Keywords
Computer Science and Digital Science
- A3.3. Data and knowledge analysis
- A3.3.2. Data mining
- A3.3.3. Big data analysis
- A3.4. Machine learning and statistics
- A3.4.1. Supervised learning
- A3.4.2. Unsupervised learning
- A3.4.4. Optimization and learning
- A3.4.5. Bayesian methods
- A3.4.6. Neural networks
- A3.4.7. Kernel methods
- A3.4.8. Deep learning
- A5.3.2. Sparse modeling and image representation
- A5.3.3. Pattern recognition
- A5.9.1. Sampling, acquisition
- A5.9.2. Estimation, modeling
- A5.9.3. Reconstruction, enhancement
- A5.9.6. Optimization tools
- A6.2.4. Statistical methods
- A6.2.6. Optimization
- A9.2. Machine learning
- A9.3. Signal analysis
- A9.7. AI algorithmics
Other Research Topics and Application Domains
- B1.2. Neuroscience and cognitive science
- B1.2.1. Understanding and simulation of the brain and the nervous system
- B1.2.2. Cognitive science
- B2.2.6. Neurodegenerative diseases
- B2.6.1. Brain imaging
1 Team members, visitors, external collaborators
Research Scientists
- Bertrand Thirion [Team leader, Inria, Senior Researcher, HDR]
- Philippe Ciuciu [CEA, Researcher, HDR]
- Benedicte Colnet [Inria, Researcher]
- Denis Alexander Engemann [Inria, Advanced Research Position, until Oct 2021]
- Alexandre Gramfort [Inria, Senior Researcher, HDR]
- Marine Le Morvan [Inria, Researcher, from Oct 2021]
- Thomas Moreau [Inria, Researcher]
- Gaël Varoquaux [Inria, Senior Researcher, HDR]
- Demian Wassermann [Inria, Researcher, HDR]
Faculty Member
- Matthieu Kowalski [Univ Paris-Saclay, Associate Professor]
Post-Doctoral Fellows
- Majd Abdallah [Inria]
- Judith Abecassis [Inria]
- Jerome Alexis Chevalier [Inria, until Jun 2021]
- Pedro Luiz Coelho Rodrigues [Inria, until Sep 2021]
- Marine Le Morvan [CNRS, until Sep 2021]
- Cédric Rommel [Inria]
PhD Students
- Cedric Allain [Inria]
- Zaineb Amor [CEA]
- Thomas Bazeille [Inria, until Sep 2021]
- Quentin Bertrand [Inria, until Oct 2021]
- Alexandre Blain [Univ Paris-Saclay, from Nov 2021]
- Samuel Brasil De Albuquerque [INSERM, from Sep 2021]
- Charlotte Caucheteux [Facebook, CIFRE]
- Ahmad Chamma [Inria]
- Thomas Chapalain [Ecole normale supérieure Paris-Saclay, from Oct 2021]
- L Emir Omar Chehab [Inria]
- Hamza Cherkaoui [CEA, until Mar 2021]
- Pierre Antoine Comby [Univ Paris-Saclay, from Oct 2021]
- Alexis Cvetkov-Iliev [Inria]
- Mathieu Dagreou [Inria, from Oct 2021]
- Guillaume Daval-Frerot [CEA]
- Matthieu Doutreligne [Haute autorité de santé, from May 2021]
- Merlin Dumeur [Univ Paris-Saclay]
- Chaithya Giliyar Radhkrishna [CEA]
- Leo Grinsztajn [Inria, from Oct 2021]
- Valentin Iovene [Inria, until Oct 2021]
- Hubert Jacob Banville [Interaxon Inc]
- Maeliss Jallais [Inria]
- Hicham Janati [Inria, until Mar 2021]
- Julia Linhart [Ecole normale supérieure Paris-Saclay, from Nov 2021]
- Benoit Malezieux [Inria]
- Apolline Mellot [Inria, from Oct 2021]
- Raphael Meudec [Inria]
- Thomas Meunier [Inria, from Oct 2021]
- Tuan Binh Nguyen [Inria]
- Alexandre Pasquiou [Inria]
- Alexandre Perez [Inria, from Sep 2021]
- Zaccharie Ramzi [CEA]
- Hugo Richard [Univ Paris-Saclay]
- Louis Rouillard–Odera [Inria]
- David Sabbagh [INSERM]
- Alexis Thual [CEA]
- Gaston Zanitti [Inria]
Technical Staff
- Alexandre Abadie [Inria, Engineer]
- Himanshu Aggarwal [Inria, Engineer]
- David Arturo Amor Quiroz [Inria, Engineer, from Jul 2021]
- Loïc Estève [Inria, Engineer]
- Guillaume Favelier [Inria, Engineer]
- Nicolas Gensollen [Inria, Engineer]
- Olivier Grisel [Inria, Engineer]
- Benjamin Habert [Inria, Engineer]
- Richard Höchenberger [Inria, Engineer]
- Julien Jerphanion [Inria, Engineer, from Apr 2021]
- Guillaume Lemaitre [Inria, Engineer, until Mar 2021]
- Chiara Marmo [Inria, Engineer, until Jun 2021]
- Jonas Renault [Inria, Engineer]
- Tomas Rigaux [Inria, Engineer, Dec 2021]
- Swetha Shankar [Inria, Engineer]
- Maria Telenczuk [Inria, Engineer, until Apr 2021]
- Jérémie du Boisberranger [Inria, Engineer]
Interns and Apprentices
- Pierre Antoine Bannier [Inria, from Mar 2021 until Sep 2021]
- Mathis Batoul [Inria, from Mar 2021 until Sep 2021]
- Alexandre Blain [Inria, from May 2021 until Nov 2021]
- Lilian Boulard [Inria, Apprentice]
- Thomas Chapalain [Ecole normale supérieure Paris-Saclay, from Apr 2021 until Sep 2021]
- Tomas D'amelio [Inria, until Jun 2021]
- Mathieu Dagreou [Inria, from Apr 2021 until Sep 2021]
- Nanxin Feng [Inria, from Mar 2021 until Jul 2021]
- Theo Gnassounou [Ecole normale supérieure Paris-Saclay, from Feb 2021 until Jun 2021]
- Apolline Mellot [Inria, from Feb 2021 until Aug 2021]
- Joseph Paillard [Inria, from Jun 2021]
- Alexandre Perez [Inria, from Apr 2021 until Aug 2021]
- Kumari Pooja [CEA, from Mar 2021 until Oct 2021]
- Jeanne Ramambason [Inria, from May 2021 until Aug 2021]
- Charbel Raphael Segerie [Inria, from Apr 2021 until Aug 2021]
- Maelys Solal [École polytechnique, until Mar 2021]
- Hassiba Tej [Inria, from Jul 2021 until Aug 2021]
Administrative Assistant
- Corinne Petitot [Inria]
Visiting Scientists
- Michael Betancourt [Symplectomorphic, Sep 2021]
- Joseph Hellerstein [University of California-Berkeley, Sep 2021]
- Neil David Lawrence [Université de Cambridge - Royaume Uni, Sep 2021]
- Madeleine Udell [Cornell University, Sep 2021]
External Collaborators
- Hamza Cherkaoui [INSERM, from May 2021 until Sep 2021]
- Samuel Davenport [Univ de Toulouse 1 Capitole, from Feb 2021]
- Elvis Dohmatob [Criteo, Jan 2021]
- Denis Alexander Engemann [F. Hoffmann-La Roche A.G., from Nov 2021]
- Jiaping Liu [Bureau of Meteoroly - Australie, until May 2021]
- Romuald Menuet [Owkin France, until May 2021]
- Sofiane Mrah [Institut du Cerveau et de la Moelle Epinière, Jan 2021]
- Joseph Salmon [Institut Telecom ex GET Groupe des Écoles des Télécommunications , until Oct 2021, HDR]
- Juan Jesus Torre Tresols [Institut supérieur de l'aéronautique et de l'espace , until Sep 2021]
2 Overall objectives
The Parietal team focuses on mathematical methods for modeling and statistical inference based on neuroimaging data, with a particular interest in machine learning techniques and applications of human functional imaging. This general theme splits into four research axes:
- Modeling for neuroimaging population studies,
- Encoding and decoding models for cognitive imaging,
- Statistical and machine learning methods for large-scale data,
- Compressed-sensing for MRI.
Parietal is also strongly involved in open-source software development in scientific Python (machine learning) and for neuroimaging applications.
3 Research program
3.1 Inverse problems in Neuroimaging
Many problems in neuroimaging can be framed as forward and inverse problems. For instance, brain population imaging is concerned with the inverse problem that consists in predicting individual information (behavior, phenotype) from neuroimaging data, while the corresponding forward problem boils down to explaining neuroimaging data with the behavioral variables. Solving these problems entails the definition of two terms: a loss that quantifies the goodness of fit of the solution (does the model explain the data well enough?), and a regularization scheme that represents a prior on the expected solution of the problem. These priors can be used to enforce some properties on the solutions, such as sparsity, smoothness or being piece-wise constant.
Let us detail the model used in typical inverse problem: Let be a neuroimaging dataset as an matrix, where and are the number of subjects under study, and the image size respectively, a set of values that represent characteristics of interest in the observed population, written as matrix, where is the number of characteristics that are tested, and an array of shape that represents a set of pattern-specific maps. In the first place, we may consider the columns of independently, yielding problems to be solved in parallel:
where the vector contains is the row of . As the problem is clearly ill-posed, it is naturally handled in a regularized regression framework:
where is an adequate penalization used to regularize the solution:
with (this formulation particularly highlights the fact that convex regularizers are norms or quasi-norms). In general, only one or two of these constraints is considered (hence is enforced with a non-zero coefficient):
- When only (LASSO), and to some extent, when only (elastic net), the optimal solution is (possibly very) sparse, but may not exhibit a proper image structure; it does not fit well with the intuitive concept of a brain map.
- Total Variation regularization (see Fig. 1) is obtained for ( only), and typically yields a piece-wise constant solution. It can be associated with Lasso to enforce both sparsity and sparse variations.
- Smooth lasso is obtained with ( and only), and yields smooth, compactly supported spatial basis functions.
Note that, while the qualitative aspect of the solutions are very different, the predictive power of these models is often very close.
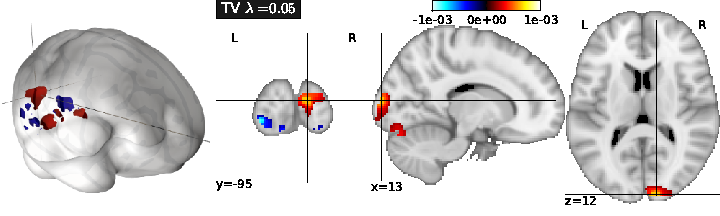
The performance of the predictive model can simply be evaluated as the amount of variance in fitted by the model, for each . This can be computed through cross-validation, by learning on some part of the dataset, and then estimating using the remainder of the dataset.
This framework is easily extended by considering
- Grouped penalization, where the penalization explicitly includes a prior clustering of the features, i.e. voxel-related signals, into given groups. This amounts to enforcing structured priors on the solution.
- Combined penalizations, i.e. a mixture of simple and group-wise penalizations, that allow some variability to fit the data in different populations of subjects, while keeping some common constraints.
- Logistic and hinge regression, where a non-linearity is applied to the linear model so that it yields a probability of classification in a binary classification problem.
- Robustness to between-subject variability to avoid the learned model overly reflecting a few outlying particular observations of the training set. Note that noise and deviating assumptions can be present in both and
-
Multi-task learning: if several target variables are thought to be related, it might be useful to constrain the estimated parameter vector to have a shared support across all these variables.
For instance, when one of the variables is not well fitted by the model, the estimation of other variables may provide constraints on the support of and thus, improve the prediction of .
3then
4
3.2 Multivariate decompositions
Multivariate decompositions provide a way to model complex data such as brain activation images: for instance, one might be interested in extracting an atlas of brain regions from a given dataset, such as regions exhibiting similar activity during a protocol, across multiple protocols, or even in the absence of protocol (during resting-state). These data can often be factorized into spatial-temporal components, and thus can be estimated through regularized Principal Components Analysis (PCA) algorithms, which share some common steps with regularized regression.
Let be a neuroimaging dataset written as an matrix, after proper centering; the model reads
where represents a set of spatial maps, hence a matrix of shape , and the associated subject-wise loadings. While traditional PCA and independent components analysis (ICA) are limited to reconstructing components within the space spanned by the column of , it seems desirable to add some constraints on the rows of , that represent spatial maps, such as sparsity, and/or smoothness, as it makes the interpretation of these maps clearer in the context of neuroimaging. This yields the following estimation problem:
where represents the columns of . can be chosen such as in Eq. (2) in order to enforce smoothness and/or sparsity constraints.
The problem is not jointly convex in all the variables but each penalization given in Eq (2) yields a convex problem on for fixed, and conversely. This readily suggests an alternate optimization scheme, where and are estimated in turn, until convergence to a local optimum of the criterion. As in PCA, the extracted components can be ranked according to the amount of fitted variance. Importantly, also, estimated PCA models can be interpreted as a probabilistic model of the data, assuming a high-dimensional Gaussian distribution (probabilistic PCA).
