2023Activity reportProject-TeamMONC
RNSR: 201521155J- Research center Inria Centre at the University of Bordeaux
- In partnership with:CNRS, Institut Polytechnique de Bordeaux
- Team name: Mathematical modeling for Oncology
- In collaboration with:Institut de Mathématiques de Bordeaux (IMB)
- Domain:Digital Health, Biology and Earth
- Theme:Modeling and Control for Life Sciences
Keywords
Computer Science and Digital Science
- A6. Modeling, simulation and control
- A6.1. Methods in mathematical modeling
- A6.1.1. Continuous Modeling (PDE, ODE)
- A6.1.4. Multiscale modeling
- A6.1.5. Multiphysics modeling
- A6.2. Scientific computing, Numerical Analysis & Optimization
- A6.2.1. Numerical analysis of PDE and ODE
- A6.2.4. Statistical methods
- A6.2.6. Optimization
- A6.2.7. High performance computing
- A6.3. Computation-data interaction
- A6.3.1. Inverse problems
- A6.3.2. Data assimilation
- A6.3.3. Data processing
- A6.3.4. Model reduction
- A6.5. Mathematical modeling for physical sciences
- A6.5.2. Fluid mechanics
- A9. Artificial intelligence
- A9.2. Machine learning
Other Research Topics and Application Domains
- B1.1.7. Bioinformatics
- B1.1.8. Mathematical biology
- B1.1.10. Systems and synthetic biology
- B2.2.3. Cancer
- B2.4.2. Drug resistance
- B2.6.1. Brain imaging
- B2.6.3. Biological Imaging
1 Team members, visitors, external collaborators
Research Scientists
- Clair Poignard [Team leader, INRIA, Senior Researcher, HDR]
- Baudouin Denis de Senneville [CNRS, Researcher, HDR]
- Christele Etchegaray [INRIA, Researcher]
- Nicolas Papadakis [CNRS, Senior Researcher, from Jul 2023, HDR]
- Olivier Saut [CNRS, Senior Researcher, HDR]
Faculty Members
- Annabelle Collin [BORDEAUX INP, Associate Professor, HDR]
- David Dean [Université de Bordeaux, HDR]
Post-Doctoral Fellows
- Giorgia Ciavolella [INRIA, Post-Doctoral Fellow]
- Van Linh Le [Institut Bergonie]
- Emma Leschiera [Inria, until Oct 2023]
PhD Students
- Simon Bihoreau [INRIA]
- Khaoula Chahdi [INRIA]
- Kylian Desier [UNIV BORDEAUX]
- Audrey Gossard [INRIA, from Nov 2023]
- Julien Granet [CNRS]
- Eloise Inacio [INRIA]
- Pedro Jaramillo Aguayo [BORDEAUX INP, ATER]
- Guillaume Martinroche [UNIV BORDEAUX]
- Virginie Montalibet [UNIV BORDEAUX]
- Simone Nati Poltri [BORDEAUX INP]
- Yannis Petitpas [UNIV BORDEAUX, from Oct 2023]
- Florian Robert [UNIV BORDEAUX]
- Olivier Sutter [CHU AVICIENNE AP-HP]
Technical Staff
- Jerome Faure [Inria, until Jul 2023]
- Luc Lafitte [INRIA, Engineer]
Interns and Apprentices
- Victor Bousch [Inria, from Mar 2023 until May 2023]
- Maria Correa Abendano [Inria]
- Mouna Naim [Inria]
- Camilla Paleari [Inria, from Oct 2023]
Administrative Assistant
- Fabienne Cuyollaa [BORDEAUX INP, from Aug 2023]
External Collaborator
- Damien Voyer [EIGSI]
2 Overall objectives
2.1 Objectives
The MONC project-team aims at developing new mathematical models from partial differential equations and statistical methods and based on biological and medical knowledge. Our goal is ultimately to be able to help clinicians and/or biologists to better understand, predict or control the evolution of the disease and possibly evaluate the therapeutic response, in a clinical context or for pre-clinical studies. We develop patient-specific approaches (mainly based on medical images) as well as population-type approaches in order to take advantage of large databases.
In vivo modeling of tumors is limited by the amount of information available. However, recently, there have been dramatic increases in the scope and quality of patient-specific data from non-invasive imaging methods, so that several potentially valuable measurements are now available to quantitatively measure tumor evolution, assess tumor status as well as anatomical or functional details. Using different techniques from biology or imaging - such as CT scan, magnetic resonance imaging (MRI), or positron emission tomography (PET) - it is now possible to evaluate and define tumor status at different levels or scales: physiological, molecular and cellular.
In the meantime, the understanding of the biological mechanisms of tumor growth, including the influence of the micro-environment, has greatly increased. Medical doctors now have access to a wide spectrum of therapies (surgery, mini-invasive techniques, radiotherapies, chemotherapies, targeted therapies, immunotherapies...).
Our project aims at helping oncologists in their followup of patients via the development of novel quantitative methods for evaluation cancer progression. The idea is to build phenomenological mathematical models based on data obtained in the clinical imaging routine like CT scans, MRIs and PET scans. We therefore want to offer medical doctors patient-specific tumor evolution models, which are able to evaluate – on the basis of previously collected data and within the limits of phenomenological models – the time evolution of the pathology at subsequent times and the response to therapies. More precisely, our goal is to help clinicians answer the following questions thanks to our numerical tools:
- When is it necessary to start a treatment?
- What is the best time to change a treatment?
- When to stop a treatment?
We also intend to incorporate real-time model information for improving the accuracy and efficacy of non invasive or micro-invasive tumor ablation techniques like acoustic hyperthermia, electroporation, radio-frequency, cryo-ablation and of course radiotherapies.
There is therefore a dire need of integrating biological knowledge into mathematical models based on clinical or experimental data. A major purpose of our project is also to create new mathematical models and new paradigms for data assimilation that are adapted to the biological nature of the disease and to the amount of multi-modal data available.
