Keywords
Computer Science and Digital Science
- A3.1.1. Modeling, representation
- A3.3.2. Data mining
- A3.3.3. Big data analysis
- A3.4.1. Supervised learning
- A3.4.2. Unsupervised learning
- A3.4.5. Bayesian methods
- A6.1.1. Continuous Modeling (PDE, ODE)
- A9.2. Machine learning
Other Research Topics and Application Domains
- B1.1.8. Mathematical biology
- B2.2.3. Cancer
- B2.4.1. Pharmaco kinetics and dynamics
- B2.4.2. Drug resistance
1 Team members, visitors, external collaborators
Research Scientist
- Sebastien Benzekry [Team leader, Inria, Researcher, from May 2021, HDR]
Faculty Members
- Dominique Barbolosi [Aix-Marseille University, Professor, HDR]
- Joseph Ciccolini [Aix-Marseille Université, Professor, from Jul 2021, HDR]
- Raphaëlle Fanciullino [Aix-Marseille University, AP-HM, Associate Professor, HDR]
- Florence Gattacceca [Aix-Marseille Université, Associate Professor, from Sept 2017, HDR]
- Laurent Greillier [Aix-Marseille Université, Professor, from Jul 2021, HDR]
- Athanassios Iliadis [Aix-Marseille Université, Emeritus, from Jul 2021, HDR]
- Xavier Muracciole [AP-HM, Hospital Staff]
- Anne Rodallec [Aix-Marseille Université, Associate Professor, from Aug 2021]
- Sébastien Salas [AP-HM, Professor]
Post-Doctoral Fellows
- Kokou Atsou [Inria, from May 2021]
- Emilia Kozlowska [Silesian Institute of Technology, from Sep 2021]
PhD Students
- Celestin Bigarre [Inria, from May 2021]
- Anthéa Deschamps [Aix-Marseille University]
- Melanie Donnette [Aix-Marseille Université, from Jul 2021]
- Mourad Hamimed [Aix-Marseille Université, from Aug 2021]
- Clemence Marin [Aix-Marseille Université, from Jul 2021]
- Jessica Ou [Aix-Marseille Université, from Oct 2021]
Technical Staff
- Sarah Giacometti [Aix-Marseille Université, Technician, from Jul 2021]
- Melanie Karlsen [INSERM, Engineer, from Jul 2021]
Interns and Apprentices
- Maria Alexa [ENSAE, from Jun 2021 until Jul 2021]
- Isabella Bicalho-Frazeto [CENTURI]
- Pauline Fleury [Inria, from Aug 2021, UTC Compiègne]
- Benoit Immordino [AMU]
- Ruben Taieb [ENS Lyon]
- Anita Vergnaud [Inria, from Jul 2021 until Aug 2021, EPFL]
Administrative Assistant
- Sandrine Boute [Inria, from May 2021]
External Collaborator
- Laurent Bourguignon [Université Claude Bernard Lyon 1, from Sep 2021]
2 Overall objectives
We aim to optimize therapeutic approaches (i.e., controlling toxicities while ensuring a maximal efficacy) in oncology using mechanistic and statistical modeling (see Figure 1). These therapeutic approaches include immunotherapy, radiotherapy, chemotherapy, targeted therapies and their planification: combinations, sequences, intensification – densification, dosing and scheduling. Specifically, our research will be organized along three main axes:
- Quantitative modeling for personalized clinical oncology.
- Individualizing anticancer drugs regimen.
- Optimizing combinatorial strategies with immune checkpoint inhibitors.
Of note, in the Research Priorities document released by the American Society of Clinical Oncology in February 2021, “Developing and Integrating Artificial Intelligence in Cancer Research”, “Identifying Strategies That Predict Response and Resistance to Immunotherapies” and “Optimizing Multimodality Treatment for Solid Tumors” are listed as top-priorities, which fit quite well with our 3 axes.
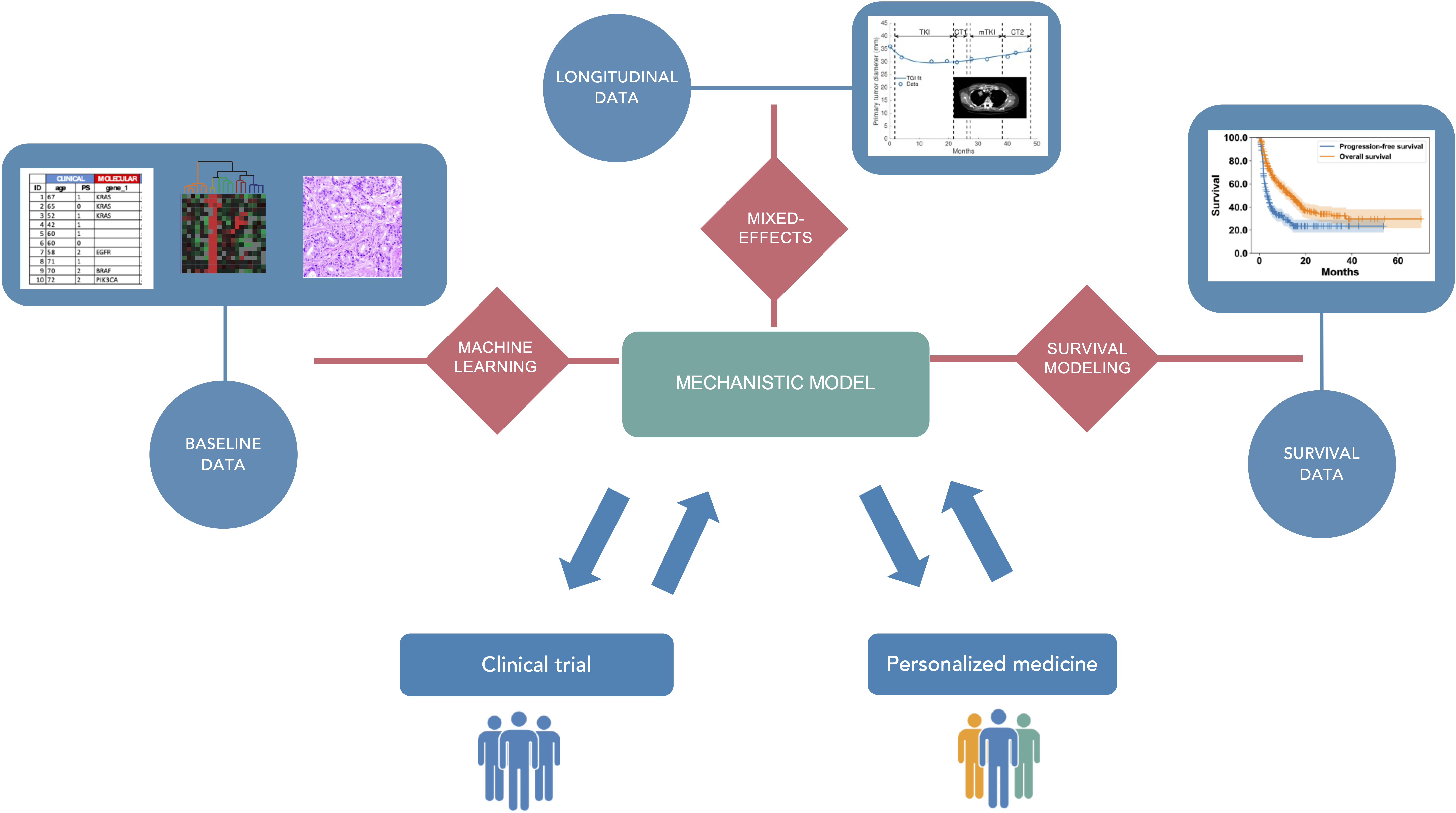
Mechanistic and statistical modeling for pharmacological and clinical oncology.
3 Research program
3.1 Scientific context and motivations
The project-team is based upon the development of model-driven clinical oncology as a means to optimize anticancer therapies. Despite continuous efforts to make available novel drugs beyond traditional cytotoxic chemotherapy (i.e., oral targeted therapies, biologics, immune checkpoint inhibitors), prognosis of outcome remains poor for many cancers. The dosing regimen of anticancer drugs given today remains largely empirical, because dose-finding studies are often performed using outdated, sub-optimal protocols (such as modified-Fibonacci dose-ranging protocols) or because concomitant administration is the rule when combining several drugs. Consequently, clinical oncologists struggle to refine the way they use the anticancer agents made at their disposal. For instance, it took several years of bedside practice to understand that paclitaxel in breast cancer patients should not be administrated using the officially approved 150 mg/m² every 3 weeks scheduling, but rather with an alternate 75 mg/m² weekly dosing 90. Similarly, multi-targets sunitinib is now given on a 50 mg two-weeks on / one-week off basis, rather than the officially approved four-weeks on / two-weeks off schedule 76. Elsewhere, several combinatorial strategies trials have failed to yield convincing results, mostly because of the lack of a strong rationale regarding the best way to sequence treatments 70. Globally, clinical oncology today is still all about finding the best way to treat patients ensuring an optimal efficacy / safety balance.
After having long been limited to cytotoxic chemotherapy (in addition to surgery and radiotherapy), the arsenal of anti-cancer agents has dramatically increased over the last two decades. Indeed, major advances in the understanding of cancer biology, including: 1) the discovery and quantification of (epi)genetic alterations leading to targeted therapy and 2) the realization of the importance of the non-cancer cell components of tumors, i.e., the tumor micro-environment and tumor immunity, have helped to identify novel targets. Drugs targeting the tumor vasculature (e.g., first-in-class bevacizumab, approved in the mid-2000’s 58) or tumor immunity (e.g., immune checkpoint inhibitors (ICI) such as first-in-class ipilimumab, approved in the early 2010’s 88) represent groundbreaking innovations in oncology. ICIs in particular are considered as game-changing drugs because diseases with once dismal prognosis (e.g., metastatic melanoma, non-small cell lung cancer (NSCLC), kidney cancer or head and neck cancer 110) now show 20-40% of 5-years survival. Nevertheless, these impressive results are limited to a minority of patients in a limited number of cancers. In addition, no validated biomarker predictive of response has yet been identified, thus highlighting how early prediction of response and probability of future relapse are a critical, unmet medical need. The encouraging yet still insufficient clinical results of ICIs have led current clinical oncology to consider combinations of such immunotherapies with preexisting anti-cancer modalities: radiation therapy 97, cytotoxic 84, targeted 102 or anti-angiogenic therapies 109. However, the near-infinite possibilities of combinations in terms of sequencing, dosing and scheduling challenge the ability of classical trial-and-error methods to find appropriate modes of combination 69.
In addition, day-to-day clinical decisions made by oncologists are based on a large amount of information, coming from: 1) their own knowledge integrating years of clinical practice combined to updated literature and 2) objective data coming from multiple sources (demographic data, radiology, functional imaging, molecular biology, histology, biomarkers, blood counts, etc…). The large amount of clinical and biological data generated now in clinical oncology is not properly analyzed, because of the lack of appropriate models to picture the complexity of longitudinal observations. Oncologists lack a comprehensive framework and numerical software that could support decision of therapeutic strategy (e.g., to treat or not? to what extent? with what treatment (surgery, radiotherapy, systemic therapy)? in what order? etc.), especially when their time dedicated to examination of a given patient case is limited (e.g., in multidisciplinary meetings (RCP), or individual consultations). Furthermore, modeling is the only way to retrieve similar characteristics from very different experimental conditions and clinical protocols.
To address these major issues, our project-team aims at:
- guiding anticancer therapy by developing patient-specific predictive models (individual level);
- better designing clinical trials, in particular regarding combinatorial trials (population level).
3.2 Data
We use non-clinical and clinical data related with the pharmacology of anti-cancer agents and medical monitoring of the disease status. The former includes pharmacokinetics (drug levels in plasma (patients) and full body pharmacokinetics (animal models)), pharmacodynamics (efficacy, safety), pharmacogenetics (i.e., constitutional genetic polymorphisms affecting drug transport and metabolizing enzymes), pharmacogenomics (i.e., molecular and genetic alterations affecting tumor cells). The latter include demographics, anatomical imaging (e.g., tumor sizes derived from CT scan or MRI), functional imaging (e.g., positron emission tomography), histopathology quantifications, biological variables (such as kidney and liver functions or blood counts), immuno-monitoring data (flow cytometry) or cell free DNA. We will especially rely on real-world data (also termed fragmentary data) collected from patient routine monitoring by our members with hospital activity.
Experimental data are generated by the experimental wet-lab group (AR, RF, JC), relying on state-of-the-art experimental pharmacokinetic laboratory fully equipped to perform in vitro and in vivo explorations of drug metabolism, pharmacokinetics and experimental therapeutics in oncology, including bioanalytical support and fluorescence/bioluminescence monitoring in rodents with highly specialized staff. Clinical pharmacokinetics and pharmacogenetics data are generated by the clinical pharmacology group (JC, RF), relying on the expertise of the clinical pharmacokinetics laboratory of the La Timone University Hospital of Marseille, an FDA-labelled, ISO15189-labelled facility with state-of-the-art bioanalytical resources to assay any kind of drugs or drug metabolites in patients. Specific data regarding cancer biology and pharmacodynamics (immunomonitoring, pharmacogenomics) are generated in collaboration with other CRCM teams.
Clinical data and additional biomarker data are collected from either clinical trials or real-world studies performed by hospital pharmacists and oncologists of the joint-team (RF, JC, SS, LG, XM) and their residents, or by other medical oncologist partners. We have strong collaborations and ongoing projects with pediatrics (Pr N. Andre), hematology (Dr G. Venton), nuclear medicine (Pr D. Taïeb) and radiotherapy (Pr L. Padovani). Importantly, the project-team is located near the INCa-labeled center for early clinical trials (CLIP2), thus facilitating the data collection and later, the implementation of modeling approaches in early clinical trials.
In addition, we also rely on publicly available data from online databases such as the TCGA (genomic data), the TCIA (imaging data) or data from clinical trials.
3.3 Mathematical methodology
Our primary objective is centered on the improvement of therapeutic strategies in oncology. Nevertheless, this brings novel methodological challenges requiring developments at the formal level within the generic field of modeling biological and pharmaco-patho-physiological systems. Difficulties to take into account include: the longitudinal profile of the quantities of interest; measurement uncertainty (requiring statistical considerations); difficulties in sampling the real processes leading to scarcity of the observed data and large inter-individual variability. Many specific problems in life science systems are very different from that encountered from physical modeling in industrial applications (e.g., mechanical engineering or energy).
To summarize our methodology, we are interested in modeling the dynamics of pharmaco-oncological processes (mechanistic modeling) and their inter-individual variability (statistical modeling). Our intended methodological contributions are: 1) to invent novel mechanistic models for complex physiological processes able to describe the effect of therapeutic intervention, 2) to design appropriate statistical frameworks for parameter estimation and description of inter-individual variability, 3) to test and validate the models against experimental and clinical data, 4) to combine state-of-the art machine learning (ML) methods with mechanistic models to integrate large dimension data.
3.3.1 Mechanistic modeling
Mechanistic models are defined here as mathematical constructs that describe physiological variables (e.g., plasma drug concentration, tumor size, or biomarkers) and their dynamics based on physical and biological principles (e.g., law of mass conservation). They describe the time profiles of the variables of interest by means of ordinary or partial differential equations (ODEs and PDEs, respectively) and are thus deterministic.
