Keywords
Computer Science and Digital Science
- A3.1.1. Modeling, representation
- A3.4. Machine learning and statistics
- A5.3. Image processing and analysis
- A5.4. Computer vision
- A5.10. Robotics
- A9. Artificial intelligence
- A9.1. Knowledge
- A9.2. Machine learning
- A9.3. Signal analysis
- A9.5. Robotics
Other Research Topics and Application Domains
- B9.5.1. Computer science
- B9.5.6. Data science
1 Team members, visitors, external collaborators
Research Scientists
- Jean Ponce [Team leader, Inria, Senior Researcher, on leave from Ecole Normale Supérieure]
- Justin Carpentier [Inria, Researcher]
- Ivan Laptev [Inria, Senior Researcher, HDR]
- Jean-Paul Laumond [CNRS, HDR]
- Cordelia Schmid [Inria, Senior Researcher, from Apr 2020, HDR]
- Josef Sivic [Inria, Senior Researcher, until Jul 2020, HDR]
Post-Doctoral Fellows
- Pierre-Yves Masse [CTU Pragues]
- Vladimir Petrik [CTU Pragues]
- Makarand Tapaswi [Inria]
PhD Students
- Minttu Alakuijala [Google, CIFRE]
- Alaaeldin Ali [Facebook, CIFRE, from Aug 2020]
- Antoine Bambade [Corps des , from Sept 2020]
- Adrien Bardes [Facebook, CIFRE, from Oct 2020]
- Oumayma Bounou [Inria, from Oct 2020]
- Elliot Chane-Sane [Inria, from Sept 2020]
- Hugo Cisneros [CTU Prague, from Sept 2020]
- Yann Dubois De Mont-Marin [Inria, from Sept 2020]
- Thomas Eboli [École Normale Supérieure de Paris]
- Aamr El Kazdadi [Inria]
- Pierre-Louis Guhur [Université Paris-Saclay]
- Yana Hasson [Inria]
- Yann Labbé [École Normale Supérieure de Paris]
- Guillaume Le Moing [Inria, from Nov 2020]
- Bruno Lecouat [Inria]
- Zongmian Li [Inria]
- Antoine Miech [Inria, until Aug 2020]
- Alexander Pashevich [Inria]
- Ronan Riochet [Inria]
- Ignacio Rocco Spremolla [Inria]
- Robin Strudel [École Normale Supérieure de Paris]
- Van Huy Vo [Valeo, CIFRE]
- Antoine Yang [Inria, from Sep 2020]
- Dimitri Zhukov [Inria]
Technical Staff
- Rohan Bundiraja [Inria, Engineer, Plan IA]
- Marie Heurtevent [Inria, from Oct 2020, Pre-thèse]
- Wilson Jallet [Inria, Engineer, From Sept 2020, Pre-thèse]
- Igor Kalevatykh [Inria, Engineer, SED]
- Quentin Le Lidec [Inria, Engineer, From Sept 2020, Pre-thèse]
Interns and Apprentices
- Adrien Bardes [Inria, from Apr 2020 until Sep 2020]
- Marie Heurtevent [Inria, from Apr 2020 until Sep 2020]
- Wilson Jallet [Inria, from Apr 2020 until Aug 2020]
- Quentin Le Lidec [Inria, from Apr 2020 until Sept 2020]
- Antoine Yang [Inria, from Apr 2020 until Aug 2020]
Administrative Assistant
- Mathieu Mourey [Inria]
External Collaborators
- Mathieu Aubry [ENPC]
- Josef Sivic [CTU Prague, from A 2020]
2 Overall objectives
2.1 Statement
Object recognition —or, in a broader sense, scene understanding— is the ultimate scientific challenge of computer vision: After 40 years of research, robustly identifying the familiar objects (chair, person, pet), scene categories (beach, forest, office), and activity patterns (conversation, dance, picnic) depicted in family pictures, news segments, or feature films is still beyond the capabilities of today's vision systems. On the other hand, truly successful object recognition and scene understanding technology will have a broad impact in application domains as varied as defense, entertainment, health care, human-computer interaction, image retrieval and data mining, industrial and personal robotics, manufacturing, scientific image analysis, surveillance and security, and transportation.
Despite the limitations of today's scene understanding technology, tremendous progress has been accomplished in the past ten years, due in part to the formulation of object recognition as a statistical pattern matching problem. The emphasis is in general on the features defining the patterns and on the algorithms used to learn and recognize them, rather than on the representation of object, scene, and activity categories, or the integrated interpretation of the various scene elements. WILLOW complements this approach with an ambitious research program explicitly addressing the representational issues involved in object recognition and, more generally, scene understanding.
Concretely, our objective is to develop geometric, physical, and statistical models for all components of the image interpretation process, including illumination, materials, objects, scenes, and human activities. These models will be used to tackle fundamental scientific challenges such as three-dimensional (3D) object and scene modeling, analysis, and retrieval; human activity capture and classification; and category-level object and scene recognition. They will also support applications with high scientific, societal, and/or economic impact in domains such as quantitative image analysis in science and humanities; film post-production and special effects; and video annotation, interpretation, and retrieval. Machine learning is a key part of our effort, with a balance of practical work in support of computer vision application and methodological research aimed at developing effective algorithms and architectures.
WILLOW was created in 2007: It was recognized as an INRIA team in January 2007, and as an official project-team in June 2007. WILLOW is a joint research team between INRIA Paris, Ecole Normale Supérieure (ENS) and Centre National de la Recherche Scientifique (CNRS).
Following the 12-years cycle, in 2020 we have proposed a new Inria project-team. A new team will continue addressing the challenges in visual recognition with particular focus on weakly-supervised learning and learning multi-modal representations. The new research axis of the team will target the synergy between robotics and computer vision by learning embodied representations and sensorimotor control policies. The team will also continue its efforts advancing image restoration and enhancement. The team proposal has been reviewed and accepted at the Inria Comité des Projets meeting on December 3 2020.
3 Research program
3.1 3D object and scene modeling, analysis,and retrieval
This part of our research focuses on geometric models of specific 3D objects at the local (differential) and global levels, physical and statistical models of materials and illumination patterns, and modeling and retrieval of objects and scenes in large image collections. Our past work in these areas includes research aimed at recognizing rigid 3D objects in cluttered photographs taken from arbitrary viewpoints (Rothganger et al., 2006), segmenting video sequences into parts corresponding to rigid scene components before recognizing these in new video clips (Rothganger et al., 2007), retrieval of particular objects and buildings from images and videos (Sivic and Zisserman, 2003) and (Philbin et al., 2007), and a theoretical study of a general formalism for modeling central and non-central cameras using the formalism and terminology of classical projective geometry (Ponce, 2009 and Batog et al., 2010).
We have also developed multi-view stereopsis algorithms that have
proven remarkably effective at recovering intricate details and thin
features of compact objects and capturing the overall structure of
large-scale, cluttered scenes.
We have obtained a US patent 8,331,615
1
for the corresponding software
(PMVS, https://
Our current efforts in this area are outlined in detail in Section. 7.1.
3.2 Category-level object and scene recognition
The objective in this core part of our research is to learn and recognize quickly and accurately thousands of visual categories, including materials, objects, scenes, and broad classes of temporal events, such as patterns of human activities in picnics, conversations, etc. The current paradigm in the vision community is to model/learn one object category (read 2D aspect) at a time. If we are to achieve our goal, we have to break away from this paradigm, and develop models that account for the tremendous variability in object and scene appearance due to texture, material, viewpoint, and illumination changes within each object category, as well as the complex and evolving relationships between scene elements during the course of normal human activities.
Our current work in this area is outlined in detail in Section 7.2.
3.3 Image restoration, manipulation and enhancement
The goal of this part of our research is to develop models, and methods for image/video restoration, manipulation and enhancement. The ability to “intelligently" manipulate the content of images and video is just as essential as high-level content interpretation in many applications: This ranges from restoring old films or removing unwanted wires and rigs from new ones in post production, to cleaning up a shot of your daughter at her birthday party, which is lovely but noisy and blurry because the lights were out when she blew the candles, or editing out a tourist from your Roman holiday video. Going beyond the modest abilities of current “digital zoom" (bicubic interpolation in general) so you can close in on that birthday cake, “deblock" a football game on TV, or turn your favorite DVD into a blue-ray, is just as important.
In this context, we believe there is a new convergence between computer vision, machine learning, and signal processing. For example: The idea of exploiting self-similarities in image analysis, originally introduced in computer vision for texture synthesis applications (Efros and Leung, 1999), is the basis for non-local means (Buades et al., 2005), one of today's most successful approaches to image restoration. In turn, by combining a powerful sparse coding approach to non-local means (Dabov et al., 2007) with modern machine learning techniques for dictionary learning (Mairal et al., 2010), we have obtained denoising and demosaicking results that are the state of the art on standard benchmarks (Mairal et al., 2009).
Our current work is outlined in detail in Section 7.3.
3.4 Human activity capture and classification
From a scientific point of view, visual action understanding is a computer vision problem that until recently has received little attention outside of extremely specific contexts such as surveillance or sports. Many of the current approaches to the visual interpretation of human activities are designed for a limited range of operating conditions, such as static cameras, fixed scenes, or restricted actions. The objective of this part of our project is to attack the much more challenging problem of understanding actions and interactions in unconstrained video depicting everyday human activities such as in sitcoms, feature films, or news segments. The recent emergence of automated annotation tools for this type of video data (Everingham, Sivic, Zisserman, 2006; Laptev, Marszałek, Schmid, Rozenfeld, 2008; Duchenne, Laptev, Sivic, Bach, Ponce, 2009) means that massive amounts of labelled data for training and recognizing action models will at long last be available.
Our research agenda in this scientific domain is described below and our recent results are outlined in detail in Section 7.4.
- Weakly-supervised learning and annotation of human actions in video. We aim to leverage the huge amount of video data using readily-available annotations in the form of video scripts. Scripts, however, often provide only imprecise and incomplete information about the video. We address this problem with weakly-supervised learning techniques both at the text and image levels.
- Descriptors for video representation. Video representation has a crucial role for recognizing human actions and other components of a visual scene. Our work in this domain aims to develop generic methods for representing video data based on realistic assumptions. In particular, we develop deep learning methods and design new trainable representations for various tasks such as human action recognition, person detection, segmentation and tracking.
3.5 Learning embodied representations
Computer vision has come a long way toward understanding images and videos in terms of scene geometry, object labels, locations and poses of people or classes of human actions. This “understanding”, however, remains largely disconnected from reasoning about the physical world. For example, what will happen if removing a tablecloth from a setted table? What actions will be needed to resume an interrupted meal? We believe that a true embodied understanding of dynamic scenes from visual observations is the next major research challenge. We plan to address this challenge by developing new models and algorithms with an emphasis on the synergy between vision, learning, robotics and natural language understanding. If successful, this research direction will bring significant advances in high-impact applications such as autonomous driving, home robotics and personal visual assistance.
Learning embodied representations is planned to be a major research axis for the successor of the Willow team. Meanwhile we have already started work in this direction and report our first results in Section 7.5.
4 Application domains
4.1 Introduction
We believe that foundational modeling work should be grounded in applications. This includes (but is not restricted to) the following high-impact domains.
4.2 Quantitative image analysis in science and humanities
We plan to apply our 3D object and scene modeling and analysis technology to image-based modeling of human skeletons and artifacts in anthropology, and large-scale site indexing, modeling, and retrieval in archaeology and cultural heritage preservation. Most existing work in this domain concentrates on image-based rendering, that is, the synthesis of good-looking pictures of artifacts and digs. We plan to focus instead on quantitative applications. We are engaged in a project involving the archaeology laboratory at ENS and focusing on image-based artifact modeling and decorative pattern retrieval in Pompeii. Application of our 3D reconstruction technology is now being explored in the field of cultural heritage and archeology by the start-up Iconem, founded by Y. Ubelmann, a Willow collaborator.
4.3 Video Annotation, Interpretation, and Retrieval
Both specific and category-level object and scene recognition can be used to annotate, augment, index, and retrieve video segments in the audiovisual domain. The Video Google system developed by Sivic and Zisserman (2005) for retrieving shots containing specific objects is an early success in that area. A sample application, suggested by discussions with Institut National de l'Audiovisuel (INA) staff, is to match set photographs with actual shots in film and video archives, despite the fact that detailed timetables and/or annotations are typically not available for either medium. Automatically annotating the shots is of course also relevant for archives that may record hundreds of thousands of hours of video. Some of these applications will be pursued in our MSR-Inria project.
5 Highlights of the year
5.1 Awards
- IEEE CVPR Longuet-Higgins prize to Y. Furukawa and J. Ponce for a CVPR paper from ten years before with signicant impact on computer vision research (2020).
- Winner of the BOP 6D object pose estimation challenge at ECCV for Y. Labbé, J. Carpentier and J. Sivic.
- Royal Society Milner award, 2020 for C. Schmid.
- Winner of CVPR 2020 Video Pentathlon challenge for C. Schmid.
6 New software and platforms
6.1 New software
6.1.1 Pinocchio
- Name: Pinocchio
- Keywords: Robotics, Biomechanics, Mechanical multi-body systems
- Functional Description: Pinocchio instantiates state-of-the-art Rigid Body Algorithms for poly-articulated systems based on revisited Roy Featherstone's algorithms. In addition, Pinocchio instantiates analytical derivatives of the main Rigid-Body Algorithms like the Recursive Newton-Euler Algorithms or the Articulated-Body Algorithm. Pinocchio is first tailored for legged robotics applications, but it can be used in extra contexts. It is built upon Eigen for linear algebra and FCL for collision detection. Pinocchio comes with a Python interface for fast code prototyping.
-
URL:
https://
github. com/ stack-of-tasks/ pinocchio - Contact: Justin Carpentier
- Partner: CNRS
6.1.2 MImE
- Name: Manipulation Imitation Environments
- Keywords: Robotics, Simulator, Computer vision
- Functional Description: Simulation environment for learning robotics manipulation policies.
-
URL:
https://
github. com/ ikalevatykh/ mime/ - Contact: Igor Kalevatykh
6.1.3 Real2Sim
- Name: Approximate State Estimation from Real Videos
- Keywords: Machine learning, Computer vision, Robotics
- Functional Description: The code is divided into a few main - estimating/optimizing course states from a - reinforcement learning of the control - benchmarking.
-
URL:
https://
data. ciirc. cvut. cz/ public/ projects/ 2020Real2Sim/ - Publication: hal-03017607
- Contact: Makarand Tapaswi
- Participants: Vladimir Petrik, Makarand Tapaswi, Ivan Laptev, Josef Sivic
6.1.4 Sparse-NCNet
- Keyword: Matching
- Functional Description: This is the implementation of the paper "Efficient Neighbourhood Consensus Networks via Submanifold Sparse Convolutions" by Ignacio Rocco, Relja Arandjelović and Josef Sivic, accepted to ECCV 2020
-
URL:
https://
github. com/ ignacio-rocco/ sparse-ncnet - Contact: Ignacio Rocco Spremolla
6.1.5 LIReC
- Name: Learning Interactions and Relationships between Movie Characters
- Keywords: Computer vision, Machine learning
- Functional Description: Interactions between people are often governed by their relationships. On the flip side, social relationships are built upon several interactions. Two strangers are more likely to greet and introduce themselves while becoming friends over time. We are fascinated by this interplay between interactions and relationships, and believe that it is an important aspect of understanding social situations. In this work, we propose neural models to learn and jointly predict interactions, relationships, and the pair of characters that are involved.