2.2 General strategy
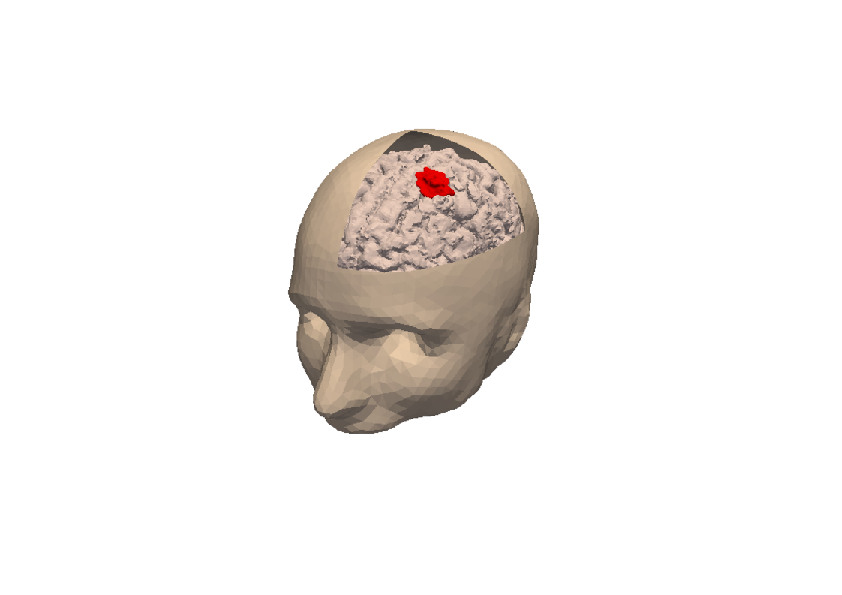
3D numerical simulation of a meningioma.
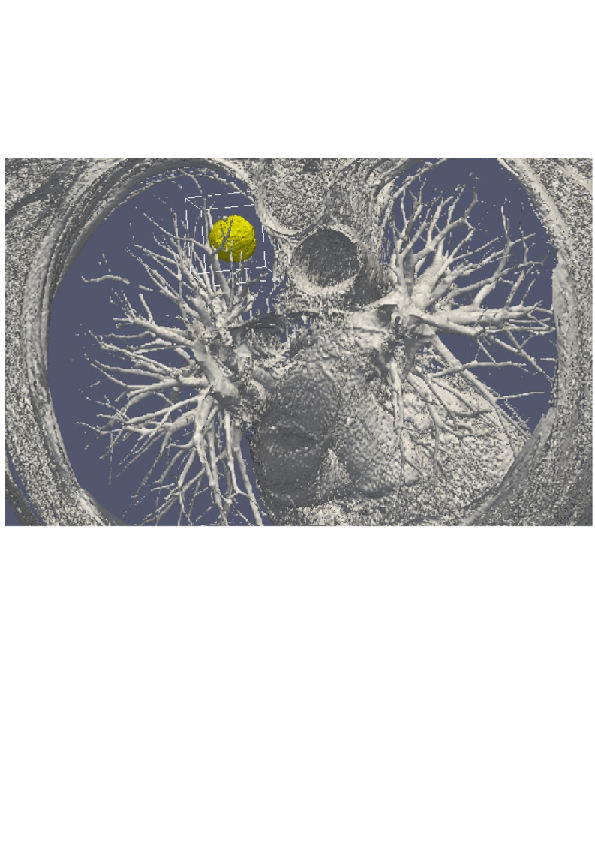
3D numerical simulation of a lung tumor.
Our general strategy may be described with the following sequence:
- Stage 1: Derivation of mechanistic models based on the biological knowledge and the available observations. The construction of such models relies on the up-to-date biological knowledge at the cellular level including description of the cell-cycle, interaction with the microenvironement (angiogenesis, interaction with the stroma). Such models also include a "macroscopic" description of specific molecular pathways that are known to have a critical role in carcinogenesis or that are targeted by new drugs. We emphasize that for this purpose, close interactions with biologists are crucial. Lots of works devoted to modeling at the cellular level are available in the literature. However, in order to be able to use these models in a clinical context, the tumor is also to be described at the tissue level. The in vitro mechanical characterization of tumor tissues has been widely studied. Yet no description that could be patient specific or even tumor specific is available. It is therefore necessary to build adapted phenomenological models, according to the biological and clinical reality.
-
Stage 2: Data collection. In the clinical context, data may come from medical imaging (MRI, CT-Scan, PET scan) at different time points. We need longitudinal data in time in order to be able to understand or describe the evolution of the disease. Data may also be obtained from analyses of blood samples, biopsies or other quantitative biomarkers. A close collaboration with clinicians is required for selecting the specific cases to focus on, the understanding of the key points and data, the classification of the grades of the tumors, the understanding of the treatment,...In the preclinical context, data may for instance be macroscopic measurements of the tumor volume for subcutaneous cases, green fluorescence protein (GFP) quantifications for total number of living cells, non-invasive bioluminescence signals or even imaging obtained with devices adapted to small animals.
- Data processing: Besides selection of representative cases by our collaborators, most of the time, data has to be processed before being used in our models. We develop novel methods for semi-automatic (implemented in SegmentIt) as well as supervized approaches (machine learning or deep learning) for segmentation, non-rigid registration and extraction of image texture information (radiomics, deep learning).
- Stage 3: Adaptation of the model to data. The model has to be adapted to data: it is useless to have a model considering many biological features of the disease if it cannot be reliably parameterized with available data. For example, very detailed descriptions of the angiogenesis process found in the literature cannot be used, as they have too much parameters to determine for the information available. A pragmatic approach has to be developed for this purpose. On the other hand, one has to try to model any element that can be useful to exploit the image. Parameterizing must be performed carefully in order to achieve an optimal trade-off between the accuracy of the model, its complexity, identifiability and predictive power. Parameter estimation is a critical issue in mathematical biology: if there are too many parameters, it will be impossible to estimate them but if the model is too simple, it will be too far from reality.
-
Stage 4: Data assimilation. Because of data complexity and scarcity - for example multimodal, longitudinal medical imaging - data assimilation is a major challenge. Such a process is a combination of methods for solving inverse problems and statistical methods including machine learning strategies.
- Personalized models: Currently, most of the inverse problems developed in the team are solved using a gradient method coupled with some MCMC type algorithm. We are now trying to use more efficient methods as Kalman type filters or so-called Luenberger filter (nudging). Using sequential methods could also simplify Stage 3 because they can be used even with complex models. Of course, the strategy used by the team depends on the quantity and the quality of data. It is not the same if we have an homogeneous population of cases or if it is a very specific isolated case.
