Keywords
Computer Science and Digital Science
- A5.1.1. Engineering of interactive systems
- A5.1.2. Evaluation of interactive systems
- A5.1.4. Brain-computer interfaces, physiological computing
- A5.1.5. Body-based interfaces
- A5.1.6. Tangible interfaces
- A5.1.7. Multimodal interfaces
- A5.3.3. Pattern recognition
- A5.4.1. Object recognition
- A5.4.2. Activity recognition
- A5.7.3. Speech
- A5.8. Natural language processing
- A5.10.5. Robot interaction (with the environment, humans, other robots)
- A5.10.7. Learning
- A5.10.8. Cognitive robotics and systems
- A5.11.1. Human activity analysis and recognition
- A6.3.1. Inverse problems
- A9. Artificial intelligence
- A9.2. Machine learning
- A9.5. Robotics
- A9.7. AI algorithmics
Other Research Topics and Application Domains
- B1.2.1. Understanding and simulation of the brain and the nervous system
- B1.2.2. Cognitive science
- B5.6. Robotic systems
- B5.7. 3D printing
- B5.8. Learning and training
- B9. Society and Knowledge
- B9.1. Education
- B9.1.1. E-learning, MOOC
- B9.2. Art
- B9.2.1. Music, sound
- B9.2.4. Theater
- B9.6. Humanities
- B9.6.1. Psychology
- B9.6.8. Linguistics
- B9.7. Knowledge dissemination
1 Team members, visitors, external collaborators
Research Scientists
- Pierre-Yves Oudeyer [Team leader, Inria, Senior Researcher, HDR]
- Natalia Diaz Rodriguez [École Nationale Supérieure de Techniques Avancées, Researcher, until May 2021]
- Cécile Mazon [Univ de Bordeaux, Researcher, until Feb 2021]
- Clément Moulin-Frier [Inria, Researcher]
- Mai Nguyen [École Nationale Supérieure de Techniques Avancées, Researcher, until Nov 2021]
Faculty Members
- Helene Sauzeon [Team leader, Univ de Bordeaux, Professor, HDR]
- David Filliat [École Nationale Supérieure de Techniques Avancées, Professor, HDR]
- Cécile Mazon [Univ de Bordeaux, Associate Professor, from Mar 2021]
Post-Doctoral Fellows
- Eric Meyer [Inria, from Sep 2021]
- Eleni Nisioti [Inria]
- Masataka Sawayama [Inria]
- Guillermo Jorge Valle Perez [Inria, from Apr 2021]
PhD Students
- Rania Abdelghani [Evidenceb]
- Maxime Adolphe [Onepoint]
- Thomas Carta [Univ de Bordeaux, from Oct 2021]
- Hugo Caselles-Dupre [Softbank Robotics, until Feb 2021]
- Cedric Colas [Inria, until Jun 2021]
- Mayalen Etcheverry [Poietis]
- Tristan Karch [Inria]
- Vyshakh Palli Thaza [École Nationale Supérieure de Techniques Avancées, until Feb 2021]
- Remy Portelas [Inria]
- Thomas Rojat [Renault, CIFRE]
- Isabeau Saint-Supery [Univ de Bordeaux, from Sep 2021]
- Julius Taylor [Inria]
- Alexandr Ten [Inria]
- Maria Teodorescu [Inria]
- Guillermo Jorge Valle Perez [Inria, until Mar 2021]
Technical Staff
- Florence Carton [École Nationale Supérieure de Techniques Avancées, Engineer, until Apr 2021]
- Benjamin Clément [Inria, Engineer]
- Grgur Kovac [Inria, Engineer]
- Clement Romac [Inria, Engineer]
- Didier Roy [Inria, Engineer]
Interns and Apprentices
- Maxime Balan [Inria, from Feb 2021 until Jul 2021]
- Clea Gardin [Inria, from Jun 2021 until Jul 2021]
- Paul Germon [Inria, from Feb 2021 until Jul 2021]
- Gautier Hamon [Inria, from Apr 2021 until Oct 2021]
- Katia Jodogne–Del Litto [Inria, from Mar 2021 until Aug 2021]
- Tianwei Lan [Inria, from Mar 2021 until Aug 2021]
- Yoann Lemesle [Inria, from May 2021 until Aug 2021]
- Mateo Mahaut [Inria, from Feb 2021 until Aug 2021]
- Thomas Michel [Ecole normale supérieure Paris-Saclay, from Jun 2021 until Jul 2021]
- Marie Pelletier [Univ de Bordeaux, from Jun 2021 until Jul 2021]
- Mathieu Perie [Inria, Apprentice, from Mar 2021]
- Miliana Rahouadj [Inria, from Apr 2021 until Jul 2021]
- Isabeau Saint-Supery [Inria, until Jun 2021]
- Emma Tison [Inria, until Jun 2021]
Administrative Assistant
- Nathalie Robin [Inria]
Visiting Scientist
- Paul Barde [Quebec AI institute (Mila), until Aug 2021]
External Collaborator
- Wang Chak Chan [Automated Systems Limited-Hong Kong]
2 Overall objectives
Abstract:The Flowers project-team studies models of open-ended development and learning. These models are used as tools to help us understand better how children learn, as well as to build machines that learn like children, i.e. developmental artificial intelligence, with applications in educational technologies, assisted scientific discovery, video games, robotics and human-computer interaction.
Context: Great advances have been made recently in artificial intelligence concerning the topic of how autonomous agents can learn to act in uncertain and complex environments, thanks to the development of advanced Deep Reinforcement Learning techniques. These advances have for example led to impressive results with AlphaGo 177 or algorithms that learn to play video games from scratch 158, 132. However, these techniques are still far away from solving the ambitious goal of lifelong autonomous machine learning of repertoires of skills in real-world, large and open environments. They are also very far from the capabilities of human learning and cognition. Indeed, developmental processes allow humans, and especially infants, to continuously acquire novel skills and adapt to their environment over their entire lifetime. They do so autonomously, i.e. through a combination of self-exploration and linguistic/social interaction with their social peers, sampling their own goals while benefiting from the natural language guidance of their peers, and without the need for an “engineer” to open and retune the brain and the environment specifically for each new task (e.g. for providing a task-specific external reward channel). Furthermore, humans are extremely efficient at learning fast (few interactions with their environment) skills that are very high-dimensional both in perception and action, while being embedded in open changing environments with limited resources of time, energy and computation.
Thus, a major scientific challenge in artificial intelligence and cognitive sciences is to understand how humans and machines can efficiently acquire world models, as well as open and cumulative repertoires of skills over an extended time span. Processes of sensorimotor, cognitive and social development are organized along ordered phases of increasing complexity, and result from the complex interaction between the brain/body with its physical and social environment. Making progress towards these fundamental scientific challenges is also crucial for many downstream applications. Indeed, autonomous lifelong learning capabilities similar to those shown by humans are key requirements for developing virtual or physical agents that need to continuously explore and adapt skills for interacting with new or changing tasks, environments, or people. This is crucial for applications like assistive technologies with non-engineer users, such as robots or virtual agents that need to explore and adapt autonomously to new environments, adapt robustly to potential damages of their body, or help humans to learn or discover new knowledge in education settings, and need to communicate through natural language with human users, grounding the meaning of sentences into their sensorimotor representations.
The Developmental AI approach: Human and biological sciences have identified various families of developmental mechanisms that are key to explain how infants can acquire so robustly a wide diversity of skills 134, 156, in spite of the complexity and high-dimensionality of the body 97 and the open-endedness of its potential interactions with the physical and social environment. To advance the fundamental understanding of these mechanisms of development as well as their transposition in machines, the FLOWERS team has been developing an approach called Developmental artificial intelligence, leveraging and integrating the ideas and techniques from developmental robotics (193, 149, 102, 162, the team was already a key player of the creation and development of this field), Deep (Reinforcement) Learning and developmental psychology. This approach consists in developing computational models that leverage advanced machine learning techniques such as intrinsically motivated Deep Reinforcement Learning, in strong collaboration with developmental psychology and neuroscience. In particular, the team focuses on models of intrinsically motivated learning and exploration (also called curiosity-driven learning), with mechanisms enabling agents to learn to represent and generate their own goals, self-organizing a learning curriculum for efficient learning of world models and skill repertoire under limited resources of time, energy and compute. The team also studies how autonomous learning mechanisms can enable humans and machines to acquire grounded language skills, using neuro-symbolic architectures for learning structured representations and handling systematic compositionality and generalization.
Our fundamental research is organized along three strands:
-
Strand 1: Lifelong autonomous learning in machines.
Understanding how developmental mechanisms can be functionally formalized/transposed in machines and explore how they can allow these machines to acquire efficiently open-ended repertoires of skills through self-exploration and social interaction.
-
Strand 2: Computational models as tools to understand human development in cognitive sciences.
The computational modelling of lifelong learning and development mechanisms achieved in the team centrally targets to contribute to our understanding of the processes of sensorimotor, cognitive and social development in humans. In particular, it provides a methodological basis to analyze the dynamics of interactions across learning and inference processes, embodiment and the social environment, allowing to formalize precise hypotheses and later on test them in experimental paradigms with animals and humans. A paradigmatic example of this activity is the Neurocuriosity project achieved in collaboration with the cognitive neuroscience lab of Jacqueline Gottlieb, where theoretical models of the mechanisms of information seeking, active learning and spontaneous exploration have been developped in coordination with experimental evidence and investigation 17, 46.
-
Strand 3: Applications.
Beyond leading to new theories and new experimental paradigms to understand human development in cognitive science, as well as new fundamental approaches to developmental machine learning, the team explores how such models can find applications in robotics, human-computer interaction, multi-agent systems, automated discovery and educational technologies. In robotics, the team studies how artificial curiosity combined with imitation learning can provide essential building blocks allowing robots to acquire multiple tasks through natural interaction with naive human users, for example in the context of assistive robotics. The team also studies how models of curiosity-driven learning can be transposed in algorithms for intelligent tutoring systems, allowing educational software to incrementally and dynamically adapt to the particularities of each human learner, and proposing personalized sequences of teaching activities.
3 Research program
Research in artificial intelligence, machine learning and pattern recognition has produced a tremendous amount of results and concepts in the last decades. A blooming number of learning paradigms - supervised, unsupervised, reinforcement, active, associative, symbolic, connectionist, situated, hybrid, distributed learning... - nourished the elaboration of highly sophisticated algorithms for tasks such as visual object recognition, speech recognition, robot walking, grasping or navigation, the prediction of stock prices, the evaluation of risk for insurances, adaptive data routing on the internet, etc... Yet, we are still very far from being able to build machines capable of adapting to the physical and social environment with the flexibility, robustness, and versatility of a one-year-old human child.
Indeed, one striking characteristic of human children is the nearly open-ended diversity of the skills they learn. They not only can improve existing skills, but also continuously learn new ones. If evolution certainly provided them with specific pre-wiring for certain activities such as feeding or visual object tracking, evidence shows that there are also numerous skills that they learn smoothly but could not be “anticipated” by biological evolution, for example learning to drive a tricycle, using an electronic piano toy or using a video game joystick. On the contrary, existing learning machines, and robots in particular, are typically only able to learn a single pre-specified task or a single kind of skill. Once this task is learnt, for example walking with two legs, learning is over. If one wants the robot to learn a second task, for example grasping objects in its visual field, then an engineer needs to re-program manually its learning structures: traditional approaches to task-specific machine/robot learning typically include engineer choices of the relevant sensorimotor channels, specific design of the reward function, choices about when learning begins and ends, and what learning algorithms and associated parameters shall be optimized.
As can be seen, this requires a lot of important choices from the engineer, and one could hardly use the term “autonomous” learning. On the contrary, human children do not learn following anything looking like that process, at least during their very first years. Babies develop and explore the world by themselves, focusing their interest on various activities driven both by internal motives and social guidance from adults who only have a folk understanding of their brains. Adults provide learning opportunities and scaffolding, but eventually young babies always decide for themselves what activity to practice or not. Specific tasks are rarely imposed to them. Yet, they steadily discover and learn how to use their body as well as its relationships with the physical and social environment. Also, the spectrum of skills that they learn continuously expands in an organized manner: they undergo a developmental trajectory in which simple skills are learnt first, and skills of progressively increasing complexity are subsequently learnt.
A link can be made to educational systems where research in several domains have tried to study how to provide a good learning or training experience to learners. This includes the experiences that allow better learning, and in which sequence they must be experienced. This problem is complementary to that of the learner who tries to progress efficiently, and the teacher here has to use as efficiently the limited time and motivational resources of the learner. Several results from psychology 96 and neuroscience 123 have argued that the human brain feels intrinsic pleasure in practicing activities of optimal difficulty or challenge. A teacher must exploit such activities to create positive psychological states of flow 112 for fostering the indivual engagement in learning activities. A such view is also relevant for reeducation issues where inter-individual variability, and thus intervention personalization are challenges of the same magnitude as those for education of children.
A grand challenge is thus to be able to build machines that possess this capability to discover, adapt and develop continuously new know-how and new knowledge in unknown and changing environments, like human children. In 1950, Turing wrote that the child's brain would show us the way to intelligence: “Instead of trying to produce a program to simulate the adult mind, why not rather try to produce one which simulates the child's” 187. Maybe, in opposition to work in the field of Artificial Intelligence who has focused on mechanisms trying to match the capabilities of “intelligent” human adults such as chess playing or natural language dialogue 128, it is time to take the advice of Turing seriously. This is what a new field, called developmental (or epigenetic) robotics, is trying to achieve 149193. The approach of developmental robotics consists in importing and implementing concepts and mechanisms from developmental psychology 155, cognitive linguistics 111, and developmental cognitive neuroscience 133 where there has been a considerable amount of research and theories to understand and explain how children learn and develop. A number of general principles are underlying this research agenda: embodiment 100166, grounding 126, situatedness 181, self-organization 183161, enaction 190, and incremental learning 107.
Among the many issues and challenges of developmental robotics, two of them are of paramount importance: exploration mechanisms and mechanisms for abstracting and making sense of initially unknown sensorimotor channels. Indeed, the typical space of sensorimotor skills that can be encountered and learnt by a developmental robot, as those encountered by human infants, is immensely vast and inhomogeneous. With a sufficiently rich environment and multimodal set of sensors and effectors, the space of possible sensorimotor activities is simply too large to be explored exhaustively in any robot's life time: it is impossible to learn all possible skills and represent all conceivable sensory percepts. Moreover, some skills are very basic to learn, some other very complicated, and many of them require the mastery of others in order to be learnt. For example, learning to manipulate a piano toy requires first to know how to move one's hand to reach the piano and how to touch specific parts of the toy with the fingers. And knowing how to move the hand might require to know how to track it visually.
Exploring such a space of skills randomly is bound to fail or result at best on very inefficient learning 163. Thus, exploration needs to be organized and guided. The approach of epigenetic robotics is to take inspiration from the mechanisms that allow human infants to be progressively guided, i.e. to develop. There are two broad classes of guiding mechanisms which control exploration:
- internal guiding mechanisms, and in particular intrinsic motivation, responsible of spontaneous exploration and curiosity in humans, which is one of the central mechanisms investigated in FLOWERS, and technically amounts to achieve online active self-regulation of the growth of complexity in learning situations;
- social learning and guidance, a learning mechanisms that exploits the knowledge of other agents in the environment and/or that is guided by those same agents. These mechanisms exist in many different forms like emotional reinforcement, stimulus enhancement, social motivation, guidance, feedback or imitation, some of which being also investigated in FLOWERS.
Internal guiding mechanisms
In infant development, one observes a progressive increase of the complexity of activities with an associated progressive increase of capabilities 155, children do not learn everything at one time: for example, they first learn to roll over, then to crawl and sit, and only when these skills are operational, they begin to learn how to stand. The perceptual system also gradually develops, increasing children perceptual capabilities other time while they engage in activities like throwing or manipulating objects. This make it possible to learn to identify objects in more and more complex situations and to learn more and more of their physical characteristics.
Development is therefore progressive and incremental, and this might be a crucial feature explaining the efficiency with which children explore and learn so fast. Taking inspiration from these observations, some roboticists and researchers in machine learning have argued that learning a given task could be made much easier for a robot if it followed a developmental sequence and “started simple” 91117. However, in these experiments, the developmental sequence was crafted by hand: roboticists manually build simpler versions of a complex task and put the robot successively in versions of the task of increasing complexity. And when they wanted the robot to learn a new task, they had to design a novel reward function.
Thus, there is a need for mechanisms that allow the autonomous control and generation of the developmental trajectory. Psychologists have proposed that intrinsic motivations play a crucial role. Intrinsic motivations are mechanisms that push humans to explore activities or situations that have intermediate/optimal levels of novelty, cognitive dissonance, or challenge 96112114. Futher, the exploration of critical role of intrinsic motivation as lever of cognitive developement for all and for all ages is today expanded to several fields of research, closest to its original study, special education or cognitive aging, and farther away, neuropsychological clinical research. The role and structure of intrinsic motivation in humans have been made more precise thanks to recent discoveries in neuroscience showing the implication of dopaminergic circuits and in exploration behaviours and curiosity 113129176. Based on this, a number of researchers have began in the past few years to build computational implementation of intrinsic motivation 16316417494130151175. While initial models were developed for simple simulated worlds, a current challenge is to manage to build intrinsic motivation systems that can efficiently drive exploratory behaviour in high-dimensional unprepared real world robotic sensorimotor spaces 164, 163, 165, 173. Specific and complex problems are posed by real sensorimotor spaces, in particular due to the fact that they are both high-dimensional as well as (usually) deeply inhomogeneous. As an example for the latter issue, some regions of real sensorimotor spaces are often unlearnable due to inherent stochasticity or difficulty, in which case heuristics based on the incentive to explore zones of maximal unpredictability or uncertainty, which are often used in the field of active learning 110127 typically lead to catastrophic results. The issue of high dimensionality does not only concern motor spaces, but also sensory spaces, leading to the problem of correctly identifying, among typically thousands of quantities, those latent variables that have links to behavioral choices. In FLOWERS, we aim at developing intrinsically motivated exploration mechanisms that scale in those spaces, by studying suitable abstraction processes in conjunction with exploration strategies.
Socially Guided and Interactive Learning
Social guidance is as important as intrinsic motivation in the cognitive development of human babies 155. There is a vast literature on learning by demonstration in robots where the actions of humans in the environment are recognized and transferred to robots 90. Most such approaches are completely passive: the human executes actions and the robot learns from the acquired data. Recently, the notion of interactive learning has been introduced in 184, 99, motivated by the various mechanisms that allow humans to socially guide a robot 170. In an interactive context the steps of self-exploration and social guidance are not separated and a robot learns by self exploration and by receiving extra feedback from the social context 184, 141, 152.
Social guidance is also particularly important for learning to segment and categorize the perceptual space. Indeed, parents interact a lot with infants, for example teaching them to recognize and name objects or characteristics of these objects. Their role is particularly important in directing the infant attention towards objects of interest that will make it possible to simplify at first the perceptual space by pointing out a segment of the environment that can be isolated, named and acted upon. These interactions will then be complemented by the children own experiments on the objects chosen according to intrinsic motivation in order to improve the knowledge of the object, its physical properties and the actions that could be performed with it.
In FLOWERS, we are aiming at including intrinsic motivation system in the self-exploration part thus combining efficient self-learning with social guidance 159, 160. We also work on developing perceptual capabilities by gradually segmenting the perceptual space and identifying objects and their characteristics through interaction with the user 150 and robots experiments 131. Another challenge is to allow for more flexible interaction protocols with the user in terms of what type of feedback is provided and how it is provided 146.
Exploration mechanisms are combined with research in the following directions:
Cumulative learning, reinforcement learning and optimization of autonomous skill learning
FLOWERS develops machine learning algorithms that can allow embodied machines to acquire cumulatively sensorimotor skills. In particular, we develop optimization and reinforcement learning systems which allow robots to discover and learn dictionaries of motor primitives, and then combine them to form higher-level sensorimotor skills.
Autonomous perceptual and representation learning
In order to harness the complexity of perceptual and motor spaces, as well as to pave the way to higher-level cognitive skills, developmental learning requires abstraction mechanisms that can infer structural information out of sets of sensorimotor channels whose semantics is unknown, discovering for example the topology of the body or the sensorimotor contingencies (proprioceptive, visual and acoustic). This process is meant to be open- ended, progressing in continuous operation from initially simple representations towards abstract concepts and categories similar to those used by humans. Our work focuses on the study of various techniques for:
- autonomous multimodal dimensionality reduction and concept discovery;
- incremental discovery and learning of objects using vision and active exploration, as well as of auditory speech invariants;
- learning of dictionaries of motion primitives with combinatorial structures, in combination with linguistic description;
- active learning of visual descriptors useful for action (e.g. grasping).
Embodiment and maturational constraints
FLOWERS studies how adequate morphologies and materials (i.e. morphological computation), associated to relevant dynamical motor primitives, can importantly simplify the acquisition of apparently very complex skills such as full-body dynamic walking in biped. FLOWERS also studies maturational constraints, which are mechanisms that allow for the progressive and controlled release of new degrees of freedoms in the sensorimotor space of robots.
Discovering and abstracting the structure of sets of uninterpreted sensors and motors
FLOWERS studies mechanisms that allow a robot to infer structural information out of sets of sensorimotor channels whose semantics is unknown, for example the topology of the body and the sensorimotor contingencies (proprioceptive, visual and acoustic). This process is meant to be open-ended, progressing in continuous operation from initially simple representations to abstract concepts and categories similar to those used by humans.
Emergence of social behavior in multi-agent populations
FLOWERS studies how populations of interacting learning agents can collectively acquire cooperative or competitive strategies in challenging simulated environments. This differs from "Social learning and guidance" presented above: instead of studying how a learning agent can benefit from the interaction with a skilled agent, we rather consider here how social behavior can spontaneously emerge from a population of interacting learning agents. We focus on studying and modeling the emergence of cooperation, communication and cultural innovation based on theories in behavioral ecology and language evolution, using recent advances in multi-agent reinforcement learning.
Cognitive variability across Lifelong development and (re)educational Technologies
Over the past decade, the progress in the field of curiosity-driven learning generates a lot of hope, especially with regard to a major challenge, namely the inter-individual variability of developmental trajectories of learning, which is particularly critical during childhood and aging or in conditions of cognitive disorders. With the societal purpose of tackling of social inegalities, FLOWERS deals to move forward this new research avenue by exploring the changes of states of curiosity across lifespan and across neurodevelopemental conditions (neurotypical vs. learning disabilities) while designing new educational or rehabilitative technologies for curiosity-driven learning. The information gaps or learning progress, and their awareness are the core mechanisms of this part of research program due to high value as brain fuel by which the individual's internal intrinsic state of motivation is maintained and leads him/her to pursue his/her cognitive efforts for acquisitions /rehabilitations. Accordingly, a main challenge is to understand these mechanisms in order to draw up supports for the curiosity-driven learning, and then to embed them into (re)educational technologies. To this end, two-ways of investigations are carried out in real-life setting (school, home, work place etc): 1) the design of curiosity-driven interactive systems for learning and their effectiveness study ; and 2) the automated personnalization of learning programs through new algorithms maximizing learning progress in ITS.
4 Application domains
Neuroscience, Developmental Psychology and Cognitive Sciences The computational modelling of life-long learning and development mechanisms achieved in the team centrally targets to contribute to our understanding of the processes of sensorimotor, cognitive and social development in humans. In particular, it provides a methodological basis to analyze the dynamics of the interaction across learning and inference processes, embodiment and the social environment, allowing to formalize precise hypotheses and later on test them in experimental paradigms with animals and humans. A paradigmatic example of this activity is the Neurocuriosity project achieved in collaboration with the cognitive neuroscience lab of Jacqueline Gottlieb, where theoretical models of the mechanisms of information seeking, active learning and spontaneous exploration have been developed in coordination with experimental evidence and investigation, see https://flowers.inria.fr/neurocuriosityproject/. Another example is the study of the role of curiosity in learning in the elderly, with a view to assessing its positive value against the cognitive aging as a protective ingredient (i.e, Industrial project with Onepoint and joint project with M. Fernendes from the Cognitive neursocience Lab of the University of Waterloo).
Personal and lifelong learning assistive agents Many indicators show that the arrival of personal assistive agents in everyday life, ranging from digital assistants to robots, will be a major fact of the 21st century. These agents will range from purely entertainment or educative applications to social companions that many argue will be of crucial help in our society. Yet, to realize this vision, important obstacles need to be overcome: these agents will have to evolve in unpredictable environments and learn new skills in a lifelong manner while interacting with non-engineer humans, which is out of reach of current technology. In this context, the refoundation of intelligent systems that developmental AI is exploring opens potentially novel horizons to solve these problems. In particular, this application domain requires advances in artificial intelligence that go beyond the current state-of-the-art in fields like deep learning. Currently these techniques require tremendous amounts of data in order to function properly, and they are severely limited in terms of incremental and transfer learning. One of our goals is to drastically reduce the amount of data required in order for this very potent field to work when humans are in-the-loop. We try to achieve this by making neural networks aware of their knowledge, i.e. we introduce the concept of uncertainty, and use it as part of intrinsically motivated multitask learning architectures, and combined with techniques of learning by imitation.
Educational technologies that foster curiosity-driven and personalized learning. Optimal teaching and efficient teaching/learning environments can be applied to aid teaching in schools aiming both at increase the achievement levels and the reduce time needed. From a practical perspective, improved models could be saving millions of hours of students' time (and effort) in learning. These models should also predict the achievement levels of students in order to influence teaching practices. The challenges of the school of the 21st century, and in particular to produce conditions for active learning that are personalized to the student's motivations, are challenges shared with other applied fields. Special education for children with special needs, such as learning disabilities, has long recognized the difficulty of personalizing contents and pedagogies due to the great variability between and within medical conditions. More remotely, but not so much, cognitive rehabilitative carers are facing the same challenges where today they propose standardized cognitive training or rehabilitation programs but for which the benefits are modest (some individuals respond to the programs, others respond little or not at all), as they are highly subject to inter- and intra-individual variability. The curiosity-driven technologies for learning and STIs could be a promising avenue to address these issues that are common to (mainstream and specialized)education and cognitive rehabilitation.