The main challenge of the modeling exercise is to find the appropriate balance between the degree of integration of biological phenomena (model complexity) and granularity of the data available (i.e., sampling time resolution, observed variables, spatio-temporal or only temporal measurements) ensuring the feasibility of parameter estimation. Indeed, cancer biology is extremely complex, involving processes at multiple temporal and spatial scales (intra- and inter-cellular, tissular, organism). It is thus tempting to build intricate models integrating as many phenomena as possible. Along these lines, the last decades have witnessed the proliferation of multiple such complex models. We see two shortcomings to this approach. First, in contrast with models of physical phenomena, the parameters of biological models are often not directly measurable and thus have to be estimated from fitting the models to experimental or clinical data. Therefore, their number has to be commensurate to the available data in order to ensure identifiability. Unfortunately, many complex models from mathematical oncology have too many parameters to be reasonably identified and have thus had a limited application in terms of biological insights or clinical applications. Second, complex, multiscale models are characterized by a reductionist point-of-view whereby general phenomena could be explained by decomposing them into elementary pieces. However, corresponding elementary experiments would not be suitable for quantification of the several homeostatic mechanisms involved in the whole real process. Thereby, we do not adhere to this reductionist vision and for modeling purposes we rather adopt a holistic approach considering the process as an indivisible whole.
To avoid the above-mentioned caveats, our methodology always starts from: 1) a clinically relevant medical problem but more importantly 2) the data available to build models.
In several instances, the mechanistic models are ordinary differential equations (ODEs). This is the case for the simplest type of experimental data that we generate, i.e., tumor growth kinetics. Departing from previous works establishing models for untreated experimental growth, we are now actively engaged into designing PK/PD models of the effect of multiple therapies. These models have to account the specificities of the drug delivery (e.g., nanoparticles), the biological effect of the treatments (e.g., cytotoxics, antiangiogenics or immunotherapies) and resistance to the therapy (either innate or acquired). The resulting models are novel nonlinear ODEs that need to be validated against the data and, when necessary, theoretically studied for their qualitative behavior. With the advent of immunotherapies, there has been a regain of interest to modeling tumor-immune interactions. Again, despite a wide literature on the subject, very few models have been validated against empirical data. A methodological objective is to establish and validate such models, including effect of immunotherapies.
Description of other phenomena are more adapted to partial differential equations (PDE) models. For instance, following an approach initiated by Iwata et al. 93, structured PDE models can be written for description of a population of metastases (see 4.3.2). Indeed, at the organism scale, cancer diseases are often characterized by a generalized (metastatic) state. However, few modeling efforts are currently focused on this aspect. The only validated models in large cohorts for systemic disease concern the sum of largest diameters as defined by the RECIST criteria 66. We aim to go beyond this state of the art by: 1) providing models of coupled tumor growth with interactions (and quantification of inter-lesion variability) 63, 64 and more importantly 2) developing models accounting not only for growth of the tumors, but also dissemination (birth of new lesions).
To date, most of the available and collectable clinical data about tumor growth and response to therapy consist of scalar data, often even limited to lesion diameters or sum of diameters. This is why we primarily focus our efforts on developing kinetic models of such data, the novelty coming from integrating other longitudinal biological data (e.g., from blood counts). Nevertheless, imaging data are now increasingly accessible and recent advances in image analysis allow the automatic segmentation of lesions make it possible to quantify the spatial shape and texture of tumors without a prohibitive cost for radiologists. This opens the way to develop spatially distributed PDE models of tumor dynamics. The existing models have largely remained unconfronted to data, apart from notable exceptions from the Inria MONC 73 and EPIONE 71 teams, as well as the Swanson 59 and Yankeelov 89 groups. Radiomics approaches quantifying heterogeneity in the images could bring additional information. We will rely on existing or establish collaborations with other dedicated Inria teams (EPIONE, MONC) for such purpose. COMPO would ideally bridge the gap between clinical studies and the Inria ecosystem. Finally, PDE models are also well adapted to describe intra-tumor drug penetration and we have recently developed such models for description of intra-tumor fluid flow and transport of antibody nano-conjugates 111.
3.3.2 Statistical modeling
Statistical models are defined here as mathematical constructs that describe the stochastic sources of variability in the data. They comprise both: 1) classical statistical models defining the functional and probabilistic relationship explicitly and 2) machine learning (ML) algorithms highly based on the data alone (e.g., tree-based models and associated ensemble methods or support vector machines) 86. We use such models for the following purposes: defining appropriate frameworks for parameter estimation; quantitative testing of biological hypothesis; addressing interindividual variability (using nonlinear mixed-effects (NLME) modeling); and building predictive models.
NLME – also termed the population approach in PK/PD modeling, or hierarchical modeling 95 – consists in assuming a statistical distribution of the parameters of the structural (often mechanistic) model, in order to describe longitudinal observations within a population of individuals. Instead of estimating individual parameters on a subject per subject basis – leading to identifiability issues in sparse data situations characteristic of longitudinal measurements in oncology – all data can be pooled together and a joint likelihood is obtained. Likelihood maximization becomes more complex than for classical nonlinear regression, nevertheless this problem has already been addressed by means of algorithms such as the deterministic first-order conditional expansion (FOCE) algorithm 96 or the stochastic approximation of the expectation-maximization algorithm (SAEM) 75. These algorithms are implemented in widely used software in the PMX community such as NONMEM® (Icon) or Monolix® (Lixoft), or R packages (e.g., saemix or nlme). Once the population distribution is estimated, empirical Bayes estimates (EBEs) can be derived for estimation of individual parameters. We also use the language Stan that implements state-of-the art Bayesian methods 67.
Departing from a general distribution of the parameters (often assumed log-normal) with quantified but unexplained interindividual variability, covariates are incorporated to explain this variability and build predictive models. This is traditionally done by means of linear models (possibly up to a functional transformation). However, with the increase in number of such covariates, the traditional tools and algorithms are limited. We thus develop advanced covariate models in NLME incorporating ML algorithms. Such methods require novel contributions. A possible lead is to first identify the EBEs and then use ML algorithms to predict these from the covariates 103. In other cases, ensemble models could be built from the heterogeneous sources of data, integrating one sub-model from EBEs identified from early data. Another, more challenging avenue would be to adapt the parameter estimation algorithms like SAEM to include ML models in the covariate part.
In addition, because few data have been available longitudinally so far (i.e., small number of quantities measured at each time point), the current use of NLME relies on models with a small number of output variables. In this respect, modern clinical oncology studies bring new modeling and statistical challenges because many more quantitative data are collected at each time point (e.g., hundreds of variables from immuno-monitoring or possibly tens of thousands from circulating DNA, or radiomics features from imaging). Defining high-dimensional ODE models describing all the physiologically meaningful variables becomes intractable, therefore new methods are required. A possible avenue is to have a sequential approach, using first ML methods to reduce the dimension, then model the reduced number of variables. Another, more challenging, avenue would be to perform the two tasks (dimension reduction and temporal modeling) at the same time, and include this in an NLME framework for population estimation. The first part could be done using tools from unsupervised learning such as auto-encoders.
Following the availability of longitudinal tumor measurements, recent developments in the field of NLME have concerned joint modeling 77. This consists in modeling the longitudinal kinetics of a biomarker (e.g., tumor size) together with censored time-to-event data (e.g., overall survival) in a single step. Promising results have been obtained so far and we intend to develop methods beyond the state-of-the art in this area. This includes, in connection with above: 1) extension to models with emergence of new metastases, 2) integration of high-dimensional covariates and 3) high-dimensional longitudinal data. This Bayesian integration of data for updated survival predictions could lead to high impact results, as demonstrated by a recent publication in Cell 94.
Finally, we intend to bring the use of established ML tools to address concrete clinical problems emerging from the data collected in routine or clinical trials. Indeed, such data is so far analyzed using traditional statistical methods. ML algorithms could bring added value for predicting efficacy or toxicity from demographic, clinical and biological data.
3.4 Experimental therapeutics in oncology
The project-team is based upon generating experimental and clinical data to identify and test the models, and to provide proof-of-concept studies so as to validate the model-based dosing and scheduling prior to transposing them in patients. Historically, experimental therapeutics in oncology has relied on a wide variety of in vitro and in vivo models mimicking human cancer disease. In oncology, hundreds of in vitro models using cancer cell lines cultivated following 2D or 3D (spheroids) fashion, plus more sophisticated models with cancer cells enriched with fibroblasts or endothelial cells 104, eventually leading to complex organoids 83. Similarly, almost all kind of tumors can be tested in vivo, mostly in small rodents. In oncology, in vivo models are mostly based upon xenografting human tumors from established cell lines or from patient biopsies (patient-derived xenografts or PDX) so as to better mimic human pharmacology when testing active compounds next. To achieve this, several strains of immune-compromised mice have been successfully developed. Because immune checkpoint inhibitors do not exert direct anti-proliferative activity on cancer cells but are rather expected to harness tumor immunity, human xenografts in immuno-compromised mice is not anymore a suitable model. This has led investigators to shift towards immune-competent syngeneic mice models. Non-clinical experiments with drug candidates in immunotherapy mostly focus on deciphering the pharmacology of the targeted pathways, assessing the cytokine release potential, studying receptor occupancy, by using models the most likely to mimic tumor immunity in human. More sophisticated animal models such as human knock-in mice, immuno-avatar, hemato-lymphoid humanized mice or immune-PDX mice have been developed 107 (i.e., allowing to test immune checkpoint inhibitors in mice models combining human xenograft with relevant, humanized immunity and stroma cells) have been made available as well. Beyond generating data on efficacy such as reduction in primary tumor mass or metastatic spreading, experimental models help providing as well in depth knowledge on human and animal target cells, in vitro and in vivo concentration-effect studies, search for biomarkers, plus the most comprehensive knowledge on animal vs. human differences on dose – exposure – effects relationships and finally drug distribution throughout the body, target expression, affinity of target-binding and intrinsic efficacy, duration and reversibility of the effects. In particular, animal drug metabolism and pharmacokinetics (i.e., exploration of liver metabolism and distribution / absorption processes using in vitro or in vivo dedicated models) help understanding the disposition and distribution of the drug in the body throughout time, especially its ability to target tumor tissues (i.e., in vivo distribution in tumor-bearing mice) and helps understanding sources of pharmacokinetic variability. All this information requires state-of-the-art techniques for measuring drugs and drug metabolites into biological fluids in tissues, such as fluorescence-imaging, high-performance liquid chromatography or liquid-chromatography-mass spectrometry bioanalysis. Our team has proven track records in the field of experimental therapeutics in oncology, with two PhDs on developing anticancer nanoparticles in breast and colorectal cancer 81, 106 plus experiments on model-driven way to combine anti-angiogenics with cytotoxics in breast and lung cancers 92, 99, 108, model-driven determination of alternate dosing in neuroblastoma, or methodological studies on monitoring tumor growth 100.
3.5 Axis 1: Quantitative modeling for personalized clinical oncology
The different steps of patient therapeutic management by clinicians consist mostly of: diagnosis, estimation of the extension of the disease, choice of therapy and evaluation of the therapy (efficacy, toxicity). This axis is specifically concerned with such clinical problems, apart from the pharmacological aspects addressed in the other axes.
In this axis, we aim to develop mathematical and statistical models and methods able to process this information to bring added value by inferring hidden parameters and provide simulations and predictions about the past and future behavior of the disease.
In the short-term (4 years), our research projects are: (1) modeling large-scale longitudinal data from immuno-oncology for prediction of response to immune checkpoint inhibition (QUANTIC and TGI-ML projects), (2) developing clinically relevant mathematical models of metastasis and (3) modeling the kinetics of clinical biomarkers.
3.6 Axis 2: Individualizing anticancer drugs dosing regimen
This axis fits within the “population approach” introduced in the 80’s by L. Sheiner 121 and aims at gathering exhaustive information about the multiple sources for variability in response in patients (including but not limited to drug-drug interactions, pharmacogenetics, and cormorbidities affecting renal and liver functions), build specific mechanistic models including relevant covariates and determine the PK/PD relationships of drugs used in oncology-hematology or for treating solid tumors. This covers cytotoxics, oral targeted therapies, biologics or immune checkpoint inhibitors. The overall goal is to achieve precision and personalized drug administration, i.e., the right dosing and scheduling regimen for the right patient.
In the short-term (4 years), our research projects will be focused on the following objectives: 1) predict response or toxicity variability dependent on pharmacogenetic (PGx) and pharmacogenomic data, 2) assess PMX of anticancer agents such as biologics, including ICI, and 3) develop physiologically-based PK models of nanoparticles distribution. Together, these objectives will allow to gain insights in the variability in drug response that depends on PK (1, 2 and 3) or germinal genetic alterations (1).
3.7 Axis 3: Optimizing combinatorial strategies with immune checkpoint inhibitors
Our hypothesis is that so many attempts to combine drugs fail not because the underlying pharmacological concepts are wrong (such as immunogenic cell death triggered by cytotoxics or radiation therapy, or increase in T cells infiltration with anti-angiogenics) but because these combinations probably require fine tuning in terms of dosing, scheduling and sequencing, whereas in practice all the drugs are given the same day. The goal of this second axis is therefore to shift from current empirical and suboptimal combinatorial regimen to model-informed designs to best combine drugs and therapeutic approaches so as to maximize efficacy while controlling toxicities. To do so, we will rely on our pioneering work about model-driven scheduling in early phase trials for combination of cytotoxic agents in metastatic breast cancer (MODEL1 trial) 87, 98 and metronomic vinorelbine in lung cancers (MetroVino trial) 62, 80. Leveraging the unique multidisciplinary aspect of our team, we implement a fully translational approach going from experimental therapeutics, PMX and quantitative systems pharmacology, to clinical trials either in early (phase I/II) or late (phase III) settings. Of note, our group has already an expertise in developing mathematical models determining the best sequencing between chemotherapy and anti-angiogenics.
In the short-term (4 years), our research projects will be focused on providing model-informed designs for combining ICI with: (1) cytotoxics, (2) an experimental immunoliposome and (3) radiotherapy.
3.8 Mid-term objectives
3.8.1 Modeling
We plan to achieve two main things on the modeling side: 1) the development of effective numerical tools (either as web applications or as part of simulation software) and 2) the empirical validation of the models.
For 1), this includes a tool able to predict response to ICI monotherapy in NSCLC from baseline and early response data . We will work with our industrial partners (in particular, HalioDX from the PIONeeR consortium), to transfer the tool for commercial use. Second, We plan to have a validated numerical tool able to predict metastatic relapse from clinical biomarkers at diagnosis, using our mechanistic model. This model will integrate the effect of adjuvant therapy (hormonotherapy or cytotoxic therapy) and will be able to simulate the long-term impact of alternative treatments (e.g., number of cycles to be administered to prevent distant relapse). It will have been validated from our local databases and will be implemented in a clinically-usable online tool such as PREDICT 112. The main difference with this tool will be the ability to mechanistically simulate the effect of therapy. We plan to have initiated a larger initiative at the national or European level to collect large data bases, validate further the predictive power of the model, and refine its structure if required. We will also extend this tool to other pathologies that share the same problematic (diagnosis at early-stage, important probability of future distant relapse) such as kidney cancer, following our initial work from preclinical data 60, 65, 74.
The pharmacometric models that will have been developed will also be implemented as clinically effective dose adaptation numerical tools directly usable to personalize the dose and scheduling of multiple anti-cancer agents not only by the clinicians and pharmacists from our group, but also by others, at least at a regional level.
For 2), our strategy of validation is the following. First, during the
development phase, a proportion of the dataset (usually, 30%) is left
aside unused for establishment of the model and initial calibration, and
then used as a test set. When the sample size is too small (n
3.8.2 Pharmacological and clinical oncology
In axis 2, in addition to standard drugs, developing tools for similarly better understanding the sources of therapeutic and PK variability and understanding the PK/PD relationships of cell therapy in oncology such as CAR-T cell therapies, is a challenging task. The challenge with CAR-T cells is that first, developing bioanalytical tools to monitor them in patients is not trivial, and second, little but nothing is known regarding their PK properties and possible sources impacting on PK/PD relationships such as disease status or immune status of the patient. We aim at developing both a platform to monitor CAR-T cells and future cell therapies in plasma and mechanistic models to describe the disposition of these new therapies in the body.