- Statistical learning: In some clinical cases, there is no longitudinal data available to build a mathematical model describing the evolution of the disease. In these cases (e.g. in our collaboration with Humanitas Research Hospital on low grade gliomas or Institut Bergonié on soft-tissue sarcoma), we use machine learning techniques to correlate clinical and imaging features with clinical outcome of patients (radiomics). When longitudinal data and a sufficient number of patients are available, we combine this approach and mathematical modeling by adding the personalized model parameters for each patient as features in the statistical algorithm. Our goal is then to have a better description of the evolution of the disease over time (as compared to only taking temporal variations of features into account as in delta-radiomics approaches). We also plan to use statistical algorithms to build reduced-order models, more efficient to run or calibrate than the original models.
- Data assimilation of gene expression. "Omics" data become more and more important in oncology and we aim at developing our models using this information as well. For example, in our work on GIST, we have taken the effect of a Ckit mutation on resistance to treatment into account. However, it is still not clear how to use in general gene expression data in our macroscopic models, and particularly how to connect the genotype to the phenotype and the macroscopic growth. We expect to use statistical learning techniques on populations of patients in order to move towards this direction, but we emphasize that this task is very prospective and is a scientific challenge in itself.
- Stage 5: Patient-specific Simulation and prediction, Stratification. Once the mechanistic models have been parametrized, they can be used to run patient-specific simulations and predictions. The statistical models offer new stratifications of patients (i.t. an algorithm that tells from images and clinical information wheter a patient with soft-tissue sarcoma is more likely to be a good or bad responder to neoadjuvant chemotherapy). Building robust algorithms (e.g. that can be deployed over multiple clinical centers) also requires working on quantifying uncertainties.
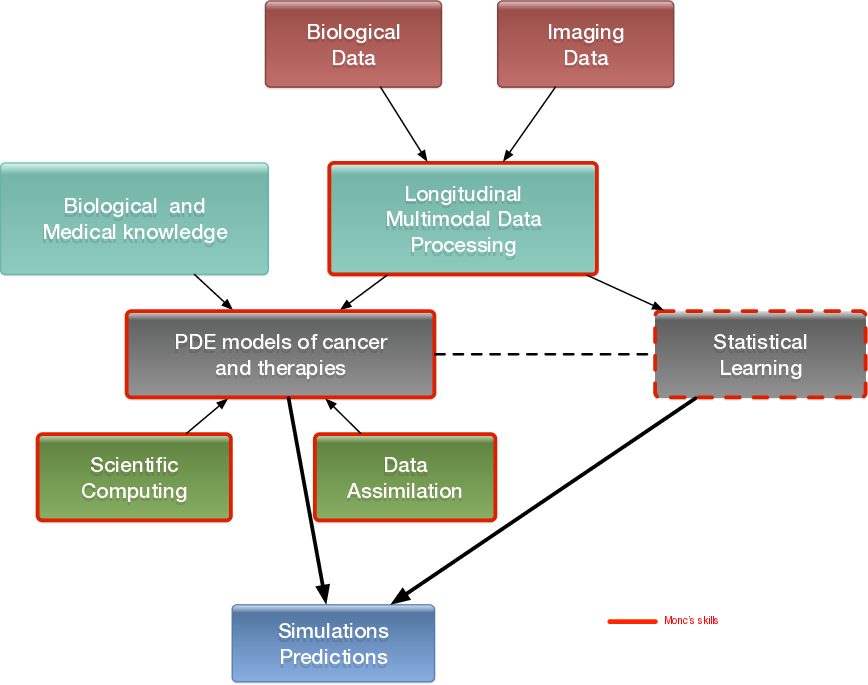
General strategy of the team to build meaningful models in oncology.
3 Research program
3.1 Introduction
We are working in the context of data-driven medicine against cancer. We aim at coupling mathematical models with data to address relevant challenges for biologists and clinicians in order for instance to improve our understanding in cancer biology and pharmacology, assist the development of novel therapeutic approaches or develop personalized decision-helping tools for monitoring the disease and evaluating therapies.
More precisely, our research on mathematical oncology is three-fold:
- Axis 1: Tumor modeling for patient-specific simulations: Clinical monitoring. Numerical markers from imaging data. Radiomics.
- Axis 2: Bio-physical modeling for personalized therapies: Electroporation from cells to tissue. Radiotherapy.
- Axis 3: Quantitative cancer modeling for biological studies: Biological mechanisms. Metastatic dissemination. Physical properties of microtumors.
In the first axis, we aim at producing patient-specific simulations of the growth of a tumor or its response to treatment starting from a series of images. We hope to be able to offer a valuable insight on the disease to the clinicians in order to improve the decision process. This would be particularly useful in the cases of relapses or for metastatic diseases.
The second axis aims at modeling biophysical therapies like irreversible electroporation, but also radiotherapy, thermo-ablations, radio-frequency ablations or electrochemotherapies that play a crucial role for a local treatment of the disease.
The third axis is essential since it is a way to better understand and model the biological reality of cancer growth and the (possibly complex) effects of therapeutic intervention. Modeling in this case also helps to interpret the experimental results and improve the accuracy of the models used in Axis 1. Technically speaking, some of the computing tools are similar to those of Axis 1.
Since our models are higly data driven, a transverse axis dedicated to data assimilation has been recently added to our research program.
