical Multiscale Formalism.IEEE Transactions on Audio, Speech and Language ProcessingDecember 2014HAL
- 11 articleEdges, Transitions and Criticality.Pattern RecognitionJanuary 2014, URL: http://hal.inria.fr/hal-00924137
- 12 articleA Multifractal-based Wavefront Phase Estimation Technique for Ground-based Astronomical Observations.IEEE Transactions on Geoscience and Remote SensingNovember 2015, 11HALDOI
- 13 articleSingularity analysis in digital signals through the evaluation of their Unpredictable Point Manifold.International Journal of Computer Mathematics2012, URL: http://hal.inria.fr/hal-00688715
- 14 articleOcean Turbulent Dynamics at Superresolution From Optimal Multiresolution Analysis and Multiplicative Cascade.IEEE Transactions on Geoscience and Remote Sensing5311June 2015, 12HALDOIback to text
- 15 articleMicrocanonical multifractal formalism: a geometrical approach to multifractal systems. Part I: singularity analysis.Journal of Physics A: Math. Theor412008, URL: http://dx.doi.org/10.1088/1751-8113/41/1/015501back to text
- 16 articleMotion analysis in oceanographic satellite images using multiscale methods and the energy cascade.Pattern Recognition43102010, 3591-3604URL: http://dx.doi.org/10.1016/j.patcog.2010.04.011back to text
9.2 Publications of the year
International journals
- 17 articleReduced-rank Spectral Mixtures Gaussian Processes for Probabilistic Time-frequency Representations.Signal ProcessingDecember 2023HALDOI
- 18 articleImage denoising in fluorescence microscopy using feature based gradient reconstruction.Journal of Medical Imaging1006December 2023HALDOI
- 19 articleImproved mapping of flood affected villages in India: a novel three-stage approach using PolSAR polarization signatures and ensembled dilated CNNs.International Journal of Remote SensingNovember 2023, 1-26HALDOI
- 20 articleSynergistic exploitation of localized spectral-spatial and temporal information with DNNs for multisensor-multitemporal image-based crop classification.International Journal of Applied Earth Observation and Geoinformation125December 2023, 103595HALDOI
Scientific book chapters
- 21 inbook3D Volumetric Computed Tomography from 2D X-RaysA Deep Learning Perspective.Handbook of AI-Based Models in Healthcare and Medicine1CRC PressDecember 2023, 23HALDOI
- 22 inbookA Review on Despeckling of the Earth's Surface Visuals Captured by Synthetic Aperture Radar.Multimedia Data Processing and ComputingMultimedia Data Processing and Computing1CRC PressSeptember 2023, 196HALDOI
9.3 Cited publications
- 23 bookOndelettes, multifractales et turbulence.Paris, FranceDiderot Editeur1995back to text
- 24 bookModeling Complex Systems.New-York Dordrecht Heidelberg LondonSpringer2010back to text
- 25 articlePredictability: a way to characterize complexity.Physics Report356arXiv:nlin/0101029v12002, 367--474URL: http://dx.doi.org/10.1016/S0370-1573(01)00025-4back to text
- 26 articleDiscovering causal structure with reproducing-kernel Hilbert space ε-machines.Chaos: An Interdisciplinary Journal of Nonlinear Science3222022back to text
- 27 articleRECONSTRUCTION OF EPSILON-MACHINES IN PREDICTIVE FRAMEWORKS AND DECISIONAL STATES.Advances in Complex Systems14052011, 761-794URL: https://doi.org/10.1142/S0219525911003347DOIback to text
- 28 articleModern Koopman Theory for Dynamical Systems.SIAM Review6422022, 229-340URL: https://doi.org/10.1137/21M1401243DOIback to text
- 29 bookChaos and Coarse Graining in Statistical Mechanics.Cambridge University Press2008DOIback to text
- 30 articleBetween order and chaos.Nature Physics82012, 17–24back to textback to text
- 31 articleEquations of Motion from a Data Series.Complex Syst.11987back to text
- 32 articleInferring statistical complexity.Phys. Rev. Lett.632Jul 1989, 105--108URL: https://link.aps.org/,doi/10.1103/PhysRevLett.63.105DOIback to textback to text
- 33 bookMotor Speech Disorders Substrates, Differential Diagnosis, and Management.Elsevier2013back to text
- 34 articleVoice for Health: The Use of Vocal Biomarkers from Research to Clinical Practice.Digit. Biomark512021DOIback to text
- 35 articleAbout the role of chaos and coarse graining in statistical mechanics.Physica A: Statistical Mechanics and its Applications418Proceedings of the 13th International Summer School on Fundamental Problems in Statistical Physics2015, 94-104URL: https://www.sciencedirect.com/science/article/pii/S0378437114004038DOIback to text
- 36 articleMeasures of statistical complexity: Why?Physics Letters A23841998, 244-252URL: https://www.sciencedirect.com/science/article/pii/S0375960197008554DOIback to text
- 37 articleApproaching complexity by stochastic methods: From biological systems to turbulence.Physics Reports50652011, 87-162URL: https://www.sciencedirect.com/science/article/pii/S0370157311001530DOIback to text
- 38 articleMixed LICORS: A Nonparametric Algorithm for Predictive State Reconstruction.2012, URL: https://arxiv.org/abs/1211.3760DOIback to text
- 39 articleMultifield visualization using local statistical complexity.IEEE Transactions on Visualization and Computer Graphics1362007, 1384-1391DOIback to text
- 40 book Statistical physics, statics, dynamics & renormalization.World Scie,tific2000back to text
- 41 articleMultiscale entropy analysis of complex physiologic time series.Phys Rev Lett.89(6)2002DOIback to text
- 42 article Can the original equations of a dynamical system be retrieved from observational time series? Chaos 29 2019 DOI back to text
- 43 articleKernel learning for robust dynamic mode decomposition: linear and nonlinear disambiguation optimization.Proc. R. Soc.A.47820210830202108302022DOIback to text
- 44 articleGeneralized statistical complexity measures: Geometrical and analytical properties.Physica A: Statistical Mechanics and its Applications36922006, 439-462URL: https://www.sciencedirect.com/science/article/pii/S0378437106001324DOIback to textback to text
- 45 bookFrom Statistical Physics to Statistical Inference and Back.New York Heidelberg BerlinSpringer1994, URL: http://www.springer.com/physics/complexity/book/978-0-7923-2775-2back to text
- 46 bookGaussian Processes for Machine Learning.The MIT Press11 2005, URL: https://doi.org/10.7551/mitpress/3206.001.0001DOIback to text
- 47 articleBlind Construction of Optimal Nonlinear Recursive Predictors for Discrete Sequences.2004, URL: https://arxiv.org/abs/cs/0406011DOIback to text
- 48 bookSparse Image and Signal Processing: Wavelets, Curvelets, Morphological Diversity.ISBN:9780521119139Cambridge University Press2010back to text
- 49 articleDetecting Strange Attractors in Turbulence.Non Linear Optimization8981981, 366--381URL: http://www.springerlink.com/content/b254x77553874745/back to text
- 50 articleTime-Frequency Analysis as Probabilistic Inference.IEEE Transactions on Signal Processing62232014, 6171-6183DOIback to textback to text
- 51 articleRevisting multifractality of high resolution temporal rainfall using a wavelet-based formalism.Water Resources Research422006back to text