Keywords
Computer Science and Digital Science
- A3.3. Data and knowledge analysis
- A3.3.2. Data mining
- A3.3.3. Big data analysis
- A3.4. Machine learning and statistics
- A3.4.1. Supervised learning
- A3.4.2. Unsupervised learning
- A3.4.4. Optimization and learning
- A3.4.5. Bayesian methods
- A3.4.6. Neural networks
- A3.4.7. Kernel methods
- A3.4.8. Deep learning
- A5.3.2. Sparse modeling and image representation
- A5.3.3. Pattern recognition
- A5.9.1. Sampling, acquisition
- A5.9.2. Estimation, modeling
- A5.9.3. Reconstruction, enhancement
- A5.9.6. Optimization tools
- A6.2.4. Statistical methods
- A6.2.6. Optimization
- A9.2. Machine learning
- A9.3. Signal analysis
- A9.7. AI algorithmics
Other Research Topics and Application Domains
- B1.2. Neuroscience and cognitive science
- B1.2.1. Understanding and simulation of the brain and the nervous system
- B1.2.2. Cognitive science
- B2.2.6. Neurodegenerative diseases
- B2.6.1. Brain imaging
1 Team members, visitors, external collaborators
Research Scientists
- Bertrand Thirion [Team leader, Inria, Senior Researcher, HDR]
- Philippe Ciuciu [CEA, Researcher, HDR]
- Benedicte Colnet [Inria, Researcher, from Sep 2020]
- Denis Alexander Engemann [Inria, Starting Research Position]
- Alexandre Gramfort [Inria, Senior Researcher, HDR]
- Thomas Moreau [Inria, Researcher]
- Gaël Varoquaux [Inria, Senior Researcher, HDR]
- Demian Wassermann [Inria, Researcher, HDR]
Faculty Members
- Anna Kazeykina [Univ Paris-Saclay, Associate Professor, until Jun 2020]
- Matthieu Kowalski [Univ Paris-Saclay, Associate Professor, from Sep 2020]
Post-Doctoral Fellows
- Judith Abecassis [Inria, from Jul 2020]
- Pedro Luiz Coelho Rodrigues [Inria, from Mar 2020]
- Kamalaker Reddy Dadi [Inria, from Oct 2020]
- Marine Le Morvan [CNRS]
- Cédric Rommel [Inria, from Dec 2020]
PhD Students
- Thomas Bazeille [Inria]
- Quentin Bertrand [Inria]
- Charlotte Caucheteux [Facebook, CIFRE, from Nov 2020]
- L Emir Omar Chehab [Inria, from Nov 2020]
- Hamza Cherkaoui [CEA]
- Jerome Alexis Chevalier [Inria]
- Alexis Cvetkov-Iliev [Inria]
- Kamalaker Reddy Dadi [Inria, until Sep 2020]
- Guillaume Daval-Frerot [CEA]
- Merlin Dumeur [Univ Paris-Saclay, from Dec 2020]
- Chaithya Giliyar Radhkrishna [CEA, from Jul 2020]
- Valentin Iovene [Inria]
- Hubert Jacob Banville [Interaxon Inc]
- Maeliss Jallais [Inria]
- Hicham Janati [Inria]
- Antonia Machlouzarides Shalit [Inria]
- Benoit Malezieux [Inria, from Oct 2020]
- Lucas Martin [Inria, until Apr 2020]
- Tuan Binh Nguyen [Inria]
- Alexandre Pasquiou [Inria, from Mar 2020]
- Nicolas Prost [École polytechnique, until Jan 2020]
- Zaccharie Ramzi [CEA]
- Hugo Richard [Univ Paris-Saclay]
- Louis Rouillard–Odera [Inria, from Sep 2020]
- David Sabbagh [INSERM]
- Badr Tajini [Inria, from Mar 2020 until Nov 2020]
- Alexis Thual [CEA, from Oct 2020]
- Gaston Zanitti [Inria]
Technical Staff
- Alexandre Abadie [Inria, Engineer]
- Himanshu Aggarwal [Inria, Engineer, from Dec 2020]
- Kshitij Chawla [Inria, Engineer, until Mar 2020]
- L Emir Omar Chehab [Inria, Engineer, until Oct 2020]
- Merlin Dumeur [École Normale Supérieure de Lyon, Engineer, until Nov 2020]
- Loïc Estève [Inria, Engineer, from Dec 2020]
- Guillaume Favelier [Inria, Engineer]
- Nicolas Gensollen [Inria, Engineer, from Nov 2020]
- Chaithya Giliyar Radhkrishna [CEA, Engineer, until Jul 2020]
- Ana Luisa Grilo Pinho [Inria, Engineer]
- Olivier Grisel [Inria, Engineer]
- Benjamin Habert [Inria, Engineer, from Dec 2020]
- Richard Höchenberger [Inria, Engineer, from Mar 2020]
- Guillaume Lemaitre [Inria, Engineer]
- Jiaping Liu [Inria, Engineer, until Nov 2020]
- Chiara Marmo [Inria, Engineer]
- Thomas Schmitt [Inria, Engineer, until Oct 2020]
- Swetha Shankar [Inria, Engineer]
- Maria Telenczuk [Inria, Engineer]
- Juan Jesus Torre Tresols [Inria, Engineer, until Aug 2020]
- Jérémie du Boisberranger [Inria, Engineer]
Interns and Apprentices
- Cedric Allain [Inria, from Jul 2020 until Nov 2020]
- Lilian Boulard [Inria, Apprentice, from Oct 2020]
- Benedicte Colnet [Mines ParisTech, from Feb 2020 until Jun 2020]
- Tomas D'amelio [Inria, from Dec 2020]
- Simon Freyburger [Inria, from May 2020 until Nov 2020]
- Benoit Malezieux [Inria, from Apr 2020 until Oct 2020]
- Manon Rivoire [Inria, from May 2020 until Oct 2020]
- Nikita Zdanovitch [Inria, from Mar 2020 until Aug 2020]
Administrative Assistant
- Corinne Petitot [Inria]
External Collaborators
- Danilo Bzdok [McGill University - Canada, until Jun 2020]
- Pierre Glaser [École Normale Supérieure de Cachan]
- Sofiane Mrah [Institut du Cerveau et de la Moelle Epinière, from Oct 2020]
- Joseph Salmon [Institut Telecom ex GET Groupe des Écoles des Télécommunications , HDR]
2 Overall objectives
The Parietal team focuses on mathematical methods for modeling and statistical inference based on neuroimaging data, with a particular interest in machine learning techniques and applications of human functional imaging. This general theme splits into four research axes:
- Modeling for neuroimaging population studies,
- Encoding and decoding models for cognitive imaging,
- Statistical and machine learning methods for large-scale data,
- Compressed-sensing for MRI.
Parietal is also strongly involved in open-source software development in scientific Python (machine learning) and for neuroimaging applications.
3 Research program
3.1 Inverse problems in Neuroimaging
Many problems in neuroimaging can be framed as forward and inverse problems. For instance, brain population imaging is concerned with the inverse problem that consists in predicting individual information (behavior, phenotype) from neuroimaging data, while the corresponding forward problem boils down to explaining neuroimaging data with the behavioral variables. Solving these problems entails the definition of two terms: a loss that quantifies the goodness of fit of the solution (does the model explain the data well enough?), and a regularization scheme that represents a prior on the expected solution of the problem. These priors can be used to enforce some properties on the solutions, such as sparsity, smoothness or being piece-wise constant.
Let us detail the model used in typical inverse problem: Let
where the vector contains
where
with
- When
only (LASSO), and to some extent, when only (elastic net), the optimal solution is (possibly very) sparse, but may not exhibit a proper image structure; it does not fit well with the intuitive concept of a brain map. - Total Variation regularization (see Fig. 1) is obtained for
(
only), and typically yields a piece-wise constant solution. It can be associated with Lasso to enforce both sparsity and sparse variations. - Smooth lasso is obtained with (
and only), and yields smooth, compactly supported spatial basis functions.
Note that, while the qualitative aspect of the solutions are very different, the predictive power of these models is often very close.
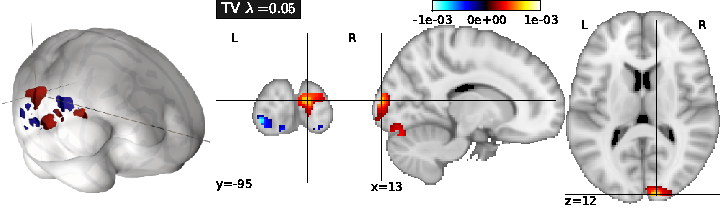
The performance of the predictive model can simply be evaluated as the
amount of variance in
This framework is easily extended by considering
- Grouped penalization, where the penalization explicitly includes a prior clustering of the features, i.e. voxel-related signals, into given groups. This amounts to enforcing structured priors on the solution.
- Combined penalizations, i.e. a mixture of simple and group-wise penalizations, that allow some variability to fit the data in different populations of subjects, while keeping some common constraints.
- Logistic and hinge regression, where a non-linearity is applied to the linear model so that it yields a probability of classification in a binary classification problem.
-
Robustness to between-subject variability to avoid the
learned model overly reflecting a few outlying particular observations
of the training set. Note that noise and deviating assumptions can be
present in both
and -
Multi-task learning: if several target variables are thought to be related, it might be useful to constrain the estimated parameter vector
to have a shared support across all these variables.For instance, when one of the variables
is not well fitted by the model, the estimation of other variables may provide constraints on the support of and thus, improve the prediction of . 3then
4
3.2 Multivariate decompositions
Multivariate decompositions provide a way to model complex data such as brain activation images: for instance, one might be interested in extracting an atlas of brain regions from a given dataset, such as regions exhibiting similar activity during a protocol, across multiple protocols, or even in the absence of protocol (during resting-state). These data can often be factorized into spatial-temporal components, and thus can be estimated through regularized Principal Components Analysis (PCA) algorithms, which share some common steps with regularized regression.
Let
where
where
The problem is not jointly convex in all the variables but each
penalization given in Eq (2) yields a convex problem on
Ultimately, the main limitations to these algorithms is the cost due to the memory requirements: holding datasets with large dimension and large number of samples (as in recent neuroimaging cohorts) leads to inefficient computation. To solve this issue, online methods are particularly attractive 1.
3.3 Covariance estimation
Another important estimation problem stems from the general issue of learning the relationship between sets of variables, in particular their covariance. Covariance learning is essential to model the dependence of these variables when they are used in a multivariate model, for instance to study potential interactions among them and with other variables. Covariance learning is necessary to model latent interactions in high-dimensional observation spaces, e.g. when considering multiple contrasts or functional connectivity data.
The difficulties are two-fold: on the one hand, there is a shortage of data to learn a good covariance model from an individual subject, and on the other hand, subject-to-subject variability poses a serious challenge to the use of multi-subject data. While the covariance structure may vary from population to population, or depending on the input data (activation versus spontaneous activity), assuming some shared structure across problems, such as their sparsity pattern, is important in order to obtain correct estimates from noisy data. Some of the most important models are:
- Sparse Gaussian graphical models, as they express meaningful conditional independence relationships between regions, and do improve conditioning/avoid overfit.
- Decomposable models, as they enjoy good computational properties and enable intuitive interpretations of the network structure. Whether they can faithfully or not represent brain networks is still an open question.
- PCA-based regularization of covariance which is powerful when modes of variation are more important than conditional independence relationships.
Adequate model selection procedures are necessary to achieve the right level of sparsity or regularization in covariance estimation; the natural evaluation metric here is the out-of-sample likelihood of the associated Gaussian model. Another essential remaining issue is to develop an adequate statistical framework to test differences between covariance models in different populations. To do so, we consider different means of parametrizing covariance distributions and how these parametrizations impact the test of statistical differences across individuals.
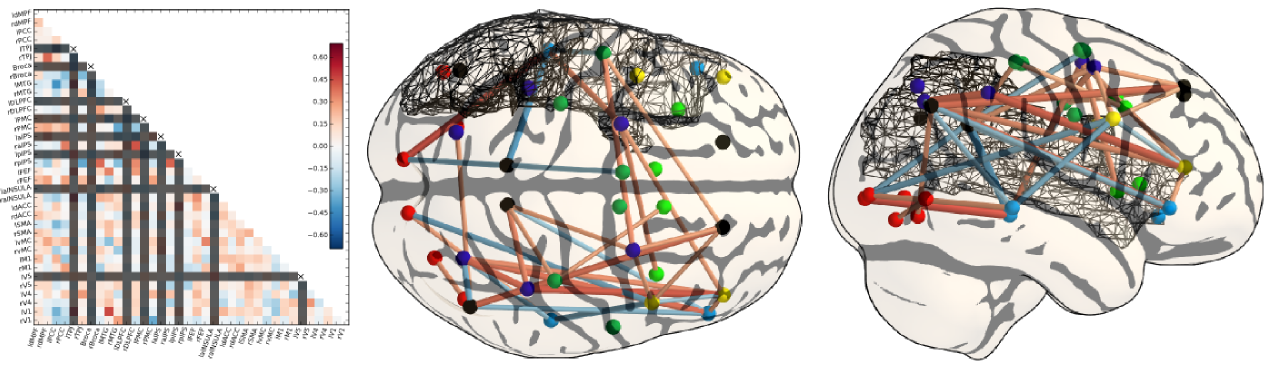
4 Application domains
4.1 Cognitive neuroscience
Macroscopic Functional cartography with functional Magnetic Resonance Imaging (fMRI)
The brain as a highly structured organ, with both functional specialization and a complex network organization. While most of the knowledge historically comes from lesion studies and animal electophysiological recordings, the development of non-invasive imaging modalities, such as fMRI, has made it possible to study routinely high-level cognition in humans since the early 90's. This has opened major questions on the interplay between mind and brain , such as: How is the function of cortical territories constrained by anatomy (connectivity) ? How to assess the specificity of brain regions ? How can one characterize reliably inter-subject differences ?
Analysis of brain Connectivity
Functional connectivity is defined as the interaction structure that underlies brain function. Since the beginning of fMRI, it has been observed that remote regions sustain high correlation in their spontaneous activity, i.e. in the absence of a driving task. This means that the signals observed during resting-state define a signature of the connectivity of brain regions. The main interest of resting-state fMRI is that it provides easy-to-acquire functional markers that have recently been proved to be very powerful for population studies.
Modeling of brain processes (MEG)
While fMRI has been very useful in defining the function of regions at the mm scale, Magneto-encephalography (MEG) provides the other piece of the puzzle, namely temporal dynamics of brain activity, at the ms scale. MEG is also non-invasive. It makes it possible to keep track of precise schedule of mental operations and their interactions. It also opens the way toward a study of the rhythmic activity of the brain. On the other hand, the localization of brain activity with MEG entails the solution of a hard inverse problem.
Current challenges in human neuroimaging (acquisition+analysis)
Human neuroimaging targets two major goals: i) the study of neural responses involved in sensory, motor or cognitive functions, in relation to models from cognitive psychology, i.e. the identification of neurophysiological and neuroanatomical correlates of cognition; ii) the identification of markers in brain structure and function of neurological or psychiatric diseases. Both goals have to deal with a tension between
- the search for higher spatial 1 resolution to increase spatial specificity of brain signals, and clarify the nature (function and structure) of brain regions. This motivates efforts for high-field imaging and more efficient acquisitions, such as compressed sensing schemes, as well as better source localization methods from M/EEG data.
- the importance of inferring brain features with population-level validity, hence, contaminated with high variability within observed cohorts, which blurs the information at the population level and ultimately limits the spatial resolution of these observations.
Importantly, the signal-to-noise ratio (SNR) of the data remains limited due to both resolution improvements 2 and between-subject variability. Altogether, these factors have led to realize that results of neuroimaging studies were statistically weak, i.e. plagued with low power and leading to unreliable inference 79, and particularly so due to the typically number of subjects included in brain imaging studies (20 to 30, this number tends to increase 80): this is at the core of the neuroimaging reproducibility crisis. This crisis is deeply related to a second issue, namely that only few neuroimaging datasets are publicly available, making it impossible to re-assess a posteriori the information conveyed by the data. Fortunately, the situation improves, lead by projects such as NeuroVault or OpenfMRI. A framework for integrating such datasets is however still missing.
5 Highlights of the year
5.1 Awards
Mathurin Massias got the PGMO PhD Prize for his work “Sparse high dimensional regression in the presence of colored heteroscedastic noise : application to M/EEG source imaging” and implementation of his ideas in CELER https://
6 New software and platforms
6.1 New software
6.1.1 Mayavi
- Functional Description: Mayavi is the most used scientific 3D visualization Python software. Mayavi can be used as a visualization tool, through interactive command line or as a library. It is distributed under Linux through Ubuntu, Debian, Fedora and Mandriva, as well as in PythonXY and EPD Python scientific distributions. Mayavi is used by several software platforms, such as PDE solvers (fipy, sfepy), molecule visualization tools and brain connectivity analysis tools (connectomeViewer).
-
URL:
http://
mayavi. sourceforge. net/ - Contacts: Gaël Varoquaux, Prabhu Ramachandran
6.1.2 Nilearn
- Name: NeuroImaging with scikit learn
- Keywords: Health, Neuroimaging, Medical imaging
- Functional Description: NiLearn is the neuroimaging library that adapts the concepts and tools of scikit-learn to neuroimaging problems. As a pure Python library, it depends on scikit-learn and nibabel, the main Python library for neuroimaging I/O. It is an open-source project, available under BSD license. The two key components of NiLearn are i) the analysis of functional connectivity (spatial decompositions and covariance learning) and ii) the most common tools for multivariate pattern analysis. A great deal of efforts has been put on the efficiency of the procedures both in terms of memory cost and computation time.