Ultimately, the main limitations to these algorithms is the cost due to the memory requirements: holding datasets with large dimension and large number of samples (as in recent neuroimaging cohorts) leads to inefficient computation. To solve this issue, online methods are particularly attractive 1.
3.3 Covariance estimation
Another important estimation problem stems from the general issue of learning the relationship between sets of variables, in particular their covariance. Covariance learning is essential to model the dependence of these variables when they are used in a multivariate model, for instance to study potential interactions among them and with other variables. Covariance learning is necessary to model latent interactions in high-dimensional observation spaces, e.g. when considering multiple contrasts or functional connectivity data.
The difficulties are two-fold: on the one hand, there is a shortage of data to learn a good covariance model from an individual subject, and on the other hand, subject-to-subject variability poses a serious challenge to the use of multi-subject data. While the covariance structure may vary from population to population, or depending on the input data (activation versus spontaneous activity), assuming some shared structure across problems, such as their sparsity pattern, is important in order to obtain correct estimates from noisy data. Some of the most important models are:
- Sparse Gaussian graphical models, as they express meaningful conditional independence relationships between regions, and do improve conditioning/avoid overfit.
- Decomposable models, as they enjoy good computational properties and enable intuitive interpretations of the network structure. Whether they can faithfully or not represent brain networks is still an open question.
- PCA-based regularization of covariance which is powerful when modes of variation are more important than conditional independence relationships.
Adequate model selection procedures are necessary to achieve the right level of sparsity or regularization in covariance estimation; the natural evaluation metric here is the out-of-sample likelihood of the associated Gaussian model. Another essential remaining issue is to develop an adequate statistical framework to test differences between covariance models in different populations. To do so, we consider different means of parametrizing covariance distributions and how these parametrizations impact the test of statistical differences across individuals.
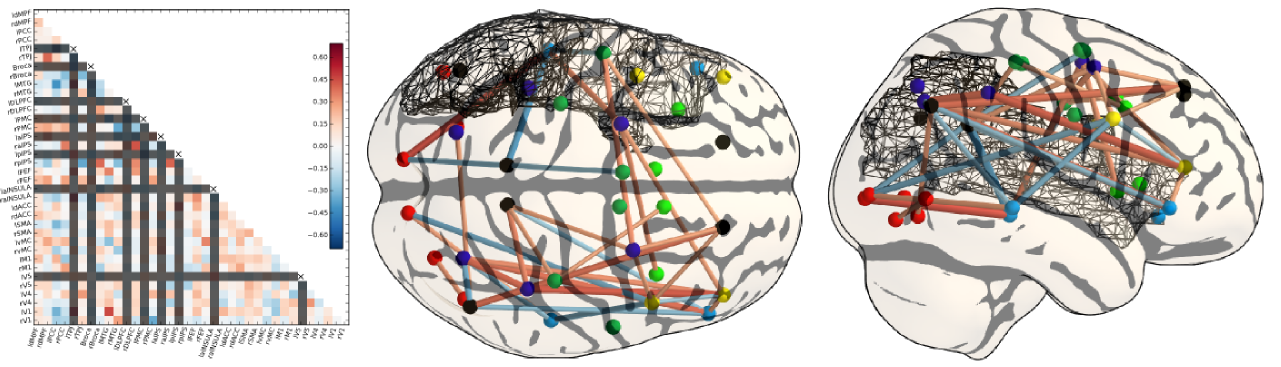
4 Application domains
4.1 Cognitive neuroscience
Macroscopic Functional cartography with functional Magnetic Resonance Imaging (fMRI)
The brain as a highly structured organ, with both functional specialization and a complex network organization. While most of the knowledge historically comes from lesion studies and animal electophysiological recordings, the development of non-invasive imaging modalities, such as fMRI, has made it possible to study routinely high-level cognition in humans since the early 90's. This has opened major questions on the interplay between mind and brain , such as: How is the function of cortical territories constrained by anatomy (connectivity) ? How to assess the specificity of brain regions ? How can one characterize reliably inter-subject differences ?
Analysis of brain Connectivity
Functional connectivity is defined as the interaction structure that underlies brain function. Since the beginning of fMRI, it has been observed that remote regions sustain high correlation in their spontaneous activity, i.e. in the absence of a driving task. This means that the signals observed during resting-state define a signature of the connectivity of brain regions. The main interest of resting-state fMRI is that it provides easy-to-acquire functional markers that have recently been proved to be very powerful for population studies.
Modeling of brain processes (MEG)
While fMRI has been very useful in defining the function of regions at the mm scale, Magneto-encephalography (MEG) provides the other piece of the puzzle, namely temporal dynamics of brain activity, at the ms scale. MEG is also non-invasive. It makes it possible to keep track of precise schedule of mental operations and their interactions. It also opens the way toward a study of the rhythmic activity of the brain. On the other hand, the localization of brain activity with MEG entails the solution of a hard inverse problem.
Current challenges in human neuroimaging (acquisition+analysis)
Human neuroimaging targets two major goals: i) the study of neural responses involved in sensory, motor or cognitive functions, in relation to models from cognitive psychology, i.e. the identification of neurophysiological and neuroanatomical correlates of cognition; ii) the identification of markers in brain structure and function of neurological or psychiatric diseases. Both goals have to deal with a tension between
- the search for higher spatial 1 resolution to increase spatial specificity of brain signals, and clarify the nature (function and structure) of brain regions. This motivates efforts for high-field imaging and more efficient acquisitions, such as compressed sensing schemes, as well as better source localization methods from M/EEG data.
- the importance of inferring brain features with population-level validity, hence, contaminated with high variability within observed cohorts, which blurs the information at the population level and ultimately limits the spatial resolution of these observations.
Importantly, the signal-to-noise ratio (SNR) of the data remains limited due to both resolution improvements 2 and between-subject variability. Altogether, these factors have led to realize that results of neuroimaging studies were statistically weak, i.e. plagued with low power and leading to unreliable inference 60, and particularly so due to the typically number of subjects included in brain imaging studies (20 to 30, this number tends to increase 61): this is at the core of the neuroimaging reproducibility crisis. This crisis is deeply related to a second issue, namely that only few neuroimaging datasets are publicly available, making it impossible to re-assess a posteriori the information conveyed by the data. Fortunately, the situation improves, lead by projects such as NeuroVault or OpenfMRI. A framework for integrating such datasets is however still missing.
5 Highlights of the year
5.1 Awards
- Hugo Richard, PhD student of the team, got the STIC « Doctorants » Prize delivered by DigiCosme Labex, the STIC doctoral school of Paris Saclay University, and the IP Paris doctoral school. This prize concerns the works on the MultiViewICA work.
- Maëliss Jallais, PhD student of the team, got the cum laude award for her abstract submitted to the International Symposium of Magnetic Resonance in Medicine 2021 concerning a simulation-based inference system for diffusion MRI analyses.
- Bertrand Thirion got the Ordre National du Mérite Award.
6 New software and platforms
Parietal has a long tradition of software development.
6.1 New software
6.1.1 Mayavi
-
Functional Description:
Mayavi is the most used scientific 3D visualization Python software. Mayavi can be used as a visualization tool, through interactive command line or as a library. It is distributed under Linux through Ubuntu, Debian, Fedora and Mandriva, as well as in PythonXY and EPD Python scientific distributions. Mayavi is used by several software platforms, such as PDE solvers (fipy, sfepy), molecule visualization tools and brain connectivity analysis tools (connectomeViewer).
- URL:
-
Contact:
Gael Varoquaux
6.1.2 Nilearn
-
Name:
NeuroImaging with scikit learn
-
Keywords:
Health, Neuroimaging, Medical imaging
-
Functional Description:
NiLearn is the neuroimaging library that adapts the concepts and tools of scikit-learn to neuroimaging problems. As a pure Python library, it depends on scikit-learn and nibabel, the main Python library for neuroimaging I/O. It is an open-source project, available under BSD license. The two key components of NiLearn are i) the analysis of functional connectivity (spatial decompositions and covariance learning) and ii) the most common tools for multivariate pattern analysis. A great deal of efforts has been put on the efficiency of the procedures both in terms of memory cost and computation time.
- URL:
-
Contact:
Bertrand Thirion
-
Participants:
Alexandre Abraham, Alexandre Gramfort, Bertrand Thirion, Elvis Dohmatob, Fabian Pedregosa Izquierdo, Gael Varoquaux, Loic Esteve, Michael Eickenberg, Virgile Fritsch
6.1.3 Scikit-learn
-
Keywords:
Regession, Clustering, Learning, Classification, Medical imaging
-
Scientific Description:
Scikit-learn is a Python module integrating classic machine learning algorithms in the tightly-knit scientific Python world. It aims to provide simple and efficient solutions to learning problems, accessible to everybody and reusable in various contexts: machine-learning as a versatile tool for science and engineering.
-
Functional Description:
Scikit-learn can be used as a middleware for prediction tasks. For example, many web startups adapt Scikitlearn to predict buying behavior of users, provide product recommendations, detect trends or abusive behavior (fraud, spam). Scikit-learn is used to extract the structure of complex data (text, images) and classify such data with techniques relevant to the state of the art.
Easy to use, efficient and accessible to non datascience experts, Scikit-learn is an increasingly popular machine learning library in Python. In a data exploration step, the user can enter a few lines on an interactive (but non-graphical) interface and immediately sees the results of his request. Scikitlearn is a prediction engine . Scikit-learn is developed in open source, and available under the BSD license.
- URL:
-
Contact:
Olivier Grisel
-
Participants:
Alexandre Gramfort, Bertrand Thirion, Fabian Pedregosa Izquierdo, Gael Varoquaux, Loic Esteve, Michael Eickenberg, Olivier Grisel
-
Partners:
CEA, Logilab, Nuxeo, Saint Gobain, Tinyclues, Telecom Paris
6.1.4 MODL
-
Name:
Massive Online Dictionary Learning
-
Keywords:
Pattern discovery, Machine learning
-
Functional Description:
Matrix factorization library, usable on very large datasets, with optional sparse and positive factors.
- URL:
- Publications:
-
Contact:
Arthur Mensch
-
Participants:
Arthur Mensch, Gael Varoquaux, Bertrand Thirion, Julien Mairal
6.1.5 MNE
-
Name:
MNE-Python
-
Keywords:
Neurosciences, EEG, MEG, Signal processing, Machine learning
-
Functional Description:
Open-source Python software for exploring, visualizing, and analyzing human neurophysiological data: MEG, EEG, sEEG, ECoG, and more.
-
Release Contributions:
https://mne.tools/stable/whats_new.html
- URL:
-
Contact:
Alexandre Gramfort
-
Partners:
HARVARD Medical School, New York University, University of Washington, CEA, Aalto university, Telecom Paris, Boston University, UC Berkeley
6.1.6 Dmipy
-
Name:
Diffusion MRI Multi-Compartment Modeling and Microstructure Recovery Made Easy
-
Keywords:
Diffusion MRI, Multi-Compartment Modeling, Microstructure Recovery
-
Functional Description:
Non-invasive estimation of brain microstructure features using diffusion MRI (dMRI) – known as Microstructure Imaging – has become an increasingly diverse and complicated field over the last decades. Multi-compartment (MC)-models, representing the measured diffusion signal as a linear combination of signal models of distinct tissue types, have been developed in many forms to estimate these features. However, a generalized implementation of MC-modeling as a whole, providing deeper insights in its capabilities, remains missing. To address this fact, we present Diffusion Microstructure Imaging in Python (Dmipy), an open-source toolbox implementing PGSE-based MC-modeling in its most general form. Dmipy allows on-the-fly implementation, signal modeling, and optimization of any user-defined MC-model, for any PGSE acquisition scheme. Dmipy follows a “building block”-based philosophy to Microstructure Imaging, meaning MC-models are modularly constructed to include any number and type of tissue models, allowing simultaneous representation of a tissue's diffusivity, orientation, volume fractions, axon orientation dispersion, and axon diameter distribution. In particular, Dmipy is geared toward facilitating reproducible, reliable MC-modeling pipelines, often allowing the whole process from model construction to parameter map recovery in fewer than 10 lines of code. To demonstrate Dmipy's ease of use and potential, we implement a wide range of well-known MC-models, including IVIM, AxCaliber, NODDI(x), Bingham-NODDI, the spherical mean-based SMT and MC-MDI, and spherical convolution-based single- and multi-tissue CSD. By allowing parameter cascading between MC-models, Dmipy also facilitates implementation of advanced approaches like CSD with voxel-varying kernels and single-shell 3-tissue CSD. By providing a well-tested, user-friendly toolbox that simplifies the interaction with the otherwise complicated field of dMRI-based Microstructure Imaging, Dmipy contributes to more reproducible, high-quality research.