Automated discovery in science. Machine learning algorithms integrating intrinsically-motivated goal exploration processes (IMGEPs) with flexible modular representation learning are very promising directions to help human scientists discover novel structures in complex dynamical systems, in fields ranging from biology to physics. The automated discovery project lead by the FLOWERS team aims to boost the efficiency of these algorithms for enabling scientist to better understand the space of dynamics of bio-physical systems, that could include systems related to the design of new materials or new drugs with applications ranging from regenerative medicine to unraveling the chemical origins of life. As an example, Grizou et al. 124 recently showed how IMGEPs can be used to automate chemistry experiments addressing fundamental questions related to the origins of life (how oil droplets may self-organize into protocellular structures), leading to new insights about oil droplet chemistry. Such methods can be applied to a large range of complex systems in order to map the possible self-organized structures. The automated discovery project is intended to be interdisciplinary and to involve potentially non-expert end-users from a variety of domains. In this regard, we are currently collaborating with Poietis (a bio-printing company) and Bert Chan (an independant researcher in artificial life) to deploy our algorithms. To encourage the adoption of our algorithms by a wider community, we are also working on an interactive software which aims to provide tools to easily use the automated exploration algorithms (e.g. curiosity-driven) in various systems.
Human-Robot Collaboration. Robots play a vital role for industry and ensure the efficient and competitive production of a wide range of goods. They replace humans in many tasks which otherwise would be too difficult, too dangerous, or too expensive to perform. However, the new needs and desires of the society call for manufacturing system centered around personalized products and small series productions. Human-robot collaboration could widen the use of robot in this new situations if robots become cheaper, easier to program and safe to interact with. The most relevant systems for such applications would follow an expert worker and works with (some) autonomy, but being always under supervision of the human and acts based on its task models.
Environment perception in intelligent vehicles. When working in simulated traffic environments, elements of FLOWERS research can be applied to the autonomous acquisition of increasingly abstract representations of both traffic objects and traffic scenes. In particular, the object classes of vehicles and pedestrians are if interest when considering detection tasks in safety systems, as well as scene categories (”scene context”) that have a strong impact on the occurrence of these object classes. As already indicated by several investigations in the field, results from present-day simulation technology can be transferred to the real world with little impact on performance. Therefore, applications of FLOWERS research that is suitably verified by real-world benchmarks has direct applicability in safety-system products for intelligent vehicles.
5 Social and environmental responsibility
5.1 Footprint of research activities
AI is a field of research that currently requires a lot of computational resources, which is a challenge as these resources have an environmental cost. In the team we try to address this challenge in two ways:
- by working on developmental machine learning approaches that model how humans manage to learn open-ended and diverse repertoires of skills under severe limits of time, energy and compute: for example, curiosity-driven learning algorithms can be used to guide agent's exploration of their environment so that they learn a world model in a sample efficient manner, i.e. by minimizing the number of runs and computations they need to perform in the environment;
- by monitoring the number of CPU and GPU hours required to carry out our experiments. For instance, our work 9 used a total of 2.5 cpu years. More globally, our work uses large scale computational resources, such as the Jean Zay supercomputer platform, for which we obtained a credit of 2 millions hours of GPU and CPU for year 2021.
5.2 Impact of research results
Our research activities are organized along two fundamental research axis (models of human learning and algorithms for developmental machine learning) and one application research axis (involving multiple domains of application, see the Application Domains section). This entails different dimensions of potential societal impact:
- Towards autonomous agents that can be shaped to human preferences and be explainable We work on reinforcement learning architectures where autonomous agents interact with a social partner to explore a large set of possible interactions and learn to master them, using language as a key communication medium. As a result, our work contributes to facilitating human intervention in the learning process of agents (e.g. digital assistants, video games characters, robots), which we believe is a key step towards more explainable and safer autonomous agents.
- Reproducibility of research: By releasing the codes of our research papers, we believe that we help efforts in reproducible science and allow the wider community to build upon and extend our work in the future. In that spirit, we also provide clear explanations on the statistical testing methods when reporting the results.
- AI and personalized educational technologies that support inclusivity and diversity and reduce inequalities The Flowers team develops AI technologies aiming to personalize sequences of educationa activities in digital educational apps: this entails the central challenge of designing systems which can have equitable impact over a diversity of students and reduce inequalitie. Using models of curiosity-driven learning to design AI algorithms for such personalization, we have been working to enable them to be positively and equitably impactful across several dimensions of diversity: for young learners or for aging populations; for learners with low initial levels as well as for learners with high initial levels; for "normally" developping children and for children with developmental disorders; and for learners of different socio-cultural backgrounds (e.g. we could show in the KidLearn project that the system is equally impactful along these various kinds of diversities).
- Health: Bio-printing The Flowers team is studying the use of curiosity-driven exploraiton algorithm in the domain of automated discovery, enabling scientists in physics/chemistry/biology to efficiently explore and build maps of the possible structures of various complex systems. One particular domain of application we are studying is bio-printing, where a challenge consists in exploring and understanding the space of morphogenetic structures self-organized by bio-printed cell populations. This could facilitate the design and bio-printing of personalized skins or organoids for people that need transplants, and thus could have major impact on the health of people needing such transplants.
- Tools for human creativity and the arts Curiosity-driven exploration algorithms could also in principle be used as tools to help human users in creative activities ranging from writing stories to painting or musical creation, which are domains we aim to consider in the future, and thus this constitutes another societal and cultural domain where our research could have impact.
- Education to AI As artificial intelligence takes a greater role in human society, it is of foremost importance to empower individuals with understanding of these technologies. For this purpose, the Flowers lab has been actively involved in educational and popularization activities, in particular by designing educational robotics kits that form a motivating and tangible context to understand basic concepts in AI: these include the Inirobot kit (used by >30k primary school students in France, see https://pixees.fr/dm1r.fr/ and the Poppy Education kit (https://www.poppy-education.org) now supported by the Poppy Station educational consortium (see https://www.poppy-station.org)
- Health: optimization of intervention strategies during pandemic events Modelling the dynamics of epidemics helps proposing control strategies based on pharmaceutical and non-pharmaceutical interventions (contact limitation, lock down, vaccination, etc). Hand-designing such strategies is not trivial because of the number of possible interventions and the difficulty to predict long-term effects. This task can be cast as an optimization problem where state-of-the-art machine learning algorithms such as deep reinforcement learning, might bring significant value. However, the specificity of each domain – epidemic modelling or solving optimization problem – requires strong collaborations between researchers from different fields of expertise. Due to its fundamental multi-objective nature, the problem of optimizing intervention strategies can benefit from the goal-conditioned reinforcement learning algorithms we develop at Flowers. In this context, we have developped EpidemiOptim, a Python toolbox that facilitates collaborations between researchers in epidemiology and optimization. https://epidemioptim.bordeaux.inria.fr/.
6 Highlights of the year
The team reached a major scientific milestone in its research program aiming to model human curiosity-driven learning, associated to an article published in Nature Communication 46: this paper presented the first experimental study in the literature directly testing the Learning Progress hypothesis in humans, formulated by PY Oudeyer and F. Kaplan around 15 years ago 136, 163. This new result is the outcome of a key collaboration with J. Gottlieb and her cognitive neuroscience lab at Columbia University, NY, and of the PhD work of Alexander Ten (co-supervised by PY Oudeyer and J Gottlieb).
The team continued to develop the developmental artificial intelligence perspective and introduce it to the machine learning community, in particular publishing papers at ICML 53, ICLR 47, AAMAS 54 and NeurIPS 50, as well as through blog posts (see http://developmentalsystems.org/language_as_cognitive_tool_vygotskian_rl and http://developmentalsystems.org/teacher_algorithms_for_drl_learners). The team also released the TeachMyAgent benchmark 53, providing to the scientific community a benchmark enabling to compare automated curriculum learning algorithms https://developmentalsystems.org/TeachMyAgent/.
The team also achieved several major societal contributions. In 2021, the team collaborated with the Inria/BPH team SISTM to build a software tool leveraging advanced deep reinforcement learning techniques to assess various intervention strategies for the Covid pandemic, associated to a journal paper in JAIR 33.
The team also organized the CREATE workshop - Designing technologies for older adults (see https://www.inria.fr/fr/technologies-personnes-agees-vieillesse-dependance to work on improving digital access for elderly population.
Didier Roy was manager editor of a 370-pages computer science school textbook for kindergarten and elementary schools (collaboration Inria/EPFL/Canton de Vaud, Switzerland).
The team also reached a major industrial transfer milestone. Together with the edTech industrial consortium Adaptiv'Maths (https://www.adaptivmath.fr), we integrated our ZPDES machine learning algorithm, leveraging models of intrinsic motivation in humans, to personalize sequences of exercises in an educational software aiming to be used at large scale in the French educational system and beyond. This work was achieved by Benjamin Clément, co-supervised by Didier Roy and PY Oudeyer. We also started a new line of research investigating technologies that can help children to practice skills that are essential to foster curiosity-driven learning, such as question asking and meta-cognitive monitoring. This work is made through the PhD of Rania Abdelghani, co-supervised by Hélène Sauzéon and PY Oudeyer in collaboration with Edith Law's team at the University of Waterloo.
6.1 Awards
Didier Roy and Pierre-Yves Oudeyer were finalist of the Roberval prize in the category "Jeunesse" (http://prixroberval.utc.fr/ for their popular science book introducing artificial intelligence and its societal implications to primary school children https://site.nathan.fr/livres/les-robots-et-lintelligence-artificielle-questionsreponses-doc-des-7-ans-9782092593295.html.
7 New software and platforms
7.1 New software
7.1.1 Kidlearn: money game application
-
Functional Description:
The games is instantiated in a browser environment where students are proposed exercises in the form of money/token games (see Figure 1). For an exercise type, one object is presented with a given tagged price and the learner has to choose which combination of bank notes, coins or abstract tokens need to be taken from the wallet to buy the object, with various constraints depending on exercises parameters. The games have been developed using web technologies, HTML5, javascript and Django.
Figure 1: Four principal regions are defined in the graphical interface. The first is the wallet location where users can pick and drag the money items and drop them on the repository location to compose the correct price. The object and the price are present in the object location. Four different types of exercises exist: M : customer/one object, R : merchant/one object, MM : customer/two objects, RM : merchant/two objects. - URL:
-
Contact:
Benjamin Clement
7.1.2 Kidlearn: script for Kidbreath use
-
Keyword:
PHP
-
Functional Description:
A new way to test Kidlearn algorithms is to use them on Kidbreath Plateform. The Kidbreath Plateform use apache/PHP server, so to facilitate the integration of our algorithm, a python script have been made to allow PHP code to use easily the python library already made which include our algorithms.
- URL:
-
Contact:
Benjamin Clement
7.1.3 KidLearn
-
Keyword:
Automatic Learning
-
Functional Description:
KidLearn is a software which adaptively personalize sequences of learning activities to the particularities of each individual student. It aims at proposing to the student the right activity at the right time, maximizing concurrently his learning progress and its motivation.
- URL:
-
Contact:
Pierre-Yves Oudeyer
-
Participants:
Benjamin Clement, Didier Roy, Manuel Lopes, Pierre Yves Oudeyer
7.1.4 teachDeepRL
-
Name:
Teacher algorithms for curriculum learning of Deep RL in continuously parameterized environments
-
Keywords:
Machine learning, Git
-
Functional Description:
Codebase from our CoRL2019 paper https://arxiv.org/abs/1910.07224
This github repository provides implementations for the following teacher algorithms: - Absolute Learning Progress-Gaussian Mixture Model (ALP-GMM), our proposed teacher algorithm - Robust Intelligent Adaptive Curiosity (RIAC), from Baranes and Oudeyer, R-IAC: robust intrinsically motivated exploration and active learning. - Covar-GMM, from Moulin-Frier et al., Self-organization of early vocal development in infants and machines: The role of intrinsic motivation.
- URL:
-
Author:
Remy Portelas
-
Contact:
Remy Portelas
7.1.5 ZPDES_ts
-
Name:
ZPDES in typescript
-
Keywords:
Machine learning, Education
-
Functional Description:
ZPDES is a machine learning-based algorithm that allows you to customize the content of training courses for each learner's level. It has already been implemented in the Kidlern software in python with other algorithms. Here, ZPDES is implemented in typescript.
- URL:
-
Authors:
Benjamin Clement, Pierre-Yves Oudeyer, Didier Roy, Manuel Lopes
-
Contact:
Benjamin Clement
7.1.6 GEP-PG
-
Name:
Goal Exploration Process - Policy Gradient
-
Keywords:
Machine learning, Deep learning
-
Functional Description:
Reinforcement Learning algorithm working with OpenAI Gym environments. A first phase implements exploration using a Goal Exploration Process (GEP). Samples collected during exploration are then transferred to the memory of a deep reinforcement learning algorithm (deep deterministic policy gradient or DDPG). DDPG then starts learning from a pre-initialized memory so as to maximize the sum of discounted rewards given by the environment.
- URL:
-
Contact:
Cedric Colas
7.1.7 EpidemiOptim
-
Name:
EpidemiOptim: a toolbox for the optimization of control policies in epidemiological models
-
Keywords:
Epidemiology, Optimization, Dynamical system, Reinforcement learning, Multi-objective optimisation
-
Functional Description:
This toolbox proposes a modular set of tools to optimize intervention strategies in epidemiological models. The user can define or use a pre-coded epidemiological model to represent an epidemic. He/she can define a set of cost functions to define a particular optimization problem. Finally, given an optimization problem (epidemiological model and cost functions and action modalities), the user can define/reuse optimization algorithms to optimize intervention strategies that minimize the costs. Finally, the toolbox contains visualization and comparison tools. This allows to investigate various hypotheses easily.
- URL:
-
Contact:
Cedric Colas
7.1.8 IMAGINE
-
Keywords:
Exploration, Reinforcement learning, Modeling language, Artificial intelligence
-
Functional Description:
This software provides: 1. An environment modelling the social interaction between an autonomous agent and a social partner. The social partner gives natural language descriptions when the agent performs something interesting in the environment. 2. A modular architecture allowing the autonomous agent to manipulate and to target goals expressed in natural language. This architecture is divided into three modules: 2.a. A goal achievement function mapping language descriptions and the agent's observations to a reward signal 2.b. A goal conditioned-policy that uses the reward signal in order to learn the behaviour required to reach the goal (expressed in natural language). This module is trained via Reinforcement Learning 2.c. A goal imagination module allowing the agent to compose known goals into new sentences in order to creatively explore new outcomes in its environment.
- URL:
-
Contact:
Tristan Karch
7.1.9 DECSTR
-
Name:
Grouding Language to Autonomously-Acquired Skills via Goal Generation
-
Keywords:
Reinforcement learning, Curiosity, Intrinsic motivations
-
Functional Description:
DECSTR is a learning algorithm that trains an agent to reach semantic goals made of predicates characterizing spatial relations between pairs of blocks. After this first skill learning phase, the agent trains a language generation module that converts linguistic inputs into semantic goals. This module enables efficient language grounding.
- URL:
-
Contact:
Cedric Colas
7.1.10 holmes
-
Name:
IMGEP-HOLMES, an algorithm for meta-diversity search applied to the automated discovery of novel structures in complex dynamical systems
-
Keywords:
Exploration, Incremental learning, Unsupervised learning, Hierarchical architecture, Intrinsic motivations, Cellular automaton, Complexity
-
Functional Description:
Python source code to reproduce the experiments and data analysis for the paper "Hierarchically Organized Latent Modules for Exploratory Search in Morphogenetic Systems" (Mayalen Echeverry, Clément Moulin-Frier and Pierre-Yves Oudeyer, published at NeurIPS 2020). The user can define a complex system he would like to explore, or use the Lenia environment which is already provided. He/she can select an explorer to explore this system (Random or IMGEP explorer). For the IMGEP explorer, many variants of goal space representations are provided in the source code: hand-defined descriptors of the Lenia system, unsupervisedly learned descriptors that can be trained online during the course of exploration (VAE variants and Contrastive Learning variants) and the hierarchical progressively-learned architecture presented in the paper (HOLMES). To this purpose, the software includes tools and configurations to run experiments and for data analysis and comparison of the results, as well as for running the scripts on super-computers (SLURM job manager).
- URL:
-
Contact:
Mayalen Etcheverry
7.1.11 metaACL
-
Name:
Meta Automatic Curriculum Learning
-
Keywords:
Machine learning, Git
-
Functional Description:
Codebase from our arxiv paper https://arxiv.org/abs/2011.08463
This github repository provides implementations for AGAIN (Alp-Gmm and Inferred Progress Niches), our proposed Meta automatic curriculum learning teacher algorithm.
- URL:
-
Contact:
Remy Portelas
7.1.12 EmComPartObs
-
Name:
Studying the joint role of partial observability and channel reliability in emergent communication
-
Keywords:
Multi-agent, Reinforcement learning, Emergent communication
-
Functional Description:
This source code contains a new grid-world environment where two agents interact to solve a task, Multi-Agent Reinforcement algorithms that solve that task, as well as plotting utilities.
- URL:
- Publication:
-
Contact:
Clément Moulin-Frier
7.1.13 grimgep
-
Name:
GRIMGEP: Learning Progress for Robust Goal Sampling in Visual Deep Reinforcement Learning
-
Keywords:
Machine learning, Reinforcement learning, Artificial intelligence, Exploration, Intrinsic motivations, Git, Deep learning
-
Functional Description:
Source code for the GRIMGEP paper (https://arxiv.org/abs/2008.04388) Contains: - Implementation of the GRIMGEP framework on top of three different underlying imgeps (Skew-fit, CountBased, OnlineRIG). - image-based 2D environment (PlaygroundRGB)
- URL:
-
Contact:
Grgur Kovac
7.1.14 flowers-OL
-
Name:
flowers-open-lab
-
Keyword:
Experimentation
-
Functional Description:
This web platform designed for planning and implementing remote behavioural studies provides the following features: - Registration and login of participants - Presentation of the instructions concerning the experience and get informed consent - Behavioural task and questionnaires - Automatic management of a participant's schedule (sends emails before the user's appointments) - Quick and easy addition of new experimental conditions
- URL:
-
Authors:
Maxime Adolphe, Maxime Adolphe, Alexandr Ten
-
Contact:
Maxime Adolphe
-
Partner:
Onepoint
7.1.15 SocialAI
-
Name:
SocialAI: Benchmarking Socio-Cognitive Abilities in Deep Reinforcement Learning Agents
-
Keywords:
Artificial intelligence, Deep learning, Reinforcement learning
-
Functional Description:
Source code for the paper https://arxiv.org/abs/2107.00956.
A suite of environments for testing socio-cognitive abilities of RL agents. Simple RL baselines.
- URL:
-
Contact:
Grgur Kovac
7.1.16 Spatio-Temporal-Transformers
-
Name:
Grounding Spatio-Temporal Language with Transformers
-
Keywords:
Transformer, Artificial intelligence, Modeling language, Machine learning
-
Functional Description:
Source code for the paper Grounding Spatio-Temporal Language with Transformers.
This software provided: 1) An environment modeling the social interaction between an autonomous agent and a social partner. The social partner gives sentences in natural language describing the spatio-temporal behavior of the agent. The descriptions contain spatial references to the objects, predicates that span several time steps as well as spatiotemporal references to the objects. 2) A grammar and a temporal logic that control the generation of the spatio-temporal descriptions. 3) Several architectures based on Transformers that learn multimodal truth functions that predict the compatibility between a spatio-temporal description and a behavioural trace of an agent
- URL:
-
Contact:
Tristan Karch
7.1.17 SocSRL
-
Name:
Socially Supervised Representation Learning
-
Keyword:
Multi-agent
-
Scientific Description:
Code related to work on socially supervised representation learning (SocSRL). SocSRL is a multi-agent representation learning technique that exploits the inherent subjectivity of multi-agent systems to improve upon representations.
-
Functional Description:
Open source code associated with research paper SocSRL
- URL:
-
Contact:
Julius Taylor
7.1.18 Transflower
-
Name:
Transflower: probabilistic autoregressive dance generation with multimodal attention
-
Keywords:
Probability, Artificial intelligence, 3D animation, Motion capture, Neural networks
-
Scientific Description:
The model uses a type of neural network called a transformer to represent the recent history of motion, and music context. This representation is passed to a normalizing flow, which can flexibily model probability distributions over next poses. Running this iteratively generates the motion. The code is made with generality in mind, so that the model can be used for parametrizing probability distributions over general continuous signals.
-
Functional Description:
The code is able to probabilistically model continuous signals, such as movement. After training on a dataset of dance motion (captured using a variety of mocap techniques), the model, through a sampling/inference process, can be used to generate new pieces of dance for any given piece of music.
- URL:
- Publication:
-
Contact:
Guillermo Jorge Valle Perez
-
Participants:
Guillermo Jorge Valle Perez, Simon Alexanderson, Gustav Eje Henter, Jonas Beskow, André Holzapfel, Pierre-Yves Oudeyer
-
Partner:
KTH Royal Institute of Technology
7.1.19 evocraftsearch
-
Name:
Open-ended artefact generation in Minecraft
-
Keywords:
Exploration, Intrinsic motivations, Unsupervised learning, Cellular automaton, Complexity
-
Functional Description:
Python source code whose general structure is inspired from OpenAI's Gym library. The user can define a (System, OutputRepresentation, OutputFitness and Explorer) either using the already provided classes or implementing its own from the provided templates. Among others, the user can select the system (LeniaChem), explorer (IMGEP_HOLMES) and output representation (HOLMES) that were used for the challenge submission.Then the user can launch an exploration (following the script provided in the examples). The source code implements the interaction with the Evocraft API (https://github.com/real-itu/Evocraft-py) allowing to send and test the discoveries in the Minecraft server. The software includes tools and configurations to reproduce the experiments and for data analysis of the results.
- URL:
-
Contact:
Mayalen Etcheverry
7.1.20 TeachMyAgent
-
Name:
TeachMyAgent: a Benchmark for Automatic Curriculum Learning in Deep RL
-
Keywords:
Reinforcement learning, Machine learning, Curriculum Learning
-
Functional Description:
We release our platform as an open-source repository along with APIs allowing one to extend our testbed. We currently provide the following elements: - Two parametric Box2D environments: Stump Tracks (an extension of this environment) and Parkour - Multiple embodiments with different locomotion skills (e.g. bipedal walker, spider, climbing chimpanzee, fish) - Two Deep RL students: SAC and PPO - Several ACL algorithms: ADR, ALP-GMM, Covar-GMM, SPDL, GoalGAN, Setter-Solver, RIAC - Two benchmark experiments using elements above: Skill-specific comparison and global performance assessment - A notebook for systematic analysis of results using statistical tests along with visualisation tools (plots, videos...)
- URL:
- Publication:
-
Contact:
Clément Romac
-
Participants:
Clément Romac, Remy Portelas, Pierre-Yves Oudeyer
7.1.21 AutoDisc
-
Keyword:
Complex Systems
-
Functional Description:
AutoDisc is a software built for automated scientific discoveries in complex systems (e.g. self-organizing systems). It can be used as a tool to experiment automated discovery of various systems using exploration algorithms (e.g. curiosity-driven). Our software is fully Open Source and allows user to add their own systems, exploration algorithms or visualization methods.
- URL:
-
Contact:
Clément Romac
7.1.22 RL Stats
-
Name:
Library for the statistical comparison of RL algorithms.
-
Keywords:
Reinforcement learning, Statistic analysis
-
Functional Description:
This code allows to replicate the paper A Hitchhiker's Guide to Statistical Comparisons of Reinforcement Learning Algorithms.
It also facilitates the comparison of RL algorithms by using existing statistical tests.
- URL:
-
Contact:
Cedric Colas
7.1.23 Kids Ask
-
Keywords:
Human Computer Interaction, Cognitive sciences
-
Functional Description:
Kids Ask is a web-based educational platform that involves an interaction between a child and a conversational agent. The platform is designed to teach children how to generate curiosity-based questions and use them in their learning in order to gain new knowledge in an autonomous way.
-
News of the Year:
The kids Ask platform was used during two experiments with two different French primary schools, with a total of 53 participants that used the different functions of it.
- URL:
-
Contact:
Rania Abdelghani
7.1.24 SBDRL
-
Name:
Symmetry-Based Disentangled Representation Learning
-
Keywords:
Machine learning, Robotics
-
Functional Description:
Reproduction of the experiment of the paper : Caselles-Dupré, H., Garcia Ortiz, M., & Filliat, D. (2019). Symmetry-based disentangled representation learning requires interaction with environments. Advances in Neural Information Processing Systems, 32, 4606-4615.
- URL:
-
Contact:
Hugo Caselles-Dupre
7.1.25 AD-RobustnessEval
-
Name:
Evaluating Robustness over High Level Driving Instruction for Autonomous Driving
-
Keywords:
Robotics, Machine learning
-
Functional Description:
We propose a benchmark to evaluate the behavior of autonomous driving agents in unforeseen situations. Description in the paper : "Florence Carton, David Filliat, Jaonary Rabarisoa, Quoc Pham. Evaluating Robustness over High Level Driving Instruction for Autonomous Driving. IV 2021"
- URL:
-
Contact:
Florence Carton
7.1.26 humans-monitor-LP
-
Name:
Humans monitor learning progress in curiosity-driven exploration
-
Keywords:
Statistic analysis, Behavior modeling
-
Functional Description:
The repository contains jupyter notebooks with python code that replicate data processing, data analyses, and data visualizations reported in the study. The code for fitting the study's computational model is also included.