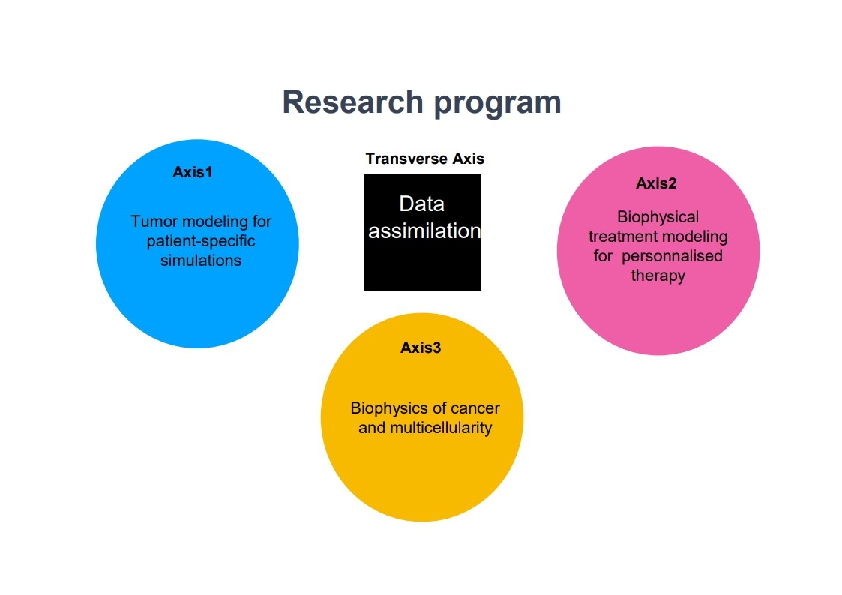
Research program organisation into 3 axes and 1 transverse axis.
3.2 Axis 1: Tumor modeling for patient-specific simulations
The gold standard treatment for most cancers is surgery. In the case where total resection of the tumor is possible, the patient often benefits from an adjuvant therapy (radiotherapy, chemotherapy, targeted therapy or a combination of them) in order to eliminate the potentially remaining cells that may not be visible. In this case personalized modeling of tumor growth is useless and statistical modeling will be able to quantify the risk of relapse, the mean progression-free survival time...However if total resection is not possible or if metastases emerge from distant sites, clinicians will try to control the disease for as long as possible. A wide set of tools are available. Clinicians may treat the disease by physical interventions (radiofrequency ablation, cryoablation, radiotherapy, electroporation, focalized ultrasound,...) or chemical agents (chemotherapies, targeted therapies, antiangiogenic drugs, immunotherapies, hormonotherapies). One can also decide to monitor the patient without any treatment (this is the case for slowly growing tumors like some metastases to the lung, some lymphomas or for some low grade glioma). A reliable patient-specific model of tumor evolution with or without therapy may have different uses:
- Case without treatment: the evaluation of the growth of the tumor would offer a useful indication for the time at which the tumor may reach a critical size. For example, radiofrequency ablation of pulmonary lesion is very efficient as long as the diameter of the lesion is smaller than 3 cm. Thus, the prediction can help the clinician plan the intervention. For slowly growing tumors, quantitative modeling can also help to decide at what time interval the patient has to undergo a CT-scan. CT-scans are irradiative exams and there is a challenge for decreasing their occurrence for each patient. It has also an economical impact. And if the disease evolution starts to differ from the prediction, this might mean that some events have occurred at the biological level. For instance, it could be the rise of an aggressive phenotype or cells that leave a dormancy state. This kind of events cannot be predicted, but some mismatch with respect to the prediction can be an indirect proof of their existence. It could be an indication for the clinician to start a treatment.
- Case with treatment: a model can help to understand and to quantify the final outcome of a treatment using the early response. It can help for a redefinition of the treatment planning. Modeling can also help to anticipate the relapse by analyzing some functional aspects of the tumor. Again, a deviation with respect to reference curves can mean a lack of efficiency of the therapy or a relapse. Moreover, for a long time, the response to a treatment has been quantified by the RECIST criteria which consists in (roughly speaking) measuring the diameters of the largest tumor of the patient, as it is seen on a CT-scan. This criteria is still widely used and was quite efficient for chemotherapies and radiotherapies that induce a decrease of the size of the lesion. However, with the systematic use of targeted therapies and anti-angiogenic drugs that modify the physiology of the tumor, the size may remain unchanged even if the drug is efficient and deeply modifies the tumor behavior. One better way to estimate this effect could be to use functional imaging (Pet-scan, perfusion or diffusion MRI, ...), a model can then be used to exploit the data and to understand in what extent the therapy is efficient.
- Optimization: currently, we do not believe that we can optimize a particular treatment in terms of distribution of doses, number, planning with the model that we will develop in a medium term perspective.
The scientific challenge is therefore as follows: given the history of the patient, the nature of the primitive tumor, its histopathology, knowing the treatments that patients have undergone, some biological facts on the tumor and having a sequence of images (CT-scan, MRI, PET or a mix of them), are we able to provide a numerical simulation of the extension of the tumor and of its metabolism that fits as best as possible with the data (CT-scans or functional data) and that is predictive in order to address the clinical cases described above?
Our approach relies on the elaboration of PDE models and their parametrization with images by coupling deterministic and stochastic methods. The PDE models rely on the description of the dynamics of cell populations. The number of populations depends on the pathology. For example, for glioblastoma, one needs to use proliferative cells, invasive cells, quiescent cells as well as necrotic tissues to be able to reproduce realistic behaviors of the disease. In order to describe the relapse for hepatic metastases of gastro-intestinal stromal tumor (gist), one needs three cell populations: proliferative cells, healthy tissue and necrotic tissue.
The law of proliferation is often coupled with a model for the angiogenesis. However such models of angiogenesis involve too many non measurable parameters to be used with real clinical data and therefore one has to use simplified or even simplistic versions. The law of proliferation often mimics the existence of an hypoxia threshold, it consists of an ODE. or a PDE that describes the evolution of the growth rate as a combination of sigmoid functions of nutrients or roughly speaking oxygen concentration. Usually, several laws are available for a given pathology since at this level, there are no quantitative argument to choose a particular one.
The velocity of the tumor growth differs depending on the nature of the tumor. For metastases, we will derive the velocity thanks to Darcy's law in order to express that the extension of the tumor is basically due to the increase of volume. This gives a sharp interface between the metastasis and the surrounding healthy tissues, as observed by anatomopathologists. For primitive tumors like glioma or lung cancer, we use reaction-diffusion equations in order to describe the invasive aspects of such primitive tumors.
The modeling of the drugs depends on the nature of the drug: for chemotherapies, a death term can be added into the equations of the population of cells, while antiangiogenic drugs have to be introduced in a angiogenic model. Resistance to treatment can be described either by several populations of cells or with non-constant growth or death rates. As said before, it is still currently difficult to model the changes of phenotype or mutations, we therefore propose to investigate this kind of phenomena by looking at deviations of the numerical simulations compared to the medical observations.