-
URL:
https://
annusha. github. io/ LIReC/ - Publication: hal-03017606
- Contact: Makarand Tapaswi
- Participants: Anna Kukleva, Makarand Tapaswi, Ivan Laptev
6.1.6 diffqcqp
- Name: Differentiable QP/QCQP solver
- Keyword: Optimization
- Functional Description: This solver proposes an implementation of the ADMM algorithm for QP and QCQP problems appearing in the Staggered projections algorithm. This solver also implements derivatives of the solution by using implicit differentiation.
-
URL:
https://
github. com/ quentinll/ diffqcqp - Publication: hal-03025616
- Contact: Quentin Le Lidec
- Participants: Quentin Le Lidec, Justin Carpentier, Ivan Laptev, Igor Kalevatykh, Cordelia Schmid
6.1.7 LCHQS
- Name: Fast and efficient non-blind image deblurring
- Keywords: Image processing, Deep learning
- Functional Description: A non-blind deblurring approach based on splitting algorithms, fixed-point equations and learnable prior term. Code of the "End-to-end interpretable learning of non-blind image deblurring" paper accepted at ECCV2020.
-
URL:
https://
github. com/ teboli/ CPCR - Publication: hal-02966204
- Contact: Thomas Eboli
- Participants: Thomas Eboli, Jian Sun, Jean Ponce
6.1.8 CosyPose
- Name: CosyPose: Consistent multi-view multi-object 6D pose estimation
- Keywords: Pose estimation, Deep learning, Robotics, Visual servoing (VS)
- Functional Description: Given an RGB image and a 2D bounding box of an object with a known 3D model, the 6D pose estimator predicts the full 6D pose of the object with respect to the camera. Our method is inspired by DeepIM with several simplifications and technical improvements. It is fully implemented in PyTorch and achieve single-view state-of-the-art on YCB-Video and T-LESS. We provide pre-trained models used in our experiments on both datasets. We make the training code that we used to train them available. It can be parallelized on multiple GPUs and multiple nodes.
-
URL:
https://
github. com/ ylabbe/ cosypose - Contact: Yann Labbé
- Partner: Ecole des Ponts ParisTech
6.2 New platforms
In 2020, we have made the acquisition of a fully instrumented bi-manual wheeled robotic platform (Tiago++) developed by PAL Robotics (Barcelona, Spain) and dedicated for navigation and manipulation. This new robot will strengthen our research and will enable the development of complex manipulation skills involving locomotion. Our ambition is to advance state of the art which currently addresses navigation and manipulation, but can not perform both at the same time.
In addition to that, together with the SED of Inria Paris, we have set up the new robotics laboratory of Inria Paris, currently located on the 5th floor of the main building. This laboratory is now composed of two robotic anthropomorphic arms for manipulation experiments mounted on a fixed frame basement, as well as the new Tigao++ robot.
7 New results
7.1 3D object and scene modeling, analysis, and retrieval
7.1.1 Leveraging Photometric Consistency over Time for Sparsely Supervised Hand-Object Reconstruction
Participants: Hasson Yana, Bugra Tekin, Federica Bogo, Ivan Laptev, Marc Pollefeys, Cordelia Schmid.
Modeling hand-object manipulations is essential for understanding how humans interact with their environment. While of practical importance, estimating the pose of hands and objects during interactions is challenging due to the large mutual occlusions that occur during manipulation. Recent efforts have been directed towards fully-supervised methods that require large amounts of labeled training samples. Collecting 3D ground-truth data for hand-object interactions, however, is costly, tedious, and error-prone. To overcome this challenge, in 13 we present a method to leverage photometric consistency across time when annotations are only available for a sparse subset of frames in a video. Our model is trained end-to-end on color images to jointly reconstruct hands and objects in 3D by inferring their poses. Given our estimated reconstructions, we differentiably render the optical flow between pairs of adjacent images and use it within the network to warp one frame to another. We then apply a self-supervised photometric loss that relies on the visual consistency between nearby images. Examples of improvements obtained by our method are shown in Figure 1. We obtain state-of-the-art results on 3D hand-object reconstruction benchmarks and demonstrate that our approach allows to improve the pose estimation accuracy by leveraging information from neighboring frames in low-data regimes.
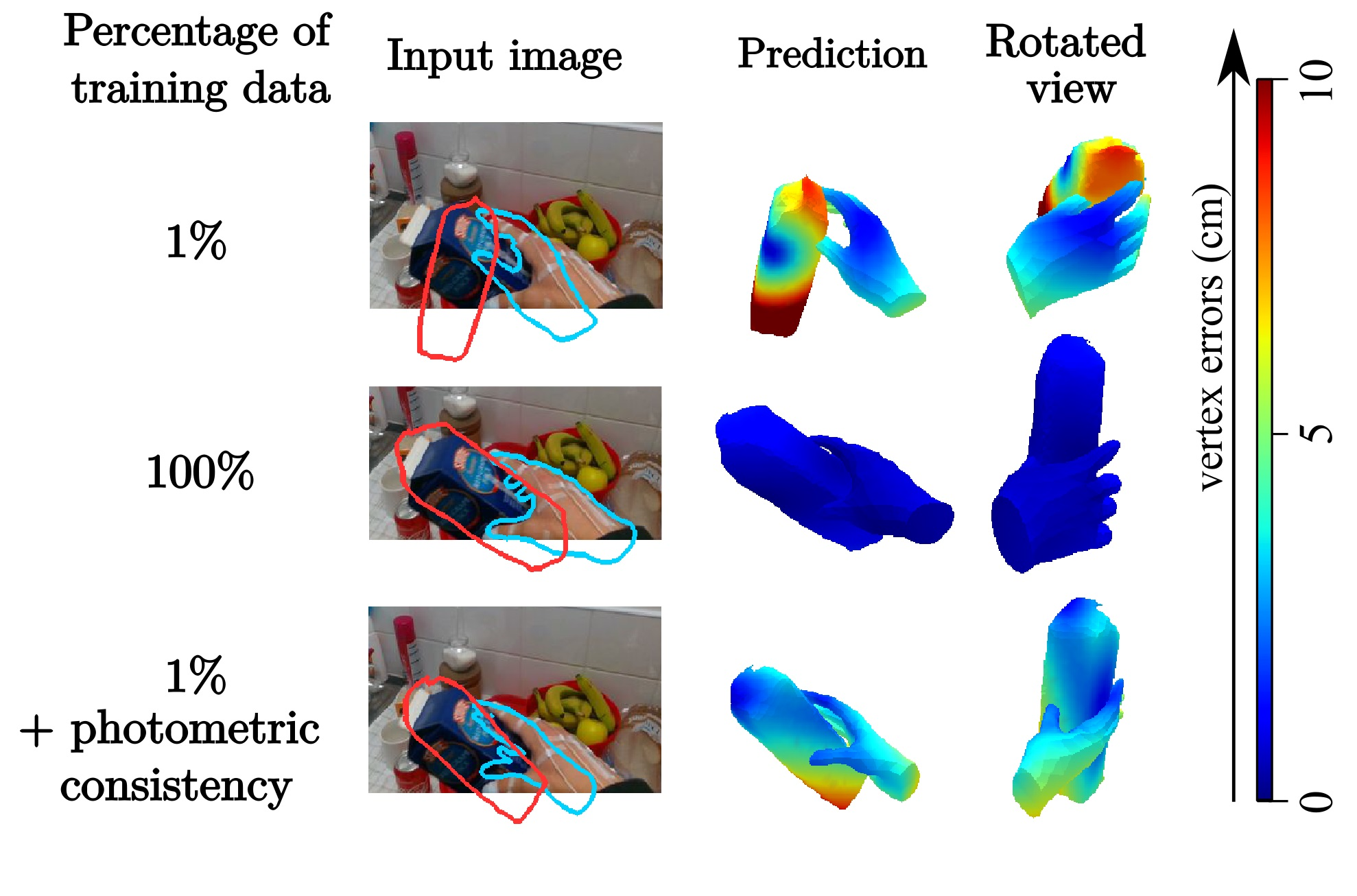
7.1.2 CosyPose: Consistent multi-view multi-object 6D pose estimation
Participants: Yann Labbé, Justin Carpentier, Mathieu Aubry, Josef Sivic.
In 15, we introduce an approach for recovering the 6D pose of multiple known objects in a scene captured by a set of input images with unknown camera viewpoints.
First, we present a single-view single-object 6D pose estimation method, which we use to generate 6D object pose hypotheses. Second, we develop a robust method for matching individual 6D object pose hypotheses across different input images in order to jointly estimate camera viewpoints and 6D poses of all objects in a single consistent scene. Our approach explicitly handles object symmetries, does not require depth measurements, is robust to missing or incorrect object hypotheses, and automatically recovers the number of objects in the scene.
Third, we develop a method for global scene refinement given multiple object hypotheses and their correspondences across views. This is achieved by solving an object-level bundle adjustment problem that refines the poses of cameras and objects to minimize the reprojection error in all views.
We demonstrate that the proposed method, dubbed CosyPose, outperforms current state-of-the-art results for single-view and multi-view 6D object pose estimation by a large margin on two challenging benchmarks: the YCB-Video and T-LESS datasets. Code and pre-trained models are available on the project webpage https://
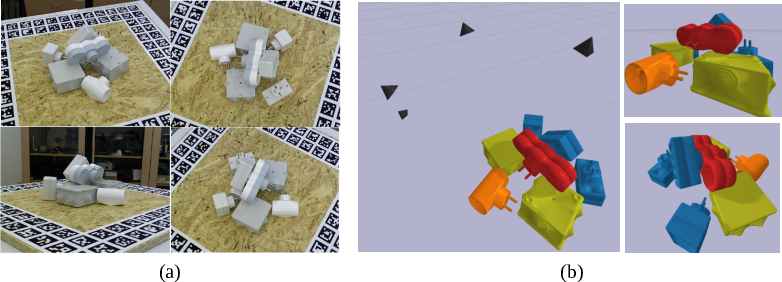
7.2 Category-level object and scene recognition
7.2.1 Efficient Neighbourhood Consensus Networks via Submanifold Sparse Convolutions
Participants: Ignacio Rocco, Relja Arandjelovic, Josef Sivic.
In 23 we target the problem of estimating accurately localised correspondences between a pair of images. We adopt the recent Neighbourhood Consensus Networks that have demonstrated promising performance for difficult correspondence problems and propose modifications to overcome their main limitations: large memory consumption, large inference time and poorly localised correspondences. Our proposed modifications can reduce the memory footprint and execution time more than 10×, with equivalent results. This is achieved by sparsifying the correlation tensor containing tentative matches, and its subsequent processing with a 4D CNN using submanifold sparse convolutions. Localisation accuracy is significantly improved by processing the input images in higher resolution, which is possible due to the reduced memory footprint, and by a novel two-stage correspondence relocalisation module. The proposed Sparse-NCNet method obtains state-of-the-art results on the HPatches Sequences and InLoc visual localisation benchmarks as well as competitive results in the Aachen Day-Night benchmark. Results of our method are illustrated in Figure 3.
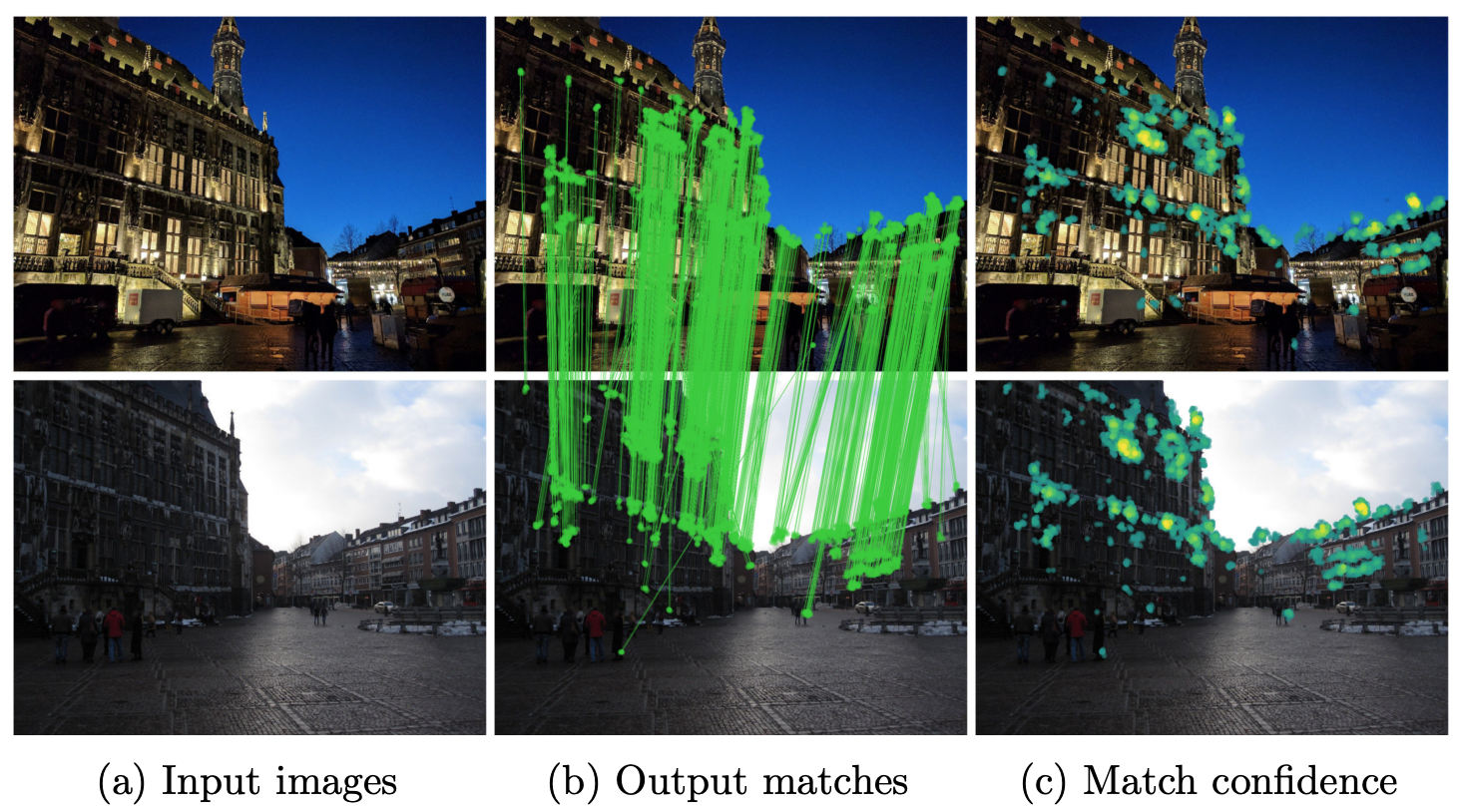
7.2.2 NCNet: Neighbourhood Consensus Networks for Estimating Image Correspondences
Participants: Ignacio Rocco, Mircea Cimpoi, Relja Arandjelovic, Akihiko Torii, Tomas Pajdla, Josef Sivic.