The nanoPBPK model will be extrapolated to humans and used to determine the specifications of an optimal nanosystem in order to penetrate solid tumors such as pancreatic tumors. The rationally designed nanosystem will be evaluated in vitro and in vivo in order to validate the approach. The nanoPBPK model will be interfaced to become a software and be shared with the scientific community. To achieve this goal, a partnership with ESQlabs, the company developing the opensource PBPK platform PKSim, has already been approved by both sides. The nanoPBPK model will be combined with pharmacodynamic modelling describing the effect of the loaded anticancer drug on tumor growth and metastases spread, on the immune system, and on dose-limiting toxicities.
Our mid-term objective in axis 3 is to assist the design of scheduling regimen for combinatorial treatments in early phase clinical trials, which represent an important clinical challenge of the next 10 years. To do so, we will benefit from our close connection to the INCa-labeled AP-HM's center for early phase clinical trials (CLIP2). Our aim is to design model-based, individualized and adaptive scheduling regimen that depend on the monitoring of the disease evolution. We plan to run phase I/II trials based on the model recommendations. Depending on our achievements and success in phase I/II trials our mid-term goal would be to lead a prospective, randomized, phase III trial comparing a model-based adaptive regimen to the standard of care for combination of immune checkpoint inhibition with chemotherapy and/or anti-angiogenic and targeted therapy. According to our team expertise, the target malignancies would be primarily lung cancer (LG) and head and neck cancer (SS).
3.9 Long-term objectives
At long-term, we globally wish to have established a worldwide leader position in the fields of quantitative mathematical oncology and PMX, as well as the pharmacokinetics of nanoparticles. We hope that this would translate into the achievement of three goals: (1) the development of software effectively used for clinical decision-making and dosing adjustment (estimated achievable), (2) the initiation of prospective, phase III clinical trials comparing model-guided therapy versus standard of care (highly challenging), (3) clinical trials of nanosystems designed by our group (estimated achievable). In addition, we foresee several avenues both in terms of modeling opportunities and applications.
3.9.1 Modeling
Our short-term program is devoted to the development of new models and their confrontation to empirical data. The mid-term program will focus on the validation and refinement of these models. In the long-term, we foresee that this will bring novel questions in terms of mathematical analysis of the models. For instance, metastatic modeling (4.3.2) will establish validated models for tumor-tumor interactions, including immune-mediated interactions (4.3.1). In turn, this leads to nonlinear, size-structured, renewal PDEs. Study of the asymptotic behavior of such equations is non-trivial.
More generally, we expect that physiologically structured PDEs (psPDE) can become relevant to practical modeling in oncology, from two types of data: flow cytometry and single-cell sequencing. Flow cytometry is currently becoming of increasing relevance to characterize multiple populations of cells, for instance in the context of immuno-oncology. In the QUANTIC project (4.3.1), we are starting to interact with such data, only by means of scalar quantities so far. However, the structure of this data is to have, for each cell, a quantitative measure (e.g., a surface marker). Measuring these in a population of millions or billions of cells makes it adapted to modeling by such psPDE. Similarly, single-cell sequencing is a technique by which every cell of a population (e.g., in a tumor) is sequenced, thus having mutation information. In turn, this allows to quantify subclones in the population. Such data has already generated fascinating results, for instance in the study of metastatic development theories 85, 91. Although evolutionary modeling is a wide field with established groups (M. Nowak or F. Michor in Harvard, C. Curtis in Stanford, T. Graham at the CRUK), few groups are modeling dynamical data at single-cell resolution. To this regard, the theoretical work initiated by J. Clairambault and B. Perthame (Inria MAMBA) suggesting to use psPDEs to model evolution in cancer cell populations could be appropriate 68. Parameter estimation in such models is a challenging task 79 and data assimilation from flow cytometry or single-cell sequencing data sets would represent an important avenue. Dynamical data can be provided by circulating tumor DNA and we have already initiated contacts with an important clinical and biological study in Marseille on this topic (the SCHISM study, PIs: SS and F. Fina). The recent developments of technology enabling spatial resolution of single-cell sequencing also paves the way to exciting avenues in terms of modeling 101.
We will also build models that can optimize the effectiveness of treatments incorporating new criteria (other than the evolution of tumor mass) of diagnostic and therapeutic evaluation, especially those we have forged around the information provided by functional imaging (T80 computational algorithm time at which 80% of FDG is metabolized 61, 72, 105.
3.9.2 Pharmacological and clinical oncology
A general, challenging, long-term objective, would be to run prospective clinical trials in which a model-informed arm would be compared to the standard of care. In the model-informed arm, therapeutic decision would be based on the recommendation of the model. This applies to the models developed in all axes. For instance, in breast cancer, the number of cycles of chemotherapy would be adapted based on the model indication (axis 1), decision of the maximum tolerated dose in the treatment of leukemia patients would be based on the PGx/PK/PD model (axis 2) or the combination scheduling regimen would be given by model calculations (axis 3).
Regarding nanosystems initially designed by our group based on the nanoPBPK modelling, they will also be tested in early clinical trials. Our group will drive the design of these based on simulations performed with the nanoPBPK and pharmacodynamic model, in order to guarantee the highest chances of success while ensuring patients safety. In particular, nanoparticles specifically transporting cytoxics could replace standard systemic myelo-ablation in hematopoietic stem cell transplantation, a risky strategy with frequent life-threatening, when not lethal, toxicities. Because of the fully controlled distribution phase in the body, nanoparticles encapsulating several drugs could thus be implemented in the preparative regimens for allogeneic stem cell transplantation in leukemia or myeloid malignancies.
In addition, several groups predict that in addition to standard drugs or biologics, or rising gene therapy and cell therapy strategies, new devices such as nanobots will be developed to treat cancers. Nanobots are entities which are not designed to interact with standard pharmacological targets or genes like current anticancer treatments, but could fix the cancer cell, either by providing a missing protein, or ultimately trigger a mechanic cell-death using radiation, thermal wave, or by disrupting cell membrane. These new entities should exhibit totally new pharmacokinetics, because they are unlikely to be metabolized in the liver or to be cleared by the kidney or the biliary tract. Therefore, new models for PK/PD should be developed, because neither behavior in the body or intrinsic mechanisms of action are known yet. In addition, the issue of nanosafety with such devices will be particularly critical and will require extensive PMX resources, to predict long-term effects or to keep under control the mechanism of action. Should such nanobots be developed, the COMPO joint-team should develop specific resources to monitor their fate in the body, specific resources to write equations describing nanobots/body, nanobots/immune system, and nanobots/cancer cells interactions, plus new global models encapsulating all the interactions, and pharmacodynamics impact of such devices.
Depending on the expertise we gain in the PK/PD knowledge of those cell therapies as part of the mid-term objectives, optimizing combinatorial strategies to such cell therapies will be another long-term objective.
Last, pharmaco-economic studies including impact of quality of life, increase in both tolerability and efficacy will be performed to determine whether PMX-based dosing is a cost-saving strategy. For instance, by refining the scheduling of immune checkpoint inhibitors, such as determining, using modeling and simulation strategies, when the plasma concentration of the drug reaches the threshold in trough levels necessary to ensure maximal target engagement, it will be possible to customize the frequency of the administrations, with possible strong impact on treatment costs. By using real-life data, we propose to use dedicated models to quickly define alternate and more appropriate dosing and/or scheduling with newly marketed anticancer agents, either chemotherapy, targeted therapies or biologics including immune checkpoint inhibitors.
4 Application domains
The COMPO research team's projects all focus on a serial of complementary and inter-related domains described in an itemized fashion below:
- Health: all the models to be developed within the framework of the COMPO team are related to improving healthcare;
- Cancer: in particular, the models will be developed to address specific issues related to cancerous diseases;
- Precision Medicine: in particular, in cancer the developed models will be part of the implementation of precision medicine in oncology focusing on the following items;
- Combinatorial regimen: developing model-informed strategies to determine the optimal modalities when combining several treatments altogether. With the increasingly diversified arsenal of therapeutic approaches to treat cancers (surgery, radiotherapy, chemotherapy, targeted and anti-angiogenic therapy and immunotherapy), defining optimal combination protocols is highly challenging 69. This spans the issues of sequencing, scheduling and dosing of those therapies, which are to date largely addressed using a trial-and-error approaches. Consequently, too many combinatorial trials fail, and attrition rate with combinatorial immunotherapy is now a rising issue in clinical oncology and we hypothesize that extensive modeling and pharmacometrics could help refining the way anticancer drugs are combined;
- Tools for decision-making: developing model-informed strategies to forecast clinical outcome, i.e., during clinical trials. Assessing the predictive power of markers not only at baseline but also in their change over time is a current challenge. The information available, on the basis of which decision is made, includes clinico-demographic variables, classical biomarkers (e.g., blood counts, thyroglobulin, lactate dehydrogenase levels, etc...) but also an increasing amount of data from other sources (e.g., immuno-monitoring, anatomical functional imaging or genomics) that require state-of-the-art modeling to analyze extremely dense and longitudinal data;
- Adaptive dosing strategies: developing model-informed strategies to customize dosing so as to ensure an optimal toxicity-efficacy ratio. All anticancer agents are approved upon registration trials performed in highly selected patients (i.e., with controlled lifestyle, little comorbidities, controlled polymedication and restricted range of age), thus smoothing the interindividual variability. In real-world practice however, patients are all heavily co-treated with a wide variety of other drugs plus herbal medications, likely to interact with drug metabolism and transport, and are frequently older than in clinical trials. In addition, genetic polymorphisms affecting genes coding for drug transport proteins or drug-metabolizing enzymes in the liver, or transcriptional factors can impact as well on dose-exposure relationships. Consequently, standard dosing may not be suitable in non-standard patients to reach the adequate drug exposure levels associated with optimal toxicity/efficacy balance;
- Nanomedicines: developing model-informed strategies to conceptualize drug-loaded nanoparticles likely to improve the toxicity-efficacy ratio over conventional treatments. As of today, the biodistribution phase of anticancer agents is totally aspecific, making "on-taget off-site" actions an issue because it is associated with drug-related side effects affecting healthy tissues. Nanoparticles present unique features likely to deliver specifically a high amount of payload directly on a tumor site, thus improving the antiproliferative action while sparing healthy tissues. In addition, nanoparticles are expected to reshape the tumor micro-environment, making them good candidates to be further associated with immunotherapy (see Combinatorial Regimen above).
5 Highlights of the year
Creation of the project-team as of May 1st, 2021.
5.1 Awards
Coline Sentis received the bronze medal for her MD thesis: “Descriptive and prognosis value of a mechanistic mathematical model of metastatic development in high-risk neuroblastoma" (Supervisors: N. André and S. Benzekry). Associated publication: 15
Clemence Marin received the 2d prize for the young Investigator Award for her presentation "A Multiplex Mass Spec Method for Assaying mAbs Plasma Levels in Patients with Cancer", GPCO-Unicancer, Dijon Nov. 2021 (supervisor: J. Ciccolini). Associated publication: 41
6 New software and platforms
Let us describe here new software and platforms.
6.1 New software
6.1.1 nlml_onco
-
Name:
Nonlinear mixed-effects modeling and machine learning for oncology
-
Keywords:
Nonlinear mixed effects models, Machine learning, Oncology, Survival analysis
-
Functional Description:
This software analyses multiple data arising from clinical oncology (routine care and clinical trials). This data can be of two types: 1) static (e.g., baseline features), from clinical, biological, molecular (e.g., transcriptomic or mutation data) 2) longitudinal (multiple time points per individual): tumor kinetics, biomarkers The second type is modeled using the framework of nonlinear mixed-effects modeling. All features are then analyzed using data science techniques (preprocess, feature selection, machine learning algorithms), in order to predict survival outcome.
-
News of the Year:
Initial development of the software - Exploratory data analysis - Automated mixed-effects modeling analysis - Preprocess - Analysis of high dimensional transcriptomic data - Dimension reduction - Feature selection - Survival machine algorithms - Applications (
,000 patients) - Individual predictions of survival - Predictions of hazard ratios for applications in drug development, for both monotherapy and combinatorial treatments - URL:
-
Contact:
Sebastien Benzekry
-
Participant:
Sebastien Benzekry
6.1.2 metastats
-
Keywords:
Nonlinear mixed effects models, Mechanistic modeling, Metastasis, Cancer, Survival analysis, Data analysis
-
Functional Description:
Code to fit a mechanistic model of time to metastatic relapse to clinical data, using mixed-effects statistical learning. It contains simulation of the natural history of primary and secondary tumors. Statistical population distributions of the parameters can be fitted to describe right censored time-to-event data. Covariates can be included to perform predictive modeling. Evaluation of predictive performances (c-index, calibration plots and classification metrics) are implemented.Cox and survival random forest analysis are also implemented.
-
News of the Year:
The code was totally reworked and bundled as an R package to run and fit the model. The simulation code was ported from a python prototype to an optimized c++ version directly available from R. This new version of the model code enabled considerable gains of computational time when fitting the mixed effect model. A particular attention was ported on the package architecture to make it as easy as possible to use and maintain. The model was used on breast cancer data from several databases (Bergonié Institute, AP-HM, open-access data) to assess its performances. The results will be the subject of a communication in the 2022 AACR annual connference and of a later publication.
- URL:
- Publication:
-
Contact:
Sebastien Benzekry
-
Participants:
Celestin Bigarre, Chiara Nicolo, Sebastien Benzekry
-
Partner:
Institut Bergonié
6.1.3 stats_pioneer
-
Keywords:
PIONeeR, Statistical analysis, Biostatistics
-
Functional Description:
This software was built to analyse the PIONeeR (Precision Immuno-Oncology for advanced Non-small cell lung cancer patients with PD-(L) 1 ICI Resistance) data. PIONeeR is a prospective, multicenter study with primary objective being to validate the existence of a hypothetical immune profile explaining resistance to immunotherapy in non-small cell lung cancer patients.
This software corresponds to the very first step of the data analysis, which is the statistical analysis. Some of its functions aim at: - preprocessing the data (creation of clinical variables, dictionary, outcome variables, clinical biomarkers, treatment of the variables types) - computing statistical tests, logistic or Cox regression, or performing a correlation analysis - visualising the data (boxplots, barplots, survival curves, ROC curves, volcano plots)
-
News of the Year:
Reception of the PIONeeR data in october 2021, first exploratory data analysis, basic statistical analysis, establishment of the first version of the statistical analysis software, use of this package to create a detailed report of basic survival analysis and classification. Multiple outcomes have been studied, both in classification (several definitions of responders/ non-responders are possible) and in survival analysis (overall survival and progression-free survival).
-
Authors:
Sebastien Benzekry, Melanie Karlsen, Kokou Atsou
-
Contact:
Sebastien Benzekry
6.1.4 nsclc_predict
-
Keywords:
Machine learning, Oncology, Biomarkers, Prediction, Immunotherapy
-
Functional Description:
Prediction of efficacy and toxicity in response to immune-checkpoint inhibition from baseline clinico-biological values in non-small cell lung carcinoma. Written in R and based on tidymodels
-
News of the Year:
Exploratory data analysis, basic statistical analysis, study and comparison of several classification algorithms and several performance metrics, survival analysis for the OS (overall survival) and PFS (progression-free survival) using cox regression.
