Keywords
Computer Science and Digital Science
- A5. Interaction, multimedia and robotics
- A5.3. Image processing and analysis
- A5.4. Computer vision
- A5.9. Signal processing
- A9. Artificial intelligence
Other Research Topics and Application Domains
- B6. IT and telecom
1 Team members, visitors, external collaborators
Research Scientists
- Christine Guillemot [Team leader, Inria, Senior Researcher, HDR]
- Xiaoran Jiang [Inria, Starting Research Position]
- Maja Krivokuca [Inria, Starting Research Position]
- Mikael Le Pendu [Inria, Starting Research Position, from Nov 2020]
- Thomas Maugey [Inria, Researcher]
- Aline Roumy [Inria, Senior Researcher, HDR]
Post-Doctoral Fellows
- Ehsan Miandji [Inria, until Oct 2020]
- Anju Jose Tom [Inria, from Sep 2020]
PhD Students
- Denis Bacchus [Inria, from Sep 2020]
- Nicolas Charpenay [Univ de Rennes I, from Oct 2020]
- Simon Evain [Inria]
- Rita Fermanian [Inria, from Oct 2020]
- Patrick Garus [Orange Labs, CIFRE]
- Reda Kaafarani [Inria, from Dec 2020]
- Brandon Le Bon [Inria, from Nov 2020]
- Arthur Lecert [Inria, from Oct 2020]
- Yiqun Liu [Ateme Rennes, CIFRE, from Aug 2020]
- Xuan Hien Pham [Technicolor, CIFRE]
- Jinglei Shi [Inria]
Technical Staff
- Pierre Allain [Inria, Engineer, until Feb 2020]
- Sebastien Bellenous [Inria, Engineer, from Oct 2020]
- Fatma Hawary [Inria, Engineer, until Jun 2020]
- Guillaume Le Guludec [Inria, Engineer, from Apr 2020]
- Navid Mahmoudian Bidgoli [Inria, Engineer]
- Hoai Nam Nguyen [Inria, Engineer]
- Mira Rizkallah [Inria, Engineer, until Jun 2020]
- Alexander Sagel [Inria, Engineer, until Jan 2020]
Interns and Apprentices
- Alban Marie [Inria, from Mar 2020 until Aug 2020]
Administrative Assistant
- Huguette Bechu [Inria]
Visiting Scientist
- Saghi Hajisharif [Université de Linköping - Suède, from Sep 2020 until Oct 2020]
2 Overall objectives
2.1 Introduction
Efficient processing, i.e., analysis, storage, access and transmission of visual content, with continuously increasing data rates, in environments which are more and more mobile and distributed, remains a key challenge of the signal and image processing community. New imaging modalities, High Dynamic Range (HDR) imaging, multiview, plenoptic, light fields, 360o videos, generating very large volumes of data contribute to the sustained need for efficient algorithms for a variety of processing tasks.
Building upon a strong background on signal/image/video processing and information theory, the goal of the SIROCCO team is to design mathematically founded tools and algorithms for visual data analysis, modeling, representation, coding, and processing, with for the latter area an emphasis on inverse problems related to super-resolution, view synthesis, HDR recovery from multiple exposures, denoising and inpainting. Even if 2D imaging is still within our scope, the goal is to give a particular attention to HDR imaging, light fields, and 360o videos. The project-team activities are structured and organized around the following inter-dependent research axes:
- Visual data analysis
- Signal processing and learning methods for visual data representation and compression
- Algorithms for inverse problems in visual data processing
- Distributed coding for interactive communication.
While aiming at generic approaches, some of the solutions developed are applied to practical problems in partnership with industry (InterDigital, Ateme, Orange) or in the framework of national projects. The application domains addressed by the project are networked visual applications taking into account their various requirements and needs in terms of compression, of network adaptation, of advanced functionalities such as navigation, interactive streaming and high quality rendering.
2.2 Visual Data Analysis
Most visual data processing problems require a prior step of data analysis, of discovery and modeling of correlation structures. This is a pre-requisite for the design of dimensionality reduction methods, of compact representations and of fast processing techniques. These correlation structures often depend on the scene and on the acquisition system. Scene analysis and modeling from the data at hand is hence also part of our activities. To give examples, scene depth and scene flow estimation is a cornerstone of many approaches in multi-view and light field processing. The information on scene geometry helps constructing representations of reduced dimension for efficient (e.g. in interactive time) processing of new imaging modalities (e.g. light fields or 360o videos).
2.3 Signal processing and learning methods for visual data representation and compression
Dimensionality reduction has been at the core of signal and image processing methods, for a number of years now, hence have obviously always been central to the research of Sirocco. These methods encompass sparse and low-rank models, random low-dimensional projections in a compressive sensing framework, and graphs as a way of representing data dependencies and defining the support for learning and applying signal de-correlating transforms. The study of these models and signal processing tools is even more compelling for designing efficient algorithms for processing the large volumes of high-dimensionality data produced by novel imaging modalities. The models need to be adapted to the data at hand through learning of dictionaries or of neural networks. In order to define and learn local low-dimensional or sparse models, it is necessay to capture and understand the underlying data geometry, e.g. with the help of manifolds and manifold clustering tools. It also requires exploiting the scene geometry with the help of disparity or depth maps, or its variations in time via coarse or dense scene flows.
2.4 Algorithms for inverse problems in visual data processing
Based on the above models, besides compression, our goal is also to develop algorithms for solving a number of inverse problems in computer vision. Our emphasis is on methods to cope with limitations of sensors (e.g. enhancing spatial, angular or temporal resolution of captured data, or noise removal), to synthesize virtual views or to reconstruct (e.g. in a compressive sensing framework) light fields from a sparse set of input views, to recover HDR visual content from multiple exposures, and to enable content editing (we focus on color transfer, re-colorization, object removal and inpainting). Note that view synthesis is a key component of multiview and light field compression. View synthesis is also needed to support user navigation and interactive streaming. It is also needed to avoid angular aliasing in some post-capture processing tasks, such as re-focusing, from a sparse light field. Learning models for the data at hand is key for solving the above problems.
2.5 Distributed coding for interactive communication
The availability of wireless camera sensors has also been spurring interest for a variety of applications ranging from scene interpretation, object tracking and security environment monitoring. In such camera sensor networks, communication energy and bandwidth are scarce resources, motivating the search for new distributed image processing and coding solutions suitable for band and energy limited networking environments. Our goal is to address theoretical issues such as the problem of modeling the correlation channel between sources, and to practical coding solutions for distributed processing and communication and for interactive streaming.
3 Research program
3.1 Introduction
The research activities on analysis, compression and communication of visual data mostly rely on tools and formalisms from the areas of statistical image modeling, of signal processing, of machine learning, of coding and information theory. Some of the proposed research axes are also based on scientific foundations of computer vision (e.g. multi-view modeling and coding). We have limited this section to some tools which are central to the proposed research axes, but the design of complete compression and communication solutions obviously rely on a large number of other results in the areas of motion analysis, transform design, entropy code design, etc which cannot be all described here.
3.2 Data Dimensionality Reduction
Keywords: Manifolds, graph-based transforms, compressive sensing.
Dimensionality reduction encompasses a variety of methods for low-dimensional data embedding, such as sparse and low-rank models, random low-dimensional projections in a compressive sensing framework, and sparsifying transforms including graph-based transforms. These methods are the cornerstones of many visual data processing tasks (compression, inverse problems).
Sparse representations, compressive sensing, and dictionary learning have been shown to be powerful tools for efficient processing of visual data. The objective of sparse representations is to find a sparse approximation of a given input data. In theory, given a dictionary matrix
The recent theory of compressed sensing, in the context of discrete signals, can be seen as an effective dimensionality reduction technique. The idea behind compressive sensing is that a signal can be accurately recovered from a small number of linear measurements, at a rate much smaller than what is commonly prescribed by the Shannon-Nyquist theorem, provided that it is sparse or compressible in a known basis. Compressed sensing has emerged as a powerful framework for signal acquisition and sensor design, with a number of open issues such as learning the basis in which the signal is sparse, with the help of dictionary learning methods, or the design and optimization of the sensing matrix. The problem is in particular investigated in the context of light fields acquisition, aiming at novel camera design with the goal of offering a good trade-off between spatial and angular resolution.
While most image and video processing methods have been developed for cartesian sampling grids, new imaging modalities (e.g. point clouds, light fields) call for representations on irregular supports that can be well represented by graphs. Reducing the dimensionality of such signals require designing novel transforms yielding compact signal representation.
One example of transform is the Graph Fourier transform
whose basis functions are given by the eigenvectors of the graph Laplacian matrix
3.3 Deep neural networks
Keywords: Autoencoders, Neural Networks, Recurrent Neural Networks.
From dictionary learning which we have investigated a lot in the past, our activity is now evolving towards deep learning techniques which we are considering for dimensionality reduction. We address the problem of unsupervised learning of transforms and prediction operators that would be optimal in terms of energy compaction, considering autoencoders and neural network architectures.