The calibration of the model is achieved by using a series (at least 2) of images of the same patient and by minimizing a cost function. The cost function contains at least the difference between the volume of the tumor that is measured on the images with the computed one. It also contains elements on the geometry, on the necrosis and any information that can be obtained through the medical images. We will pay special attention to functional imaging (PET, perfusion and diffusion MRI). The inverse problem is solved using a gradient method coupled with some Monte-Carlo type algorithm. If a large number of similar cases is available, one can imagine to use statistical algorithms like random forests to use some non quantitative data like the gender, the age, the origin of the primitive tumor...for example for choosing the model for the growth rate for a patient using this population knowledge (and then to fully adapt the model to the patient by calibrating this particular model on patient data) or for having a better initial estimation of the modeling parameters. We have obtained several preliminary results concerning lung metastases including treatments and for metastases to the liver.
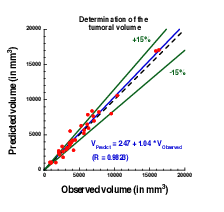
Plot showing the accuracy of our prediction on meningioma volume. Each point corresponds to a patient whose two first exams were used to calibrate our model. A patient-specific prediction was made with this calibrated model and compared with the actual volume as measured on a third time by clinicians. A perfect prediction would be on the black dashed line. Medical data was obtained from Prof. Loiseau, CHU Pellegrin.
3.3 Axis 2: Bio-physical modeling for personalized therapies
In this axis, we investigate locoregional therapies such as radiotherapy, irreversible electroporation. Electroporation consists in increasing the membrane permeability of cells by the delivery of high voltage pulses. This non-thermal phenomenon can be transient (reversible) or irreversible (IRE). IRE or electro-chemotherapy – which is a combination of reversible electroporation with a cytotoxic drug – are essential tools for the treatment of a metastatic disease. Numerical modeling of these therapies is a clear scientific challenge. Clinical applications of the modeling are the main target, which thus drives the scientific approach, even though theoretical studies in order to improve the knowledge of the biological phenomena, in particular for electroporation, should also be addressed. However, this subject is quite wide and we focus on two particular approaches: some aspects of radiotherapies and electro-chemotherapy. This choice is motivated partly by pragmatic reasons: we already have collaborations with physicians on these therapies. Other treatments could be probably treated with the same approach, but we do not plan to work on this subject on a medium term.
-
Radiotherapy (RT) is a common therapy for cancer. Typically, using a CT scan of the patient with the structures of interest (tumor, organs at risk) delineated, the clinicians optimize the dose delivery to treat the tumor while preserving healthy tissues. The RT is then delivered every day using low resolution scans (CBCT) to position the beams. Under treatment the patient may lose weight and the tumor shrinks. These changes may affect the propagation of the beams and subsequently change the dose that is effectively delivered. It could be harmful for the patient especially if sensitive organs are concerned. In such cases, a replanification of the RT could be done to adjust the therapeutical protocol. Unfortunately, this process takes too much time to be performed routinely. The challenges faced by clinicians are numerous, we focus on two of them:
- Detecting the need of replanification: we are using the positioning scans to evaluate the movement and deformation of the various structures of interest. Thus we can detect whether or not a structure has moved out of the safe margins (fixed by clinicians) and thus if a replanification may be necessary. In a retrospective study, our work can also be used to determine RT margins when there are no standard ones. A collaboration with the RT department of Institut Bergonié is underway on the treatment of retroperitoneal sarcoma and ENT tumors (head and neck cancers). A retrospective study was performed on 11 patients with retro-peritoneal sarcoma. The results have shown that the safety margins (on the RT) that clinicians are currently using are probably not large enough. The tool used in this study was developed by an engineer funded by INRIA (Cynthia Périer, ADT Sesar). We used well validated methods from a level-set approach and segmentation / registration methods. The originality and difficulty lie in the fact that we are dealing with real data in a clinical setup. Clinicians have currently no way to perform complex measurements with their clinical tools. This prevents them from investigating the replanification. Our work and the tools developed pave the way for easier studies on evaluation of RT plans in collaboration with Institut Bergonié. There was no modeling involved in this work that arose during discussions with our collaborators. The main purpose of the team is to have meaningful outcomes of our research for clinicians, sometimes it implies leaving a bit our area of expertise.
- Evaluating RT efficacy and finding correlation between the radiological responses and the clinical outcome: our goal is to help doctors to identify correlation between the response to RT (as seen on images) and the longer term clinical outcome of the patient. Typically, we aim at helping them to decide when to plan the next exam after the RT. For patients whose response has been linked to worse prognosis, this exam would have to be planned earlier. This is the subject of collaborations with Institut Bergonié and CHU Bordeaux on different cancers (head and neck, pancreas). The response is evaluated from image markers (e.g. using texture information) or with a mathematical model developed in Axis 1. The other challenges are either out of reach or not in the domain of expertise of the team. Yet our works may tackle some important issues for adaptive radiotherapy.
-
Both IRE and electrochemotherapy are anticancerous treatments based on the same phenomenon: the electroporation of cell membranes. This phenomenon is known for a few decades but it is still not well understood, therefore our interest is two fold:
- We want to use mathematical models in order to better understand the biological behavior and the effect of the treatment. We work in tight collaboration with biologists and bioeletromagneticians to derive precise models of cell and tissue electroporation, in the continuity of the research program of the Inria team-project MC2. These studies lead to complex non-linear mathematical models involving some parameters (as less as possible). Numerical methods to compute precisely such models and the calibration of the parameters with the experimental data are then addressed. Tight collaborations with the Vectorology and Anticancerous Therapies (VAT) of IGR at Villejuif, Laboratoire Ampère of Ecole Centrale Lyon and the Karlsruhe Institute of technology will continue, and we aim at developing new collaborations with Institute of Pharmacology and Structural Biology (IPBS) of Toulouse and the Laboratory of Molecular Pathology and Experimental Oncology (LMPEO) at CNR Rome, in order to understand differences of the electroporation of healthy cells and cancer cells in spheroids and tissues.