-
URL:
http://
nilearn. github. io/ - Contacts: Gaël Varoquaux, Bertrand Thirion
- Participants: Alexandre Abraham, Alexandre Gramfort, Bertrand Thirion, Elvis Dohmatob, Fabian Pedregosa Izquierdo, Gaël Varoquaux, Loïc Estève, Michael Eickenberg, Virgile Fritsch
6.1.3 Scikit-learn
- Keywords: Regession, Clustering, Learning, Classification, Medical imaging
- Scientific Description: Scikit-learn is a Python module integrating classic machine learning algorithms in the tightly-knit scientific Python world. It aims to provide simple and efficient solutions to learning problems, accessible to everybody and reusable in various contexts: machine-learning as a versatile tool for science and engineering.
-
Functional Description:
Scikit-learn can be used as a middleware for prediction tasks. For example, many web startups adapt Scikitlearn to predict buying behavior of users, provide product recommendations, detect trends or abusive behavior (fraud, spam). Scikit-learn is used to extract the structure of complex data (text, images) and classify such data with techniques relevant to the state of the art.
Easy to use, efficient and accessible to non datascience experts, Scikit-learn is an increasingly popular machine learning library in Python. In a data exploration step, the user can enter a few lines on an interactive (but non-graphical) interface and immediately sees the results of his request. Scikitlearn is a prediction engine . Scikit-learn is developed in open source, and available under the BSD license.
-
URL:
http://
scikit-learn. org - Contacts: Bertrand Thirion, Olivier Grisel
- Participants: Alexandre Gramfort, Bertrand Thirion, Fabian Pedregosa Izquierdo, Gaël Varoquaux, Loïc Estève, Michael Eickenberg, Olivier Grisel
- Partners: CEA, Logilab, Nuxeo, Saint Gobain, Tinyclues, Telecom Paris
6.1.4 MODL
- Name: Massive Online Dictionary Learning
- Keywords: Pattern discovery, Machine learning
- Functional Description: Matrix factorization library, usable on very large datasets, with optional sparse and positive factors.
-
URL:
http://
github. com/ arthurmensch/ modl - Publications: hal-01405058, hal-01431618v3
- Contact: Arthur Mensch
- Participants: Arthur Mensch, Gaël Varoquaux, Bertrand Thirion, Julien Mairal
6.1.5 MNE
- Name: MNE-Python
- Keywords: Neurosciences, EEG, MEG, Signal processing, Machine learning
- Functional Description: Open-source Python software for exploring, visualizing, and analyzing human neurophysiological data: MEG, EEG, sEEG, ECoG, and more.
- Release Contributions: http://martinos.org/mne/stable/whats_new.html
-
URL:
http://
martinos. org/ mne/ - Contact: Alexandre Gramfort
- Partners: HARVARD Medical School, New York University, University of Washington, CEA, Aalto university, Telecom Paris, Boston University, UC Berkeley
6.1.6 Dmipy
- Name: Diffusion MRI Multi-Compartment Modeling and Microstructure Recovery Made Easy
- Keywords: Diffusion MRI, Multi-Compartment Modeling, Microstructure Recovery
- Functional Description: Non-invasive estimation of brain microstructure features using diffusion MRI (dMRI) – known as Microstructure Imaging – has become an increasingly diverse and complicated field over the last decades. Multi-compartment (MC)-models, representing the measured diffusion signal as a linear combination of signal models of distinct tissue types, have been developed in many forms to estimate these features. However, a generalized implementation of MC-modeling as a whole, providing deeper insights in its capabilities, remains missing. To address this fact, we present Diffusion Microstructure Imaging in Python (Dmipy), an open-source toolbox implementing PGSE-based MC-modeling in its most general form. Dmipy allows on-the-fly implementation, signal modeling, and optimization of any user-defined MC-model, for any PGSE acquisition scheme. Dmipy follows a “building block”-based philosophy to Microstructure Imaging, meaning MC-models are modularly constructed to include any number and type of tissue models, allowing simultaneous representation of a tissue's diffusivity, orientation, volume fractions, axon orientation dispersion, and axon diameter distribution. In particular, Dmipy is geared toward facilitating reproducible, reliable MC-modeling pipelines, often allowing the whole process from model construction to parameter map recovery in fewer than 10 lines of code. To demonstrate Dmipy's ease of use and potential, we implement a wide range of well-known MC-models, including IVIM, AxCaliber, NODDI(x), Bingham-NODDI, the spherical mean-based SMT and MC-MDI, and spherical convolution-based single- and multi-tissue CSD. By allowing parameter cascading between MC-models, Dmipy also facilitates implementation of advanced approaches like CSD with voxel-varying kernels and single-shell 3-tissue CSD. By providing a well-tested, user-friendly toolbox that simplifies the interaction with the otherwise complicated field of dMRI-based Microstructure Imaging, Dmipy contributes to more reproducible, high-quality research.
- Authors: Rutger Fick, Demian Wassermann, Rachid Deriche
- Contacts: Rachid Deriche, Demian Wassermann
6.1.7 PySAP
- Name: Python Sparse data Analysis Package
- Keywords: Image reconstruction, Image compression
-
Functional Description:
The PySAP (Python Sparse data Analysis Package, https://github.com/CEA-COSMIC/pysap) open-source image processing software package has been developed for the 3 years between the Compressed Sensing group at Iniria-CEA Parietal team led by Philippe Ciuciu and the CosmoStat team (CEA/IRFU) led by Jean-Luc Statck. It has been developed for the COmpressed Sensing for Magnetic resonance Imaging and Cosmology (COSMIC) project. This package provides a set of flexible tools that can be applied to a variety of compressed sensing and image reconstruction problems in various research domains. In particular, PySAP offers fast wavelet transforms and a range of integrated optimisation algorithms. It also offers a variety of plugins for specific application domains: on top of Pysap-MRI and PySAP-astro plugins, several complementary modules are now in development for electron tomography and electron microscopy for CEA colleagues. In October 2019, PySAP has been released on PyPi (https://pypi.org/project/python-pySAP/, currently version 0.0.3) and in conda (https://anaconda.org/agrigis/python-pysap).
The Pysap-MRI has been advertised through a specific abstract accepted to the next workshop of ISMRM on Data Sampling & Image Reconstruction in late January 2020. It will be presented during a power pitch session together wih an hands-on demo session using JuPyter notebooks.
- Contact: Philippe Ciuciu
- Partner: CEA
7 New results
7.1 Subject‐specific segregation of functional territories based on deep phenotyping
Functional Magnetic Resonance Imaging (fMRI) has opened the possibility to investigate how brain activity is modulated by behavior. Most studies so far are bound to one single task, in which functional responses to a handful of contrasts are analyzed and reported as a group average brain map. Contrariwise, recent data-collection efforts have started to target a systematic spatial representation of multiple mental functions. In this paper, we leverage the Individual Brain Charting (IBC) dataset—a high-resolution task-fMRI dataset acquired in a fixed environment—in order to study the feasibility of individual mapping. First, we verify that the IBC brain maps reproduce those obtained from previous, large-scale datasets using the same tasks. Second, we confirm that the elementary spatial components, inferred across all tasks, are consistently mapped within and, to a lesser extent, across participants. Third, we demonstrate the relevance of the topographic information of the individual contrast maps, showing that contrasts from one task can be predicted by contrasts from other tasks. At last, we showcase the benefit of contrast accumulation for the fine functional characterization of brain regions within a pre-specified network. To this end, we analyze the cognitive profile of functional territories pertaining to the language network and prove that these profiles generalize across participants.
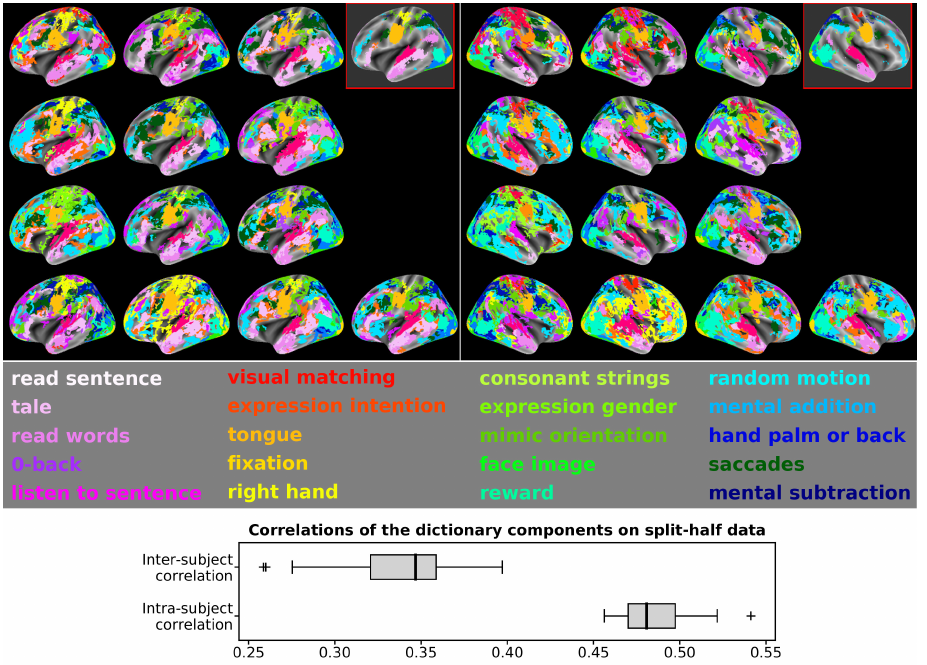
More information can be found in 22.
7.2 Fine-grain atlases of functional modes for fMRI analysis
Population imaging markedly increased the size of functional-imaging datasets, shedding new light on the neural basis of inter-individual differences. Analyzing these large data entails new scalability challenges, computational and statistical. For this reason, brain images are typically summarized in a few signals, for instance reducing voxel-level measures with brain atlases or functional modes. A good choice of the corresponding brain networks is important, as most data analyses start from these reduced signals. We contribute finely-resolved atlases of functional modes, comprising from 64 to 1024 networks. These dictionaries of functional modes (DiFuMo) are trained on millions of fMRI functional brain volumes of total size 2.4TB, spanned over 27 studies and many research groups. We demonstrate the benefits of extracting reduced signals on our fine-grain atlases for many classic functional data analysis pipelines: stimuli decoding from 12,334 brain responses, standard GLM analysis of fMRI across sessions and individuals, extraction of resting-state functional-connectomes biomarkers for 2,500 individuals, data compression and meta-analysis over more than 15,000 statistical maps. In each of these analysis scenarii, we compare the performance of our functional atlases with that of other popular references, and to a simple voxel-level analysis. Results highlight the importance of using high-dimensional "soft" functional atlases, to represent and analyse brain activity while capturing its functional gradients. Analyses on high-dimensional modes achieve similar statistical performance as at the voxel level, but with much reduced computational cost and higher interpretability. In addition to making them available, we provide meaningful names for these modes, based on their anatomical location. It will facilitate reporting of results.
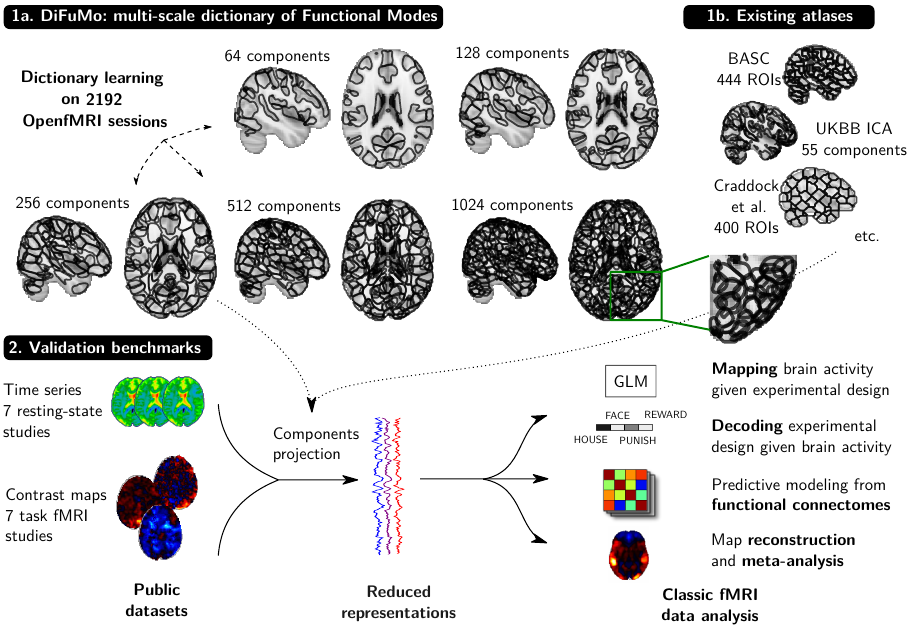
More information can be found in 66.
7.3 Microstructural organization of human insula is linked to its macrofunctional circuitry and predicts cognitive control
The human insular cortex is a heterogeneous brain structure which plays an integrative role in guiding behavior. The cytoarchitectonic organization of the human insula has been investigated over the last century using postmortem brains but there has been little progress in noninvasive in vivo mapping of its microstructure and large-scale functional circuitry. Quantitative modeling of multi-shell diffusion MRI data from 413 participants revealed that human insula microstructure differs significantly across subdivisions that serve distinct cognitive and affective functions. Insular microstructural organization was mirrored in its functionally interconnected circuits with the anterior cingulate cortex that anchors the salience network, a system important for adaptive switching of cognitive control systems. Furthermore, insular microstructural features, confirmed in Macaca mulatta, were linked to behavior and predicted individual differences in cognitive control ability. Our findings open new possibilities for probing psychiatric and neurological disorders impacted by insular cortex dysfunction, including autism, schizophrenia, and fronto-temporal dementia.
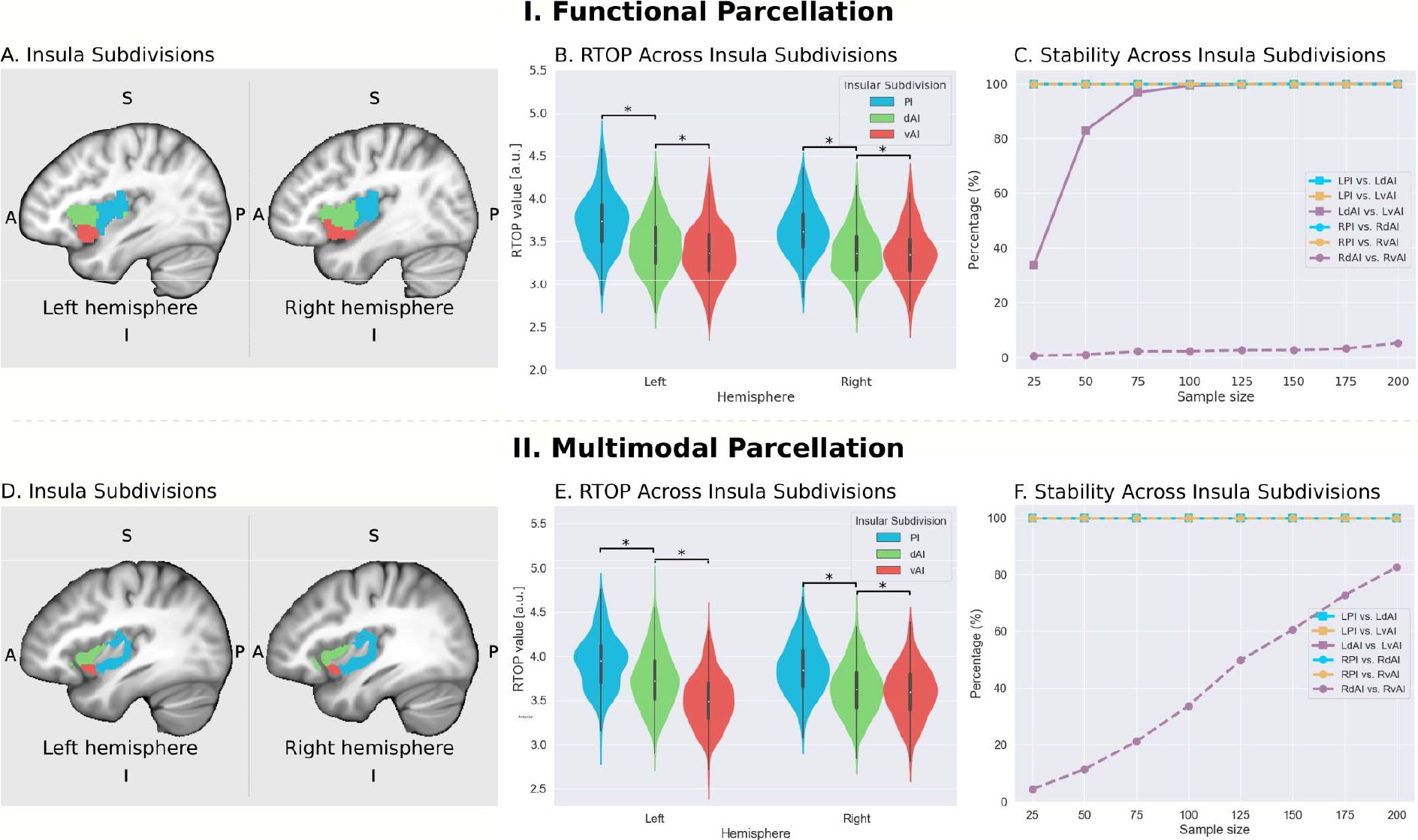
More information can be found in 20.