An autoencoder is a neural network with an encoder
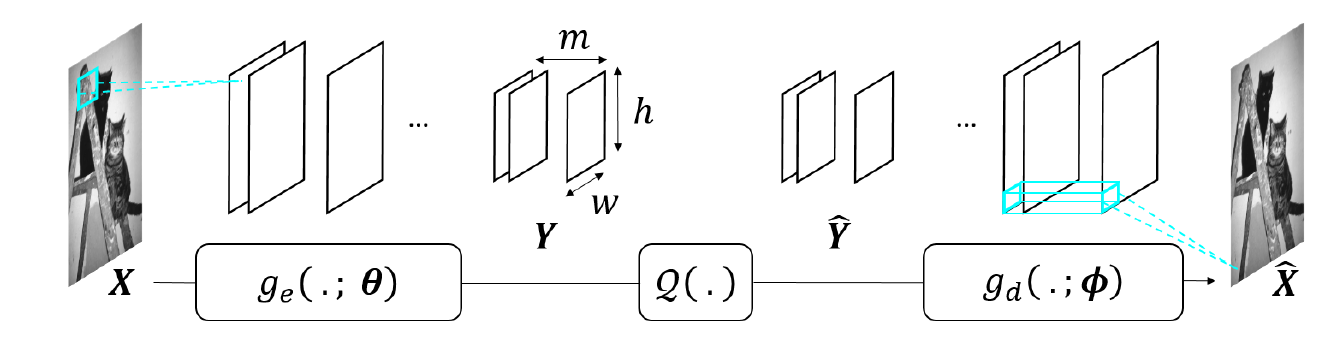
To avoid this limitation, architectures without fully-connected layer and comprising instead convolutional layers and non-linear operators, forming convolutional neural networks (CNN) may be preferrable. The obtained representation is thus a set of so-called feature maps.
The other problems that we address with the help of neural networks are scene geometry and scene flow estimation, view synthesis, prediction and interpolation with various imaging modalities. The problems are posed either as supervised or unsupervised learning tasks. Our scope of investigation includes autoencoders, convolutional networks, variational autoencoders and generative adversarial networks (GAN) but also recurrent networks and in particular Long Short Term Memory (LSTM) networks. Recurrent neural networks attempting to model time or sequence dependent behaviour, by feeding back the output of a neural network layer at time t to the input of the same network layer at time t+1, have been shown to be interesting tools for temporal frame prediction. LSTMs are particular cases of recurrent networks made of cells composed of three types of neural layers called gates.
Deep neural networks have also been shown to be very promising for solving inverse problems (e.g. super-resolution, sparse recovery in a compressive sensing framework, inpainting) in image processing. Variational autoencoders, generative adversarial networks (GAN), learn, from a set of examples, the latent space or the manifold in which the images, that we search to recover, reside. The inverse problems can be re-formulated using a regularization in the latent space learned by the network. For the needs of the regularization, the learned latent space may need to verify certain properties such as preserving distances or neighborhood of the input space, or in terms of statistical modeling. GANs, trained to produce images that are plausible, are also useful tools for learning texture models, expressed via the filters of the network, that can be used for solving problems like inpainting or view synthesis.
3.4 Coding theory
Keywords: OPTA limit (Optimum Performance Theoretically Attainable), Rate allocation, Rate-Distortion optimization, lossy coding, joint source-channel coding multiple description coding, channel modelization, oversampled frame expansions, error correcting codes..
Source coding and channel coding theory 1 is central to our compression and communication activities, in particular to the design of entropy codes and of error correcting codes. Another field in coding theory which has emerged in the context of sensor networks is Distributed Source Coding (DSC). It refers to the compression of correlated signals captured by different sensors which do not communicate between themselves. All the signals captured are compressed independently and transmitted to a central base station which has the capability to decode them jointly. DSC finds its foundation in the seminal Slepian-Wolf 2 (SW) and Wyner-Ziv 3 (WZ) theorems. Let us consider two binary correlated sources
In 1976, Wyner and Ziv considered the problem of coding of two correlated sources
4 Application domains
4.1 Overview
The application domains addressed by the project are:
- Compression with advanced functionalities of various imaging modalities
- Networked multimedia applications taking into account needs in terms of user and network adaptation (e.g., interactive streaming, resilience to channel noise)
- Content editing, post-production, and computational photography.
4.2 Compression of emerging imaging modalities
Compression of visual content remains a widely-sought capability for a large number of applications. This is particularly true for mobile applications, as the need for wireless transmission capacity will significantly increase during the years to come. Hence, efficient compression tools are required to satisfy the trend towards mobile access to larger image resolutions and higher quality. A new impulse to research in video compression is also brought by the emergence of new imaging modalities, e.g. high dynamic range (HDR) images and videos (higher bit depth, extended colorimetric space), light fields and omni-directional imaging.
Different video data formats and technologies are envisaged for interactive and immersive 3D video applications using omni-directional videos, stereoscopic or multi-view videos. The "omni-directional video" set-up refers to 360-degree view from one single viewpoint or spherical video. Stereoscopic video is composed of two-view videos, the right and left images of the scene which, when combined, can recreate the depth aspect of the scene. A multi-view video refers to multiple video sequences captured by multiple video cameras and possibly by depth cameras. Associated with a view synthesis method, a multi-view video allows the generation of virtual views of the scene from any viewpoint. This property can be used in a large diversity of applications, including Three-Dimensional TV (3DTV), and Free Viewpoint Video (FVV). In parallel, the advent of a variety of heterogeneous delivery infrastructures has given momentum to extensive work on optimizing the end-to-end delivery QoS (Quality of Service). This encompasses compression capability but also capability for adapting the compressed streams to varying network conditions. The scalability of the video content compressed representation and its robustness to transmission impairments are thus important features for seamless adaptation to varying network conditions and to terminal capabilities.
4.3 Networked visual applications
Free-viewpoint Television (FTV) is a system for watching videos in which the user can choose its viewpoint freely and change it at anytime. To allow this navigation, many views are proposed and the user can navigate from one to the other. The goal of FTV is to propose an immersive sensation without the disadvantage of Three-dimensional television (3DTV). With FTV, a look-around effect is produced without any visual fatigue since the displayed images remain 2D. However, technical characteristics of FTV are large databases, huge numbers of users, and requests of subsets of the data, while the subset can be randomly chosen by the viewer. This requires the design of coding algorithms allowing such a random access to the pre-encoded and stored data which would preserve the compression performance of predictive coding. This research also finds applications in the context of Internet of Things in which the problem arises of optimally selecting both the number and the position of reference sensors and of compressing the captured data to be shared among a high number of users.
Broadband fixed and mobile access networks with different radio access technologies have enabled not only IPTV and Internet TV but also the emergence of mobile TV and mobile devices with internet capability. A major challenge for next internet TV or internet video remains to be able to deliver the increasing variety of media (including more and more bandwidth demanding media) with a sufficient end-to-end QoS (Quality of Service) and QoE (Quality of Experience).
4.4 Editing, post-production and computational photography
Editing and post-production are critical aspects in the audio-visual production process. Increased ways of “consuming” visual content also highlight the need for content repurposing as well as for higher interaction and editing capabilities. Content repurposing encompasses format conversion (retargeting), content summarization, and content editing. This processing requires powerful methods for extracting condensed video representations as well as powerful inpainting techniques. By providing advanced models, advanced video processing and image analysis tools, more visual effects, with more realism become possible. Our activies around light field imaging also find applications in computational photography which refers to the capability of creating photographic functionalities beyond what is possible with traditional cameras and processing tools.
5 Highlights of the year
5.1 Awards
- C. Guillemot has be award an ANR Chair on Artificial Intelligence forthe project DeepCIM (Deep learning for computational imaging with emerging image modalities), Sept. 2020- Août 2023.
- Together with Tampere UNiv., MidSweden Univ. and TTechnical Univ. Berlin, the SIROCCO team has been awarded a European Joint Doctorate project, Plenoptima, building on the ERC-CLIM and financing 15 joint PhD degrees.
6 New software and platforms
6.1 New software
6.1.1 LMVS-Net
- Name: LMVS-Net: Lightweight Neural Network for Monocular View Synthesis with Occlusion Handling
- Keywords: Light fields, View synthesis, Deep learning
- Functional Description: This code implements the method described in A Lightweight Neural Network for Monocular View Synthesis with Occlusion Handling, allowing to perform monocular view synthesis in a stereo setting. From one input image, it computes a view laterally located, left-side or right-side, and with the required disparity range depending on user input. It is also able to retrieve a disparity map from the input image, as well as a confidence map to distinguish the occluded regions, as well as to evaluate the pixelwise accuracy of the prediction. The code was developed using Keras and TensorFlow.
- Publication: hal-02428602
- Contact: Simon Evain
- Participants: Simon Evain, Christine Guillemot
6.1.2 Compression of omnidirectional images on the sphere
- Keywords: Image compression, Omnidirectional image
- Functional Description: This code implements a compression scheme of omnidirectional images. The approach operates directly on the sphere, without the need to project the data on a 2D image. More specifically, from the sphere pixelization, called healpix, the code implements the partition of the set of pixels into blocks, a block scanning order, an intra prediction between blocks, and a Graph Fourier Transform for each block residual. Finally, the image to be displayed in the viewport is generated.
- Authors: Navid Mahmoudian Bidgoli, Thomas Maugey, Aline Roumy
- Contacts: Navid Mahmoudian Bidgoli, Thomas Maugey, Aline Roumy
6.1.3 Interactive compression for omnidirectional images and texture maps of 3D models
- Keywords: Image compression, Random access
-
Functional Description:
This code implements a new image compression algorithm that allows to navigate within a static scene. To do so, the code provides access in the compressed domain to any block and therefore allows extraction of any subpart of the image. This codec implements this interactive compression for two image modalities: omnidirectional images and texture maps of 3D models. For omnidirectional images the input is a 2D equirectangular projection of the 360 image. The output is the image seen in the viewport. For 3D models, the input is a texture map and the 3D mesh. The output is also the image seen in the viewport.