-
Authors:
Rutger Fick, Demian Wassermann, Rachid Deriche, Samuel Deslauriers-Gauthier
-
Contact:
Rachid Deriche
6.1.7 PySAP
-
Name:
Python Sparse data Analysis Package
-
Keywords:
Image reconstruction, Image compression
-
Functional Description:
The PySAP (Python Sparse data Analysis Package, https://github.com/CEA-COSMIC/pysap) open-source image processing software package has been developed for the 3 years between the Compressed Sensing group at Iniria-CEA Parietal team led by Philippe Ciuciu and the CosmoStat team (CEA/IRFU) led by Jean-Luc Statck. It has been developed for the COmpressed Sensing for Magnetic resonance Imaging and Cosmology (COSMIC) project. This package provides a set of flexible tools that can be applied to a variety of compressed sensing and image reconstruction problems in various research domains. In particular, PySAP offers fast wavelet transforms and a range of integrated optimisation algorithms. It also offers a variety of plugins for specific application domains: on top of Pysap-MRI and PySAP-astro plugins, several complementary modules are now in development for electron tomography and electron microscopy for CEA colleagues. In October 2019, PySAP has been released on PyPi (https://pypi.org/project/python-pySAP/, currently version 0.0.3) and in conda (https://anaconda.org/agrigis/python-pysap).
The Pysap-MRI has been advertised through a specific abstract accepted to the next workshop of ISMRM on Data Sampling & Image Reconstruction in late January 2020. It will be presented during a power pitch session together wih an hands-on demo session using JuPyter notebooks.
-
Contact:
Philippe Ciuciu
-
Partner:
CEA
6.2 New platforms
Parietal is involved in the Neurospin platform.
Participants: Philippe Ciuciu.
7 New results
Participants: Bertrand Thirion, Gael Varoquaux, Thomas Moreau, Alexandre Gramfort, Demian Wassermann, Olivier Grisel, Philippe Ciuciu.
7.1 An empirical evaluation of functional alignment using inter-subject decoding
Inter-individual variability in the functional organization of the brain presents a major obstacle to identifying generalizable neural coding principles. Functional alignment—a class of methods that matches subjects’ neural signals based on their functional similarity—is a promising strategy for addressing this variability. To date, however, a range of functional alignment methods have been proposed and their relative performance is still unclear. In this work, we benchmark five functional alignment methods for inter-subject decoding on four publicly available datasets. Specifically, we consider three existing methods: piecewise Procrustes, searchlight Procrustes, and piecewise Optimal Transport. We also introduce and benchmark two new extensions of functional alignment methods: piecewise Shared Response Modelling (SRM), and intra-subject alignment. We find that functional alignment generally improves inter-subject decoding accuracy though the best performing method depends on the research context. Specifically, SRM and Optimal Transport perform well at both the region-of-interest level of analysis as well as at the whole-brain scale when aggregated through a piecewise scheme. We also benchmark the computational efficiency of each of the surveyed methods, providing insight into their usability and scalability. Taking inter-subject decoding accuracy as a quantification of inter-subject similarity, our results support the use of functional alignment to improve inter-subject comparisons in the face of variable structure-function organization. We provide open implementations of all methods used.
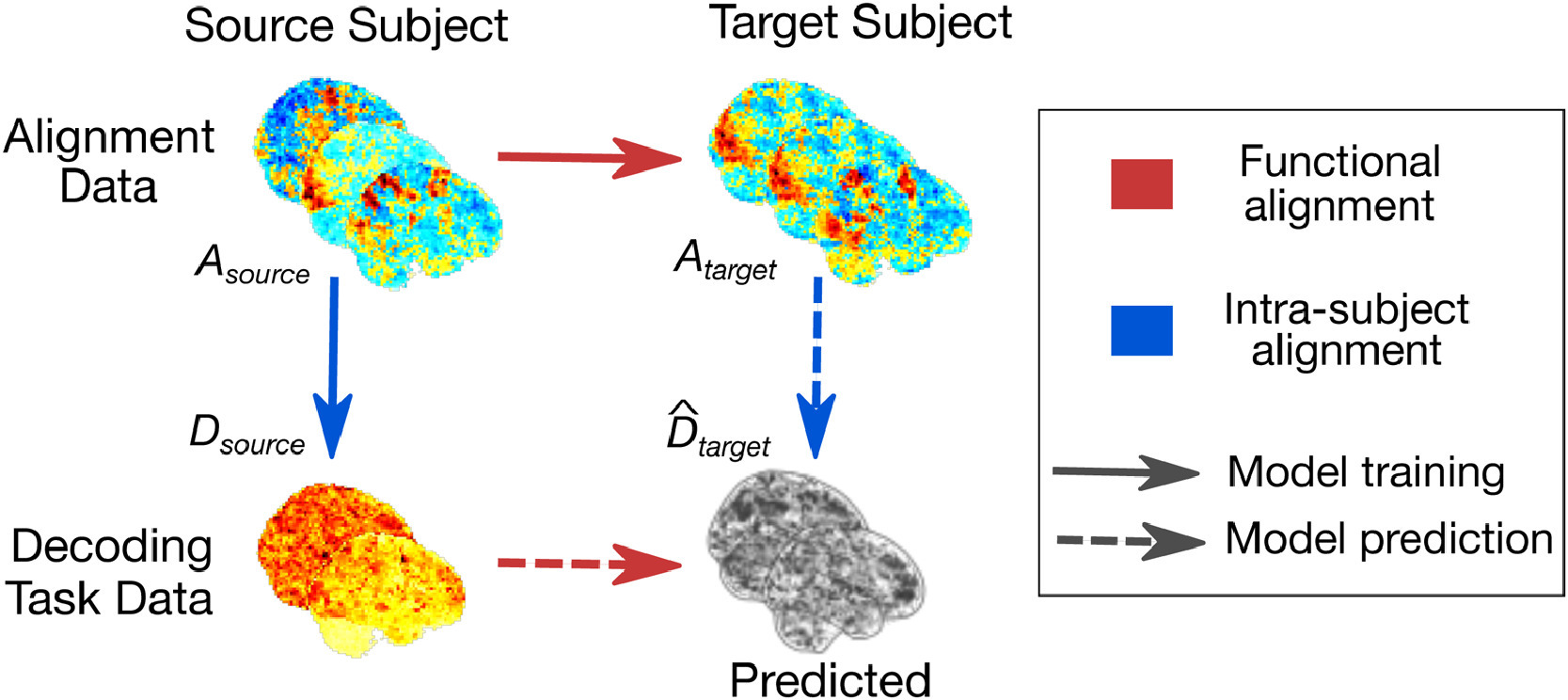
Principle of functional alignment The goal of functional alignment is to learn correspondence between data drawn from two subjects
7.2 Extracting representations of cognition across neuroimaging studies improves brain decoding
Cognitive brain imaging is accumulating datasets about the neural substrate of many different mental processes. Yet, most studies are based on few subjects and have low statistical power. Analyzing data across studies could bring more statistical power; yet the current brain-imaging analytic framework cannot be used at scale as it requires casting all cognitive tasks in a unified theoretical framework. We introduce a new methodology to analyze brain responses across tasks without a joint model of the psychological processes. The method boosts statistical power in small studies with specific cognitive focus by analyzing them jointly with large studies that probe less focal mental processes. Our approach improves decoding performance for 80% of 35 widely-different functional-imaging studies. It finds commonalities across tasks in a data-driven way, via common brain representations that predict mental processes. These are brain networks tuned to psychological manipulations. They outline interpretable and plausible brain structures. The extracted networks have been made available; they can be readily reused in new neuro-imaging studies. We provide a multi-study decoding tool to adapt to new data.
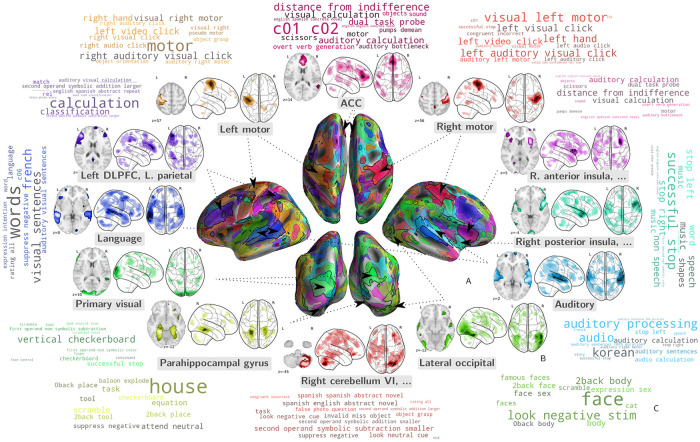
Our approach learns networks that are important for decoding across studies
7.3 Uncovering the structure of clinical EEG signals with self-supervised learning
Supervised learning paradigms are often limited by the amount of labeled data that is available. This phenomenon is particularly problematic in clinically-relevant data, such as electroencephalography (EEG), where labeling can be costly in terms of specialized expertise and human processing time. Consequently, deep learning architectures designed to learn on EEG data have yielded relatively shallow models and performances at best similar to those of traditional feature-based approaches. However, in most situations, unlabeled data is available in abundance. By extracting information from this unlabeled data, it might be possible to reach competitive performance with deep neural networks despite limited access to labels.
We investigated self-supervised learning (SSL), a promising technique for discovering structure in unlabeled data, to learn representations of EEG signals. Specifically, we explored two tasks based on temporal context prediction as well as contrastive predictive coding on two clinically-relevant problems: EEG-based sleep staging and pathology detection. We conducted experiments on two large public datasets with thousands of recordings and performed baseline comparisons with purely supervised and hand-engineered approaches.
Linear classifiers trained on SSL-learned features consistently outperformed purely supervised deep neural networks in low-labeled data regimes while reaching competitive performance when all labels were available. Additionally, the embeddings learned with each method revealed clear latent structures related to physiological and clinical phenomena, such as age effects.
We demonstrate the benefit of SSL approaches on EEG data. Our results suggest that self-supervision may pave the way to a wider use of deep learning models on EEG data.
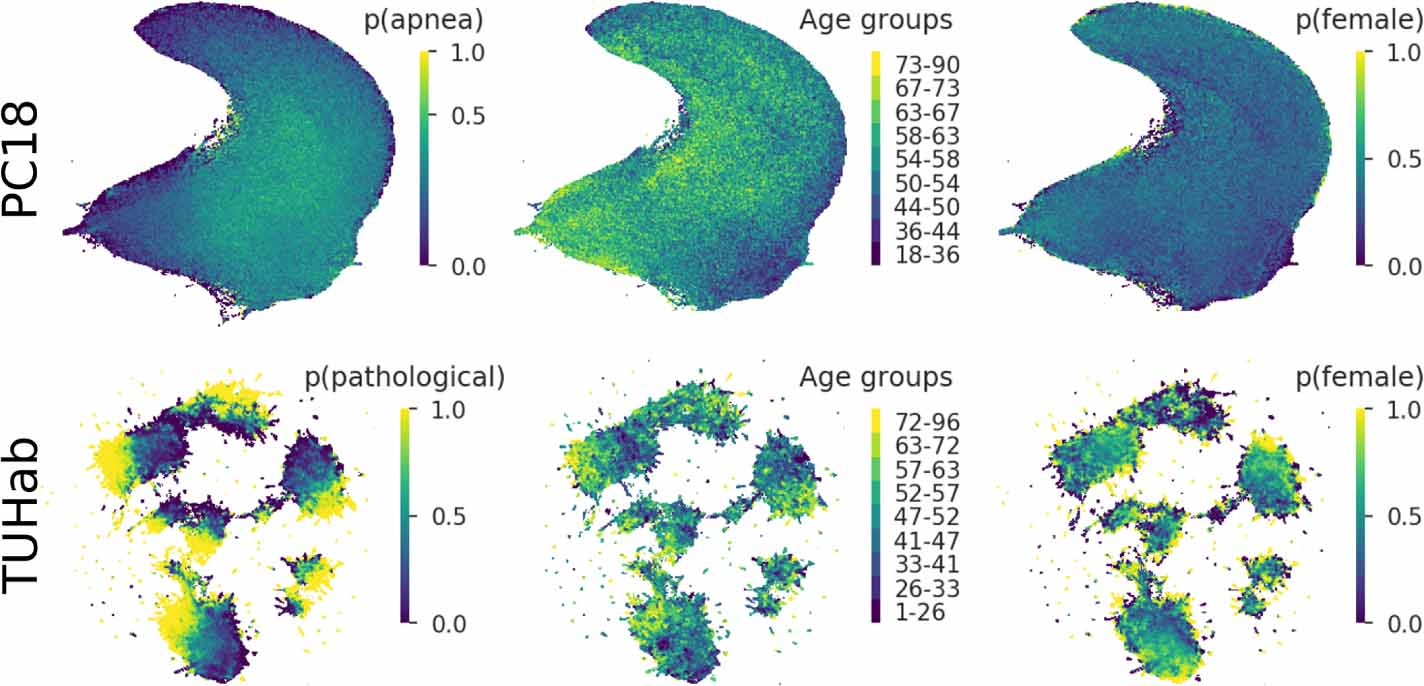
Structure learned by the embedders trained on the temporal shuffling task
7.4 What's a good imputation to predict with missing values?
How to learn a good predictor on data with missing values? Most efforts focus on first imputing as well as possible and second learning on the completed data to predict the outcome. Yet, this widespread practice has no theoretical grounding. Here we show that for almost all imputation functions, an impute-then-regress procedure with a powerful learner is Bayes optimal. This result holds for all missing-values mechanisms, in contrast with the classic statistical results that require missing-at-random settings to use imputation in probabilistic modeling. Moreover, it implies that perfect conditional imputation is not needed for good prediction asymptotically. In fact, we show that on perfectly imputed data the best regression function will generally be discontinuous, which makes it hard to learn. Crafting instead the imputation so as to leave the regression function unchanged simply shifts the problem to learning discontinuous imputations. Rather, we suggest that it is easier to learn imputation and regression jointly. We propose such a procedure, adapting NeuMiss, a neural network capturing the conditional links across observed and unobserved variables whatever the missing-value pattern. Experiments confirm that joint imputation and regression through NeuMiss is better than various two step procedures in our experiments with finite number of samples.