7.4 Combining magnetoencephalography with magnetic resonance imaging enhances learning of surrogate-biomarkers
Electrophysiological methods, that is M/EEG, provide unique views into brain health. Yet, when building predictive models from brain data, it is often unclear how electrophysiology should be combined with other neuroimaging methods. Information can be redundant, useful common representations of multimodal data may not be obvious and multimodal data collection can be medically contraindicated, which reduces applicability. Here, we propose a multimodal model to robustly combine MEG, MRI and fMRI for prediction. We focus on age prediction as a surrogate biomarker in 674 subjects from the Cam-CAN dataset. Strikingly, MEG, fMRI and MRI showed additive effects supporting distinct brain-behavior associations. Moreover, the contribution of MEG was best explained by cortical power spectra between 8 and 30 Hz. Finally, we demonstrate that the model preserves benefits of stacking when some data is missing. The proposed framework, hence, enables multimodal learning for a wide range of biomarkers from diverse types of brain signals.
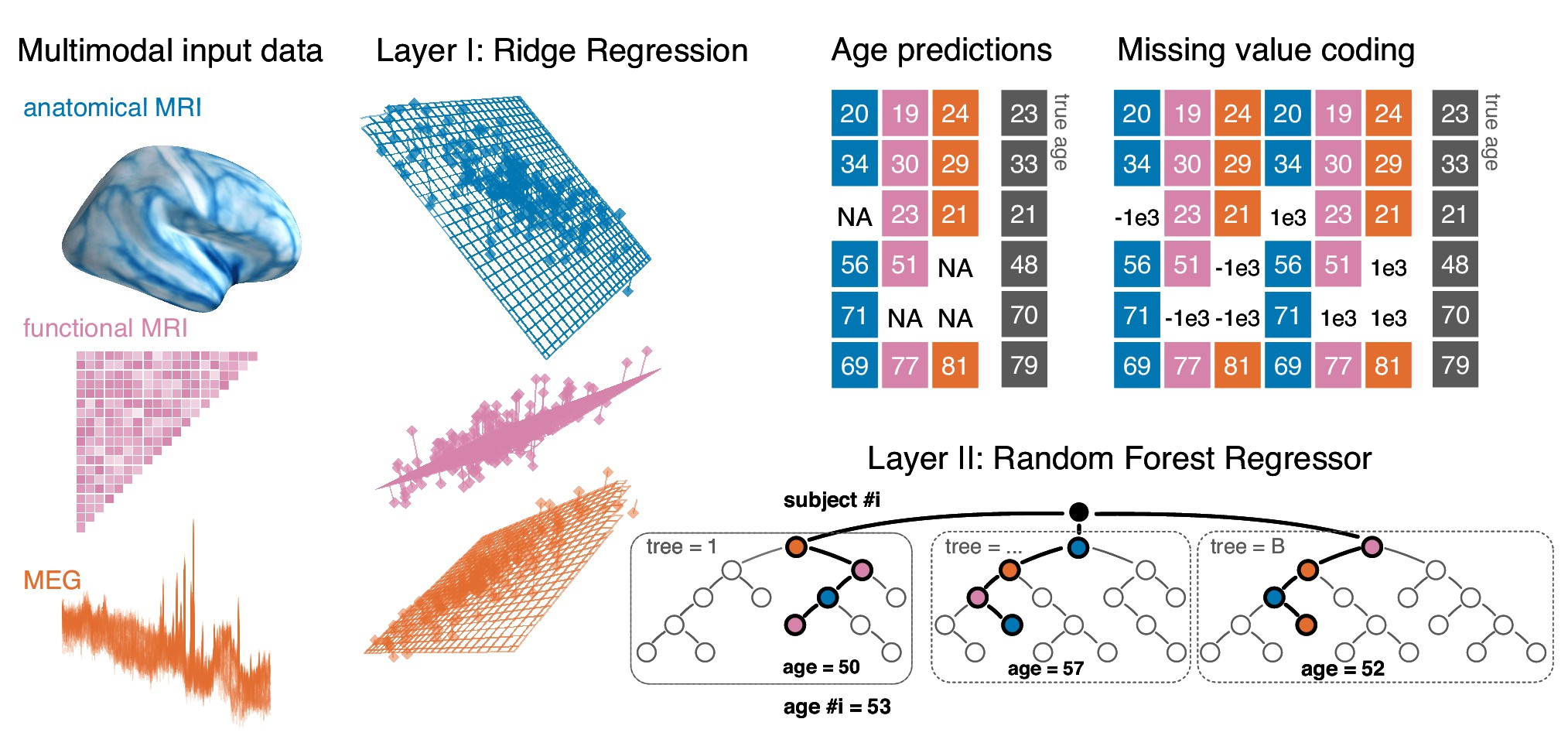
More information can be found in 11.
7.5 3D variable-density SPARKLING trajectories for high-resolution T2 -weighted Magnetic Resonance imaging
We have recently proposed a new optimization algorithm called SPARKLING (Spreading Projection Algorithm for Rapid K-space sampLING) to design efficient Com-pressive Sampling patterns for Magnetic Resonance Imaging. This method has a few advantages over conventional non-Cartesian trajectories such as radial lines or spirals: i) it allows to sample the k-space along any arbitrary density while the other two are restricted to radial densities and ii) it optimizes the gradient waveforms for a given readout time. Here, we introduce an extension of the SPARKLING method for 3D imaging by considering both stacks-of-SPARKLING and fully 3D SPARKLING trajectories. Our method allowed to achieve an isotropic resolution of 600
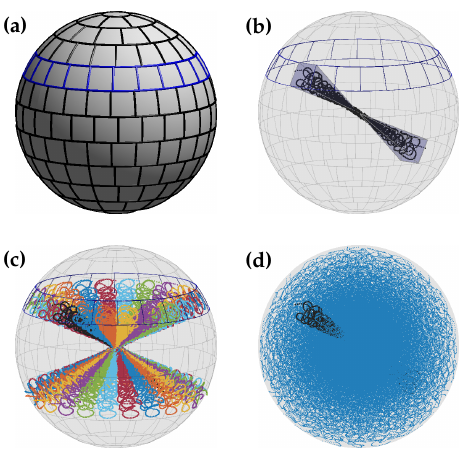
More information can be found in 18.
7.6 Predictive regression modeling with MEG/EEG: from source power to signals and cognitive states
Predicting biomedical outcomes from Magnetoencephalography and Electroencephalography (M/EEG) is central to applications like decoding, brain-computer-interfaces (BCI) or biomarker development and is facilitated by supervised machine learning. Yet, most of the literature is concerned with classification of outcomes defined at the event-level. Here, we focus on predicting continuous outcomes from M/EEG signal defined at the subject-level, and analyze about 600 MEG recordings from Cam-CAN dataset and about 1000 EEG recordings from TUH dataset. Considering different generative mechanisms for M/EEG signals and the biomedical outcome, we propose statistically-consistent predictive models that avoid source-reconstruction based on the covariance as representation. Our mathematical analysis and ground-truth simulations demonstrated that consistent function approximation can be obtained with supervised spatial filtering or by embedding with Riemannian geometry. Additional simulations revealed that Riemannian methods were more robust to model violations, in particular geometric distortions induced by individual anatomy. To estimate the relative contribution of brain dynamics and anatomy to prediction performance, we propose a novel model inspection procedure based on biophysical forward modeling. Applied to prediction of outcomes at the subject-level, the analysis revealed that the Riemannian model better exploited anatomical information while sensitivity to brain dynamics was similar across methods. We then probed the robustness of the models across different data cleaning options. Environmental denoising was globally important but Riemannian models were strikingly robust and continued performing well even without preprocessing. Our results suggest each method has its niche: supervised spatial filtering is practical for event-level prediction while the Riemannian model may enable simple end-to-end learning.
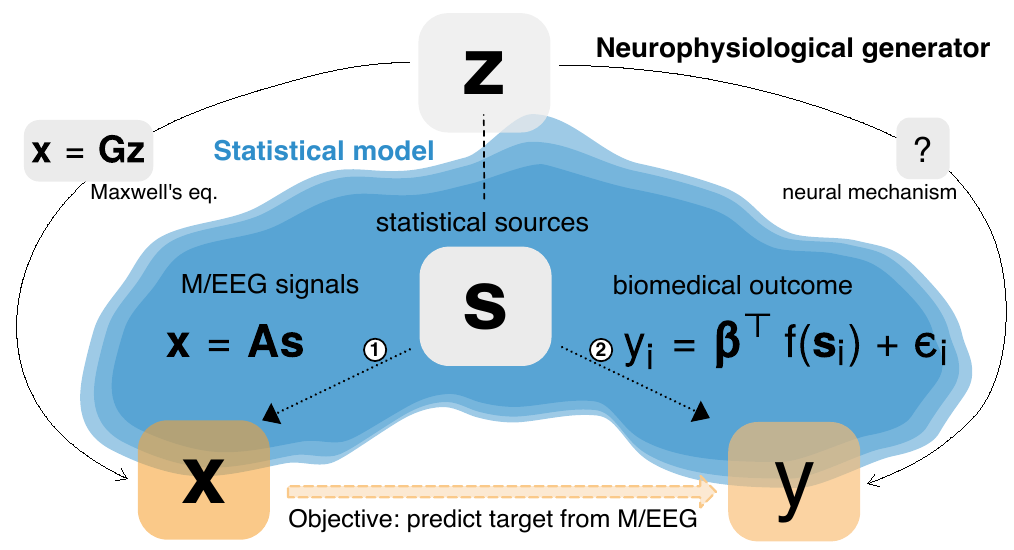
More information can be found in 26.
7.7 NeuroQuery, comprehensive meta-analysis of human brain mapping
Reaching a global view of brain organization requires assembling evidence on widely different mental processes and mechanisms. The variety of human neuroscience concepts and terminology poses a fundamental challenge to relating brain imaging results across the scientific literature. Existing meta-analysis methods perform statistical tests on sets of publications associated with a particular concept. Thus, large-scale meta-analyses only tackle single terms that occur frequently. We propose a new paradigm, focusing on prediction rather than inference. Our multivariate model predicts the spatial distribution of neurological observations, given text describing an experiment, cognitive process, or disease. This approach handles text of arbitrary length and terms that are too rare for standard meta-analysis. We capture the relationships and neural correlates of 7 547 neuroscience terms across 13 459 neuroimaging publications. The resulting meta-analytic tool, neuroquery.org, can ground hypothesis generation and data-analysis priors on a comprehensive view of published findings on the brain.
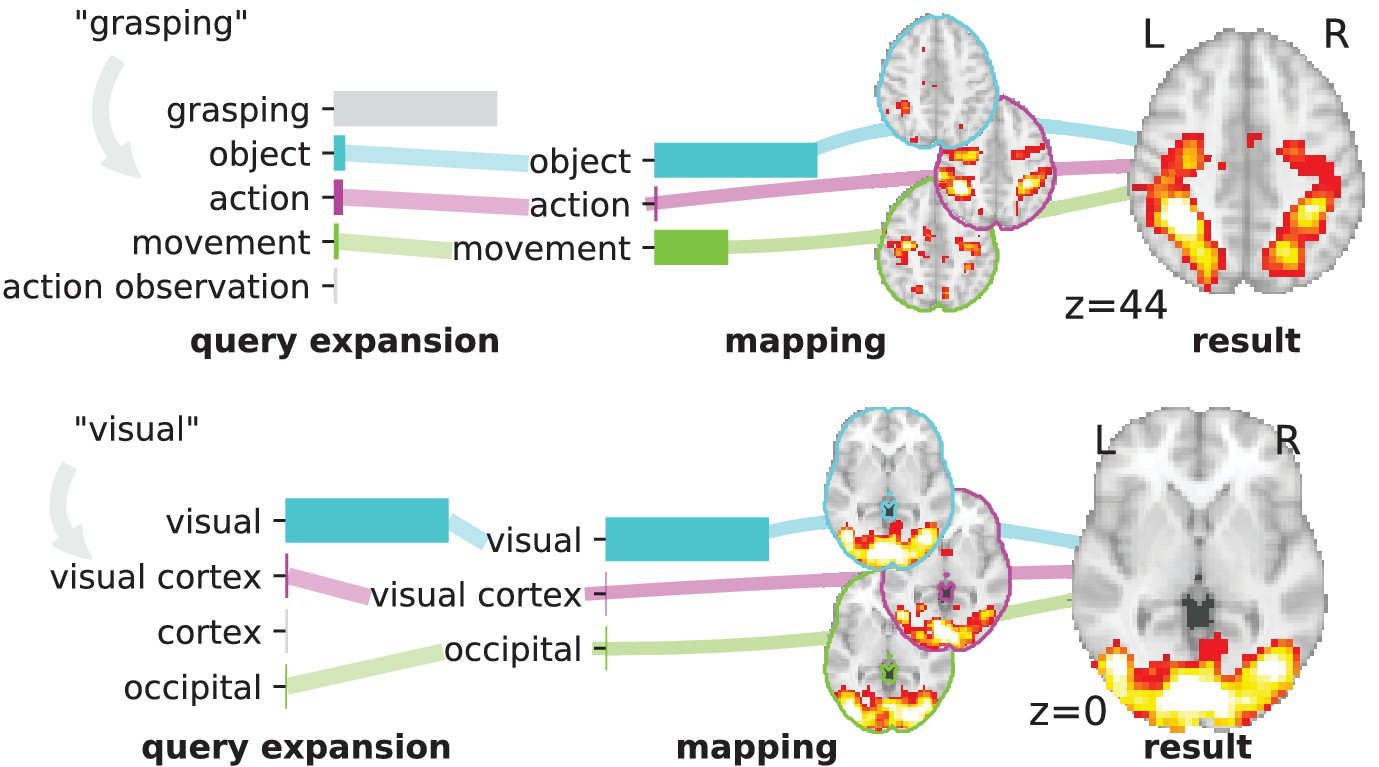
More information can be found in 8.
7.8 DiCoDiLe: Distributed Convolutional Dictionary Learning
Convolutional dictionary learning (CDL) estimates shift invariant basis adapted to represent signals or images. CDL has proven useful for image denoising or inpainting, as well as for pattern discovery on multivariate signals. Contrarily to standard patch-based dictionary learning, patterns estimated by CDL can be positioned anywhere in signals or images. Optimization techniques consequently face the difficulty of working with extremely large inputs with millions of pixels or time samples. To address this optimization problem, we propose a distributed and asynchronous algorithm, employing locally greedy coordinate descent and a soft-locking mechanism that does not require a central server. Computation can be distributed on a number of workers which scales linearly with the size of the data. The parallel computation accelerates the parameter estimation and the distributed setting allows our algorithm be used with data that does not fit into a single computer's RAM. Experiments confirm the theoretical scaling properties of the algorithm, allowing us to learn patterns on images from the Hubble Space Telescope containing tens of millions of pixels.
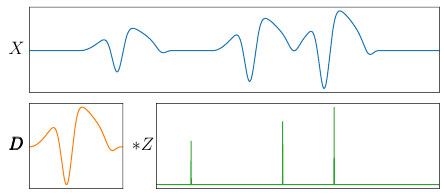
More information can be found in 21.
7.9 NeuMiss networks: differentiable programming for supervised learning with missing values
The presence of missing values makes supervised learning much more challenging. Indeed, previous work has shown that even when the response is a linear function of the complete data, the optimal predictor is a complex function of the observed entries and the missingness indicator. As a result, the computational or sample complexities of consistent approaches depend on the number of missing patterns, which can be exponential in the number of dimensions. In this work, we derive the analytical form of the optimal predictor under a linearity assumption and various missing data mechanisms including Missing at Random (MAR) and self-masking (Missing Not At Random). Based on a Neumann-series approximation of the optimal predictor, we propose a new principled architecture, named NeuMiss networks. Their originality and strength come from the use of a new type of non-linearity: the multiplication by the missingness indicator. We provide an upper bound on the Bayes risk of NeuMiss networks, and show that they have good predictive accuracy with both a number of parameters and a computational complexity independent of the number of missing data patterns. As a result they scale well to problems with many features, and remain statistically efficient for medium-sized samples. Moreover, we show that, contrary to procedures using EM or imputation, they are robust to the missing data mechanism, including difficult MNAR settings such as self-masking.
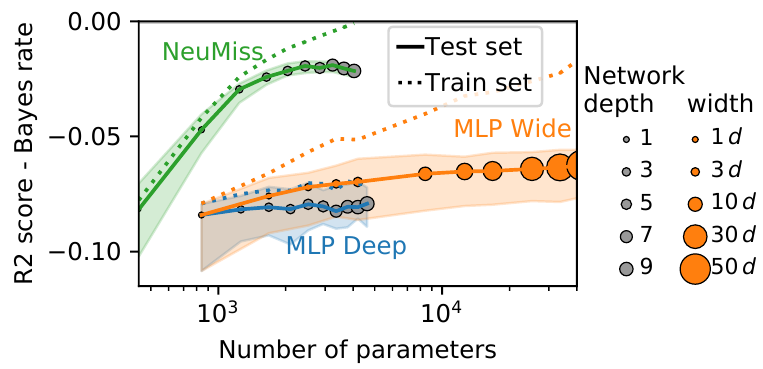
More information can be found in 49.