The code consists of three parts: (A) an offline encoder (B) an online bit extractor and (C) a decoder. The offline encoder (i) partitions the image into blocks, (ii) optimizes the positions of the access blocks, (iii) computes a set of geometry aware predictions for each block (to cover all possible navigation paths), (iv) implements transform quantization for all blocks and their predictions, and finally (v) evaluates the encoding rates. The online bit extractor (Part B) first computes the optimal and geometry aware scanning order. Then it extracts in the bitstream, the sufficient amount of information to allow the decoding of the requested blocks. The last part of the code is the decoder (Part C). The decoder reconstructs the same scanning order as the one computed at the online bit extractor. Then, the blocks are decoded (inverse transform, geometry aware predictions, ...) and reconstructed. Finally the image in the viewport is generated.
- Authors: Navid Mahmoudian Bidgoli, Thomas Maugey, Aline Roumy
- Contacts: Navid Mahmoudian Bidgoli, Thomas Maugey, Aline Roumy
6.1.4 Monocular-LF-VS
- Name: Neural Network with Adversarial Loss for Light Field Synthesis from a Single Image
- Keywords: Light fields, Deep learning, View synthesis
- Functional Description: The code implement a lightweight neural network architecture with an adversarial loss for generating a fulllight field from one single image. The method is able to estimate disparity maps and automatically identify occluded regions from one single image thanks to a disparity confidence map based on forward-backward consistency checks. The disparity confidence map also controls the use of an adversarial loss for occlusion handling. The approach outperforms reference methods when trained and tested on light field data. Besides,we also designed the method so that it can efficiently generate a full light field from one single image, even when trained only on stereo data. This allows us to generalize our approach for view synthesis to more diversedata and semantics.
-
URL:
http://
clim. inria. fr/ DataSoftware. html - Publication: hal-03024210
- Contact: Simon Evain
- Participants: Simon Evain, Christine Guillemot
6.1.5 FDL-Compress
- Name: FDL-Compress: Light Field Compression using Fourier Disparity Layers
- Keywords: Light fields, Compression, Sparse decomposition
- Functional Description: This code implements a compression method for light fields based on the Fourier Disparity Layer representation. This light field representation consists in a set of layers that can be efficiently constructed in the Fourier domain from a sparse set of views, and then used to reconstruct intermediate viewpoints without requiring a disparity map. In the proposed compression scheme, a subset oflight field views is encoded first and used to construct a Fourier Disparity Layer model from which a second subset of views is predicted. After encoding and decoding the residual of those pre-dicted views, a larger set of decoded views is available, allowing us to refine the layer model in order to predict the next views with increased accuracy. The procedure is repeated until the complete set of light field views is encoded.
-
URL:
http://
clim. inria. fr/ DataSoftware. html - Publication: hal-02130487
- Contact: Christine Guillemot
- Participants: Elian Dib, Mikael Le Pendu, Christine Guillemot
6.1.6 LF-Slomo
- Name: LF-Slomo: LF video temporal interpolation method
- Keywords: Light fields, Learning, Temporal interpolation
- Functional Description: This code implements a method for temporal interpolation of sparsely sampled video light fields using dense scene flows. Given light fields at two time instants, the goal is to interpolate an intermediate light field to form a spatially, angularly and temporally coherent light field video sequence. We first compute angularly coherent bidirectional scene flows (4D-SFE method) between the two input light fields. We then use the optical flows and the two light fields as inputs to a convolutional neural network that synthesizes independently the views of the light field at an intermediate time.
-
URL:
http://
clim. inria. fr/ DataSoftware. html - Publication: hal-02924662v1
- Contact: Christine Guillemot
- Participants: Pierre David, Mikael Le Pendu, Christine Guillemot
6.1.7 LFVS
- Name: LFVS: Light Field View Synthesis
- Keywords: Light fields, View synthesis, Deep learning
- Functional Description: The code implements a learning-based framework for light field view synthesis from a subset of input views. Building upon a light-weight optical flow estimation network toobtain depth maps, our method employs two reconstruction modules in pixel and feature domains respectively. For the pixel-wise reconstruction, occlusions are explicitly handled by a disparity-dependent interpolation filter, whereas in-painting on disoccluded areas is learned by convolutional layers. Due to disparity inconsistencies, the pixel-based reconstruction may lead to blurriness in highly textured areasas well as on object contours. On the contrary, the feature-based reconstruction well performs on high frequencies,making the reconstruction in the two domains complementary. End-to-end learning is finally performed including a fusion module merging pixel and feature-based reconstruc-tions.
-
URL:
http://
clim. inria. fr/ DataSoftware. html - Publication: hal-02507722
- Contact: Christine Guillemot
- Participants: Jinglei Shi, Xiaoran Jiang, Christine Guillemot
6.1.8 LFDE-FLEX
- Name: LFDE-FLEX: A Framework for Learning Based Depth from a Flexible Subset of Dense and Sparse Light Field Views
- Keywords: Light fields, Depth estimation, Deep learning
- Functional Description: The code implements a learning based depth estimation framework suitable for both densely and sparsely sampled light fields. The proposed framework consists of three processing steps: initial depth estimation, fusion with occlusion handling, and refinement. The estimation can be performed from a flexible subset of input views. The fusion of initial disparity estimates, relying on two warping error measures, allows us to have an accurate estimation in occluded regions and along the contours. In contrast with methods relying on the computation of cost volumes, the proposed approach does not need any prior information on the disparity range.
- Publication: hal-02155040
- Contact: Jinglei Shi
- Participants: Jinglei Shi, Xiaoran Jiang, Christine Guillemot
6.1.9 Rate-distortion optimized motion estimation for on the sphere compression of 360 videos
- Keywords: Image compression, Omnidirectional video
-
Functional Description:
This code implements a new video compression algorithm for omnidirectional (360°) videos. The main originality of this algorithm is that the compression is performed directly on the sphere. First, it saves computational complexity as it avoids to project the sphere onto a 2D map, as classically done. Second, and more importantly, it allows to achieve a better rate-distortion tradeoff, since neither the visual data nor its domain are distorted. This code implements an extension from still images to videos of the on-the-sphere compression for omnidirectional still images. In particular, it implements a novel rate-distortion optimized motion estimation algorithm to perform motion compensation. The optimization is performed among a set of existing motion models and a novel motion model called tangent-linear+t. Moreover, a finer search pattern, called spherical-uniform, is also implemented for the motion parameters, which leads to a more accurate block prediction. The novel algorithm leads to rate-distortion gains compared to methods based on a unique motion model.
More precisely, this algorithm performs inter-prediction and contains (i) a motion estimation, (ii) a motion compensation and computation of a residue, (iii) the encoding of this residue, (iv) estimation of rate and distortion. Several visualization tools are also provided such as rate-distortion curves, but also search pattern centers, and motion field.
- Authors: Alban Marie, Navid Mahmoudian Bidgoli, Thomas Maugey, Aline Roumy
- Contacts: Navid Mahmoudian Bidgoli, Thomas Maugey, Aline Roumy
6.1.10 4D-SFE
- Name: 4D-SFE: 4D Scene Flow Estimator from Light Fields
- Keywords: Light fields, Scene Flow, Motion analysis, Depth estimation
- Functional Description: This software implements a method for scene flow estimation from light fields by computing an optical flow, a disparity map and a disparity variation map for the whole light field. It takes as inputs two consecutive frames from a light field video, as well as optical flow and disparity maps estimated for each view of the light field (e.g. with a deep model like PWC-Net) and saved as .flo files. First the light field is divided into 4D clusters called superrays, then a neighboring weighted graph is built between the different clusters and finally a 4D affine model is fitted for every cluster, using the initial estimations from the optical flow and disparity estimations that are contained in the cluster and in the neighboring clusters.
- Publication: hal-02506355
- Contact: Christine Guillemot
- Participants: Pierre David, Christine Guillemot, Mikael Le Pendu
6.2 New platforms
6.2.1 Acquisition of multi-view sequences for Free viewpoint Television
Participants: Laurent Guillo, Thomas Maugey.
The scientific and industrial community is nowadays exploring new multimedia applications using 3D data (beyond stereoscopy). In particular, Free Viewpoint Television (FTV) has attracted much attention in the recent years. In those systems, user can choose in real time its view angle from which he wants to observe the scene. Despite the great interest for FTV, the lack of realistic and ambitious datasets penalizes the research effort. The acquisition of such sequences is very costly in terms of hardware and working effort, which explains why no multi-view videos suitable for FTV has been proposed yet.
In the context of the project ADT ATeP 2016-2018 (funded by Inria), such datasets were acquired and some calibration tools have been developed. First 40 omnidirectional cameras and their associated equipments have been acquired by the team (thanks to Rennes Metropole funding). We have first focused on the calibration of this camera, i.e., the development of the relationship between a 3D point and its projection in the omnidirectional image. In particular, we have shown that the unified spherical model fits the acquired omnidirectional cameras. Second, we have developed tools to calibrate the cameras in relation to each other. Finally, we have made a capture of 3 multiview sequences that have been made available to the community via a public web site.
6.2.2 CLIM processing toolbox
Participants: Pierre Allain, Christine Guillemot, Laurent Guillo.