In 6 we address the problem of finding reliable dense correspondences between a pair of images. This is a challenging task due to strong appearance differences between the corresponding scene elements and ambiguities generated by repetitive patterns. The contributions of this work are threefold. First, inspired by the classic idea of disambiguating feature matches using semi-local constraints, we develop an end-to-end trainable convolutional neural network architecture that identifies sets of spatially consistent matches by analyzing neighbourhood consensus patterns in the 4D space of all possible correspondences between a pair of images without the need for a global geometric model. Second, we demonstrate that the model can be trained effectively from weak supervision in the form of matching and non-matching image pairs without the need for costly manual annotation of point to point correspondences. Third, we show the proposed neighbourhood consensus network can be applied to a range of matching tasks including both category- and instance-level matching, obtaining the state-of-the-art results on the PF, TSS, InLoc and HPatches benchmarks. Figure 4 presents qualitative results of NCNet.
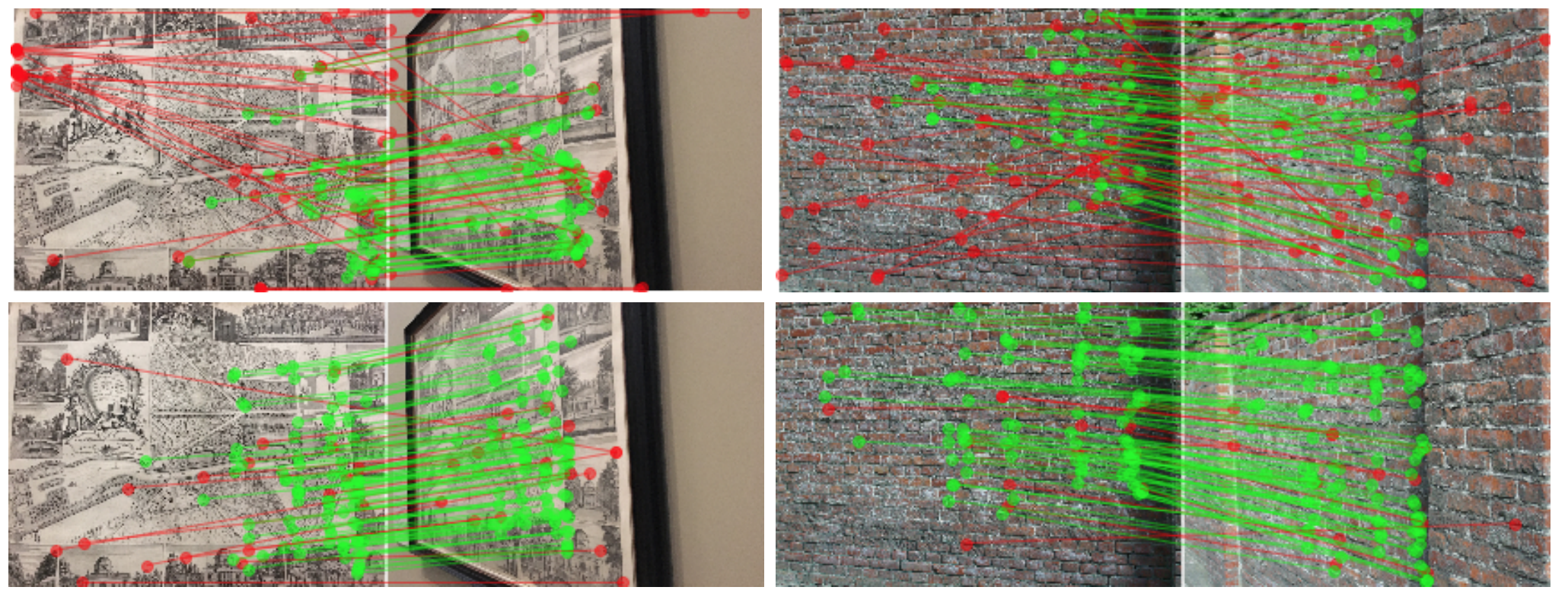
7.2.3 Toward unsupervised, multi-object discovery in large-scale image collections
Participants: Van Huy Vo, Patrick Pérez, Jean Ponce.
27 addresses the problem of discovering the objects present in a collection of images without any supervision. We build on the optimization approach of Vo et al. (CVPR'19) with several key novelties: (1) We propose a novel saliency-based region proposal algorithm that achieves significantly higher overlap with ground-truth objects than other competitive methods. This procedure leverages off-the-shelf CNN features trained on classification tasks without any bounding box information, but is otherwise unsupervised. (2) We exploit the inherent hierarchical structure of proposals as an effective regularizer for the approach to object discovery of Vo et al., boosting its performance to significantly improve over the state of the art on several standard benchmarks. (3) We adopt a two-stage strategy to select promising proposals using small random sets of images before using the whole image collection to discover the objects it depicts, allowing us to tackle, for the first time (to the best of our knowledge), the discovery of multiple objects in each one of the pictures making up datasets with up to 20,000 images, an over five-fold increase compared to existing methods, and a first step toward true large-scale unsupervised image interpretation. Figure 5 presents an illustration of our proposed method.
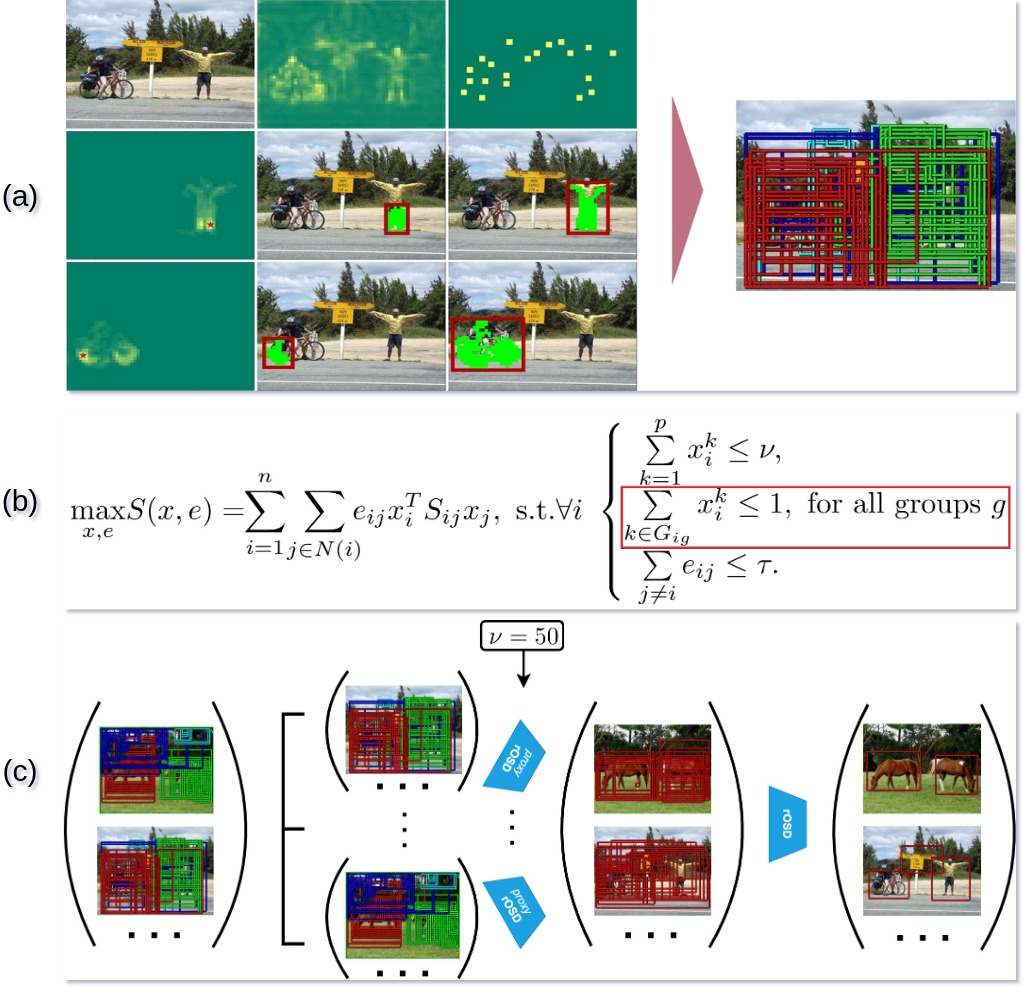
7.3 Image restoration, manipulation and enhancement
7.3.1 End-to-end interpretable learning of non-blind image deblurring
Participants: Thomas Eboli, Jian Sun, Jean Ponce.
Non-blind image deblurring is typically formulated as a linear least-squares problem regularized by natural priors on the corresponding sharp picture's gradients, which can be solved, for example, using a half-quadratic splitting method with Richardson fixed-point iterations for its least-squares updates and a proximal operator for the auxiliary variable updates. In 12 We propose to precondition the Richardson solver using approximate inverse filters of the (known) blur and natural image prior kernels. Using convolutions instead of a generic linear preconditioner allows extremely efficient parameter sharing across the image, and leads to significant gains in accuracy and/or speed compared to classical FFT and conjugate-gradient methods. More importantly, the proposed architecture is easily adapted to learning both the preconditioner and the proximal operator using CNN embeddings. This yields a simple and efficient algorithm for non-blind image deblurring which is fully interpretable, can be learned end to end, and whose accuracy matches or exceeds the state of the art, quite significantly, in the non-uniform case. Figure 6 shows a deblurring comparison with two other state-of-the-art non-blind deblurring methods where the kernels have been predicted directly from the blurry picture. It illustrates the robustness of our method to both lage and approximated kernels occuring in realistic scenarios where the ground-truth blur kernel is not available.
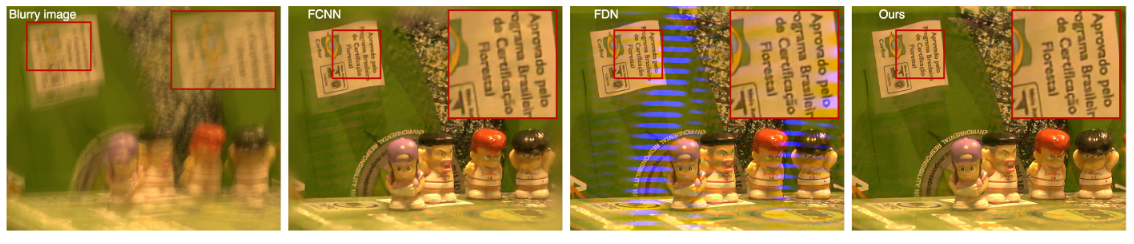
7.3.2 Deformable Kernel Networks for Joint Image Filtering
Participants: Beomjun Kim, Jean Ponce, Bumsub Ham.
Joint image filters are used to transfer structural details from a guidance picture used as a prior to a target image, in tasks such as enhancing spatial resolution and suppressing noise. Previous methods based on convolutional neural networks (CNNs) combine nonlinear activations of spatially-invariant kernels to estimate structural details and regress the filtering result. In 3 we instead learn explicitly sparse and spatially-variant kernels. We propose a CNN architecture and its efficient implementation, called the deformable kernel network (DKN), that outputs sets of neighbors and the corresponding weights adaptively for each pixel. The filtering result is then computed as a weighted average. We also propose a fast version of DKN that runs about four times faster for an image of size 640 × 480. We demonstrate the effectiveness and flexibility of our models on the tasks of depth map upsampling, saliency map upsampling, cross-modality image restoration, texture removal, and semantic segmentation. In particular, we show that the weighted averaging process with sparsely sampled 3 × 3 kernels outperforms the state of the art by a significant margin.
7.3.3 Revisiting Non Local Sparse Models for Image Restoration
Participants: Bruno Lecouat, Jean Ponce, Julien Mairal.
In 17, we propose a differentiable algorithm for image restoration inspired by the success of sparse models and self-similarity priors for natural images. Our approach builds upon the concept of joint sparsity between groups of similar image patches, and we show how this simple idea can be implemented in a differentiable architecture, allowing end-to-end training. The algorithm has the advantage of being interpretable, performing sparse decompositions of image patches, while being more parameter efficient than recent deep learning methods. We evaluate our algorithm on grayscale and color denoising, where we achieve competitive results, and on demoisaicking, where we outperform the most recent state-of-the-art deep learning model with 47 times less parameters and a much shallower architecture. Figure 7 shows results of the proposed approach.
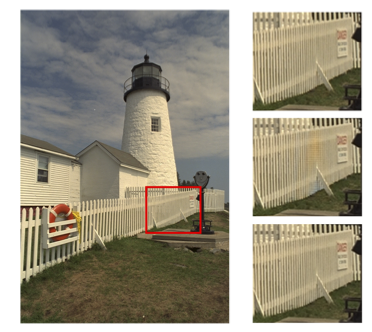
7.3.4 A Web Application for Watermark Recognition
Participants: Oumayma Bounou, Tom Monnier, Ilaria Pastrolin, Xi Shen, Christine Bénévent, Marie-Françoise Limon-Bonnet, François Bougard, Mathieu Aubry, Marc Smith, Olivier Poncet, Pierre-Guillaume Raverdy.
The study of watermarks is a key step for archivists and historians as it enables them to reveal the origin of paper. Although highly practical, automatic watermark recognition comes with many difficulties and is still considered an unsolved challenge. Nonetheless, recent work introduced a new approach for this specific task which showed promising results. Building upon this approach, in 1 we propose a new public web application dedicated to automatic watermark recognition entitled Filigranes pour tous. The application not only hosts a detailed catalog of more than 17k watermarks manually collected from the French National Archives (Minutier central) or extracted from existing online resources (Briquet database), but it also enables non-specialists to identify a watermark from a simple photograph in a few seconds as described in Figure 8.