7.10 Implicit differentiation of Lasso-type models for hyperparameter optimization
Setting regularization parameters for Lasso-type estimators is notoriously difficult, though crucial in practice. The most popular hyperparam-eter optimization approach is grid-search using held-out validation data. Grid-search however requires to choose a predefined grid for each parameter , which scales exponentially in the number of parameters. Another approach is to cast hyperparameter optimization as a bi-level optimization problem, one can solve by gradient descent. The key challenge for these methods is the estimation of the gradient w.r.t. the hyperpa-rameters. Computing this gradient via forward or backward automatic differentiation is possible yet usually suffers from high memory consumption. Alternatively implicit differentiation typically involves solving a linear system which can be prohibitive and numerically unstable in high dimension. In addition, implicit differentiation usually assumes smooth loss functions, which is not the case for Lasso-type problems. This work introduces an efficient implicit differentiation algorithm, without matrix inversion, tailored for Lasso-type problems. Our approach scales to high-dimensional data by leveraging the sparsity of the solutions. Experiments demonstrate that the proposed method outperforms a large number of standard methods to optimize the error on held-out data, or the Stein Unbiased Risk Esti-mator (SURE).
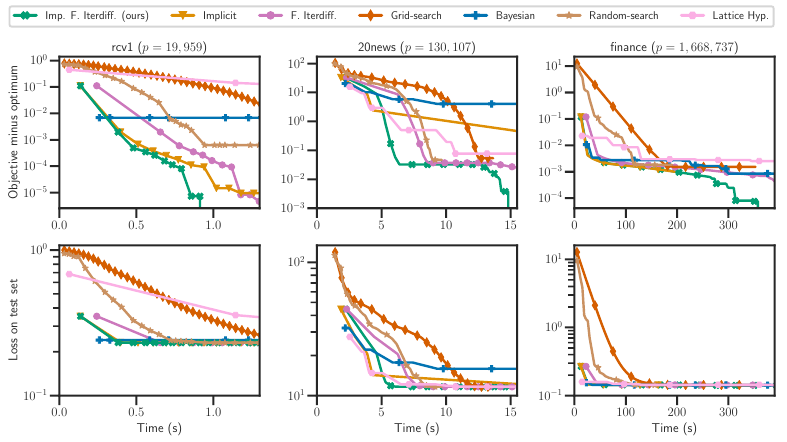
More information can be found in 41.
8 Bilateral contracts and grants with industry
8.1 Bilateral contracts with industry
Since 2020, a CIFRE PhD thesis has been launched with Facebook AI Research France. This contract supports the PhD thesis of Charlotte Caucheteux.
Since 2019, a CIFRE PhD thesis has been launched with Siemens-Healthineers France. This contract supports the PhD thesis of Guillaume Daval-Frérot.
Since 2018, a CIFRE PhD thesis has been launched with InteraXon, Ca. This contract supports the PhD thesis of Hubert Banville.
since 2020, Thomas Moreau is a consultant on machine learning for health care for Qynapse, France. The consulting sessions take place approximately once a month.
8.2 Bilateral grants with industry
Cython+
The Cython+ grant, funded by BPI France, and Region Ile de France, unites Inria, Telecom Paristech, Nexedis, and Abilian to improve parallel computing in Python.
9 Partnerships and cooperations
9.1 International initiatives
9.1.1 Inria International Labs
Meta&Co
- Title: Machine learning for meta-analysis of functional neuroimaging data
- Duration: 2018 - 2020
- Coordinator: Bertrand Thirion
-
Partners:
- Psychology department., Stanford (United States)
- Inria contact: Bertrand Thirion
- Summary: Cognitive science and psychiatry describe mental operations: cognition, emotion, perception and their dysfunction. Cognitive neuroimaging bridge these mental concepts to their implementation in the brain, neural firing and wiring, by relying on functional brain imaging. Yet aggregating results from experiments probing brain activity into a consistent description faces the roadblock that cognitive concepts and brain pathologies are ill-defined. Separation between them is often blurry. In addition, these concepts and subdivisions may not correspond to actual brain structures or systems. To tackle this challenge, we propose to adapt data-mining techniques used to learn relationships in computational linguistics. Natural language processing uses distributional semantics to build semantic relationships and ontologies. New models are needed to learn relationships from heterogeneous signals: functional magnetic resonance images (fMRI), on the one hand, combined with related psychology and neuroimaging annotations or publications, on the other hand. Such a joint effort will rely on large publicly-available fMRI databases shared by Podrack Lab, as well as literature mining.
LargeSmallBrainNets
- Title: CharacterizingLarge and Small-scale Brain Networks in Typical and Atypical populations Using Novel Computational Methods for dMRI and fMRI-based Connectivity and Microstructure.
- Duration: 2019 - 2022
- Coordinator: Demian Wassermann
-
Partners:
- Stanford Cognitive and Systems Neuroscience Laboratory, Stanford (United States)
- Inria contact: Demian Wassermann
-
Summary:
In the past two decades, brain imaging of neurotypical individuals and clinical populations has primarily focused on localization of function and structures in the brain, revealing activation in specific brain regions during performance of cognitive tasks through modalities such as functional MRI. In parallel, technologies to identify white matter structures have been developed using diffusion MRI. Lately, interest has shifted towards developing a deeper understanding of the brain's macroscopic and microscopic architectures and their influence on cognitive and affective information processing. Using for this resting state fMRI and diffusion MRI to build the functional and structural networks of the human brain.
The human brain is a complex patchwork of interconnected regions, and graph-theoretical approaches have become increasingly useful for understanding how functionally connected systems engender, and constrain, cognitive functions. The functional nodes of the human brain, i.e. cortical regions, and their structural inter-connectivity, collectively the brain’s macrostructure or "connectome", are, however, poorly understood. Quantifying in vivo how these nodes’ microstructure, specifically cellular composition or cytoarchitecture, influences the cognitive tasks in which these are involved is fundamental problem in understanding the connectome. Furthermore, the coupling between within and across-subject contributions to the connectome and cognitive differences hampers the identification and understanding of the link between brain structure and function, and human cognition.
Critically, there is a dearth of computational methods for reliably identifying functional nodes of the brain, their micro and macrostructure in vivo, and separating the population and subject-specific effects. Devising and validating methods for investigating the human connectome has therefore taken added significance.
The first major goal of this project is to develop and validate appropriate sophisticated computational and mathematical tools relate the brain’s macrostructure with its function. Specifically, we will focus on being able to separate population and subject-specific contributions within these models using state-of-the-art human brain imaging techniques and open-source data from the Human Connectome Project (HCP) and the Adolescent Brain Cognitive Development study (ABCD). To this end, we will first develop and validate novel computational tools for (1) formulating and fitting large scale random effect models on graphs derived from functional and structural connectivity and (2) implement techniques enabling us to impose different regularization schemes based on sparsity and multicollinearity of the model parameters.
The second major goal of this project is characterizing the cytoarchitecture of the nodes, i.e. cortical regions, at the microscopic level and their relationship with the brain’s hemodynamical function and cognition. For this, we will (1) identify cortical areas with specific cytoarchitecture in the human cortex and use them to develop diffusion MRI-based models, (2) validate these models with numerical simulations of the dMRI signal and animal models, and (3) establish the relationship between cytoarchitecture and hemodynamical function measured from fMRI and cognition. For this we will leverage multi-shell high-angular diffusion MRI from public databases such as HCP and ABCD.
Finally, we will use to use our newly developed computational tools to characterize normal structural and functional brain networks in neurotypical adults. Due to the complementarity of the cognitive science and imaging techniques expertise the synergy between the two laboratories of this associate team will allow us to reveal in unprecedented detail the structural and functional connectivity of the human brain and its relation to cognition.
9.1.2 Inria international partners
Informal international partners
ReSynCed: REdundancy and SYNergy between environmentally entrained and spontaneous brain activity in Cognitive Dysfunction
Denis Engemann holds a guest affiliation (2020-2022) at the Max-Planck Institute Leipzig, Neurology department https://
9.1.3 Participation in other international programs
Gaël Varoquaux is delegate for France at the OCDE GPAI (Global Partnership on AI), in the task force on AI and the pandemics.
Bertrand Thirion is delegate for France at the OCDE GPAI (Global Partnership on AI).
SILIDOC
- Title: In silico modeling of neuroimaging data at the single patient level for the characterization and prognosis of disorders of consciousness
- Duration: 2020 - 2023
- Coordinator: Demian Wassermann; Jacobo Sitt; Enzo Tagliazucchi; Rodrigo Cofre Torres.
-
Partners:
- Computational Cognitive Neuroscience Lab,Facultad de Ciencias Exactas y Naturales, Universidad de Buenos Aires, Argentina
- School of Sciences, Universidad de Valparaíso, Chile
- PICNIC Team, Brain and Spine Institute, Hôpital Pitié-Salpêtrière
- Inria contact: Demian Wassermann
- Summary: Studying the brain mechanisms behind consciousness is a major challenge for neuroscience and medicine. Yet so far, there is no such thing as a unique biomarker that can precisely define the state of consciousness of a disorders of consciousness (DOC) patient. All the biomarkers proposed so far are theory-based but empirically defined (EBM; empirical biomarkers): the thresholds that separate categories are set in a data-driven way. In this project, we propose a novel approach using model-based biomarkers (MBM). This new family of biomarkers (MBMs) will not only complement the EBMs but mainly will naturally address the knowledge gaps associated with the understanding of the underlying causal mechanisms behind the different states of consciousness. The modelling of the structural and functional connectivity will be combined with novel, systematic perturbational approaches that can provide new insights into the human brain’s ability to integrate and segregate information over time. In particular, with this approach we will address the hypothesis that MBMs provide functional fingerprinting of conscious states and insights into the underlying necessary and sufficient brain networks as well as their neural mechanisms. To address the development of these biomarkers, we propose a highly interdisciplinary project that combines basic and clinical neuroscience with whole-brain computational modelling, DTI SOMETHING, and clinical neuroscience. This project will benefit of proposing a complementary synergy between fourthree groups with large expertise in each area to address a common question We will develop computational whole-brain models based on single-patient neuroimaging data. We will extract MBM from the adjusted model parameters and from in-silico simulations. We will test the utility of these biomarkers for the diagnosis of patients with chronic DOC. Then, we will contrast the MBM with a set of previously developed EBM. Finally, we will analyze the diagnostic and prognostic capacity of these biomarkers in DOC patients in both chronic and acute stages.
9.2 International research visitors
9.2.1 Visits of international scientists
Aapo Huvarinen visited the team from April 1st, 2019 to March 31st, 2020.
9.3 European initiatives
9.3.1 FP7 & H2020 Projects
HBP SGA2
- Title: Human Brain Project Specific Grant Agreement 2
- Duration: April 2018 - March 2020
- Coordinator: EPFL
-
Partners:
- AALTO KORKEAKOULUSAATIO SR (Finland)
- ACADEMISCH ZIEKENHUIS LEIDEN (Netherlands)
- ATHENS UNIVERSITY OF ECONOMICS AND BUSINESS - RESEARCH CENTER
- ATHINA-EREVNITIKO KENTRO KAINOTOMIAS STIS TECHNOLOGIES TIS PLIROFORIAS, TON EPIKOINONION KAI TIS GNOSIS
- BARCELONA SUPERCOMPUTING CENTER - CENTRO NACIONAL DE SUPERCOMPUTACION (Spain)
- BAUHAUS-UNIVERSITAET WEIMAR (Germany)
- BERGISCHE UNIVERSITAET WUPPERTAL (Germany)
- Bloomfield Science Museum Jerusalem (BSMJ) (Israel)
- CARDIFF UNIVERSITY
- CENTRE HOSPITALIER REGIONAL DE MARSEILLE ASSISTANCE PUBLIQUE-HOPITAUX MARSEILLE (France)
- CENTRE HOSPITALIER REGIONAL ET UNIVERSITAIRE DE LILLE (France)
- CENTRE HOSPITALIER UNIVERSITAIRE DE GRENOBLE (France)
- CENTRE HOSPITALIER UNIVERSITAIRE VAUDOIS (Switzerland)
- CENTRE NATIONAL DE LA RECHERCHE SCIENTIFIQUE CNRS (France)
- CINECA CONSORZIO INTERUNIVERSITARIO (Italy)
- COMMISSARIAT A L ENERGIE ATOMIQUE ET AUX ENERGIES ALTERNATIVES (France)
- CONSIGLIO NAZIONALE DELLE RICERCHE (Italy)
- CONSORCI INSTITUT D'INVESTIGACIONS BIOMEDIQUES AUGUST PI I SUNYER (Spain)
- CYBERBOTICS SARL (Switzerland)
- DANMARKS TEKNISKE UNIVERSITET (Denmark)
- DE MONTFORT UNIVERSITY
- DEBRECENI EGYETEM (Hungary)
- DEUTSCHES ZENTRUM FUR NEURODEGENERATIVE ERKRANKUNGEN EV (Germany)
- EBRAINS (Belgium)
- ECOLE NORMALE SUPERIEURE (France)
- ECOLE POLYTECHNIQUE FEDERALE DE LAUSANNE (Switzerland)
- EIDGENOESSISCHE TECHNISCHE HOCHSCHULE ZUERICH (Switzerland)
- ERASMUS UNIVERSITAIR MEDISCH CENTRUM ROTTERDAM (Netherlands)
- ETHNIKO KAI KAPODISTRIAKO PANEPISTIMIO ATHINON
- EUROPEAN MOLECULAR BIOLOGY LABORATORY (Germany)
- FONDAZIONE EUROPEAN BRAIN RESEARCHINSTITUTE RITA LEVI (Italy)
- FONDAZIONE ISTITUTO ITALIANO DI TECNOLOGIA (Italy)
- FONDEN TEKNOLOGIRADET (Denmark)
- FORSCHUNGSZENTRUM JULICH GMBH (Germany)
- FRAUNHOFER GESELLSCHAFT ZUR FOERDERUNG DER ANGEWANDTEN FORSCHUNG E.V. (Germany)
- FUNDACAO D. ANNA SOMMER CHAMPALIMAUD E DR. CARLOS MONTEZ CHAMPALIMAUD (Portugal)
- FUNDACIO INSTITUT DE BIOENGINYERIA DE CATALUNYA (Spain)
- FZI FORSCHUNGSZENTRUM INFORMATIK (Germany)
- GEORG-AUGUST-UNIVERSITAT GOTTINGENSTIFTUNG OFFENTLICHEN RECHTS (Germany)
- HEINRICH-HEINE-UNIVERSITAET DUESSELDORF (Germany)
- HITS GGMBH (Germany)
- HOCHSCHULE STRALSUND (Germany)
- HUMBOLDT-UNIVERSITAET ZU BERLIN (Germany)
- IDRYMA IATROVIOLOGIKON EREUNON AKADEMIAS ATHINON
- IMPERIAL COLLEGE OF SCIENCE TECHNOLOGY AND MEDICINE
- INSTITUT DU CERVEAU ET DE LA MOELLE EPINIERE (France)
- INSTITUT JOZEF STEFAN (Slovenia)
- INSTITUT PASTEUR (France)
- INSTITUTE OF SCIENCE AND TECHNOLOGY AUSTRIA (Austria)
- ISTITUTO NAZIONALE DI FISICA NUCLEARE (Italy)
- JOHANN WOLFGANG GOETHE-UNIVERSITATFRANKFURT AM MAIN (Germany)
- KARLSRUHER INSTITUT FUER TECHNOLOGIE (Germany)
- KAROLINSKA INSTITUTET (Sweden)
- KATHOLIEKE UNIVERSITEIT LEUVEN (Belgium)
- KIRSERLETI ORVOSTUDOMANYI KUTATOINTEZET (Hungary)