Publication : Machine learning for prediction of immunotherapy efficacy in non-small cell lung cancer from simple clinical and biological data S. Benzekry, M. Grangeon, M. Karlsen, M. Alexa, I. Bicalho-Frazeto, S. Chaleat, P. Tomasini, D. Barbolosi, F. Barlesi, L. Greillier Cancers, Volume 5, pp. 81-90, medRxiv, 2021
- URL:
- Publications:
-
Contact:
Sebastien Benzekry
-
Participants:
Sebastien Benzekry, Melanie Karlsen
6.1.5 metamats_size
-
Name:
Fitting longitudinal data of size and number of metastases using mechanistic models
-
Keywords:
Cancer, Mechanistic modeling, Metastasis, Regression, Simulation, Data assimilation
-
Scientific Description:
This software fits models of metastatic development to longitudinal data of metastatic sizes and provides simulation and visualization tools for metastatic modeling.
-
Functional Description:
This software fits models of metastatic development to longitudinal data of metastatic sizes and provides simulation and visualization tools for metastatic modeling.
-
News of the Year:
Development of the software to analyze a database of 30+ patients. Addition of features to predict overall and prediction free survival from the mathematical parameters
- URL:
- Publication:
-
Contact:
Sebastien Benzekry
-
Participants:
Sebastien Benzekry, Mariia Bilous
-
Partners:
Centre de Recherches en Cancérologie de Marseille, Institut Bergonié, Assistance Publique - Hôpitaux de Marseille
6.2 New platforms
6.2.1 LC-MS/MS platform for bioanalysis of mAbs
Participants: Clémence Marin, Joseph Ciccolini.
Name: MassSpec_for_mAbsKeyword: mass spectrometry, bioanalysis, ISO15189
Scientific description: this mass spec plateform is dedicated to the measurement of therapeutic mAbs in plasma (i.e., atezolizumab, bevacizumab, cetuximab, ipilimumab, nivolumab, pembrolizumab, rituximab) during investigational PK studies and real-world therapeutic drug monitoring.
Functional description: MassSpec_for_mAbs is a multiplex, liquid chromatography method coupled to tandem mass spectrometry detection covering a 1-100 µg/ml range with a 15% precision, fully validated following ICH guidelines.
Publications: 41
Contact: Joseph Ciccolini
7 New results
7.1 Pharmacometrics modeling coupled with machine learning for early prediction of overall survival following atezolizumab monotherapy in non-small cell lung cancer
Participants: Sébastien Benzekry, Mélanie Karlsen, Abdessamad El Kaoutari, Suresh Vatakuti, Peter Curle, Candice Jamois.
Funding and data: Roche pRED
Publication: Abstract sent to the world conference on pharmacometrics 2022.
BACKGROUND: Pharmacometrics (PMx) and Machine Learning (ML) approaches could help to predict survival based on patients’ and disease characteristics and early response to treatment.
METHODS: We developed a predictive algorithm of survival following atezolizumab (ATZ) therapy based on the combination of nonlinear mixed-effects modeling (NLME) and ML. The data consisted of three phase 2 trials (training set: 862 patients) and one phase 3 trial (test set: 553 patients). Longitudinal data included tumor kinetics (5,570 data points) and four pharmacodynamic (PD) markers: neutrophils, C-reactive protein, lactate deshydrogenase and albumin (61,296 data points). Baseline data included: clinical factors (p = 73 variables), transcriptomic data (p = 58,311 transcripts) and mutation data (p = 395 genes). Double-exponential and hyperbolic models were used for NLME and a random survival forest for ML. Cross-validation was used to assess the predictive performances of the model (c-index, landmark time classification metrics and calibration curves).
SOFTWARE: This project led to the development of the software nlml_onco. It is an R package that implements the following tasks: 1) preprocess of the data (including missing data imputation and scaling), 2) NLME fit and individual empirical Bayes estimations (possibly using an external prior), 3) dimensionality reduction, 4) feature selection (selected among 5 methods), 5) ML (train, cross-validation or predict) and 6) post-processing. In addition, the software allows data truncation either after completion of a number of cycles or a given study duration. As output, the software generates either individual- or study-level predictions
RESULTS: The best models were double-exponential (TK, neutrophils, CRP, LDH) and hyperbolic (albumin) models for NLME and a random survival forest for ML. A minimal ML model was derived that contains 11 routine clinical variables (CRP, heart rate, neutrophils-to-lymphocyte ratio, neutrophils, lymphocytes-to-leucocytes ratio, hepatic metastases, ECOG, PDL1, hemoglobin, baseline tumor size and LDH), together with 3 TK and 9 PD model derived parameters obtained from Bayesian estimation. Predictive power was significantly improved when using the PD data compared with models based either on clinical variables or TK parameters for both discrimination (c-index = 0.83 ± 0.02 vs 0.72 ± 0.04 vs 0.72 ± 0.02 in cross-validation, 0.79 vs 0.69 vs 0.70 in test, 1-year AUC = 0.92 ± 0.02 vs 0.80 ± 0.05 vs 0.81 ± 0.05) and calibration metrics.
CONCLUSION: Our novel prediction algorithm was able to predict accurately survival following atezolizumab monotherapy, both at the study and individual levels.
7.2 Comprehensive biomarkers analysis to explain resistances to PD1/L1 ICIs: The Precision Immuno-Oncology for advanced Non-Small CEll Lung CancER (PIONeeR) trial.
Participants: Laurent Greillier, Florence Monville, Vanina Leca, Frédéric Vely, Stéphane Garcia, Joseph Ciccolini, Florence Sabatier, Gilbert Ferrani, Nawel Boudai, Lamia Ghezali, Marcellin Landri, Clémence Marin, Mourad Hamimed, Laurent Arnaud, Arnaud Boyer, Mélanie Karlsen, Kevin Atsou, Pauline Fleury, Clarisse Audigier Valette, Stéphanie Martinez, Hervé Pégliasco, Louisiane Lebas, Patricia Barré, Sarah Zahi, Ahmed Frika, Pierre Bory, Maryannick Le Ray, Lilian Laborde, Virginie Martin, Richard Malkoun, Marie Roumieux, Julien Mazières, Maurice Perol, Eric Vivier, Sébastien Benzekry, Jacques Fieschi, Fabrice Barlési.
Funding : This work is supported by French National Research Agency (ANR-17-RHUS-0007, a partnership of AMU, APHM, AstraZeneca, Centre Léon Bérard, CNRS, Veracyte, ImCheck Therapeutics, Innate Pharma, Inserm, Institut Paoli Calmettes and sponsored by AP HM and initiated by Marseille Immunopole. It is also supported by Plan Cancer Inserm grant number 19CM148-00 as part of the QUANTIC project.
Publication: Abstract sent to the AACR conference 2022.
BACKGROUND: Resistance to PD1/L1 immune checkpoint inhibitors (ICIs) in advanced NSCLC patients is observed in about 80% of individuals with no robust predictive biomarker yet. The PIONeeR trial (NCT03493581) aims to predict such resistances through a comprehensive multiparametric biomarkers analysis.
METHODOLOGY: Among the >300 advanced NSCLC patients (pts) recruited in PIONeeR, we focused on the first 137
Immune contexture was characterized in tumor and blood of each pt through FACS for circulating immune cell subtypes quantification, dual- and multiplex IHC / digital pathology to quantify activating & suppressive immune cells infiltrating the tumor, standardized methods for endothelial activation assessment, blood soluble factors dosage and tumor & blood WES (TMB and other). ICI plasma levels and pharmacokinetics parameters were also monitored, leading to 331 measured biomarkers in addition to routine clinical parameters. Multivariable (MV) logistic regression was used to examine the association of each biomarker (controlled by sex, age, smoking status, histological type & PD-L1 TC expression) with the risk of Early Progression (EP), i.e. within 3.5 months of treatment. Multivariable Cox regression analysis was conducted for association with PFS and OS.
SOFTWARE: This project is associated to the development of the stats_pioneer software.
RESULTS: Overall, the 137 pts were mainly male (64%), smokers (92%) and <70yrs (68%). Tumors were mainly non-squamous (79%) with >1% PDL1+ TC in 36% of the cases. 21% were still on treatment at data cut-off. Archived samples were available for 109 patients (80%) at inclusion and re-biopsy was available in 52.9% of these cases. The median follow up was 19.8 months, 22.5% of pts did not progress at data cut-off, and 85 patients present with EPs.
Tumor Cytotoxic T-cells density, especially PD1+ were lower in EP (MV OR=0.45, p=0.022); conversely, higher proportions of activated cytotoxic T-cells were observed among circulating lymphocytes in EP (MV OR=3.8, p<0.001). Among other biomarkers, Tregs (MV OR=0.44, p=0.018), 3 NK sub-populations (MV OR
CONCLUSION: The PIONeeR trial provides with the 1st comprehensive biomarkers’ analysis of to establish predictive models of resistance in advanced NSCLC pts treated with PD1/L1 ICIs and highlights how tumor and circulating biomarkers are complementary.
7.3 Machine Learning for Prediction of Immunotherapy Efficacy in Non-Small Cell Lung Cancer from Simple Clinical and Biological Data
Participants: Sébastien Benzekry, Mathieu Granjeon, Mélanie Karlsen, Maria Alexa, Isabella Bicalho-Frazeto, Solène Chaleat, Pascale Tomasini, Dominique Barbolosi, Fabrice Barlési, Laurent Greillier.
Publication: Cancers14
Funding : This work is supported by Plan Cancer Inserm grant number 19CM148-00 as part of the QUANTIC project.
Simple Summary: We studied determinants of response to immune-checkpoint inhibition in ad-vanced non-small cell lung cancer patients. Specifically, we evaluated the association with responseof multiple simple pre-treatment blood markers available from routine examination. We first usedclassical statistical tools and then developed a machine learning algorithm for individual predictions.We obtained a 69% accuracy. Hemoglobin levels and performance status were the strongest predic-tors. Neutrophil-to-lymphocyte ratio was also associated with outcome. A benchmark of 8 machinelearning models also evidenced that the best model performed almost equally well than a logisticregression (basic statistical learning model).
Keywords:blood counts; lung cancer; response; survival; prediction; machine learning
BACKGROUND: Immune checkpoint inhibitors (ICIs) are now a therapeutic standard inadvanced non-small cell lung cancer (NSCLC), but strong predictive markers for ICIs efficacy arestill lacking. We evaluated machine learning models built on simple clinical and biological data toindividually predict response to ICIs.
METHODS: Patients with metastatic NSCLC who received ICIin second line or later were included. We collected clinical and hematological data and studied theassociation of this data with disease control rate (DCR), progression free survival (PFS) and overallsurvival (OS). Multiple machine learning (ML) algorithms were assessed for their ability to predictresponse.
RESULTS: Overall, 298 patients were enrolled. The overall response rate and DCR were 15.3% and 53%, respectively. Median PFS and OS were 3.3 and 11.4 months, respectively. In multivariable analysis, DCR was significantly associated with performance status (PS) and hemoglobin level(OR 0.58,p< 0.0001; OR 1.8,p< 0.001). These variables were also associated with PFS and OS and ranked top in random forest-based feature importance. Neutrophil-to-lymphocyte ratio was also associated with DCR, PFS and OS. The best ML algorithm was a random forest. It could predict DCR with satisfactory efficacy based on these three variables. Ten-fold cross-validated performances were: accuracy 0.68 ± 0.04, sensitivity 0.58 ± 0.08; specificity 0.78 ± 0.06; positive predictive value 0.70 ± 0.08; negative predictive value 0.68 ± 0.06; AUC 0.74 ± 0.03. Conclusion: Combination of simple clinical and biological data could accurately predict disease control rate at the individual level.
7.4 A mechanistic model for prediction of metastatic relapse in early-stage breast cancer using routine clinical features
Participants: Célestin Bigarré, Pascal Finetti, François Bertucci, Dominique Barbolosi, Xavier Muracciole, Sébastien Benzekry.
Authors: Célestin Bigarré, Pascal Finetti, François Bertucci, Dominique Barbolosi, Xavier Muracciole, Sébastien Benzekry
Funding: Inria-Inserm Phd Grant
Publication: Abstract sent to the AACR Anual meeting 2022.
BACKGROUND: Estimation of the risk of metastatic relapse is a major challenge to decide treatment options for early-stage breast cancer patients. To date, metastatic free survival (MFS) analysis mainly relies on classical – agnostic – statistical models (e.g., Cox regression). Instead, we propose to derive mechanistic models to predict MFS.
METHODS: The data consisted of patients who did not receive adjuvant systemic therapy from two databases: one (data 1, N = 163) with routine clinical features and the PAI-1 and uPA biomarkers and two (data 2, N = 692) with 11 routine clinical features. The mathematical models are based on a partial differential equation describing a size-structured population of metastases. They predict MFS from the size of the tumor at diagnosis and two mathematical parameters,
SOFTWARE: The model was implemented as an R package using optimized C++ code for the mechanistic part and enabling parallelization, resulting in a 4-fold reduction of the computing compared to a former python implementation.
RESULTS: The model selection procedure on data 1 revealed an association of PAI1 and age with
CONCLUSION: Mechanistic modeling was able to unravel the biological and predictive role of PAI-1 but showed limited predictive power when using only on routine clinical features.
7.5 Development and Validation of a Prediction Model of Overall Survival in High-Risk Neuroblastoma Using Mechanistic Modeling of Metastasis
Participants: Sébastien Benzekry, Coline Sentis, Carole Coze, Laëtitia Tessonnier, Nicolas André.
Publication: JCO: Clinical Cancer Informatics15
Prognosis of high-risk neuroblastoma (HRNB) remains poor despite multimodal therapies. Better prediction of survival could help to refine patient stratification and better tailor treatments. We established a mechanistic model of metastasis in HRNB relying on two processes: growth and dissemination relying on two patient-specific parameters: the dissemination rate μ and the minimal visible lesion size Svis. This model was calibrated using diagnosis values of primary tumor size, lactate dehydrogenase circulating levels, and the meta-iodobenzylguanidine International Society for Paediatric Oncology European (SIOPEN) score from nuclear imaging, using data from 49 metastatic patients. It was able to describe the data of total tumor mass (lactate dehydrogenase, R2 > 0.99) and number of visible metastases (SIOPEN, R2 = 0.96). A prediction model of overall survival (OS) was then developed using Cox regression. Clinical variables alone were not able to generate a model with sufficient OS prognosis ability (P = .507). The parameter μ was found to be independent of the clinical variables and positively associated with OS (P = .0739 in multivariable analysis). Critically, addition of this computational biomarker significantly improved prediction of OS with a concordance index increasing from 0.675 (95% CI, 0.663 to 0.688) to 0.733 (95% CI, 0.722 to 0.744, P < .0001), resulting in significant OS prognosis ability (P = .0422).
7.6 Machine learning and mechanistic modeling for the prediction of Overall Survival on the basis of first line tumor kinetics in Head and Neck Squamous Cell Carcinoma
Participants: Kevin Atsou, Anne Auperin, Joël Guigay, Sébastien Salas, Sébastien Benzekry.
Funding: This work is supported by Plan Cancer Inserm grant number 19CM148-00 as part of the QUANTIC project.
Publication: to be submitted
BACKGROUND: The prediction of Overall Survival and Response to 2nd line treatment is a major challenge in treatment of Head and Neck Squamous Cell Carcinoma (HNSCC). Existing prediction algorithms are only based on baseline features and depend on mere survival analysis statistical tools which are agnostic. Here we used tumor kinetics measured by the Sum of the Largest Diameters (SLD) of the lesions in the patients during the first line to provide interesting predictive metrics for Overall Survival.