Moreover, additional watermarks can easily be added by the users making the enrichment of
the existing catalog possible through crowdsourcing. Our Web application is available at http://
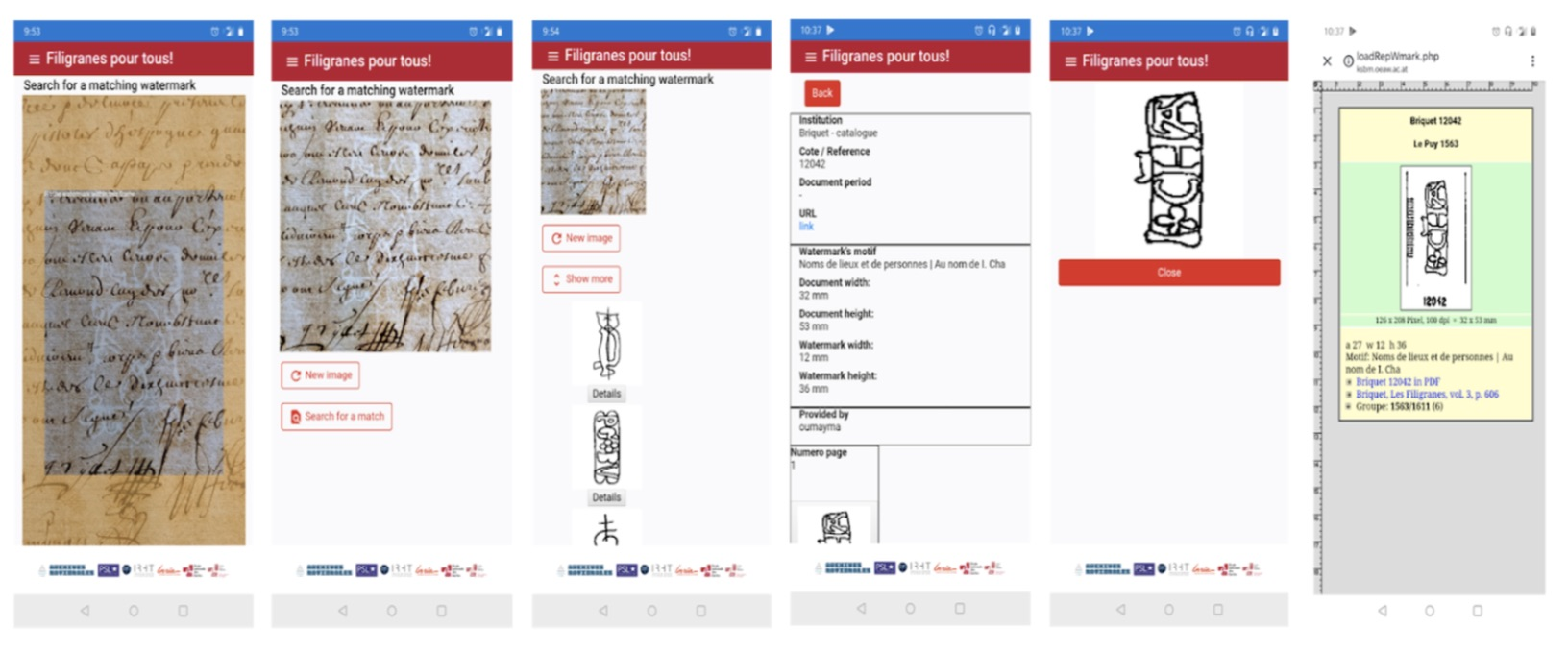
7.3.5 Fully Trainable and Interpretable Non-Local Sparse Models for Image Restoration
Participants: Bruno Lecouat, Jean Ponce, Julien Mairal.
In 17 we propose a novel differentiable relaxation of joint sparsity that exploits both principles and leads to a general framework for image restoration which is (1) trainable end to end, (2) fully interpretable, and (3) much more compact than competing deep learning architectures. We apply this approach to the problems of image denoising, blind denoising, jpeg deblocking, and demosaicking. With as few as 100K parameters our method performs on par or better compared to the state of the art on several standard benchmarks, while previous approaches may have orders of magnitude more parameters. Figure 9 illustrates results of our method.
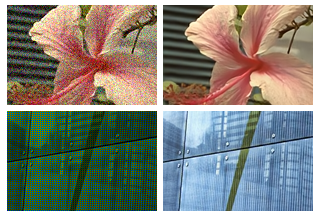
7.3.6 A Flexible Framework for Designing Trainable Priors with Adaptive Smoothing and Game Encoding
Participants: Bruno Lecouat, Jean Ponce, Julien Mairal.
In 16 we introduce a general framework for designing and training neural network layers whose forward passes can be interpreted as solving non-smooth convex optimization problems, and whose architectures are derived from an optimization algorithm. We focus on convex games, solved by local agents represented by the nodes of a graph and interacting through regularization functions. This approach is appealing for solving imaging problems, as it allows the use of classical image priors within deep models that are trainable end to end. The priors used in this presentation include variants of total variation, Laplacian regularization, bilateral filtering, sparse coding on learned dictionaries, and non-local self similarities, see Figure 10. Our models are fully interpretable as well as parameter and data efficient. Our experiments demonstrate their effectiveness on a large diversity of tasks ranging from image denoising and compressed sensing for fMRI to dense stereo matching.
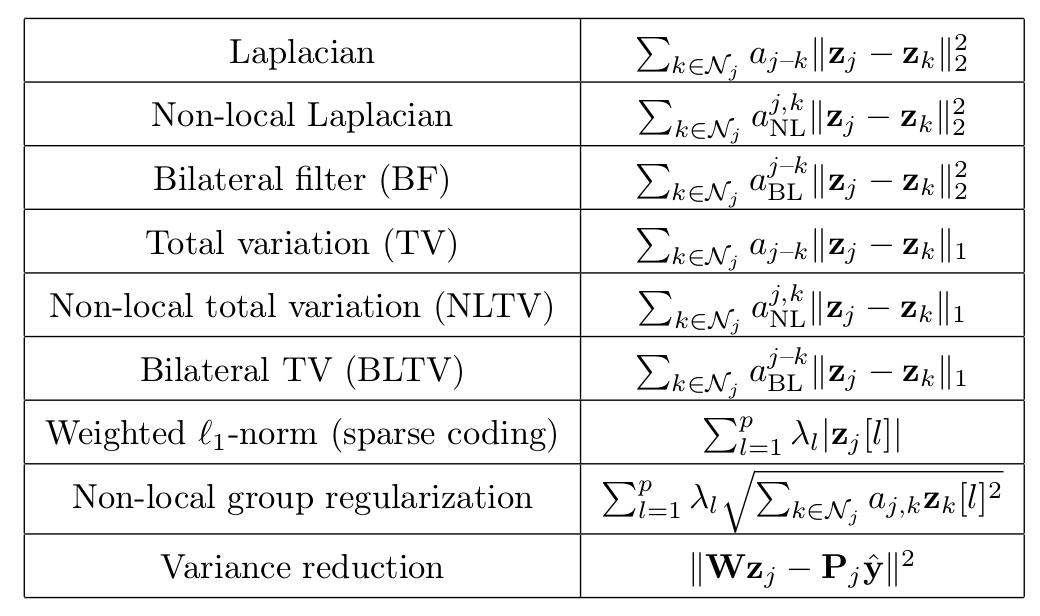
7.4 Human activity capture and classification
7.4.1 Learning Actionness via Long-range Temporal Order
Participants: Dimitri Zhukov, Jean-Baptiste Alayrac, Ivan Laptev, Josef Sivic.
In 28 we propose a new model that learns to separate temporal intervals that contain actions from their background in the videos. Our model is trained via a self-supervised proxy task of order verification, as shown in Figure 11. The model assigns high actionness scores to clips which order is easy to predict from other clips in the video. To obtain a powerful and action-agnostic model, we train it on the large-scale unlabeled HowTo100M dataset with highly diverse actions from instructional videos. We validate our method on the task of action localization and demonstrate consistent improvements when combined with other recent weakly-supervised methods.
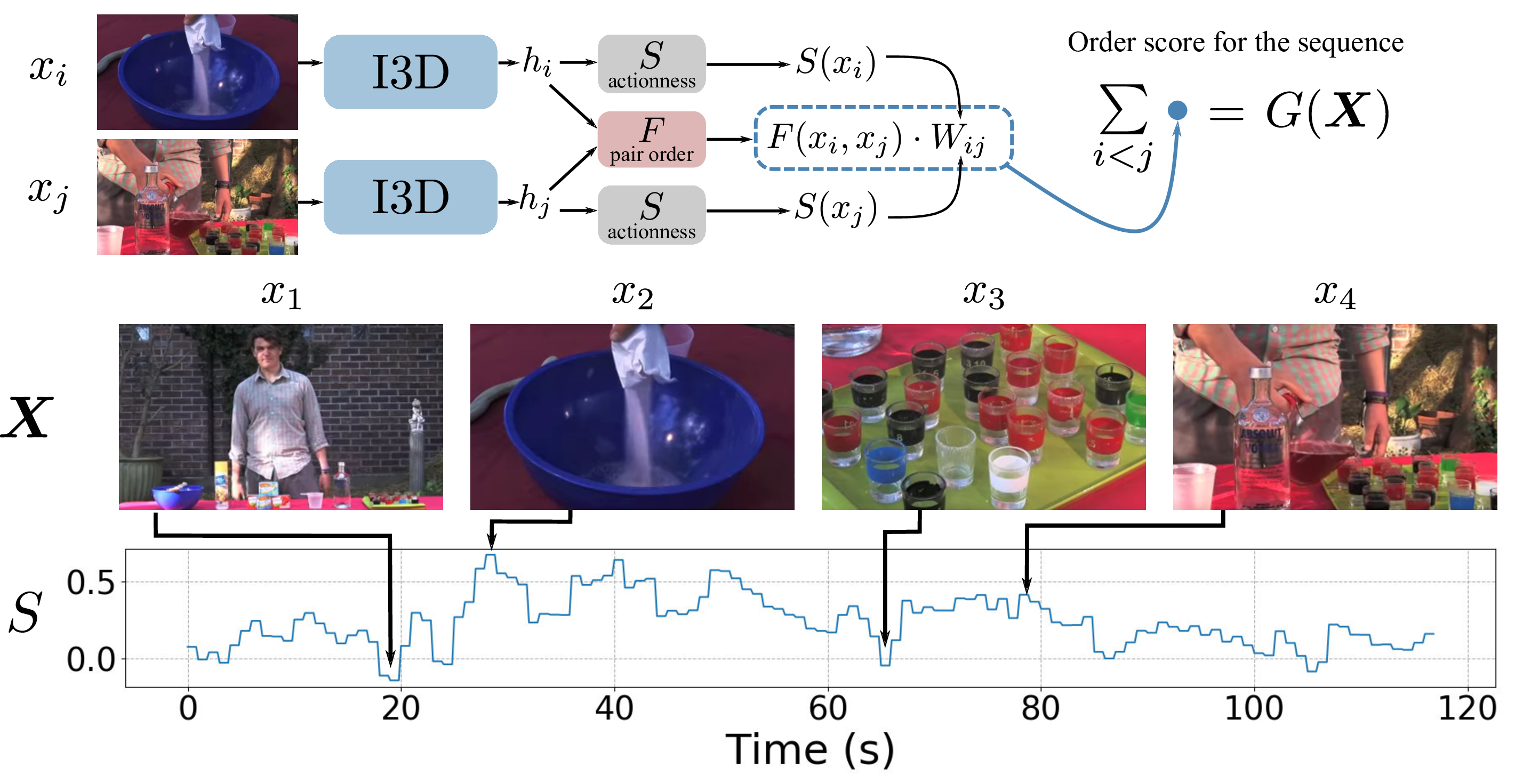
7.4.2 Leveraging the Present to Anticipate the Future in Videos
Participants: Antoine Miech, Ivan Laptev, Josef Sivic, Heng Wang, Lorenzo Torresani, Du Tran.
Anticipating actions before they are executed is crucial for a wide range of practical applications including autonomous driving and the moderation of live video streaming. While most prior work in this area requires partial observation of executed actions, in 33 we focus on anticipating actions seconds before they start (see Figure 12). Our proposed approach is the fusion of a purely anticipatory model with a complementary model constrained to reason about the present. In particular, the latter predicts present action and scene attributes, and reasons about how they evolve over time. By doing so, we aim at modeling action anticipation at a more conceptual level than directly predicting future actions. Our model outperforms previously reported methods on the EPIC-KITCHENS and Breakfast datasets. We have participated in the EPIC-KITCHENS action anticipation challenge where our method has obtained the second place.
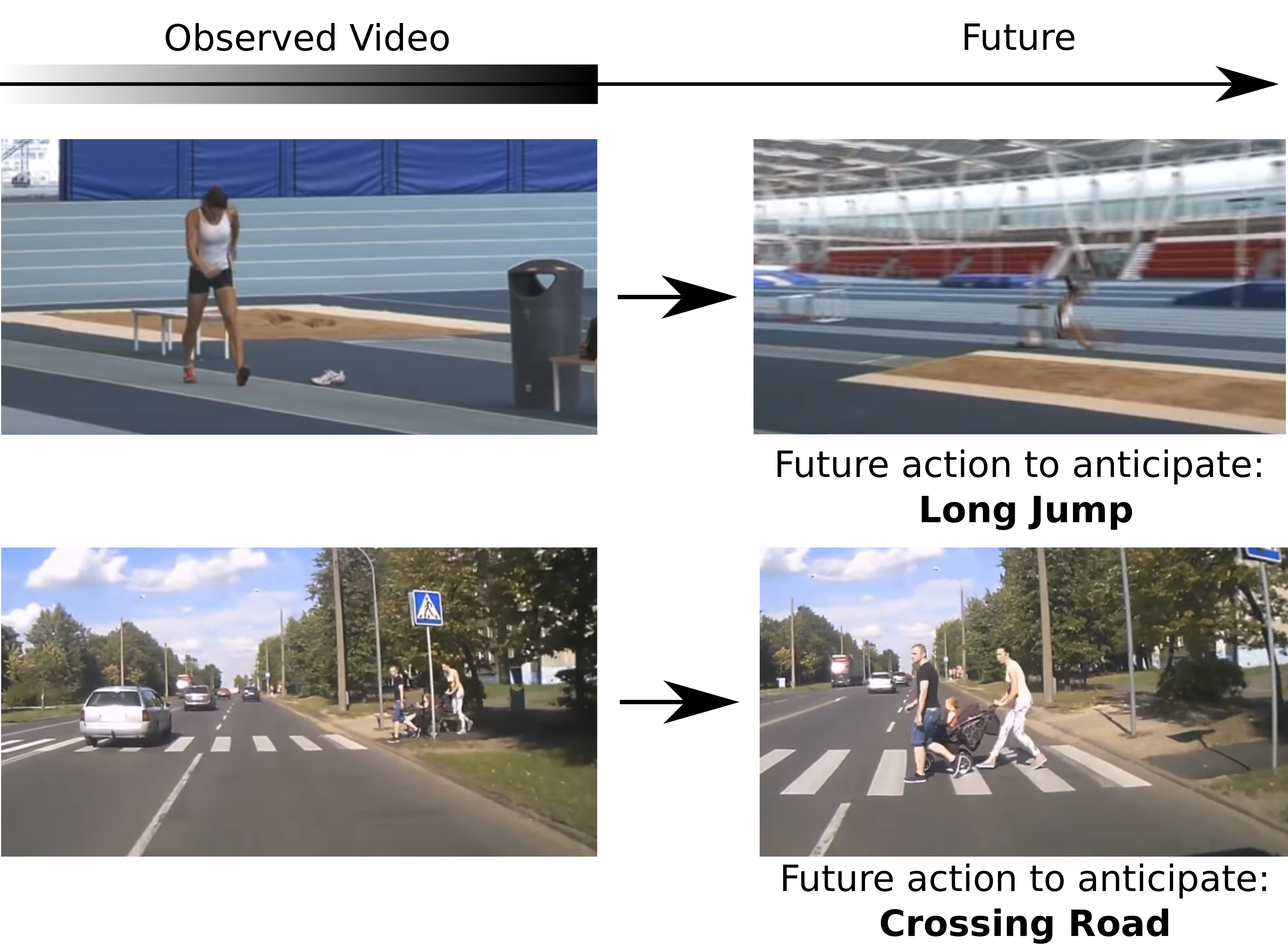
7.4.3 End-to-End Learning of Visual Representations from Uncurated Instructional Videos
Participants: Antoine Miech, Jean-Baptiste Alayrac, Lucas Smaira, Ivan Laptev, Josef Sivic, Andrew Zisserman.