- KONINKLIJKE NEDERLANDSE AKADEMIE VAN WETENSCHAPPEN - KNAW (Netherlands)
- KUNGLIGA TEKNISKA HOEGSKOLAN (Sweden)
- LABORATORIO EUROPEO DI SPETTROSCOPIE NON LINEARI (Italy)
- LINNEUNIVERSITETET (Sweden)
- MAX-PLANCK-GESELLSCHAFT ZUR FORDERUNG DER WISSENSCHAFTEN EV (Germany)
- MEDIZINISCHE UNIVERSITAT INNSBRUCK (Austria)
- MIDDLESEX UNIVERSITY HIGHER EDUCATION CORPORATION
- NORGES MILJO-OG BIOVITENSKAPLIGE UNIVERSITET (Norway)
- OSTERREICHISCHE STUDIENGESELLSCHAFTFUR KYBERNETIK VEREIN (Austria)
- POLITECNICO DI MILANO (Italy)
- POLITECNICO DI TORINO (Italy)
- POLYTECHNEIO KRITIS
- RHEINISCH-WESTFAELISCHE TECHNISCHE HOCHSCHULE AACHEN (Germany)
- RUPRECHT-KARLS-UNIVERSITAET HEIDELBERG (Germany)
- SABANCI UNIVERSITESI (Turkey)
- SCUOLA INTERNAZIONALE SUPERIORE DI STUDI AVANZATI DI TRIESTE (Italy)
- SCUOLA NORMALE SUPERIORE (Italy)
- SCUOLA SUPERIORE DI STUDI UNIVERSITARI E DI PERFEZIONAMENTO S ANNA (Italy)
- SIB INSTITUT SUISSE DE BIOINFORMATIQUE (Switzerland)
- STICHTING NEDERLANDSE WETENSCHAPPELIJK ONDERZOEK INSTITUTEN (Netherlands)
- TAMPEREEN KORKEAKOULUSAATIO SR (Finland)
- TECHNISCHE UNIVERSITAET DRESDEN (Germany)
- TECHNISCHE UNIVERSITAET GRAZ (Austria)
- TECHNISCHE UNIVERSITAET MUENCHEN (Germany)
- TEL AVIV UNIVERSITY (Israel)
- THE CHANCELLOR, MASTERS AND SCHOLARS OF THE UNIVERSITY OF OXFORD
- THE HEBREW UNIVERSITY OF JERUSALEM (Israel)
- THE UNIVERSITY COURT OF THE UNIVERSITY OF ABERDEEN
- THE UNIVERSITY OF EDINBURGH
- THE UNIVERSITY OF HERTFORDSHIRE HIGHER EDUCATION CORPORATION
- THE UNIVERSITY OF MANCHESTER
- THE UNIVERSITY OF SHEFFIELD
- THE UNIVERSITY OF SUSSEX
- UNIVERSIDAD AUTONOMA DE MADRID (Spain)
- UNIVERSIDAD DE CASTILLA - LA MANCHA (Spain)
- UNIVERSIDAD DE GRANADA (Spain)
- UNIVERSIDAD POLITECNICA DE MADRID (Spain)
- UNIVERSIDAD POMPEU FABRA (Spain)
- UNIVERSIDAD REY JUAN CARLOS (Spain)
- UNIVERSIDADE DO MINHO (Portugal)
- UNIVERSITA DEGLI STUDI DI MILANO (Italy)
- UNIVERSITA DEGLI STUDI DI NAPOLI FEDERICO II (Italy)
- UNIVERSITA DEGLI STUDI DI PAVIA (Italy)
- UNIVERSITAET BIELEFELD (Germany)
- UNIVERSITAET HAMBURG (Germany)
- UNIVERSITAETSKLINIKUM FREIBURG (Germany)
- UNIVERSITAETSKLINIKUM HAMBURG-EPPENDORF (Germany)
- UNIVERSITAT DE BARCELONA (Spain)
- UNIVERSITAT ZURICH (Switzerland)
- UNIVERSITATSKLINIKUM BONN (Germany)
- UNIVERSITATSMEDIZIN GREIFSWALD KORPERSCHAFT DES OFFENTLICHEN RECHTS (Germany)
- UNIVERSITATSSPITAL BASEL (Switzerland)
- UNIVERSITE D'AIX MARSEILLE (France)
- UNIVERSITE DE BORDEAUX (France)
- UNIVERSITE DE GENEVE (Switzerland)
- UNIVERSITE DE LIEGE (Belgium)
- UNIVERSITE GRENOBLE ALPES (France)
- UNIVERSITEIT ANTWERPEN (Belgium)
- UNIVERSITEIT GENT (Belgium)
- UNIVERSITEIT VAN AMSTERDAM (Netherlands)
- UNIVERSITETET I OSLO (Norway)
- UNIVERSITY OF LEEDS
- UNIVERSITY OF SURREY
- UNIVERSITY OF THE WEST OF ENGLAND, BRISTOL
- UPPSALA UNIVERSITET (Sweden)
- WEIZMANN INSTITUTE OF SCIENCE (Israel)
- Inria contact: Bertrand Thirion
- Summary: The Human Brain Project (HBP) is a major European scientific research initiative to improve our understanding of the brain and the role it plays in making us human, and to exploit the opportunities offered by the resulting knowledge. The size and complexity of the brain make this an expensive undertaking, but the costs associated with our current ignorance are rising and the potential gains from better insight into the brain are increasing. Brain-related diseases, many of which are age-related, now represent a major part of the global health burden and there are both ethical and economic imperatives to keep the growing number of older people healthier and more productive. Economic advantage is increasingly linked to artificial intelligence (AI), our ability to create technology to extract, manipulate and harness knowledge. The HBP’s comprehension of what makes the human brain so efficient and flexible should help to maintain Europe’s competitiveness and innovation potential in this area. The HBP is one of several brain research initiatives and projects around the world, albeit one of the first, but it is unique in a number of ways. Only the HBP has an explicit focus on both neuroscience and computing. It is also the broadest and most integrated brain initiative, and the only one aiming to build a research infrastructure to accelerate brain research. The HBP is a FET Flagship which started under FP7 and continues under H2020 with a succession of Specific Grant Agreements (SGAs) under a Framework Partnership Agreement (FPA). In its FP7 Ramp-Up Phase (2013-16) and subsequent SGA1 funding period (2016-18), the HBP implemented a scientific project of rare ambition, breadth and scale, and forged its diverse constituents into a functioning entity. On the scientific side, it not only identified critical gaps in our understanding of the brain, but also created tools and obtained data to fill many of them. It designed, built and demonstrated six ICT research platforms, supporting neuroinformatics, brain simulation, high-performance analytics and computing, medical informatics, brain-inspired computing and linking of simulated brains to robotic bodies. The results have been made available to the scientific community. The HBP also learnt to address underperformance and conflicts, and opened up the Project via competitive calls and the integration of Partnering Projects. In the upcoming SGA2 funding period (2018-20), the HBP will continue to strengthen global brain research efforts by extending coordination with other brain initiatives and projects. Internally, it will continue its unique inter-disciplinary co-design approach, developing research infrastructure capabilities via use cases built around specific research needs. This approach will underpin its critical scientific work of understanding how to bridge between the different scales of brain organisation, a key prerequisite to understand the principles of brain organisation. It will include gathering data to support detailed modelling, notably of the human hippocampus, as well as structural, functional and connectivity data to improve systemic understanding of the whole brain. The HBP will also investigate brain similarities and differences between individuals and between species. It will model key brain functions, including visual recognition, slow-wave activity, episodic memory and consciousness in rodents and humans, and elaborate their cognitive architectures. In addition, it will develop simplified brain models to support further development of brain-inspired computing. SGA2 will see the individual infrastructure platforms extended and integrated into the HBP Joint Platform (HBP-JP). The JP will make HBP services more robust and improve the user experience, encouraging wider use of its tools.
ICEI
- Title: Interactive Computing E-Infrastructure for the Human Brain Project
- Duration: January 2018 - March 2023
-
Coordinator:
EPFL
- AALTO KORKEAKOULUSAATIO SR (Finland)
- ACADEMISCH ZIEKENHUIS LEIDEN (Netherlands)
- ATHENS UNIVERSITY OF ECONOMICS AND BUSINESS - RESEARCH CENTER
- ATHINA-EREVNITIKO KENTRO KAINOTOMIAS STIS TECHNOLOGIES TIS PLIROFORIAS, TON EPIKOINONION KAI TIS GNOSIS
- BARCELONA SUPERCOMPUTING CENTER - CENTRO NACIONAL DE SUPERCOMPUTACION (Spain)
- BAUHAUS-UNIVERSITAET WEIMAR (Germany)
- BERGISCHE UNIVERSITAET WUPPERTAL (Germany)
- Bloomfield Science Museum Jerusalem (BSMJ) (Israel)
- CARDIFF UNIVERSITY
- CENTRE HOSPITALIER REGIONAL DE MARSEILLE ASSISTANCE PUBLIQUE-HOPITAUX MARSEILLE (France)
- CENTRE HOSPITALIER REGIONAL ET UNIVERSITAIRE DE LILLE (France)
- CENTRE HOSPITALIER UNIVERSITAIRE DE GRENOBLE (France)
- CENTRE HOSPITALIER UNIVERSITAIRE VAUDOIS (Switzerland)
- CENTRE NATIONAL DE LA RECHERCHE SCIENTIFIQUE CNRS (France)
- CINECA CONSORZIO INTERUNIVERSITARIO (Italy)
- COMMISSARIAT A L ENERGIE ATOMIQUE ET AUX ENERGIES ALTERNATIVES (France)
- CONSIGLIO NAZIONALE DELLE RICERCHE (Italy)
- CONSORCI INSTITUT D'INVESTIGACIONS BIOMEDIQUES AUGUST PI I SUNYER (Spain)
- CYBERBOTICS SARL (Switzerland)
- DANMARKS TEKNISKE UNIVERSITET (Denmark)
- DE MONTFORT UNIVERSITY
- DEBRECENI EGYETEM (Hungary)
- DEUTSCHES ZENTRUM FUR NEURODEGENERATIVE ERKRANKUNGEN EV (Germany)
- EBRAINS (Belgium)
- ECOLE NORMALE SUPERIEURE (France)
- ECOLE POLYTECHNIQUE FEDERALE DE LAUSANNE (Switzerland)
- EIDGENOESSISCHE TECHNISCHE HOCHSCHULE ZUERICH (Switzerland)
- ERASMUS UNIVERSITAIR MEDISCH CENTRUM ROTTERDAM (Netherlands)
- ETHNIKO KAI KAPODISTRIAKO PANEPISTIMIO ATHINON
- EUROPEAN MOLECULAR BIOLOGY LABORATORY (Germany)
- FONDAZIONE EUROPEAN BRAIN RESEARCHINSTITUTE RITA LEVI (Italy)
- FONDAZIONE ISTITUTO ITALIANO DI TECNOLOGIA (Italy)
- FONDEN TEKNOLOGIRADET (Denmark)
- FORSCHUNGSZENTRUM JULICH GMBH (Germany)
- FRAUNHOFER GESELLSCHAFT ZUR FOERDERUNG DER ANGEWANDTEN FORSCHUNG E.V. (Germany)
- FUNDACAO D. ANNA SOMMER CHAMPALIMAUD E DR. CARLOS MONTEZ CHAMPALIMAUD (Portugal)
- FUNDACIO INSTITUT DE BIOENGINYERIA DE CATALUNYA (Spain)
- FZI FORSCHUNGSZENTRUM INFORMATIK (Germany)
- GEORG-AUGUST-UNIVERSITAT GOTTINGENSTIFTUNG OFFENTLICHEN RECHTS (Germany)
- HEINRICH-HEINE-UNIVERSITAET DUESSELDORF (Germany)
- HITS GGMBH (Germany)
- HOCHSCHULE STRALSUND (Germany)
- HUMBOLDT-UNIVERSITAET ZU BERLIN (Germany)
- IDRYMA IATROVIOLOGIKON EREUNON AKADEMIAS ATHINON
- IMPERIAL COLLEGE OF SCIENCE TECHNOLOGY AND MEDICINE
- INSTITUT DU CERVEAU ET DE LA MOELLE EPINIERE (France)
- INSTITUT JOZEF STEFAN (Slovenia)
- INSTITUT PASTEUR (France)
- INSTITUTE OF SCIENCE AND TECHNOLOGY AUSTRIA (Austria)
- ISTITUTO NAZIONALE DI FISICA NUCLEARE (Italy)
- JOHANN WOLFGANG GOETHE-UNIVERSITATFRANKFURT AM MAIN (Germany)
- KARLSRUHER INSTITUT FUER TECHNOLOGIE (Germany)
- KAROLINSKA INSTITUTET (Sweden)
- KATHOLIEKE UNIVERSITEIT LEUVEN (Belgium)
- KIRSERLETI ORVOSTUDOMANYI KUTATOINTEZET (Hungary)
- KONINKLIJKE NEDERLANDSE AKADEMIE VAN WETENSCHAPPEN - KNAW (Netherlands)
- KUNGLIGA TEKNISKA HOEGSKOLAN (Sweden)
- LABORATORIO EUROPEO DI SPETTROSCOPIE NON LINEARI (Italy)
- LINNEUNIVERSITETET (Sweden)
- MAX-PLANCK-GESELLSCHAFT ZUR FORDERUNG DER WISSENSCHAFTEN EV (Germany)
- MEDIZINISCHE UNIVERSITAT INNSBRUCK (Austria)
- MIDDLESEX UNIVERSITY HIGHER EDUCATION CORPORATION
- NORGES MILJO-OG BIOVITENSKAPLIGE UNIVERSITET (Norway)
- OSTERREICHISCHE STUDIENGESELLSCHAFTFUR KYBERNETIK VEREIN (Austria)
- POLITECNICO DI MILANO (Italy)
- POLITECNICO DI TORINO (Italy)
- POLYTECHNEIO KRITIS
- RHEINISCH-WESTFAELISCHE TECHNISCHE HOCHSCHULE AACHEN (Germany)
- RUPRECHT-KARLS-UNIVERSITAET HEIDELBERG (Germany)
- SABANCI UNIVERSITESI (Turkey)
- SCUOLA INTERNAZIONALE SUPERIORE DI STUDI AVANZATI DI TRIESTE (Italy)
- SCUOLA NORMALE SUPERIORE (Italy)
- SCUOLA SUPERIORE DI STUDI UNIVERSITARI E DI PERFEZIONAMENTO S ANNA (Italy)
- SIB INSTITUT SUISSE DE BIOINFORMATIQUE (Switzerland)
- STICHTING NEDERLANDSE WETENSCHAPPELIJK ONDERZOEK INSTITUTEN (Netherlands)
- TAMPEREEN KORKEAKOULUSAATIO SR (Finland)
- TECHNISCHE UNIVERSITAET DRESDEN (Germany)
- TECHNISCHE UNIVERSITAET GRAZ (Austria)
- TECHNISCHE UNIVERSITAET MUENCHEN (Germany)
- TEL AVIV UNIVERSITY (Israel)
- THE CHANCELLOR, MASTERS AND SCHOLARS OF THE UNIVERSITY OF OXFORD
- THE HEBREW UNIVERSITY OF JERUSALEM (Israel)
- THE UNIVERSITY COURT OF THE UNIVERSITY OF ABERDEEN
- THE UNIVERSITY OF EDINBURGH
- THE UNIVERSITY OF HERTFORDSHIRE HIGHER EDUCATION CORPORATION
- THE UNIVERSITY OF MANCHESTER
- THE UNIVERSITY OF SHEFFIELD
- THE UNIVERSITY OF SUSSEX
- UNIVERSIDAD AUTONOMA DE MADRID (Spain)
- UNIVERSIDAD DE CASTILLA - LA MANCHA (Spain)
- UNIVERSIDAD DE GRANADA (Spain)
- UNIVERSIDAD POLITECNICA DE MADRID (Spain)
- UNIVERSIDAD POMPEU FABRA (Spain)
- UNIVERSIDAD REY JUAN CARLOS (Spain)
- UNIVERSIDADE DO MINHO (Portugal)
- UNIVERSITA DEGLI STUDI DI MILANO (Italy)
- UNIVERSITA DEGLI STUDI DI NAPOLI FEDERICO II (Italy)
- UNIVERSITA DEGLI STUDI DI PAVIA (Italy)
- UNIVERSITAET BIELEFELD (Germany)
- UNIVERSITAET HAMBURG (Germany)
- UNIVERSITAETSKLINIKUM FREIBURG (Germany)
- UNIVERSITAETSKLINIKUM HAMBURG-EPPENDORF (Germany)
- UNIVERSITAT DE BARCELONA (Spain)
- UNIVERSITAT ZURICH (Switzerland)
- UNIVERSITATSKLINIKUM BONN (Germany)
- UNIVERSITATSMEDIZIN GREIFSWALD KORPERSCHAFT DES OFFENTLICHEN RECHTS (Germany)
- UNIVERSITATSSPITAL BASEL (Switzerland)
- UNIVERSITE D'AIX MARSEILLE (France)
- UNIVERSITE DE BORDEAUX (France)
- UNIVERSITE DE GENEVE (Switzerland)
- UNIVERSITE DE LIEGE (Belgium)
- UNIVERSITE GRENOBLE ALPES (France)
- UNIVERSITEIT ANTWERPEN (Belgium)
- UNIVERSITEIT GENT (Belgium)
- UNIVERSITEIT VAN AMSTERDAM (Netherlands)
- UNIVERSITETET I OSLO (Norway)
- UNIVERSITY OF LEEDS
- UNIVERSITY OF SURREY
- UNIVERSITY OF THE WEST OF ENGLAND, BRISTOL
- UPPSALA UNIVERSITET (Sweden)
- WEIZMANN INSTITUTE OF SCIENCE (Israel)
- Inria contact: Bertrand Thirion
-
Summary:
Five leading European supercomputing centres are committed to develop, within their respective national programs and service portfolios, a set of services that will be federated across a consortium. The work will be undertaken by the following supercomputing centres, which form the High Performance Analytics and Computing (HPAC) Platform of the Human Brain Project (HBP):
- Barcelona Supercomputing Centre (BSC) in Spain,
- The Italian supercomputing centre CINECA,
- The Swiss National Supercomputing Centre CSCS,
- The Jülich Supercomputing Centre in Germany, and
- Commissariat à l'énergie atomique et aux énergies alternatives (CEA), France (joining in April 2018). The new consortium will be called Fenix and it aims at providing scalable compute and data services in a federated manner. The neuroscience community is of particular interest in this context and the HBP represents a prioritised driver for the Fenix infrastructure design and implementation. The Interactive Computing E-Infrastructure for the HBP (ICEI) project will realise key elements of this Fenix infrastructure that are targeted to meet the needs of the neuroscience community. The participating sites plan for cloud-like services that are compatible with the work cultures of scientific computing and data science. Specifically, this entails developing interactive supercomputing capabilities on the available extreme computing and data systems. Key features of the ICEI infrastructure are:
- Scalable compute resources;
- A federated data infrastructure; and
- Interactive Compute Services providing access to the federated data infrastructure as well as elastic access to the scalable compute resources. The ICEI e-infrastructure will be realised through a coordinated procurement of equipment and R&D services. Furthermore, significant additional parts of the infrastructure and R&D services will be realised within the ICEI project through in-kind contributions from the participating supercomputing centres.