- This basic research aims at providing new understanding of electroporation, however it is necessary to address, particular questions raised by radio-oncologists that apply such treatments. One crucial question is "What pulse or what train of pulses should I apply to electroporate the tumor if the electrodes are located as given by the medical images"? Even if the real-time optimization of the placement of the electrodes for deep tumors may seem quite utopian since the clinicians face too many medical constraints that cannot be taken into account (like the position of some organs, arteries, nerves...), one can expect to produce real-time information of the validity of the placement done by the clinician. Indeed, once the placement is performed by the radiologists, medical images are usually used to visualize the localization of the electrodes. Using these medical data, a crucial goal is to provide a tool in order to compute in real-time and visualize the electric field and the electroporated region directly on theses medical images, to give the doctors a precise knowledge of the region affected by the electric field. In the long run, this research will benefit from the knowledge of the theoretical electroporation modeling, but it seems important to use the current knowledge of tissue electroporation – even quite rough –, in order to rapidly address the specific difficulty of such a goal (real-time computing of non-linear model, image segmentation and visualization). Tight collaborations with CHU Pellegrin at Bordeaux, and CHU J. Verdier at Bondy are crucial.
- Radiofrequency ablation. In a collaboration with Hopital Haut Leveque, CHU Bordeaux we are trying to determine the efficacy and risk of relapse of hepatocellular carcinoma treated by radiofrequency ablation. For this matter we are using geometrical measurements on images (margins of the RFA, distance to the boundary of the organ) as well as texture information to statistically evaluate the clinical outcome of patients.
- Intensity focused ultrasound. In collaboration with Utrecht Medical center, we aim at tackling several challenges in clinical applications of IFU: target tracking, dose delivery...
3.4 Axis 3: Quantitative cancer modeling for biological studies
With the emergence and improvement of a plethora of experimental techniques, the molecular, cellular and tissue biology has operated a shift toward a more quantitative science, in particular in the domain of cancer biology. These quantitative assays generate a large amount of data that call for theoretical formalism in order to better understand and predict the complex phenomena involved. Indeed, due to the huge complexity underlying the development of a cancer disease that involves multiple scales (from the genetic, intra-cellular scale to the scale of the whole organism), and a large number of interacting physiological processes (see the so-called "hallmarks of cancer"), several questions are not fully understood. Among these, we want to focus on the most clinically relevant ones, such as the general laws governing tumor growth and the development of metastases (secondary tumors, responsible of 90% of the deaths from a solid cancer), and the physics of tumors which is crucial to quantify drug uptake for instance.
In this context, it is thus challenging to exploit the diversity of the data available in experimental settings (such as in vitro tumor spheroids or in vivo mice experiments) in order to improve our understanding of the disease and its dynamics, which in turn lead to validation, refinement and better tuning of the macroscopic models used in the axes 1 and 2 for clinical applications.
In recent years, several new findings challenged the classical vision of the metastatic development biology, in particular by the discovery of organism-scale phenomena that are amenable to a dynamical description in terms of mathematical models based on differential equations. These include the angiogenesis-mediated distant inhibition of secondary tumors by a primary tumor the pre-metastatic niche or the self-seeding phenomenon Building a general, cancer type specific, comprehensive theory that would integrate these dynamical processes remains an open challenge.
Starting from the available multi-modal data and relevant biological or therapeutic questions, our purpose is to develop adapted mathematical models (i.e. identifiable from the data) that recapitulate the existing knowledge and reduce it to its more fundamental components, with two main purposes:
- to generate quantitative and empirically testable predictions that allow to assess biological hypotheses or
- to investigate the therapeutic management of the disease and profile optimal experimental strategies for anti-cancerous therapies (drug uptakes or electric field parameters for instance).
We believe that the feedback loop between theoretical modeling and experimental studies can help to generate new knowledge and improve our predictive abilities for clinical diagnosis, prognosis, and therapeutic decision. Let us note that the first point is in direct link with the axes 1 and 2 of the team since it allows us to experimentally validate the models at the biological scale (in vitro and in vivo experiments) for further clinical applications.
More precisely, we first base ourselves on a thorough exploration of the biological literature of the biological phenomena we want to model: growth of tumor spheroids, in vivo tumor growth in mice, initiation and development of the metastases, distribution of anti-cancerous drugs. Then we investigate, using basic statistical tools, the data we dispose, which can range from: spatial distribution of heterogeneous cell population within tumor spheroids, expression of cell markers (such as green fluorescent protein for cancer cells or specific antibodies for other cell types), bioluminescence, direct volume measurement or even intra-vital images obtained with specific imaging devices. According to the data type, we further build dedicated mathematical models that are based either on PDEs (when spatial data is available, or when time evolution of a structured density can be inferred from the data, for instance for a population of tumors) or ODEs (for scalar longitudinal data). These models are confronted to the data by two principal means:
- when possible, experimental assays can give a direct measurement of some parameters (such as the proliferation rate or the migration speed) or
- statistical tools to infer the parameters from observables of the model.
This last point is of particular relevance to tackle the problem of the large inter-animal variability and we use adapted statistical tools such as the mixed-effects modeling framework.
Once the models are shown able to describe the data and are properly calibrated, we use them to test or simulate biological hypotheses. Based on our simulations, we then aim at proposing to our biological collaborators new experiments to confirm or infirm newly generated hypotheses, or to test different administration protocols of the drugs for in vivo and in vitro protocols.
Another motivation of this axis deals with the link between cancer evolution and multicellularity. The goals is somehow to revisit the cancer description under the prisms of multicellularity and metabolism. A tight collaboration with B. Daignan-Fornier 's team at IBGC has just started with the 80 prime's project driven by C. Etchegaray on this topics.
4 Application domains
4.1 Tumor growth monitoring and therapeutic evaluation
Each type of cancer is different and requires an adequate model. More specifically, we are currently working on the following diseases:
- Glioma (brain tumors) of various grades,
- Metastases to the lung, liver and brain from various organs,
- Soft-tissue sarcoma,
- Kidney cancer and its metastases,
- Non small cell lung carcinoma.
In this context our application domains are:
- Image-driven patient-specific simulations of tumor growth and treatments,
- Parameter estimation and data assimilation of medical images,
- Machine and deep learning methods for delineating the lesions and stratifying patients according to their responses to treatment or risks of relapse.
4.2 Biophysical therapies
- Modeling of irreversible electroporation adn electrochemotherapy on biological and clinical scales.
- Evaluation of radiotherapy and radiofrequency ablation.