METHODS: The data we used comes from a multicentre, open-label, randomised, phase 2 trial, done in 68 centres in France, Spain and Germany aiming to compare the efficacy and safety of the TPEx(platinum-docetaxel-cetuximab) regimen against the standard of care regimen: EXTREME (platinum-fluorouracil-cetuximab). 528 patients were included in the clinical trial and the data used in our analysis consist of 526 patients with 38 baseline clinical features. First, a mechanistic model of tumor growth inhibition was fitted to a training data (70% of the full data) using mixed-effects modeling. Then, different machine learning algorithms for survival analysis (such as Random Survival Forest, DeepSurv, Cox-Lasso, …) were assessed on the training data set for their ability to predict the Overall Survival of the patients. Subsequently, the best performing model on the training set was used to predict the overall survival on a test data set (30 % of the full data).
SOFTWARE: The model was implemented as an R package using keras and pytorch for the implementation of some machine learning algorithms for survival analysis.
RESULTS: Using a mechanistic mathematical model, we were able to fit the clinical data of SLD measurements. The fitted mathematical model revealed a significant association between the treatment arms and the tumor growth rate. Which were not possible with a mere Cox regression analysis. The Random Survival Forest Algorithm combined with the mechanistic model was able to predict the Overall Survival with a C-index of 0.75 in 10-folds cross-validation on the train set and 0.67 on the test set. Likewise, the most impacting parameters for the prediction of OS are the parameters resulting from the mathematical model describing the kinetics of tumors (the tumor growth rate and Time to Growth after shrinkage).
CONCLUSION: By using only the first line SLD measurements, the proposed model could be used to predict the Survival of patients as a surrogate of their response to specific first line treatments.
7.7 Pharmacogenetics of cytarabine in AML patients
Participants: Mélanie Donnette, Joseph Ciccolini, Geoffroy Venton, Regis Costello, Laure Flarnault, Raphaelle Fanciullino.
Funding and data: AORC Junior, APHM
BACKGROUND: Cytarabine is a mainstay to treat AML patients and erratic pharmacokinetics could explain unpredctible clinical outcome, including lethal toxicities.
METHODS: This is a clinical trial registred as EudraCT 2017-A00070-54 (PI: Dr Raphaelle Fanciullino) aiming at studying the pharmacokinetics of adult AML patients treated with the standard 7+3 induction and consolidation regimen. Additional PGx testing on CDA and CdK enzymes are performed.
SOFTWARE: PK modeling and PK/PGx/PD relationships are investigated on MonoLix (Lixoft France).
RESULTS: Results showed that incidence of CDA deficiency is significantly higher in patients with blood disorders as compared with patients with solid tumors or patients with no cancers. CDA deficiency was associated with marked over-exposure un cytarabine, a decrease in metabolization, and a trend towards more frequent and more severe toxicities, including lethal toxicities.
CONCLUSION: Prior search for the CDA deficiency syndrome could help to customize cytarabine dosing to limit the risk of life-threatening toxicities.
7.8 Pharmacogenetics of CPX-351 in AML patients
Participants: Mélanie Donnette, Mourad Hamimed, Geoffroy Venton, Laure Flarnault, Joseph Ciccolini, Raphaelle Fanciullino.
Funding and data: AORC Junior, APHM
Publication: 31, abstract submitted to AACR 2022
BACKGROUND: CPX-351 is a liposomal combination between daunorubicin and cytarabine approved in AML patients. Little is known about the impact of CDA gene polymorphism on the fate and of CPX-351 and resulting clinical outcome.
METHODS: This is an ancillary study to the clinical trial registered as EudraCT 2017-A00070-54 (PI: Dr Raphaelle Fanciullino) on a subset of adult AML patients treated with CPX-351. Full PK and PGx investigatiosn were performed.
SOFTWARE: PK modeling and PK/PGx/PD relationships are investigated on MonoLix (Lixoft France).
RESULTS: Results showed that surprisingly, CDA deficiency has strong impact on the PK of liposomal CPX-351. CDA-deficient patients had a trend towards decreased clearance, sharp overexposure and non-hematological toxicities when treated with CPX-351.
CONCLUSION: Unexpectedly, CDA deficiency could have a deleterious impact on the clinical outcome of patients treated with liposomal CPX-351.
7.9 Implementing adaptive dosing strategy with targeted therapies in mRCC patients
Participants: Florent Ferrer, Jean-Laurent Deville, Jonathan Chauvin, Gwenaelle Gravis, Joseph Ciccolini.
Funding and data: Canceropole Emergence, APHM and Institut Paoli Calmettes
Publication: 33, 82, Abstract submitted to AACR 2022
BACKGROUND: oral targeted therapies (i.e., sunitinib, cabozantinib) are pivotal drugs to treat metastatic renal cell carcinoma currently administered as flat dosing. To what extent adaptive dosing strategies could help improving clinical outcome is yet to be established.
METHODS: This is a real-world, observational study in mRCC patients from the APHM and the IPC cancer Center. Data from routine therapeutic drug monitoring of sunitinib and cabozantinib were used retrospectively in an adaptive dosing procedure implemented in Monolix. Models recommendation were compared with real-world data regarding efficacy and safety.
SOFTWARE: PK modeling and adaptive dosing algorithm was performed on MonoLix (Lixoft France)
RESULTS: Results show that model recommendations would have allowed to quickly reduce the dosing based upon early TDM sampling, thus avoiding the occurrence of severe toxicities in mRCC patients. Similarly, the model would have proposed to increase dosing in most patients for which progressive dosing was observed, thus suggesting that efficacy of the treatment could have been improved using model-based dosing.
CONCLUSION: This study calls for the implementation of prospective adaptive dosing with sunitinib and cabozantinib in mRCC patients.
7.10 Cetuximax: maximizing Cetuximab efficacy in head and neck cancer patients through PK/PD modeling
Participants: Clémence Marin, Mourad Hamimed, Joseph Ciccolini, Sébastien Salas.
Funding and data: Merck Serono, APHM, GPCO-Unicancer network
Publication: IATDMCT, Rome, 2021 and Abstract submitted to AACR 2022
BACKGROUND: anti-EGFR cetuximab is a pivotal mAb in head and neck cancer patients in association with chemotherapy. Therapeutic window of cetuximab and possible room for adaptive dosing strategies are unknown.
METHODS: In this multicentric clinical trial registered as NCT4218136 (P.I.: Pr Sebastien Salas), pharmacokinetics and pharmacodynamics of cetuximab will be both assessed so as to help determining the metrics (e.g., trough levels, Cmax, AUC) associated with both efficacy and toxicity of cetuximab in head and neck cancer patients. Once the therapeutic window is established, an adaptive dosing algorithm will be propose to customize drug dosing and/or scheduling.
SOFTWARE: PK/PD modeling and adaptive dosing algorithm will be performed on MonoLix (Lixoft France)
RESULTS: Early Results suggest that 34 µg/ml could be the threshold associated with efficacy upon Recist criteria. Conversely, it is not possible yet to identify exposure levels associated with severe toxicities. A large inter-individual variability in PK parameters and exposure levels has been observed (i.e., > 60%), calling for developing therapeutic drug monitoring strategies to check exposure levels.
CONCLUSION: the early results of this study confirm that PK/PD relationships with cetuximab plus large variability observed in exposure levels warrants the need for customizing dosing to ensure a maximum efficacy.
7.11 BUSEQ
Participants: Joseph Ciccolini, Sabine Furst, Didier Blaise, Raynier Devillier.
Funding and data: IPC and CHU Nice
Publication: ASH Meeting, 2019
BACKGROUND: High dose busulfan (HD Busulfan) is the backbone for bone marrow transplant conditioning but the best way to schedule its administration remains unknown. HD Busulfan is associated with up to 30% of toxic-death.
METHODS: In this multicentric clinical trial registered NCT 2020-003392-17 (P.I.: Pr Rainier Devillier IPC), pharmacokinetics and pharmacodynamics of HD Busulfan administered in a sequential fashion are investigated. Dosing is customized using a pop-PK approach so as to reach a predefined target exposure. Treatment-unrelated mortality.
SOFTWARE: PK modeling and adaptive dosing algorithm will be performed on KineticPro (MIPPS, France).
RESULTS: Preliminary results show that pop-PK identification of individual parameters after the first injection of HD Busulfan allows to customize the dosing in an iterative fashion to target a global AUC defined as the exposure to be reached.
CONCLUSION: PK-guided dosing of HD Busulfan is feasible and allows to target accurately a predefined exposure level.
8 Bilateral contracts and grants with industry
8.1 Research contracts
D-LIGHT
- Partner: Roche
- Title: Tumor growth inhibition modeling coupled with machine learning for early response prediction and identification of common features of response, to optimize combination therapies in Oncology
- Funding: 76.5 k€
- Duration: Jan 2021 - Jan 2022
- Principal investigator: S. Benzekry
NanoImmuno
- Partner: Institut Roche France and Genentech US
- Title: NanoImmuno: a nanoparticle to harness tumor immunity in her2+ breast cancer
- Funding: 80 k€
- Duration: Jan 2020 - March 2023
- Principal investigator: Raphaelle Fanciullino (Senior), Anne Rodallec (Junior)
8.2 Clinical trials
Cetuximax
- Registration: NCT4218136
- Partner: Merck Serono
- Title: Maximizing Cetuximab efficacy in head and neck cancer patients through PK/PD modeling
- Funding: 40 k€
- Duration: 2020 - 2023
- Principal investigator: Sébastien Salas
MOVIE
- Registration: NCT03518606
- Partner: Astra Zeneca and INCa
- Title: Metronomic Oral Vinorelbine Plus Anti-PD-L1/Anti-CTLA4 ImmunothErapy in Patients With Advanced Solid Tumours
- Funding: 500 k€
- Duration: 2019-2022
- Principal investigator: Anthony Goncalves (IPC)
- Compo investigator: Joseph Ciccolini
MOIO
- Registration: NCT05078047
- Partner: BMS and INCa
- Title: Study Comparing the Standard Administration of IO Versus the Same IO Administered Each 3 Months in Patients With Metastatic Cancer in Response After 6 Months of Standard IO
- Funding: 500 k€
- Duration: 2020-2024
- Principal investigator: Gwenaelle Gravis (IPC)
- Compo investigator: Joseph Ciccolini
CytaPGx
- Registration: EudraCT 2017-A00070-53
- Partner: APHM and CAL Nice
- Title: Constitutive biomarkers in AML patients treated with cytarabine
- Funding: 80 k€ (APHM)
- Duration: 2018-2021
- Principal investigator: Raphaelle Fanciullino
VIDAZAML
- Registration: EudraCT 2020-A02042-37
- Partner: APHM and CAL Nice
- Title: Role of CDA and dCK metabolic enzymes in clinical response of patients treated with azacitidine for blood disorders
- Funding: 80 k€ (APHM)
- Duration: 2021-2023
- Principal investigator: Geoffroy Venton - Compo Investigator: Raphaelle Fanciullino
Morpheus-Lung
- Registration: Eudract 2017-001267-21 / NCT03337698
- Partner: Hoffmann-La Roche
- Title: A Study to Evaluate Efficacy and Safety of Multiple Immunotherapy-Based Treatment Combinations in Patients with Metastatic Non-Small Cell Lung Cancer
- Duration: 2018-2023
- Compo investigator: Laurent Greillier
PDC-LUNG-101
- Registration: Eudract 2018-002382-19 / NCT03970746
- Partner: PDC*line Pharma SAS
- Title: Safety, immunogenicity and preliminary clinical activity study of PDC*lung01 cancer vaccine in NSCLC
- Duration: 2022-2024
- Principal investigator: Johan Vansteenkiste, Prof KU Leuven
- Compo investigator: Laurent Greillier
NCT03840915
- Registration: Eudract 2018-004040-28 / NCT03840915
- Partner: EMD Serono Research and Development Institute, Inc., Merck KGaA
- Title: A Phase Ib/II, Open-Label Study of M7824 in Combination With Chemotherapy in Participants With Stage IV Non-small Cell Lung Cancer
- Duration: 2019-2022
- Compo investigator: Laurent Greillier
NCT03708328
- Registration: Eudract 2018-000982-35 / NCT03708328
- Partner: Hoffmann-La Roche
- Title: A Dose Escalation and Expansion Study of RO7121661, a PD-1/TIM-3 Bispecific Antibody, in Participants With Advanced and/or Metastatic Solid Tumors
- Duration: 2018-2023
- Compo investigator: Laurent Greillier
PROPEL
- Registration: Eudract 2019-003474-35 / NCT03138889
- Partner: Nektar Therapeutics
- Title: Bempegaldesleukin and Pembrolizumab With or Without Chemotherapy in Locally Advanced or Metastatic Solid Tumors
- Duration: 2017-2024
- Compo investigator: Laurent Greillier
TRIDENT-1
- Registration: Eudract 2016-003616-13 / NCT03093116
- Partner: Turning Point Therapeutics, Inc.