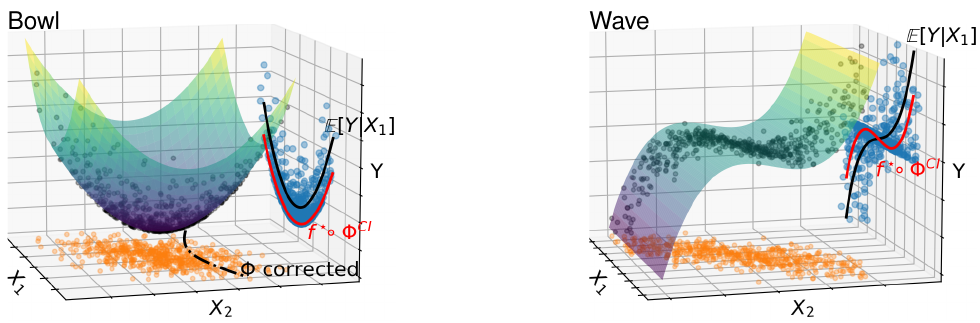
Discussion on the appropriateness of imputation models in non-linear regression.
7.5 HNPE: Leveraging Global Parameters for Neural Posterior Estimation
Inferring the parameters of a stochastic model based on experimental observations is central to the scientific method. A particularly challenging setting is when the model is strongly indeterminate, i.e. when distinct sets of parameters yield identical observations. This arises in many practical situations, such as when inferring the distance and power of a radio source (is the source close and weak or far and strong?) or when estimating the amplifier gain and underlying brain activity of an electrophysiological experiment. In this work, we present hierarchical neural posterior estimation (HNPE), a novel method for cracking such indeterminacy by exploiting additional information conveyed by an auxiliary set of observations sharing global parameters. Our method extends recent developments in simulation- based inference (SBI) based on normalizing flows to Bayesian hierarchical models. We validate quantitatively our proposal on a motivating example amenable to analytical solutions and then apply it to invert a well known non-linear model from computational neuroscience.
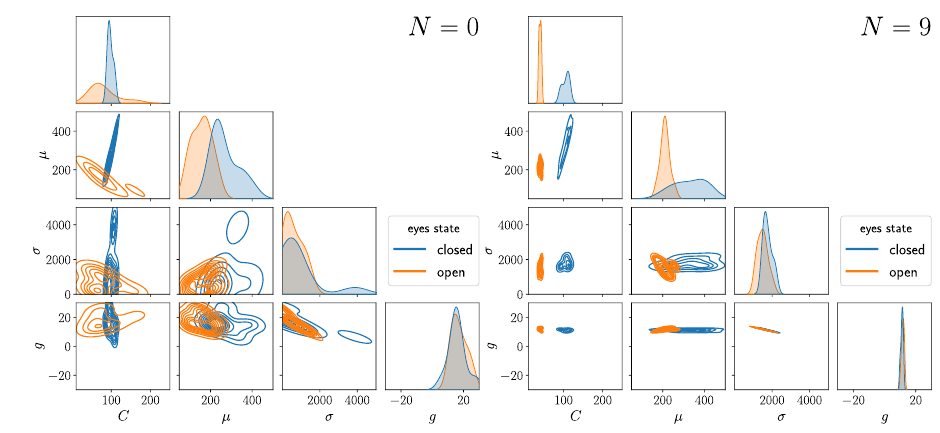
Posterior estimates for the parameters of the neural mass model computed on human EEG signals.
7.6 Shared Independent Component Analysis for Multi-Subject Neuroimaging
We consider shared response modeling, a multi-view learning problem where one wants to identify common components from multiple datasets or views. We introduce Shared Independent Component Analysis (ShICA) that models each view as a linear transform of shared independent components contaminated by additive Gaussian noise. We show that this model is identifiable if the components are either non-Gaussian or have enough diversity in noise variances. We then show that in some cases multi-set canonical correlation analysis can recover the correct unmixing matrices, but that even a small amount of sampling noise makes Multiset CCA fail. To solve this problem, we propose to use joint diagonalization after Multiset CCA, leading to a new approach called ShICA-J. We show via simulations that ShICA-J leads to improved results while being very fast to fit. While ShICA-J is based on second-order statistics, we further propose to leverage non-Gaussianity of the components using a maximum-likelihood method, ShICA-ML, that is both more accurate and more costly. Further, ShICA comes with a principled method for shared components estimation. Finally, we provide empirical evidence on fMRI and MEG datasets that ShICA yields more accurate estimation of the components than alternatives.
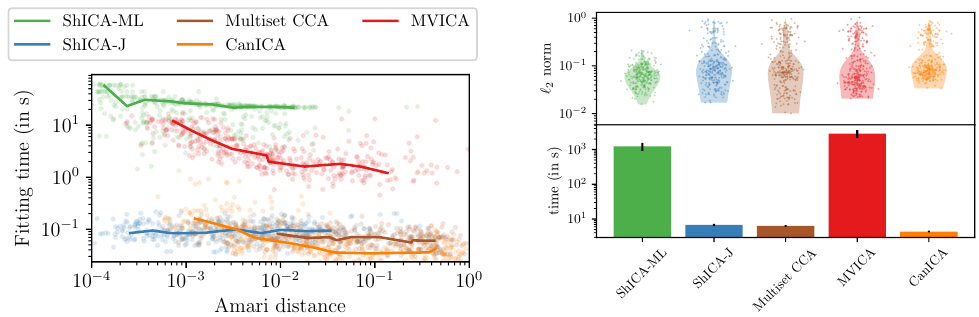
Empirical performance of shared Independent Components Analysis Models
7.7 Disentangling Syntax and Semantics in the Brain with Deep Networks
The activations of language transformers like GPT-2 have been shown to linearly map onto brain activity during speech comprehension. However, the nature of these activations remains largely unknown and presumably conflate distinct linguistic classes. Here, we propose a taxonomy to factorize the high-dimensional activations of language models into four combinatorial classes: lexical, compositional, syntactic, and semantic representations. We then introduce a statistical method to decompose, through the lens of GPT-2's activations, the brain activity of 345 subjects recorded with functional magnetic resonance imaging (fMRI) during the listening of 4.6 hours of narrated text. The results highlight two findings. First, compositional representations recruit a more widespread cortical network than lexical ones, and encompass the bilateral temporal, parietal and prefrontal cortices. Second, contrary to previous claims, syntax and semantics are not associated with separated modules, but, instead, appear to share a common and distributed neural substrate. Overall, this study introduces a versatile framework to isolate, in the brain activity, the distributed representations of linguistic constructs.
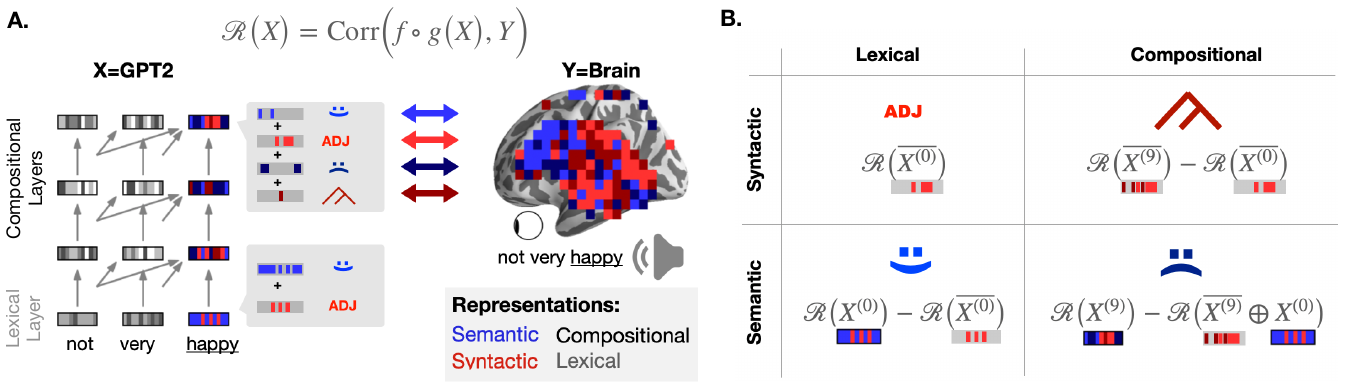
Method to decompose the language representations shared between brains and deep language models
7.8 Cytoarchitecture Measurements in Brain Gray Matter using Likelihood-Free Inference
Effective characterisation of the brain grey matter cytoarchitecture with quantitative sensitivity to soma density and volume remains an unsolved challenge in diffusion MRI (dMRI). Solving the problem of relating the dMRI signal with cytoarchitectural characteristics calls for the definition of a mathematical model that describes brain tissue via a handful of physiologically-relevant parameters and an algorithm for inverting the model. To address this issue, we propose a new forward model, specifically a new system of equations, requiring six relatively sparse b-shells. These requirements are a drastic reduction of those used in current proposals to estimate grey matter cytoarchitecture. We then apply current tools from Bayesian analysis known as likelihood-free inference (LFI) to invert our proposed model. As opposed to other approaches from the literature, our LFI-based algorithm yields not only an estimation of the parameter vector that best describes a given observed data point, but also a full posterior distribution over the parameter space. This enables a richer description of the model inversion results providing indicators such as confidence intervals for the estimations, and better understanding of the parameter regions where the model may present indeterminacies. We approximate the posterior distribution using deep neural density estimators, known as normalizing flows, and fit them using a set of repeated simulations from the forward model. We validate our approach on simulations using dmipy and then apply the whole pipeline to the HCP MGH dataset.
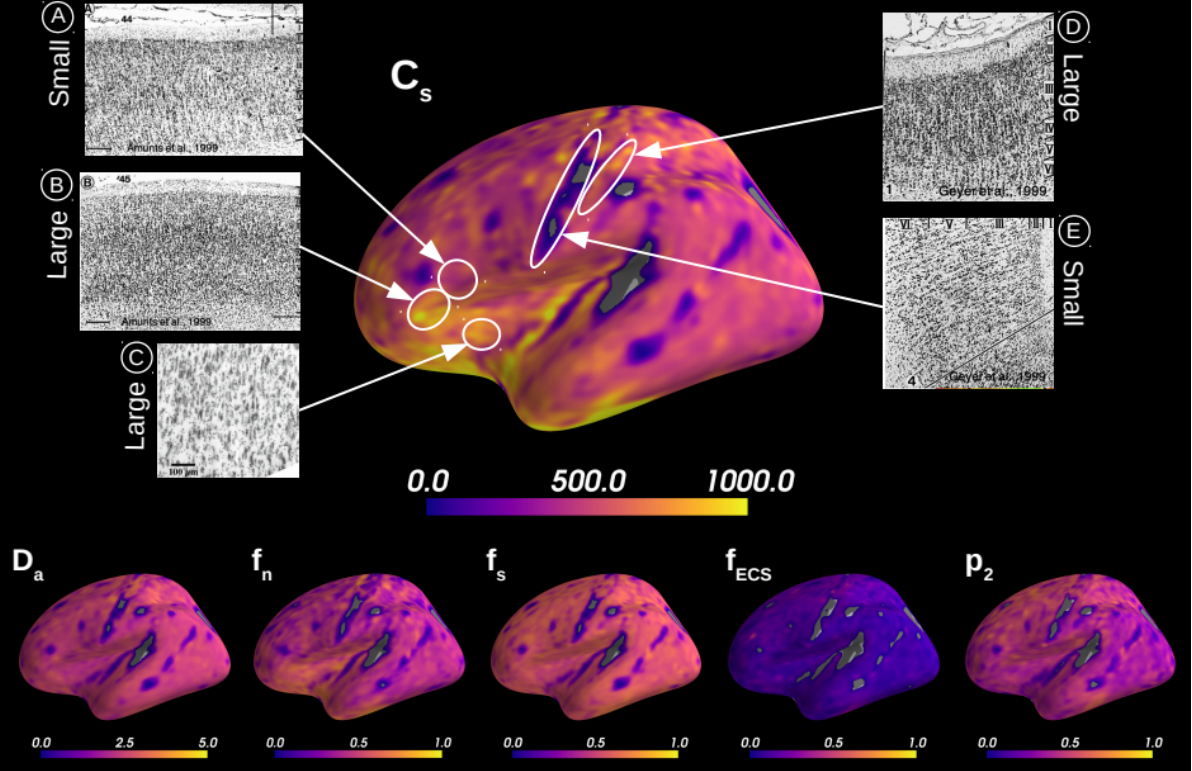
Microstructural measurements averaged over 31 HCP MGH subjects
7.9 Results of the 2020 fastMRI Challenge for Machine Learning MR Image Reconstruction
Accelerating MRI scans is one of the principal outstanding problems in the MRI research community. Towards this goal, we hosted the second fastMRI competition targeted towards reconstructing MR images with subsampled k-space data. We provided participants with data from 7,299 clinical brain scans (de-identified via a HIPAA-compliant procedure by NYU Langone Health), holding back the fully-sampled data from 894 of these scans for challenge evaluation purposes. In contrast to the 2019 challenge, we focused our radiologist evaluations on pathological assessment in brain images. We also debuted a new Transfer track that required participants to submit models evaluated on MRI scanners from outside the training set. We received 19 submissions from eight different groups. Results showed one team scoring best in both SSIM scores and qualitative radiologist evaluations. We also performed analysis on alternative metrics to mitigate the effects of background noise and collected feedback from the participants to inform future challenges. Lastly, we identify common failure modes across the submissions, highlighting areas of need for future research in the MRI reconstruction community.