As part of the ERC Clim project, the EPI Sirocco is developing a light field processing toolbox. The toolbox and libraries are developed in C++ and the graphical user interface relies on Qt. As input data, this tool accepts both sparse light fields acquired with High Density Camera Arrays (HDCA) and denser light fields captured with plenoptic cameras using microlens arrays (MLA). At the time of writing, in addition to some simple functionalities, such as re-focusing, change of viewpoints, with different forms of visualization, the toolbox integrates more advanced tools for scene depth estimation from sparse and dense light fields, for super-ray segmentation and scene flow estimation, and for light field denoising and angular interpolation using anisotropic diffusion in the 4D ray space. The toolbox is now being interfaced with the C/C++ API of the tensorflow platform, in order to execute deep models developed in the team for scene depth and scene flow estimation, view synthesis, and axial super-resolution.
6.2.3 ADT: Interactive Coder of Omnidirectional Videos
Participants: Sebastien Bellenous, Navid Mahmoudian Bidgoli, Thomas Maugey.
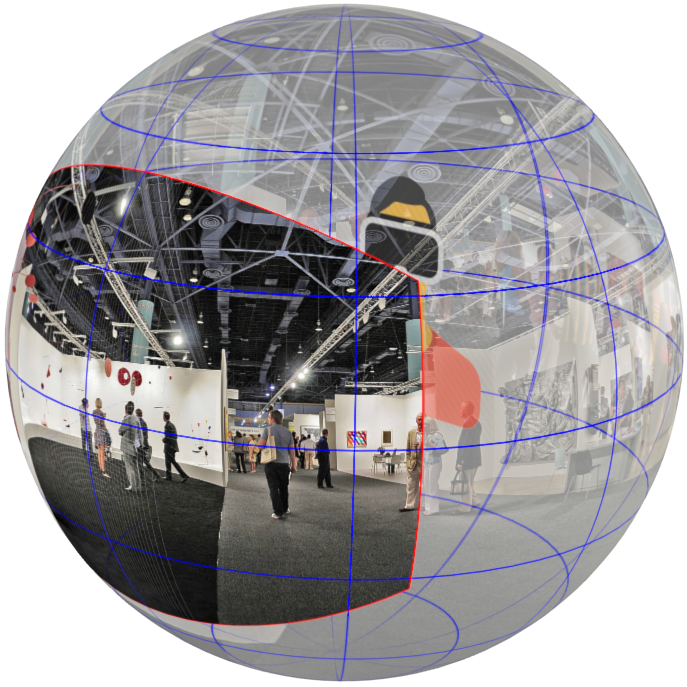
In the Intercom project, we have studied the impact of interactivity on the coding performance. We have, for example, tackled the following problem: is it possible to compress a 360
7 New results
7.1 Visual Data Analysis
Keywords: Scene depth, Scene flows, 3D modeling, Light-fields, camera design, 3D point clouds.
7.1.1 Single sensor light field acquisition using coded masks
Participants: Christine Guillemot, Guillaume Le Guludec, Ehsan Miandji, Hoai Nam Nguyen.
We designed novel light field acquisition solutions based on coded masks placed in a compressive sensing framework. We first developed a variational approach for reconstructing color light fields in the compressed sensing framework with very low sampling ratio, using both coded masks and color filter arrays (CFA) 21. A coded mask is placed in front of the camera sensor to optically modulate incoming rays, while a color filter array is assumed to be implemented at the sensor level to compress color information. Hence, the light field coded projections, operated by a combination of the coded mask and the CFA, measure incomplete color samples with a three times lower sampling ratio than reference methods that assume full color (channel-by-channel) acquisition. We then derived adaptive algorithms to directly reconstruct the light field from raw sensor measurements by minimizing a convex energy composed of two terms. The first one is the data fidelity term which takes into account the use of CFAs in the imaging model, and the second one is a regularization term which favors the sparse representation of light fields in a specific transform domain. Experimental results show that the proposed approach produces a better reconstruction both in terms of visual quality and quantitative performance when compared to reference reconstruction methods that implicitly assume prior color interpolation of coded projections.
We then developed a unifying image formation model that abstracts the architecture of most existing compressive-sensing light-field cameras, equipped with single lens and coded masks, as an equivalent multi-mask camera 15. It allows to compare different designs with a number of criteria: compression rate, light efficiency, measurement incoherence, as well as acquisition quality. Moreover, the underlying multi-mask camera can be flexibly adapted for various applications, such as single and multiple acquisitions, spatial super-resolution, parallax reconstruction, and color restoration. We also derived a generic variational algorithm solving all these concrete problems by considering appropriate sampling operators. We have also designed solutions for light field video cameras 9. We have pursued this study by designing deep learning architectures for light field reconstruction from a compressed set of measurements, and for optilizing the color coded mask distribution.
7.1.2 Deep Light Field Acquisition Using Learned Coded Mask Distributions for Color Filter Array Sensors
Participants: Christine Guillemot, Guillaume Le Guludec, Ehsan Miandji.
Compressive light field photography enables light field acquisition using a single sensor by utilizing a color coded mask. This approach is very cost effective since consumer-level digital cameras can be turned into a light field camera by simply placing a coded mask between the sensor and the aperture plane and solving an inverse problem to obtain an estimate of the original light field. We have developed a deep learning architecture for compressive light field acquisition using a color coded mask and a sensor with Color Filter Array (CFA). Unlike previous methods where a fixed mask pattern is used, our deep network learns the optimal distribution of the color coded mask pixels. The proposed solution enables end-to-end learning of the color-coded mask distribution and the reconstruction network, taking into account the sensor CFA. Consequently, the resulting network can efficiently perform joint demosaicing and light field reconstruction of images acquired with color-coded mask and a CFA sensor. Compared to previous methods based on deep learning with monochrome sensors, as well as traditional compressive sensing approaches using CFA sensors, we obtain superior color reconstruction of the light fields.7.1.3 Compressive HDR Light Field Camera with Multiple ISO Sensors
Participants: Christine Guillemot, Ehsan Miandji, Hoai Nam Nguyen.
We have proposed a new design for compressive HDR light field cameras, combining multiple ISO photography with coded mask acquisition, placed in a compressive sensing framework. The proposed camera model is based on a main lens, a multi-ISO sensor and a coded mask located in the optical path between the main lens and the sensor (close to the sensor) that projects the spatio-angular information of the light field onto the 2D sensor. The model encompasses different acquisition scenarios with different ISO patterns and gains. In the experiments, we assume that the sensor has an in-built color filter array (CFA), even if the model can enable other types of sensors (e.g. monochrome). We developed a dictionary-based algorithm to jointly perform color demosaicing, light field angular information reconstruction and HDR samples recovery from the multiple ISO measurements. We also created two HDR light field data sets: one synthetic dataset created using blender and a real light field dataset created from low dynamic range (LDR) captures at multiple exposures. Experimental results show that, with a high compression rate, the proposed method yields a higher light field reconstruction quality compared with a multiple LDR captures, and compared with the fusion of light fields captured at different ISOs.
7.1.4 Scene flow estimation from light fields
Participants: Pierre David, Christine Guillemot, Mikael Le Pendu.
Scene flows can be seen as 3D extensions of optical flows by also giving the variation in depth along time in addition to the optical flow. Scene flows are tools needed for temporal processing of light fields. Estimating dense scene flows in light fields poses obvious problems of complexity due to the very large number of rays or pixels. This is even more difficult when the light field is sparse, i.e., with large disparities, due to the problem of occlusions.
We have developed a local 4D affine model to represent scene flows, taking into account light field epipolar geometry. The model parameters are estimated per cluster in the 4D ray space. This model led to the development of a dense scene flow estimation method from light fields 6. The local 4D affine parameters are in this case derived by fitting the model on initial motion and disparity estimates obtained by using 2D dense optical flow estimation techniques. We have shown that the model is very effective for estimating scene flows from 2D optical flows (see Fig.3). The model regularizes the optical flows and disparity maps, and interpolates disparity variation values in occluded regions. The proposed model allows us to benefit from deep learning-based 2D optical flow estimation methods while ensuring scene flow geometry consistency in the 4 dimensions of the light field 24.