AI4EU
- Title: A European AI On Demand Platform and Ecosysteme
- Duration: January 2019 - December 2021
- Coordinator: THALES
-
Partners:
- AGENCIA ESTATAL CONSEJO SUPERIOR DEINVESTIGACIONES CIENTIFICAS (Spain)
- ALMA MATER STUDIORUM - UNIVERSITA DI BOLOGNA (Italy)
- ARISTOTELIO PANEPISTIMIO THESSALONIKIS
- ASSOCIACAO DO INSTITUTO SUPERIOR TECNICO PARA A INVESTIGACAO E DESENVOLVIMENTO (Portugal)
- BARCELONA SUPERCOMPUTING CENTER - CENTRO NACIONAL DE SUPERCOMPUTACION (Spain)
- BLUMORPHO SAS (France)
- BUDAPESTI MUSZAKI ES GAZDASAGTUDOMANYI EGYETEM (Hungary)
- BUREAU DE RECHERCHES GEOLOGIQUES ET MINIERES (France)
- CENTRE NATIONAL DE LA RECHERCHE SCIENTIFIQUE CNRS (France)
- CINECA CONSORZIO INTERUNIVERSITARIO (Italy)
- COMMISSARIAT A L ENERGIE ATOMIQUE ET AUX ENERGIES ALTERNATIVES (France)
- CONSIGLIO NAZIONALE DELLE RICERCHE (Italy)
- DEUTSCHES FORSCHUNGSZENTRUM FUR KUNSTLICHE INTELLIGENZ GMBH (Germany)
- DEUTSCHES ZENTRUM FUR LUFT - UND RAUMFAHRT EV (Germany)
- EOTVOS LORAND TUDOMANYEGYETEM (Hungary)
- ETHNIKO KAI KAPODISTRIAKO PANEPISTIMIO ATHINON
- ETHNIKO KENTRO EREVNAS KAI TECHNOLOGIKIS ANAPTYXIS
- EUROPEAN ORGANISATION FOR SECURITY (Belgium)
- FONDATION DE L'INSTITUT DE RECHERCHE IDIAP (Switzerland)
- FONDAZIONE BRUNO KESSLER (Italy)
- FORUM VIRIUM HELSINKI OY (Finland)
- FRANCE DIGITALE (France)
- FRAUNHOFER GESELLSCHAFT ZUR FOERDERUNG DER ANGEWANDTEN FORSCHUNG E.V. (Germany)
- FUNDACION CARTIF (Spain)
- FUNDINGBOX ACCELERATOR SP ZOO (Poland)
- FUNDINGBOX RESEARCH APS (Denmark)
- GOODAI RESEARCH SRO (Czech Republic)
- Hochschule für Technik und Wirtschaft Berlin (Germany)
- IDRYMA TECHNOLOGIAS KAI EREVNAS
- IMT TRANSFERT (France)
- INSTITUT JOZEF STEFAN (Slovenia)
- INSTITUT POLYTECHNIQUE DE GRENOBLE (France)
- INTERNATIONAL DATA SPACES EV (Germany)
- KARLSRUHER INSTITUT FUER TECHNOLOGIE (Germany)
- KNOW-CENTER GMBH RESEARCH CENTER FOR DATA-DRIVEN BUSINESS & BIG DATA ANALYTICS (Austria)
- NATIONAL CENTER FOR SCIENTIFIC RESEARCH "DEMOKRITOS"
- NATIONAL UNIVERSITY OF IRELAND GALWAY (Ireland)
- NORGES TEKNISK-NATURVITENSKAPELIGE UNIVERSITET NTNU (Norway)
- OFFICE NATIONAL D'ETUDES ET DE RECHERCHES AEROSPATIALES (France)
- ORANGE SA (France)
- OREBRO UNIVERSITY (Sweden)
- QWANT (France)
- TECHNICKA UNIVERZITA V KOSICIACH (Slovakia)
- TECHNISCHE UNIVERSITAET MUENCHEN (Germany)
- TECHNISCHE UNIVERSITAET WIEN (Austria)
- TECHNISCHE UNIVERSITAT BERLIN (Germany)
- THALES (France)
- THALES ALENIA SPACE FRANCE SAS (France)
- THALES SIX GTS FRANCE SAS (France)
- THOMSON LICENSING (France)
- TILDE SIA (Latvia)
- TWENTY COMMUNICATIONS SRO (Slovakia)
- UNIVERSIDAD POLITECNICA DE MADRID (Spain)
- UNIVERSIDADE DE COIMBRA (Portugal)
- UNIVERSITA CA' FOSCARI VENEZIA (Italy)
- UNIVERSITA DEGLI STUDI DI SIENA (Italy)
- UNIVERSITAT POLITECNICA DE CATALUNYA (Spain)
- UNIVERSITE DE LORRAINE (France)
- UNIVERSITE GRENOBLE ALPES (France)
- UNIVERSITY COLLEGE CORK - NATIONAL UNIVERSITY OF IRELAND, CORK (Ireland)
- UNIVERSITY OF LEEDS
- VRIJE UNIVERSITEIT BRUSSEL (Belgium)
- WAVESTONE (France)
- WAVESTONE ADVISORS (France)
- WAVESTONE LUXEMBOURG SA (Luxembourg)
- Inria contact: James CROWLEY
- Summary: Artificial Intelligence is a disruptive technology of our times with expected impacts rivalling those of electricity or printing. Resources for innovation are currently dominated by giant tech companies in North America and China. To ensure European independence and leadership, we must invest wisely by bundling, connecting and opening our AI resources. AI4EU will efficiently build a comprehensive European AI-on-demand platform to lower barriers to innovation, to boost technology transfer and catalyse the growth of start-ups and SMEs in all sectors through Open calls and other actions. The platform will act as a broker, developer and one-stop shop providing and showcasing services, expertise, algorithms, software frameworks, development tools, components, modules, data, computing resources, prototyping functions and access to funding. Training will enable different user communities (engineers, civic leaders, etc.) to obtain skills and certifications. The AI4EU Platform will establish a world reference, built upon and interoperable with existing AI and data components (e.g. the Acumos open-source framework, QWT search engine..) and platforms. It will mobilize the whole European AI ecosystem and already unites 80 partners in 21 countries including researchers, innovators and related talents. Eight industry-driven AI pilots will demonstrate the value of the platform as an innovation tool. In order to enhance the platform, research on five key interconnected AI scientific areas will be carried out using platform technologies and results will be implemented. The pilots and research will showcase how AI4EU can stimulate scientific discovery and technological innovation. The AI4EU Ethical Observatory will be established to ensure the respect of human centred AI values and European regulations. Sustainability will be ensured via the creation of the AI4EU Foundation. The results will feed a new and comprehensive Strategic Research Innovation Agenda for Europe.
VirtualBrainCloud
- Title: Personalized Recommendations for Neurodegenerative Disease
- Duration: December 2018 - November 2022
- Coordinator: CHARITE - UNIVERSITAETSMEDIZIN BERLIN
-
Partners:
- ALZHEIMER EUROPE (Luxembourg)
- CENTRE NATIONAL DE LA RECHERCHE SCIENTIFIQUE CNRS (France)
- CODEMART SRL (Romania)
- EODYNE SYSTEMS SL (Spain)
- FORSCHUNGSZENTRUM JULICH GMBH (Germany)
- FRAUNHOFER GESELLSCHAFT ZUR FOERDERUNG DER ANGEWANDTEN FORSCHUNG E.V. (Germany)
- FUNDACIO INSTITUT DE BIOENGINYERIA DE CATALUNYA (Spain)
- INSTITUT DU CERVEAU ET DE LA MOELLE EPINIERE (France)
- THE CHANCELLOR, MASTERS AND SCHOLARS OF THE UNIVERSITY OF OXFORD
- TP21 GMBH (Germany)
- UNIVERSIDAD COMPLUTENSE DE MADRID (Spain)
- UNIVERSITA DEGLI STUDI DI GENOVA (Italy)
- UNIVERSITAT WIEN (Austria)
- UNIVERSITE D'AIX MARSEILLE (France)
- Inria contact: Bertrand Thirion
- Summary: The annual worldwide cost of Alzheimer’s dementia was 777.81 billion Euro in 2015. This number will rise to 7.41 trillion Euro in 2050. Early diagnosis would save up to $7.9 trillion in medical and care costs by 2050 in the US alone. However, the emergent pathology is highly variable across people, necehighly variable across people, necessitating individualized diagnostics and interventions. The VirtualBrainCloud addresses this by bridging the gap between computational neuroscience and subcellular systems biology, integrating both research streams into a unifying computational model that supports personalized diagnostics and treatments in NDD. TheVirtualBrainCloud not only integrates existing software tools, it also merges the efforts of two big EU initiatives, namely The Virtual Brain large scale simulation platform of the EU Flagship Human Brain Project and IMI-EPAD initiative (European prevention of Alzheimer’s dementia consortium). VirtualBrainCloud will develop and validate a decision support system that provides access to high quality multi-disciplinary data for clinical practice. The result will be a cloud-based brain simulation platform to support personalized diagnostics and treatments in NDD. The EU PRACE (Partnership for Advanced Computing in Europe) initiative, will provide the required computing infrastructure. The VirtualBrainCloud will develop robust solutions for legal and ethical matters by interacting with EU projects such as European Open Science Cloud (EOSC), ‘cloud4health’, Alzheimer’s Europe patient organizations and ELIXIR, an organization that manages and safeguards EU research data. Our software developers have already produced highly successful brain simulation and clinical decision support tools. The resulting software will be a cloud based computational modeling system that is tailored to the individual, and bridges multiple scales to identify key mechanisms that predict NDD progression and serves as Precision Decision Support System.
9.3.2 Collaborations with major European organizations
PhD thesis in cotutelle with Univ. Aalto
Merlin Dumeur has obtained an ADI2020 fellowship from Univ. of Paris-Saclay for starting his 4-year PhD thesis in co-tutelle between this university and Univ Aalto under the joint supervision of Dr Philippe Ciuciu and Prof. Matias Palva. His PhD project is entitled a “New framework for critical brain dynamics” during which he will be working on new computational models of neural networks that are able to reproduce important features of human brain dynamics such as bistability, criticality and multifractality. These models will be tested on EEG and MEG data in healthy and pathological condition (epilepsy).
9.4 National initiatives
9.4.1 ANR
Neuroref: Mathematical Models of Anatomy / Neuroanatomy / Diffusion MRI
Participants: Demian Wassermann, Antonia Machlouzarides Shalit, Valentin Iovene.
While mild traumatic brain injury (mTBI) has become the focus of many neuroimaging studies, the understanding of mTBI, particularly in patients who evince no radiological evidence of injury and yet experience clinical and cognitive symptoms, has remained a complex challenge. Sophisticated imaging tools are needed to delineate the kind of subtle brain injury that is extant in these patients, as existing tools are often ill-suited for the diagnosis of mTBI. For example, conventional magnetic resonance imaging (MRI) studies have focused on seeking a spatially consistent pattern of abnormal signal using statistical analyses that compare average differences between groups, i.e., separating mTBI from healthy controls. While these methods are successful in many diseases, they are not as useful in mTBI, where brain injuries are spatially heterogeneous.
The goal of this proposal is to develop a robust framework to perform subject-specific neuroimaging analyses of Diffusion MRI (dMRI), as this modality has shown excellent sensitivity to brain injuries and can locate subtle brain abnormalities that are not detected using routine clinical neuroradiological readings. New algorithms will be developed to create Individualized Brain Abnormality (IBA) maps that will have a number of clinical and research applications. In this proposal, this technology will be used to analyze a previously acquired dataset from the INTRuST Clinical Consortium, a multi-center effort to study subjects with Post- Traumatic Stress Disorder (PTSD) and mTBI. Neuroimaging abnormality measures will be linked to clinical and neuropsychological assessments. This technique will allow us to tease apart neuroimaging differences between PTSD and mTBI and to establish baseline relationships between neuroimaging markers, and clinical and cognitive measures.
DirtyData: Data integration and cleaning for statistical analysis
Participants: Gaël Varoquaux, Pierre Glaser.
Machine learning has inspired new markets and applications by extracting new insights from complex and noisy data. However, to perform such analyses, the most costly step is often to prepare the data. It entails correcting errors and inconsistencies as well as transforming the data into a single matrix-shaped table that comprises all interesting descriptors for all observations to study. Indeed, the data often results from merging multiple sources of informations with different conventions. Different data tables may come without names on the columns, with missing data, or with input errors such as typos. As a result, the data cannot be automatically shaped into a matrix for statistical analysis.
This proposal aims to drastically reduce the cost of data preparation by integrating it directly into the statistical analysis. Our key insight is that machine learning itself deals well with noise and errors. Hence, we aim to develop the methodology to do statistical analysis directly on the original dirty data. For this, the operations currently done to clean data before the analysis must be adapted to a statistical framework that captures errors and inconsistencies. Our research agenda is inspired from the data-integration state of the art in database research combined with statistical modeling and regularization from machine learning.
Data integrating and cleaning is traditionally performed in databases by finding fuzzy matches or overlaps and applying transformation rules and joins. To incorporate it in the statistical analysis, an thus propagate uncertainties, we want to revisit those logical and set operations with statistical-learning tools. A challenge is to turn the entities present in the data into representations well-suited for statistical learning that are robust to potential errors but do not wash out uncertainty.
Prior art developed in databases is mostly based on first-order logic and sets. Our project strives to capture errors in the input of the entries. Hence we formulate operations in terms of similarities. We address typing entries, deduplication -finding different forms of the same entity- building joins across dirty tables, and correcting errors and missing data.
Our goal is that these steps should be generic enough to digest directly dirty data without user-defined rules. Indeed, they never try to build a fully clean view of the data, which is something very hard, but rather include in the statistical analysis errors and ambiguities in the data.
The methods developed will be empirically evaluated on a variety of
dataset, including the French public-data repository,
http://
FastBig Project
Participants: Bertrand Thirion, Jerome-Alexis Chevalier, Tuan Binh Nguyen.
In many scientific applications, increasingly-large datasets are being acquired to describe more accurately biological or physical phenomena. While the dimensionality of the resulting measures has increased, the number of samples available is often limited, due to physical or financial limits. This results in impressive amounts of complex data observed in small batches of samples.
A question that arises is then : what features in the data are really informative about some outcome of interest ? This amounts to inferring the relationships between these variables and the outcome, conditionally to all other variables. Providing statistical guarantees on these associations is needed in many fields of data science, where competing models require rigorous statistical assessment. Yet reaching such guarantees is very hard.
FAST-BIG aims at developing theoretical results and practical estimation procedures that render statistical inference feasible in such hard cases. We will develop the corresponding software and assess novel inference schemes on two applications : genomics and brain imaging.
MultiFracs project
Participants: Philippe Ciuciu.
The scale-free concept formalizes the intuition that, in many systems, the analysis of temporal dynamics cannot be grounded on specific and characteristic time scales. The scale-free paradigm has permitted the relevant analysis of numerous applications, very different in nature, ranging from natural phenomena (hydrodynamic turbulence, geophysics, body rhythms, brain activity,...) to human activities (Internet traffic, population, finance, art,...).
Yet, most successes of scale-free analysis were obtained in contexts where data are univariate, homogeneous along time (a single stationary time series), and well-characterized by simple-shape local singularities. For such situations, scale-free dynamics translate into global or local power laws, which significantly eases practical analyses. Numerous recent real-world applications (macroscopic spontaneous brain dynamics, the central application in this project, being one paradigm example), however, naturally entail large multivariate data (many signals), whose properties vary along time (non-stationarity) and across components (non-homogeneity), with potentially complex temporal dynamics, thus intricate local singular behaviors.
These three issues call into question the intuitive and founding identification of scale-free to power laws, and thus make uneasy multivariate scale-free and multifractal analyses, precluding the use of univariate methodologies. This explains why the concept of scale-free dynamics is barely used and with limited successes in such settings and highlights the overriding need for a systematic methodological study of multivariate scale-free and multifractal dynamics. The Core Theme of MULTIFRACS consists in laying the theoretical foundations of a practical robust statistical signal processing framework for multivariate non homogeneous scale-free and multifractal analyses, suited to varied types of rich singularities, as well as in performing accurate analyses of scale-free dynamics in spontaneous and task-related macroscopic brain activity, to assess their natures, functional roles and relevance, and their relations to behavioral performance in a timing estimation task using multimodal functional imaging techniques.
This overarching objective is organized into 4 Challenges:
- Multivariate scale-free and multifractal analysis,
- Second generation of local singularity indices,
- Scale-free dynamics, non-stationarity and non-homogeneity,
- Multivariate scale-free temporal dynamics analysis in macroscopic brain activity.
DARLING: Distributed adaptation and learning over graph signals
Participants: Philippe Ciuciu.
The project will be starting in 2020 with a post-doc to be hired probably in 2021.
The DARLING project will aim to propose new adaptive learning methods, distributed and collaborative on large dynamic graphs in order to extract structured information of the data flows generated and/or transiting at the nodes of these graphs. In order to obtain performance guarantees, these methods will be systematically accompanied by an in-depth study of random matrix theory. This powerful tool , never exploited so far in this context although perfectly suited for inference on random graphs, will thereby provide even avenues for improvement. Finally, in addition to their evaluation on public data sets, the methods will be compared with each other using two advanced imaging techniques in which two of the partners are involved: radio astronomy with the giant SKA instrument (Obs. Côte d'Azur) and magnetoencephalographic brain imaging (Inria Parietal at NeuroSpin, CEA Saclay). These involve the processing of time series on graphs while operating at extreme observation scales.
VLFMRI: Very low field MRI for babies
Participants: Philippe Ciuciu.
The project will be starting in 2021 with a post-doc or PhD student to be hired probably in fall 2021 or 2022.