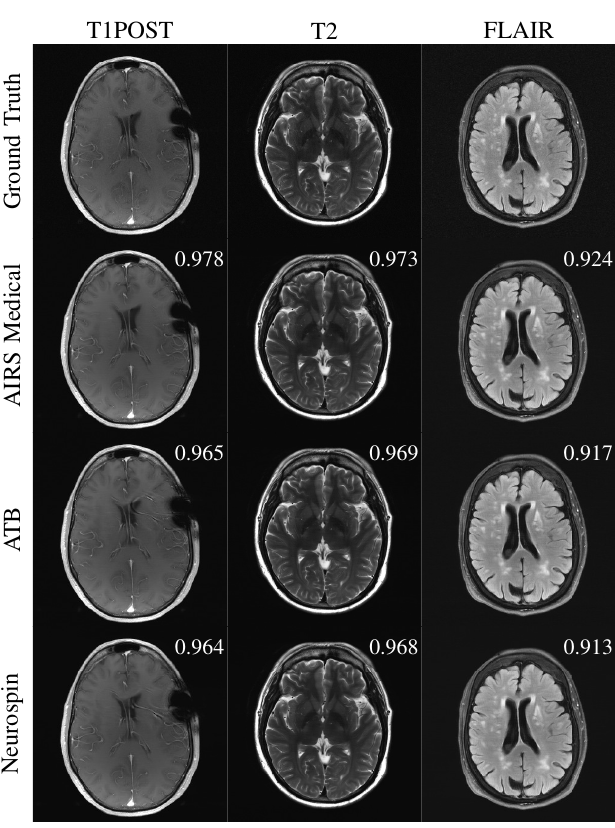
Examples of 4X submissions evaluated by radiologists with slice-level SSIM scores
8 Bilateral contracts and grants with industry
Participants: Gael Varoquaux, Thomas Moreau, Alexandre Gramfort, Philippe Ciuciu.
8.1 Bilateral contracts with industry
- Since 2020, a CIFRE PhD thesis has been launched with Facebook AI Research France. This contract supports the PhD thesis of Charlotte Caucheteux.
- Since 2019, a CIFRE PhD thesis has been launched with Siemens-Healthineers France. This contract supports the PhD thesis of Guillaume Daval-Frérot.
- Since 2018, a CIFRE PhD thesis has been launched with InteraXon, Ca. This contract supports the PhD thesis of Hubert Banville.
- Since 2020, Thomas Moreau is a consultant on machine learning for health care for Qynapse, France. The consulting sessions take place approximately once a month.
8.2 Bilateral grants with industry
Cython+
The Cython+ grant, funded by BPI France, and Region Ile de France, unites Inria, Telecom Paristech, Nexedis, and Abilian to improve parallel computing in Python.
9 Partnerships and cooperations
9.1 International initiatives
9.1.1 Associate Teams in the framework of an Inria International Lab or in the framework of an Inria International Program
LargeSmallBrainNets
-
Title:
Characterizing Large and Small-scale Brain Networks in Typical Populations Using Novel Computational Methods for dMRI and fMRI-based Connectivity and Microstructure
-
Duration:
2019 -> 2022
-
Coordinator:
Vinod Menon (menon@stanford.edu)
-
Partners:
- Stanford University
-
Inria contact:
Demian Wassermann
-
Summary:
The major goal of this project is to develop and validate sophisticated computational tools for identifying functional nodes at the whole-brain level and measuring structural and functional connectivity between them, using state-of-the-art human brain MR imaging techniques and open-source datasets such as Human Connectome Project data. Our proposed methods will reveal in unprecedented detail the structural and functional connectivity of the human brain. Furthermore, our innovative computational approach to brain connectomics will help create the building blocks for shaping the next generation of research on brain function and psychopathology.
Meta&Co
-
Title:
Meta-Analysis of Neuro-Cognitive Associations
-
Duration:
2018 ->
-
Coordinator:
Russel Poldrack (poldrack@stanford.edu)
-
Partners:
- Stanford University
-
Inria contact:
Bertrand Thirion
-
Summary:
Cognitive science and psychiatry describe mental operations: cognition, emotion, perception and their dysfunction. Cognitive neuroimaging bridge these mental concepts to their implementation in the brain, neural firing and wiring, by relying on functional brain imaging. Yet aggregating results from experiments probing brain activity into a consistent description faces the roadblock that cognitive concepts and brain pathologies are ill-defined. Separation between them is often blurry. In addition, these concepts and subdivisions may not correspond to actual brain structures or systems. To tackle this challenge, we propose to adapt data-mining techniques used to learn relationships in computational linguistics. Natural language processing uses distributional semantics to build semantic relationships and ontologies. New models are needed to learn relationships from heterogeneous signals: functional magnetic resonance images (fMRI), on the one hand, combined with related psychology and neuroimaging annotations or publications, on the other hand. Such an effort will rely on large publicly-available fMRI databases, as well as literature mining.
9.1.2 STIC/MATH/CLIMAT AmSud project
SILIDOC
-
Title:
SILIDOC, In silico modeling of single-subject neuroimaging data for the characterization and prognosis of patients with disorders of consciousness
-
Begin date:
Fri Jan 01 2021
-
End date:
Sat Dec 31 2022
-
Local supervisor:
Demian Wassermann
-
Partners:
- Universidad de Buenos Aires
- Universidad de Valparaiso
-
Inria contact:
Demian Wassermann
-
Summary:
Studying the brain mechanisms behind consciousness is a major challenge for neuroscience and medicine. Yet so far, there is no such thing as a unique biomarker that can precisely define the state of consciousness of a disorders of consciousness (DOC) patient. All the biomarkers proposed so far are theory-based but empirically defined (EBM; empirical biomarkers): the thresholds that separate categories are set in a data-driven way. In this project, we propose a novel approach using model-based biomarkers (MBM). This new family of biomarkers (MBMs) will not only complement the EBMs but mainly will naturally address the knowledge gaps associated with the understanding of the underlying causal mechanisms behind the different states of consciousness. The modelling of the structural and functional connectivity will be combined with novel, systematic perturbational approaches that can provide new insights into the human brain’s ability to integrate and segregate information over time. In particular, with this approach we will address the hypothesis that MBMs provide functional fingerprinting of conscious states and insights into the underlying necessary and sufficient brain networks as well as their neural mechanisms. To address the development of these biomarkers, we propose a highly interdisciplinary project that combines basic and clinical neuroscience with whole-brain computational modelling, DTI SOMETHING, and clinical neuroscience. This project will benefit of proposing a complementary synergy between fourthree groups with large expertise in each area to address a common question We will develop computational whole-brain models based on single-patient neuroimaging data. We will extract MBM from the adjusted model parameters and from in-silico simulations. We will test the utility of these biomarkers for the diagnosis of patients with chronic DOC. Then, we will contrast the MBM with a set of previously developed EBM. Finally, we will analyze the diagnostic and prognostic capacity of these biomarkers in DOC patients in both chronic and acute stages.
New framework for critical brain dynamics
- Title: New framework for critical brain dynamics
-
Begin date:
Dec 2020
-
End date:
Nov 2024
-
Local supervisor:
Philippe Ciuciu
-
Partners:
- Aalto University, Finland
-
Inria contact:
Philippe Ciuciu
-
Summary:
This project entitled “New framework for critical brain dynamics” actually corresponds to a Merlin Dumeur's PhD in cotutelle between Univ. Paris-Saclay (Dr Philippe Ciuciu) and Aalto University (Prof. Matias Palva), which has been funded by an ADI scholarship in 2020. This collaboration will be also supported by hosting Dr Sheng Wang as a postdoc fellow in Ph. Ciuciu's group in May 2022 for 2 years. This line of research aims to unify scarce models of brain dynamics that rely either on the concept of bistable and critical systems like in physics or on the multifractal characterization of brain activity from EEG and MEG data.
9.2 European initiatives
9.2.1 FP7 & H2020 projects
NeuroLang
-
Title:
Accelerating Neuroscience Research by Unifying Knowledge Representation and Analysis Through a Domain Specific Language
-
Duration:
2018-2024
-
Coordinator:
Inria
-
Inria contact:
Demian Wassermann
-
Summary:
Neuroscience is at an inflection point. The 150-year old cortical specialization paradigm, in which cortical brain areas have a distinct set of functions, is experiencing an unprecedented momentum with over 1000 articles being published every year. However, this paradigm is reaching its limits. Recent studies show that current approaches to atlas brain areas, like relative location, cellular population type, or connectivity, are not enough on their own to characterize a cortical area and its function unequivocally. This hinders the reproducibility and advancement of neuroscience.
Neuroscience is thus in dire need of a universal standard to specify neuroanatomy and function: a novel formal language allowing neuroscientists to simultaneously specify tissue characteristics, relative location, known function and connectional topology for the unequivocal identification of a given brain region.
The vision of NeuroLang is that a unified formal language for neuroanatomy will boost our understanding of the brain. By defining brain regions, networks, and cognitive tasks through a set of formal criteria, researchers will be able to synthesize and integrate data within and across diverse studies. NeuroLang will accelerate the development of neuroscience by providing a way to evaluate anatomical specificity, test current theories, and develop new hypotheses.
NeuroLang will lead to a new generation of computational tools for neuroscience research. In doing so, we will be shedding a novel light onto neurological research and possibly disease treatment and palliative care. Our project complements current developments in large multimodal studies across different databases. This project will bring the power of Domain Specific Languages to neuroscience research, driving the field towards a new paradigm articulating classical neuroanatomy with current statistical and machine learning-based approaches.
SLAB (698)
-
Title:
Signal processing and Learning Applied to Brain data
-
Duration:
2016 - 2021
-
Coordinator:
Inria
-
Partners:
- INSTITUT MINES-TELECOM (France)
-
Inria contact:
Alexandre Gramfort
-
Summary:
Understanding how the brain works in healthy and pathological conditions is considered as one of the challenges for the 21st century. After the first electroencephalography (EEG) measurements in 1929, the 90’s was the birth of modern functional brain imaging with the first functional MRI (fMRI) and full head magnetoencephalography (MEG) system. By offering noninvasively unique insights into the living brain, imaging has revolutionized in the last twenty years both clinical and cognitive neuroscience. After pioneering breakthroughs in physics and engineering, the field of neuroscience has to face two major challenges. The size of the datasets keeps growing with ambitious projects such as the Human Connectome Project (HCP) which will release terabytes of data. The answers to current neuroscience questions are limited by the complexity of the observed signals: non-stationarity, high noise levels, heterogeneity of sensors, lack of accurate models for the signals. SLAB will provide the next generation of models and algorithms for mining electrophysiology signals which offer unique ways to image the brain at a millisecond time scale. SLAB will develop dedicated machine learning and statistical signal processing methods and favor the emergence of new challenges for these fields focussing on five open problems: 1) source localization with M/EEG for brain imaging at high temporal resolution 2) representation learning from multivariate (M/EEG) signals to boost statistical power and reduce acquisition costs 3) fusion of heterogeneous sensors to improve spatiotemporal resolution 4) modeling of non-stationary spectral interactions to identify functional coupling between neural ensembles 5) development of algorithms tractable on large datasets and easy to use by non-experts. SLAB aims to strengthen mathematical and computational foundations of neuroimaging data analysis. The methods developed will have applications across fields (e.g. computational biology, astronomy, econometrics). Yet, the primary users of the technologies developed will be in the cognitive and clinical neuroscience community. The tools and high quality open software produced in SLAB will facilitate the analysis of electrophysiology data, offering new perspectives to understand how the brain works at a mesoscale, and for clinical applications (epilepsy, autism, essential tremor, sleep disorders).
VirtualBrainCloud
-
Title:
VirtualBrainCLoud
-
Duration:
Jan 2019 - Jan 2023
-
Coordinator:
Charité, Berlin
-
Partners:
- Fraunhofer
- University of Oxford
- Forschungzentrum Juelich
- University of Genova
- CodeBox
- CODEMART
- TP21
- Alzheimer Europe AISBL
- University of Vienna
- Institut du Cerveau et de la Moelle Epiniere
- Université d'Aix Marseille (AMU)
- INRIA
- Fundacio Institut De Bioenginyera De Catalunya (IBEC)
- Helsinki University
- University Madrid
- EODYNE
-
Inria contact:
Bertrand Thirion
-
Summary:
The overarching goal of The Virtual Brain Cloud (TVB-Cloud) is personalized prevention and treatment of dementia. To achieve generalizable results that help individual patients, The Virtual Brain Cloud integrates the data of large cohorts of patients and healthy controls through multi-scale brain simulation using The Virtual Brain (or TVB) simulator. There is a need for infrastructures for sharing and processing health data at a large scale that comply with the EU general data protection regulations (or GDPR). The VirtualBrainCloud consortium closes this gap, making health data actionable. Elaborated data protection concepts minimize the risks for data subjects and allow scientists to use sensitive data for research and clinical translation.