- Publication:
-
Contact:
Alexandr Ten
7.2 New platforms
7.2.1 ToGather application
-
Name:
Application for Specialized education
-
Keywords:
Parent-professional relationships; user-centered design; school inclusion; autism spectrum disorder; ecosystemic approach
-
Participants:
Isabeau Saint-supery, Cécile Mazon, Hélène Sauzéon, Agilonaute
-
Scientific Description:
With participatory design methods, we have designed an interactive website application for educational purposes. This application aims to provide interactive services with continuously updated content for the stakeholders of school inclusion of children with specific educational needs. Especially, the services provide: 1) the student's profile with strengths and weaknesses; 2) an evaluation and monitoring over time of the student's repertoire of acquired, emerging or targeted skills; 3) a shared notebook of effective psycho-educational solutions for the student ; 4) a shared messaging system for exchanging "news" about the student and his/her family and, 5) a meeting manager allowing updates of evaluations (student progress). This application is currently assessed with a field study. Then, it will be transferred to the Academy of Nouvelle-Aquitaine-Bordeaux of the National Education Ministery.
-
URL:
The website is not online yet.
- Publication:
8 New results
8.1 Computational Models of Curiosity-Driven Learning in Humans
8.1.1 Testing the Learning Progres Hypothesis in Curiosity-Driven explortion in Human Adults
Participants: Pierre-Yves Oudeyer [correspondant], Alexandr Ten.
This project involves a collaboration between the Flowers team and the Cognitive Neuroscience Lab of J. Gottlieb at Columbia Univ. (NY, US), on the understanding and computational modeling of mechanisms of curiosity, attention and active intrinsically motivated exploration in humans.
It is organized around the study of the hypothesis that subjective meta-cognitive evaluation of information gain (or control gain or learning progress) could generate intrinsic reward in the brain (living or artificial), driving attention and exploration independently from material rewards, and allowing for autonomous lifelong acquisition of open repertoires of skills. The project combines expertise about attention and exploration in the brain and a strong methodological framework for conducting experimentations with monkeys, human adults and children together with computational modeling of curiosity/intrinsic motivation and learning.
Such a collaboration paves the way towards a central objective, which is now a central strategic objective of the Flowers team: designing and conducting experiments in animals and humans informed by computational/mathematical theories of information seeking, and allowing to test the predictions of these computational theories.
Context
. Curiosity can be understood as a family of mechanisms that evolved to allow agents to maximize their knowledge (or their control) of the useful properties of the world - i.e., the regularities that exist in the world - using active, targeted investigations. In other words, we view curiosity as a decision process that maximizes learning/competence progress (rather than minimizing uncertainty) and assigns value ("interest") to competing tasks based on their epistemic qualities - i.e., their estimated potential allows discovery and learning about the structure of the world.
Because a curiosity-based system acts in conditions of extreme uncertainty (when the distributions of events may be entirely unknown) there is in general no optimal solution to the question of which exploratory action to take 147, 165, 172. Therefore,e we hypothesize that, rather than using a single optimization process as it has been the case in most previous theoretical work 123, curiosity is comprised of a family of mechanisms that include simple heuristics related to novelty/surprise and measures of learning progress over longer time scales 16393, 157. These different components are related to the subject's epistemic state (knowledge and beliefs) and may be integrated with fluctuating weights that vary according to the task context. Our aim is to quantitatively characterize this dynamic, multi-dimensional system in a computational framework based on models of intrinsically motivated exploration and learning.
Because of its reliance on epistemic currencies, curiosity is also very likely to be sensitive to individual differences in personality and cognitive functions. Humans show well-documented individual differences in curiosity and exploratory drives 145, 171, and rats show individual variation in learning styles and novelty seeking behaviors 119, but the basis of these differences is not understood. We postulate that an important component of this variation is related to differences in working memory capacity and executive control which, by affecting the encoding and retention of information, will impact the individual's assessment of learning, novelty and surprise and ultimately, the value they place on these factors 167, 182, 88, 188. To start understanding these relationships, about which nothing is known, we will search for correlations between curiosity and measures of working memory and executive control in the population of children we test in our tasks, analyzed from the point of view of a computational models of the underlying mechanisms.
A final premise guiding our research is that essential elements of curiosity are shared by humans and non-human primates. Human beings have a superior capacity for abstract reasoning and building causal models, which is a prerequisite for sophisticated forms of curiosity such as scientific research. However, if the task is adequately simplified, essential elements of curiosity are also found in monkeys 145, 137 and, with adequate characterization, this species can become a useful model system for understanding the neurophysiological mechanisms.
Objectives
. Our studies have several highly innovative aspects, both with respect to curiosity and to the traditional research field of each member team.
- Linking curiosity with quantitative theories of learning and decision making: While existing investigations examined curiosity in qualitative, descriptive terms, here we propose a novel approach that integrates quantitative behavioral and neuronal measures with computationally defined theories of learning and decision making.
- Linking curiosity in children and monkeys: While existing investigations examined curiosity in humans, here we propose a novel line of research that coordinates its study in humans and non-human primates. This will address key open questions about differences in curiosity between species, and allow access to its cellular mechanisms.
- Neurophysiology of intrinsic motivation: Whereas virtually all the animal studies of learning and decision making focus on operant tasks (where behavior is shaped by experimenter-determined primary rewards) our studies are among the very first to examine behaviors that are intrinsically motivated by the animals' own learning, beliefs or expectations.
- Neurophysiology of learning and attention: While multiple experiments have explored the single-neuron basis of visual attention in monkeys, all of these studies focused on vision and eye movement control. Our studies are the first to examine the links between attention and learning, which are recognized in psychophysical studies but have been neglected in physiological investigations.
- Computer science: biological basis for artificial exploration: While computer science has proposed and tested many algorithms that can guide intrinsically motivated exploration, our studies are the first to test the biological plausibility of these algorithms.
- Developmental psychology: linking curiosity with development: While it has long been appreciated that children learn selectively from some sources but not others, there has been no systematic investigation of the factors that engender curiosity, or how they depend on cognitive traits.
Results
. In a new milestone paper published in Nature Communications 46, and a follow-up article in the Cognitive Science conference 55, we provide empirical evidence that humans are sensitive to variation learning progress (LP) by means of a novel experimental paradigm 2 and computational modeling. We show that while humans rely on competence information to avoid easy tasks, models that include a learning-progress component provide the best fit to task selection data. These results bridge the research in artificial and biological curiosity, reveal strategies that are used by humans but have not been considered in computational research, and introduce tools for probing how humans become intrinsically motivated to learn and acquire interests and skills on extended time scales.
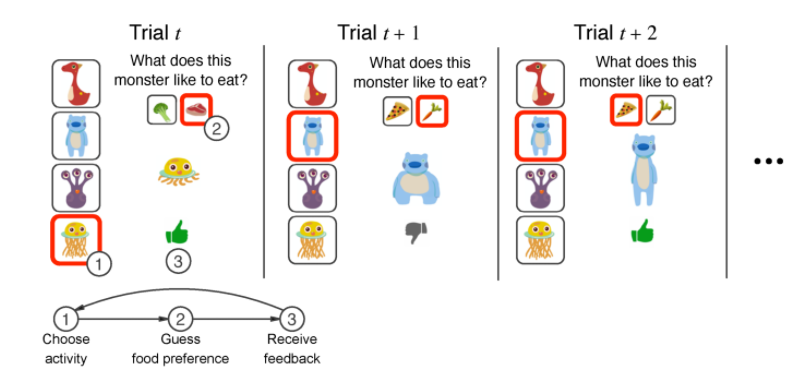
Task design. The panels show 3 example free-choice trials consisting of 3 steps each. Each trial begins with a choice of the stimulus family among the 4 icons on the left (1). This is followed by presentation of a randomly drawn individual from that family and a prompt to guess which food the individual likes to eat (2). After making the guess (2), the participant receives immediate feedback (3) and the next trial begins. For the next trial, the participant can either switch to a new monster family (e.g. trial t+1t+1) or repeat the previously sampled activity (e.g. trial t+2t+2).
8.1.2 Formation of subjective judgments of learning progress
Participants: Alexandr Ten [correspondant], Pierre-Yves Oudeyer, Hélène Sauzéon, Maxime Balan.
Although direct and unequivocal demonstration of LP computation in humans is still lacking, there are compelling theoretical 179, 148, 123 and empirical 154, 169, 14446 reasons to believe that active learning in humans depends on LP. On the other hand, metacognition research suggests that human reasoning about their own learning is not always accurate, particularly when it comes to improvement judgments 185, 186. To reconcile the tension between these views, we need not only a good definition (or a comprehensive taxonomy) for the concept of LP, but also authentic and reliable measurement tools. To be able to measure and model subjective LP, we need to address two important questions.
One question is how do humans subjectively represent tasks and task performance? Measures of LP based on the researcher's performance standards may differ from what people consider when judging how well they are doing and if they are improving. Understanding general principles behind subjective representations of competence across different tasks is key to being able to procure valid measurements of subjective performance and performance progress.
Another question is, what determines the time extent of progress judgments? To explain, when making a judgment of progress (or regress), one needs to compare two states of knowledge or competence. For computing LP, we assume that one compares one's current state to a state in the past. However, it is not obvious how this comparison is parameterized in humans. Is there a fixed time window that humans compute LP over? Or do we flexibly allocate our time to practicing particular tasks in order to get reliable LP estimates? What we can say for certain is that without knowing how humans choose what to compare their current level of knowledge/competence to, we cannot accurately measure subjective LP and study how it forms.
We have begun developing a behavioral study to address these questions. Because we wanted to study LP-judgments within the context of a naturalistic learning process, our study is built around a video-game task that requires an extended period of time to master. The task is based on an arcade game called Lunar Lander, where the goal is to control a spaceship and land it safely on the ground 3.
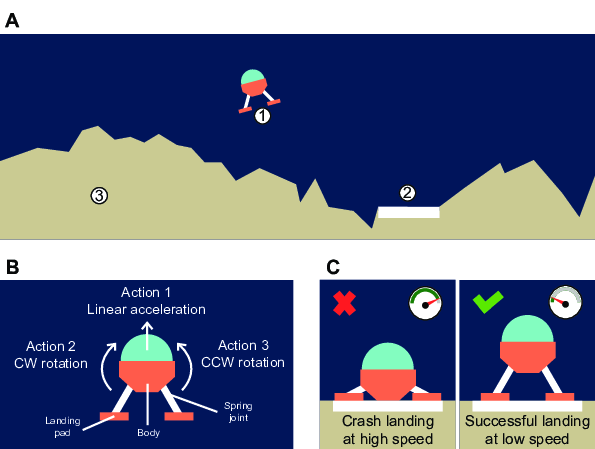
While the details of the main study are still being specified, we have conducted a pilot study aiming to (1) assess the effects of game initialization parameters on task achievement, (2) explore the relationships between several performance measures and improvement judgments, and (3) explore the relationships between improvement judgments and motivation. The results provide important lessons and pose intriguing questions for future work.
First, we have obtained some understanding of how several game parameters affect task-achievement rates. Manipulating objective difficulty is important to test causal relationships between learning and motivation/attitudes. Our results provide useful approximations of the effect sizes of game-difficulty parameters on task achievement. We also gained a sense of the learning dynamics and individual variability for the task. This knowledge be used for manipulating group-level learning profiles in independent-group designs. Given adequate tools for measuring the relevant data, we should be able to examine how self-evaluated performance dynamics relate to motivation and learning beliefs.
Our pilot study also showed that people might rely on the objective success-rate and/or subjective-competence dynamics in verbally reporting their improvement. Moreover, competence changes, measured over different temporal intervals, correlated with the corresponding self-reported judgments of improvement. We collected judgments of improvement about different time intervals (e.g., improvement within one session; improvement relative to the previous session). The idea was to evaluate whether judgments of some duration(s) would be better calibrated with reality than others, but our analyses failed to reveal such differences. The reported improvement judgments of different temporal sizes were similarly correlated with the corresponding objective improvement measures. It remains to be shown if there is a "basic" temporal interval which people tend to use naturally to gauge improvement for self-regulated learning, or if LP is temporally flexible.
Finally, we explored how different operationalizations of LP, including objective and subjective variables, relate to motivational and attitudinal measures. While LP correlated rather weakly and not reliably with intrinsic motivation, we found that it was a good predictor of beliefs about learning control and self-efficacy.
8.2 Developmental AI: Autotelic agents, intrinsically motivated goal-conditioned Deep RL, language learning
Participants: Pierre-Yves Oudeyer [correspondant], Olivier Sigaud, Cédric Colas, Adrien Laversanne-Finot, Rémy Portelas, Tristan Karch, Grgur Kovac.
8.2.1 Intrinsically Motivated Goal-Conditioned Reinforcement Learning: a Short Survey
Building autonomous machines that can explore open-ended environments, discover possible interactions and autonomously build repertoires of skills is a general objective of artificial intelligence. Developmental approaches argue that this can only be achieved by autonomous and intrinsically motivated learning agents that can generate, select and learn to solve their own problems. In recent years, we have seen a convergence of developmental approaches, and developmental robotics in particular, with deep reinforcement learning (RL) methods, forming the new domain of developmental machine learning. Within this new domain, we review here a set of methods where deep RL algorithms are trained to tackle the developmental robotics problem of the autonomous acquisition of open-ended repertoires of skills. Intrinsically motivated goal-conditioned RL algorithms train agents to learn to represent, generate and pursue their own goals. The self-generation of goals requires the learning of compact goal encodings as well as their associated goal-achievement functions, which results in new challenges compared to traditional RL algorithms designed to tackle pre-defined sets of goals using external reward signals. In a survey paper 71, we have proposed a typology of these methods at the intersection of deep RL and developmental approaches, surveyed recent approaches and discussed future avenues.
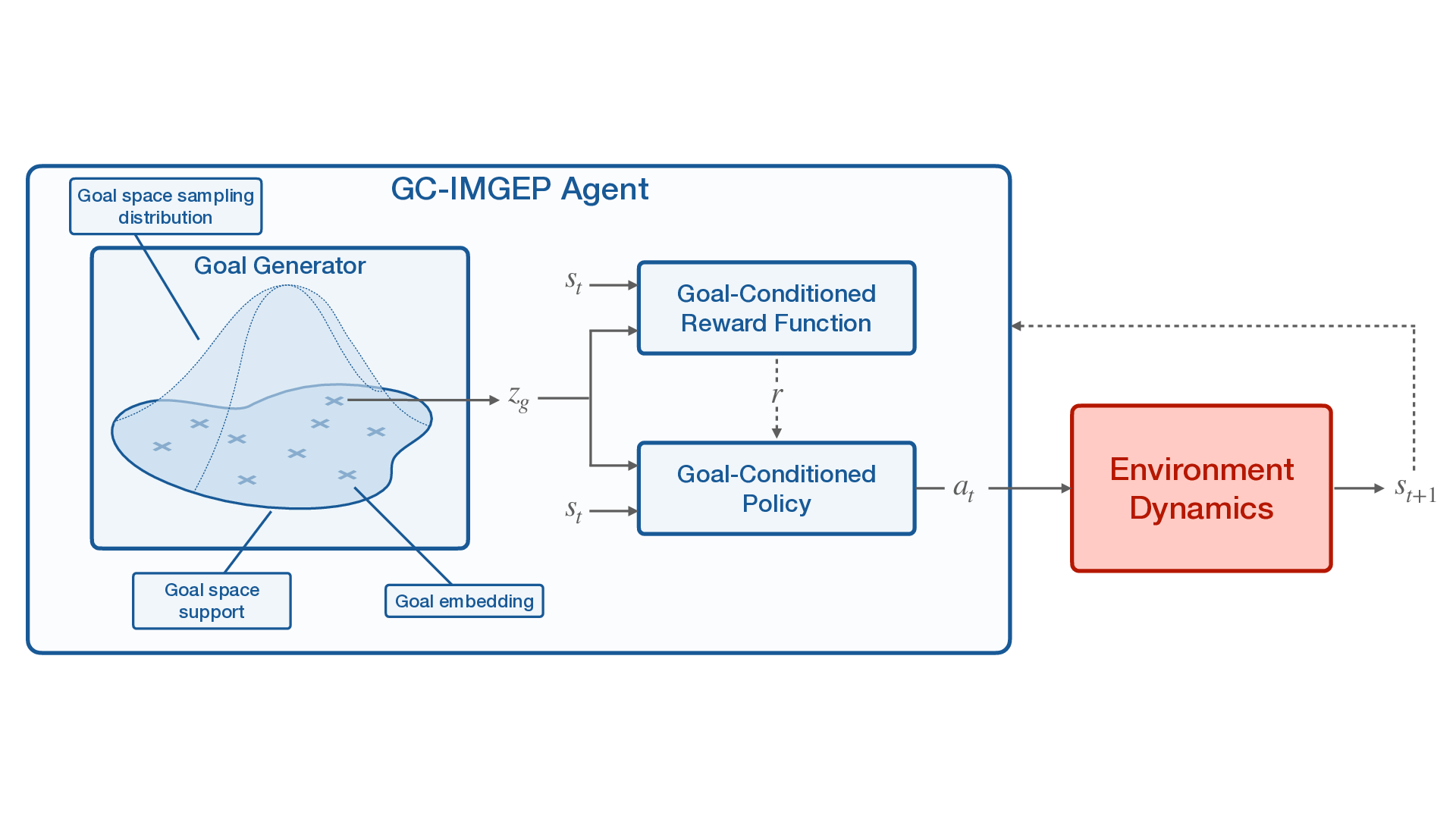
8.2.2 Intrinsically Motivated Exploration of Learned Goal Spaces
Participants: Adrien Laversanne-Finot [correspondant], Pierre-Yves Oudeyer.
Finding algorithms that allow agents to discover a wide variety of skills efficiently and autonomously, remains a challenge of Artificial Intelligence. Intrinsically Motivated Goal Exploration Processes (IMGEPs) have been shown to enable real world robots to learn repertoires of policies producing a wide range of diverse effects. They work by enabling agents to autonomously sample goals that they then try to achieve. In practice, this strategy leads to an efficient exploration of complex environments with high-dimensional continuous actions. Until recently, it was necessary to provide the agents with an engineered goal space containing relevant features of the environment. In this article we show that the goal space can be learned using deep representation learning algorithms, effectively reducing the burden of designing goal spaces. Our results pave the way to autonomous learning agents that are able to autonomously build a representation of the world and use this representation to explore the world efficiently. We present experiments in two environments using population-based IMGEPs. The first experiments are performed on a simple, yet challenging, simulated environment. Then, another set of experiments tests the applicability of those principles on a real-world robotic setup, where a 6-joint robotic arm learns to manipulate a ball inside an arena, by choosing goals in a space learned from its past experience. This work was published in 40
8.2.3 GRIMGEP: Learning Progress for Robust Goal Sampling in Visual Deep Reinforcement Learning
Participants: Grgur Kovač [correspondant], Adrien Laversanne-Finot, Pierre-Yves Oudeyer.
Autonomous agents, using novelty based goal exploration, are often efficient in environments that require exploration. However, they get attracted to various forms of distracting unlearnable regions. To address this problem, Absolute Learning Progress (ALP) has been used in reinforcement learning agents with predefined goal features and access to expert knowledge. This work extends those concepts to unsupervised image-based goal exploration.
We present the GRIMGEP framework: it provides a learned robust goal sampling prior that can be used on top of current state-of-the-art novelty seeking goal exploration approaches, enabling them to ignore noisy distracting regions while searching for novelty in the learnable regions. It clusters the goal space and estimates ALP for each cluster. These ALP estimates can then be used to detect the distracting regions, and build a prior that enables further goal sampling mechanisms to ignore them.
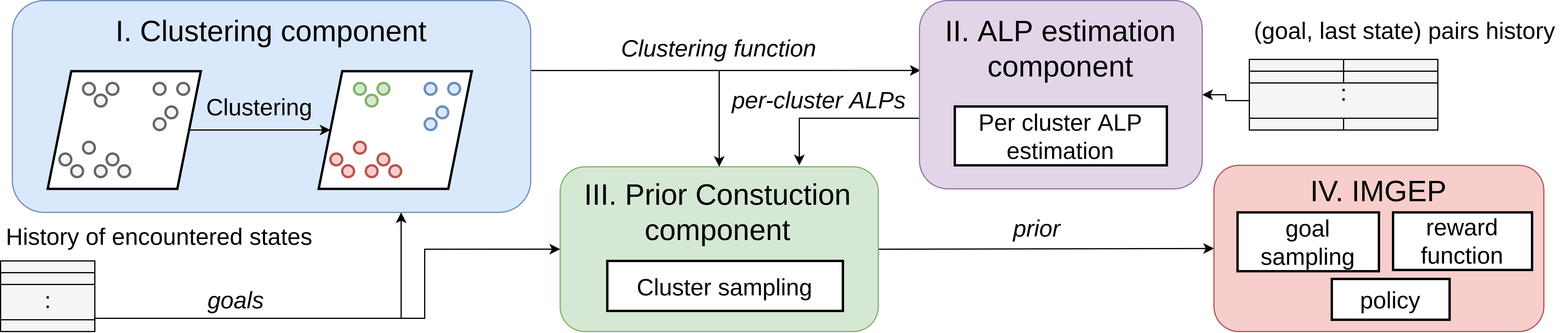
We construct an image based environment with distractors, on which we show that wrapping current state-of-the-art goal exploration algorithms with our framework allows them to concentrate on interesting regions of the environment and drastically improve performances.
In our experiments shown on figure 6, we compare the performance of two novelty-based exploration approaches: Countbased, and Skewfit (with two different values of its hyperparameter ). We can see that wrapping all baselines with the GRIMGEP framework drastically improves their performance.
0.25 |
0.25 |
0.25 |
(a) b | (b) b | (c) b |
This work is available as a preprint in 76, and the source code is available at https://gitlab.com/Grg/grimgep.
8.2.4 Language Augmented Intrinsically Motivated Agents
Participants: Cédric Colas [correspondant], Tristan Karch, Pierre-Yves Oudeyer.
Language as a Cognitive Tool to Imagine Goals in Curiosity-Driven Exploration
In this project, we investigate how autonomous multi-goal reinforcement learning agents can use language as a cognitive tool in order to creatively explore their environment and grow repertoires of skills. We follow a developmental approach inspired by how children learn to manipulate language, using it as a way to represent goals and to make plans in their heads. We developped this general vision in this blog post: http://developmentalsystems.org/language_as_cognitive_tool_vygotskian_rl.
We develop an algorithm called IMAGINE 9 enabling an intrinsically motivated agent to build a repertoire of skills only from natural language descriptions given by a Social Partner. In our setup, the agent starts without knowing any potential goal and acts randomly. As it reaches outcomes that are meaningful for the social partner, the social partner provides descriptions of the scene in natural language. The agent then converts these natural descriptions into targetable goals and learns to reach them.
This new learning algorithm offers several benefits over previous intrinsically motivated multi-goal reinforcement learning agents that do not use language to describe goals.
First, using linguistic descriptions as sole supervision helps get rid of the need to define hand-crafted reward functions for each of the reachable goals in the environment. In curious, for instance, the agent needed to have access to the description of each of the goal types as well as their associated reward functions in order to reach them. In IMAGINE, the agent builds its own internal reward function mapping natural language descriptions to binary rewards and uses this signal to train a goal-conditioned policy.
Second, using language to represent goals enables the agent to leverage language compositionality so as to imagine new goals, assembling pieces of descriptions communicated by the social partner in order to form new targetable goals. For instance, consider an agent that received the following descriptions: “Grasp red cat”, “Grow red cat” and “Grasp red plant”. This agent can imagine the goal “Grow red plant” and use it as a target in order to discover new outcomes in its environment. We call this mechanism goal imagination. We argue that goal imagination is key to be able to make creative discoveries because the corresponding targeted behaviors are out of the distribution of the outcomes communicated by the social partner. This sort of out-of-distribution goal generation can only be achieved with goals represented as language.
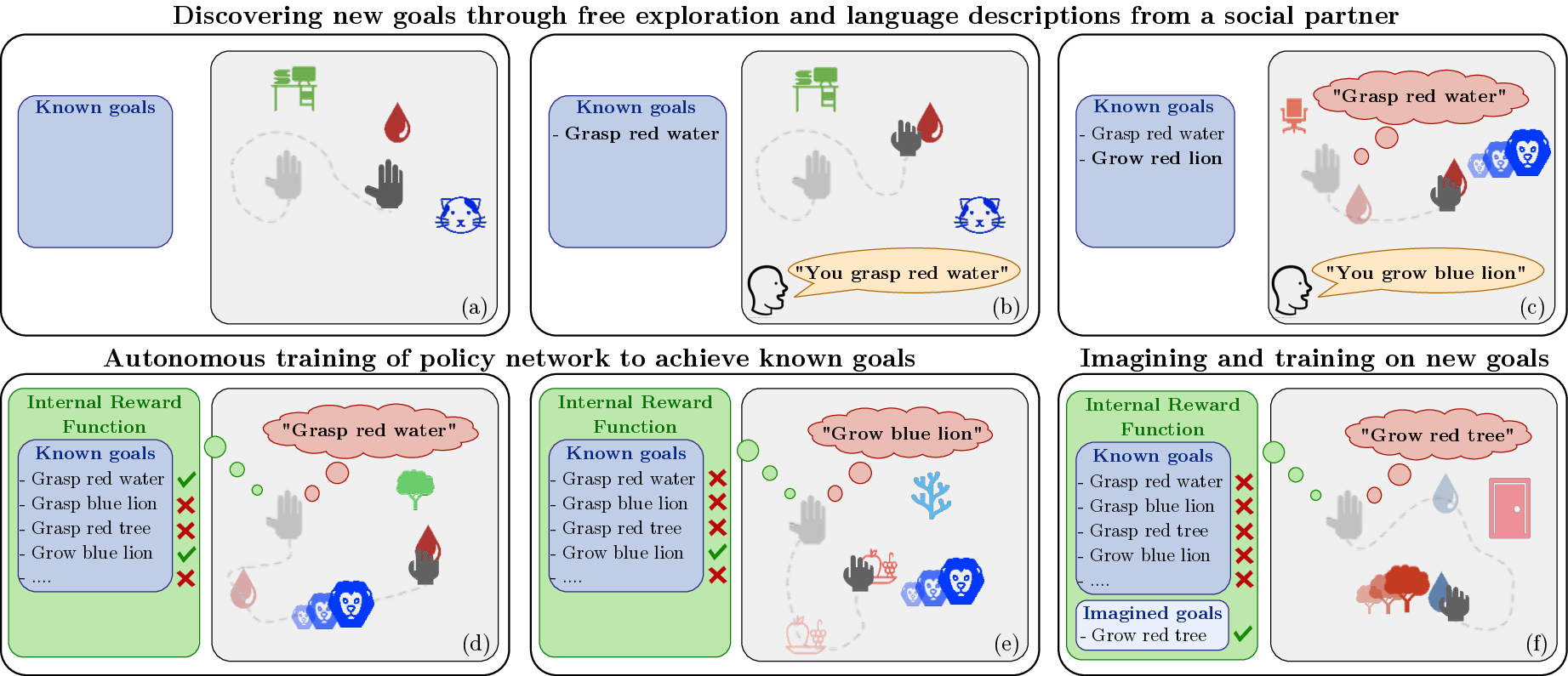
We carried out experiments in order to evaluate the benefits from goal imagination in intrinsically motivated learning. Experiments are split into two phases. In the first one, the agent interacts with the social partners, collects descriptions of goals and stores them in a set of known goal descriptions. The agent uses these descriptions paired with its observations in order to learn an internal reward function that detects when the goal represented by the descriptions are achieved in a given scene. Once this internal reward function is obtained, the agent uses its output (the reward signal) in order to train a goal-conditioned policy enabling it to reach any goal.