- Title: A Study of Repotrectinib (TPX-0005) in Patients With Advanced Solid Tumors Harboring ALK, ROS1, or NTRK1-3 Rearrangements
- Duration: 2017-2023
- Principal investigator: Shanna Stopatschinskaja, Dan Liu
- Compo investigator: Laurent Greillier
NCT04721015
- Registration: Eudract 2020-004953-57 / NCT04721015
- Partner: AbbVie
- Title: Study of Intravenous (IV) ABBV-637 Alone or in Combination With IV Docetaxel/Osimertinib to Assess Adverse Events and Change in Disease Activity in Adult Participants With Relapsed/Refractory (R/R) Solid Tumors
- Duration: 2021-2026
- Compo investigator: Laurent Greillier
PIONeeR
- Registration: NCT03493581
- Partner: APHM, HalioDx, Innate Pharma, AMU
- Title: Precision Immuno-Oncology for Advanced Non-small Cell Lung Cancer Patients With PD-1 ICI Resistance
- Duration: 2018-2022
- Principal investigator: Laurent Greillier, Jean-Olivier Arnaud
ELDERLY
- Registration: NCT03977194
- Partner: Intergroupe Francophone de Cancerologie Thoracique
- Title: Atezolizumab in Elderly Patients With Advanced Non-Small-Cell Lung Cancer and Receiving Carboplatin Paclitaxel Chemotherapy
- Duration: 2019-2023
- Principal investigator: Elisabeth QUOIX, Céline MASCAUX
- Compo investigator: Laurent Greillier
NCT01817192
- Registration: NCT01817192
- Partner: Razor Genomics
- Title: Adjuvant Chemotherapy in Patients With Intermediate or High Risk Stage I or Stage IIA Non-squamous Non-Small Cell Lung Cancer
- Duration: 2020-2025
- Principal investigator: David R Spigel, Sarah Cannon
- Compo investigator: Laurent Greillier
SAVIMMUNE
- Registration: NCT04108026
- Partner: Intergroupe Francophone de Cancerologie Thoracique
- Title: Immunotherapy in Patient With Poor General Condition
- Duration: 2020-2023
- Principal investigator: Valérie GOUNANT, Michael DURUISSEAUX
- Compo investigator: Laurent Greillier
NCT04042558
- Registration: NCT04042558
- Partner: Centre Francois Baclesse
- Title: A Study Evaluating Platinum-Pemetrexed-Atezolizumab (+/-Bevacizumab) for Patients With Stage IIIB/IV Non-squamous Non-small Cell Lung Cancer With EGFR Mutations, ALK Rearrangement or ROS1 Fusion Progressing After Targeted Therapies
- Duration: 2019-2024
- Compo investigator: Laurent Greillier
NIPINEC
- Registration: NCT03591731
- Partner: Intergroupe Francophone de Cancerologie Thoracique, Federation Francophone de Cancerologie Digestive, GERCOR - Multidisciplinary Oncology Cooperative Group
- Title: Nivolumab +/- Ipilimumab in Patients With Advanced, Refractory Pulmonary or Gastroenteropancreatic Poorly Differentiated Neuroendocrine Tumors (NECs)
- Duration: 2019-2023
- Principal investigator: Nicolas GIRARD, Thomas WALTER
- Compo investigator: Laurent Greillier
RESILIENT
- Registration: NCT03088813
- Partner: Ipsen
- Title: Study of Irinotecan Liposome Injection (ONIVYDE®) in Patients With Small Cell Lung Cancer
- Duration: 2018-2022
- Compo investigator: Laurent Greillier
Canopy-A
- Registration: NCT03447769
- Partner: Novartis Pharmaceuticals
- Title: Study of Efficacy and Safety of Canakinumab as Adjuvant Therapy in Adult Subjects With Stages AJCC/UICC v. 8 II-IIIA and IIIB (T>5cm N2) Completely Resected Non-small Cell Lung
- Duration: 2018-2027
- Compo investigator: Laurent Greillier
NCT04350463
- Registration: NCT04350463
- Partner: Celgene
- Title: A Safety and Efficacy Study of CC-90011 in Combination With Nivolumab in Subjects With Advanced Cancers
- Duration: 2020-2024
- Compo investigator: Laurent Greillier
TROPION-LUNG01
- Registration: NCT04656652
- Partner: Daiichi Sankyo, Inc., AstraZeneca
- Title: Study of DS-1062a Versus Docetaxel in Previously Treated Advanced or Metastatic Non-small Cell Lung Cancer Without Actionable Genomic Alterations
- Duration: 2020-2024
- Compo investigator: Laurent Greillier
MERMAID-1
- Registration: NCT04385368
- Partner: AstraZeneca
- Title: Phase III Study to Determine the Efficacy of Durvalumab in Combination With Chemotherapy in Completely Resected Stage II-III Non-small Cell Lung Cancer (NSCLC)
- Duration: 2020-2026
- Principal investigator: Solange Peters, Charles Swanton
- Compo investigator: Laurent Greillier
NCT03840902
- Registration: NCT03840902
- Partner: EMD Serono Research and Development Institute, Inc., Merck KGaA
- Title: M7824 With cCRT in Unresectable Stage III Non-small Cell Lung Cancer (NSCLC)
- Duration: 2019-2023
- Compo investigator: Laurent Greillier
CARMEN-LC03
- Registration: NCT04154956
- Partner: Sanofi
- Title: SAR408701 Versus Docetaxel in Previously Treated, Carcinoembryonic Antigen-related Cell Adhesion Molecule 5 (CEACAM5) Positive Metastatic Non-squamous Non-small Cell Lung Cancer Patients
- Duration: 2020-2024
- Compo investigator: Laurent Greillier
SKYSCRAPER-03
- Registration: NCT04513925
- Partner: Hoffmann-La Roche
- Title: A Study of Atezolizumab and Tiragolumab Compared With Durvalumab in Participants With Locally Advanced, Unresectable Stage III Non-Small Cell Lung Cancer (NSCLC)
- Duration: 2020-2027
- Compo investigator: Laurent Greillier
NCT03899155
- Registration: NCT03899155
- Partner: Bristol-Myers Squibb
- Title: Pan Tumor Nivolumab Rollover Study
- Duration: 2019-2025
NCT03798535
- Registration: NCT03798535
- Partner: AstraZeneca
- Title: First Real-world Data on Unresectable Stage III NSCLC Patients Treated With Durvalumab After Chemoradiotherapy
- Duration: 2018-2023
- Compo investigator: Laurent Greillier
IMbrella A
- Registration: NCT03148418
- Partner: Hoffmann-La Roche
- Title: A Study in Participants Previously Enrolled in a Genentech- and/or F. Hoffmann-La Roche Ltd-Sponsored Atezolizumab Study
- Duration: 2017-2030
- Compo investigator: Laurent Greillier
BEAT-meso
- Registration: NCT03762018
- Partner: European Thoracic Oncology Platform, Hoffmann-La Roche
- Title: Bevacizumab and Atezolizumab in Malignant Pleural Mesothelioma
- Duration: 2019-2024
- Principal investigator: Enriqueta Felip, Sanjay Popat
- Compo investigator: Laurent Greillier
SPECTA
- Registration: NCT02834884
- Partner: European Organisation for Research and Treatment of Cancer - EORTC
- Title: Screening Cancer Patients for Efficient Clinical Trial Access
- Duration: 2017-2026
- Principal investigator: Vassilis Golfinopoulos
- Compo investigator: Laurent Greillier
TROPION-Lung05
- Registration: EudraCT 2020-002774-27
- Partner: Daiichi Sankyo, Inc.
- Title: Phase 2 Study of DS-1062a in Advanced or Metastatic Non-small Cell Lung Cancer with Actionable Genomic Alterations
- Starting year: 2021
- Compo investigator: Laurent Greillier
NeoCOAST
- Registration: EudraCT 2018-002932-26
- Partner: MedImmune, LLC, a wholly owned subsidiary of AstraZeneca PLC
- Title: Neoadjuvant Durvalumab Alone or in Combination with Novel Agents in Resectable Non-Small Cell Lung Cancer
- Duration: 2019 - 2021
- Compo investigator: Laurent Greillier
PIVOT-02
- Registration: EudraCT 2016-003543-11
- Partner: Nektar Therapeutics
- Title: A study to investigate the use of the study drug NKTR-214 in combination with other approved treatments for solid tumours
- Duration: 2016 - 2021
- Compo investigator: Laurent Greillier
SKYSCRAPER-06
- Registration: NCT04619797
- Partner: Hoffmann-La Roche
- Title: A Study of Tiragolumab in Combination With Atezolizumab Plus Pemetrexed and Carboplatin/Cisplatin Versus Pembrolizumab Plus Pemetrexed and Carboplatin/Cisplatin in Participants With Previously Untreated Advanced Non-Squamous Non-Small Cell Lung Cancer
- Duration: 2020 - 2025
- Compo investigator: Laurent Greillier
PERSEE
- Registration: EudraCT 2020-002626-86
- Partner: CHRU de Brest
- Title: A trial comparing the pembrolizumab platinum based chemotherapy combination with pembrolizumab monotherapy in first line treatment of non small-cell lung cancer (NSCLC) patients
- Starting year: 2020
- Principal investigator: Renaud Descourt, Chantal Decroisette, Christos Chouaid
- Compo investigator: Laurent Greillier
SAPPHIRE
- Registration: EudraCT 2019-001043-41
- Partner: Mirati Therapeutics, Inc.
- Title: A Randomized Phase 3 Study of Sitravatinib in Combination with Nivolumab Versus Docetaxel in Patients with Advanced Non-Squamous Non-Small Cell Lung Cancer with Disease Progression On or After Platinum-Based Chemotherapy and Checkpoint Inhibitor Therapy
- Starting year: 2020
- Compo investigator: Laurent Greillier
Nivothym
- Registration: EudraCT 2015-005504-28
- Partner: European Organisation for Research and Treatment of Cancer
- Title: Single-arm, multicenter, phase II study of immunotherapy in patients with type B3 thymoma and thymic carcinoma previously treated with chemotherapy
- Starting year: 2017
- Principal investigator: Nicolas Girard, Solange Peters
- Compo investigator: Laurent Greillier
9 Partnerships and cooperations
9.1 Visits of international scientists
Emilia Kozlowska
-
Status:
Post-doc
-
Institution of origin:
Silesian Institute of Technology
-
Country:
Poland
-
Dates:
October - December 2021
-
Context of the visit:
Scientific collaboration on prediction of metastasis in lung cancer
-
Mobility program/type of mobility:
EMBO scholarship
9.2 International collaborations
REZOLVE TRIAL
- Registration: ANZGOG11-01
- Partner: Sydney University, Australia
- Title: Phase 2 study of intraperitoneal Bevacizumab in patients with refractory ovarian cancer
- Funding: 40 k€
- Duration: 2020-2022
- Principal investigator: Sonia Yip (Sydney) - COMPO investigator: Joseph Ciccolini , Clémence Marin, Mourad Hamimed
TAX TRIAL
- Registration: ongoing
- Partner: EORTC, Belgium
- Title: Trastuzumab-deruxtecan in older patients with Her2+ breast cancer
- Funding: 62 k€
- Duration: 2022-2024
- Principal investigator: Hans Wielder (Luven) - COMPO investigator: Joseph Ciccolini , Mourad Hamimed
9.3 National initiatives
QUANTIC
-
Title:
QUANTitative modeling combined to statistical learning to understand and predict resistance to Immune-checkpoint inhibition in non-small cell lung Cancer
-
Partner Institutions:
- AP-HM, Marseille
- Inserm, Marseille
- Aix-Marseille University, Marseille
- Veracyte, Marseille
- InnatePharma, Marseille
-
Date/Duration:
2019 - 2022
-
Funding:
338 k€, Inserm Plan Cancer MIC
-
Principal investigator:
S. Benzekry
-
COMPO members involved:
L. Greillier, K. Atsou, P. Fleury, M. Karlsen.
PIONeeR - biomarkers
-
Title:
Precision Immuno-Oncology for advanced Non-small cell lung cancer patients with PD-1 ICI Resistance
-
Partner Institutions:
- AP-HM, Marseille
- 13 national cancer centers, including Centre Lyon Bérard and IUCT in Toulouse
- Marseille Immmunopôle (immuno-monitoring platform)
- Vasculo-monitoring platform of AP-HM
- Inserm
- Aix-Marseille University
- Veracyte, Marseille
- InnatePharma, Marseille
-
Date/Duration:
2018 - 2023
-
Funding:
8 M€, ANR -
Principal investigator:
F. Barlesi (IGR)
-
COMPO members involved:
S. Benzekry, J. Ciccolini, L. Greillier, K. Atsou, P. Fleury, M. Hamimed, C. Marin, M. Karlsen.
THERMONANO
-
Title:
Nanoassemblies for the subcutaneous self-administration of anticancer drugs
-
Partner Institution:
- Institut Galien Paris-Saclay (UMR CNRS 8612)
-
Date/Duration:
2019 - 2024
-
Funding:
1.8 M€, ERC
-
Principal investigator:
J. Nicolas (Institut Galien, Paris-Sud)
-
COMPO members involved:
A. Rodallec, S. Benzekry
PAIR PANCREAS
-
Title:
Determination of the sensitivity to anticancer drugs of pancreatic cancers: development of a platform for precision medicine based upon patients organoids.
-
Partner Institution:
- Pancreas Cancer Team (CRCM)
-
Date/Duration:
2019 - 2022
-
Funding:
500 K€, INCa
-
Principal investigator:
N. Dusetti (CRCM)
-
COMPO members involved:
J. Ciccolini, S. Elkaoutari
9.4 Regional initiatives
SMART project
- Partner: A*MIDEX
- Title: Optimizing pemetrexed-cisplatin-bevacizumab dosing and scheduling with anti-PD1 in NSCLC models
- Funding: 350 k€
- Duration: 2018-2022
- Principal investigator: Fabrice Barlesi (IGR) COMPO investigator: Joseph Ciccolini, Guillaume Sicard, Raphaelle Fanciullino
Cardiotoxicity of immuno-oncology drugs
- Partner: Maison du Coeur, PRT-K (INCa)
- Title: Myocardites induites par les immunothérapies anticancéreuses : approche translationnelle des mécanismes physiopathologiques et des facteurs pronostiques
- Funding: 47 k€ + 250 K€
- Duration: 2021-2023
- Principal investigator: Franck Thuny (APHM) COMPO investigator: Joseph Ciccolini, Raphaelle Fanciullino
ETP Program
- Partner: APHM
- Title: Therapeutic Education of the patient in oncology- hematology
- Funding: none (APHM own resources)
- Duration: 2018-present
- Principal investigator: Raphaelle Fanciullino
10 Dissemination
10.1 Promoting scientific activities
10.1.1 Scientific events: organisation
Member of the organizing committees
- A. Rodallec: CRS BNLF ECS Meeting, Avr. 2022
- J. Ciccolini: Journées du GPCO-Unicancer , Dijon, France Nov. 2021
- J. Ciccolini: PAMM-EORTC e-Meeting, January 2021
- J. Ciccolini: PAMM-EORTC Winter Meeting, Verona, Italy, April 2022.
10.1.2 Scientific events: selection
Member of the conference program committees
- A. Rodallec: CRS BNLF ECS Meeting, Avr. 2022
- J. Ciccolini: PAMM-EORTC e-Meeting, Janurary 2021
- J. Ciccolini: PAMM-EORTC Winter Meeting, Verona, Italy, April 2022.
- F. Gattacceca: Group of Metabolism and Pharmacokinetics Symposium, Paris, France, October 2021.
10.1.3 Journal
Member of the editorial boards
- S. Benzekry, Mathematical Biosciences
- A. Rodallec, Pharmaceutics Section "Pharmacokinetics and Pharmacodynamics" (MDPI)
- J. Ciccolini: Cancer Chemotherapy and Pharmacology (Springer)
- J. Ciccolini: Pharmaceuticals - Guest Editor special issue "Precision Medicine in Oncology: controlling the pharmacokinetics variability", 2021 (MDPI)
Reviewer - reviewing activities
- S. Benzekry: Scientific Reports
- A. Rodallec: ALTEX, Pharmaceutics, Journal of Pharmacy and Pharmacology
- J. Ciccolini: Cancer Chemotherapy and Pharmacology, Cancers, Pharmaceutics, Pharmaceuticals, Bone Marrow Transplant, British Journal of Clinical Pharmacology, Clinical Cancer Research, Clinical Pharmacokinetics, Scientific Reports, Investigational New Drugs, Expert Opinion on Drug Discovery, Pharmacogenomics, Britih Journal of Cancer, Annals of Oncology, Pharmaceutical Research
- Florence Gattacceca: Nanomedicine
10.1.4 Invited talks
- S. Benzekry. October, 2021. Seminar at the Center for Cancer Research of Marseille. Mechanistic learning : combining mechanistic modeling and statistical learning to predict outcome in clinical oncology. Marseille, France
- S. Benzekry. October, 2021. Multidisciplinary Approaches in Cancer Research. Sophia Antipolis, France
- S. Benzekry. June, 2021. Annual seminar of the regional Canceropole Provence-Alpes Cote d'Azur. COMPO: COMPutational pharmacology and clinical Oncology.
- S. Benzekry. June, 2021. Mini-symposium "Mathematical approaches to advance clinical studies in oncology", Society of Mathematical Biology annual Conference</a>.(online).
- S. Benzekry. May 2021, Seminar at the pharmacometrics group of Merck. COMPO - COMPutational pharmacology and clinical Oncology: Optimization of therapeutic strategies by mechanistic and statistical modeling.
- S. Benzekry. Apr 2021, Seminar of the Pharma - Informatics Unit of the Athena research group, Athens, Greece. COMPO - COMPutational pharmacology and clinical Oncology.
- S. Benzekry. Mar 2021, Applied statistics seminar of the mathematics department. Marseille, France. COMPO - COMPutational pharmacology and clinical Oncology.
- S. Benzekry. Jan 2021, Biomathematics seminar of the mathematics department. Marseille, France. COMPO - COMPutational pharmacology and clinical Oncology.
- J. Ciccolini. December 2021, Annual Congress of Hospital Pharmacists, Porto, Portugal : PK/PD of immune checkpoint inhibitors : any room for pharmacometrics ?