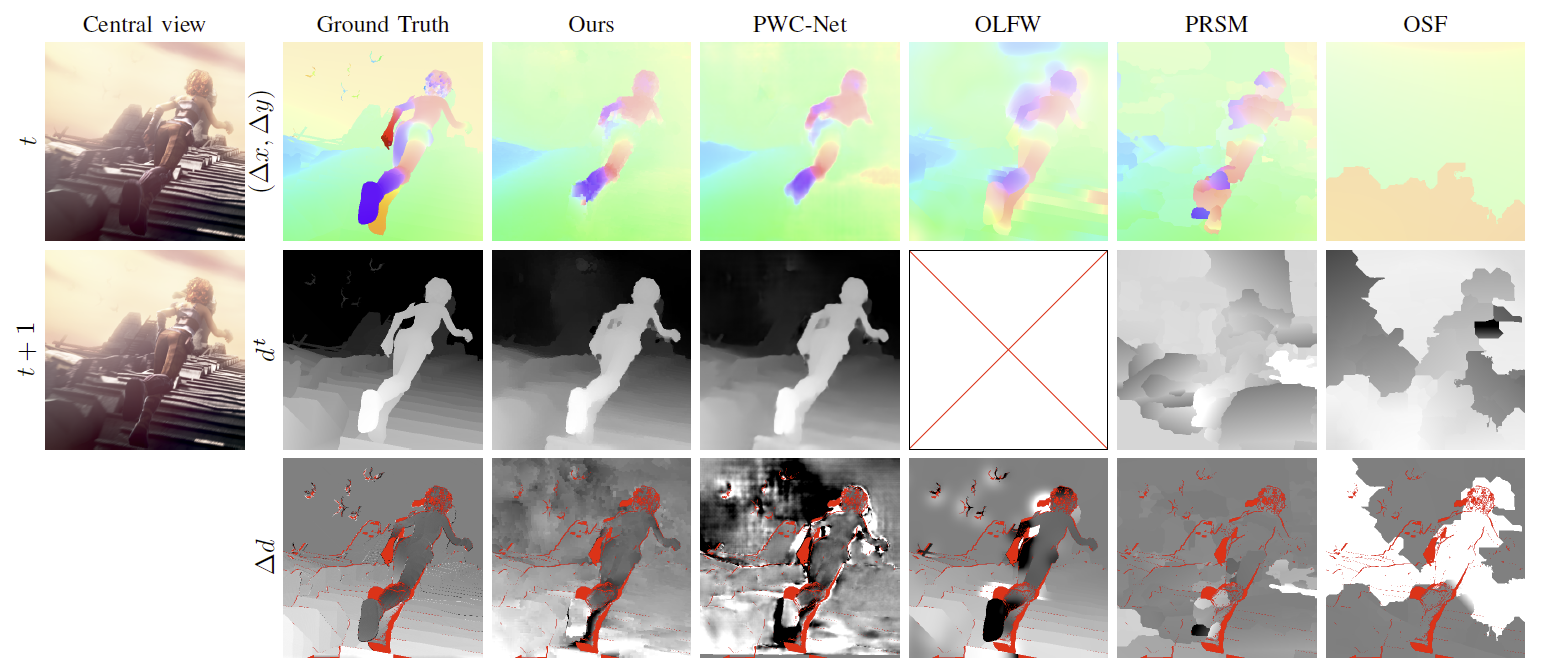
7.1.5 Depth estimation at the decoder in the MPEG-I standard
Participants: Patrick Garus, Christine Guillemot, Thomas Maugey.
Immersive video coding enables depth image-based
rendering at the client side while minimizing bitrate, pixel rate
and complexity. In collaboration with Orange labs, we investigate whether the classical approach of
sending the geometry of a scene as depth maps is appropriate
to serve this purpose. Previous work shows that bypassing depth
transmission entirely and estimating depths at the client side
improves the synthesis performance while saving bitrate and
pixel rate. In order to understand if the encoder side depth
maps contain information that is beneficial to be transmitted,
we have explored a hybrid approach which enables partial depth
map transmission using an RD-based decision in the depth
coding process. For each coding block, depth maps can either
be transmitted or derived at the decoder side, thus providing
more control of the quality of rendered views. This approach
reveals that partial depth map transmission may improve the
rendering performance but does not present a good compromise
in terms of compression efficiency. Building on this conclusion,
we have addressed the remaining drawbacks of decoder side depth
estimation: complexity and depth map inaccuracy. We propose a
novel system that takes advantage of high quality depth maps at
the server side by encoding them into lightweight features that
support the depth estimator at the client side. These features
allow the reduction of the amount of data that has to be handled
during decoder side depth estimation by 87
7.1.6 Spherical feature extraction for 360 light field reconstruction from omni-directional fish-eye camera captures
Participants: Christine Guillemot, Fatma Hawary, Thomas Maugey.
With the increasing interest in wide-angle or 360° scene captures, the extraction of descriptors well suited to the geometry of this content is a key problem for a variety of processing tasks. Algorithms designed for feature extraction in 2D images are hardly applicable to 360
In a work published in 19, we have proposed to adapt the spherical model to the geometry of the 360
7.2 Signal processing and learning methods for visual data representation and compression
Keywords: Sparse representation, data dimensionality reduction, compression, scalability, rate-distortion theory.
7.2.1 A non-parametric sparse BRDF model
Participants: Christine Guillemot, Ehsan Miandji.
Accurate modeling of bidirectional reflectance distribution functions (BRDFs) is a key component in photo-realistic and physically-based rendering. This paper presents a novel non-parametric BRDF model based on a dictionary-learning approach, in which a set of basis functions (or atoms) is trained to adapt to the space of BRDFs The proposed model is capable of accurately representing a wide range of different materials. The basis functions are optimized to enable a high degree of sparsity in the BRDF representation, i.e. most of the coefficients can be nullified while keeping the reconstruction error at minimum. We evaluate our method on the MERL, DTU and RGL-EPFL BRDF databases. Experimental results show that our approach results in
Our |
Our |
Bagher et al. | reference |
![]() |
![]() |
![]() |
![]() |
46.1956 dB | 37.4564 dB | 29.7606 dB |
7.2.2 3D point cloud processing and plenoptic point cloud compression
Participants: Christine Guillemot, Maja Krivokuca.
We have been developing a new method for the efficient representation and compression of colours in plenoptic point clouds. A plenoptic point cloud is essentially a Surface Light Field representation on a 3D point cloud, such that for each spatial point (x, y, z) that defines the point cloud's shape, there are multiple colour values associated with this point, which represent the colour of that point as seen from different viewpoints in 3D space 20 (see Fig.4 for a visual illustration of a plenoptic point cloud). The few existing algorithms for plenoptic colour compression rely on the availability of a dense distribution of viewpoints arranged in a regular, predictable manner around the 3D object represented by the point cloud. However, in real-life capture scenarios, this assumption is not realistic. Real-life captures are more likely to result in a sparse sampling of viewpoints, which may not be distributed in a regular manner around the 3D object. Moreover, at each viewpoint, only certain spatial (x, y, z) points will be visible; the remaining spatial points will be occluded. Therefore, the valid viewpoints are also likely to be in different locations (angular positions) for different spatial points. For these reasons, we have been working on a new representation and compression method for plenoptic point cloud colours, which can work with both: the irregular, sparse viewpoints that are more likely in real-life captures; and the regular, dense viewpoints that are more typical of computer-generated Surface Light Field data. Results are showing that our compression algorithm may also be able to provide a notable improvement in rate-distortion performance over the current state-of-the-art point cloud colour compression methods.
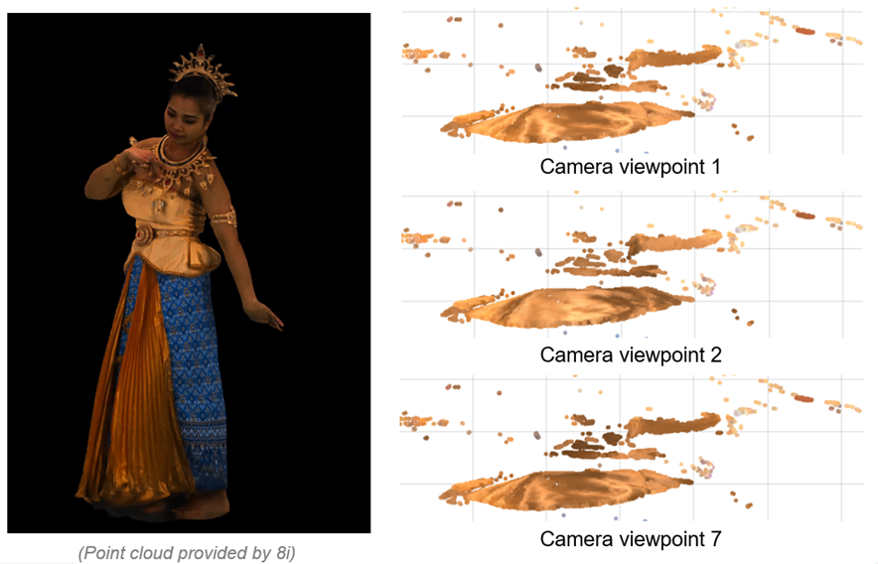
7.2.3 Low-rank models and representations for light fields
Participants: Elian Dib, Christine Guillemot, Xiaoran Jiang, Mikael Le Pendu.
We have introduced a local low-rank approximation method using a parameteric disparity model 7. The local support of the approximation is defined by super-rays. Superrays can be seen as a set of super-pixels that are coherent across all light field views. The light field low-rank assumption depends on how much the views are correlated, i.e. on how well they can be aligned by disparity compensation. We have therefore introduced a disparity estimation method using a low-rank prior. We have considered a parametric model describing the local variations of disparity within each super-ray, and alternatively search for the best parameters of the disparity model and of the low-rank approximation. We have assessed the proposed disparity parametric model, by considering an affine disparity model. We have shown that using the proposed disparity parametric model and estimation algorithm gives an alignment of superpixels across views that favours the low-rank approximation compared with using disparity estimated with classical computer vision methods. The low-rank matrix approximation is then computed on the disparity compensated super-rays using a singular value decomposition (SVD). A coding algorithm has been developed for the different components of the proposed disparity-compensated low-rank approximation 7.
7.2.4 Graph coarsening and dimensionality reduction for graph transforms of reduced complexity
Participants: Christine Guillemot, Thomas Maugey, Mira Rizkallah.