In the second phase, the social partner disappears and the agent starts imagining new goals by composing the descriptions stored in the set of known goals. The agent then targets these new goals and by doing so, discovers new interactions. This creative goal exploration process can only be efficient if imagined goal descriptions have a sufficient probability to be meaningful in the environment. As a result, we leveraged the construction grammar framework used to model child language acquisition with discovery of word equivalence classes in order to make sure that imagined goals follow the same construction rules as the descriptions communicated by the social partner. It is also important to note, that in order for goal imagination to work, the internal reward function trained from the social partner’s description must generalize. In other words, it should be able to detect if imagined goals are reached without receiving any new description from the social partner. To this end, we developed an object-factored learning architecture coupled with attention mechanisms 59 that facilitates generalization to new descriptions.
Finally, we measured the success rate of agents on a wide set of different skills and observed that agents that do not imagine goals (that stop at phase 1) master a smaller set of skills than agents that do imagine goals.
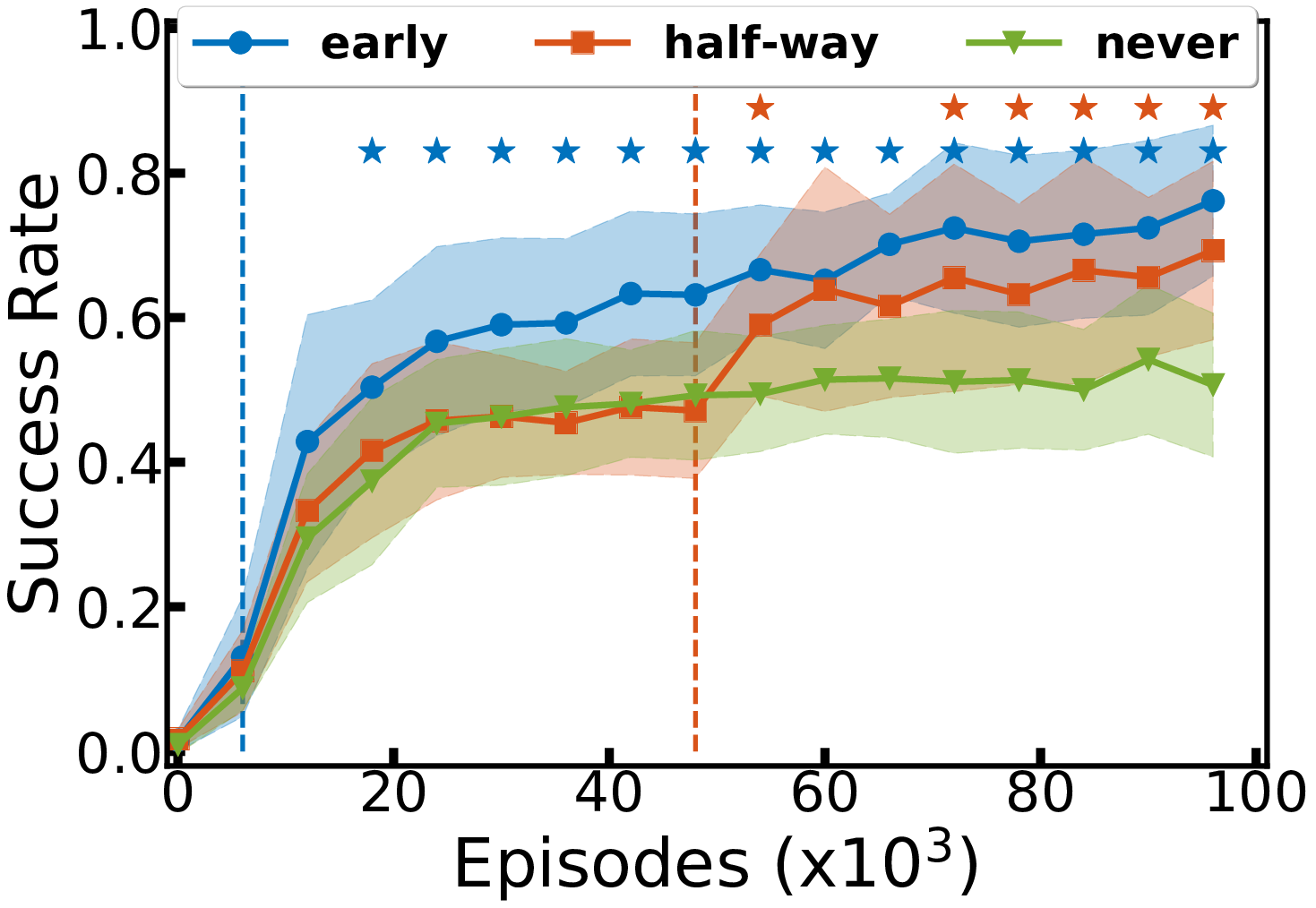
Grounding Language to Autonomously-Acquired Skills via Goal Generation
.
We are interested in the autonomous acquisition of repertoires of skills. Language-conditioned reinforcement learning (lc-rl) approaches are great tools in this quest, as they allow us to express abstract goals as sets of constraints on the states. However, most lc-rl agents are not autonomous and cannot learn without external instructions and feedback. Besides, their direct language condition cannot account for the goal-directed behavior of pre-verbal infants and strongly limits the expression of behavioral diversity for a given language input. To resolve these issues, we propose a new conceptual approach to language-conditioned rl: the Language-Goal-Behavior architecture (lgb). lgb decouples skill learning and language grounding via an intermediate semantic representation of the world—see Figure 9. To showcase the properties of lgb, we present a specific implementation called decstr. decstr is an intrinsically motivated learning agent endowed with an innate semantic representation describing spatial relations between physical objects–see Figure 10. In a first stage (gb), it freely explores its environment and targets self-generated semantic configurations. In a second stage (lg), it trains a language-conditioned goal generator to generate semantic goals that match the constraints expressed in language-based inputs. We showcase the additional properties of lgb w.r.t. both an end-to-end lc-rl approach and a similar approach leveraging non-semantic, continuous intermediate representations. Intermediate semantic representations help satisfy language commands in a diversity of ways, enable strategy switching after a failure and facilitate language grounding. This project led to a publication in the ICLR conference proceeding 70, 47.
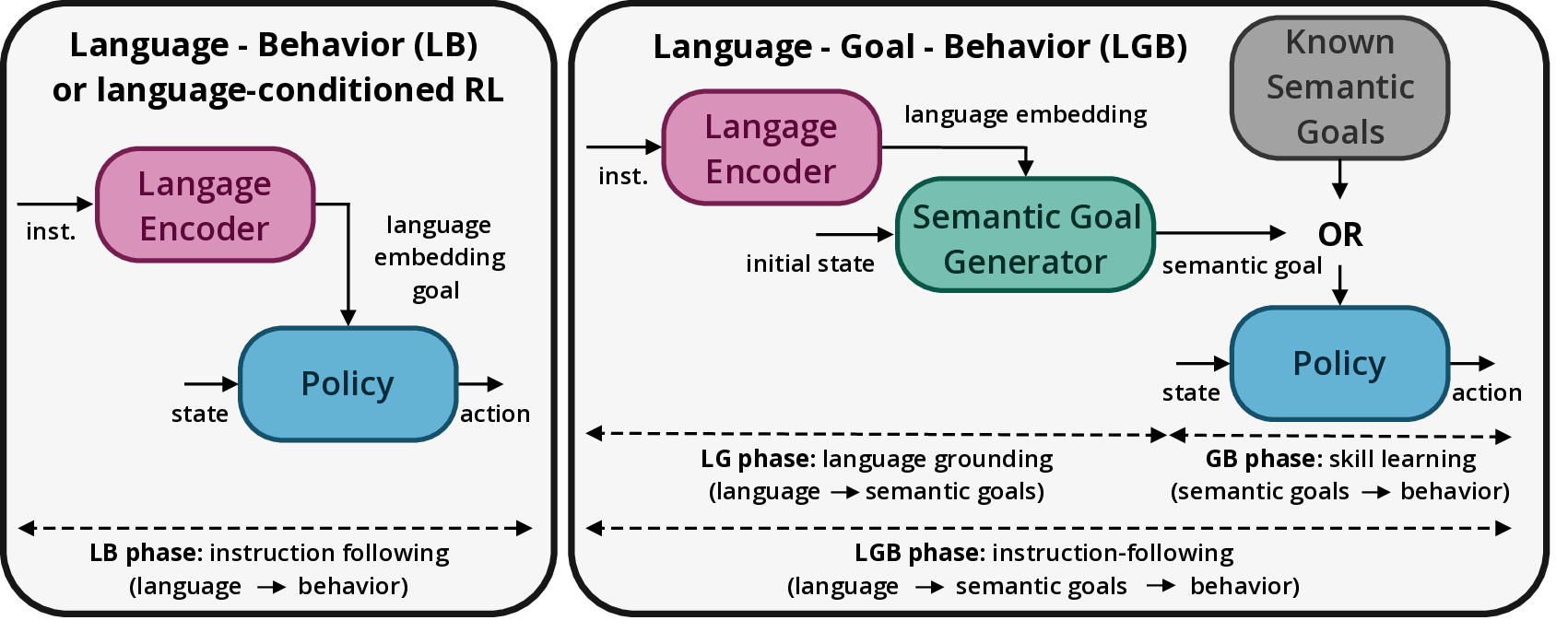
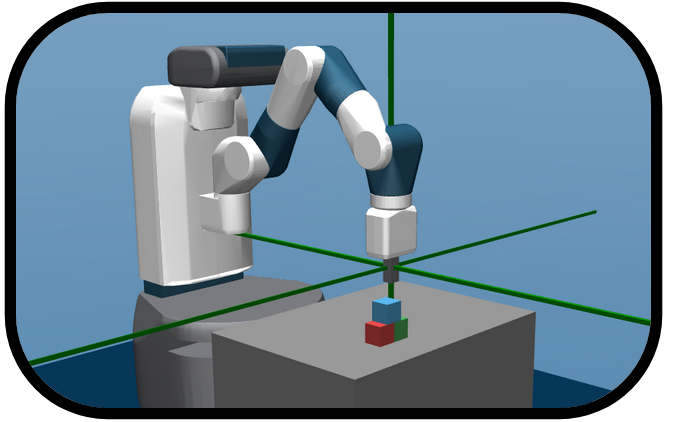
8.2.5 Grounding Spatio-Temporal Language with Transformers
Participants: Tristan Karch, Laetitia Teodorescu, Katja Hofmann, Pierre-Yves Oudeyer.
The previous study on IMAGINE revealed the powerful use of language as a cognitive tool in intrinsically-motivated agents. However, both the language considered in the study and the states this language is grounded in are very simple. The language only contains predicates that are verifiable by looking at an instantaneous state: this is unrealistic when we consider that natural language often describes actions that occur over several time steps: think of dancing, giving, waiting; these are actions that need to be observed over several time steps have their truth values decided. Similarly, another aspect of language that wasn't represented in the IMAGINE language space concerned spatial relations between objects: humans naming objects, use the surrounding spatial context to uniquely identify the reference they are speaking about, especially in ambiguous cases where a word could refer to several objects. Another temporal aspect of language that was not represented in the IMAGINE study is the past tense: in natural language humans are able to indicate whether the actions they are describing are happening right now or have happened in the past. These limitations have motivated us to define and systematically study a form of simplified spatio-temporal language. Since this language describes actions unfolding over several time steps, we need to ground it in time-extended traces of the behavior of an agent, e.g. the observation needs to be an entire trajectory instead of simply an end-state. This grounding problem thus raises the issue of what neural architecture to use for language grounding.
In this work, we frame the language grounding problem as a classification problem: the problem of learning whether a given trajectory and linguistic description match or not (See Figure 11 for a graphical overview of the problem). Alternatively this can be seen as the problem of learning a reward function over temporally-states and language. We place ourselves in the 2d environment described in 9; and we define a synthetic spatio-temporal language composed of:
- Temporal predicates (such as grow and shake);
- Spatial relations (such as grasp thing left of dog);
- Past-tense predicates, indicated through the was modifier (such as was grasp plant for describing a past-tense version of the predicate grasp);
- Past-tense spatial relations, for indicating spatial relations that were true in the past but no longer in the present due to motion of objects (such as shake thing was left of dog for referring to an object that was to the left of a dog.)
Since grounding this language is a relational problem, we use variants of a relational architecture 95 to tackle it. We represent our inputs as the union of a set of linguistic tokens, representing the input linguistic description, with a set of vectors representing object features over time, representing temporally-extended observations. Our output is a single number that is 1 when the description matches the state and 0 otherwise. To process these inputs and produce our output we instantiate three architectures based on Transformers with different inductive biases and evaluate which ones are best for this problem. See Figure 12 for a visual illustration of our input and output space, as well as the architectures used.
We split our language descriptions in 4 categories: Base, Spatial, Temporal and Spatio-temporal and evaluate our models on a set of randomly withheld test descriptions for each category (random split). We additionally evaluate our models on a series of systematic splits where certain word combinations are forbidden in the train set. See Figures 13 for the results on the random split and 14 for results on the systematic split. Overall, we find that the Unstructured Transformer variant performs consistently best over all types of splits, followed by the Temporal-first architecture; this suggests that a form of object permanence in object processing (the model being able to relate successive temporal observations of objects between them with self-attention) is necessary in learning to ground spatio-temporal language.
This work was presented at Neurips 2021.
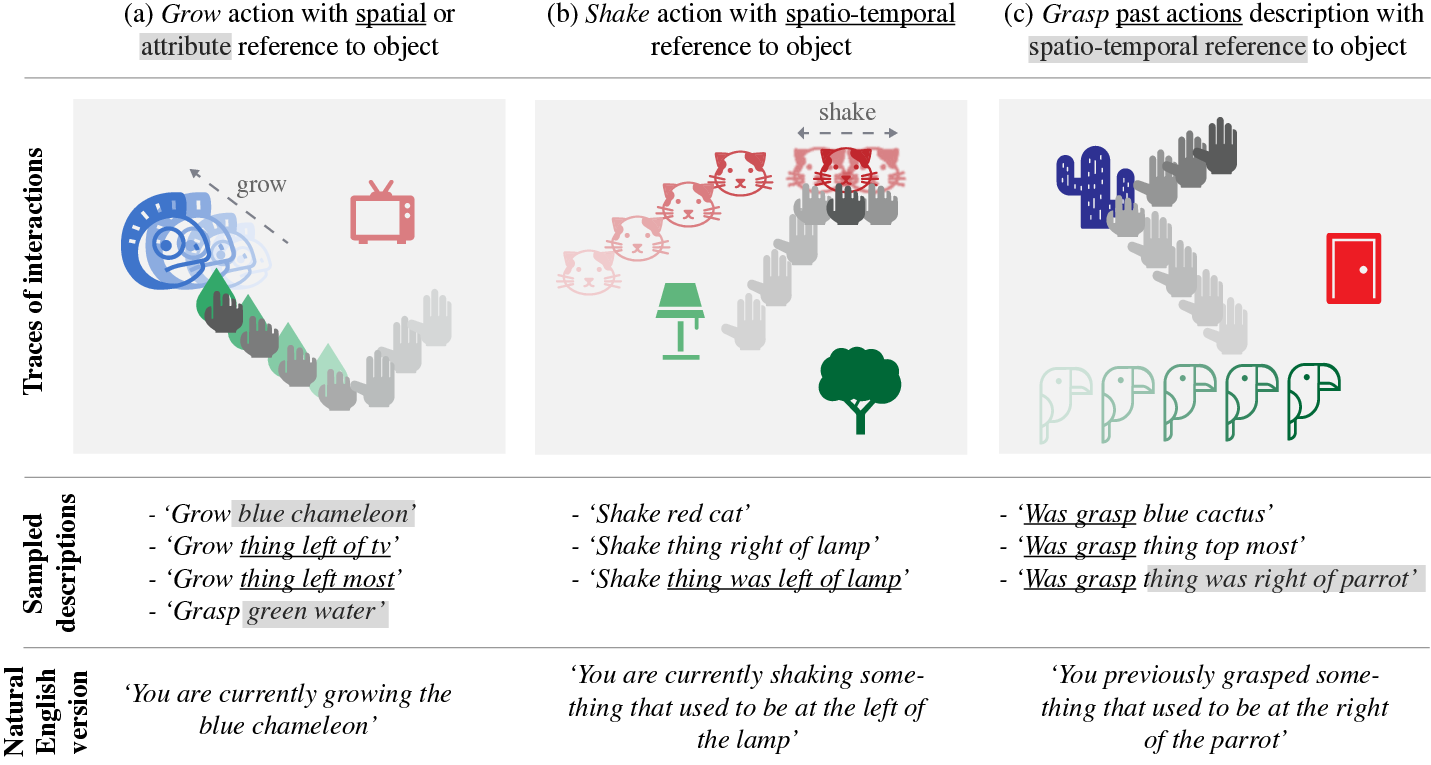
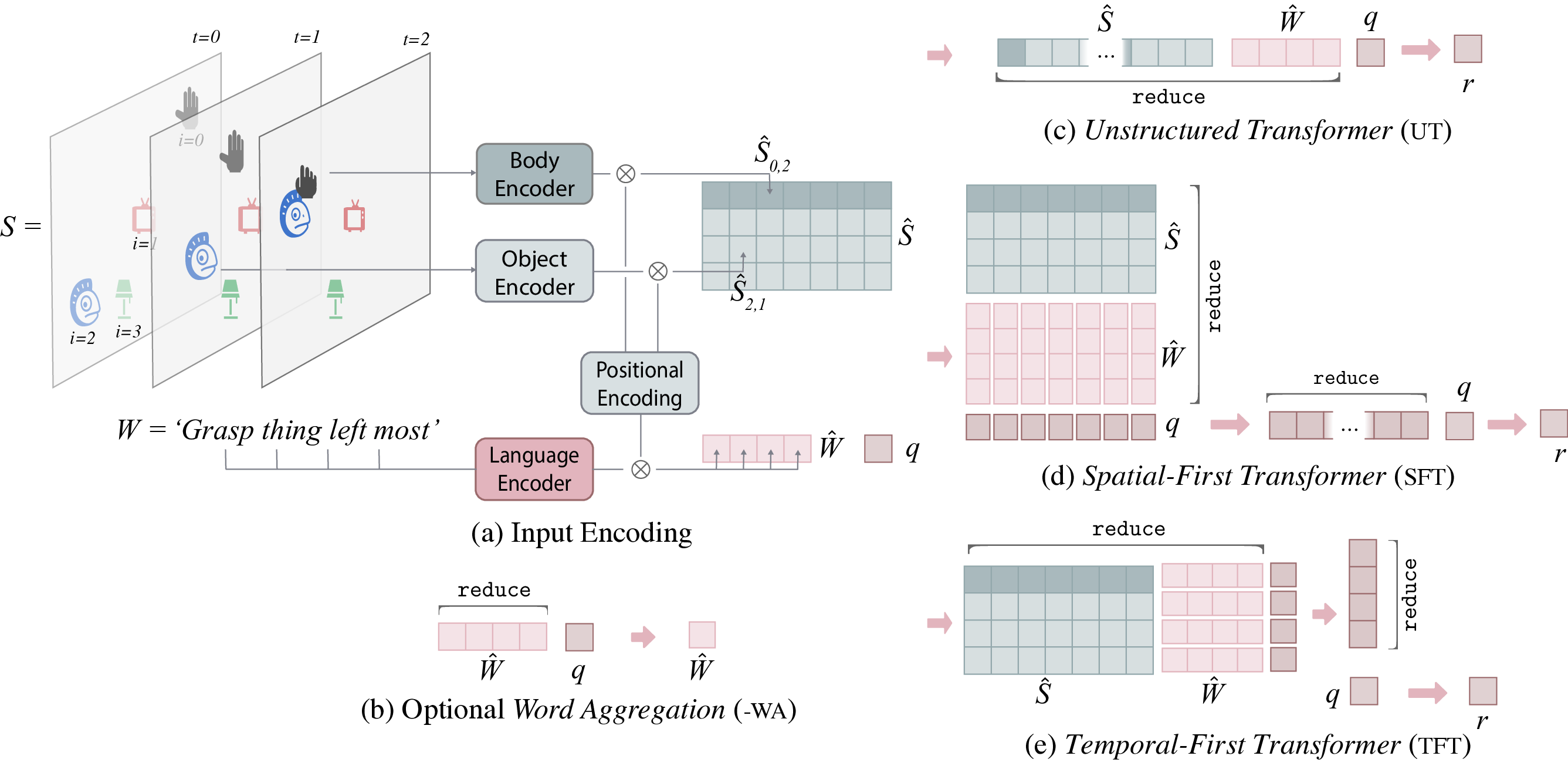
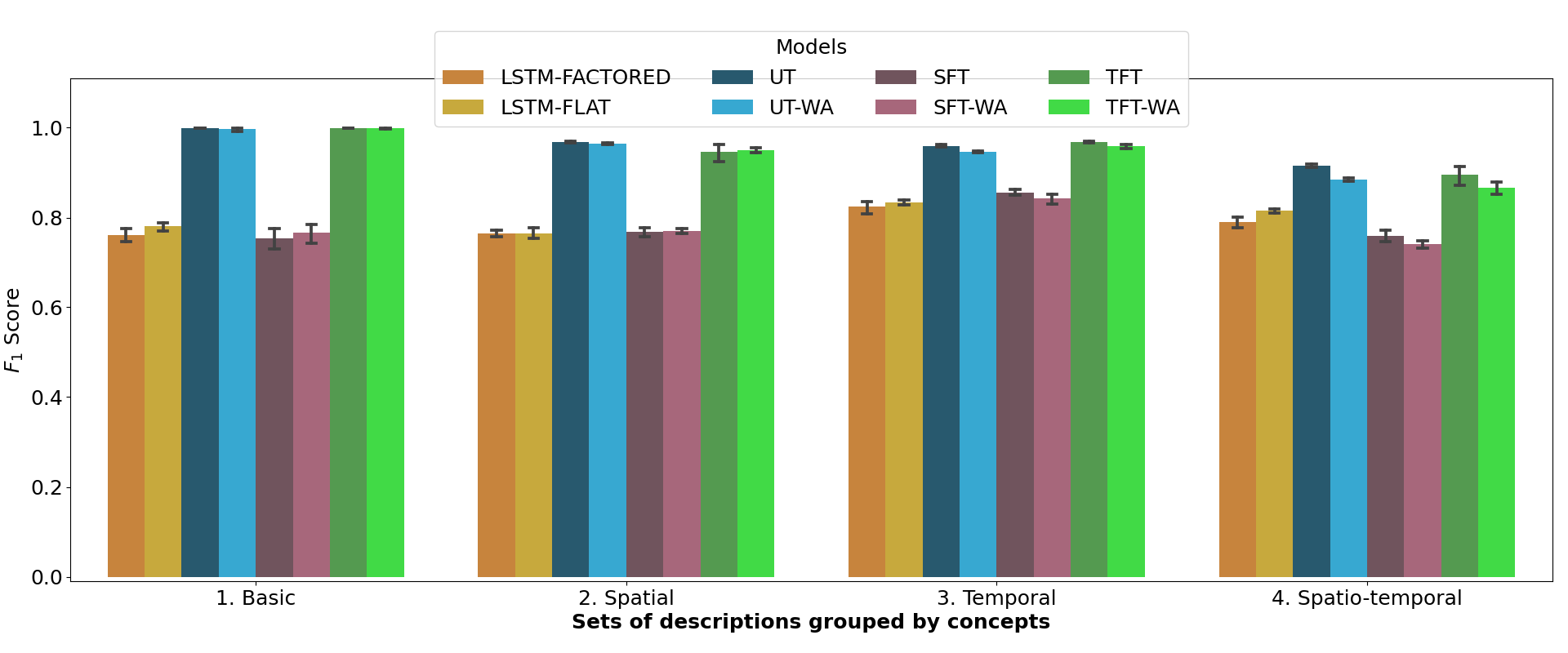
Generalization F1F_{1} scores for the random split of all language categories.