Annotating videos is cumbersome, expensive and not scalable. Yet, many strong video models still rely on manually annotated data. With the recent introduction of the HowTo100M dataset, narrated videos now offer the possibility of learning video representations without manual supervision. In 19 we propose a new learning approach, MIL-NCE, capable of addressing misalignments inherent to narrated videos (see Figure 13). With this approach we are able to learn strong video representations from scratch, without the need for any manual annotation. We evaluate our representations on a wide range of four downstream tasks over eight datasets: action recognition (HMDB-51, UCF-101, Kinetics-700), text-to-video retrieval (YouCook2, MSR-VTT), action localization (YouTube-8M Segments, CrossTask) and action segmentation (COIN). Our method outperforms all published self-supervised approaches for these tasks as well as several fully supervised baselines.

7.4.4 Discovering Actions by Jointly Clustering Video and Narration Streams Across Tasks
Participants: Minttu Alakuijala, Julien Mairal, Jean Ponce, Cordelia Schmid.
We address the problem of discovering actions in narrated tutorial videos by jointly clustering visual features and text, without any external supervision. Our method does not assume any prior grouping of the videos into distinct tasks, or that videos depicting the same task share an identical sequence of actions. In this work, only the narration and the visual stream of each individual video are assumed to depict the same sequence of actions with approximate temporal alignment, as shown in Figure 14. Our method is based on discriminative clustering, with a penalty term corresponding to the distance between the sequence of actions assigned to frames and that assigned to words in each video. This encourages the order and timing of actions in each assignment to become consistent over the course of optimization. Our experimental evaluation on Inria Instruction Videos and CrossTask shows comparable performance to existing task-specific methods while greatly improving on their generality by relaxing the assumption of a shared script, and by requiring less supervision.
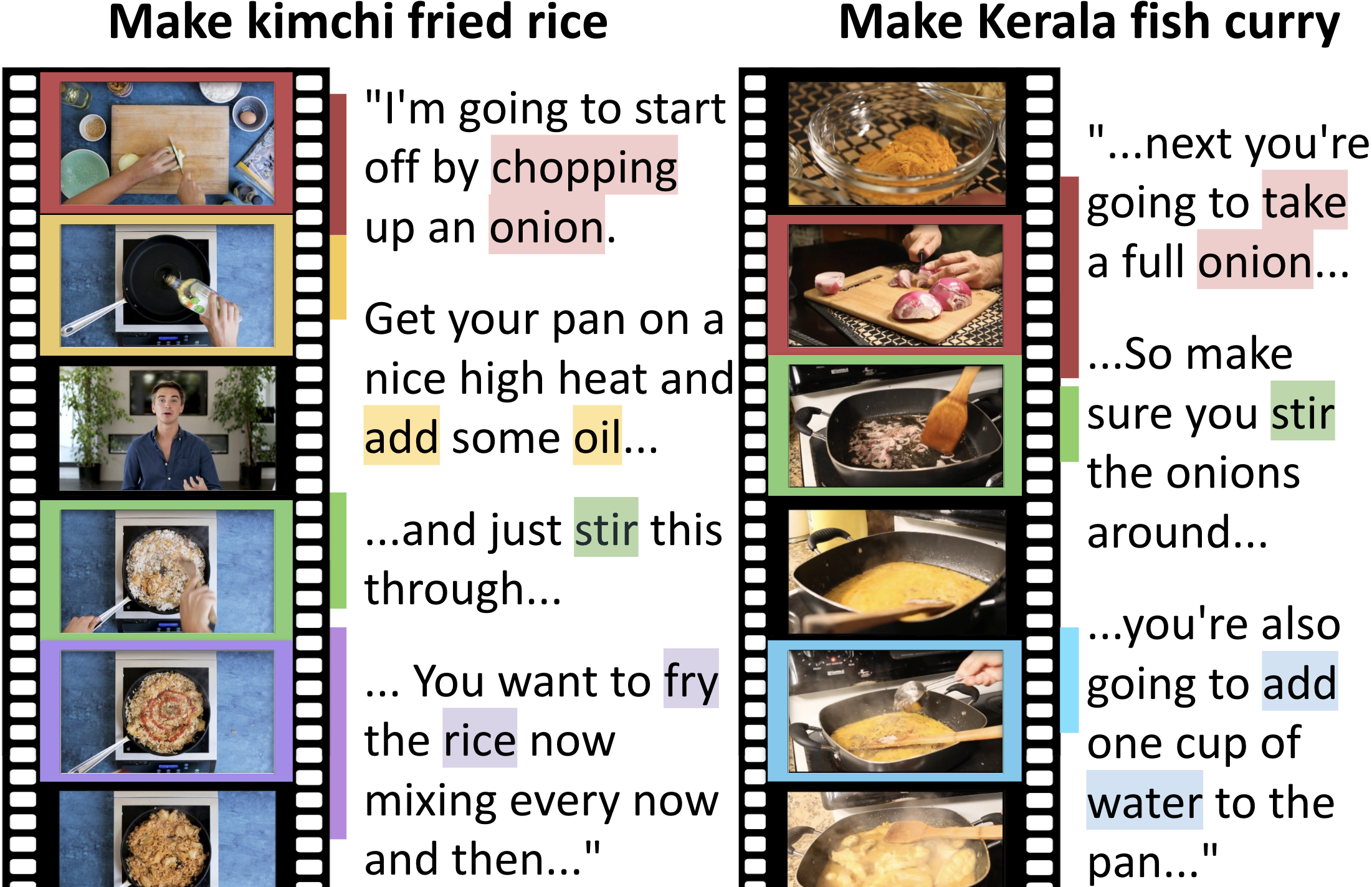
7.4.5 Synthetic Humans for Action Recognition from Unseen Viewpoints
Participants: Gul Varol, Ivan Laptev, Cordelia Schmid, Andrew Zisserman.
In 7 we use synthetic training data to improve the performance of human action recognition for viewpoints unseen during training. Although synthetic data has been shown to be beneficial for tasks such as human pose estimation, its use for RGB human action recognition is relatively unexplored. We make use of the recent advances in monocular 3D human body reconstruction from real action sequences to automatically render synthetic training videos for the action labels. We make the following contributions: (i) we investigate the extent of variations and augmentations that are beneficial to improving performance at new viewpoints. We consider changes in body shape and clothing for individuals, as well as more action relevant augmentations such as non-uniform frame sampling, and interpolating between the motion of individuals performing the same action; (ii) We introduce a new dataset, SURREACT, that allows supervised training of spatio-temporal CNNs for action classification; (iii) We substantially improve the state-of-the-art action recognition performance on the NTU RGB+D and UESTC standard human action multi-view benchmarks; Finally, (iv) we extend the augmentation approach to in-the-wild videos from a subset of the Kinetics dataset to investigate the case when only one-shot training data is available, and demonstrate improvements in this case as well. Figure 15 presents an illustration of the approach.
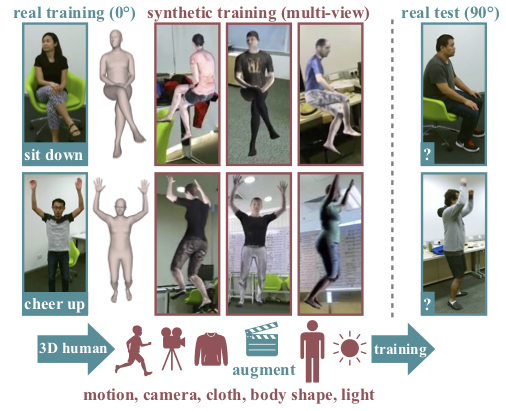
7.4.6 Occlusion resistant learning of intuitive physics from videos
Participants: Ronan Riochet, Josef Sivic, Ivan Laptev, Emmanuel Dupoux.
To reach human performance on complex tasks, a key ability for artificial systems is to understand physical interactions between objects, and predict future outcomes of a situation. This ability, often referred to as intuitive physics, has recently received attention and several methods were proposed to learn these physical rules from video sequences. Yet, most of these methods are restricted to the case where no, or only limited, occlusions occur. In 35 we propose a probabilistic formulation of learning intuitive physics in 3D scenes with significant inter-object occlusions. In our formulation, object positions are modelled as latent variables enabling the reconstruction of the scene. We then propose a series of approximations that make this problem tractable. Object proposals are linked across frames using a combination of a recurrent interaction network, modeling the physics in object space, and a compositional renderer, modeling the way in which objects project onto pixel space (see Figure 16). We demonstrate significant improvements over state-of-the-art in the intuitive physics benchmark of IntPhys. We apply our method to a second dataset with increasing levels of occlusions, showing it realistically predicts segmentation masks up to 30 frames in the future. Finally, we also show results on predicting motion of objects in real videos.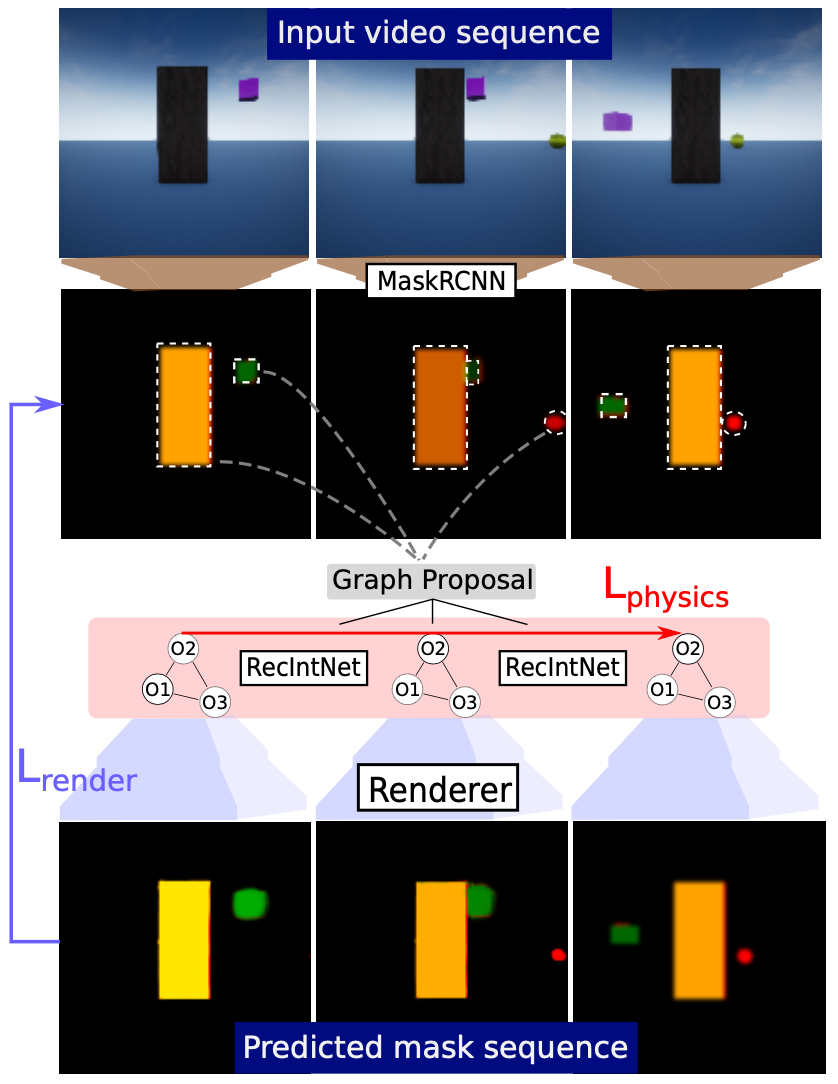
7.5 Learning embodied representations and robotics
7.5.1 Learning to combine primitive skills: A step towards versatile robotic manipulation
Participants: Robin Strudel, Alexander Pashevich, Igor Kalevatykh, Ivan Laptev, Josef Sivic, Cordelia Schmid.
Manipulation tasks such as preparing a meal or assembling furniture remain highly challenging for robotics and vision. Traditional task and motion planning (TAMP) methods can solve complex tasks but require full state observability and are not adapted to dynamic scene changes. Recent learning methods can operate directly on visual inputs but typically require many demonstrations and/or task-specific reward engineering. In 26 we aim to overcome previous limitations and propose a reinforcement learning (RL) approach to task planning that learns to combine primitive skills. First, compared to previous learning methods, our approach requires neither intermediate rewards nor complete task demonstrations during training. Second, we demonstrate the versatility of our vision-based task planning in challenging settings with temporary occlusions and dynamic scene changes. Third, we propose an efficient training of basic skills from few synthetic demonstrations by exploring recent CNN architectures and data augmentation. Notably, while all of our policies are learned on visual inputs in simulated environments, we demonstrate the successful transfer and high success rates when applying such policies to manipulation tasks on a real UR5 robotic arm. Figure 17 presents an overview of the approach.