Graph-based transforms are powerful tools for signal representation and energy compaction. However, their use for high dimensional signals such as light fields poses obvious problems of complexity. To overcome this difficulty, one can consider local graph transforms defined on supports of limited dimension, which may however not allow us to fully exploit long-term signal correlation. We have developed methods to optimize local graph supports in a rate distortion sense for efficient light field compression. A large graph support can be well adapted for compression efficiency, however at the expense of high complexity. In this case, we use graph reduction techniques to make the graph transform feasible. We also consider spectral clustering to reduce the dimension of the graph supports while controlling both rate and complexity (see Fig.5 for an example of segmentation resulting from spectral clustering). We derived the distortion and rate models which are then used to guide the graph optimization. We developped a complete light field coding scheme based on the proposed graph optimization tools. Experimental results show rate-distortion performance gains compared to the use of fixed graph support. The method also provides competitive results when compared against HEVC-based and the JPEG Pleno light field coding schemes. WE also assess the method against a homography-based low rank approximation and a Fourier disparity layer based coding method.
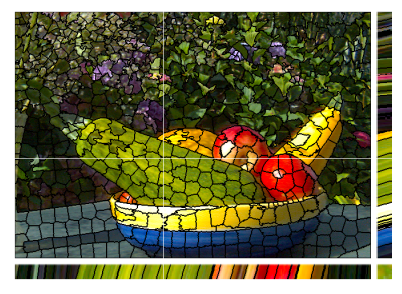
7.2.5 Quantization-aware and attention-based deep decoder for image and light field compression
Participants: Christine Guillemot, Xiaoran Jiang.
We present an image compression solution using a simple feed-forward neural network that can generate images from randomly chosen and fixed noise. The network is composed of several elementary blocks, each block containing a sequence of different operations: one convolutional layer, one upsampling layer with scale factor 2, one non-linear activation layer (rectified linear units) ReLU6, one batch normalization layer and finally a lightweight attention module which is used to better exploit cross-channel and spatial meaningful features. Quantization-aware learning is applied, and the quantified network parameters are then transmitted after applying entropy coding. The fact that the network is highly under parameterized (the number of weight parameters is much smaller than the number of pixels) makes the model a good candidate for image compression. We have observed that our method can outperform the HEVC standard and be competitive against other state-of-the-art deep learning-based compression methods. The scheme is now being extended to light field compression.
7.3 Algorithms for inverse problems in visual data processing
Keywords: Inpainting, view synthesis, super-resolution.
7.3.1 View synthesis in light fields and stereo set-ups
Participants: Simon Evain, Christine Guillemot, Xiaoran Jiang, Jinglei Shi.
We have developed a learning-based framework for light field view synthesis from a subset of input views 23. Building upon a light-weight optical flow estimation network to obtain depth maps, our method employs two reconstruction modules in pixel and feature domains respectively. For the pixel-wise reconstruction, occlusions are explicitly handled by a disparity-dependent interpolation filter, whereas inpainting on disoccluded areas is learned by convolutional layers. Due to disparity inconsistencies, the pixel-based reconstruction may lead to blurriness in highly textured areas as well as on object contours. On the contrary, the feature-based reconstruction performs well on high frequencies, making the reconstruction in the two domains complementary. End-to-end learning is finally performed including a fusion module merging pixel and feature-based reconstructions. Experimental results show that our method achieves state-of-the-art performance on both synthetic and real-world datasets, moreover, it is even able to extend light fields baseline by extrapolating high quality views without additional training.
We have also designed s lightweight neural network architecture with an adversarial loss for generating a fulllight field from one single image 18. The method is able to estimate disparity maps and automatically identifyoccluded regions from one single image thanks to a disparity confidence map based on forward-backwardconsistency checks. The disparity confidence map also controls the use of an adversarial loss for occlusionhandling. The approach outperforms reference methods when trained and tested on light field data. Besides,we also designed the method so that it can efficiently generate a full light field from one single image, evenwhen trained only on stereo data. This allows us to generalize our approach for view synthesis to more diversedata and semantics.
7.3.2 Light field video temporal interpolation
Participants: Pierre David, Christine Guillemot, Mikael Le Pendu.
We have addressed the problem of temporal interpola-tion of sparsely sampled video light fields using dense sceneflows 17. Given light fields at two time instants, the goal is to in-terpolate an intermediate light field to form a spatially, angu-larly and temporally coherent light field video sequence. Wefirst compute angularly coherent bidirectional scene flows be-tween the two input light fields. We then use the optical flowsand the two light fields as inputs to a convolutional neuralnetwork that synthesizes independently the views of the lightfield at an intermediate time. In order to measure the angularconsistency of a light field, we propose a new metric based onepipolar geometry. Experimental results show that the pro-posed method produces light fields that are angularly coher-ent while keeping similar temporal and spatial consistency asstate-of-the-art video frame interpolation methods
7.3.3 Neural networks for inverse problems in 2D imaging
Participants: Christine Guillemot, Aline Roumy, Alexander Sagel.
The Deep Image Prior has been recently introduced to solve inverse problems in image processing with no need for training data other than the image itself. However, the original training algorithm of the Deep Image Prior constrains the reconstructed image to be on a manifold described by a convolutional neural network. For some problems, this neglects prior knowledge and can render certain regularizers ineffective. We have developed an alternative approach that relaxes this constraint and fully exploits all prior knowledge 22. We have evaluated our algorithm on the problem of reconstructing a high-resolution image from a downsampled version and observed a significant improvement over the original Deep Image Prior algorithm.7.4 Distributed coding for interactive communication
Keywords: Information theory, interactive communication, model selection, robust detection, Slepian-Wolf coding, Wyner-Ziv coding.
7.4.1 Random Access in standardized video compression schemes: the random access versus compression efficiency tradeoff and the optimal reference positioning problem
Participants: Navid Mahmoudian Bidgoli, Thomas Maugey, Aline Roumy.
Data acquired over long periods of time like High Definition (HD) videos or records from a sensor over long time intervals, have to be efficiently compressed, to reduce their size. The compression has also to allow efficient access to random parts of the data upon request from the users. Efficient compression is usually achieved with prediction between data points at successive time instants. However, this creates dependencies between the compressed representations, which is contrary to the idea of random access. Prediction methods rely in particular on reference data points, used to predict other data points, and the placement of these references balances compression efficiency and random access. Existing solutions to position the references use ad hoc methods. In 16, we study this joint problem of compression efficiency and random access. We introduce the storage cost as a measure of the compression efficiency and the transmission cost for the random access ability. We show that the reference placement problem that trades off storage with transmission cost is an integer linear programming problem, that can be solved by standard optimizer. Moreover, we show that the classical periodic placement of the references is optimal, when the encoding costs of each data point are equal and when requests of successive data points are made. In this particular case, a closed form expression of the optimal period is derived. Finally, the optimal proposed placement strategy is compared with an ad hoc method, where the references correspond to sources where the prediction does not help reducing significantly the encoding cost. The optimal proposed algorithm shows a bit saving of -20% with respect to the ad hoc method.
7.4.2 Interactive compression of omnidirectional images: a complete new and optimal framework to solve the random access versus compression efficiency tradeoff problem
Participants: Navid Mahmoudian Bidgoli, Thomas Maugey, Aline Roumy.
The random access versus compression efficiency tradeoff is the core of interactive compression. In the preceding paragraph, the problem is solved while keeping standardized predictive coding schemes. In 12 instead, we propose a new and optimal interactive compression scheme for omnidirectional images. This requires two characteristics: efficient compression of data, to lower the storage cost, and random access ability to extract part of the compressed stream requested by the user (for reducing the transmission rate). In standardized compression schemes, data is predicted by a series of references that have been pre-defined and compressed. There references are the only access points in the data. This contrasts with the spirit of random accessibility. We propose a solution for this problem based on incremental codes implemented by rate-adaptive channel codes. This scheme encodes the image while adapting to any user request and leads to an efficient coding that is flexible in extracting data depending on the available information at the decoder. Therefore, only the information that is needed to be displayed at the user’s side is transmitted during the user’s request, as if the request was already known at the encoder. The experimental results demonstrate that our coder obtains a better transmission rate than the state-of-the-art tile-based methods at a small cost in storage. Moreover, the transmission rate grows gradually with the size of the request and avoids a staircase effect, which shows the perfect suitability of our coder for interactive transmission. This work has been cited in the IEEE MMTC Review Letter of October 2020.
7.4.3 Model and rate selection in interactive compression: duality with the mismatched channel coding problem and conjecture
Participants: Navid Mahmoudian Bidgoli, Thomas Maugey, Aline Roumy.
Interactive compression refers to the problem of compressing data while sending only the part requested by the user. In this context, the challenge is to perform the extraction in the compressed domain directly. Theoretical results exist, but they assume that the true distribution is known. In practical scenarios instead, the distribution must be estimated. In 11, we first formulate the model selection problem for interactive compression and show that it requires to estimate the excess rate incurred by mismatched decoding. Then, we propose a new expression to evaluate the excess rate of mismatched decoding in a practical case of interest: when the decoder is the belief- propagation algorithm. We also propose a novel experimental setup to validate this closed-form formula. We show a good match for practical interactive compression schemes based on fixed-length Low-Density Parity-Check (LDPC) codes. This new formula is of great importance to perform model and rate selection.
7.4.4 Random access to a compressed database, under the constraint of massive requests
Participants: Thomas Maugey, Aline Roumy.
In 13, we study the compression problem with Massive Random Access (MRA). A set of correlated sources is encoded once for all and stored on a server while a large number of clients access various subsets of these sources. Due to the number of concurrent requests, the server is only able to extract a bitstream from the stored data: no re-encoding can be performed before the transmission of the data requested by the clients. First, we formally define the MRA framework and propose to model the constraints on the way subsets of sources may be accessed by a navigation graph. We introduce both storage and transmission costs to characterize the performance of MRA. We then propose an Incremental coding Based Extractable Compression (IBEC) scheme. We first show that this scheme is optimal in terms of achievable storage and transmission costs. Second, we propose a practical implementation of our IBEC scheme based on rate-compatible LDPC codes. Experimental results show that our IBEC scheme can almost reach the same transmission costs as in traditional point-to-point source coding schemes, while having a reasonable overhead in terms of storage cost.