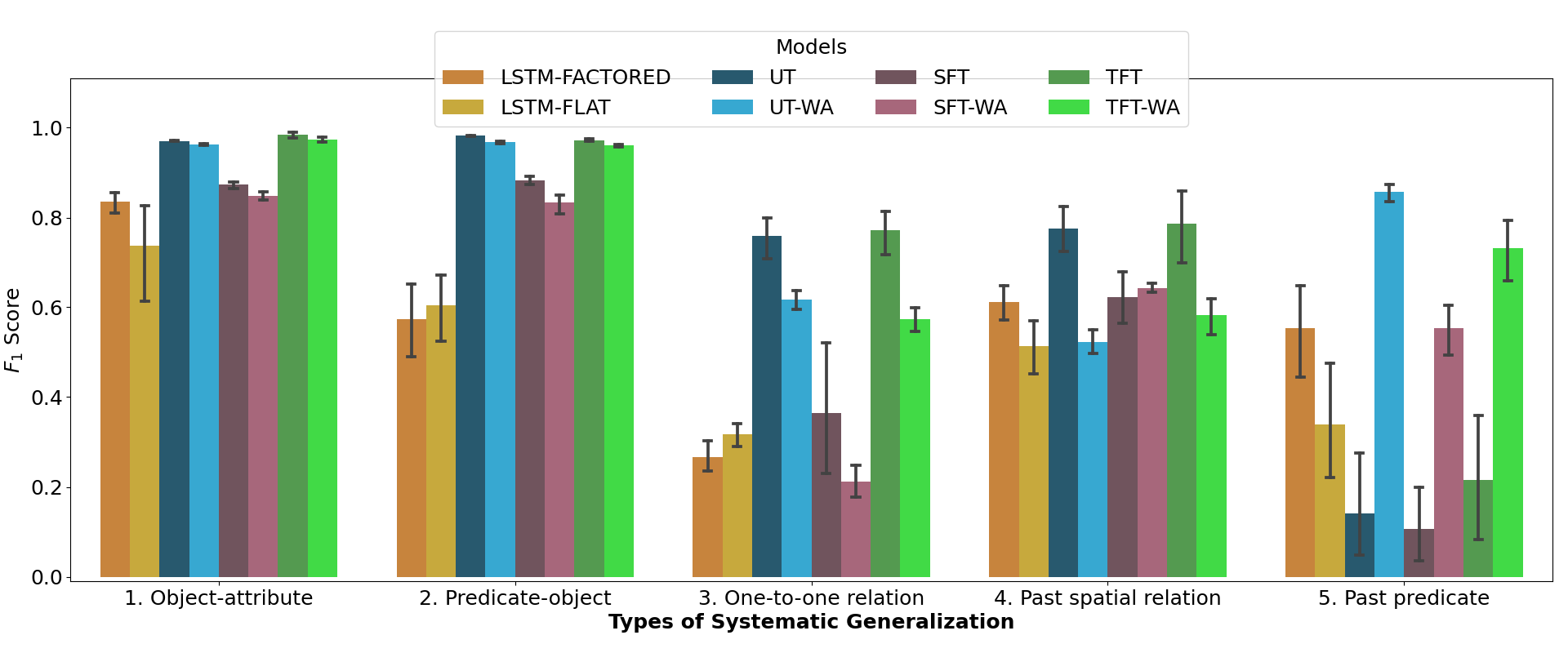
Generalization F1F_{1} scores for the systematic split.
8.2.6 Intrinsically Motivated Open-Ended Multi-Task Learning Using Transfer Learning to Discover Task Hierarchy
Participants: Nicolas Duminy [correspondant], Sao Mai Nguyen [correspondant], Junshuai Zhu, Dominique Duhaut, Jerome Kerdreux.
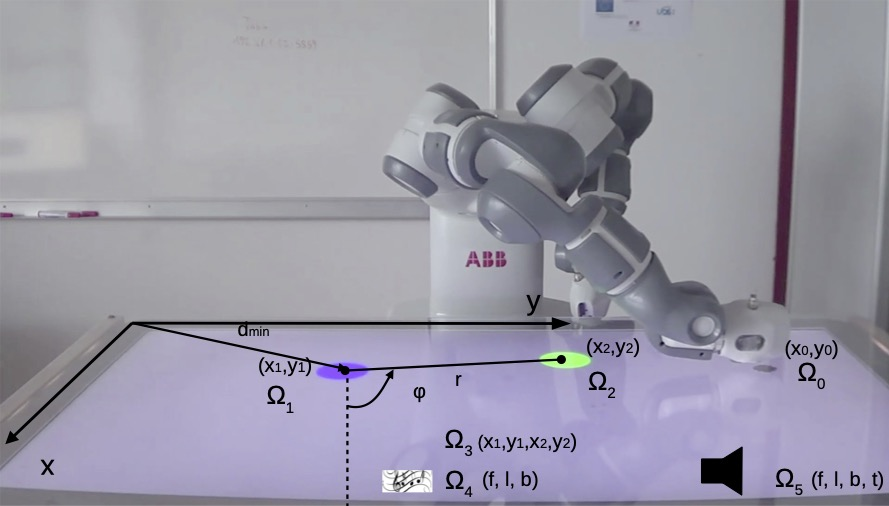
In open-ended continuous environments, robots need to learn multiple parameterized control tasks in hierarchical reinforcement learning. We hypothesize that the most complex tasks can be learned more easily by transferring knowledge from simpler tasks, and faster by adapting the complexity of the actions to the task. We propose a task-oriented representation of complex actions, called procedures, to learn online task relationships and unbounded sequences of action primitives to control the different observables of the environment. Combining both goal-babbling with imitation learning, and active learning with transfer of knowledge based on intrinsic motivation, the algorithm SGIM-PB self-organizes its learning process. It chooses at any given time a task to focus on; and what, how, when and from whom to transfer knowledge. We show with a industrial robot arm with a simulation and in real-life (see Figure 15), in cross-task and cross-learner transfer settings, that task composition is key to tackle highly complex tasks. Task decomposition is also efficiently transferred across different embodied learners and by active imitation, where the robot requests just a small amount of demonstrations and the adequate type of information. The robot learns and exploits task dependencies so as to learn tasks of every complexity.
This work lead to a publication in MDPI Applied Sciences 115.
8.3 Object-Based and Relational Representations for Autonomous Agents
Participants: Laetitia Teodorescu [correspondant], Tristan Karch, Cedric Colas, Katja Hoffman, Pierre-Yves Oudeyer.
In deep reinforcement learning, especially in approaches operating in symbolic observation spaces (the inputs are not images but the list of all object's x-y positions for instance), it is common to feed the agent's networks with a vector of the concatenation of all the symbolic features. However, in practice there is a lot of redundant structure in this observation space: if the first object has a feature describing it as "red" or if the second object has a feature describing it as "red", there should be a prior (or inductive bias) in the architecture reflecting the fact that these two situations should be processed in the same way. All objects share the same semantics no matter in what order they are listed. We can call this the object-centered prior. In addition to that, for acting on collections of objects, an agent often has to process information about the relations between objects. We can call this the relational prior (or inductive bias). A detailed discussion of these inductive biases can be found in 95.
8.3.1 Relational inductive biases for recognizing configurations of objects
Since the structure "objects + relations" is naturally present in the world, a good idea is to implement it into the neural networks we are training. Set structures can be used for representing collections of objects, and the Deep Set architecture is well-suited for learning on sets. Graph structures can be used for representing collections of objects and their relations; the Graph Neural Network (GNN) family is well-suited for learning on graphs. Additionally, we should observe differences between performance and sample efficiency of architectures having only the object-centered prior versus the ones that have the object-centered and relational priors in tasks that require processing of relational information.
We have tested this hypothesis in the case of learning to recognize spatial configurations of symbolic objects. For this purpose, we have created a benchmark dataset called SpatialSim that defines two tasks. The first task, called Identification, is learning to recognize a reference configuration of objects (up to an affine transformation) from a scene with the same objects but with their positions randomly reshuffled. The second task, called Comparison, consists in comparing two different configurations of objects and deciding if they are the same (up to an affine transformation).
In this context, we have trained architectures implementing increasing levels of relational computation: Deep Sets, Recurrent Deep Sets and Message-Passing GNNs. We have observed that the models with more relational computation perform better, especially in the Comparison task where Deep Set performance is very poor. This suggests that relational models are crucial for learning to compare configurations of objects.
This work has been presented as a spotlight talk at the Bridge Between Perception and Reasoning, Graph Neural Networks and Beyond workshop at ICLR 2020 83.
8.3.2 Extracting object representations from images
The previous work was concerned with symbolic objects described by their features such as position, orientation, etc. In a realistic setting we need to be able to learn to extract these object representations directly from raw images in an unsupervised representation learning scheme, and in a disentangled manner, such that each object is represented by a unique vector, and that each of that vector's coordinates represents a unique factor of variation (such as x or y position, color, etc). In the best case, this would recover the symbolic representations such as the ones used in the approach above.
Two architectures for object-centered unsupervised representation learning have been investigated: MONet 101 (an object-based variational autoencoder) and Contrastive-Structured World Models 139 (an architecture learning to extract objects from images by learning a world model expressed as an interaction graph). Integrating these approaches (along with mechanisms for object permanence) into an intrinsically motivated deep RL setting is still ongoing work.
8.3.3 In language-conditioned Deep RL agents
The impact of object-centered architectures in a deep RL setting has also been investigated. We have benchmarked their importance in the language-imagination deep rl setting given in 8.2.4. We have observed dramatic improvements in sample efficiency in this setting when we use Deep Sets as opposed to flat, unstructured architectures (such as regular Multi-Layer Perceptrons).
In addition to that, we observe increased generalization performance in this setting (see Figures 16 and 17), suggesting that the bias that all objects should be represented and processed in the same way (and the weight-sharing that is implied by this bias in the neural networks) is helpful for transferring skills across objects.
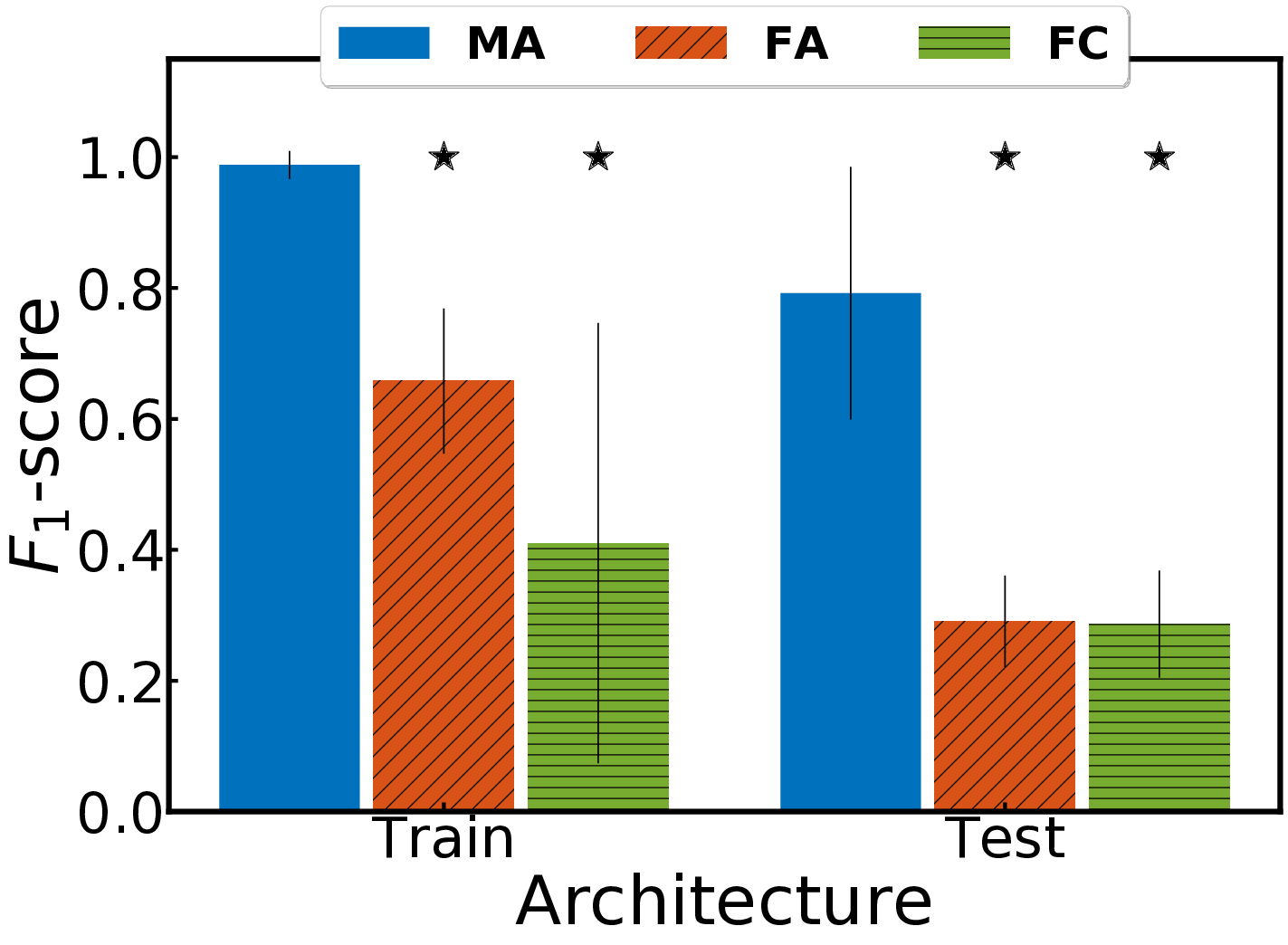
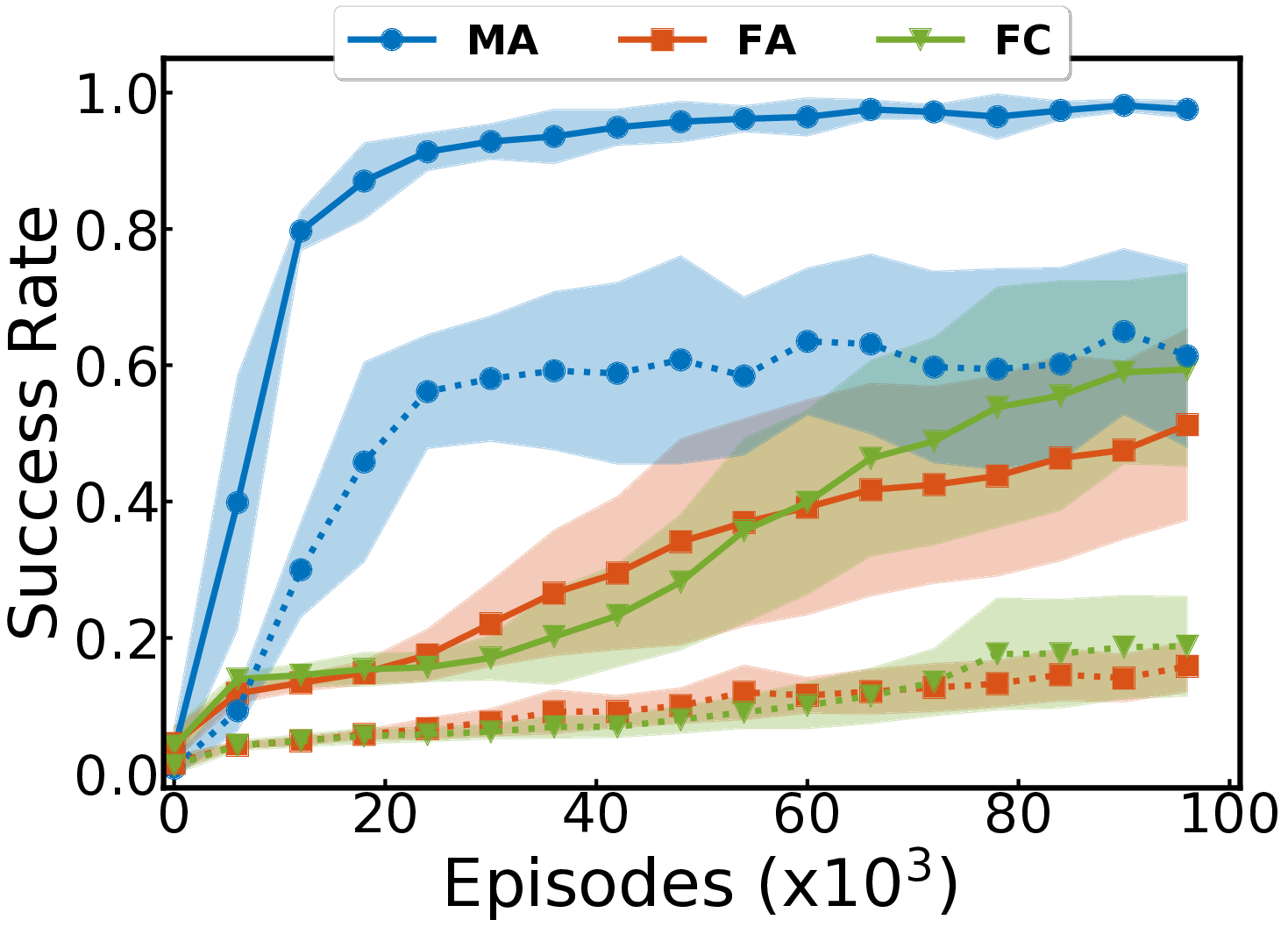
These object-based architectures are robust to the number of objects, contrary to their flat counterparts. Additionally, architectures that present biases for encoding relations between objects demonstrate increased performance in tasks that require interaction between objects, such as grasping objects that are identified by their position relative to another object.
This work was presented at the Beyond Tabula Rasa in RL ICLR 2020 workshop 59.
8.4 Automatic Curriculum Learning in Deep RL
8.4.1 Teacher algorithms for curriculum learning of Deep RL in continuously parameterized environments
Participants: Remy Portelas [correspondant], Katja Hoffman, Pierre-Yves Oudeyer.
In this work we considered the problem of how a teacher algorithm can enable an unknown Deep Reinforcement Learning (DRL) student to become good at a skill over a wide range of diverse environments. To do so, we studied how a teacher algorithm can learn to generate a learning curriculum, whereby it sequentially samples parameters controlling a stochastic procedural generation of environments. Because it does not initially know the capacities of its student, a key challenge for the teacher is to discover which environments are easy, difficult or unlearnable, and in what order to propose them to maximize the efficiency of learning over the learnable ones. To achieve this, this problem is transformed into a surrogate continuous bandit problem where the teacher samples environments in order to maximize absolute learning progress of its student. We presented ALP-GMM (see figure 18), a new algorithm modeling absolute learning progress with Gaussian mixture models. We also adapted existing algorithms and provided a complete study in the context of DRL. Using parameterized variants of the BipedalWalker environment, we studied their efficiency to personalize a learning curriculum for different learners (embodiments), their robustness to the ratio of learnable/unlearnable environments, and their scalability to non-linear and high-dimensional parameter spaces. Videos and code are available at https://github.com/flowersteam/teachDeepRL.
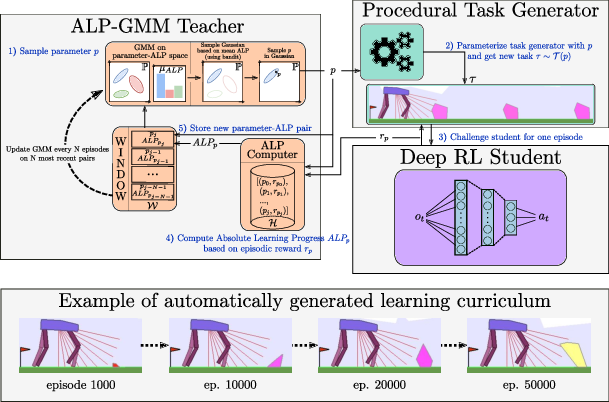
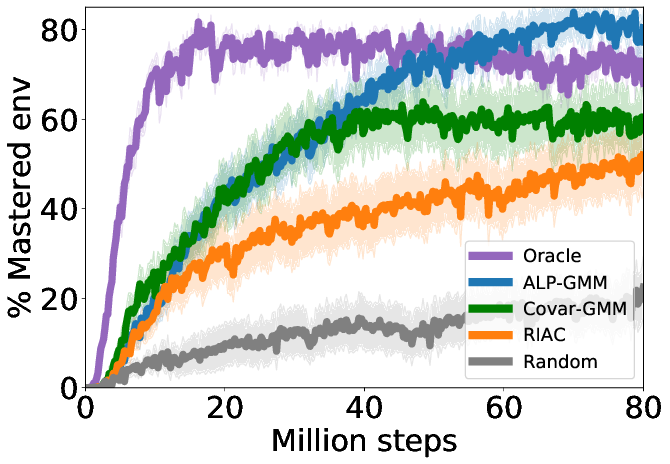
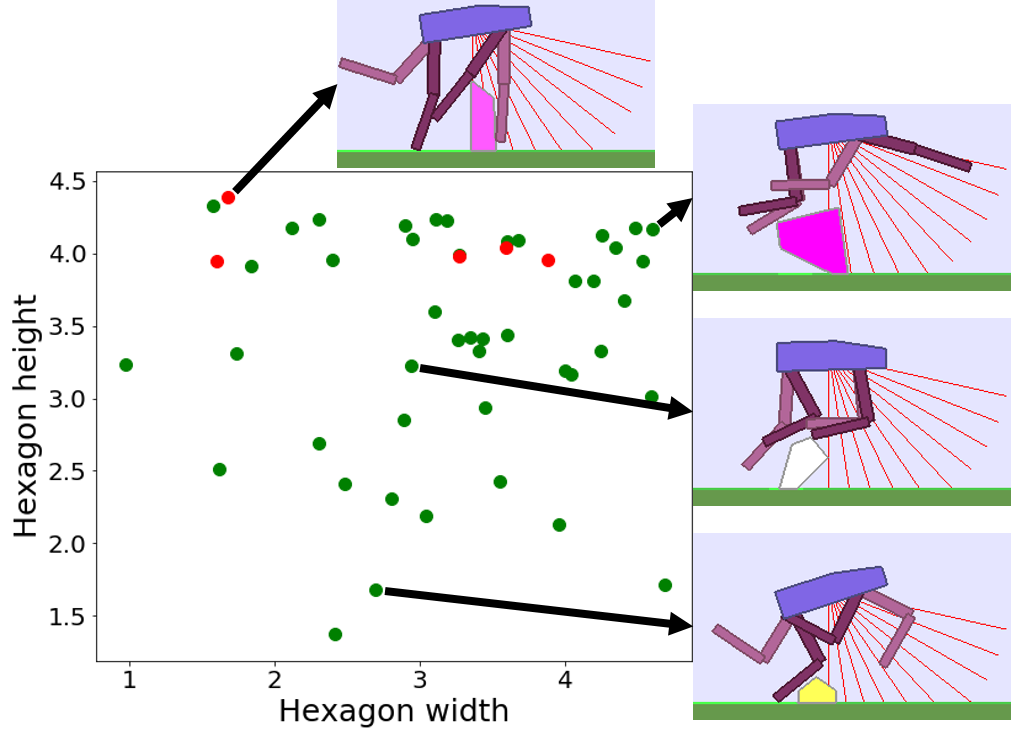
Overall, this work demonstrated that LP-based teacher algorithms could successfully guide DRL agents to learn in difficult continuously parameterized environments with irrelevant dimensions and large proportions of unfeasible tasks. With no prior knowledge of its student's abilities and only loose boundaries on the task space, ALP-GMM, our proposed teacher, consistently outperformed random heuristics and occasionally even expert-designed curricula (see figure 19). This work was presented at CoRL 2019 25.
ALP-GMM, which is conceptually simple and has very few crucial hyperparameters, opens-up exciting perspectives inside and outside DRL for curriculum learning problems. Within DRL, it could be applied to previous work on autonomous goal exploration through incremental building of goal spaces 19. In this case several ALP-GMM instances could scaffold the learning agent in each of its autonomously discovered goal spaces. Another domain of applicability is assisted education, for which current state of the art relies heavily on expert knowledge 109 and is mostly applied to discrete task sets.
8.4.2 Meta Automatic Curriculum Learning
Participants: Remy Portelas [correspondant], Clement Romac, Katja Hoffman, Pierre-Yves Oudeyer.
In this work we identified that a major challenge in the Deep RL (DRL) community is to train agents able to generalize their control policy over situations never seen in training. Training on diverse tasks has been identified as a key ingredient for good generalization, which pushed researchers towards using rich procedural task generation systems controlled through complex continuous parameter spaces. In such complex task spaces, it is essential to rely on some form of Automatic Curriculum Learning (ACL) to adapt the task sampling distribution to a given learning agent, instead of randomly sampling tasks, as many could end up being either trivial or unfeasible. Since it is hard to get prior knowledge on such task spaces, many ACL algorithms explore the task space to detect progress niches over time, a costly tabula-rasa process that needs to be performed for each new learning agents, although they might have similarities in their capabilities profiles.
To address this limitation, we introduced the concept of Meta-ACL (see fig. 20, and formalized it in the context of black-box RL learners, i.e. algorithms seeking to generalize curriculum generation to an (unknown) distribution of learners. We then presented AGAIN (see fig. 21), a first instantiation of Meta-ACL, and showcased its benefits for curriculum generation over classical ACL in multiple simulated environments including procedurally generated parkour environments with learners of varying morphologies. Videos and code are available at https://sites.google.com/view/meta-acl.