- J. Ciccolini. November, 2021, GPCO-Unicancer, Dijon France : Spécificités PK/PD des inhibiteurs de BRAF et utilité du Suivi Thérapeutique Pharmacologique.
- J. Ciccolini. November, 2021, Incyte Symposium, Marseille France : Médecine de précision et thérapies ciblées orales en hématologie.
- J. Ciccolini. January 2021, Esai Symposium (Webinar) : Pharmacokinetics of antibody-drug conjugates in oncology.
- J. Ciccolini. October 2021, Société Française des Pharmaciens Oncologues, Nantes, France : Variabilité des anticorps monoclonaux thérapeutiques, quelles répercussions pour le patient ?
- J. Ciccolini. September 2021, International Congress of Therapeutic Drug Monitoring and clinical toxicology, Roma, Italy: Pharmacometrics of biologics in oncology: any room for Therapeutic Drug Monitoring.
- J. Ciccolini. July 2021, Canceropole PACA Annual symposium, St Raphael France: Unlocking the potential of ADCs for HER2 signaling pathway.
- F. Gattacceca. Sept 2021, webinar of SFNano (French Society of Nanomedicine): nanos in Marseille, France: Pharmacokinetic challenges for an optimized nanomedicine development: specific quantitation methods and physiologically-based pharmacokinetic modelling
- F. Gattacceca. Nov 2021, ANSM: Introduction to PBPK modeling
10.1.5 Leadership within the scientific community
- S. Benzekry: Expert of the national multi-thematic institute (ITMO) Cancer of Aviesan
- S. Benzekry: Expert in the national reflexion group for in silico pharmacology
- J. Ciccolini: Board Member of the PAMM EORTC group
- J.Ciccolini: Board Member of the GPCO-Unicancer group
- J.Ciccolini: Board Member of the Task Force at Immuno-Onco-Unicancer group
- J.Ciccolini: Board Member of the Task Force at Head and Neck cancer- Unicancer group
- J.Ciccolini: Expert of the national Technology for Health Theme at Inserm.
- F. Gattacceca: Elected member of the GMP board (Group of Metabolism and Pharmacokinetics) from 2016 to 2021. Secretary in 2019, President in 2020
- R.Fanciullino: Board Member of the Clinical Pharmacy Group of the Societe Francaise des Pharmaciens Oncologues (SFPO).
- F. Gattacceca: Elected member of the GEPK board (Group of Teachers in Pharmacokinetics) since its creation in 2015
10.1.6 Scientific expertise
- S. Benzekry: Reviewer for the Inria - Inserm 2021 PhD committee
- S. Benzekry: Reviewer for the ANR
- J. Ciccolini: member of the INCa CLIP2 “Innovative Drugs” scientific committee, Boulogne Billancourt France
- J. Ciccolini: member of the INCa CLIP2 “New Combination” scientific committee, Boulogne Billancourt France
- J. Ciccolini: member of the ARC CN5 scientific committee, Villejuif, France
- J. Ciccolini: reviewer for the University of Sydney Ph.D. defense , Australia
- J. Ciccolini: reviewer for the University of Oxford Children's Cancer and Leukaemia Group grant application, England
- J. Ciccolini: reviewer for the Canceropole Grand Sud Ouest grants applications
- J. Ciccolini: reviewer for the Canceropole IdF grants applications
- J. Ciccolini: reviewer for the Canceropole CLARA grants applications
- J. Ciccolini: Expert at the national workgroup "DPD deficiency" at the Inca-ANSM-HAS steering committee, Paris France
- R. Fanciullino: reviewer for CIVIS grants applications.
- L. Greillier: reviewer for INCa and GIRCI Méditerranée; reviewer for scientific journals: Respir Med Res, Lung Cancer JTO Clin Cancer Reports, Rev Mal Respir
- F. Gattacceca: Expert at ANSM (National Drug Agency) in the “Security and quality of drugs” committee
10.1.7 Research administration
- A. Rodallec: Website and Social Media coordinator for the CRS benelux Local Chapter
- J. Ciccolini: secretary to the PAMM EORTC European group
10.2 Teaching - Supervision - Juries
10.2.1 Teaching
- Master S. Benzekry: M2 Pharmacokinetics of Aix-Marseille University (20h).
- DESU S. Benzekry: "Advances courses in pharmacometrics" (10h).
- A. Rodallec: lectures in MSc in Pharmacokinetics, MSc in Digipharm, MSc in Innovative Diagnostic and therapeutic Drug Products, DESU "Advances courses in pharmacometrics", DES in animal experiments, Pharm.D. studies (2d, 3d, 4th and 6th year), -> 190 h a year + additional lectures at University of Paris Saclay.
- J. Ciccolini: Founder and Head, Digipharm MSc degree (Aix Marseille Univ). Lectures at Aix Marseille univ in: MSc (2d year) in Oncology, MSc (2d year) in Oncogenetics, MSc (2d year) in Pharmacokinetics, MSc (2d year) in Digipharm, MSc (1st year) in Drugs & Health Products, D.U. in Animal Experiments, D.U. in Genetic Councelling, Master Class in Lung Cancer, Pharm.D. studies (2d, 3d, 4th and 6th year) -> 150 h a year + additional lectures in pharmacokinetics at Université Catholique de Lyon, University of Amsterdam NL and University of Verona Italy.
- R. Fanciullino: Lectures in: MSc (2d year) in Pharmacokinetics, MSc (2d year) in Digipharm, CESU in Oncogeriatry, DES in PK Variability, Pharm.D. studies (3d, 4th and 5th year) -> 190 h a year .
- L. Greillier: M2 Recherche clinique et Simulation en Santé.
- L. Greillier: oncology and pulmonology for 3rd – 11 year medical students.
- F. Gattacceca: Director of the Master Program “Pharmacokinetics”.
- F. Gattacceca: Aix-Marseille University school of pharmacy (260h), teaching in other universities (Nîmes, Angers, Montpellier): 90% at a post-graduate level.
- F. Gattacceca: Director of two international University Diplomas: “Modeling and simulation: population approaches in pharmacokinetics/pharmacodynamics” and “Modeling and simulation: physiologically-based pharmacokinetic modeling for pharmacology and toxicology” (the latter created in 2021: first session in January-May 2022).
10.2.2 Supervision
- Postdoc: K. Atsou, 2021 - 2022, QUANTIC, supervision S. Benzekry
- PhD: C. Bigarré, 2020 - 2023, Mathematical modeling for prediction of metastatic relapse in breast cancer, supervision S. Benzekry, X. Muracciole
- PhD: Mélanie Donnette, 2018-2021: Pharmacogenetics and Pharmacokinetics of cytarabine in AML patients. Supervision: R. Fanciullino and J. Ciccolini
- PhD: Guillaume Sicard, 2020-2023: optimization of the combination between cytotoxics, anti-VEGF and anti-PD1 in NSCLC models. Supervision: R. Fanciullino and J. Ciccolini
- PhD: Mathilde Dacos, 2021-2024: Nanimmuno: immunomodulating properties of immunoliposomes in breast cancer models. Supervision: R. Fanciullino
- PhD: Clémence Marin, 2018-2022: Bioanalytical Development and PK studies of mabs in oncology. Supervision: J. Ciccolini
- PhD: Florent Ferrer, 2019-2021: Precision medicine with oral target therapies in mRCC patients. Supervision: J. Ciccolini
- Engineer: M. Karlsen, 2021 - 2023, QUANTIC, supervision S. Benzekry
- Engineer: M. Hamimed 2021-2022 (PIONeeR project), supervision J. Ciccolini
- PhD: Anna Chan-Kwong, 2019-2021, supervision F. Gattacceca, S. Khier;
- PhD: Jessica Ou, 2021-2024, supervision F. Gattacceca, P. Garrigue
- PhD: Anthéa Deschamps (2019-2023), supervision F. Gattacceca, R. Guilhaumou
- Master 2: Athina Marcos (5th year pharmacy, host: ANSM, March-July 2021), supervision P. Houeto, F. Gattacceca
- Master 2: Jessica Ou (host: CERIMED, January-June 2021), supervision P. Garrigue, F. Gattacceca
- Master 2: Benoit Immordino (Nanimmuno Project, 2021), supervision R. Fanciullino
- Master 2: Loic Osanno (Vidaza project 2021-2022), supervision R. Fanciullino
- Master 2: Alexis Plan (Predicting MTX toxicity in Neuro-Oncology 2021-2022), supervision J.Ciccolini
- Master 1: Moussa Ndiaye, Tornado (May-June 2021), supervision F. Gattacceca
- Master 1: Céline Delansay, Thermonano (May-June 2021), supervision F. Gattacceca
10.2.3 Juries
- S. Benzekry: reviewer of the PhD of Maximilian Strobl, Oxford University
- J. Ciccolini: reviewer for the PhD of Emilie Molins, U Lyon France Dec 2021
- J. Ciccolini, reviewer for the PhD of Jeffry Adiwidjaja, U Sydney Australia Sept 2021
- J. Ciccolini, reviewer for the Pharm.D. thesis of 10-12 students/year..
- R. Fanciullino, reviewer for the PhD of Fanny Lenhardt, Université de Montpellier June 2021
- A. Rodallec, reviewer for the PhD of Corentin Franco, Aix Marseille Univ. Jan 2021
- A. Rodallec, reviewer for the Pham.D. thesis of 3-5 students/year
- R. Fanciullino, reviewer for the Pharm.D. thesis of 8-10 students/year
- L. Greillier: reviewer of the “HDR” of Florian Guisier, Université de Rouen Normandie
- F. Gattacceca: HDR examiner for S. Khier, Montpellier University, June 2021
- F. Gattacceca: director for the PharmD of C. Perret, AMU, Dec 2021,
- F. Gattacceca: reviewer for the PharmD of L. Mioux, University Lyon 1, Sept 2021
- F. Gattacceca: reviewer for the PharmD of R. Garreau, University Lyon 1, Sept 2021
10.3 Popularization
10.3.1 Articles and contents
- S. Benzekry: recording of a podcast for Intestices.
- R. Fanciullino: recording of a podcast for the Société Française de Pharmacie Clinique (SFPC).
- A. Rodallec: interview for "Sciences & Mines", Nancy.
- J. Ciccolini: Podcast "Le métier de Pharmacien-chercheur" AMUpod Marseille.
- J. Ciccolini: Podcast OncLive "Residual concentrations of cetuximab in head and neck cancer" Washington DC USA.
10.3.2 Education
- F. Gattacceca: Organizer and Chair of the Student Webinar of the GMP: “Les métiers de la PK”, May 2021.
- A. Rodallec: Round-table "Pharmaceutical Research and Development" at the "Forum des métiers de la pharmacie", School of Pharmacy of Marseille 2018.
- A. Rodallec: France-Poland TWINET, Conference "Nanoparticles in oncology" school of Pharmacy of Marseille 2018.
- J. Ciccolini: Booth and animation "Les métiers de la pharmacie” at the Forum Métiers de la Santé, School of Medicine of Marseille 2021.
- D. Barbolosi: Booth and animation "La modélisation mathématique au service de la médecine” at the Forum Fête de la Science, Ajaccio 2021.
- D. Barbolosi: Booth and animation "Maths & Cancer” at the Forum des Mathématiques, Marseille 2021.