8 Bilateral contracts and grants with industry
8.1 Bilateral contracts with industry
CIFRE contract with Orange labs. on compression of immersive content
Participants: Patrick Garus, Christine Guillemot, Thomas Maugey.
- Title : Compression of immersive content
- Research axis : 7.1.5
- Partners : Orange labs. (F. Henry), Inria-Rennes.
- Funding : Orange, ANRT.
- Period : Jan.2019-Dec.2021.
The goal of this Cifre contract is to develop novel compression methods for 6 DoF immersive video content. This implies investigating depth estimation and view synthesis methods that would be robust to quantization noise, for which deep learning solutions are being considered. This also implies developing the corresponding coding mode decisions based on rate-distortion criteria.
CIFRE contract with Ateme on neural networks for video compression
Participants: Christine Guillemot, Yiqun Liu, Aline Roumy.
- Title : Neural networks for video compression of reduced complexity
- Partners : Ateme (T. Guionnet, M. Abdoli), Inria-Rennes.
- Funding : Ateme, ANRT.
- Period : Aug.2020-Jul.2023.
The goal of this Cifre contract is to investigate deep learning architectures for the inference of coding modes in video compression algorithms with the ultimate goal of reducing the encoder complexity. The first step addresses the problem of Intra coding modes and quad-tree partitioning inference. The next step will consider Inter coding modes taking into account motion and temporal information.
Contract LITCHIE with Airbus on deep learning for satellite imaging
Participants: Denis Bacchus, Christine Guillemot, Arthur Lecert, Aline Roumy.
- Title : Deep learning methods for low light vision
- Partners : Airbus (R. Fraisse), Inria-Rennes.
- Funding : BPI.
- Period : Sept.2020-Aug.2023.
The goal of this contract is to investigate deep learning methods for low light vision with sattelite imaging. The SIROCCO team focuses on two complementary problems: compression of low light images and restoration under conditions of low illumination, and hazing. The problem of low light image enhancement implies handling various factors simultaneously including brightness, contrast, artifacts and noise. We investigate solutions coupling the retinex theory, assuming that observed images can be decomposed into reflectance and illumination, with machine learning methods. We address the compression problem taking into account the processing tasks considered on the ground such as the restoration task, leading to an end-to-end optimization approach.
Research collaboration contract with Mediakind on Multiple profile encoding optimization
Participants: Reda Kaafarani, Thomas Maugey, Aline Roumy.
- Title : Multiple profile encoding optimization
- Partners : Mediakind, Inria-Rennes.
- Funding : Mediakind.
- Period : Dec.2020-May.2021.
The goal of the research contract is to compress and deliver video, while adapting the quality to the available bandwidth and/or the user screen resolution. This contract is a pre-thesis contract.
9 Partnerships and cooperations
9.1 International initiatives
9.1.1 Inria international partners
Declared Inria International Partners:
The SIROCCO team is collaborating with the following international partners:
- Univ. of MidSweden, in the context of the Guest Professorship of C. Guillemot.
Informal International Partners:
The SIROCCO team is collaborating with the following international partners:
- Univ. of Linkoping, with the team of Prof. J. Unger, on HDR light field imaging with multi-ISO sensors
- EPFL, with the team of Prof. P. Frossard and his student R. Azevedo, on graph signal processing and learning on graphs
- X'ian Univ., with the team of Prof. Z. Xiao, on light field descriptors and axial super-resolution
- University College London (UCL), with Prof. L. Toni.
Informal National Partners:
The SIROCCO team is collaborating with the following national partners:
- L2S, CentraleSupelec, with Prof. Michel Kieffer,
- ETIS laboratory UMR 8051, Cergy Pontoise, with Mael Le Treust.
9.2 International research visitors
The team has had the visit of
- S. Hajisharif, Univ. of Linkoping, in the context of the ERC-CLIM project.
9.2.1 Visits of international scientists
Research Stays Abroad:
Three members of the SIROCCO team (A. Roumy, N. Mahmoudian Bidgoli, and T. Maugey) have spent 2 weeks visiting the team of P. Frossard at EPFL, in Feb. 2020.
9.3 European initiatives
9.3.1 FP7 & H2020 Projects
ERC-CLIM
Participants: Pierre David, Elian Dib, Simon Evain, Christine Guillemot, Laurent Guillo, Fatma Hawary, Xiaoran Jiang, Maja Krivokuca, Guillaume Le Guludec, Ehsan Miandji, Hoai Nam Nguyen, Mira Rizkallah, Jinglei Shi.
- Title : Computational Light field Imaging.
- Research axis : 7.1.4, 7.1.6, 7.1.1, 7.2.3, 7.2.4, 7.2.2, 7.3.1, 7.3.3
- Partners : Inria-Rennes
- Funding : European Research Council (ERC) advanced grant
- Period : Sept. 2016 - Aug. 2021.
All imaging systems, when capturing a view, record different combinations of light rays emitted by the environment. In a conventional camera, each sensor element sums all the light rays emitted by one point over the lens aperture. Light field cameras instead measure the light along each ray reaching the camera sensors and not only the sum of rays striking each point in the image. In one single exposure, they capture the geometric distribution of light passing through the lens. This process can be seen as sampling the plenoptic function that describes the intensity of the light rays interacting with the scene and received by an observer at every point in space, along any direction of gaze, for all times and every wavelength.
The recorded flow of rays (the light field) is in the form of high-dimensional data (4D or 5D for static and dynamic light fields). The 4D/5D light field yields a very rich description of the scene enabling advanced creation of novel images from a single capture, e.g. for computational photography by simulating a capture with a different focus and a different depth of field, by simulating lenses with different apertures, by creating images with different artistic intents. It also enables advanced scene analysis with depth and scene flow estimation and 3D modeling. The goal of the ERC-CLIM project is to develop algorithms for the entire static and video light fields processing chain. The planned research includes the development of:
- Acquisition methods and novel coded-mask based camera models,
- Novel low-rank or graph-based models for dimensionality reduction and compression
- Deep learning methods for scene analysis (e.g. scene depth and scene flow estimation)
- Learning methods for solving a range of inverse problems: denoising, super-resolution, axial super-resolution, view synthesis.
9.4 National initiatives
9.4.1 Project Action Exploratoire "Data Repurposing"
Participants: Anju Jose Tom, Thomas Maugey.
Lossy compression algorithms trade bits for quality, aiming at reducing as much as possible the bitrate needed to represent the original source (or set of sources), while preserving the source quality. In the exploratory action "DARE", we propose a novel paradigm of compression algorithms, aimed at minimizing the information loss perceived by the final user instead of the actual source quality loss, under compression rate constraints.
In a first work published in 14, we first introduced the concept of perceived information (PI), which reflects the information perceived by a given user experiencing a data collection, and which is evaluated as the volume spanned by the sources features in a personalized latent space. This enabled us to formalize the rate-PI optimization problem and propose an algorithm to solve this compression problem. Finally, we validate our algorithm against benchmark solutions with simulation results, showing the gain in taking into account users' preferences while also maximizing the perceived information in the feature domain.
9.4.2 IA Chair: DeepCIM- Deep learning for computational imaging with emerging image modalities
Participants: Rita Fermanian, Christine Guillemot, Brandon Lebon, Mikael Le Pendu.
The project aims at leveraging recent advances in three fields: image processing, computer vision and machine (deep) learning. It will focus on the design of models and algorithms for data dimensionality reduction and inverse problems with emerging image modalities. The first research challenge will concern the design of learning methods for data representation and dimensionality reduction. These methods encompass the learning of sparse and low rank models, of signal priors or representations in latent spaces of reduced dimensions. This also includes the learning of efficient and, if possible, lightweight architectures for data recovery from the representations of reduced dimension. Modeling joint distributions of pixels constituting a natural image is also a fundamental requirement for a variety of processing tasks. This is one of the major challenges in generative image modeling, field conquered in recent years by deep learning. Based on the above models, our goal is also to develop algorithms for solving a number of inverse problems with novel imaging modalities. Solving inverse problems to retrieve a good representation of the scene from the captured data requires prior knowledge on the structure of the image space. Deep learning based techniques designed to learn signal priors, tcan be used as regularization models.
9.4.3 Interactive Communication (INTERCOM): Massive random access to subsets of compressed correlated data
Participants: Navid Mahmoudian Bidgoli, Thomas Maugey, Aline Roumy.
- Partners : Inria-Rennes (Sirocco team and i4S team); LabSTICC, IMT Atlantique, Signal & Communications Department; External partners: L2S, CentraleSupelec, Univ. Paris Sud; EPFL, Signal Processing Laboratory (LTS4).
- Funding : Labex CominLabs.
- Period : Oct. 2016 - Dec. 2020.
This project aims to develop novel compression techniques allowing massive random access to large databases. Indeed, we consider a database that is so large that, to be stored on a single server, the data have to be compressed efficiently, meaning that the redundancy/correlation between the data have to be exploited. The dataset is then stored on a server and made available to users that may want to access only a subset of the data. Such a request for a subset of the data is indeed random, since the choice of the subset is user-dependent. Finally, massive requests are made, meaning that, upon request, the server can only perform low complexity operations (such as bit extraction but no decompression/compression). Algorithms for two emerging applications of this problem are being developed: Free-viewpoint Television (FTV) and massive requests to a database collecting data from a large-scale sensor network (such as Smart Cities).