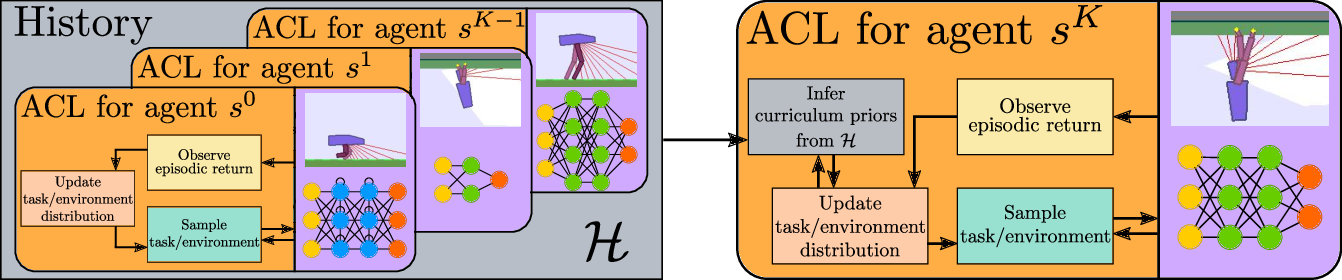
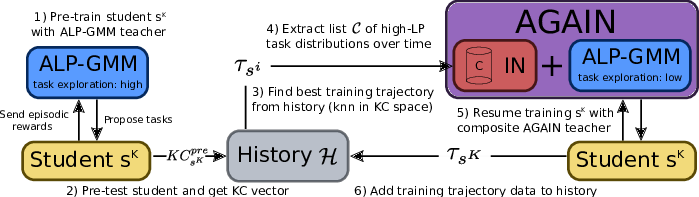
This work is available as preprint 79 and will be submitted to ICML 2021. In future work, AGAIN could be improved by using adaptive approaches to build compact pre-test sets, e.g. using decision tree based test pruning methods, or by combining curriculum priors from multiple previously trained learners. While AGAIN is built on top of an existing ACL algorithm, developing an end-to-end Meta-ACL algorithm that generates curricula using a DRL teacher policy trained across multiple students is also a promising line of work to follow. Additionally, this work opens-up exciting new perspectives in transferring Meta-ACL methods to educational data-mining, e.g. in MOOC scenarios, given a previously trained pilot classroom, one could use Meta-ACL to infer adaptive curricula for new students.
8.4.3 TeachMyAgent: a Benchmark for Automatic Curriculum Learning in Deep RL
Participants: Clement Romac [correspondant], Remy Portelas, Katja Hoffman, Pierre-Yves Oudeyer.
Training autonomous agents able to generalize to multiple tasks is a key target of Deep Reinforcement Learning (DRL) research. In parallel to improving DRL algorithms themselves, Automatic Curriculum Learning (ACL) study how teacher algorithms can train DRL agents more efficiently by adapting task selection to their evolving abilities. While multiple standard benchmarks exist to compare DRL agents, there is currently no such thing for ACL algorithms. Thus, comparing existing approaches is difficult, as too many experimental parameters differ from paper to paper.
In this work, we identify several key challenges faced by ACL algorithms. Based on these, we present TeachMyAgent, a benchmark of current ACL algorithms leveraging procedural task generation. It includes 1) challenge-specific unit-tests using variants of a procedural Box2D bipedal walker environment, and 2) a new procedural Parkour environment combining most ACL challenges, making it ideal for global performance assessment.
We then use TeachMyAgent to conduct a comparative study of representative existing approaches, showcasing the competitiveness of some ACL algorithms that do not use expert knowledge. We also show that the Parkour environment remains an open problem.
We open-source our environments, all studied ACL algorithms (collected from open-source code or re-implemented), and DRL students in a Python package available at https://github.com/flowersteam/TeachMyAgent. We provide a detailed documentation at http://developmentalsystems.org/TeachMyAgent/ and present our work in a paper accepted at ICML 2021 53.
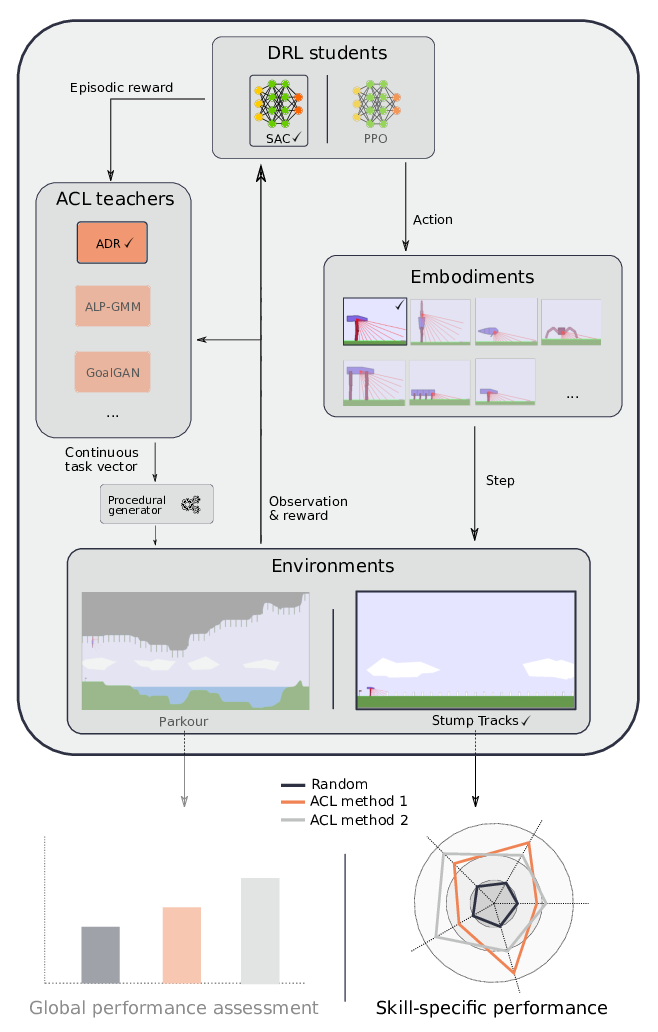
8.4.4 Other
Continual State Representation Learning via Self-Triggered Generative Replay
Participants: Hugo Caselles-Dupré [correspondant], David Filliat.
We consider the problem of building a state repre-sentation model for control, in a continual learning setting. As the environment changes, the aim is to efficiently compress the sensory state information without losing past knowledge, and then use Reinforcement Learning on the resulting features for efficient policy learning. To this end, we propose S-TRIGGER, a general method for Continual State Representation Learning applicable to Variational Auto-Encoders and its many variants. The method is based on Generative Replay, i.e. the use of generated samples to maintain past knowledge. It comes along with a statistically sound method for environment change detection, which self-triggers the Generative Replay. Our experiments on VAEs show that S-TRIGGER learns state representations that allows fast and high-performing Reinforcement Learning, while avoiding catastrophic forgetting. The resulting system has a bounded size and is capable of autonomously learning new information without using past data.
8.5 Multi-agent Reinforcement learning for Ecologically-valid Artificial Intelligence
8.5.1 Grounding Artificial Intelligence in the Origins of Human Behavior
Participants: Clément Moulin-Frier [correspondant], Eleni Nisioti, Julius Taylor.
Introduction
One of the most ambitious goal in Artificial Intelligence (AI) is the realization of a so-called Artificial General Intelligence (AGI), i.e. AI that is not limited to the realization of a predefined set of tasks but is able to generalize its capabilities to any cognitive task that can be solved by human intelligence. This is obviously a long-term objective but recent advances in AI have revived research in this field, with the vast majority of contributions focusing on
- new cognitive architectures and learning algorithms 178;
- new cost functions to be optimized 125 ;
- new databases to learn from 135
. However, although AGI is fundamentally related to the characteristics of human intelligence, research in this field rarely considers the processes that may have guided the emergence of complex cognitive capacities during the evolution of the species. Research in Human Behavioral Ecology (HBE) 98 seeks to understand how the behaviors characterizing human nature can be conceived as adaptive responses to major changes in the structure of our ecological niche. However, very little work in AI proposes to study how this long-term environmental dynamics can potentially guide and improve the acquisition of complex behaviors in artificial systems (see however recent contributions 192, including from our research group 25, 37). Moreover, to our knowledge, modern AI methods for learning behaviors in sequential environments have not yet been applied to test hypotheses in HBE (although it has been recently proposed 120).
An inter-disciplinary dialogue between AI and HBE
As a first step in our project, we conducted a targeted yet extensive literature review on HBE, in particular works studying the effect that climate complexity has had on the emergence of adaptability, cooperation and cultural repertoire in human evolution. In parallel, we have reviewed the state-of-the-art in the study of open-ended skill acquisition in, in particular, the AI sub-fields of multi-agent reinforcement and meta reinforcement learning. We have compiled our review in a position paper that summarizes the project's objectives 62. An important objective at this stage was to justify the proposed exchange of ideas between the two fields by identifying their commonalities in terms of research challenges. In Figure 23, we introduce a conceptual framework that recognizes important ecological components, as well as the feedforward and feedback links that relate them. In addition, we have derived the desiderata for an eco-valid simulation environment envisioned to enable the study of the whole spectrum of ecological hypotheses and worked towards implementing a grid-world with climate dynamics that model the ones hypothesized to have taken place during the birth of our own species 62. Figure 24 presents our design of the climate dynamics and the resource availability patterns that emerge based on them. We observe that the environments oscillates between periods of high and low resource variability instantiating conditions similar to the ones proposed by paleoclimatology data. This work was presented at the recent EcoRL workshop of the NEURips conference 62.
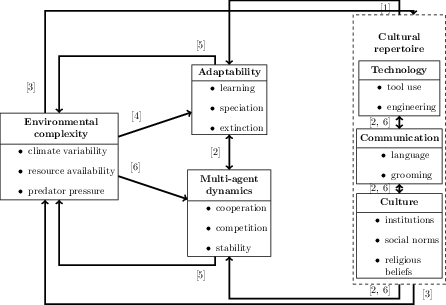
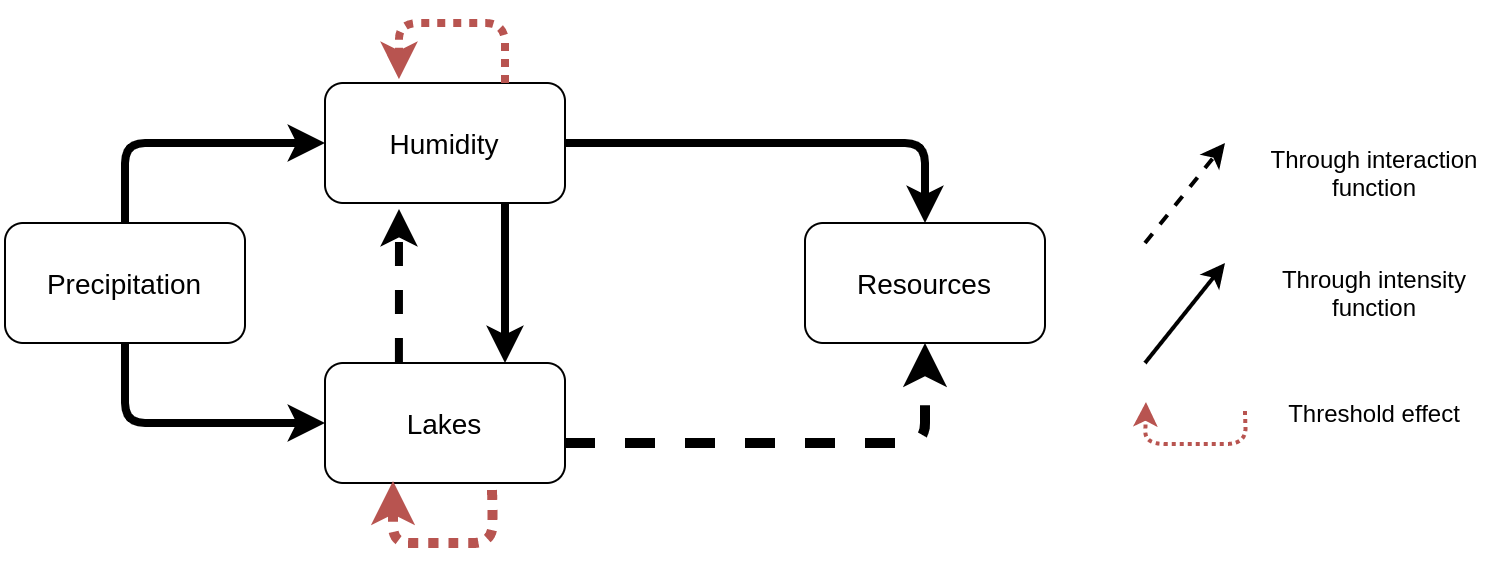
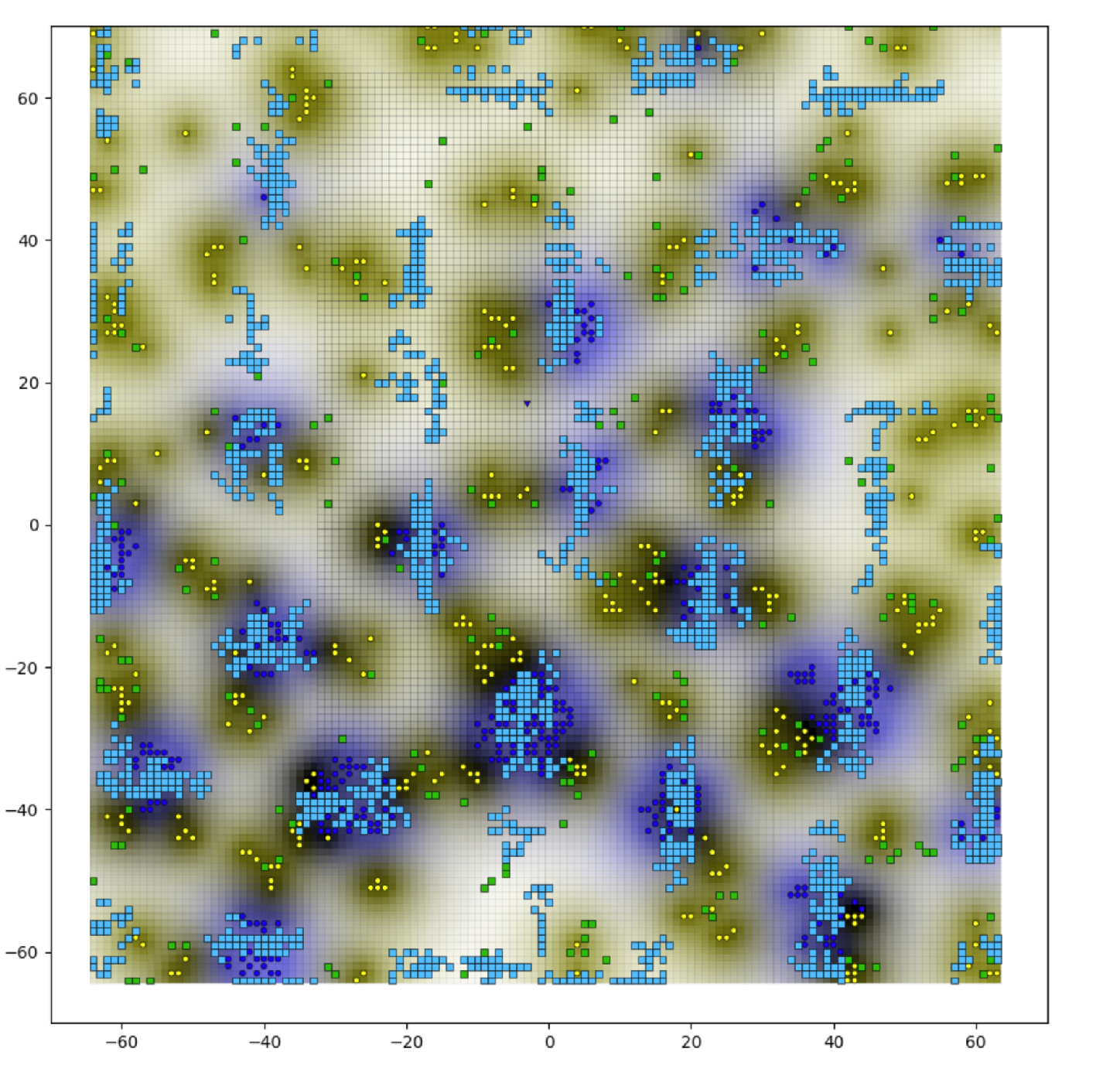
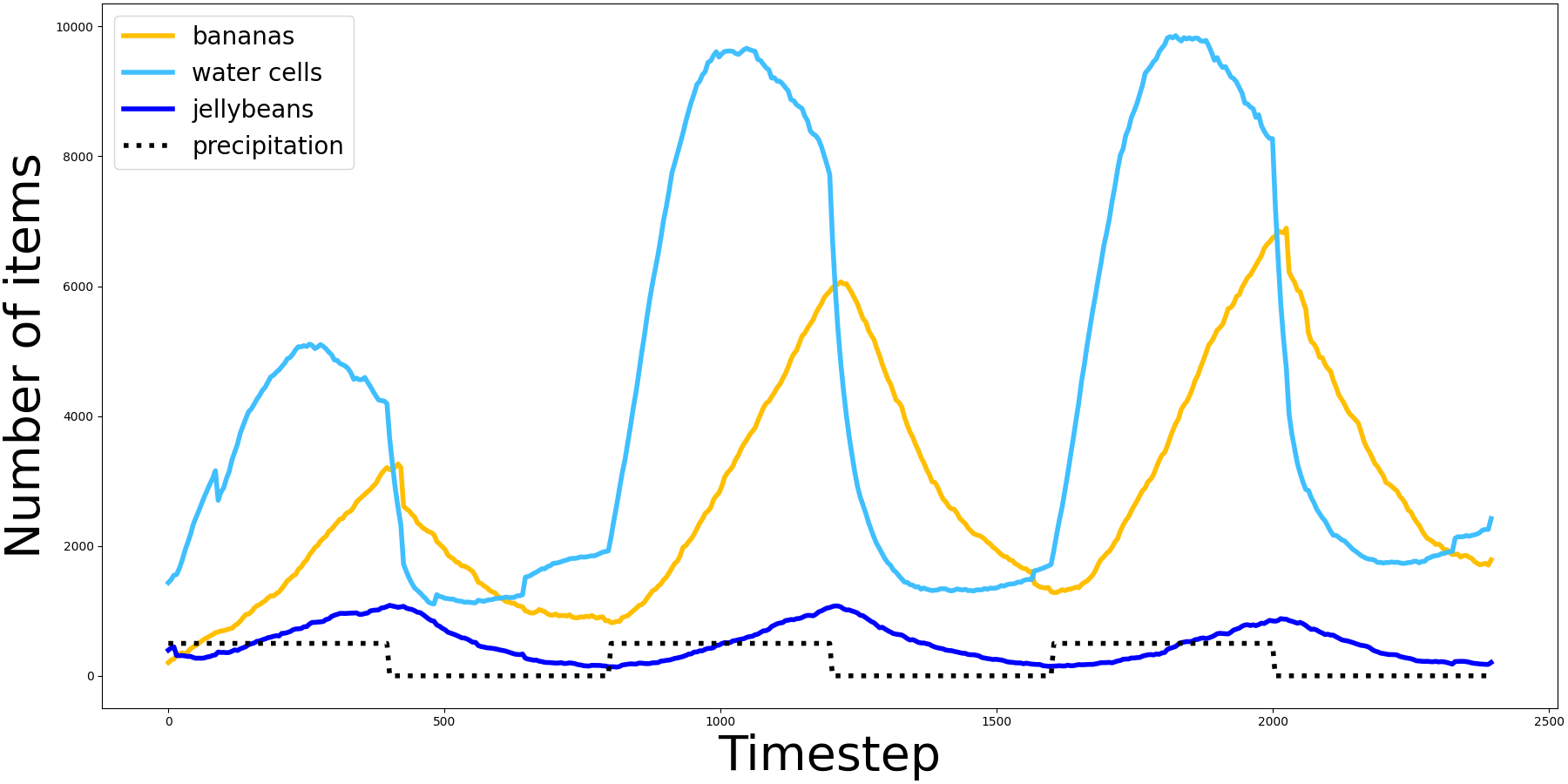
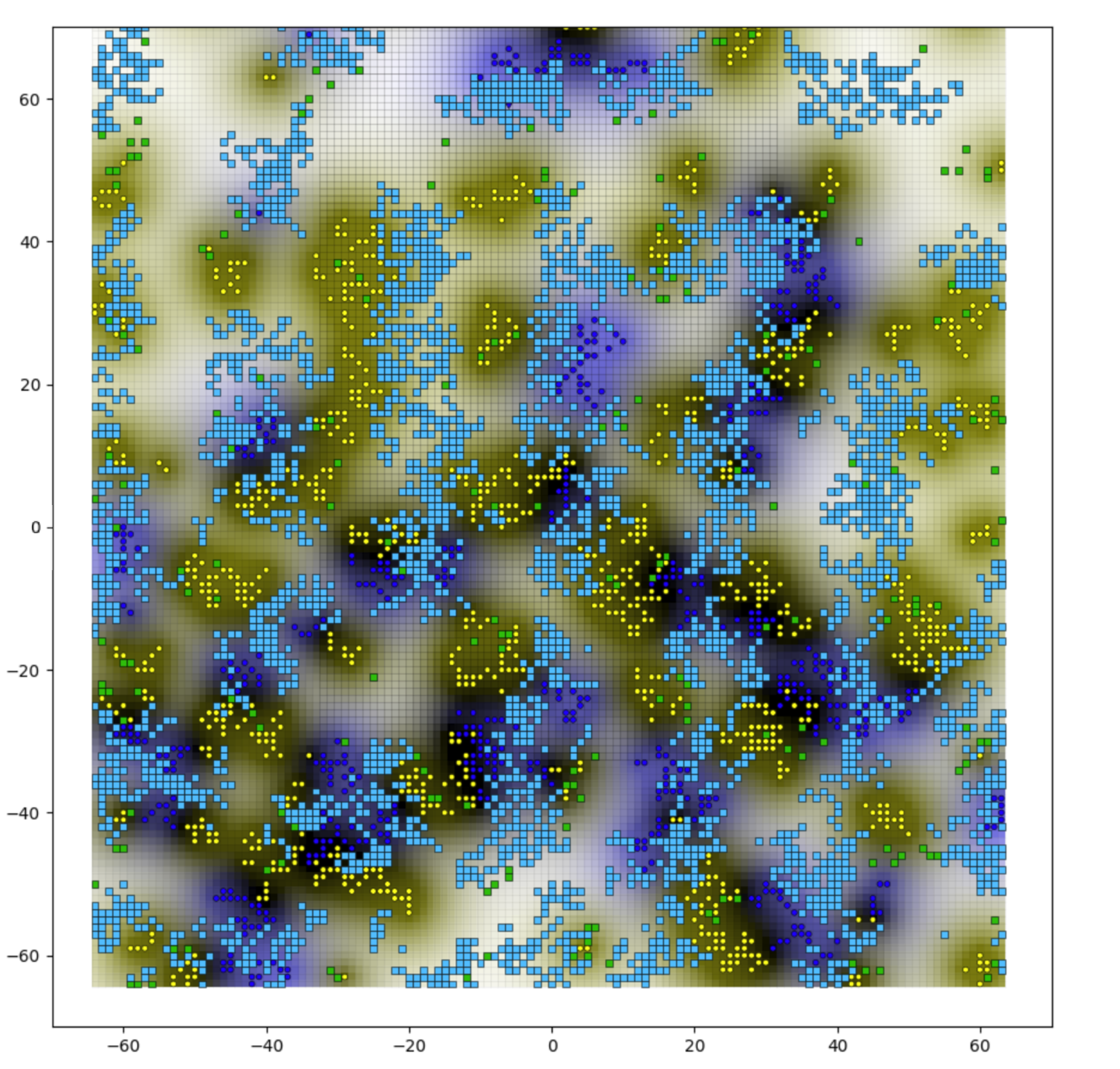
Objectives
In our next steps, we plan to work on the lines of improving the state-of-the-art in meta RL and multi-agent RL by leveraging hypotheses from HBE. Simultaneously, in a similar spirit to our group's proposal of using multi-agent RL as a computational tool for studying language development 51, we will employ RL as a computational tool for evaluating HBE hypotheses. In particular, our review has identified the following research challenges:
- identifying the effect that environmental variability has on the adaptability of meta RL agents. The rate of environmental change is an important hyper-parameter for meta RL algorithms but has only recently attracted attention 142. Our plan is to ground this investigation in HBE theories from climate variability, which state that the adaptability of species is achieved through mechanisms whose form depends on properties of the environment. If the environment is constant across time and space, natural selection may favor innate behaviors. By contrast, if the environment varies, natural selection might favor behavioral plasticity: based on environmental observations an agent may be able to switch between different behaviors following innate, and not learned instructions 120. In cases where the environment changes noticeably across generations but slowly enough within a generation, behavioral plasticity is guided by a process of developmental selection, an example of which is the learning process, where an agent’s past behavior guide its future behavior.
- studying the effect that environmental properties such as predator pressure and resource availability have on groups of RL agents. Emergent autocurricula in multi-agent RL have been observed to lead to open-ended skill acquisition in various works 92, 143. We plan to investigate how group properties, such as size and structure, are influenced by their environment and create feedback loops that lead to the emergence of autocurricula. Preliminary work was realized in this direction during the Master internship of Younès Rabii (February to August 2020), who implemented predator-preys complex systems within a multi-agent simulated environment. This work initiated a collaboration with Michael Garcia-Ortiz from City University in London (UK).
- cultural repertoire in large-scale groups of RL agents. According to the social complexity hypothesis 121, uniquely human skills such as language, social norms and institutions emerged as a need to regulate interactions in social systems of increasing size and structural complexity. We plan to study emergent communication in MARL as part of the ongoing Phd thesis of Julius Taylor (started November 2020, see also our recent position paper 51). We also recently started a collaboration with Microsoft Research New-York (USA) on a project that studies the role of fireside chats in the emergence of rich communication systems in groups of RL agent, in relation with theories of language evolution. We have recently published a paper on the emergence of social conventions in collaboration with University Pompeu Fabra in Spain 122. Finally, preliminary experiments on the role of partial observability and channel reliability in emergent communication were realized during the Master internship of Valentin Villecroze (April to August 2020). This work was published as a workshop paper (191) and an extract of the results is presented in figure 25.