VLFMRI aims at developing a very low-field Magnetic Resonance Imaging (MRI) system as an alternative to conventional high-field MRI for continuous imaging of premature newborns to detect hemorrhages or ischemia. This system is based on a combination of a new generation of magnetic sensors based on spin electronics, optimized MR acquisition sequences (based on the SPARKLING patent, Inria-CEA Parietal team at NeuroSpin) and a open and compatible system with an incubator that will allow to achieve an image resolution of 1mm
meegBIDS.fr: Standardization, sharing and analysis of MEEG data simplified by BIDS
Participants: Alexandre Gramfort.
The project accepted by ANR in 2019 started in 2020 with an engineer hired in 2020. This project is in collaboration with the MEG groups at CEA NeuroSpin and the Brain and Spine Institute (ICM) in Paris.
The neuroimaging community recently started an international effort to standardize the sharing of data recorded with magnetoencephalography (MEG) and with electroencephalography (EEG). This format, known as the Brain Imaging Data Structure (BIDS), now needs a wider adoption, notably in the French neuroimaging community, along with the development of dedicated software tools that operate seamlessly on BIDS formatted datasets. The meegBIDS.fr project has three aims: 1) accelerate the research cycles by allowing analysis software tools to work with BIDS formated data, 2) simplify data sharing with high quality standards thanks to automated validation tools, 3) train French neuroscientists to leverage existing public BIDS MEG/EEG datasets and to share their own data with little efforts.
AI-Cog: AI for Aging Societies: From Basic Concepts to Practical Tools for AI-Facilitated Cognitive Training
Participants: Alexandre Gramfort, Denis Engemann, Thomas Moreau.
The project accepted by ANR in 2020 will be starting in 2021 with an engineer and a post-doc to be hired in 2021. This project is in collaboration with the University of Freiburg in Germany and the RIKEN AIP in Japan.
Worldwide, people are living longer than ever before in history. Today, most people can expect to live into their sixties and beyond. Ageing societies, however, bring social, economical, and healthcare challenges. Japan (#1), France (#3) and Germany (#4) belong to the top five countries worldwide with the highest economic old-age dependency ratio of people aged over 65 years and more. Particularly detrimental health conditions in older age include depression, and dementia. Today, around 50 M people globally suffer from dementia and there are nearly 10 M new cases every year. According to WHO there is a new case of dementia every 3 seconds globally. Mastering the challenges associated with aging societies in general, and those associated with age-related brain disorders in particular, is therefore of outstanding global importance, and especially for the three countries involved in the present trilateral call, Japan, France, and Germany. Therefore, the aim of the present project is to leverage the potential of artificial intelligence (AI) approaches to foster healthy aging. To this aim we will study objective machine-learning-driven biomarkers to evaluate cognitive interventions as well as support personalized therapies. We will develop novel, dedicated machine learning (ML) methods and adapt them to the special signal types that can be recorded from the human brain. We will make our methods publicly available in an open-source reference software package, focussing on unsupervised learning, data augmentation, domain adaptation, and interpretable machine learning models. Our main scientific aims is to optimize the decodable information about the current functional state of the brain, to identify biomarkers of the risk for cognitive impairments and different forms of dementia, and use these improved methods to guide AI-facilitated cognitive training. These joint efforts between Japan, France and Germany will be accompanied by a focus on ethical and societal aspects of AI in the context of aging, paired with participatory, transnational outreach activities, to foster the dialog between our scientific community and the general public.
BrAIN: Bridging Artificial Intelligence and Neuroscience
Participants: Alexandre Gramfort, Denis Engemann, Thomas Moreau.
The project accepted in 2020 by ANR in the "Chaire IA" call will be starting in 2021 with an engineer, 2 PhDs and a post-doc to be hired in 2021.
The general objectives of BrAIN is to develop ML algorithms that can learn with weak or no supervision on neural time series. It will require contributions to self-supervised learning, domain adaptation and data augmentation techniques, exploiting the known underlying physical mechanisms that govern the data generating process of neurophysiological signals.
Knowledge and representations integration on the brain
Participants: Bertrand Thirion, Demian Wassermann, Badr Tajini.
The project accepted in 2020 by ANR in the "Chaire IA" call will be starting in 2021 with an engineer, 1 PhD and a starting position to be hired in 2021.
Cognitive science describes mental operations, and functional brain imaging provides a unique window into the brain systems that support these operations. A growing body of neuroimaging research has provided significant insight into the relations between psychological functions and brain activity. However, the aggregation of cognitive neuroscience results to obtain a systematic mapping between structure and function faces the roadblock that cognitive concepts are ill-defined and may not map cleanly onto the computational architecture of the brain.
To tackle this challenge, we propose to leverage rapidly increasing data sources: text and brain locations described in neuroscientific publications, brain images and their annotations taken from public data repositories, and several reference datasets. Our aim here is to develop multi-modal machine learning techniques to bridge these data sources.
LearnI: learning data integration, from discrete entities to signals
Participants: Gaël Varoquaux.
The project accepted in 2020 by ANR in the "Chaire IA" call will be starting in 2021 with an engineer, 2 PhDs and a post-doc to be hired in 2021.
The goal of LearnI is to develop machine-learning across multiple sources of relational data, with numerical and symbolic entries. LearnI will address the core challenge of joining and aggregating across tables where the information is represented with different symbols. For this, LearnI will develop methods to embed the discrete elements in vector spaces and perform data assembly across tables with these vectorial representations.
9.5 Regional initiatives
Inria - AP-HP Chaire-IA EDS_PERI-OP: Building a data warehouse for perioperative health inside the EDS/AP-HP for testing, preventing and following cardiovascular and neurocognitive complications
Participants: Étienne Gayat, Denis Engemann, Fabrice Vallée, Jona Joachim.
The project has been accepted for the Chaire IA `Donnés massives et Intelligence Artificielle en santé' by the AP-HP and its Entrepôt de Donneées de Santé (EDS, http://
LIDL: Learning priors for Inverse Problem Resolution through Deep-Learning
Participants: Thomas Moreau, Matthieu Kowalski, Benoit Malézieux.
The project has been accepted by Digiteo in 2020 and started with a PhD student who started October 2020. This project is in collaboration with the L2S from CentraleSupélec.
In many experimental science, the signal of interest can be linked to the observed signal through a system of physical equations. To invert such systems, one needs to solve inverse problems which require prior knowledge on the signal to be recovered. While some classical methods have been proposed to learn such priors, they are often slow and do not scale to large problems. In the recent year, deep learning has been used to accelerate the resolution of inverse problems with fixed priors. The goal of this thesis is to investigate how these neural network architectures could be used to also accelerate the prior learning to solve inverse problems while retaining some of the theoretical properties of the classical methods. Such methods could have implication for inverse problems in a broad range of domain, including neuroscience.
10 Dissemination
10.1 Promoting scientific activities
10.1.1 Scientific events: organisation
Nilearn days
The nilearn days, 19-22 May 2020, united 300
participants online to discuss science and software development related
to machine learning and advanced statistics on brain images. Gaël
Varoquaux was general chair and helped organizing
(https://
Missing-values workshop, ICML
Gaël Varoquaux was an organizer of the missing-values workshop at ICML 2020.
HARMONIC and MULTIFRACTAL ANALYSES: From Mathematics to Quantitative Neurosciences.
Philippe Ciuciu was a remote organizing member of this summer school that was supposed to be held on July 2020 in Montreal with the local support of the Centre de Recherches Mathématiques. Due to the pandemic, this school has been rescheduled in 2022.
BioMag2020/21
Denis Engemann is organizing the symposium `Predicting clinical endpoints from M/EEG: Challenges and opportunities of large-scale data analysis' https://
Member of the organizing committees
In 2020, Philippe Ciuciu has joined the program committee of the ESMRMB 2021.
Thomas Moreau co-organizes the Séminaire Palaisien, a machine learning and statistics seminar in Saclay that aims to gather researchers from the ecosystem of IPP and UPSa around monthly presentations. It gather an audience around 40 persons each month.
10.1.2 Scientific events: selection
Member of the conference program committees
Gaël Varoquaux was and Senior Program Committee for IJCAI (International Joint Conference on AI).
Demian Wassermann was part of the organization committee of ISMRM and IPMI
Since 2019 Philippe Ciuciu has been the representative of the IEEE Signal Processing Society at the International Symposium on Biomedical Imaging (ISBI). As such, he is member of the steering committee of this conference.
Reviewer
Gaël Varoquaux member of the program committees of ICML (International Conference on Machine Learning), NeurIPS, AISTATS, AAAI,
Alexandre Gramfort member of the program committees of ICML (International Conference on Machine Learning), NeurIPS, AISTATS, Virtual Physiological Human (VPH) and area chair at ICLR (International Conference on Learning Representation).
Thomas Moreau member of the program committees of ICML, NeurIPS, ICLR.
Demian Wassermann Member of the program and organization committees of CVPR, IPMI, MICCAI, ISMRM.
Bertrand Thirion member of the program committees of ICML, NeurIPS, AISTATS, ICLR.
10.1.3 Journal
Member of the editorial boards
elife Gaël Varoquaux is member of Board of Reviewing Editors at elife.
IEEE Open Journal on Signal Processing Philippe Ciuciu is Senior Area Editor.
Aperture Bertrand Thirion and Alexandre Gramfort are editors of the Aperture journal.
NeuroImage Alexandre Gramfort is editor of the NeuroImage journal.
JMLR Alexandre Gramfort is editor of the Journal of Machine Learning Research.
Reviewer - reviewing activities
Gael Varoquaux reviewed for MedIA (Medical Image Analysis), JMLR (Journal of Machine Learning Research), Machine Learning, Neuroscience and Behavior Reviews, and JAMA Psychiatry.
Philippe Ciuciu reviewed for IEEE TMI/TCI/TNSRE, Neuroimage, Magnetic Resonance in Medicine, MedIA, Human Brain Mapping, Frontiers in Physiology, Computers in Biology and Medicine.
Alexandre Gramfort reviewed for Nature Comms, NeuroImage, IEEE PAMI/TNSRE, JMLR, Scientific Data, eLife
Bertrand Thirion reviewed for Nature Comms, NeuroImage, IEEE TMI.
Thomas Moreau reviewed for SIAM Journal on Imaging Science.
Denis Engemann reviewed for Nature Comms, eLife, NeuroImage, Molecular Autism, Psychological Medicine, International Journal of Psychophysiology
Demian Wassermann reviewed for Nature Comms, NeuroImage, Medical Image Analysis, Brain Structure and Function.
10.1.4 Invited talks
Gaël Varoquaux
Etalab DataDrinks (online), Ray Summit (online), McGill University Biostats seminar (online), Boulder university institute for cognitive science seminar (Boulder), Cap Digital (online), John Hopkins Imaging Science institute seminar (Baltimore), NYU Tandom School of Engineering (New York), Open Data Science Conference (online), Ottawa Statistics seminar (online), PyData global (online), Laval University Economics Seminar (Quebec city)
Philippe Ciuciu
Univ. Helsinki (in-person in Feb), Heriot-Watt Univ (online), Signal & System Labs, CentraleSupélec (online), NeuroSpin Seminar Series (online), Univ. of Geneva (online), Aix-Marseille Univ (online)
Bertrand Thirion
International Research Training Group on Aggression and Impulsivity (Aachen-Philadelphia), Institut Curie, GDR Isis, Martinos Center (Boston), Recent Advances in Statistical Analysis of Imaging Data.
Alexandre Gramfort
Brown University (USA) (online), Aalto University (Finland) (online), Brain and Spine Institute (Paris), Virtual Physiological Human (VPH) conference (Paris) (online), tutorial at Pattern Recognition in NeuroImaging (PRNI) (online), tutorial at OHBM (online), tutorial at the AI4Health winter school (online), tutorial at the DS3 winter school (online), tutorial at Live MEEG workshop (France) (online).
Denis Engemann
National Multiple Sclerosis Center, Melsbroek, Belgium (online), November 2020; Higher School of Economics (HSE), Moscow, Russia (online), September 2020; Computational approaches for ageing and age-related diseases (CompAGE) Paris (online); Virtual Physiological Human (VPH) conference (Paris) (online); Feindel Virtual Seminar Series at The Neuro, Montreal, Canada (online), July 2020.
Thomas Moreau
Institut Henri Poincaré (PAris), GT PASADENA (Orsay), CIRM Optimization for ML workshop (Marseille), John Hopkins Imaging Science institute seminar (Baltimore).
10.1.5 Leadership within the scientific community
Bertrand Thirion is head of the DATAIA convergence institute and has been member of the Comité Analyse, Recherche, Expertise (CARE), created to face the Covid-19 Crisis (March-July 2020).
Alexandre Gramfort is head of the Paris-Saclay Center for Datascience (CDS).
10.1.6 Scientific expertise
In 2020, Philippe Ciuciu has been appointed by the CEA Programme Transverse de Compétences - section Simulation Numérique to join the steering committee. The latter is in charge of the annual selection of the fifteen best scientific and interdisciplinary projects in the fields of numerical simulation and artificial intelligence. The projects, which are submitted by CEA teams and external partners may receive up to 100keuros (18-month post-doc funding and seed money for experiments, etc.) for 2 years.
In 2020, Philippe Ciuciu has been appointed as CEA/DRF expert for the imagery to take part to the selection of PhD theses in the FOCUS program led by the Haut Commissaire and entitled `Experimentation et Jumeau Numérique'.
Denis Engemann has reviewed grants for several national funding bodies: Estonian Research Council; French Ministry of Europe and Foreign Affairs; Medical Research Council (MRC), UK; Swiss National Science Foundation, Switzerland.
10.1.7 Research administration
Alexandre Gramfort fait parti du comité du pole B de l'Ecole Doctorale STIC de l'Université Paris-Saclay.
10.2 Teaching - Supervision - Juries
10.2.1 Teaching
- Master: Alexandre Gramfort, Optimization for Data Science, 20h, MSc 2 Data Science Master Ecole Polytechnique, France
- Master: Alexandre Gramfort, DataCamp, 25h, Msc 2 Data Science Master Ecole Polytechnique, France
- Master: Alexandre Gramfort, Source Imaging with EEG and MEG, 10h, Msc 2 in Biomedical Imaging at Univ. Paris
- Master: Alexandre Gramfort, Source Imaging with EEG and MEG, 7h, Msc 2 in Biomedical Imaging at CentraleSupélec
- Master: Bertrand Thirion, Functional neuroimaging and BCI, 12h, Master MVA, ENS Paris-Saclay, France
- Master: Bertrand Thirion, Kshitih Chawla, Thomas Bazeille: tutorial on Nilearn, 8h, INT, Marseille.
- Master: Philippe Ciuciu, Medical Imaging course – A tour in Magnetic Resonance Imaging, 12h (9h course, 3h hands-on session), MSc 2 ATSI, CentraleSupélec, Univ. Paris-Saclay
- Master: Philippe Ciuciu, Magnetic Resonance Imaging: From data acquisition to image reconstruction and clinical applications, 20h (14h course, 6h of hands-on session), ENSEEIHT Toulouse, MSc 2 Signal & image processing
- IOGS (SupOptique) 3rd year, 3h30: A tour in Magnetic Resonance Imaging
- Bachelor: Demian Wassermann, CSE201 class, 15h, C++ programming, Ecole Polytechnique
- Master: Demian Wassermann, 7h Biomedical Engineering, Msc 2 Biomedical Engineering, Université de Paris
- Extension: Demian Wassermann, Data Science, 20h, Ecole Polytechnique
- Master: Gaël Varoquaux, machine learning on dirty data, HEC Montréal 3H
- Master: Gaël Varoquaux, Advanced machine learning for brain imaging, McGill university 2H
- Master: Gaël Varoquaux, Machine learning for digital humanities, EHESS 8h
- Doctoral school: Gaël Varoquaux, machine learning for neuroimaging, 6h, Unique days, Montréal
- Master: Thomas Moreau, DataCamp, 25h, Ms Data Science, Ecole Polytechnique, France
- Executive Master: Thomas Moreau, Machine Learning, 3h, Ms Smart Mobility, Telecom ParisTech.
- Executive Master: Thomas Moreau, Python, 9h, Ms Statistique et big Data, Université Paris-Dauphine.
- Formation continue: Thomas Moreau, Data Science, 30h, Executive Education, Ecole Polytechnique
- Master: Olivier Grisel, Deep Learning (40h), Ms Data Science, Ecole Polytechnique, France
10.2.2 Supervision
The following PhD defenses have taken place
- Kamalakar Dadi, "Machine Learning on Population Imaging for Mental Health", defended Sep 2020, directed by Gaël Varoquaux, Denis Engemman and Bertrand Thirion
- Jerome-Alexis Chevalier, “Statistical control of sparse models in high dimension”, defended Dec 2020, directed by Bertrand Thirion and Joseph Salmon.