11 Scientific production
11.1 Major publications
- 1 articleComputational oncology — mathematical modelling of drug regimens for precision medicine.Nature Reviews Clinical Oncology134April 2016, 242-254
- 2 articleArtificial Intelligence and Mechanistic Modeling for Clinical Decision Making in Oncology.Clinical Pharmacology and TherapeuticsJune 2020
- 3 articleMathematical modeling of tumor-tumor distant interactions supports a systemic control of tumor growth.Cancer Research2017
- 4 articleModeling Spontaneous Metastasis following Surgery: An In Vivo-In Silico Approach.Cancer Research763February 2016, 535 - 547
- 5 articlePharmacokinetics and Pharmacodynamics-Based Mathematical Modeling Identifies an Optimal Protocol for Metronomic Chemotherapy.Cancer Research77172017, 4723-4733
- 6 articleWomen at a Disadvantage in Fluorouracil Treatment.JAMA oncology26June 2016, 829
- 7 articleA phase Ia/Ib clinical trial of metronomic chemotherapy based on a mathematical model of oral vinorelbine in metastatic non-small cell lung cancer and malignant pleural mesothelioma: rationale and study protocol.BMC Cancer161December 2016, 278
- 8 articleCDA as a predictive marker for life-threatening toxicities in patients with AML treated with cytarabine.Blood Advances25February 2018, 462 - 469
- 9 articleTowards Rational Cancer Therapeutics: Optimizing Dosing, Delivery, Scheduling, and Combinations.Clinical Pharmacology and Therapeutics1083September 2020, 458-470
- 10 articleMachine Learning and Mechanistic Modeling for Prediction of Metastatic Relapse in Early-Stage Breast Cancer.JCO Clinical Cancer Informatics4September 2020, 259-274
11.2 Publications of the year
International journals
- 11 articleA size and space structured model of tumor growth describes a key role for protumor immune cells in breaking equilibrium states in tumorigenesis.PLoS ONE1611November 2021, e0259291
- 12 articleMulticenter phase II trial of nintedanib plus docetaxel in second-line treatment in advanced non-squamous non-small cell lung cancer patients refractory to first-line platin-based chemotherapy (REFRACT GFPC 02–15 study).Lung Cancer161November 2021, 122-127
- 13 articleA prospective behavioral and imaging study exploring the impact on long-term memory of radiotherapy delivered for a brain tumor in childhood and adolescence.Clinical and Translational Radiation Oncology33March 2022, 7-14
- 14 articleMachine Learning for Prediction of Immunotherapy Efficacy in Non-Small Cell Lung Cancer from Simple Clinical and Biological Data.Cancers1324December 2021, 6210
- 15 articleDevelopment and Validation of a Prediction Model of Overall Survival in High-Risk Neuroblastoma Using Mechanistic Modeling of Metastasis.JCO Clinical Cancer Informatics5January 2021, 81-90
- 16 articleUnraveling the complexity of therapeutic drug monitoring for monoclonal antibody therapies to individualize dose in oncology.Pharmacology Research & Perspectives92April 2021
- 17 articleMulticentric phase II trial of TI‐CE high‐dose chemotherapy with therapeutic drug monitoring of carboplatin in patients with relapsed advanced germ cell tumors.Cancer Medicine107April 2021, 2250-2258
- 18 articleDetecting DPD deficiency: when perfect is the enemy of good.Cancer Chemotherapy and Pharmacology875May 2021, 717-719
- 19 articleExperimental and computational modeling for signature and biomarker discovery of renal cell carcinoma progression.Molecular Cancer201December 2021
- 20 articleImpact of care pathway for nursing home residents treated for cancer: ONCO-EHPAD study.Supportive Care in Cancer297July 2021, 3933-3942
- 21 articleUse and impact of the G8 score in older patients with thoracic and lung cancers.European Geriatric Medicine125October 2021, 1095-1100
- 22 articleFunctional status in older patients with cancer.Journal of Geriatric Oncology131January 2022, 40-45
- 23 articleOlder Patients Treated for Lung and Thoracic Cancers: Unplanned Hospitalizations and Overall Survival.Clinical Lung Cancer223May 2021, e405-e414
- 24 articleOctogenarians treated for thoracic and lung cancers: Impact of comprehensive geriatric assessment.Journal of Geriatric Oncology123April 2021, 402-409
- 25 articleOutcome of Stage IV Completely Necrotic Wilms Tumour and Local Stage III Treated According to the SIOP 2001 Protocol.Cancers135March 2021, 976
- 26 articleHigh‐dose chemotherapy followed by autologous stem cell rescue in Wilms tumors: French report on toxicity and efficacy.Pediatric Blood and CancerNovember 2021
- 27 articleFirst-line pembrolizumab with or without platinum doublet chemotherapy in non-small-cell lung cancer patients with PD-L1 expression ≥50%.Future Oncology1723August 2021, 3007-3016
- 28 articlePopulation pharmacokinetic model of irinotecan and its four main metabolites in patients treated with FOLFIRI or FOLFIRINOX regimen.Cancer Chemotherapy and Pharmacology882August 2021, 247-258
- 29 articleMechanistic models for hematological toxicities: Small is beautiful.CPT: Pharmacometrics and Systems Pharmacology105May 2021, 396-398
- 30 articleHigh incidence of CDA deficiency in patients with hematological malignancies: perspectives and therapeutic implications.Annals of Oncology325May 2021, 684-686
- 31 articlePharmacokinetics and pharmacogenetics of liposomal cytarabine in AML patients treated with CPX-351.Journal of Controlled Release338October 2021, 244-252
- 32 articleCOVID-19 vaccine race: watch your step for cancer patients.British Journal of Cancer1245March 2021, 860-861
- 33 articleClinical-Based vs. Model-Based Adaptive Dosing Strategy: Retrospective Comparison in Real-World mRCC Patients Treated with Sunitinib.Pharmaceuticals146June 2021, 494
- 34 articleStereotactic Body Radiotherapy for Frail Patients with Primary Renal Cell Carcinoma: Preliminary Results after 4 Years of Experience.Cancers1313July 2021, 3129
- 35 articlePharmacokinetics of oral vinorelbine in French children with recurrent or progressive primary low‐grade glioma.British Journal of Clinical PharmacologyOctober 2021
- 36 articleSuccessful Lung Cryoablation for a Bulky Lung Carcinoma.JVIR: Journal of Vascular and Interventional Radiology324April 2021, 610
- 37 articleEfficacy and Safety of Rovalpituzumab Tesirine Compared With Topotecan as Second-Line Therapy in DLL3-High SCLC: Results From the Phase 3 TAHOE Study.Journal of Thoracic Oncology169September 2021, 1547-1558
- 38 articleMetabolomic profiling of pancreatic adenocarcinoma reveals key features driving clinical outcome and drug resistance.EBioMedicine66April 2021, 103332
- 39 articleAssessment of nivolumab in HIV-Infected patients with advanced non-small cell lung cancer after prior chemotherapy. The IFCT-1602 CHIVA2 phase 2 clinical trial.Lung Cancer158August 2021, 146-150
- 40 articleA Phase 1–2 Study of Rovalpituzumab Tesirine in Combination With Nivolumab Plus or Minus Ipilimumab in Patients With Previously Treated Extensive-Stage SCLC.Journal of Thoracic Oncology169September 2021, 1559-1569
- 41 articleCross-Validation of a Multiplex LC-MS/MS Method for Assaying mAbs Plasma Levels in Patients with Cancer: A GPCO-UNICANCER Study.Pharmaceuticals148August 2021, 796
- 42 articleDiscovery and 3D imaging of a novel ΔNp63-expressing basal cell type in human pancreatic ducts with implications in disease.Gut2021
- 43 articleThe association between adverse events and outcome under checkpoint inhibitors: Where is the deal?Translational Oncology141January 2021, 100952
- 44 articleTreatment strategies for thymic carcinoma in a real-life setting. Insights from the RYTHMIC network.European Journal of Cancer162February 2022, 118-127
- 45 articleMask-Based versus Frame-Based Gamma Knife ICON Radiosurgery in Brain Metastases: A Prospective Randomized Trial.Stereotactic and Functional NeurosurgeryDecember 2021, 1-9
- 46 articleCould daratumumab induce the maturation of plasmablasts in Plasmablastic lymphoma?—Potential therapeutic applications.European Journal of Haematology1064April 2021, 589-592
- 47 articleSuccessful treatment with adapted high dose methotrexate in a hemodialysis patient with primary central nervous system lymphoma: 100 mg/m2 seems sufficient.NefrologiaAugust 2021
- 48 articleAsymptotic analysis of a biphase tumor fluid flow: the weak coupling case..Applied Mathematics and Computation413January 2022, 126635
- 49 articleCirculating tumor DNA in advanced non-small-cell lung cancer patients with HIV is associated with shorter overall survival: Results from a Phase II trial (IFCT-1001 CHIVA).Lung Cancer157July 2021, 124-130
Other scientific publications
- 50 inproceedingsMachine Learning and Mechanistic Modeling for the prediction of Overall Survival on the basis of 1st line Tumor Dynamics in Head and Neck Squamous Cell Carcinoma.Colloque Science numériques et intelligence Artificielle pour la Santé, AMUMarseille, FranceNovember 2021
- 51 inproceedingsMachine learning for prediction of immunotherapy efficacy in non-small cell lung cancer from simple clinical and biological data.Colloque Sciences numériques et Intelligence Artificielle pour la Santé à Aix-Marseille UniversitéMarseille, FranceNovember 2021
- 52 inproceedingsPharmacokinetics and oral absorption of different lipid nanocapsules: new quantitative FRET technique revealing PEGylation increasing the particle’s clearance.GMP 2021 SymposiumParis, FranceOctober 2021
- 53 inproceedingsPopulation pharmacokinetic modelling of intravenous LNCs FRET in rat.GMP 2021 SymposiumParis, FranceOctober 2021
- 54 miscLooking for new broad spectrum enterovirus inhibitor.July 2021
- 55 inproceedingsCefotaxime dosage optimization in intensive care patients by population pharmacokinetic analysis.GMP 2021 SymposiumParis, FranceOctober 2021
- 56 miscPharmacokinetic studies and modeling to efficiently predict nanoradiotracer PET biodistribution.September 2021
11.3 Other
Educational activities
- 57 unpublishedQuantitative modeling of metastasis: cancer at the organism scale.June 2021, DoctoralUnited States
11.4 Cited publications
- 58 articleExploiting Cancer’s Tactics to Make Cancer a Manageable Chronic Disease.Cancers1266Jun 2020, 1649
- 59 articleFrom patient-specific mathematical neuro-oncology to precision medicine..Front Oncol3AprilJan 2013, 62–62
- 60 articleComputational Modelling of Metastasis Development in Renal Cell Carcinoma.PLoS Comput Biol111100007Nov 2015, e1004626
- 61 articleDetermination of the unmetabolized 18F-FDG fraction by using an extension of simplified kinetic analysis method: clinical evaluation in paragangliomas.Medical & biological engineering & computing541Jan 2016, 103–111
- 62 articleOncotarget829Jul 2017, 47161–47166
- 63 articleGlobal Dormancy of Metastases Due to Systemic Inhibition of Angiogenesis.PLoS ONE9100019Jan 2014, e84249-11
- 64 articleMathematical Modeling of Tumor-Tumor Distant Interactions Supports a Systemic Control of Tumor Growth..Cancer Research7718Sep 2017, 5183–5193
- 65 articleModeling Spontaneous Metastasis following Surgery: An In Vivo-In Silico Approach.Cancer Research76300000Feb 2016, 535–547
- 66 articleProgress and Opportunities to Advance Clinical Cancer Therapeutics Using Tumor Dynamic Models..Clinical cancer research : an official journal of the American Association for Cancer ResearchDec 2019, 1–22
- 67 articleStan: A Probabilistic Programming Language.Journal of Statistical Software761Jan 2017, 1–32
- 68 articleEmergence of drug tolerance in cancer cell populations: an evolutionary outcome of selection, nongenetic instability, and stress-induced adaptation.Cancer Research756Mar 2015, 930–939
- 69 articleMechanistic Learning for Combinatorial Strategies With Immuno-oncology Drugs: Can Model-Informed Designs Help Investigators?JCO Precision Oncology4May 2020, 486–491
- 70 articleCombinatorial immunotherapy strategies: most gods throw dice, but fate plays chess.Annals of Oncology3011Nov 2019, 1690–1691
- 71 articleRealistic simulation of the 3-D growth of brain tumors in MR images coupling diffusion with biomechanical deformation.IEEE Transactions on Medical Imaging2410Oct 2005, 1334–1346
- 72 articleA new methodology to derive 3D kinetic parametric FDG PET images based on a mathematical approach integrating an error model of measurement.EJNMMI Research8Nov 2018, URL: https://www.ncbi.nlm.nih.gov/pmc/articles/PMC6238015/
- 73 articlePrediction of the Evolution of Thyroidal Lung Nodules Using a Mathematical Model..82Jan 2010, 37–38
- 74 articleA multi-layered systems approach for renal cell carcinoma.bioRxivJan 2020, 2020.01.13.904235
- 75 articleConvergence of a stochastic approximation version of the EM algorithm.The Annals of Statistics271Zbl: 0932.62094Mar 1999, 94–128
- 76 articleA 2/1 Sunitinib Dosing Schedule Provides Superior Antitumor Effectiveness and Less Toxicity Than a 4/2 Schedule for Metastatic Renal Cell Carcinoma: A Systematic Review and Meta-Analysis.Frontiers in Oncology102020, 313
- 77 articleUsing the SAEM algorithm for mechanistic joint models characterizing the relationship between nonlinear PSA kinetics and survival in prostate cancer patients.Biometrics731Mar 2017, 305–312
- 78 articleSimultaneous determination of cytosine arabinoside and its metabolite uracil arabinoside in human plasma by LC-MS/MS: Application to pharmacokinetics-pharmacogenetics pilot study in AML patients.Journal of Chromatography B - Analytical Technologies in the Biomedical and Life Sciences1126-1127September 2019, 121770
- 79 articleNumerical solution of an inverse problem in size-structured population dynamics.Inverse Problems254Apr 2009, 045008–045008
- 80 articleA phase Ia/Ib clinical trial of metronomic chemotherapy based on a mathematical model of oral vinorelbine in metastatic non-small cell lung cancer and malignant pleural mesothelioma: rationale and study protocol.BMC cancer161000002016, 278
- 81 articleIn vitro and in vivo reversal of resistance to 5-fluorouracil in colorectal cancer cells with a novel stealth double-liposomal formulation.British Journal of Cancer977Oct 2007, 919–926
- 82 articleA simple and rapid liquid chromatography-mass spectrometry method to assay cabozantinib in plasma: Application to therapeutic drug monitoring in patients with renal cell carcinoma.Journal of Chromatography B - Analytical Technologies in the Biomedical and Life Sciences1138February 2020, 121968
- 83 articleMethodological aspects and pharmacological applications of three-dimensional cancer cell cultures and organoids.Life Sciences254Aug 2020, 117784
- 84 articlePembrolizumab plus Chemotherapy in Metastatic Non-Small-Cell Lung.Cancer. N Engl J Med3782078–20922018
- 85 articleThe evolutionary history of lethal metastatic prostate cancer.Nature5207547Apr 2015, 353–357
- 86 articleThe elements of statistical learning: data mining, inference, and prediction, Springer Series in Statistics.2009
- 87 articleRevisiting dosing regimen using PK/PD modeling: the MODEL1 phase I/II trial of docetaxel plus epirubicin in metastatic breast cancer patients.Breast Cancer Research and Treatment1562Mar 2016, 331–341
- 88 articleDevelopment of ipilimumab: contribution to a new paradigm for cancer immunotherapy.Seminars in Oncology375Oct 2010, 533–546
- 89 articleCalibrating a Predictive Model of Tumor Growth and Angiogenesis with Quantitative MRI.Annals of Biomedical Engineering477Jul 2019, 1539–1551
- 90 articleSingle-agent paclitaxel for the treatment of breast cancer: an overview.Seminars in Oncology231 Suppl 1Feb 1996, 4–9
- 91 articleQuantitative evidence for early metastatic seeding in colorectal cancer.Nature Genetics5177Jul 2019, 1113–1122
- 92 articleRevisiting Bevacizumab + Cytotoxics Scheduling Using Mathematical Modeling: Proof of Concept Study in Experimental Non-Small Cell Lung Carcinoma..CPT: Pharmacometrics & Systems Pharmacology71Jan 2018, 42–50
- 93 articleA Dynamical Model for the Growth and Size Distribution of Multiple Metastatic Tumors.Journal of Theoretical Biology2032Mar 2000, 177–186
- 94 articleDynamic Risk Profiling Using Serial Tumor Biomarkers for Personalized Outcome Prediction.Cell1783Jul 2019, 699-713.e19
- 95 bookMixed Effects Models for the Population Approach.CRC PressJul 2014
- 96 articleNonlinear mixed effects models for repeated measures data..Biometrics463Sep 1990, 673–687
- 97 articleA phase II randomized trial of nivolumab with stereotactic body radiotherapy (SBRT) versus nivolumab alone in metastatic (M1) head and neck squamous cell carcinoma (HNSCC)..Journal of Clinical Oncology3615_supplMay 2018, 6009–6009
- 98 articleRevisiting Dosing Regimen Using Pharmacokinetic/Pharmacodynamic Mathematical Modeling: Densification and Intensification of Combination Cancer Therapy.Clinical Pharmacokinetics55800000Aug 2016, 1015–1025
- 99 articleModel driven optimization of antiangiogenics + cytotoxics combination: application to breast cancer mice treated with bevacizumab + paclitaxel doublet leads to reduced tumor growth and fewer metastasis.Oncotarget814Feb 2017, 23087–23098
- 100 articleIn Vivo Bioluminescence Tomography for Monitoring Breast Tumor Growth and Metastatic Spreading: Comparative Study and Mathematical Modeling.Scientific Reports600000Nov 2016, 36173
- 101 articleIntegrating microarray-based spatial transcriptomics and single-cell RNA-seq reveals tissue architecture in pancreatic ductal adenocarcinomas.Nature Biotechnology3833Mar 2020, 333–342
- 102 articleAvelumab plus Axitinib versus Sunitinib for Advanced Renal-Cell Carcinoma.New England Journal of Medicine38012Mar 2019, 1103–1115
- 103 phdthesisMathematical modelling of neoadjuvant antiangiogenic therapy and prediction of post-surgical metastatic relapse in breast cancer patients.Université de BordeauxOct 2019, URL: https://tel.archives-ouvertes.fr/tel-02560543
- 104 articleMatrix metalloproteinases at cancer tumor–host interface.Seminars in Cell & Developmental Biology191Feb 2008, 52–60
- 105 articleAn alternative parameter for early forecasting clinical response in NSCLC patients during radiotherapy: proof of concept study.The British Journal of Radiology891062Jun 2016, 20160061
- 106 articleFrom 3D spheroids to tumor bearing mice: efficacy and distribution studies of trastuzumab-docetaxel immunoliposome in breast cancer.International Journal of Nanomedicine132018, 6677–6688
- 107 articleDefining the optimal murine models to investigate immune checkpoint blockers and their combination with other immunotherapies.Annals of Oncology: Official Journal of the European Society for Medical Oncology2772016, 1190–1198
- 108 articleOptimal Scheduling of Bevacizumab and Pemetrexed/cisplatin Dosing in Non-Small Cell Lung Cancer.CPT: pharmacometrics & systems pharmacologyApr 2019
- 109 articleAtezolizumab for First-Line Treatment of Metastatic Nonsquamous NSCLC..New England Journal of Medicine37824Jun 2018, 2288–2301
- 110 articleComprehensive analysis of the clinical immuno-oncology landscape..Ann. Oncol.291Jan 2018, 84–91
- 111 phdthesisMathematical modeling of antibody nanoconjugates transport in tumors.Bordeaux UniversityDec 2020
- 112 articlePREDICT: a new UK prognostic model that predicts survival following surgery for invasive breast cancer..Breast cancer research121Jan 2010, R1