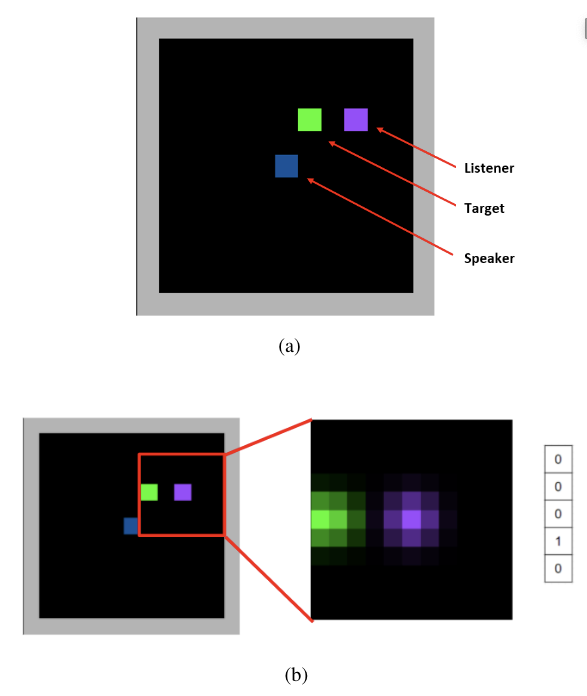
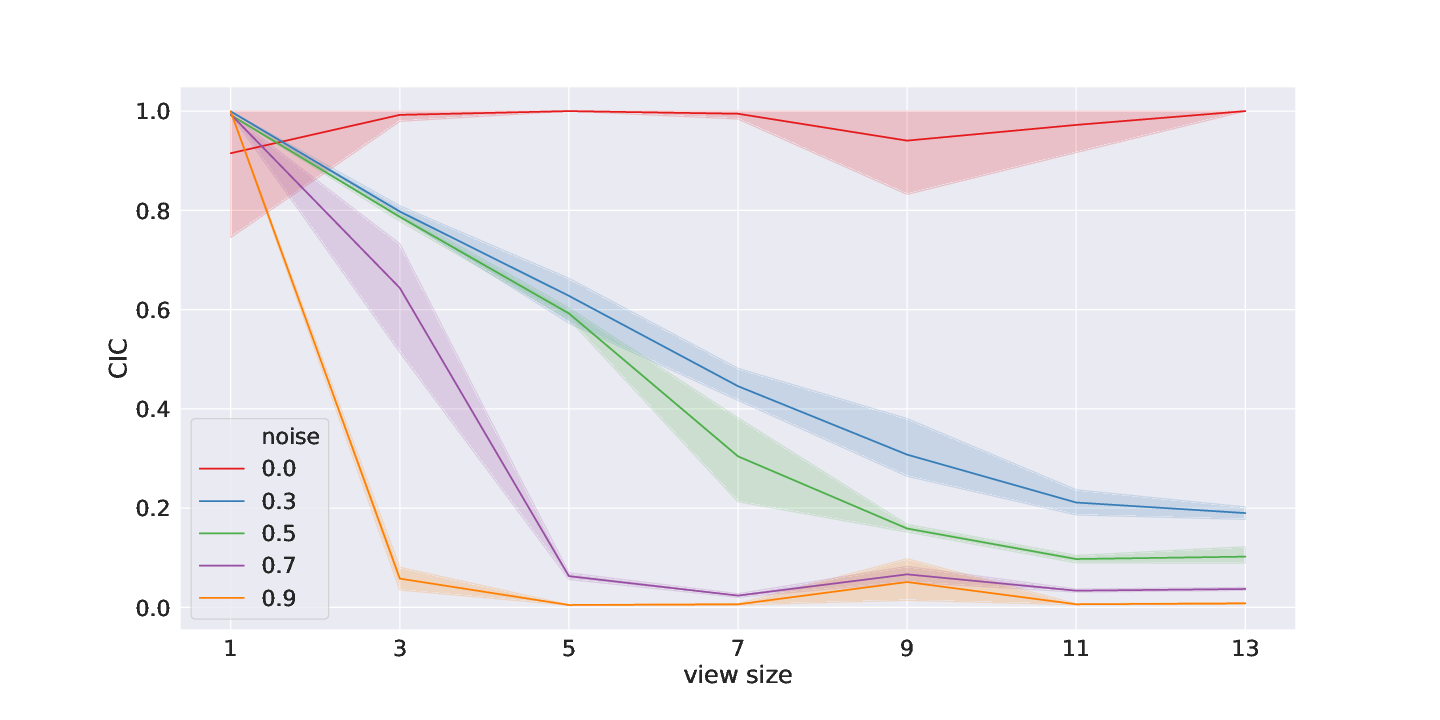
8.5.2 Socially Supervised Representation Learning: the Role of Subjectivity in Learning Efficient Representations
Participants: Julius Taylor [correspondant], Eleni Nisioti, Clément Moulin-Frier.
Introduction
In this work 54, we propose that aligning internal subjective representations, which naturally arise in a multi-agent setup where agents receive partial observations of the same underlying environmental state, can lead to more data-efficient representations. We propose that multi-agent environments, where agents do not have access to the observations of others but can communicate within a limited range, guarantees a common context that can be leveraged in individual representation learning. The reason is that subjective observations necessarily refer to the same subset of the underlying environmental states and that communication about these states can freely offer a supervised signal. To highlight the importance of communication, we refer to our setting as socially supervised representation learning. We present a minimal architecture comprised of a population of autoencoders, where we define loss functions, capturing different aspects of effective communication, and examine their effect on the learned representations.
Contributions.
We summarise our contributions as follows:
- We highlight an interesting link between data-augmentation traditionally used in single-agent self-supervised setting and a group of agents interacting in a shared environment.
- We introduce Socially Supervised Representation Learning, a new learning paradigm for unsupervised learning of efficient representations in a multi-agent setup.
- We present a detailed analysis of the conditions ensuring both the learning of efficient individual representations and the alignment of those representations across the agent population.
Methods
We consider a population of agents and environment states , hidden to the agents. Each agent receives a private observation of the state , where is an observation space. Agents are essentially convolutional autoencoders, though other self-supervised learning technique could be used (for example variational autoencoders 138). We define encoder and decoder functions and , respectively, where is a latent representation space (also called a message space, see below). Given an input observation, an agent encodes it into a latent representation and attempts at reconstructing the observation through , dropping the dependence on for brevity. Agents will use these latent vectors to communicate to other agents about their perceptual inputs (hence the term message space for ). When agent receives a message from agent they decode the message using their own decoder, i.e. .
In order to incentivise communication in our system, we define four loss functions which encourage agents to converge on a common protocol in their latent spaces. First, we define the message-to-message loss as
This loss directly incentivises that two messages (i.e. encodings) are similar. Since messages are always received in a shared context, this loss encourages agents to find a common representation for the observed state, abstracting away particularities induced by the specific viewpoint of an agent. Next, we propose the decoding-to-input loss, given by
This loss brings the decoding of agent from agent 's message closer to agent 's input observation, indirectly incentivising an alignment of representations because both agents can reconstruct from the other agents message more easily, when they agree on a common latent code i.e. they have similar representations for a given . Then, we propose the decoding-to-decoding loss:
which is computed using the reconstructed input of agent and the reconstruction of incurred from the message sent by . Lastly, the standard autoencoding loss is given by
The message of agent is defined as , with , where is a hyperparameter in our system. The total loss we optimise is thus
with , , , and being tunable hyperparameters.
Results
0.475 |
(a) |
0.475 |
0.475 |
0.475 |
(b) | (c) | (d) |
We show that our proposed architecture allows the emergence of aligned representations. This means that different agents find similar encodings for the same sensory inputs. The subjectivity introduced by presenting agents with distinct perspectives of the environment state contributes to learning abstract representations that outperform those learned by a single autoencoder and a population of autoencoders, presented with identical perspectives of the environment state, which is shown in the left column of Fig. 26. Furthermore, in Fig. 26 (right) we show that the learned representations are data-efficient, i.e. they enjoy the most benefit when evaluated on small testing splits. This is important, because good representations should allow agents to adapt to downstream tasks quickly and with few samples. Altogether, our results demonstrate how communication from subjective perspectives can lead to the acquisition of more abstract representations in multi-agent systems, opening promising perspectives for future research at the intersection of representation learning and emergent communication.
8.6 Applications in Educational Technologies
8.6.1 Machine Learning for Adaptive Personalization in Intelligent Tutoring Systems
Participants: Pierre-Yves Oudeyer [correspondant], Benjamin Clément, Didier Roy, Hélène Sauzeon.
The Kidlearn project
Kidlearn is a research project studying how machine learning can be applied to intelligent tutoring systems. It aims at developing methodologies and software which adaptively personalize sequences of learning activities to the particularities of each individual student. Our systems aim at proposing to the student the right activity at the right time, maximizing concurrently his learning progress and his motivation. In addition to contributing to the efficiency of learning and motivation, the approach is also made to reduce the time needed to design ITS systems.
We continued to develop an approach to Intelligent Tutoring Systems which adaptively personalizes sequences of learning activities to maximize skills acquired by students, taking into account the limited time and motivational resources. At a given point in time, the system proposes to the students the activity which makes them progress faster. We introduced two algorithms that rely on the empirical estimation of the learning progress, RiARiT that uses information about the difficulty of each exercise and ZPDES that uses much less knowledge about the problem.
The system is based on the combination of three approaches. First, it leverages recent models of intrinsically motivated learning by transposing them to active teaching, relying on empirical estimation of learning progress provided by specific activities to particular students. Second, it uses state-of-the-art Multi-Arm Bandit (MAB) techniques to efficiently manage the exploration/exploitation challenge of this optimization process. Third, it leverages expert knowledge to constrain and bootstrap initial exploration of the MAB, while requiring only coarse guidance information of the expert and allowing the system to deal with didactic gaps in its knowledge. The system was evaluated in several large-scale experiments relying on a scenario where 7-8 year old schoolchildren learn how to decompose numbers while manipulating money 109. Systematic experiments were also presented with simulated students.
Kidlearn Experiments 2018-2019: Evaluating the impact of ZPDES and choice on learning efficiency and motivation
An experiment was held between March 2018 and July 2019 in order to test the Kidlearn framework in classrooms in Bordeaux Metropole. 600 students from Bordeaux Metropole participated in the experiment. This study had several goals. The first goal was to evaluate the impact of the Kidlearn framework on motivation and learning compared to an Expert Sequence without machine learning. The second goal was to observe the impact of using learning progress to select exercise types within the ZPDES algorithm compared to a random policy. The third goal was to observe the impact of combining ZPDES with the ability to let children make different kinds of choices during the use of the ITS. The last goal was to use the psychological and contextual data measures to see if correlation can be observed between the students psychological state evolution, their profile, their motivation and their learning. The different observations showed that generally, algorithms based on ZPDES provided a better learning experience than an expert sequence. In particular, they provide a more motivating and enriching experience to self-determined students. Details of these new results, as well as the overall results of this project, are presented in Benjamin Clément PhD thesis 108 and are currently being processed to be published.
Kidlearn and Adaptiv'Math
The algorithms developed during the Kidlearn project and Benjamin Clement thesis 108 are being used in an innovation partnership for the development of a pedagogical assistant based on artificial intelligence intended for teachers and students of cycle 2. The algorithms are being written in typescript for the need of the project. The expertise of the team in creating the pedagogical graph and defining the graph parameters used for the algorithms is also a crucial part of the role of the team for the project. One of the main goal of the team here is to transfer technologies developed in the team in a project with the perspective of industrial scaling and see the impact and the feasibility of such scaling.
Kidlearn for numeracy skills with individuals with autism spectrum disorders
Few digital interventions targeting numeracy skills have been evaluated with individuals with autism spectrum disorder (ASD) 65153. Yet, some children and adolescents with ASD have learning difficulties and/or a significant academic delay in mathematics. While ITS are successfully developed for typically developed students to personalize learning curriculum and then to foster the motivation-learning coupling, they are not or fewly proposed today to student with specific needs. The objective of this pilot study is to test the feasibility of a digital intervention using an STI with high school students with ASD and/or intellectual disability. This application (KidLearn) provides calculation training through currency exchange activities, with a dynamic exercise sequence selection algorithm (ZPDES). 24 students with ASD and/or DI enrolled in specialized classrooms were recruited and divided into two groups: 14 students used the KidLearn application, and 10 students received a control application. Pre-post evaluations show that students using KidLearn improved their calculation performance, and had a higher level of motivation at the end of the intervention than the control group. These results encourage the use of an STI with students with specific needs to teach numeracy skills, but need to be replicated on a larger scale. Suggestions for adjusting the interface and teaching method are suggested to improve the impact of the application on students with autism. (Paper is submitted).
8.6.2 Machine learning for adaptive cognitive training
Participants: Pierre-Yves Oudeyer [correspondant], Hélène Sauzéon [correspondant], Masataka Sawayama, Benjamin Clément, Maxime Adolphe.
Because of its cross-cutting nature to all cognitive activities such as learning tasks, attention is a hallmark of good cognitive health throughout life and more particularly in the current context of societal crisis of attention. Recent works have shown the great potential of computerized attention training for an example of attention training, with efficient training transfers to other cognitive activities, and this, over a wide spectrum of individuals (children, elderly, individuals with cognitive pathology such as Attention Deficit and Hyperactivity Disorders). Despite this promising result, a major hurdle is challenging: the high inter-individual variability in responding to such interventions. Some individuals are good responders (significant improvement) to the intervention, others respond variably, and finally some respond poorly, not at all, or occasionally. A central limitation of computerized attention training systems is that the training sequences operate in a linear, non-personalized manner: difficulty increases in the same way and along the same dimensions for all subjects. However, different subjects require in principle a progression at a different, personalized pace according to the different dimensions that characterize attentional training exercises.
To tackle the issue of inter-individual variability, the present project proposes to apply some principles from intelligent tutorial systems (ITS) to the field of attention training. In this context, we have already developed automatic curriculum learning algorithms such as those developed in the KidLearn project, which allow to customize the learner's path according to his/her progress and thus optimize his/her learning trajectory while stimulating his/her motivation by the progress made. ITS are widely identified in intervention research as a successful way to address the challenge of personalization, but no studies to date have actually been conducted for attention training. Thus, whether ITS, and in particular personalization algorithms, can optimize the number of respondents to an attention training program remains an open question.
To investigate this question, an ongoing work on systematically reviewing the literature of the use of ITS in the field of cognitive training has been started. In parallel to this, a web platform has been designed for planning and implementing remote behavioural studies. This tool provides means for registering recruited participants remotely and executing complete experimental protocols: from presenting instructions and obtaining informed consents, to administering behavioural tasks and questionnaires, potentially throughout multiple sessions spanning days or weeks. In addition to this platform, a cognitive test battery composed of seven classical behavioural tasks has been developed. This battery aims to evaluate the evolution of the cognitive performance of participants before and after training. Fully open-source, it mainly targets attention and memory. A preliminary study on 30 participants showed that the developed tasks reproduced the results of previous studies, that there were large differences between individuals (no ceiling effect) and that the results were significantly reliable between two measurements taken on two days separated by one night (paper in progress).
With these tools, an ongoing pilot study involving 27 participants was launched. The objective of the study was to compare the effectiveness of a cognitive training whose difficulty is managed in a linear way (staircase procedure) to a cognitive training whose difficulty is manipulated by an ITS. In the coming months, the results of this first experiment will allow the launch of a study on a larger population of young adults as well as on an aging population.
8.6.3 Interactive systems that foster curiosity for education
Participants: Pierre-Yves Oudeyer [correspondant], Hélène Sauzéon [correspondant], Mehdi Alami, Rania Abdelghani, Didier Roy, Edith Law.
Since 2019 via the renewal of the Idex cooperation fund (between the University of Bordeaux and the University of Waterloo, Canada) led by the Flowers team and also involving F. Lotte from the Potioc team, we continue our work on the development of new curiosity-driven interaction systems. Although experiments have been slowed down by sanitary conditions, progress has been made in this area of application of FLOWERS works. In particular, three studies have been completed.
The first study regards a new interactive educational application to foster curiosity-driven question-asking in children. This study has been performed during the Master 2 internship of Mehdi Alaimi co-supervised by H. Sauzéon, E. Law and PY Oudeyer. It addresses a key challenge for 21st-century schools, i.e., teaching diverse students with varied abilities and motivations for learning, such as curiosity within educational settings. Among variables eliciting curiosity state, one is known as « knowledge gap », which is a motor for curiosity-driven exploration and learning. It leads to question-asking which is an important factor in the curiosity process and the construction of academic knowledge. However, children questions in classroom are not really frequent and don’t really necessitate deep reasoning. Determined to improve children’s curiosity, we developed a digital application aiming to foster curiosity-related question-asking from texts and their perception of curiosity. To assess its efficiency, we conducted a study with 95 fifth grade students of Bordeaux elementary schools. Two types of interventions were designed, one trying to focus children on the construction of low-level question (i.e. convergent) and one focusing them on high-level questions (i.e. divergent) with the help of prompts or questions starters models. We observed that both interventions increased the number of divergent questions, the question fluency performance, while they did not significantly improve the curiosity perception despite high intrinsic motivation scores they have elicited in children. The curiosity-trait score positively impacted the divergent question score under divergent condition, but not under convergent condition. The overall results supported the efficiency and usefulness of digital applications for fostering children’s curiosity that we need to explore further. The overall results are published in CHI'20 2. In parallel to these first experimental works, we wrote this year a review of the existing works on the subject 31.
The second study investigates the neurophysiological underpinnings of curiosity and the opportunities of their use for Brain-computer interactions 89. Understanding the neurophysiological mechanisms underlying curiosity and therefore being able to identify the curiosity level of a person, would provide useful information for researchers and designers in numerous fields such as neuroscience, psychology, and computer science. A first step to uncovering the neural correlates of curiosity is to collect neurophysiological signals during states of curiosity, in order to develop signal processing and machine learning (ML) tools to recognize the curious states from the non-curious ones. Thus, we ran an experiment in which we used electroencephalography (EEG) to measure the brain activity of participants as they were induced into states of curiosity, using trivia question and answer chains. We used two ML algorithms, i.e. Filter Bank Common Spatial Pattern (FBCSP) coupled with a Linear Discriminant Algorithm (LDA), as well as a Filter Bank Tangent Space Classifier (FBTSC), to classify the curious EEG signals from the non-curious ones. Global results indicate that both algorithms obtained better performances in the 3-to-5s time windows, suggesting an optimal time window length of 4 seconds to go towards curiosity states estimation based on EEG signals. These results have been published 89
Finally, the third study investigates the role of intrinsic motivation in spatial learning in children (paper in progress). In this study, the state curiosity is manipulated as a preference for a level of uncertainty during the exploration of new environments. To this end, a series of virtual environments have been created and is presented to children. During encoding, participants explore routes in environments according the three levels of uncertainty (low, medium, and high), thanks to a virtual reality headset and controllers and, are later asked to retrace their travelled routes. The exploration area and the wayfinding. ie the route overlap between encoding and retrieval phase, (an indicator of spatial memory accuracy) are measured. Neuropsychological tests are also performed. Preliminary results showed that there are better performances under the medium uncertainty condition in terms of exploration area and wayfinding score. These first results supports the idea that curiosity states are a learning booster (paper in progress).
At the end of 2020, we started an industrial collaboration project with EvidenceB on this topic (CIFRE contract of Rania Abdelghani currently submitted to the ANRT). The overall objective of the thesis is to propose new educational technologies driven by epistemic curiosity, and allowing children to express themselves more and learn better. To this end, a central question of the work will be to specify the impact of self-questioning aroused by states of curiosity about student performance. Another objective will be to create and study the pedagogical impact of new educational technologies in real situations (schools) promoting an active education of students based on their curiosity. To this end, a web platform called 'Kids Ask' has been designed, developed and tested in two primary schools. The tool offers an interaction with a conversational agent that trains children's abilities to generate curiosity-driven questions and use these questions to explore a learning environment and acquire new knowledge. The first results suggest that the configuration helped enhance children's questioning and exploratory behaviors; they also show that learning progress differences in children can be explained by the differences in their curiosity-driven behaviors (paper in progress).
8.6.4 Aïna : an accessible MOOC player for people with cognitive disabilities
Participants: Pascal Guitton [correspondant], Hélène Sauzéon [correspondant], Pierre-Antoine Cinquin, Damien Caselli.
New digital teaching systems such as MOOCs are taking an increasingly important place in current teaching practices. Unfortunately, accessibility for people with disabilities is often forgotten, which excludes them, particularly those with cognitive impairments for whom accessibility standards are fare from being established. This is truly unfortunate as the interest of using these specialized practices for this audience is scientifically proven (self-determination theory, Universal Design for Learning) 106(Computer & Education). To overcome these limitations, we proposed new design principles based on knowledge in the areas of accessibility (Ability-based Design and Universal Design, e.g., alternatives communication functionalities), digital pedagogy (Instruction Design with functionalities that reduce the cognitive load : navigation by concept, slowing of the flow…), specialized pedagogy (Universal Design for Learning, eg, automatic note-taking, and Self Determination Theory, e.g., configuration of the interface according to users needs and preferences) and psychoducational interventions (eg, support the joint teacher-learner attention), but also through a participatory design approach involving students with disabilities and experts in the field of disability. From these new design principles and through co-design sessions with PWD and experts, we developed Aïana, an accessible MOOC player 105. Aïana has been used in the context of a MOOC on Digital Accessibility available on the national platform FUN (with more than 5600 registered users from 60 different country nowadays). Moreover we observed how learners were using Aïana through activities follow-up and questionnaires that we proposed to them. These measures enabled us to validate three main results (32. First, in contradiction to “classic” MOOC, percentage of learners with disability following our MOOC was equivalent to the global population, which is a strong indication of its accessibility. Second, we observed a learning performance at the end of the MOOC equivalent for learners with disability and other learners. Finally, the results in terms of learning analytics (e.g., user interactions with the player features) confirm our contribution to designing a more inclusive e-learning environment. Importantly, we observed that the relationships between intrinsic motivation and learning rate is more critical for learners with disability compared to typical learners. Thus, Aïana has been particularly beneficial for learners with cognitive impairment.
8.6.5 ToGather : Interactive website to foster collaboration among stakeholders of school inclusion for pupils with neurodevelopmental disorders
Participants: Hélène Sauzéon [correspondant], Cécile Mazon, Eric Meyer, Isabeau Saint-Supery, Christelle Maillart.
Sustain and support the follow-up of the school inclusion of children with neurodevelopmental disorders (e.g., autism, attention disorders, intellectual deficiencies) has become an emergency : the higher is the school level, the lower is the amount of schooled pupils with cognitive disabilities.Technology-based interventions to improve school inclusion of children with neurodevelopmental disorders have mostly been individual centered, focusing on their socio-adaptive and cognitive impairments and implying they have to adapt themselves in order to fit in our society's expectations. Although this approach centered on the normalization of the person has some advantages (reduction of clinical symptoms), it carries social stereotypes and misconceptions of cognitive disability that are not respectful of the cognitive diversity and intrinsic motivations of the person, and in particular of the student's wishes in terms of school curriculum to achieve his or her future life project. The "ToGather" project aims at enlightening the field of educational technologies for special education by proposing an approach centered on the educational needs of the students and by bringing a concerted and informed answer between all the stakeholders including the student and all their support spheres (family, school, medico-social care). To this end, ToGather project that emanates from participatory design methods, primarily consists of having developed a pragmatic tool (interactive website) to help students with cognitive disability and their caregivers to formalize and to visualize the repertoire of academic skills of the student and to make it evolve according to his or her proximal zone of development (in the sense of Vygotsky) on the one hand, and to the intrinsic motivations of the student (his or her own educational and life project) on the other 41.
The next part of the project will have two goals: 1) to validate its usability (interaction data, user experience, motivations of different users, etc. ) for French and Belgian schools (transferability of the tool to no french socio-educational context), and 2) to validate its added value through a controlled and randomized field study evaluating the impact on the student (user experience, academic success, school-related well-being and motivation) and his/her caregivers (self-efficacy, perception of school inclusion, perceived health, communication quality etc.)
This project is in partnership with the School Academy of Bordeaux of the French Education Minestery, the ARI association, the Centre of Autism of Aquitaine. It is funded by the FIRAH (foundation) and the Nouvelle-Aquitaine Region.
8.6.6 A computer science and robotics integration model for primary school
Participants: Didier Roy [correspondant].
Integrating computer science (CS) into school curricula has become a worldwide preoccupation. Therefore, we present a CS and Robotics integration model and its validation through a large-scale pilot study in the administrative region of the Canton Vaud in Switzerland. Approximately 350 primary school teachers followed a mandatory CS continuing professional development program (CPD) of adapted format with a curriculum scaffolded by instruction modality. This included CS Unplugged activities that aim to teach CS concepts without the use of screens, and Robotics Unplugged activities that employed physical robots, without screens, to learn about robotics and CS concepts. Teachers evaluated positively the CPD and their representation of CS improved. Voluntary adoption rates reached 97 percent during the CPD and 80 percent the following year. These results combined with the underpinning literature support the generalisability of the model to other contexts. This work was published in 116 and led by our colleagues at EPFL.
8.6.7 How An Automated Gesture Imitation Game Can Improve Social Interactions With Teenagers With ASD
Participants: Linda Nanan Vallée, Sao Mai Nguyen, Christophe Lohr, Ioannis Kanellos, Olivier Asseu.