4.3 In-vitro and animals experimentations in oncology
- Theoretical biology of the rheology of microtumors: dynamics of a population of tumors in mutual interactions, uptake of drugs, effect of electric field on the growth of microtumors, growth under mechanical or chemical (hypoxia) constraints.
- Mathematical models of multicellular and unicellular yeast organism, model for metabolism.
5 Social and environmental responsibility
5.1 Footprint of research activities
Numerical computations on (GPU) clusters like Plafrim. The permanent members of the team reduced drastically their travels to scientifically relevant journey (for instance small conferences where we can really meet scientists, stay of at least 1 week for efficient collaboration etc).
A. Collin is in charge of the sustainable development and social responsability at Enseirb-Matmeca. In addition A. Collin is involved in "Fresques du climat" formation. She is also involved in EcoInfo CNRS group.
N. Papadakis is co-director of IMB, in charge of the sustainable development and social responsability.
O. Saut is in charge of sustainable development at CNRS Mathématiques and membre of the National Sustainable Development Council at CNRS.
5.2 Impact of research results
In the long run, our research could yield interesting outcomes for cancer patients. Yet we are mostly building proofs of concept that would have to be taken over by an industrial partner for any transfer towards clinics (like we did with Sophia Genetics in the past).
The softwares EVolution and IRENA are combined into PrimetimeIRE and into 3D Slicer extensions for clinical purpose. PrimetimeIRE is in its test phase at Avicenne hospital, AP-HP.
5.3 Gender equity
C. Etchegaray is in charge of the gender equity committee for IMB. She is also correspondent on this topic for CNRS (Insmi), and co-head of the local Inria parity-equality committee.
6 Highlights of the year
6.1 Starting projects
- ANR MIRE4VTACH (4 years, 250keuros, leader: A. Collin, Bdx INP, co-PI: P. Jais IHU LIRYC)
- Dielectric project (3 years, 450keuros, leader: P. Jais, IHU LIRYC, co-PIs: H. Cochet IHU, C.Poignard Inria)
- ANR Holibrain (3 years, 550keuros, leader: P. Coupé, CNRS, co-leader: N. Papadakis, CNRS)
- Univ. Bordeaux Research project MONC4AS (2 years, 90keuros, leader: A. Collin, Bdx INP, co-PI F. Moisan, CR Inserm)
6.2 Defenses
- HDR defense of A. Collin.
- PhD defense of P. Jaramillo-Aguayo.
6.3 Software testing
- The plugins EVOLUTION and Irena for 3DSlicer that enable to compute during the ablation the electric field on the medical image have been tested in clinical configuration at CHU Poitiers.
6.4 Awards
- The project AI4DEEP led by L. Lafitte, B. Denis de Senneville and C. Poignard won the DEEPTECH award at the Hackatech 2023.
7 New results
7.1 AI for medical images
Participants: Annabelle Collin, Baudouin Denis de Senneville, Olivier Saut.
-
Integration of operator-validated contours in deformable image registration for dose accumulation in radiotherapy.
L. Bosma et al. Physics and Imaging in Radioation Oncology, 2023, 5 (4).
-
Evaluation of four tumour growth models to describe the natural history of meningiomas
J. Engelhardt et al. EBioMedicine, 2023, 94
-
Generating high-resolution synthetic CT from lung MRI with ultrashort echo-times: initial evaluation in cystic fibrosis.
A. Longuefosse et al. Raiology 2023, 308(1).
-
Non-Contrast-Enhanced Functional Lung MRI to Evaluate Treatment Response of Allergic Bronchopulmonary Aspergillosis in Patients With Cystic Fibrosis: A Pilot Study
I. Benlala et al. Journal of Magnetic Resonance Imaging, In press.
-
3D motion strategy for online volumetric thermometry using simultaneous multi-slice EPI at 1.5T : an evaluation study.
V. Ozenne et al. International Journal of Hyperthermia, 2023, 40 (1),
-
Development and external validation of a prediction model for the transition from mild to moderate or severe form of COVID-19.
M. Zysman, et al. European Radiology, In press.
7.2 Electroporation modeling
Participants: Annabelle Collin, Clair Poignard.
-
Deciphering Immediate Post-Pulse Membrane Resealing from 4-Electrode Impedance Measurements by Numerical Modeling
A. Collin et al. Journal of Bioelectricity, 2023, 5 (4)
-
How impedance measurements and imaging can be used to characterize the conductivity of tissues during the workflow of an electroporation-based therapy.
O. Sutter, et al. IEEE Transactions on Biomedical Engineering, 2023.
-
Percutaneous irreversible electroporation for the treatment of pancreatic insulinoma
J.-P. Tasu et al. Letter in Radiology, 2023. 2023
-
Phase-field model of bilipid membrane electroporation
P. Jaramillo-Aguayo et al. Journal of Mathematical Biology, 2023, 87 (18).
8 Bilateral contracts and grants with industry
8.1 Bilateral contracts with industry
Participants: Olivier Saut.
- Collaboration contract with Sophia Genetics in the context of Plan de Relance.
- Contract with Roche.
9 Partnerships and cooperations
9.1 National initiatives
On-going research projects:
9.1.1 Plan Cancer MECI
Participants: Annabelle Collin, Baudouin Denis de Senneville, Christèle Etchegaray, Clair Poignard, Olivier Saut.
- Title: Mathematical Models of Electroporation Ablation Combined with Immunotherapy for Liver and Pancreas Tumors
- Partners: INRIA Monc (leader), IPBS, AP-HP Avicenne, CHU Poitiers
- Total funds: 575k€.
9.1.2 ANR Project MIRE4VTACH
Participants: Annabelle Collin (PI), Baudouin Denis de Senneville, Christèle Etchegaray, Clair Poignard.
- Title: Modeling of Irreversible Electroporation for Ventricular Tachycardia
- Partners: INRIA Monc (leader), IHU LIRYC
- Total funds: 250k€.
9.1.3 ANR Project HOLIBRAIN
Participants: Baudouin Denis de Senneville, Nicolas Papadakis (co-PI).
- Title: Holistic Brain Analysis
- Partners: Labri (leader), INRIA Monc
- Total funds: 250k€.