10 Dissemination
10.1 Promoting scientific activities
10.1.1 Scientific events: organisation
Member of the organizing committees
C. Guillemot has been a member
- as keynote chair of the organizing committee of IEEE MultiMedia Signal Processing (MMSP) workshop, Tampere, Sept. 2020.
10.1.2 Scientific events: selection
Member of the conference program committees
C. Guillemot has been a member
- of the program committe of the workshop "New Trends in Image Restoration and Enhancement and challenges" held together with CVPR, June 2020.
- of the award committee of IEEE International Conference on Image Processing, Oct. 2020.
- of the program committee of the International Conference on 3D Immersion (IC3D), Dec. 2020.
A. Roumy has been a member of
- the technical program committee of the CVPR 2020 workshop on New Trends in Image Restoration and Enhancement (NTIRE),
- the technical program committee of the ECCV 2020 workshop and challenges on Advances in Image manipulation (AIM),
- the technical program committee of the IEEE International Conference on Communications (ICC) 2020, Workshop on Machine Learning in Wireless Communications (ML4COM).
10.1.3 Journal
Member of the editorial boards
- C. Guillemot is Senior Area Editor (SAE) of EEE Trans. on Image Processing.
- C. Guillemot is associate editor of the Int. Journal of Mathematical Imaging and Vision.
- Thomas Maugey is Associate Editor of the EURASIP Journal on Advanced Signal Processing
- A. Roumy is associate editor of IEEE Trans. on Image Processing.
- A. Roumy is associate editor of the Springer Annals of Telecommunications.
10.1.4 Leadership within the scientific community
- C. Guillemot is responsible of the theme « compression et protection des données images » for the realization of the encyclopedia SCIENCES of the publisher ISTE/Wiley (2019‐2021).
- A. Roumy is a member of the IEEE IVMSP technical committee.
- A. Roumy is a Local Liaison Officer for the European Association for Signal Processing (EURASIP).
- A. Roumy is a member of the Executive board of the National Research group in Image and Signal Processing (GRETSI).
10.1.5 Research administration
- C. Guillemot has been member of the jury of recrutment of an assistant Prof. at IMT-Atlantique, May 2020.
- C. Guillemot has been a member of the Inria prizes of academy of sciences, Sept. 2020.
- C. Guillemot has been member of the CRCN jury of the Rennes Bretagne Atlantique center, Apr. 2020
- C. Guillemot has been member of the jury of admission of CRCN, July 2020.
- A. Roumy is a member of Inria evaluation committee,
- A. Roumy has been a member of the Inria Delegation committee of the Rennes Bretagne Atlantique center, Febr. 2020,
- A. Roumy has been member of the CRCN/ISFP jury of the Bordeaux Sud Ouest center, June 2020,
- A. Roumy has been member of the CRCN/ISFP jury of the Nancy Grand Est center, June 2020,
- A. Roumy has been member of the CRCN/ISFP jury of the Grenoble Rhone Alpes center, June 2020.
10.2 Teaching - Supervision - Juries
10.2.1 Teaching
- Master: C. Guillemot, courses on compression sensing and inverse problems, 6 hours, Midsweden Univ., Sweden.
- Master: T. Maugey, course on 3D models in a module on advanced video, 4 hours (2018-2019), 12 hours (2019-2020), M2 SISEA, Univ. of Rennes 1, France.
- Master: T. Maugey, course on Image compression, 10 hours (2019-2020), M2 SISEA, Univ. of Rennes 1, France.
- Master: T. Maugey, course on Representation, editing and perception of digital images, 12 hours, M2 SIF, Univ. of Rennes 1, France.
- Engineering degree: A. Roumy, Sparse methods in image and signal processing, 13 hours, INSA Rennes, 5th year, Mathematical engineering, France.
- Master: A. Roumy, Foundations of smart sensing, 13.5 hours, ENSAI, Master of Science in Statistics for Smart Data, France.
- Master: A. Roumy, Information theory, 15 hours, University Rennes 1, SIF master, France.
- Master: A. Roumy, High dimensional statistical learning, 9 hours, University Rennes 1, SIF Master, France.
10.2.2 Juries
- C. Guillemot has been reviewer of the PhDs of:
- M. P. Dimopoulou, Univ. Cöte d'Azur, Dec. 2020
- R. Verhack, Technical Univ. Berlin, Apr. 2020
- S. Vagharshakyan, Tampere Univ., Feb. 2020
- A. El Rhammad, Univ. Rennes 1, Feb. 2020
- A. Alperovich, Univ. of Konstanz, Jan. 2020
11 Scientific production
11.1 Major publications
- 1 articleA Lightweight Neural Network for Monocular View Generation with Occlusion HandlingIEEE Transactions on Pattern Analysis and Machine IntelligenceDecember 2019, 1-14
- 2 articleLight Field Super-Resolution using a Low-Rank Prior and Deep Convolutional Neural NetworksIEEE Transactions on Pattern Analysis and Machine Intelligence2019, 1-15
- 3 article Light Field Compression with Homography-based Low Rank Approximation IEEE Journal of Selected Topics in Signal Processing 2017
- 4 articleA Fourier Disparity Layer representation for Light FieldsIEEE Transactions on Image ProcessingMay 2019, 5740 - 5753
- 5 articleOut-of-sample generalizations for supervised manifold learning for classificationIEEE Transactions on Image Processing253March 2016, 15
11.2 Publications of the year
International journals
- 6 articleScene Flow Estimation from Sparse Light Fields Using a Local 4D Affine ModelIEEE Transactions on Computational ImagingMarch 2020, 1-15
- 7 articleLocal low rank approximation with a parametric disparity model for light field compressionIEEE Transactions on Image Processing292020, 9641-9653
- 8 articleA Simple Framework to Leverage State-Of-The-Art Single-Image Super-Resolution Methods to Restore Light FieldsSignal Processing: Image Communication80February 2020, 1-11
- 9 articleSingle Sensor Compressive Light Field Video CameraComputer Graphics ForumMay 2020, 1-12
- 10 article Compressively Sampled Light Field Reconstruction Using Orthogonal Frequency Selection and Refinement Signal Processing: Image Communication December 2020
- 11 articleExcess rate for model selection in interactive compression using Belief-propagation decodingAnnals of Telecommunications - annales des télécommunicationsOctober 2020, 1-18
- 12 article Fine granularity access in interactive compression of 360-degree images based on rate-adaptive channel codes IEEE Transactions on Multimedia 2020
- 13 articleIncremental Coding for Extractable Compression in the Context of Massive Random AccessIEEE transactions on Signal and Information Processing over Networks6December 2020, 251-260
- 14 articleLarge Database Compression Based on Perceived InformationIEEE Signal Processing Letters27September 2020, 1735 – 1739
- 15 article Multi-Mask Camera Model for Compressed Acquisition of Light Fields IEEE Transactions on Computational Imaging 2021
- 16 articleOptimal Reference Selection for Random Access in Predictive Coding SchemesIEEE Transactions on Communications2020, 1-20
International peer-reviewed conferences
- 17 inproceedingsAngularly Consistent Light Field Video InterpolationICME 2020 - IEEE International Conference on Multimedia and ExpoProc. IEEE International Conference on Multimedia and ExpoVitual, United KingdomJuly 2020, 1-6
- 18 inproceedings A Neural Network with Adversarial Loss for Light Field Synthesis from a Single Image VISAPP 2021 - 16th International Conference on Computer Vision Theory and Applications Proc. International Conference on Computer Vision Theory and Applications (VISAPP) Vienna, Austria February 2021
- 19 inproceedingsSphere mapping for feature extraction from 360° fish-eye capturesMMSP 2020 - 22 nd IEEE International Workshop on Multimedia Signal ProcessingTempere, FinlandSeptember 2020, 1-6
- 20 inproceedings Colour compression of plenoptic point clouds using raht-klt with prior colour clustering and specular/diffuse component separation IEEE International Conference on Acoustics, Speech, and Signal Processing Proc. IEEE International Conference on Acoustics, Speech, and Signal Processing Barcelone, Spain May 2020
- 21 inproceedings Color and angular reconstruction of light fields from incomplete-color coded projections Proc. IEEE International Conference on Acoustics, Speech, and Signal Processing IEEE International Conference on Acoustics, Speech, and Signal Processing Proc. IEEE International Conference on Acoustics, Speech, and Signal Processing Barcelone, Spain May 2020
- 22 inproceedingsSUB-DIP: Optimization on a subspace with deep image prior regularization and application to superresolutionIEEE International Conference on Acoustics, Speech, and Signal ProcessingICASSP 2020 - 45th International Conference on Acoustics, Speech, and Signal ProcessingProc. IEEE International Conference on Acoustics, Speech, and Signal ProcessingBarcelone, SpainMay 2020, 1-5
- 23 inproceedingsLearning Fused Pixel and Feature-based View Reconstructions for Light FieldsProc. IEEE Conference on Computer Vision and Pattern RecognitionCVPR 2020 - IEEE Conference on Computer Vision and Pattern RecognitionProc. International Conference on Comàputer Vision and Pattern Recognition (CVPR)Seattle, United StatesJune 2020, 1-10
Doctoral dissertations and habilitation theses
- 24 thesis Scene analysis and view rendering from light fields Université de Rennes 1 June 2020