ICEI (861)
-
Title:
Interactive Computing E-Infrastructure for the Human Brain Project
-
Duration:
2020 - 2023
-
Coordinator:
FZ Juelich.
-
Partners:
- AALTO KORKEAKOULUSAATIO SR (Finland)
- ATHENS UNIVERSITY OF ECONOMICS AND BUSINESS - RESEARCH CENTER (Greece)
- BARCELONA SUPERCOMPUTING CENTER - CENTRO NACIONAL DE SUPERCOMPUTACION (Spain)
- BAUHAUS-UNIVERSITAET WEIMAR (Germany)
- BERGISCHE UNIVERSITAET WUPPERTAL (Germany)
- Bloomfield Science Museum Jerusalem (BSMJ) (Israel)
- CARDIFF UNIVERSITY (UK)
- CENTRE HOSPITALIER UNIVERSITAIRE VAUDOIS (Switzerland)
- CENTRE NATIONAL DE LA RECHERCHE SCIENTIFIQUE CNRS (France)
- CINECA CONSORZIO INTERUNIVERSITARIO (Italy)
- COMMISSARIAT A L ENERGIE ATOMIQUE ET AUX ENERGIES ALTERNATIVES (France)
- CONSIGLIO NAZIONALE DELLE RICERCHE (Italy)
- CONSORCI INSTITUT D'INVESTIGACIONS BIOMEDIQUES AUGUST PI I SUNYER (Spain)
- CYBERBOTICS SARL (Switzerland)
- DANMARKS TEKNISKE UNIVERSITET (Denmark)
- DE MONTFORT UNIVERSITY (UK))
- DEBRECENI EGYETEM (Hungary)
- DEUTSCHES ZENTRUM FUR NEURODEGENERATIVE ERKRANKUNGEN EV (Germany)
- ECOLE NORMALE SUPERIEURE (France)
- ECOLE POLYTECHNIQUE FEDERALE DE LAUSANNE (Switzerland)
- EIDGENOESSISCHE TECHNISCHE HOCHSCHULE ZUERICH (Switzerland)
- ETHNIKO KAI KAPODISTRIAKO PANEPISTIMIO ATHINON (Greece)
- EUROPEAN MOLECULAR BIOLOGY LABORATORY (Germany)
- FONDAZIONE EUROPEAN BRAIN RESEARCHINSTITUTE RITA LEVI (Italy)
- FONDEN TEKNOLOGIRADET (Denmark)
- FORSCHUNGSZENTRUM JULICH GMBH (Germany)
- FORTISS GMBH (Germany)
- FRAUNHOFER GESELLSCHAFT ZUR FOERDERUNG DER ANGEWANDTEN FORSCHUNG E.V. (Germany)
- FUNDACAO D. ANNA SOMMER CHAMPALIMAUD E DR. CARLOS MONTEZ CHAMPALIMAUD (Portugal)
- FUNDACIO INSTITUT DE BIOENGINYERIA DE CATALUNYA (Spain)
- HEINRICH-HEINE-UNIVERSITAET DUESSELDORF (Germany)
- HITS GGMBH (Germany)
- HOSPITAL CLINICO Y PROVINCIAL DE BARCELONA (Spain)
- HUMBOLDT-UNIVERSITAET ZU BERLIN (Germany)
- IDRYMA IATROVIOLOGIKON EREUNON AKADEMIAS ATHINON (Greece)
- IMPERIAL COLLEGE OF SCIENCE TECHNOLOGY AND MEDICINE (UK)
- INSTITUT DU CERVEAU ET DE LA MOELLE EPINIERE (France)
- INSTITUT JOZEF STEFAN (Slovenia)
- INSTITUT PASTEUR (France)
- INSTITUTE OF EXPERIMENTAL MEDICINE - HUNGARIAN ACADEMY OF SCIENCES (Hungary)
- ISTITUTO NAZIONALE DI FISICA NUCLEARE (Italy)
- Institute of Science and Technology Austria (Austria)
- JOHANN WOLFGANG GOETHE-UNIVERSITATFRANKFURT AM MAIN (Germany)
- KARLSRUHER INSTITUT FUER TECHNOLOGIE (Germany)
- KAROLINSKA INSTITUTET (Sweden)
- KATHOLIEKE UNIVERSITEIT LEUVEN (Belgium)
- KONINKLIJKE NEDERLANDSE AKADEMIE VAN WETENSCHAPPEN - KNAW (Netherlands)
- KUNGLIGA TEKNISKA HOEGSKOLAN (Sweden)
- LABORATORIO EUROPEO DI SPETTROSCOPIE NON LINEARI (Italy)
- LINNEUNIVERSITETET (Sweden)
- MEDIZINISCHE UNIVERSITAT INNSBRUCK (Austria)
- MIDDLESEX UNIVERSITY HIGHER EDUCATION CORPORATION (UK)
- NORGES MILJO-OG BIOVITENSKAPLIGE UNIVERSITET (Norway)
- OSTERREICHISCHE STUDIENGESELLSCHAFTFUR KYBERNETIK VEREIN (Austria)
- POLITECNICO DI TORINO (Italy)
- POLYTECHNEIO KRITIS (Greece)
- RHEINISCH-WESTFAELISCHE TECHNISCHE HOCHSCHULE AACHEN (Germany)
- RUPRECHT-KARLS-UNIVERSITAET HEIDELBERG (Germany)
- SABANCI UNIVERSITESI (Turkey)
- SCUOLA NORMALE SUPERIORE (Italy)
- SCUOLA SUPERIORE DI STUDI UNIVERSITARI E DI PERFEZIONAMENTO SANT'ANNA (Italy)
- SIB INSTITUT SUISSE DE BIOINFORMATIQUE (Switzerland)
- STICHTING NEDERLANDSE WETENSCHAPPELIJK ONDERZOEK INSTITUTEN (Netherlands)
- STIFTUNG FZI FORSCHUNGSZENTRUM INFORMATIK AM KARLSRUHER INSTITUT FUR TECHNOLOGIE (Germany)
- TECHNISCHE UNIVERSITAET DRESDEN (Germany)
- TECHNISCHE UNIVERSITAET GRAZ (Austria)
- TECHNISCHE UNIVERSITAET MUENCHEN (Germany)
- TEL AVIV UNIVERSITY (Israel)
- THE CHANCELLOR, MASTERS AND SCHOLARS OF THE UNIVERSITY OF OXFORD (UK)
- THE HEBREW UNIVERSITY OF JERUSALEM (Israel)
- THE UNIVERSITY COURT OF THE UNIVERSITY OF ABERDEEN (UK)
- THE UNIVERSITY OF EDINBURGH (UK)
- THE UNIVERSITY OF HERTFORDSHIRE HIGHER EDUCATION CORPORATION (UK)
- THE UNIVERSITY OF MANCHESTER (UK)
- THE UNIVERSITY OF SUSSEX (UK)
- TTY-SAATIO (Finland)
- UNIVERSIDAD AUTONOMA DE MADRID (Spain)
- UNIVERSIDAD DE CASTILLA - LA MANCHA (Spain)
- UNIVERSIDAD DE GRANADA (Spain)
- UNIVERSIDAD POLITECNICA DE MADRID (Spain)
- UNIVERSIDAD POMPEU FABRA (Spain)
- UNIVERSIDAD REY JUAN CARLOS (Spain)
- UNIVERSIDADE DO MINHO (Portugal)
- UNIVERSITA DEGLI STUDI DI MILANO (Italy)
- UNIVERSITA DEGLI STUDI DI PAVIA (Italy)
- UNIVERSITAET BIELEFELD (Germany)
- UNIVERSITAET HAMBURG (Germany)
- UNIVERSITAETSKLINIKUM HAMBURG-EPPENDORF (Germany)
- UNIVERSITAT DE BARCELONA (Spain)
- UNIVERSITAT ZURICH (Switzerland)
- UNIVERSITATSSPITAL BASEL (Switzerland)
- UNIVERSITE D'AIX MARSEILLE (France)
- UNIVERSITE DE BORDEAUX (France)
- UNIVERSITE DE GENEVE (Switzerland)
- UNIVERSITE DE LIEGE (Belgium)
- UNIVERSITEIT ANTWERPEN (Belgium)
- UNIVERSITEIT GENT (Belgium)
- UNIVERSITETET I OSLO (Norway)
- UNIVERSITY OF LEEDS (UK)
- UNIVERSITY OF SHEFFIELD ROYAL CHARTER (UK)
- UNIVERSITY OF SURREY (UK)
- UNIVERSITY OF THE WEST OF ENGLAND, BRISTOL (UK)
- UPPSALA UNIVERSITET (Sweden)
- WEIZMANN INSTITUTE OF SCIENCE (Israel)
-
Inria contact:
Bertrand Thirion
-
Summary:
The Human Brain Project (HBP) is one of the three FET (Future and Emerging Technology) Flagship projects. Started in 2013, it is one of the largest research projects in the world . More than 500 scientists and engineers at over than 140 universities, teaching hospitals, and research centres across Europe come together to address one of the most challenging research targets – the human brain.
To tame brain complexity, the project is building a research infrastructure to help advance neuroscience, medicine, computing and brain-inspired technologies - EBRAINS. The HBP is developing EBRAINS to create lasting research platforms that benefit the wider community.
The HBP provides a framework where teams of researchers and technologists work together to scale up ambitious ideas from the lab, explore the different aspects of brain organisation, and understand the mechanisms behind cognition, learning, or plasticity.
Scientists in the HBP conduct targeted experimental studies and develop theories and models to shed light on the human connectome, addressing mechanisms that underlie information processing, from the molecule to cellular signaling and large-scale networks.
The project teams transfer the acquired knowledge to make an impact in health and innovation: Insights from basic research are translated into medical applications, to prepare the ground for new diagnoses and therapies. Discoveries about learning and brain plasticity mechanisms are used to inspire technologic progress, e.g., in artificial intelligence. In addition, the project studies the ethical and societal implications of the advancement of neuroscience and related fields.
In its final phase (April 2020 – March 2023) the HBP’s focus is to advance three core scientific areas – brain networks, their role in consciousness, and artificial neural nets – while further expanding EBRAINS.
Currently transitioning into a sustainable infrastructure, EBRAINS will remain available to the scientific community, as a lasting contribution of the HBP to global scientific progress.
9.3 National initiatives
9.3.1 ANR
Neuroref: Mathematical Models of Anatomy / Neuroanatomy / Diffusion MRI
Participants: Demian Wassermann [Correspondant], Antonia Machlouzarides Shalit, Valentin Iovene.
While mild traumatic brain injury (mTBI) has become the focus of many neuroimaging studies, the understanding of mTBI, particularly in patients who evince no radiological evidence of injury and yet experience clinical and cognitive symptoms, has remained a complex challenge. Sophisticated imaging tools are needed to delineate the kind of subtle brain injury that is extant in these patients, as existing tools are often ill-suited for the diagnosis of mTBI. For example, conventional magnetic resonance imaging (MRI) studies have focused on seeking a spatially consistent pattern of abnormal signal using statistical analyses that compare average differences between groups, i.e., separating mTBI from healthy controls. While these methods are successful in many diseases, they are not as useful in mTBI, where brain injuries are spatially heterogeneous.
The goal of this proposal is to develop a robust framework to perform subject-specific neuroimaging analyses of Diffusion MRI (dMRI), as this modality has shown excellent sensitivity to brain injuries and can locate subtle brain abnormalities that are not detected using routine clinical neuroradiological readings. New algorithms will be developed to create Individualized Brain Abnormality (IBA) maps that will have a number of clinical and research applications. In this proposal, this technology will be used to analyze a previously acquired dataset from the INTRuST Clinical Consortium, a multi-center effort to study subjects with Post- Traumatic Stress Disorder (PTSD) and mTBI. Neuroimaging abnormality measures will be linked to clinical and neuropsychological assessments. This technique will allow us to tease apart neuroimaging differences between PTSD and mTBI and to establish baseline relationships between neuroimaging markers, and clinical and cognitive measures.
DirtyData: Data integration and cleaning for statistical analysis
Participants: Gaël Varoquaux [Correspondant], Pierre Glaser.
Machine learning has inspired new markets and applications by extracting new insights from complex and noisy data. However, to perform such analyses, the most costly step is often to prepare the data. It entails correcting errors and inconsistencies as well as transforming the data into a single matrix-shaped table that comprises all interesting descriptors for all observations to study. Indeed, the data often results from merging multiple sources of informations with different conventions. Different data tables may come without names on the columns, with missing data, or with input errors such as typos. As a result, the data cannot be automatically shaped into a matrix for statistical analysis.