9.1.4 French Federation for Cardiology grant DIELECTRIC
Participants: Annabelle Collin, Clair Poignard.
- Title: Ablation rapide par électroporation et jumeau numérique de la tachycardie ventriculaire.
- Partners: IHU LIRYC (leader), INRIA MONC, INRIA CARMEN
- Total funds: 450keuros.
9.1.5 80Prime CNRS call
Participants: Annabelle Collin, Christèle Etchegaray (PI), Clair Poignard.
- Title: EvoMulti: Identification de conditions favorisant la transition évolutive vers la multicellularité : approche expérimentale et modélisation mathématique.
- Partners: INRIA MONC, IBGC
- Acronym: EvolMulti
- Total funds: 19k€.
10 Dissemination
10.1 Promoting scientific activities
10.1.1 Scientific events: organisation
Clair Poignard is member of the scientific and organisation committees of the Math-Bio-Santé days (Nov. 2023)
10.1.2 Journal
Member of the editorial boards
Clair Poignard is Editor-in-Chief with R. Eftimié of Math. Mod. Nat. Phenom. Journal.
Clair Poignard is member of the editorial board of AIMS Bioeng. Journal.
10.1.3 Invited talks
Christèle Etchegaray : Journées Normandes de modélisation et applications, LMNO, Caen University.
10.1.4 Scientific expertise
Olivier Saut was member of the expert panel for the ANR CES45 and for the National Science Council of Poland.
Christèle Etchegaray was a reviewer for the ANR CES45.
10.1.5 Research administration
Nicolas Papadakis is vice-director of the Insitute of math of Bordeaux.
Olivier Saut is a scientific delegate at CNRS Mathématiques (), in charge of interdiscplinariy and in the steering comittee of until September 2023 then in charge of Sustainable Development and Thematic Networks at CNRS Mathématiques. He is also a member of the Ethical comittee of University of Bordeaux.
Clair Poignard was in charge of the Commission des Emplois Recherche of the Inria Research Center at Univ. Bordeaux.
Christèle Etchegaray is a member of IMB's lab council.
10.2 Teaching - Supervision - Juries
10.2.1 Teaching
- Engineering School Enseirb-Matmeca of Bordeaux INP(undergraduate level): Annabelle Collin (270h), Baudouin Denis de Senneville (20h), Nicolas Papadakis (12h)
- Master in mathematics: Nicolas Papadakis (15h)
- Engineering School ENSAM (undergraduate): Clair Poignard , (60heq).
- Cancer Biology Master: Olivier Saut and Clair Poignard (4h)
- Autumn school GDR Mathsav : Annabelle Collin (4h).
10.2.2 Supervision
The permanent staff of the Team supervises the 14 phd students.
10.2.3 Juries
Annabelle Collin was jury member of 2 PhD defense. Clair Poignard was President of the jury of 1 HDR and 1 PhD defense, and member as reviewer of 2 PhD defenses. Nicolas Papadakis was jury member of 1 HdR defense (as reviewer) and 5 PhD defense (2 as reviewer). Olivier Saut was jury member was President of the jury of 1 PhD defence, reviewer of 2 additional PhD defenses, member of the jury of one medical thesis.
10.3 Popularization
10.3.1 Interventions
Annabelle Collin gave a talk at INSERM Bettencourt school for promoting applied mathematics for future clinicians.
Annabelle Collin gave 1 talk about the environmental impact of the digital (IA4Industry)
Christèle Etchegaray gave a talk for high schools students during the "Maths week" at Bordeaux university.
Christèle Etchegaray gave 5 talks for high schools students within the "1 scientifique, 1 classe, Chiche!" initiative, at St Jean de Luz.
Christèle Etchegaray gave 1 talk for high schools female students during the event "Filles & Maths, une équation lumineuse" at Pau.
Christèle Etchegaray took part in the Inria video initiative "Une minute avec...".
Olivier Saut gave 1 talk at Université Populaire de Bordeaux and at the SIN department of University of Bordeaux.
11 Scientific production
11.1 Major publications
- 1 articleComparison of MRI-based response criteria and radiomics for the prediction of early response to transarterial radioembolization in patients with hepatocellular carcinoma.Diagnostic and Interventional Imaging1037-8July 2022, 360-366HALDOI
- 2 articleSpatial mechanistic modeling for prediction of the growth of asymptomatic meningioma.Computer Methods and Programs in Biomedicine2020HAL
- 3 articleJoint state-parameter estimation for tumor growth model.SIAM Journal on Applied Mathematics812March 2021HALDOI
- 4 articleIntensity Harmonization Techniques Influence Radiomics Features and Radiomics-based Predictions in Sarcoma Patients.Scientific Reports101December 2020HALDOI
- 5 articleT2-based MRI Delta-Radiomics Improve Response Prediction in Soft-Tissue Sarcomas Treated by Neoadjuvant Chemotherapy.Journal of Magnetic Resonance Imaging502August 2019, 497-510HALDOI
- 6 articleViscoelastic modeling of the fusion of multicellular tumor spheroids in growth phase.Journal of Theoretical Biology454October 2018, 102-109HALDOI
- 7 articleLiver contrast-enhanced sonography: Computer-assisted differentiation between focal nodular hyperplasia and inflammatory hepatocellular adenoma by reference to microbubble transport patterns.European Radiology2020HALDOI
- 8 articleDeciphering tumour tissue organization by 3D electron microscopy and machine learning.Communications Biology412021, 1390HALDOI
- 9 articleNumerical Workflow of Irreversible Electroporation for Deep-Seated Tumor.Physics in Medicine and Biology645March 2019, 055016HALDOI
- 10 articleSpatial modelling of tumour drug resistance: the case of GIST liver metastases Mathematical Medicine and Biology Advance.Mathematical Medicine and Biology002016, 1 - 26HALDOI
- 11 articleImproved 18-FDG PET/CT diagnosis of multiple myeloma diffuse disease by radiomics analysis.Nuclear Medicine Communications4210October 2021, 1135-1143HALDOI
- 12 articleComputational Trials: Unraveling Motility Phenotypes, Progression Patterns, and Treatment Options for Glioblastoma Multiforme.PLoS ONE111January 2016HALDOI
11.2 Publications of the year
International journals
International peer-reviewed conferences
Reports & preprints
Other scientific publications