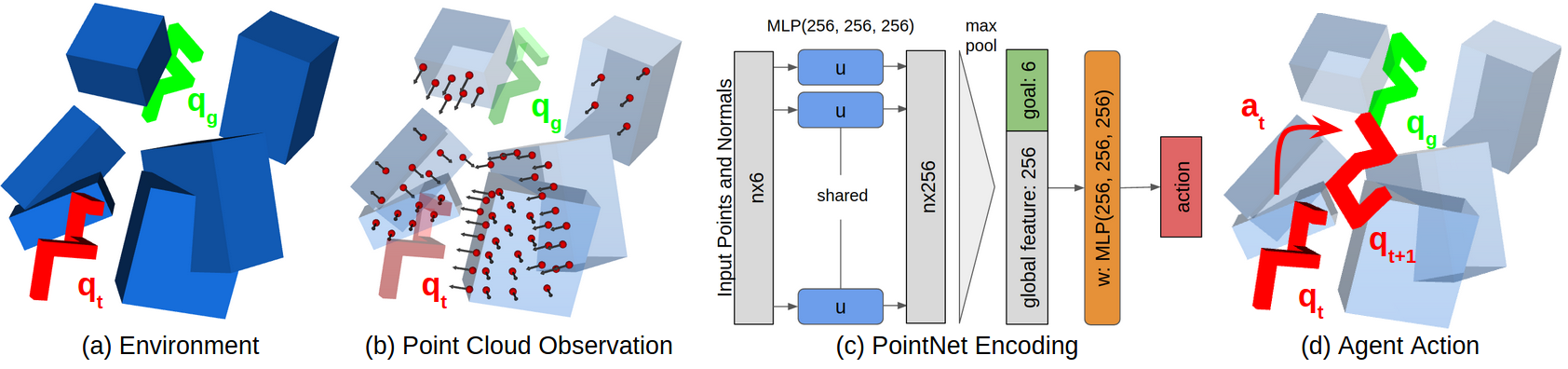
7.5.2 Monte-Carlo Tree Search for Efficient Visually Guided Rearrangement Planning
Participants: Yann Labbé, Sergey Zagoruyko, Igor Kalevatykh, Ivan Laptev, Justin Carpentier, Mathieu Aubry, Josef Sivic.
In 4 we address the problem of visually guided rearrangement planning with many movable objects, i.e., finding a sequence of actions to move a set of objects from an initial arrangement to a desired one, while relying on visual inputs coming from RGB camera. To do so, we introduce a complete pipeline relying on two key contributions. First, we introduce an efficient and scalable rearrangement planning method, based on a Monte-Carlo Tree Search exploration strategy. We demonstrate that because of its good trade-off between exploration and exploitation our method (i) scales well with the number of objects while (ii) finding solutions which require a smaller number of moves compared to the other state-of-the-art approaches. Note that on the contrary to many approaches, we do not require any buffer space to be available. Second, to precisely localize movable objects in the scene, we develop an integrated approach for robust multi-object workspace state estimation from a single uncalibrated RGB camera using a deep neural network trained only with synthetic data. We validate our multi-object visually guided manipulation pipeline with several experiments on a real UR-5 robotic arm by solving various rearrangement planning instances, requiring only 60 ms to compute the complete plan to rearrange 25 objects. In addition, we show that our system is insensitive to camera movements and can successfully recover from external perturbation. Figure 18 shows an example of the problems we consider.
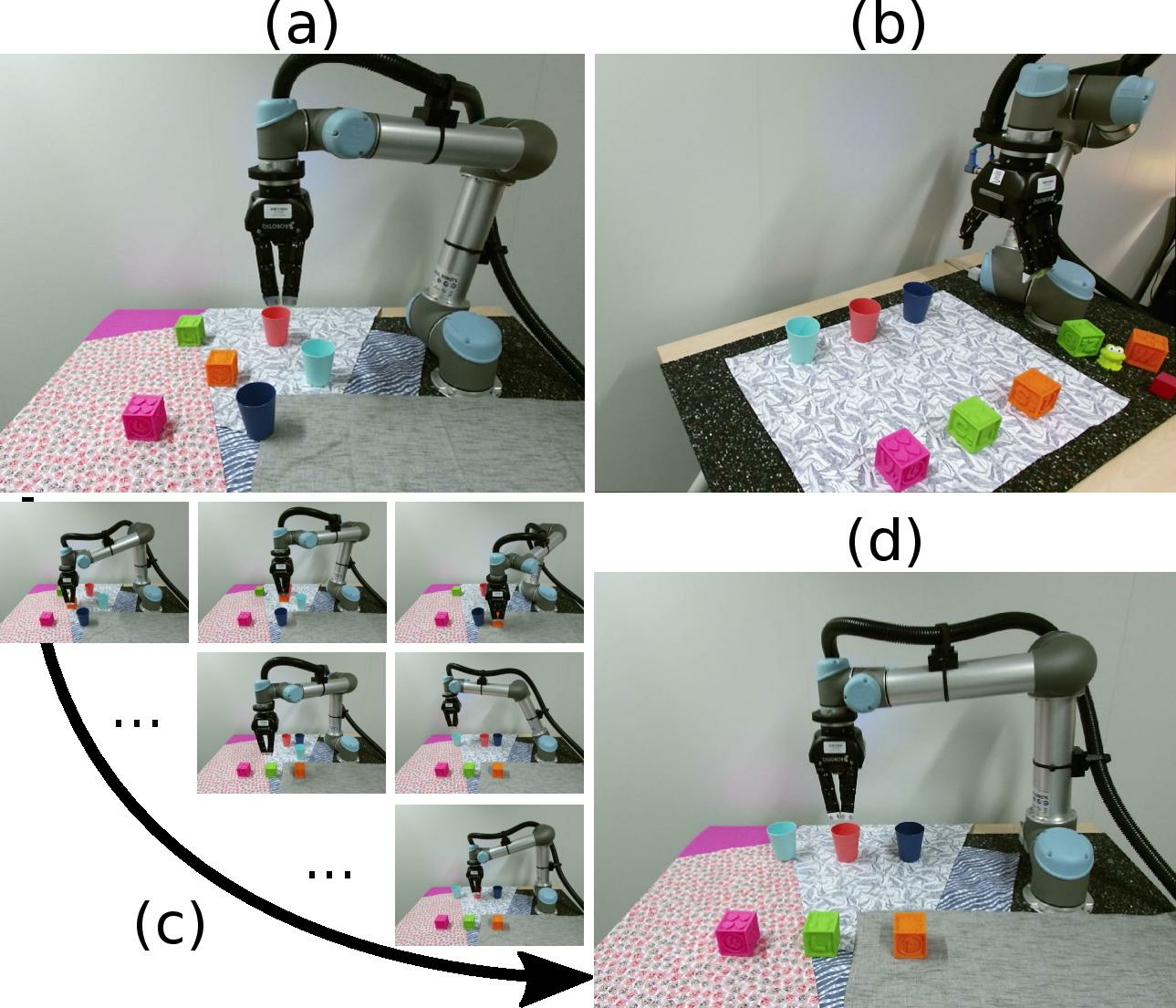
7.5.3 Crocoddyl: An Efficient and Versatile Framework for Multi-Contact Optimal Control
Participants: Carlos Mastalli, Rohan Budhiraja, Wolfgang Merkt, Guilhem Saurel, Bilal Hammoud, Maximilien Naveau, Justin Carpentier, Sethu Vijayakumar, Nicolas Mansard.
In 18 we introduce Crocoddyl (Contact RObot COntrol by Differential DYnamic Library), an open-source framework tailored for efficient multi-contact optimal control. Crocoddyl efficiently computes the state trajectory and the control policy for a given predefined sequence of contacts. Its efficiency is due to the use of sparse analytical derivatives, exploitation of the problem structure, and data sharing. It employs differential geometry to properly describe the state of any geometrical system, e.g. floating-base systems. We have unified dynamics, costs, and constraints into a single concept-action-for greater efficiency and easy prototyping. Additionally, we propose a novel multiple-shooting method called Feasibility-prone Differential Dynamic Programming (FDDP). Our novel method shows a greater globalization strategy compared to classical Differential Dynamic Programming (DDP) algorithms, and it has similar numerical behavior to state-of-the-art multiple-shooting methods. However, our method does not increase the computational complexity typically encountered by adding extra variables to describe the gaps in the dynamics. Concretely, we propose two modifications to the classical DDP algorithm. First, the backward pass accepts infeasible state-control trajectories. Second, the rollout keeps the gaps open during the early "exploratory" iterations (as expected in multiple-shooting methods). We showcase the performance of our framework using different tasks. With our method, we can compute highly-dynamic maneuvers for legged robots (e.g. jumping, front-flip) in the order of milliseconds. Figure 19 presents a resulting motion of the proposed approach.
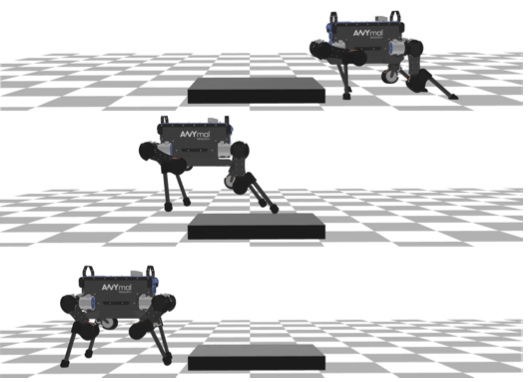
7.5.4 Fast and Robust Stability Region Estimation for Nonlinear Dynamical Systems
Participants: Eloïse Berthier, Justin Carpentier, Francis Bach.
A linear quadratic regulator can stabilize a nonlinear dynamical system with a local feedback controller around a linearization point, while minimizing a given performance criteria. An important practical problem is to estimate the region of attraction of such a controller, that is, the region around this point where the controller is certified to be valid. This is especially important in the context of highly nonlinear dynamical systems. In 32 we propose two stability certificates that are fast to compute and robust when the first, or second derivatives of the system dynamics are bounded. Associated with an efficient oracle to compute these bounds, this provides a simple stability region estimation algorithm compared to classic approaches of the state of the art. We experimentally validate that it can be applied to both polynomial and non-polynomial systems of various dimensions, including standard robotic systems, for estimating region of attractions around equilibrium points, as well as for trajectory tracking. This work is a joint contribution between Willow and Sierra teams.
7.5.5 Differentiable simulation for physical system identification
Participants: Quentin Le Lidec, Igor Kalevatykh, Ivan Laptev, Cordelia Schmid, Justin Carpentier.
Simulating frictional contacts remains a challenging research topic in robotics. Recently, differentiable physics emerged and has proven to be a key element in model-based Reinforcement Learning (RL) and optimal control fields. However, most of the current formulations deploy coarse approximations of the underlying physical principles. Indeed, the classic simulators loose precision by casting the Nonlinear Complementarity Problem (NCP) of frictional contact into a Linear Complementarity Problem (LCP) to simplify computations. Moreover, such methods deploy non-smooth operations and cannot be automatically differentiated. In 5, we propose (i) an extension of the staggered projections algorithm for more accurate solutions of the problem of contacts with friction. Based on this formulation, we introduce (ii) a differentiable simulator and an efficient way to compute the analytical derivatives of the involved optimization problems. Finally, (iii) we validate the proposed framework with a set of experiments to present a possible application of our differentiable simulator. In particular, using our approach we demonstrate accurate estimation of friction coefficients and object masses both in synthetic and real experiments. An overview of our approach is presented in Figure 20.
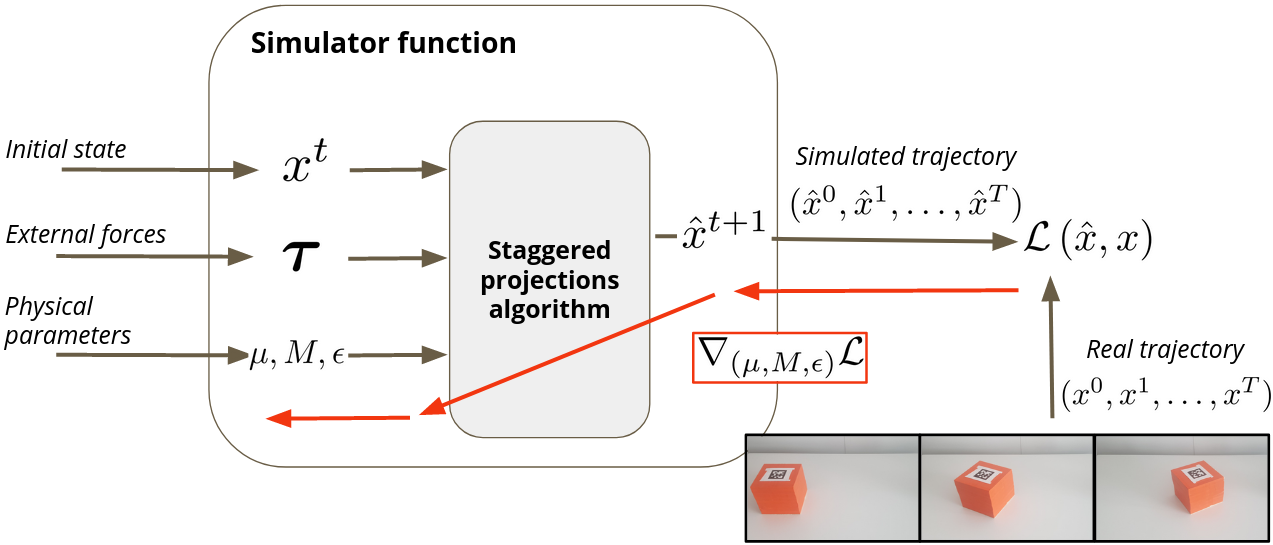
7.5.6 Learning Obstacle Representations for Neural Motion Planning
Participants: Robin Strudel, Ricardo Garcia, Justin Carpentier, Jean-Paul Laumond, Ivan Laptev, Cordelia Schmid.
Motion planning and obstacle avoidance is a key challenge in robotics applications. While previous work succeeds to provide excellent solutions for known environments, sensor-based motion planning in new and dynamic environments remains difficult. In 25 we address sensor-based motion planning from a learning perspective. Motivated by recent advances in visual recognition, we argue the importance of learning appropriate representations for motion planning. We propose a new obstacle representation based on the PointNet architecture and train it jointly with policies for obstacle avoidance. We experimentally evaluate our approach for rigid body motion planning in challenging environments and demonstrate significant improvements of the state of the art in terms of accuracy and efficiency. Figure 21 presents an overview of our approach.
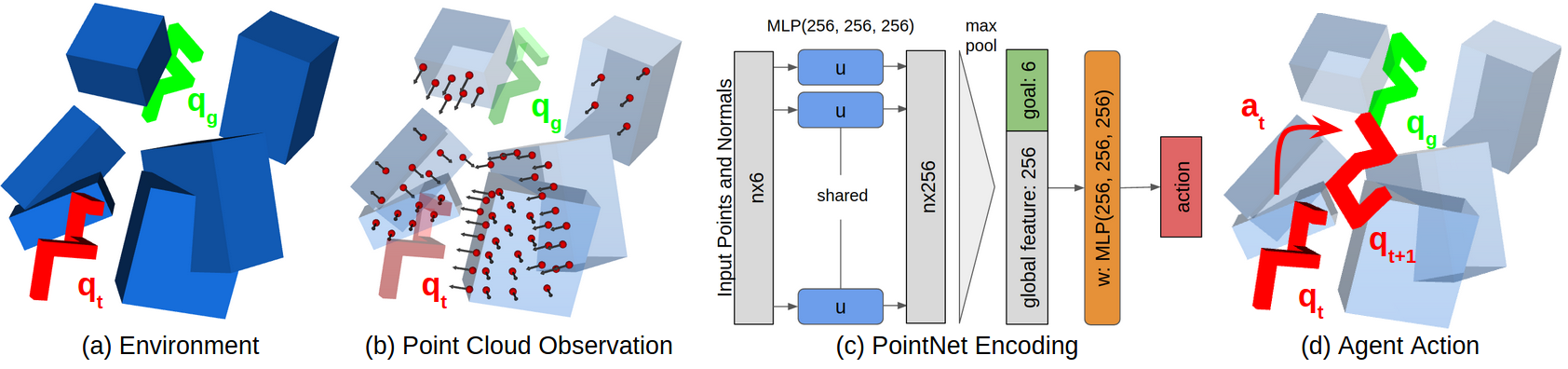
7.5.7 C-CROC: Continuous and Convex Resolution of Centroidal Dynamic Trajectories for Legged Robots in Multicontact Scenarios
Participants: Pierre Fernbach, Steve Tonneau, Olivier Stasse, Justin Carpentier, Michel Taïx.