This proposal aims to drastically reduce the cost of data preparation by integrating it directly into the statistical analysis. Our key insight is that machine learning itself deals well with noise and errors. Hence, we aim to develop the methodology to do statistical analysis directly on the original dirty data. For this, the operations currently done to clean data before the analysis must be adapted to a statistical framework that captures errors and inconsistencies. Our research agenda is inspired from the data-integration state of the art in database research combined with statistical modeling and regularization from machine learning.
Data integrating and cleaning is traditionally performed in databases by finding fuzzy matches or overlaps and applying transformation rules and joins. To incorporate it in the statistical analysis, an thus propagate uncertainties, we want to revisit those logical and set operations with statistical-learning tools. A challenge is to turn the entities present in the data into representations well-suited for statistical learning that are robust to potential errors but do not wash out uncertainty.
Prior art developed in databases is mostly based on first-order logic and sets. Our project strives to capture errors in the input of the entries. Hence we formulate operations in terms of similarities. We address typing entries, deduplication -finding different forms of the same entity- building joins across dirty tables, and correcting errors and missing data.
Our goal is that these steps should be generic enough to digest directly dirty data without user-defined rules. Indeed, they never try to build a fully clean view of the data, which is something very hard, but rather include in the statistical analysis errors and ambiguities in the data.
The methods developed will be empirically evaluated on a variety of dataset, including the French public-data repository, datagouv. The consortium comprises a company specialized in data integration, Data Publica, that guides business strategies by cross-analyzing public data with market-specific data.
FastBig Project
Participants: Bertrand Thirion [Correspondant], Jerome-Alexis Chevalier, Tuan Binh Nguyen.
In many scientific applications, increasingly-large datasets are being acquired to describe more accurately biological or physical phenomena. While the dimensionality of the resulting measures has increased, the number of samples available is often limited, due to physical or financial limits. This results in impressive amounts of complex data observed in small batches of samples.
A question that arises is then : what features in the data are really informative about some outcome of interest ? This amounts to inferring the relationships between these variables and the outcome, conditionally to all other variables. Providing statistical guarantees on these associations is needed in many fields of data science, where competing models require rigorous statistical assessment. Yet reaching such guarantees is very hard.
FAST-BIG aims at developing theoretical results and practical estimation procedures that render statistical inference feasible in such hard cases. We will develop the corresponding software and assess novel inference schemes on two applications : genomics and brain imaging.
MultiFracs project
Participants: Philippe Ciuciu [Correspondant], Merlin Dumeur.
The scale-free concept formalizes the intuition that, in many systems, the analysis of temporal dynamics cannot be grounded on specific and characteristic time scales. The scale-free paradigm has permitted the relevant analysis of numerous applications, very different in nature, ranging from natural phenomena (hydrodynamic turbulence, geophysics, body rhythms, brain activity,...) to human activities (Internet traffic, population, finance, art,...).
Yet, most successes of scale-free analysis were obtained in contexts where data are univariate, homogeneous along time (a single stationary time series), and well-characterized by simple-shape local singularities. For such situations, scale-free dynamics translate into global or local power laws, which significantly eases practical analyses. Numerous recent real-world applications (macroscopic spontaneous brain dynamics, the central application in this project, being one paradigm example), however, naturally entail large multivariate data (many signals), whose properties vary along time (non-stationarity) and across components (non-homogeneity), with potentially complex temporal dynamics, thus intricate local singular behaviors.
These three issues call into question the intuitive and founding identification of scale-free to power laws, and thus make uneasy multivariate scale-free and multifractal analyses, precluding the use of univariate methodologies. This explains why the concept of scale-free dynamics is barely used and with limited successes in such settings and highlights the overriding need for a systematic methodological study of multivariate scale-free and multifractal dynamics. The Core Theme of MULTIFRACS consists in laying the theoretical foundations of a practical robust statistical signal processing framework for multivariate non homogeneous scale-free and multifractal analyses, suited to varied types of rich singularities, as well as in performing accurate analyses of scale-free dynamics in spontaneous and task-related macroscopic brain activity, to assess their natures, functional roles and relevance, and their relations to behavioral performance in a timing estimation task using multimodal functional imaging techniques.
This overarching objective is organized into 4 Challenges:
- Multivariate scale-free and multifractal analysis,
- Second generation of local singularity indices,
- Scale-free dynamics, non-stationarity and non-homogeneity,
- Multivariate scale-free temporal dynamics analysis in macroscopic brain activity.
DARLING: Distributed adaptation and learning over graph signals
Participants: Philippe Ciuciu [Correspondant].
The project has finally started in 2021. A postdoc has been identified, Tiziana Cattai, and she will be hired in Spring 2022.
The DARLING project will aim to propose new adaptive learning methods, distributed and collaborative on large dynamic graphs in order to extract structured information of the data flows generated and/or transiting at the nodes of these graphs. In order to obtain performance guarantees, these methods will be systematically accompanied by an in-depth study of random matrix theory. This powerful tool , never exploited so far in this context although perfectly suited for inference on random graphs, will thereby provide even avenues for improvement. Finally, in addition to their evaluation on public data sets, the methods will be compared with each other using two advanced imaging techniques in which two of the partners are involved: radio astronomy with the giant SKA instrument (Obs. Côte d'Azur) and magnetoencephalographic brain imaging (Inria Parietal at NeuroSpin, CEA Saclay). These involve the processing of time series on graphs while operating at extreme observation scales.
VLFMRI: Very low field MRI for babies
Participants: Philippe Ciuciu [Correspondant], Kumari Pooja.
The project will be starting in 2021 with a post-doc or PhD student to be hired probably in fall 2021 or 2022.
VLFMRI aims at developing a very low-field Magnetic Resonance Imaging (MRI) system as an alternative to conventional high-field MRI for continuous imaging of premature newborns to detect hemorrhages or ischemia. This system is based on a combination of a new generation of magnetic sensors based on spin electronics, optimized MR acquisition sequences (based on the SPARKLING patent, Inria-CEA Parietal team at NeuroSpin) and a open and compatible system with an incubator that will allow to achieve an image resolution of 1mm on a whole baby body in a short scan time. This project is a partnership of three academic partners and two hospital departments. The different stages of the project are the finalization of the hardware development and software system, preclinical validation on small animals and clinical validation.
meegBIDS.fr: Standardization, sharing and analysis of MEEG data simplified by BIDS
Participants: Alexandre Gramfort [Correspondant], Richard Hoechenberger.
The project accepted by ANR in 2019 started in 2020 with an engineer hired in 2020. This project is in collaboration with the MEG groups at CEA NeuroSpin and the Brain and Spine Institute (ICM) in Paris.
The neuroimaging community recently started an international effort to standardize the sharing of data recorded with magnetoencephalography (MEG) and with electroencephalography (EEG). This format, known as the Brain Imaging Data Structure (BIDS), now needs a wider adoption, notably in the French neuroimaging community, along with the development of dedicated software tools that operate seamlessly on BIDS formatted datasets. The meegBIDS.fr project has three aims: 1) accelerate the research cycles by allowing analysis software tools to work with BIDS formated data, 2) simplify data sharing with high quality standards thanks to automated validation tools, 3) train French neuroscientists to leverage existing public BIDS MEG/EEG datasets and to share their own data with little efforts.
AI-Cog: AI for Aging Societies: From Basic Concepts to Practical Tools for AI-Facilitated Cognitive Training
Participants: Alexandre Gramfort [Correspondant], Denis Engemann, Thomas Moreau, Apolline Mellot.
The project accepted by ANR in 2020 started in 2021 with a PhD student. An engineer should be hired in 2022 to lead the software engineering developments. This project is in collaboration with the University of Freiburg in Germany and the RIKEN AIP in Japan.
Worldwide, people are living longer than ever before in history. Today, most people can expect to live into their sixties and beyond. Ageing societies, however, bring social, economical, and healthcare challenges. Japan (#1), France (#3) and Germany (#4) belong to the top five countries worldwide with the highest economic old-age dependency ratio of people aged over 65 years and more. Particularly detrimental health conditions in older age include depression, and dementia. Today, around 50 M people globally suffer from dementia and there are nearly 10 M new cases every year. According to WHO there is a new case of dementia every 3 seconds globally. Mastering the challenges associated with aging societies in general, and those associated with age-related brain disorders in particular, is therefore of outstanding global importance, and especially for the three countries involved in the present trilateral call, Japan, France, and Germany. Therefore, the aim of the present project is to leverage the potential of artificial intelligence (AI) approaches to foster healthy aging. To this aim we will study objective machine-learning-driven biomarkers to evaluate cognitive interventions as well as support personalized therapies. We will develop novel, dedicated machine learning (ML) methods and adapt them to the special signal types that can be recorded from the human brain. We will make our methods publicly available in an open-source reference software package, focussing on unsupervised learning, data augmentation, domain adaptation, and interpretable machine learning models. Our main scientific aims is to optimize the decodable information about the current functional state of the brain, to identify biomarkers of the risk for cognitive impairments and different forms of dementia, and use these improved methods to guide AI-facilitated cognitive training. These joint efforts between Japan, France and Germany will be accompanied by a focus on ethical and societal aspects of AI in the context of aging, paired with participatory, transnational outreach activities, to foster the dialog between our scientific community and the general public.
BrAIN: Bridging Artificial Intelligence and Neuroscience
Participants: Alexandre Gramfort [Correspondant], Denis Engemann, Thomas Moreau, Richard Hoechenberger, Omar Chehab, David Sabbagh.
The project accepted in 2020 by ANR in the "Chaire IA" call started in 2021 with the recruitment of an engineer, 1 PhD and one post-doc.
The general objectives of BrAIN is to develop ML algorithms that can learn with weak or no supervision on neural time series. It will require contributions to self-supervised learning, domain adaptation and data augmentation techniques, exploiting the known underlying physical mechanisms that govern the data generating process of neurophysiological signals.
Knowledge and representations integration on the brain
Participants: Bertrand Thirion [Correspondant], Demian Wassermann, Badr Tajini, Raphaël Meudec.
The project accepted in 2020 by ANR in the "Chaire IA" call will be starting in 2021 with an engineer, 1 PhD and a starting position to be hired in 2021.
Cognitive science describes mental operations, and functional brain imaging provides a unique window into the brain systems that support these operations. A growing body of neuroimaging research has provided significant insight into the relations between psychological functions and brain activity. However, the aggregation of cognitive neuroscience results to obtain a systematic mapping between structure and function faces the roadblock that cognitive concepts are ill-defined and may not map cleanly onto the computational architecture of the brain.
To tackle this challenge, we propose to leverage rapidly increasing data sources: text and brain locations described in neuroscientific publications, brain images and their annotations taken from public data repositories, and several reference datasets. Our aim here is to develop multi-modal machine learning techniques to bridge these data sources.
LearnI: learning data integration, from discrete entities to signals
Participants: Gaël Varoquaux [Correspondant].
The project accepted in 2020 by ANR in the "Chaire IA" call will be starting in 2021 with an engineer, 2 PhDs and a post-doc to be hired in 2021.
The goal of LearnI is to develop machine-learning across multiple sources of relational data, with numerical and symbolic entries. LearnI will address the core challenge of joining and aggregating across tables where the information is represented with different symbols. For this, LearnI will develop methods to embed the discrete elements in vector spaces and perform data assembly across tables with these vectorial representations.
10 Dissemination
Participants: Bertrand Thirion, Gael Varoquaux, Thomas Moreau, Alexandre Gramfort, Demian Wassermann, Olivier Grisel, Philippe Ciuciu.
10.1 Promoting scientific activities
10.1.1 Scientific events: organisation
Member of the organizing committees
Gael Varoquaux was member of the organizing committee of the autoDS workshop at ECML.
Bertrand Thirion organized a workshop on modern statistical methods at the OHBM 2021 conference.
10.1.2 Scientific events: selection
Member of the conference program committees
- Bertrand Thirion is Area chair for NeurIPS 2021.
- Alexandre Gramfort is Area Chair for ICML, NeurIPS and ICLR 2021.
- Philippe Ciuciu was Area Chair for EUSIPCO 2021 and member of the ESMRMB 2021 conference program.
- Demian Wassermann was Area Chair for CVPR and IPMI and a member of the ISMRM 2021 conference program.
- Gael Varoquaux is Area chair for NeurIPS 2021, Senior Program Committee for IJCAI 2021.
10.1.3 Journal
Member of the editorial boards
- Bertrand Thirion is member of the Editorial Board of MedIA and Aperture.
- Alexandre Gramfort is member of the Editorial Board of Journal of Machine Learning Research (JMLR), NeuroImage and Aperture.
- Philippe Ciuciu is Senior Area Editor of the IEEE Open Journal of Signal Processing and associate editor of Frontiers in Neuroscience, section Brain Imaging Methods.
- Gaël Varoquaux is review editor for elife.
Reviewer - reviewing activities
- Bertrand Thirion has reviewed for Nature Communications, GigaScience, Scientific Data, Nature human behavior and for the ERC.
- Alexandre Gramfort has reviewed for the European Research Council (ERC), IEEE Trans. PAMI, IEEE Journal of Biomedical and Health Informatics, Scientific Data, NeuroImage, Neuroinformatics, JMLR, Journal of Mathematical Imaging and Vision (JMIV).