- Antonia Machlouzarides Shalit, "Development of subject-specific representations of neuroanatomy via a domain-specific language”, defended dec 2020, directed by Demian Wassermann
Ongoing PhD theses:
- Benedict Colnet, "Causal inference on joint observational and experimental data", defense planned Oct 2023, directed by Gaël Varoquaux, and Julie Josse
- Matthieu Doutreligne, "Joining Eletronic Health Records for epidemiological studies joining hospital and liberal practice"
- Alexis Cvetkov Iliev, "embeddings of database entries", defense planned Oct 2022, directed by Gaël Varoquaux & Alexandre Allauzen (ESPCI)
- Hicham Janati, 01/10/2017, coadvised by Alexandre Gramfort and Marco Cuturi
- Quentin Bertrand, 01/10/2018, coadvised by Alexandre Gramfort and Joseph Salmon
- Hubert Banville, 01/10/2018, coadvised by Alexandre Gramfort and Denis Engemann
- David Sabbagh, 01/10/2018, coadvised by Alexandre Gramfort and Denis Engemann
- Charlotte Caucheteux, 01/10/2020, coadvised by Alexandre Gramfort and Jean-Rémi King
- Omar Chehab, 01/11/2020, advised by Alexandre Gramfort
- Cédric Allain, 01/01/2021, coadvised by Alexandre Gramfort and Thomas Moreau
- Jérôme-Alexis Chevalier, 01/10/2017, coadvised by Bertrand Thirion and Joseph Salmon
- Hugo Richard, 01/10/2018, coadvised by Bertrand Thirion and Olivier Grisel
- Thomas Bazeille, 01/10/2018, advised by Bertrand Thirion
- Tuan Binh Nguyen, 01/10/2018, coadvised by Bertrand thirion and Sylvain Arlot
- Jospeh Benzakoun, 01/10/2018, caodavised by Bertrand Thirion and Catherine Oppenheim
- Valentin Iovene, 01/10/2018, advised by Demian Wassermann
- Antonia Machlouzarides Shalit, 01/10/2018, coadvised by Demian Wassermann and Bertrand Thirion
- Maeliss Jallais, 01/10/2018, advised by Demian Wassermann
- Chengran Fan, 01/10/2019, co-advised by Demian Wassermann and Jing-Rebecca Li
- Gaston Zanitti, 01/11/2019, advised by Demian Wassermann
- Hamza Cherkaoui, 01/10/2017, co-advised by Philippe Ciuciu and Thomas Moreau (PhD thesis extended due to the COVID-19 pandemic, and defense planned on March 3rd 2021)
- Zaccharie Ramzi, 12/02/2019, co-advised by Philippe Ciuciu and Jean-Luc Starck
- Guillaume Daval-Frérot, 01/11/2019, co-advised by Philippe Ciuciu and Alexandre Vignaud
- Zaineb Amor, 01/09/2020, co-adivsed by Philippe Ciuciu and Alexandre Vignaud
- Chaithya G R, 19/11/2020, advised by Philippe Ciuciu,
- Merlin Dumeur, 01/12/2020, co-advised by Philippe Ciuciu and Matias Palva
- Benoit Malézieux, 01/10/2020, advised by Thomas Moreau and Matthieu Kowalski
10.2.3 Juries
Bertrand Thirion has been involved in the following committees:
- PhD - Wei Jiang /ecole Polytechnique
- PhD - Nicolas Clairis / University of Paris
- Habilitation - Cloe Azencott / Institut Curie
- Habilitation - Pierre Neuvial / University of Toulouse
Philippe Ciuciu has been involved in the following committees:
- PhD - Oumaima El Mansouri, University of Toulouse
- PhD - AntoniaMachlouzarides-Shalit, University of Paris-Saclay
Denis Engemann has been involved in the following committees:
- PhD - Laura Sophie Imperatori (reviewer), IMT School for Advanced Studies Lucca, Lucca
Gael Varoquaux has been involved in the following committees:
- PhS - Abraham Nunnes, Dalhousie university (Halifax)
Alexandre Gramfort has been involved in the following committees:
- PhD - Nathan de Lara (reviewer), Télécom ParisTech
- PhD - Baptiste Lefebvre, Université PSL ENS
- PhD - Pierre Humbert, Université Paris-Saclay
- PhD - Johann Faouzi, Sorbonne Université
- PhD - Jean Feydy, Université Paris-Saclay
- PhD - Jules Brochard, Sorbonne Université
- PhD - Mohamed El Amine Seddik, Université Paris-Saclay
- PhD - Khanh Hung Tran, Université Paris-Saclay
- PhD - Guillaume Devineau, Université PSL, Mines ParisTech
- HdR - Samuel Vaiter, Université de Bourgogne
- HdR - Bertrand Rivet (reviewer), Université Grenoble Alpes (UGA)
Demian Wassermann has been involved in the following committees:
- PhD - Katrin Rojkova / Université Paris-Sorbonne
- PhD - Céline Delettre / Université Paris-Sorbonne
Thomas Moreau has been involved in the following committees:
- PhD - Vanessa d'Amario (reviewer) / Université de Genova
10.3 Popularization
10.3.1 Internal or external Inria responsibilities
Demian Wassermann Member of the computational infrastructure group of Inria Paris Saclay-Île-de-France
Thomas Moreau Member of the computational infrastructure group of Inria Paris Saclay-Île-de-France
10.3.2 Articles and contents
Philippe Ciuciu has published an article in the special issue entitled `Sacrées Mathématiques' of the journal Clefs-CEA (number 70, March 2020). His article in French is entitled De la tête aux étoiles and reflects the joint work he has for the past four years with astrophysicists at CEA (CosmoStat lab, head: JL Starck).
Philippe Ciuciu has published a Fait Marquant on the
Joliot institute website, available both in French and English after finishing runner-up in the 2020 brain fastMRI challenge organized by Facebook AI Research and NYU, Langone Health Center. A press release has also been written by CEA, see https://
10.3.3 Education
Loïc Esteven, Olivier Grisel, Guillaume Lemaître, and Gaël Varoquaux are working on a MOOC on scikit-learn for machine learning.
11 Scientific production
11.1 Major publications
- 1 articleStochastic Subsampling for Factorizing Huge MatricesIEEE Transactions on Signal Processing661January 2018, 113-128
11.2 Publications of the year
International journals
- 2 articleVariability in the analysis of a single neuroimaging dataset by many teamsNature5827810May 2020, 84-88
- 3 articleInternational electronic health record-derived COVID-19 clinical course profiles: the 4CE consortiumnpj Digital Medicine31December 2020, #109
- 4 article Analyzing Brain Networks in Population Neuroscience: A Case for the Bayesian Philosophy Philosophical Transactions of the Royal Society of London. B (1887–1895) January 2020
- 5 article Encoding high-cardinality string categorical variables IEEE Transactions on Knowledge and Data Engineering 2020
- 6 article Predictive pattern classification can distinguish gender identity subtypes from behavior and brain imaging Cerebral Cortex February 2020
- 7 articleFine-grain atlases of functional modes for fMRI analysisNeuroImage221July 2020, 117126
- 8 article NeuroQuery, comprehensive meta-analysis of human brain mapping eLife March 2020
- 9 articleDark Control: The Default Mode Network as a Reinforcement Learning AgentHuman Brain Mapping4112August 2020, 3318-3341
- 10 articleBrain topography beyond parcellations: local gradients of functional mapsNeuroImageJanuary 2021, 117706
- 11 article Combining magnetoencephalography with magnetic resonance imaging enhances learning of surrogate-biomarkers eLife May 2020
- 12 articleDiffusion MRI simulation of realistic neurons with SpinDoctor and the Neuron ModuleNeuroImage222November 2020, 117198
- 13 articlepyts: A Python Package for Time Series ClassificationJournal of Machine Learning Research212020, 1 - 6
- 14 article Combined behavioral and electrophysiological evidence for a direct cortical effect of prefrontal tDCS on disorders of consciousness Scientific Reports March 2020
- 15 article Multi-Scale Network Regression for Brain-Phenotype Associations Human Brain Mapping 2020
- 16 article 10,000 Social Brains: Sex Differentiation in Human Brain Anatomy Annales Archéologiques Arabes Syriennes March 2020
- 17 article Revisiting Functional Connectivity for Infraslow Scale-Free Brain Dynamics using Complex Wavelet Frontiers in Physiology 2020
- 18 article 3D variable-density SPARKLING trajectories for high-resolution T2*-weighted Magnetic Resonance imaging NMR in Biomedicine May 2020
- 19 article Dual Extrapolation for Sparse GLMs Journal of Machine Learning Research 21 1-33 October 2020
- 20 article Microstructural organization of human insula is linked to its macrofunctional circuitry and predicts cognitive control eLife 9 June 2020
- 21 article DiCoDiLe: Distributed Convolutional Dictionary Learning IEEE Transactions on Pattern Analysis and Machine Intelligence 2020
- 22 article Subject‐specific segregation of functional territories based on deep phenotyping Human Brain Mapping December 2020
- 23 article Individual Brain Charting dataset extension, second release of high-resolution fMRI data for cognitive mapping Scientific Data 7 1 October 2020
- 24 article Benchmarking MRI Reconstruction Neural Networks on Large Public Datasets Applied Sciences March 2020
- 25 articleNew insights into the neural foundations mediating movement/language interactions gained from intrasurgical direct electrostimulationsBrain and Cognition142July 2020, 105583
- 26 article Predictive regression modeling with MEG/EEG: from source power to signals and cognitive states NeuroImage April 2020
- 27 article Inferring disease subtypes from clusters in explanation space Scientific Reports July 2020
- 28 article Population variability in social brain morphology for social support, household size, and friendship satisfaction Social Cognitive and Affective Neuroscience June 2020
- 29 article Finding the needle in a high-dimensional haystack: Canonical correlation analysis for neuroscientists NeuroImage April 2020
International peer-reviewed conferences
- 30 inproceedings Guidelines to use Transfer Learning for Motor Imagery Detection: an experimental study 10th International IEEE/EMBS Conference on Neural Engineering (NER). virtually, United States May 2021
- 31 inproceedings An Open Experimental Platform for Ranging, Proximity and Contact Event Tracking using Ultra-Wide-Band and Bluetooth Low-Energy CNERT 2021 - IEEE INFOCOM Workshop on Computer and Networking Experimental Research using Testbeds Virtual, France https://infocom2021.ieee-infocom.org/ May 2021
- 32 inproceedings Diffusion MRI-based Connectivity Enriched with Microstructure Information Predicts the Propagation of Cortico-Cortical Evoked Potentials ISMRM 2020 - 28th Annual Meeting & Exhibition of the International Society for Magnetic Resonance in Medicine Sydney / Virtual, Australia August 2020
- 33 inproceedings Cytoarchitecture Measurements in Brain Gray Matter using Likelihood-Free Inference IPMI 2021 Rønne, Denmark June 2021
- 34 inproceedings Indetermination-free cytoarchitecture measurements in brain gray matter via a forward diffusion MRI signal separation method ISMRM 2020 - 28th Annual Meeting & Exhibition Sydney / Virtual, Australia August 2020
- 35 inproceedingsExtraction of Nystagmus Patterns from Eye-Tracker Data with Convolutional Sparse CodingEMBC 2020 - 42nd Annual International Conferences of the IEEE Engineering in Medicine and Biology Society in conjunction with the 43rd Annual Conference of the Canadian Medical and Biological Engineering Society42Annual International Conference of the IEEE Engineering in Medicine & Biology Society (EMBC)Montreal / Virtuel, CanadaJuly 2020, 928-931
- 36 inproceedingsLinear predictor on linearly-generated data with missing values: non consistency and solutionsInternational Conference on Artificial Intelligence and StatisticsPMLR 108Proceedings of Machine Learning ResearchOnline, France2020, 3165-3174
- 37 inproceedings Multivariate analysis is sufficient for lesion-behaviour mapping MICCAI BrainLes 2020 workshop Lima, Peru October 2020
- 38 inproceedings Aggregation of Multiple Knockoffs Proceedings of the 37th International Conference on Machine Learning, PMLR 119, 2020 ICML 2020 - 37th International Conference on Machine Learning Proceedings of the ICML 37th International Conference on Machine Learning, 119 Vienne / Virtual, Austria July 2020
- 39 inproceedings Benchmarking Deep Nets MRI Reconstruction Models on the FastMRI Publicly Available Dataset ISBI 2020 - International Symposium on Biomedical Imaging Iowa City, United States April 2020
- 40 inproceedingsModeling Shared Responses in Neuroimaging Studies through MultiView ICAAdvances in Neural Information Processing Systems33Vancouver, CanadaDecember 2020, 19149--19162
Conferences without proceedings
- 41 inproceedings Implicit differentiation of Lasso-type models for hyperparameter optimization ICML 2020 - 37th International Conference on Machine Learning Vienna / Virtuel, Austria https://icml.cc/Conferences/2020 July 2020
- 42 inproceedings Learning to solve TV regularized problems with unrolled algorithms NeurIPS 2020 - 34th Conference on Neural Information Processing Systems Vancouver / Virtuel, Canada https://neurips.cc/Conferences/2020/AcceptedPapersInitial December 2020
- 43 inproceedings Statistical control for spatio-temporal MEG/EEG source imaging with desparsified multi-task Lasso NeurIPS 2020 - 34h Conference on Neural Information Processing Systems Vancouver /Virtual, Canada December 2020
- 44 inproceedings Learning summary features of time series for likelihood free inference Workshop "Machine Learning and the Physical Sciences" at the NeurIPS 2020 - 34th Conference on Neural Information Processing Systems Vancouver, Canada December 2020
- 45 inproceedings Lesion-Robust White-Matter Bundle Identification through Diffusion Driven Label Fusion ISMRM 2020 - 28th Annual Meeting & Exhibition Sydney / Virtual, Australia August 2020
- 46 inproceedings Probabilistic Programming in neurolang: Bridging the Gap Between Cognitive Science and Statistical Modeling 2020 OHBM - Annual Meeting of Organization for Human Brain Mapping Remote due to Covid-19, France January 2020
- 47 inproceedings Debiased Sinkhorn barycenters ICML 2020 - 37th International Conference on Machine Learning Vienna / Virtuel, Austria July 2020
- 48 inproceedings Entropic Optimal Transport between Unbalanced Gaussian Measures has a Closed Form NeurIPS 2020 - 34th Conference on Neural Information Processing Systems Vancouver / Virtuel, Canada December 2020
- 49 inproceedings NeuMiss networks: differentiable programming for supervised learning with missing values Advances in Neural Information Processing Systems 33 NeurIPS 2020 - 34th Conference on Neural Information Processing Systems Vancouver / Virtual, Canada December 2020
- 50 inproceedings Diffusion MRI simulation of realistic neurons with SpinDoctor and the Neuron Module ISMRM 2020 - 28th Annual Meeting & Exhibition Sydney, Australia April 2020
- 51 inproceedings Denoising Score-Matching for Uncertainty Quantification in Inverse Problems NeurIPS 2020 - 34th Conference on Neural Information Processing Systems / Workshop on Deep Learning and Inverse Problems Vancouver / Virtuel, Canada December 2020
- 52 inproceedings Wavelets in the Deep Learning ERA Eusipco Amsterdam, Netherlands January 2021
- 53 inproceedings Probabilistic Mapping of Dark Matter by Neural Score Matching NeurIPS 2020 - 3rd Workshop on Machine Learning and the Physical Sciences Vancouver / Virtuel, Canada December 2020
Scientific book chapters
- 54 inbook Single Encoding Diffusion MRI: a Probe to Brain Anisotropy Anisotropy Across Fields and Scales February 2021
Doctoral dissertations and habilitation theses
- 55 thesis Machine Learning on Population Imaging for Mental Health Université Paris-Saclay September 2020
Reports & preprints
- 56 misc Spectral independent component analysis with noise modeling for M/EEG source separation September 2020
- 57 misc Interactive Psychometrics for Autism with the Human Dynamic Clamp: Interpersonal Synchrony from Sensory-motor to Socio-cognitive Domains January 2020
- 58 misc Uncovering the structure of clinical EEG signals with self-supervised learning September 2020
- 59 misc An empirical evaluation of functional alignment using inter-subject decoding December 2020
- 60 misc Anderson acceleration of coordinate descent November 2020
- 61 misc A Lightweight Neural Model for Biomedical Entity Linking December 2020
- 62 misc Multivariate semi-blind deconvolution of fMRI time series November 2020
- 63 misc Leveraging Global Parameters for Flow-based Neural Posterior Estimation February 2021
- 64 misc Causal inference methods for combining randomized trials and observational studies: a review November 2020
- 65 misc Beyond brain age: Empirically-derived proxy measures of mental health October 2020
- 66 misc Fine-grain atlases of functional modes for fMRI analysis March 2020
- 67 misc Globally optimized 3D SPARKLING trajectories for high-resolution T2*-weighted Magnetic Resonance Imaging December 2020
- 68 misc ICU Bed Availability Monitoring and analysis in the Grand Est region of France during the COVID-19 epidemic May 2020
- 69 misc Complex Coordinate-Based Meta-Analysis with Probabilistic Programming January 2021
- 70 misc On the consistency of supervised learning with missing values June 2020
- 71 misc Model identification and local linear convergence of coordinate descent November 2020
- 72 misc Support recovery and sup-norm convergence rates for sparse pivotal estimation January 2020
- 73 misc Quantitative modeling links in vivo microstructural and macrofunctional organization of human and macaque insular cortex, and predicts cognitive control abilities January 2020
- 74 misc State-of-the-art Machine Learning MRI Reconstruction in 2020: Results of the Second fastMRI Challenge December 2020
- 75 misc XPDNet for MRI Reconstruction: an Application to the fastMRI 2020 Brain Challenge November 2020
- 76 misc Hydroxychloroquine with or without azithromycin and in-hospital mortality or discharge in patients hospitalized for COVID-19 infection: a cohort study of 4,642 in-patients in France November 2020
Other scientific publications
- 77 misc Indetermination-free cytoarchitecture measurements in brain gray matter via an inverse diffusion MRI signal separation method Sydney / virtual, Australia August 2020
- 78 misc NeuroLang: Representing Neuroanatomy with Sulcus-Specific Queries Montreal, Canada June 2020
11.3 Cited publications
- 79 articlePower failure: why small sample size undermines the reliability of neuroscienceNature Reviews Neuroscience1452013, 365--376
- 80 articleScanning the horizon: towards transparent and reproducible neuroimaging researchNature Reviews Neuroscience1822017, 115--126