Synthesizing legged locomotion requires planning one or several steps ahead (literally): when and where, and with which effector should the next contact(s) be created between the robot and the environment? Validating a contact candidate implies a minima the resolution of a slow, nonlinear optimization problem, to demonstrate that a center of mass (CoM) trajectory, compatible with the contact transition constraints, exists. In 2 we propose a conservative reformulation of this trajectory generation problem as a convex 3-D linear program, named convex resolution of centroidal dynamic trajectories (CROC). It results from the observation that if the CoM trajectory is a polynomial with only one free variable coefficient, the nonlinearity of the problem disappears. This has two consequences. On the positive side, in terms of computation times, CROC outperforms the state of the art by at least one order of magnitude, and allows to consider interactive applications (with a planning time roughly equal to the motion time). On the negative side, in our experiments, our approach finds a majority of the feasible trajectories found by a nonlinear solver, but not all of them. Still, we demonstrate that the solution space covered by CROC is large enough to achieve the automated planning of a large variety of locomotion tasks for different robots demonstrated in simulation and on the real HRP-2 robot, several of which were rarely seen before (see Figure 22). Another significant contribution is the introduction of a Bezier curve representation of the problem, which guarantees that the constraints of the CoM trajectory are verified continuously, and not only at discrete points as traditionally done. This formulation is lossless, and results in more robust trajectories. It is not restricted to CROC, but could rather be integrated with any method from the state of the art.
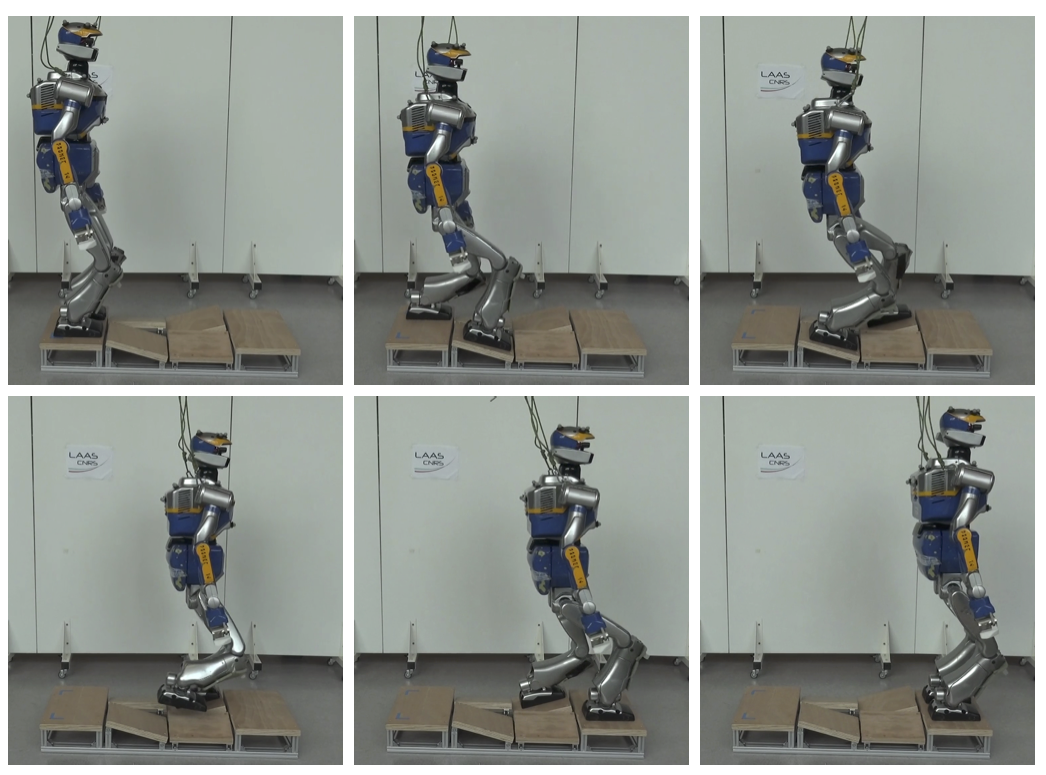
7.5.8 Visualizing computation in large-scale cellular automata
Participants: Hugo Cisneros, Josef Sivic, Tomas Mikolov.
In 8 we examine emergent processes in complex systems such as cellular automata. They can perform computations of increasing complexity, and could possibly lead to artificial evolution. Such a feat would require scaling up current simulation sizes to allow for enough computational capacity. Understanding complex computations happening in cellular automata and other systems capable of emergence poses many challenges, especially in large-scale systems. We propose methods for coarse-graining cellular automata based on frequency analysis of cell states, clustering and autoencoders. These innovative techniques facilitate the discovery of large-scale structure formation and complexity analysis in those systems. They emphasize interesting behaviors in elementary cellular automata while filtering out background patterns. Moreover, our methods reduce large 2D automata to smaller sizes and enable identifying systems that behave interestingly at multiple scales. Figure 23 presents some example filtering results.
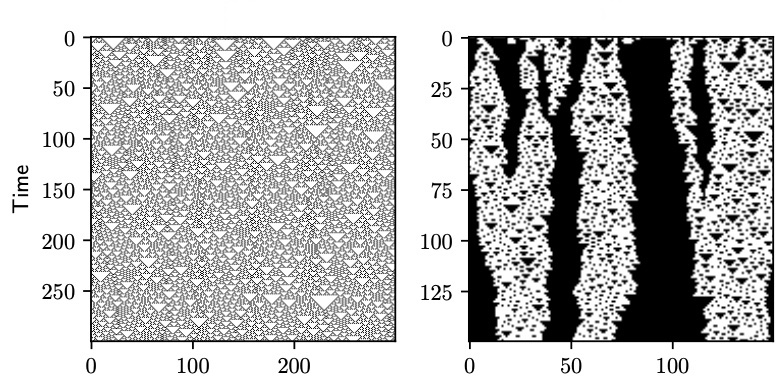
7.5.9 Residual Reinforcement Learning from Demonstrations
Participants: Minttu Alakuijala, Gabriel Dulac-Arnold, Julien Mairal, Jean Ponce, Cordelia Schmid.
Residual reinforcement learning (RL) has been proposed as a way to solve challenging robotic tasks by adapting control actions from a conventional feedback controller to maximize a reward signal. We extend the residual formulation to learn from visual inputs and sparse rewards using demonstrations (as outlined in Figure 24). Learning from pixels and a sparse task-completion reward relaxes the requirement of full state features being available. In addition, replacing the base controller with a policy learned from demonstration removes the dependency on a hand-engineered controller in favour of a dataset of demonstrations, which can be provided by non-experts. Our experimental evaluation on manipulation tasks on a simulated UR5 arm demonstrates that residual RL from demonstration is able to generalize to unseen environment conditions more flexibly than behavioral cloning, while benefiting from a vast improvement in data efficiency compared to RL from scratch.
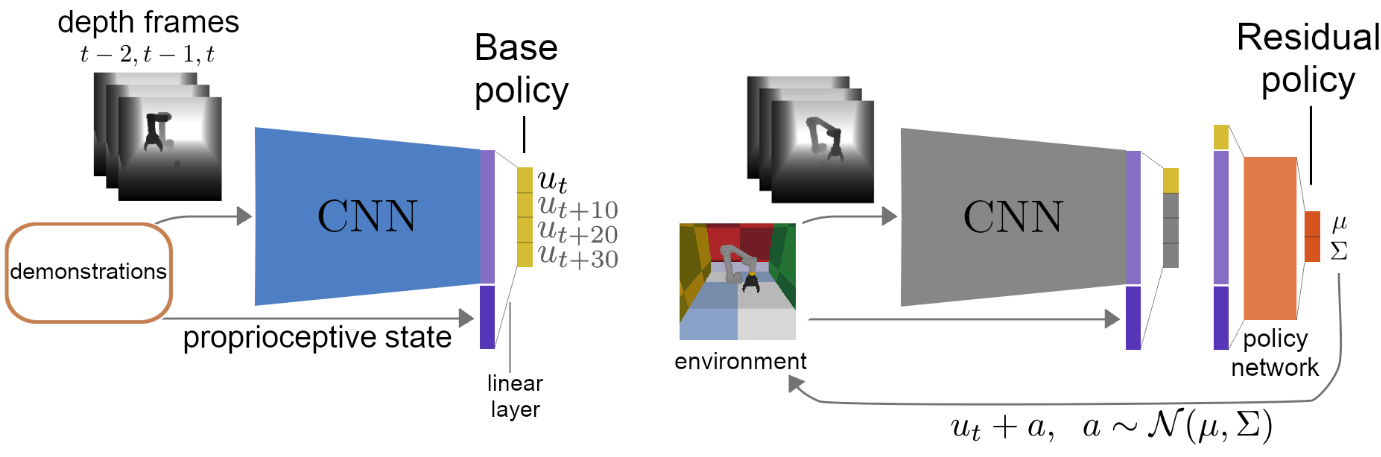
8 Bilateral contracts and grants with industry
8.1 Bilateral contracts with industry
8.1.1 MSR-Inria joint lab: Image and video mining for science and humanities (Inria)
Participants: Yana Hasson, Ivan Laptev, Jean Ponce, Josef Sivic, Dimitri Zhukov, Cordelia Schmid.
This collaborative project brings together the WILLOW and THOTH project-teams with MSR researchers in Cambridge and elsewhere. The concept builds on several ideas articulated in the 2020 Sciencea report, including the importance of data mining and machine learning in computational science. Rather than focusing only on natural sciences, however, we propose here to expand the breadth of e-science to include humanities and social sciences. The project we propose will focus on fundamental computer science research in computer vision and machine learning, and its application to archaeology, cultural heritage preservation, environmental science, and sociology, and it will be validated by collaborations with researchers and practitioners in these fields.
In 2018 a new agreement has been signed with a new focus on video understanding for personal assistants. The scientific objectives are to develop models, representations and learning algorithms for (i) automatic understanding of task-driven complex human activities from videos narrated with natural language in order to (ii) give people instructions in a new environment via an augmented reality device such as the Microsoft HoloLens. Besides the clear scientific interest of automatically understanding human activities in video streams, the main high-impact motivation of this project it to develop virtual assistants that may guide a child through simple games to improve his/her manipulation and language skills; help an elderly person to achieve everyday tasks; or facilitate the training of a new worker for highly-specialized machinery maintenance.
8.1.2 Louis Vuitton/ENS chair on artificial intelligence
Participants: Ivan Laptev, Jean Ponce, Josef Sivic.
The scientific chair Louis Vuitton - École normale supérieure in Artificial Intelligence has been created in 2017 and inaugurated on April 12, 2018 by the ENS Director Marc Mézard and the LV CEO Michael Burke. The goal of the chair is to establish a close collaboration between LV and ENS in the area of Artificial Intelligence. The chair enjoys the generous annual contribution of 200K Euros provided by LV in support of research activities in statistical learning and computer vision. In particular, the chair supports the costs of researchers, students, missions, computational resources as well as seminars and meetings, including the two days of meeting annually organized by LV and ENS. During 2020 ENS and LV have organized several joint meetings with the participation of researchers from SIERRA and WILLOW teams. The chair has also supported the hiring of one PhD student at the WILLOW team, missions to conferences and international research labs as well as data collection for research projects. In 2020 the chair has been extended to the next three-year period until 2023.
8.2 Bilateral grants with industry
8.2.1 Facebook AI Research Paris: Weakly-supervised interpretation of image and video data (Inria)
Participants: Jean Ponce, Minsu Cho, Ivan Laptev, Josef Sivic.
We will develop in this project (Facebook gift) new models of image and video content, as well as new recognition architectures and algorithms, to address the problem of understanding the visual content of images and videos using weak forms of supervision, such as the fact that multiple images contain instances of the same objects, or the textual information available in television or film scripts.
8.2.2 Google: Multimodal video representation with cross-modal learning (Inria)
Participants: Ivan Laptev.
The proposed project (Google gift) aims to learn a detailed correspondence between the text and the visual content of the video from large-scale unlabeled video collections. It will significantly extend current representations which rely on frame/clip based features and at best learn correlation based on transformers, but fail to provide the in-depth understanding of spatial and temporal structure of the visual content in the video. This will enable advanced multimodal video representations and hence will improve downstream tasks such as video captioning, search and summarization. The main challenge of the project is to build new state-of-the-art models and methods for self-supervised learning based on large-scale but imprecise textual information obtained from video transcripts and other video metadata. The project includes the collection of a dataset allowing a detailed analysis of the visual representation by extending the HowTo100Million dataset with manual annotations.
8.2.3 Google: Structured learning from video and natural language (Inria)
Participants: Simon Lacoste-Julien, Ivan Laptev, Josef Sivic.
People can easily learn how to change a flat tire of a car or assemble an IKEA shelve by observing other people doing the same task, for example, by watching a narrated instruction video. In addition, they can easily perform the same task in a different context, for example, at their home. This involves advanced visual intelligence abilities such as recognition of objects and their function as well as interpreting sequences of human actions that achieve a specific task. However, currently there is no artificial system with a similar cognitive visual competence. The goal of this proposal is to develop models, representations and learning algorithms for automatic understanding of complex human activities from videos narrated with natural language.
9 Partnerships and cooperations
9.1 National initiatives
9.1.1 PRAIRIE
Participants: Ivan Laptev, Jean-Paul Laumond, Jean Ponce, Josef Sivic, Cordelia Schmid.
The Prairie Institute (PaRis AI Research InstitutE) is one of the four French Institutes for Interdisciplinary Artificial Intelligence Research (3IA), which were created as part of the national French initiative on AI announced by President Emmanuel Macron on May 29, 2018. It brings together five academic partners (CNRS, Inria, Institut Pasteur, PSL University, and University of Paris) as well as 17 industrial partners, large corporations which are major players in AI at the French, European and international levels, as well as 45 Chair holders, including four of the members of WILLOW (Laumond, Laptev, Ponce, Sivic). Ponce is the scientific director of PRAIRIE.
9.1.2 DGA - RAPID project DRAAF
Participants: Ivan Laptev.