- Demian Wassermann has reviewed for the European Research Council (ERC), IEEE Trans PAMI, NeuroImage, Nature Communications Biology, Brain Structure and Function, NeuroImage.
- Philippe Ciuciu has reviewed for IEEE Trans. Medical Imaging/Comput. Imaging/Biomed. Eng., NeuroImage, Medical Image Analysis, Magnetic Resonance in Medicine. He has been reviewer for ISBI 2021 as well.
- Thomas Moreau has reviewed for SIAM Journal on Imaging Sciences, IMCL, Signal Processing Letters, NeurIPS and ICLR.
- Gael Varoquaux has reviewed for DAMI, Machine Learning Journal, JMLR, AAAI, ICML, ICLR, AIstats, and for funding agencies ANR and dataia.
10.1.4 Invited talks
Bertrand Thirion has given the following talks:
- Neurospin, Analysing individual brains: Individual Brain Charting project, Jan 11th
- Neuropsy Large-scale brain activity decoding: when machine learning supports cognitive neuroscience, Feb 26th
- BrainSpace Initiative, In the wild brain activity decoding March26th
- FAIR, brain activity decoding: toward a cognitive brain atlas April 15th
- HBP WP1 presentation From brain activity decoding to functional atlasing: scaling up cognitive neuroscience., March 20th
- Centrale-Supelec, séminaire IA-Santé Inference and group analysis, application to brain imaging, April 13th
- LMO, Statistical inference in high-dimension & application to brain imaging, October 14th
- Lapsyde Medical imaging for population analysis in the age of machine learning, December 10th
- OHBM 2021 Decoding with confidence: Statistical Control on Decoder Maps, June 10th.
Alexandre Gramfort has given the following talks:
- PrAIrie Inst., Bridging the gap between neuroscience and machine learning, Nov 10th
- CuttingEEG workshop, Boosting EEG data analysis with deep learning, 6 Oct.
- Journée Maths/IA Insa Rouen, Learning to learn on EEG signals: From bilevel optimization to automatic data-augmentation, Sep
- GDR ISIS, Reproducible ML: software challenges, anecdotes and some engineering solutions, Sep
- NeoBrain Workshop, Machine Learning on EEG: From sleep to brain age, Mar 8th
- BCI Workshop Korea, From supervised to self-supervised learning on EEG, Feb 22nd
Philippe Ciuciu has given the following talks:
- Aix-Marseille Universi
'e (virtual), Accelerated MR imaging: from shorter data acquisition to faster image reconstruction, Jan 21th 2021 - French Ultra-high field Network (La Timone hospital, Aix-Marseille Univ., (in person) Accelerated MR imaging: from shorter data acquisition to faster image reconstruction, Oct. 2021
- Neuroscience Center (HiLIFE, University of Helsinki, Finalnd), in person, Functional Connectivity in the Infra-slow Human Brain Activity in MEG, Nov 2021
- ABC Seminar: Human brain imaging (Aalto University, Finland), in person, Accelerated non-Cartesian MR imaging: From shorter data acquisition to faster image reconstruction, Nov 2021
- CEA Key note of the Transverse Working Program on Numerical Simulation and AI (in-person at CEA Grenoble), Compressed Sensing for Computational Imaging, Nov 22nd 2021
Thomas Moreau has given the following talks:
- MOD seminar, Tubingen University, Learning to optimize with unrolled algorithms, Apr. 1st
- ML-MTP seminar, Université de Montpellier, Learning to optimize with unrolled algorithms, Apr. 15th
- Colloque Imagerie Médicale à l'heure de l'IA, ICM, Task-Force Covid-19, L’expérience de l’AP-HP, Jun. 9th
- NeurIPS@PAris, HNPE: Leveraging Global Parameters for Neural Posterior Estimation, Dec 10th
Gael Varoquaux has given the following talks:
- AI as statistical methods for imperfect theories, NeurIPS workshop for AI for Science, Dec.
- Machine learning and health, Apr.
- Scikit-learn: la force d'une communauté, Séminaire National DGDI
- Supervised Learning with Missing Values, journée statistique de l'IHESS, Feb
- Supervised Learning with Missing Values, séminaire de statistique de P6, March
- AI, electronic records, health, journées santée et IA, Hi Paris, Apr
- Electronic Health Records, from Dirty Data to Gold Mine, rencontres Franco-Indiennes, Nov
- Scikit-learn et santé, ComEX Air Liquide, Nov
10.1.5 Scientific expertise
Bertrand Thirion has been part of a panel reviewing CEA-DRT activities in AI in nov. 2021.
Gael Varoquaux has been part of the Global Partnership on AI
10.1.6 Research administration
- Bertrand Thirion has been head of Dataia research institute till March 31st, 2021
- Bertrand Thirion is Délégué Scientifique of the Inria Saclay Center since March 1st, 2021
- Philippe Ciuciu is member of the steering committee of the working program on numerical simulation and AI at CEA
- Philippe Ciuciu was the CEA/DRF expert nominated by the High Commissioner of CEA for the 2021 PhD FOCUS program on AI and Numerical Twins.
- Alexandre Gramfort is member of the operational commitee of Hi!Paris (AI center from IP Paris).
- Alexandre Gramfort manages the data challenges supported by DataIA (supervision of one engineer).
- Alexandre Gramfort is member of the scientific committee of the Institut Henri Poincaré (IHP)
- Alexandre Gramfort is member of the Comission de Développement Technologique (CDT) du centre de Saclay.
- Demian Wassermann is the local correspondent for the ethics committee of Inria Saclay Île-de-France
- Gael Varoquaux is member of the Comission de Suivi Doctorale at Inria Saclay Ile-de-France
- Gael Varoquaux is director of the scikit-learn consortium at Inria
10.2 Teaching - Supervision - Juries
10.2.1 Teaching
- Master: Alexandre Gramfort, Optimization for Data Science, 20h, MSc 2 Data Science Master, Institut Polytechnique de Paris, France
- Master: Alexandre Gramfort, DataCamp, 30h, Msc 2 Data Science Master, Institut Polytechnique de Paris, France
- Master: Alexandre Gramfort, Source Imaging with EEG and MEG, 10h, Msc 2 in Biomedical Imaging at Univ. Paris
- Master: Alexandre Gramfort, Source Imaging with EEG and MEG, 7h, Msc 2 in Biomedical Imaging at CentraleSupélec
- Master: Bertrand Thirion, Functional neuroimaging and BCI, 12h, Master MVA, ENS Paris-Saclay, France
- Master: Bertrand Thirion, Neuroengineering master, 2h, Université Paris-Saclay.
- Master: Philippe Ciuciu, Medical Imaging course – A tour in Magnetic Resonance Imaging, 12h (9h course, 3h hands-on session), MSc 2 ATSI, CentraleSupélec, Univ. Paris-Saclay
- IOGS (SupOptique) 3rd year, 3h30: A tour in Magnetic Resonance Imaging
- Bachelor: Demian Wassermann, CSE201 class, 15h, C++ programming, Ecole Polytechnique
- Master: Demian Wassermann, 7h Biomedical Engineering, Msc 2 Biomedical Engineering, Université de Paris
- Extension: Demian Wassermann, Data Science, 20h, Ecole Polytechnique
- Master: Gaël Varoquaux, machine learning on dirty data, AI Summer School DFKI-Inria 3H
- Master: Gaël Varoquaux, representation learning in limited-data settings, Deep Learning Summer School, Gran Canaria, 4h30
- Master: Gaël Varoquaux, Machine learning for digital humanities, EHESS 8h
- Doctoral school: Gaël Varoquaux, machine learning for neuroimaging, 6h, Unique days, Montréal
- Master: Thomas Moreau, DataCamp, 30h, Ms Data Science, Ecole Polytechnique, France
- Executive Master: Thomas Moreau, Python, 9h, Ms Statistique et big Data, Université Paris-Dauphine.
- Formation continue: Thomas Moreau, Data Science, 24h, Executive Education, Ecole Polytechnique
- IDESSAI summer school: Thomas Moreau, Introduction to neuroimaging with Python (3h)
- Master: Olivier Grisel, Deep Learning (40h), Ms Data Science, Ecole Polytechnique, France
- AI4Health winter school: Alexandre Gramfort, Deep Learning on EEG (6h)
- Tutorial at CuttingEEG workshop: Alexandre Gramfort, Processing EEG data with MNE (3h)
10.2.2 Supervision
- Bertrand Thirion is PhD advisor for Thomas Bazeille, Hugo Richard, Binh Nguyen, Joseph Ben Zakoun, Thmas Chalapaon, Alexandre Bralin, Alexis Thual, Alexandre Pasquiou,
- Philippe Ciuciu is PhD advisor for Hamza Cherkaoui, Zaccharie Ramzi, Guillaume Daval-Frérot, Chaithya G R, Arthur Waguet, Merlin Dumeur, Pierre-Antoine Comby and PhD co-adivsor for Zaineb Amor and Anaïs Artiges.
- Demian Wassermann is PhD advisor for Maëliss Jallais, Valentin Iovene, Gaston Zanitti, Chengran Fang, Raphaël Meudec.
- Alexandre Gramfort is PhD advisor for Hubert Banville, David Sabbagh, Charlotte Caucheteux, Omar Chehab, Cedric Allain, Julia Linhart, Quentin Bertrand and Apolline Mellot, Hicham Janati.
- Thomas Moreau is PhD advisor for Hamza Cherkaoui, Cedric Allain, Benoit Malézieux and Mathieu Dagreou.
- Gaël Varoquaux is PhD advisor for Léo Grinsztajn, Samuel Brasil, Alexandre Perez, Matthieu Doutreligne, Bénédicte Colnet, Lihu Chen, and Alexis Cvetkov-Iliev
10.2.3 Juries
- Bertrand Thirion has been part of the PhD committee of Myriam Bontonou, Dec 3rd
- Bertrand Thirion has been part of the PhD committee of Valentin Iovene, Nov 23rd
- Philippe Ciuciu has been part of the PhD committee of Martin Jacob (CEA, Grenoble), March 11th
- Philippe Ciuciu acted as reviewer for the PhD of Serafeim Loukas (EPFL, Switzerland), Apr. 28th
- Philippe Ciuciu acted as THE opponent for the PhD defense of Sheng H. Wang (University of Helsinki), Nov. 19th
- Alexandre Gramfort acted as reviewer for the PhD of Nicolas Coquelet (Univ. Libre de Bruxelles), Sep 2nd.
- Alexandre Gramfort acted as reviewer for the PhD of Giorgia Cantisani (Telecom Paris, IP Paris), Dec 13th.
- Alexandre Gramfort acted as reviewer for the PhD of Khanh Hung TRAN (CEA, Univ. Paris Saclay), Feb 16th.
- Alexandre Gramfort acted as reviewer for the PhD of Jules Brochard (Sorbonne Univ.), Jan 15th.
- Alexandre Gramfort has been part of the PhD committee of Hugo Richard (Inria), Dec 20th
- Alexandre Gramfort has been part of the PhD committee of Malik Tiomoko (CentraleSupélec), Oct. 7th
- Alexandre Gramfort has been part of the PhD committee of Khaled Zaouk (Inria), Mar. 11th
- Hamza Cherkaoui defended his PhD thesis on March 3rd
- Thomas Bazeille defended his PhD thesis on Oct 20th
- Valentin Iovene defended his PhD thesis on Nov 23rd.
- Binh Nguyen defended his PhD thesis on Dec 10th
- Joseph Ben Zakoun defended his PhD thesis on Dec 15th
- Hugo Richard defended his PhD thesis on Dec 20th
- Quentin Bertrand defended his PhD thesis on Sep 28th
- David Sabbagh defended his PhD thesis on Dec 15th
- Hicham Janati defended his PhD thesis on Mar 23rd
10.3 Popularization
10.3.1 Articles and contents
Philippe Ciuciu has published an article in the Issue of March 2021 in the Contact SKA Magazine, entitled “When the brain meets the stars: Knowledge made visible to the naked eye” (pp. 25-26 or see here)
After the seminal publication about SPARKLING on the Dr Imago website in 20193, Philippe Ciuciu has written a novel article for this online journal dedicated to the medical doctors about Deep learning for MRI (see details here: la-recherche-en-astrophysique-faconne-les-algorithmes-dimagerie-de-demain/).
10.3.2 Education
Gaël Varoquaux, Olivier Grisel, Guillaume Lemaitre, and Loic Esteve have created and run the scikit-learn MOOC (10 000 enrolled, 1 000 finisher)
10.3.3 Interventions
Bertrand Thirion has given a talk at semaine de la science, NeuroSpin, on March 18th, entitled Le décodage de l’activité du cerveau.
11 Scientific production
11.1 Major publications
- 1 articleStochastic Subsampling for Factorizing Huge Matrices.IEEE Transactions on Signal Processing661January 2018, 113-128
11.2 Publications of the year
International journals
International peer-reviewed conferences
Conferences without proceedings
Scientific book chapters
Doctoral dissertations and habilitation theses
Reports & preprints
11.3 Cited publications
- 60 articlePower failure: why small sample size undermines the reliability of neuroscience.Nature Reviews Neuroscience1452013, 365--376
- 61 articleScanning the horizon: towards transparent and reproducible neuroimaging research.Nature Reviews Neuroscience1822017, 115--126