DGA DRAAF is a two-year collaborative effort with University of Caen (F. Jurie) and the industrial partner EVITECH (P. Bernas) focused on modelling and recognition of violent behaviour in surveillance videos. The project aims to develop image recognition models and algorithms to automatically detect weapons, gestures and actions using recent advances in computer vision and deep learning to provide an affordable real-time solution reducing effects of threats in public places.
9.2 European initiatives
9.2.1 IMPACT: Intelligent machine perception
Participants: Josef Sivic, Jean Ponce, Ivan Laptev.
IMPACT is a 5-year collaborative project with Czech Technical University, Center for Robotics, Informatics and Cybernetics (CIIRC) (2017-2022). The IMPACT project focuses on fundamental and applied research in computer vision, machine learning and robotics to develop machines that learn to perceive, reason, navigate and interact with complex dynamic environments. For example, people easily learn how to change a flat tire of a car or perform resuscitation by observing other people doing the same task. This involves advanced visual intelligence abilities such as interpreting sequences of human actions that manipulate objects to achieve a specific task. Currently, however, there is no artificial system with a similar level of cognitive visual competence. Breakthrough progress in intelligent machine perception will have profound implications on our everyday lives as well as science and commerce, with smart assistive robots that automatically learn new skills from the Internet, safer cars that autonomously navigate in difficult changing conditions, or intelligent glasses that help people navigate never seen before environments.
9.3 International initiatives
9.3.1 Associate team GAYA
Participants: Jean Ponce, Cordelia Schmid.
GAYA is a joint research team bringing together two Inria project-teams (Thoth, Grenoble and WILLOW, Paris) and Carnegie Mellon University, USA. It focuses on two research themes: (i) semantic structured interpretation of videos, and (ii) studying the geometric properties of object shapes to enhance state-of-the-art object recognition approaches.
Interpreting videos semantically in a general setting, involving various types of video content like home video clips, news broadcasts, feature films, which contain a lot of clutter, non-rigid motion, many “actors” performing actions, person-object and person-person interactions, varying viewpoints, is challenging. This task is being examined increasingly over the past decade, with the availability of large video resources, e.g., YouTube. Despite this progress, an effective video representation for recognizing actions is still missing. To address this critical challenge, we propose a joint optimization framework, wherein we learn the video representation and also develop models for action recognition. Specifically, we aim to exploit the spatio-temporal relations among pixels in a video through graphical models and novel deep learning feature representations.
The second research theme explores geometric aspects of computer vision, in particular how to model three-dimensional objects from their two-dimensional projections, and how the appearance of these objects evolves with changes in viewpoint. Beyond its theoretical interest, this work is critical for developing object recognition algorithms that take into account the three-dimensional nature of the visual world and go beyond the template-matching approaches dominant today. Duality is an important concept in this area, and we are investigating its application to the construction of visual hulls as well as the characterization of the topology of image contours using the Gauss map. Existing results are essentially limited to the Euclidean setting, and we are investigating their generalization to the general projective case.
Partners: CMU (Deva Ramanan, Martial Hebert, Abhinav Gupta, Gunnar Sigurdsson), INRIA Thoth (Karteek Alahari, Pavel Tokmakov).
9.4 International research visitors
Most of our international visits in 2020 have been cancelled due to the COVID-19 pandemic. We have nevertheless continued close remote collaboration with universities and companies including CTU in Prague (J. Sivic), DeepMind in London (J.-B. Alayrac, A. Zisserman), POSTECH in Pohang (M. Cho), Xi’an Jiaotong University in Xi’an (J. Sun) and Yonsei University in Seoul (B. Ham, B. Kim). Moreover, J. Ponce spends most of his time at New York University.
10 Dissemination
10.1 Promoting scientific activities
10.1.1 Scientific events: organisation
- I. Laptev was co-organizer of Machines Can See on-line summit on computer vision and machine learning, June 8–10, 2020.
General chair, scientific chair
- C. Schmid was general chair ECCV 2020.
10.1.2 Scientific events: selection
Area chairs
- IEEE Conference on Computer Vision and Pattern Recognition (CVPR), 2020 (I. Laptev, J. Ponce).
- European Conference on Computer Vision (ECCV), 2020 (I. Laptev, J. Ponce).
- Neural Information Processing Systems (NeurIPS), 2020 (J. Sivic, C. Schmid).
- Asian Conference on Computer Vision (ECCV), 2020 (I. Laptev).
- International Conference on Intelligent Robots and Systems (IROS), Associate Editor, 2020 (J. Carpentier)
Member of the Conference Program Committees / Reviewer
- European Conference on Computer Vision (ECCV), 2020 (J. Sivic, I. Rocco).
- IEEE Conference on Computer Vision and Pattern Recognition (CVPR), 2020 (J. Sivic, I. Rocco, Y. Hasson)
- Neur l Information Processing Systems (NeurIPS), 2020 (J. Carpentier, I. Rocco)
- International Conference on Robotics and Automation (ICRA), 2020 (J. Carpentier)
- International Conference on Intelligent Robots and Systems (IROS), 2020 (J. Carpentier)
- Robotics: Science and Systems (RSS), 2020 (J. Carpentier)
10.1.3 Journal
Member of the editorial boards
- International Journal of Computer Vision (I. Laptev, J. Ponce, J. Sivic).
- Foundations and Trends in Computer Graphics and Vision (J. Ponce).
Reviewer - reviewing activities
- IEEE Transactions on Robotics (TRO) (J. Carpentier)
- IEEE Robotics and Automation Letters (RAL) (J. Carpentier)
10.1.4 Invited talks
- I. Laptev, Invited talk, Learning from Unlabeled Videos workshop at CVPR 2020, June 2020 (virtual).
- I. Laptev, Invited talk, Compositional and Multimodal Perception workshop at ECCV 2020, August 2020 (virtual).
- I. Laptev, Invited talk, AI Journay, Moscow, December 3 (virtual).
- J.P. Laumond, Plenary Speaker, IEEE International Conference on Robotics and Automation (ICRA), May 2020 (virtual)
- J. Sivic, Invited talk, Machines that See, Moscow, June 2020 (virtual).
- J. Sivic, Invited talk, Romanian AI days, December 2020 (virtual).
- J. Sivic, Invited talk, Technological Agency of the Czech Republic.
- J. Sivic, Invited talk, GDR Machine learning and Robotics, June 2020 (virtual).
- J. Sivic, Invited talk, ActivityNet workshop at CVPR 2020, June 2020 (virtual).
- J. Ponce, Keynote speaker, Chinese Conference on Pattern Recognition and Computer Vision (Oct. 2020) (virtual).
- J. Ponce, Invited speaker, World AI Conference (July 2020) (virtual).
- J. Ponce, Invited speaker, ELLIS Workshop (July 2020) (virtual).
- J. Ponce, Invited speaker, ONERA (March 2020) (virtual).
- J. Ponce, Invited speaker, University of California at San Diego (Feb. 2020).
- J. Carpentier, Invited talk, Journées Nationales de la Robotique Humanoïde, GDR Robotique, June 2020 (virtual).
- C. Schmid, Keynote speaker at France is AI, November 2020.
- C. Schmid, Invited speaker at Tracking and its many Guises Workshop, in conjunction with ECCV,virtual, August 2020.
- C. Schmid, Invited speaker at Multi-modal Video Analysis Workshop, in conjunction with ECCV, virtual, August 2020.
- C. Schmid, Keynote speaker at Computer Vision and Deep Learning Summit “Machines Can See”, virtual, June 2020.
- C. Schmid, Keynote speaker at GdR ISIS, virtual, June 2020.
- C. Schmid, Keynote speaker at ICRA, virtual, June 2020.
- C. Schmid, Keynote speaker at Applied Machine Learning Days, Imaging Track, Lausanne, January 2020.
- C. Schmid, Talk at inaugural Bell Labs webinar on Prairie research, December 2020.
- Y. Hasson, Talk at AI in Robotics Toronto Reading group, University of Toronto, September 2020.
10.1.5 Leadership within the scientific community
- Member, ECCV awards committee (I. Laptev).
- Member, the steering committee of France AI (J. Ponce).
- Member, advisory board, Computer Vision Foundation (J. Sivic).
- Board Member Deputy, European Laboratory for Learning and Intelligent Systems (J. Sivic).
- Member of the Inria-académie des sciences award committe (S. Schmid).
10.1.6 Scientific expertise
- J. Ponce, coordinator of the AI theme for the joint French-American Committee on Science and Technology, 2018–.
- I. Laptev, head of scientific board at VisionLabs, 2019–.
10.1.7 Research administration
- Member, Bureau du comité des projets, Inria, Paris (J. Ponce)
- Member, Scientific academic council, PSL Research University (J. Ponce)
- Member, Research representative committee, PSL Research University (J. Ponce).
- Member, INRIA Cordi-S and postdoc selection committee, 2019— (I. Laptev).
- Member, INRIA Commission des emplois scientifiques (CES), 2019— (I. Laptev).
10.2 Teaching - Supervision - Juries
10.2.1 Teaching
- Master: M. Aubry, K. Alahari, I. Laptev and J. Sivic "Introduction to computer vision", M1, Ecole normale supérieure, 36h.
- Master: I. Laptev, J. Ponce, J. Sivic and C. Schmid "Object recognition and computer vision", M2, Ecole normale superieure, and MVA, Ecole normale superieure Paris-Saclay, 36h.
- Master: J-P. Laumond and J. Carpentier, "Robotics", M1 MPRI, Ecole normale supérieure and Ecole normale superieure Paris-Saclay, 48h.
- Master: I. Laptev, "Fundamentals of Machine Learning", Master IASD, PSL University, 9h.
- J. Carpentier co-organized the Memmo Summer School in Toulouse, 2020.
- Master: J-P. Laumond, "Robotics", Ecole des Mines de Paris, 4h.
- Master: J. Sivic, three lectures (3 x 1.5h) in the 3D computer vision class of V. Hlavac at Charles University in Prague.
- License: P.L. Guhur, "Developping web applications with React Js", L3, Université Grenoble Alpes, 30h.
- Bachelor: J. Ponce, "Inroduction to computer vision" MS level class, NYU Center for Data Science, Fall 2019
10.2.2 Supervision
- PhD in progress : Guillaume Le Moing, “Learning robust representations for improved visual understanding”, started in Nov 2020, J. Ponce and C. Schmid.
- PhD in progress : Antoine Bambade, started in Oct. 2020, J. Carpentier, A. Taylor (Sierra) and J. Ponce.
- PhD in progress : Adrien Bardes, started in Oct. 2020, J. Ponce.
- PhD in progress : Oumayma Bounou, started in Oct. 2020, J. Ponce and J. Carpentier.
- PhD in progress : Marie Heurtevent, started in Oct. 2020, J. Ponce.
- PhD in progress : Antoine Yang, “Multimodal video representation with cross-modal learning”, started in Oct. 2020, I. Laptev, C. Schmid, J. Sivic.
- PhD in progress : Elliot Chane-Sane, “Learning long-horizon robotics manipulations”, started in Oct. 2020, I. Laptev and C. Schmid.
- PhD in progress : Yann Dubois De Mont-Marin, started in Sept. 2020, J.-P. Laumond.
- PhD in progress : Alaaeldin Ali, “Object centric visual retrieval in the wild”, started in Aug. 2020, I. Laptev.
- PhD in progress : Vo Van Huy, started in Dec 2018, J. Ponce.
- PhD in progress : Pierre-Louis Guhur, “Learning Visual Language Manipulation”, started in Oct 2019, I. Laptev and C. Schmid.
- PhD in progress : Aamr El Kazdadi, started in Oct 2019, J. Carpentier and J. Ponce.
- PhD in progress : Bruno Lecouat, started in Sept 2019, J. Ponce and J. Mairal (Inria Grenoble).
- PhD in progress : Robin Strudel, “Learning and transferring complex robot skills from human demonstrations”, started in Oct 2018, I. Laptev, C. Schmid and J. Sivic.
- PhD in progress : Yann Labbe, “Generalizing robotic sensorimotor skills to new tasks and environments”, started in Oct 2018, J. Sivic and I. Laptev.
- PhD in progress : Minttu Alakuijala, started in Feb 2019, J. Ponce and C. Schmid.
- PhD in progress : Thomas Eboli, started in Oct 2017, J. Ponce.
- PhD in progress : Zongmian Li, “Learning to manipulate objects from instructional videos”, started in Oct 2017, I. Laptev, J. Sivic and N. Mansard (LAAS/CNRS, Toulouse).
- PhD in progress : Yana Hasson, “Reconstruction and recognition of hand-object manipulations”, started in Nov 2017, I. Laptev and C. Schmid.
- PhD in progress : Alexander Pashevich, “Learning to grasp”, started in Sept 2017, C. Schmid.
- PhD in progress : Ronan Riochet, “Unsupervised Learning of Intuitive Physics from Videos”, started in Oct 2017, E. Dupoux, I. Laptev and J. Sivic.
- PhD in progress : Dmitry Zhukov, “Learning from instruction videos for personal assistants”, started in Oct 2017, I. Laptev and J. Sivic.
- PhD in progress : Ignacio Rocco, “Estimating correspondence between images via convolutional neural networks”, gratuated in Oct. 2020, J. Sivic, R. Arandjelovic (Google DeepMind).
- PhD in progress : Antoine Miech, “Understanding long-term temporal structure of videos”, gratuated in Oct. 2020, I. Laptev, J. Sivic.
10.2.3 Juries
- PhD thesis committee:
- Fabien Baradel, Université de Lyon, 2020 (I. Laptev, rapporteur)
- Rémi Cadène, Sorbonne Université, 2020 (I. Laptev, rapporteur)
- Gunnar Atli Sigurdsson, Carnegie Mellon University (I. Laptev, examiner)
- Oriane SIMEONI, Universite de Rennes 1, 2020 (J. Sivic, rapporteur)
- Daan WYNEN, Universite Grenoble Alpes, 2020 (J. Sivic, rapporteur)
- Hazel DOUGHTY, University of Bristol, 2020 (J. Sivic, examiner)
- Arun MUKUNDAN, Czech Technical University, 2020 (J. Sivic, member of the thesis jury)
- Milan SULC, Czech Technical University, 2020 (J. Sivic, member of the thesis jury)
- Melia Boukheddimi, Université de Toulouse, 2020 (J. Carpentier, examinateur)
10.3 Popularization
10.3.1 Internal or external Inria responsibilities
J.P. Laumond is the scientific curator of the permanent exhibition at Cité des Sciences et de l'Industrie. The aim of this exhibition is to help the general audience to understand the concepts of robotics. Indeed, the notion of robotics today is packed with many preconceived notions, phobias, and utopias, all fed by literature and a rich film culture. The real challenge of the exhibition is the presentation of authentic working robots that raises awareness of our relationship to these singular machines. How do they work? What are they for? What are their performances today and what will they be tomorrow? The exhibition lays bare the actual capabilities of robots and provides insight into the current issues.
11 Scientific production
11.1 Publications of the year
International journals
International peer-reviewed conferences
Doctoral dissertations and habilitation theses
Reports & preprints