With the outlook of improving communication and social abilities of people with ASD, we propose to extend the paradigm of robot-based imitation games to ASD teenagers. In this paper 189, we present an interaction scenario adapted to ASD teenagers, propose a computational architecture using the latest machine learning algorithm Openpose for human pose detection, and present the results of our basic testing of the scenario with human caregivers. These results are preliminary due to the number of session (1) and participants (4). They include a technical assessment of the performance of Openpose, as well as a preliminary user study to confirm our game scenario could elicit the expected response from subjects.
8.7 Applications to Automated Scientific Discovery in Self-Organizing Systems
8.7.1 Curiosity-driven Learning for Automated Discovery of Physico-Chemical Structures
Participants: Mayalen Etcheverry [correspondant], Chris Reinke, Clément Moulin-Frier, Pierre-Yves Oudeyer.
In previous work, the problem of automated diversity-driven discovery in morphogenetic systems was introduced 12427. Aiming to discover a maximal diversity of patterns that can emerge in the system without relying on prior assumptions or expert knowledge, an intrinsically-motivated goal exploration processes (IMGEP) is applied to autonomously guide the system exploration. Originally developed for the learning of inverse models in developmental robotics, an IMGEP is an algorithmic process which defines a goal space (encodes relevant features of the observed patterns) and generates a sequence of experiments (to explore the parameters of a dynamical system) by targeting a diversity of self-generated goals 315. In robotics, these exploration algorithms have been shown to allow real world robots to acquire skills such as tool use 16. In other domains such as chemistry and physics, they open the possibility to automate the discovery of novel chemical or physical structures produced by complex dynamical systems 168. However, they have so far assumed that self-generated goals are sampled in a specifically engineered feature space, limiting their autonomy. Recent work has shown how unsupervised deep learning approaches could be used to learn goal space representations 24 but they have used precollected data to learn the representations. Instead of using an externally-imposed goal space, 27 developed a novel IMGEP algorithm (IMGEP-OGL) that learns goal representations online during the exploration of the system, using a online-learned Variational Auto Encoder 138.
As testbed system, the method was applied onto numerical cellular automata called Lenia, a continuous extension of the Game of Life that has shown to produce interesting patterns and dynamics resembling life-like micro-organisms 104.
A random exploration of the Lenia system tends to produce
This work is published and has been presented as an oral talk at the conference ICLR 2020 27. The project website with videos and additional results can be found at https://automated-discovery.github.io/, and the source code is available at https://github.com/flowersteam/automated_discovery_of_lenia_patterns. Additionally, a blogpost explaining and presenting the approach to a broader audience was published on the team website 118.
8.7.2 Hierarchically Organized Latent Modules for Meta-Diversity Search in Morphogenetic Systems
Participants: Mayalen Etcheverry [correspondant], Clément Moulin-Frier, Pierre-Yves Oudeyer.
In the previous paper 27, the problem of automated diversity-driven discovery in morphogenetic systems was introduced, highlighting that two key ingredients are autonomous exploration and unsupervised representation learning to describe "relevant" degrees of variations in the patterns. Yet, standard diversity-driven approaches assume that the intuitive notion of diversity can be captured within a single behavioral characterization (BC) space.
In this project, we follow the proposed experimental testbed of Reinke et al.(2020) 27 on a continuous game-of-life system (Lenia, 104). We provide empirical evidence that the discoveries of an IMGEP operating in a monolithic BC space are highly-diverse in that space, yet tend to be poorly-diverse in other potentially-interesting BC spaces (see Figure 27). This draws several limitations when it comes to applying such system as a tool for assisting discovery in morphogenetic system, as the suggested discoveries are unlikely to align with the interests of a end-user.
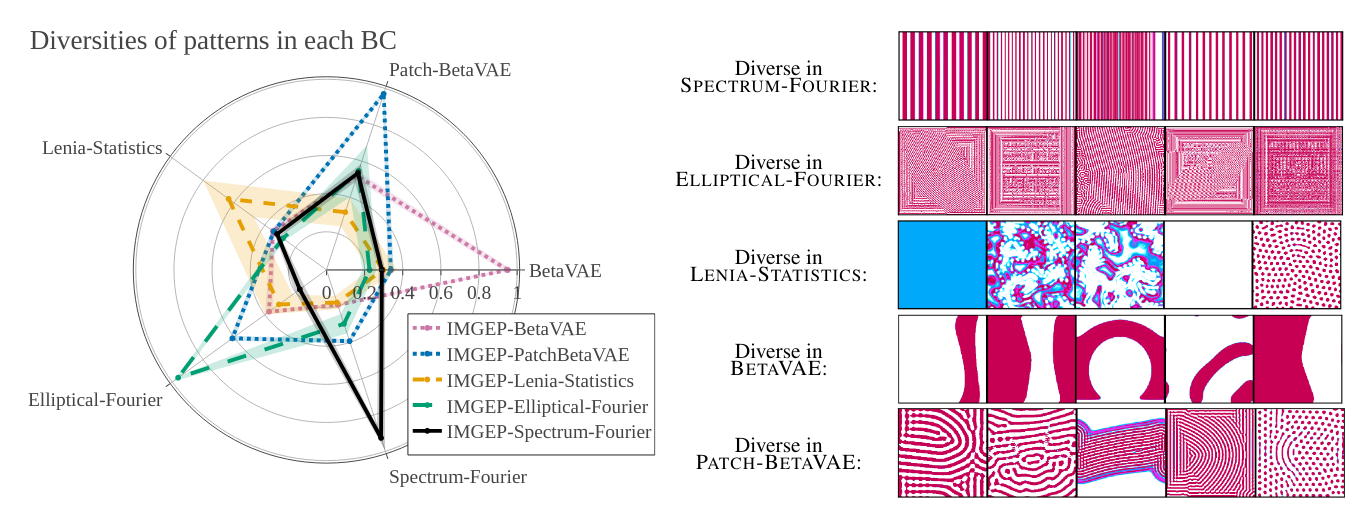
To address these limits, the contributions of this project are threefold. First, we formulate the problem of meta-diversity search as follows: an artificial “discovery assistant” incrementally learns a set of diverse BC spaces in an outer loop; and searches to discover diverse patterns within each of them in an inner loop. With minimal external feedback, a successful discovery assistant should be able to efficiently specialize the exploration strategy toward a particular type of diversity, corresponding to the initially unknown preferences of the human evaluator.
Second, we present HOLMES, a dynamic and modular model architecture for unsupervised learning of diverse representations where a hierarchy of module embedding networks is actively expanded. Additionally, we present IMGEP-HOLMES (see Figure 28) which extends the standard IMGEP framework by replacing the monolithic representation with the proposed hierarchy. We show that the hierarchical structure allows the IMGEP agent to target goals in the different nodes in order to achieve diversity in each BC space.
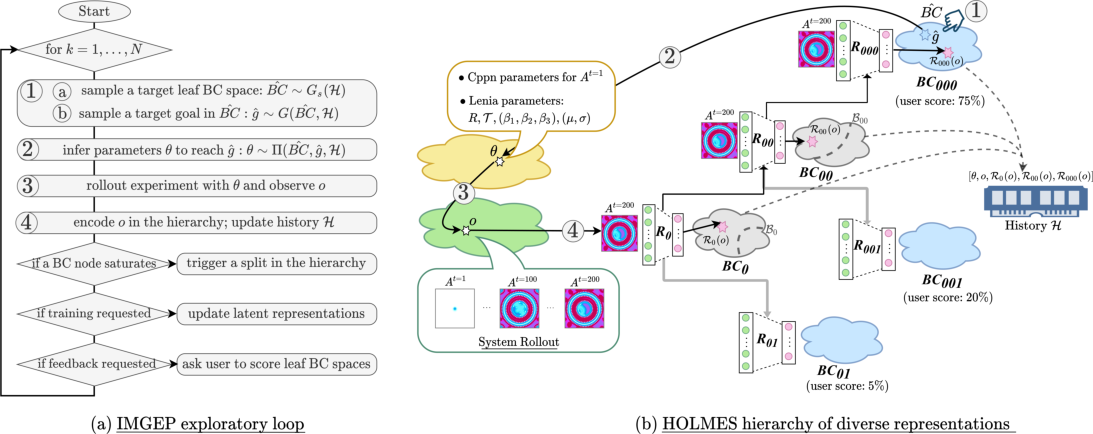
IMGEP-HOLMES framework integrates a goal-based intrinsically-motivated exploration process (IMGEP) with the incremental learning of a hierarchy of behavioral characterization spaces (HOLMES). HOLMES unsupervisedly clusters and encodes discovered patterns into the different nodes of the hierarchy of representations. The exploratory loop and its interaction with the hierarchy of behavioral characterization (BC) spaces enables the meta-diversity search.
Finally, we show how this architecture can easily be leveraged to drive exploration, opening interesting perspectives for the integration of a human in the loop.
To conclude, this work shows that integrating flexible modular representation learning with intrinsically-motivated goal exploration processes for meta-diversity search are very promising directions in the context of automated discovery in morphogenetic systems. As an example, IMGEP-HOLMES was able to discover many types of solutions including unseen pattern-emitting lifeforms in less than 15000 training steps without guidance, when their existence remained an open question raised in the original Lenia paper 104.
Initial version of this work was presented at ICLR 2020 workshop "Beyond tabula rasa in Reinforcement Learning" 58. The final version of this work is published and has been presented as an oral talk at the conference NeurIPS 2020 14. The project website with videos and additional results can be found at http://mayalenE.github.io/holmes/, and the source code is available at http://mayalenE.github.io/holmes/.
In 2021, the proposed method was applied and presented to the Minecraft Open-Endedness Challenge holded at GEGGO 2021, for which the FLOWERS team submission won the Runner-Up Prize 86. The purpose of the challenge was to highlight the progress in algorithms that can create novel and increasingly complex artefacts. It was the first contest on open-endedness within the Machine Learning community and was based on the Minecraft environment to study and compare the generated artifacts. Our submission was based on two main components: a complex system used to recursively grow and complexify artifacts over time, and a discovery algorithm that leverages the concept of meta-diversity search. As complex system, we implemented a 3D variant of the Lenia system 103 that was adapted for the Minecraft environment. The discovery algorithm was based on the IMGEP-HOLMES implementation presented in previous work 14. The video summarizing the approach and the blogpost presenting the algorithm and the obtained results can be found at https://mayalene.github.io/evocraftsearch/.
8.7.3 Automated exploration of neuro-mechanical models or arms using goal exploration algorithms
Participants: Pierre-Yves Oudeyer [correspondant].
This work was led by Daniel Cattaert, Aymar de Rugy and their collaborators at Incia, with contributions from Pierre-Yves Oudeyer.
Objective. Neuro-mechanical models are essential to increase our understanding of the fundamental mechanisms underlying natural sensorimotor control, and to foster robotic designs using them. Yet, the complexity of those models is such that current optimization methods are unsuited to establish the range of useful behaviors they could produce, and their associated parameter settings. Our goal is to provide both using recent advances in developmental machine learning. Approach. We designed a simplified neuro-mechanical model that nevertheless has the complexity that make current optimization fail. This model consists of a single (elbow) joint actuated by two muscles and their associated spindles, alpha and gamma motoneurons, receiving simple (non-dynamic) step commands. To establish the range of movements this system is capable of doing, a goal exploration process was used that built a repertoire of valid actions through iterative sampling of target behaviors, combined with stochastic variation on the parameter settings that elicited their closest behaviors in this repertoire. Results obtained with this process were compared to those obtained with alternative optimization methods. Main results. The goal exploration was found to widely outperform optimization methods in terms of its capacity to rapidly establish a repertoire of valid actions, and to find a large range of behaviors not otherwise found. The resulting repertoire also provides diverse parameter sets for any given actions, akin to what is observed in natural control. Families of solutions originating from few initial seeds should also be exploitable to generate novel behaviors through interpolation. Significance. The proposed method provides rich perspectives to explore the structure and settings of lower-level neural circuitry, and their associated descending commands, to produce a wide range of useful behaviors. Comparison of behavioral space obtained after selective manipulation of various elements of neuro-mechanical models should also help understand natural control, and promote its emulation in robotics. We have written an article under review.
8.7.4 Design of an Interactive Software for Automated Discovery in Complex Systems
Participants: Clément Romac [correspondant], Mathieu Perie, Mayalen Etcheverry, Clément Moulin-Frier, Pierre-Yves Oudeyer.
We recently showed how curiosity-driven algorithms can be used to guide the exploration of complex systems, such as morphogenetic systems 2714. While such methods could be applied to a large range of complex systems in order to map the possible self-organized structures, they remain difficult to grasp for non-experts users, limiting their deployment.Additionally, 14 also showed that adding human in the exploration loop can be a key to obtain interesting mappings. Designing interactive algorithms is thus an important step towards the adoption of automated exploration and discovery of complex systems, as users previously using hand-made heuristics would still need to add their expert knowledge in the exploration process.
Following these, we are designing a fully open-source interactive software which aims to provide tools to easily use exploration algorithms (e.g. curiosity-driven) in various systems. Our software is composed of:
- a standalone Python library allowing to define systems and exploration algorithms and launch experiments. An experiment is composed of a system (e.g. Lenia), an exploration algorithm (IMGEP-HOLMES14), in-between functions (e.g. a CPPN) and configuration for all the previously mentioned "blocks".
- a web application allowing to control the library through a user-friendly visual interface. Our application allows to create experiments and visualize their results (see fig. 29 and fig. 30). This application has a microservice architecture (see fig. 31) and leverages Docker to make the software easily installable and modifiable by non-computer scientist users.
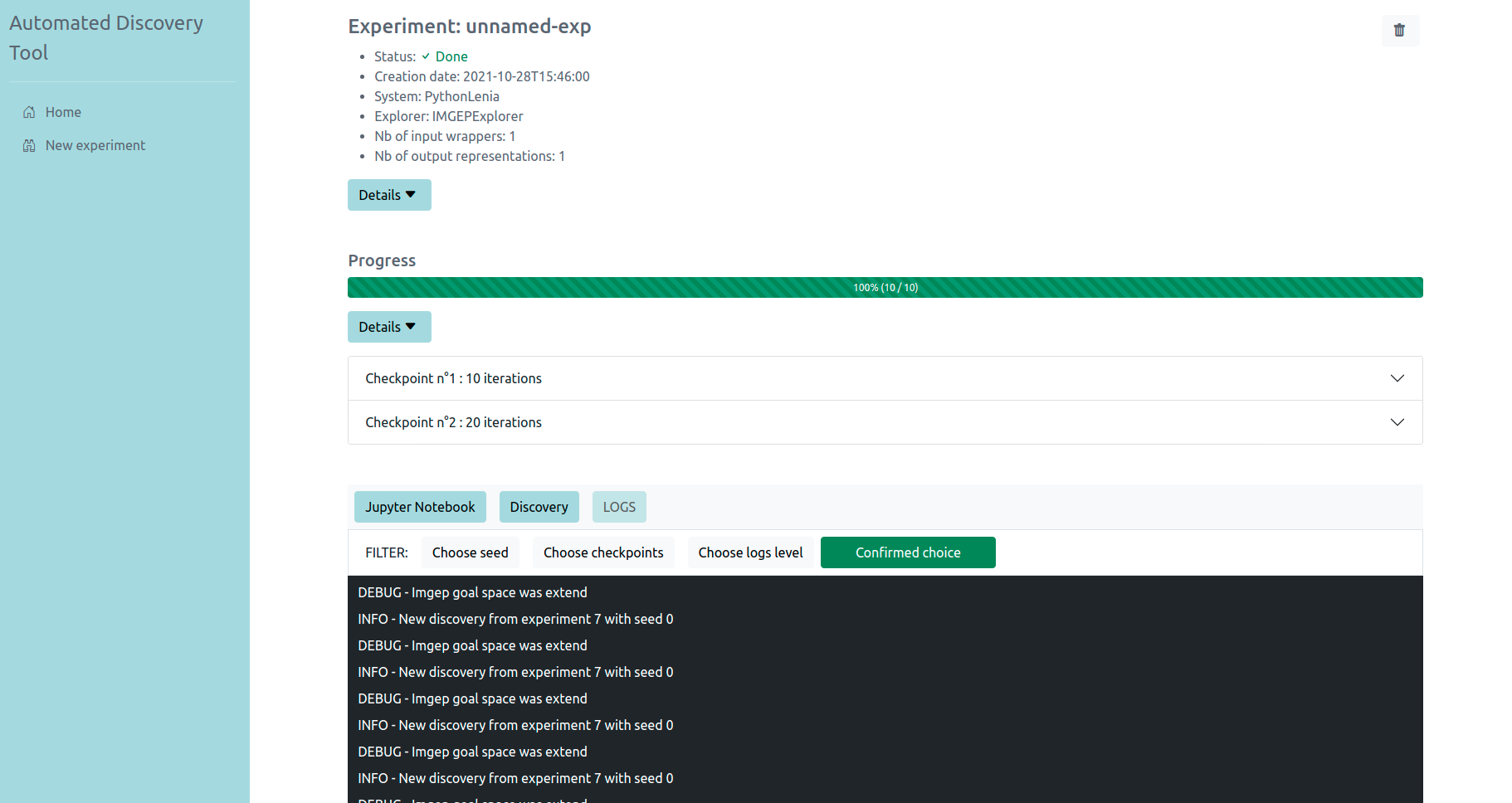
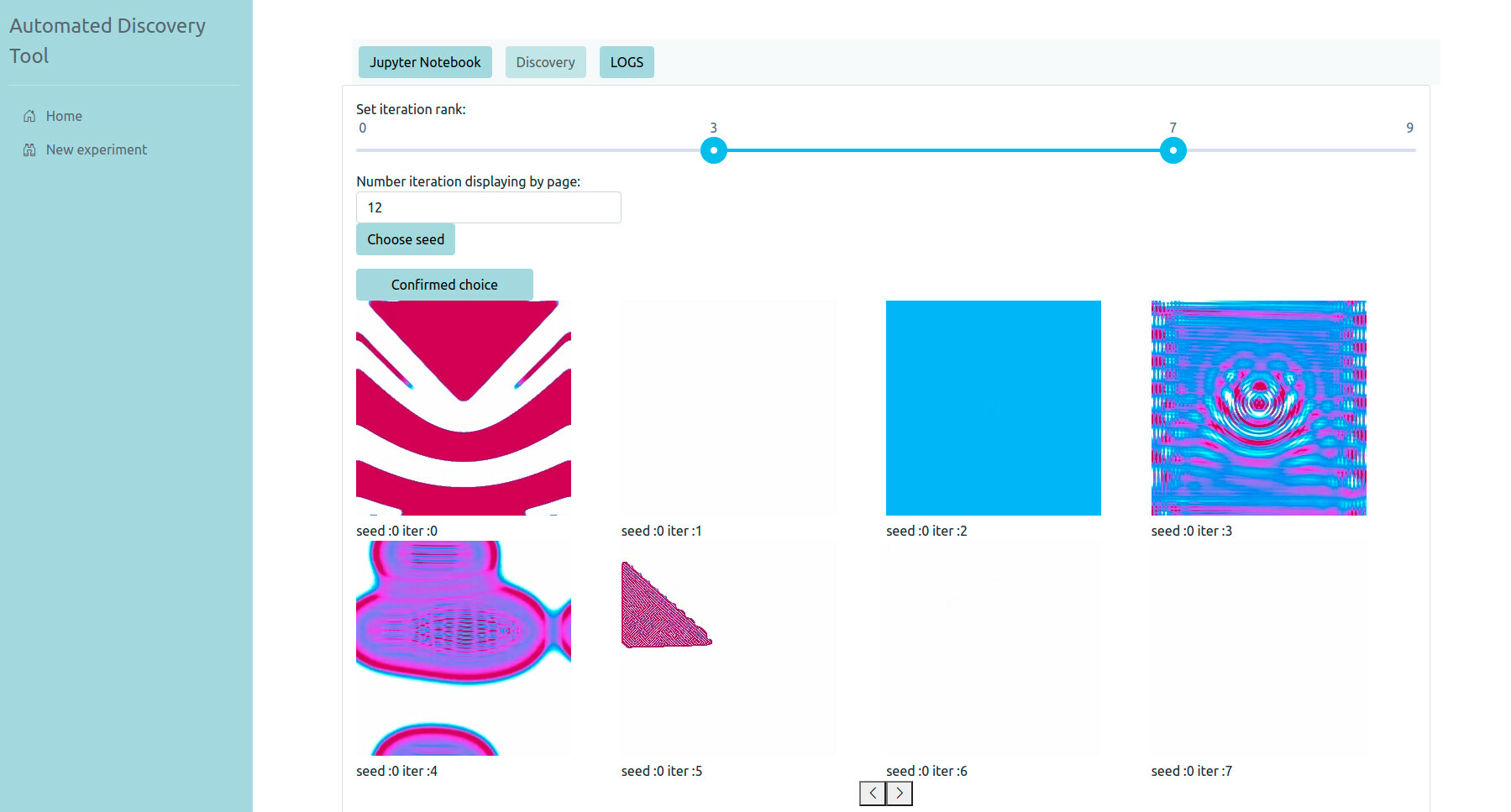
We are currently building the possibility to run experiments remotely (e.g. on a cluster) as well as adding user in the experiment loop to provide feedback and guide exploration. We plan to release this software in 2022 along with some already implemented systems (e.g. Lenia) and exploration methods (e.g. IMGEP-HOLMES) as well as experiments with them.
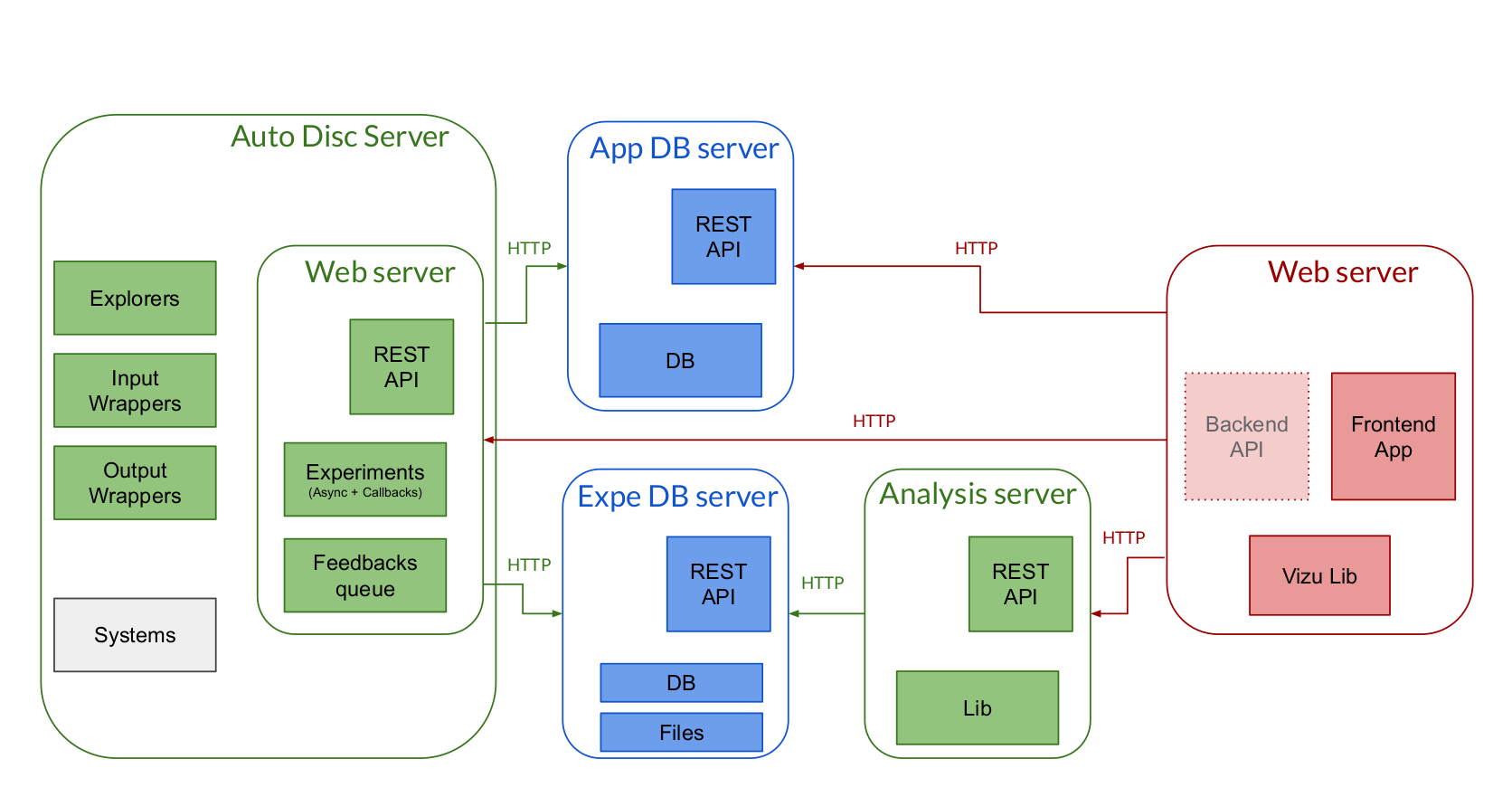
8.7.5 Learning Sensorimotor Agency in Cellular Automata
Participants: Gautier Hamon [correspondant], Mayalen Etcheverry, Bert Chan, Clément Moulin-Frier, Pierre-Yves Oudeyer.
As a continuation of the projects in 8.7, we have been working on expanding the set of discoveries of possible structures in continuous CAs such as Lenia 104, 103, and in particular we have been interested to search for emerging agents with sensorimotor capabilities. Understanding what has led to the emergence of life and sensorimotor agency as we observe in living organisms is a fundamental question. In our work, we initially only assume environments made of low-level elements of matter (called atoms, molecules or cells) locally interacting via physics-like rules. There is no predefined notion of agent embodiment and yet we aim to answer the following scientific question: is it possible to find environments in which there exists/emerge a subpart that could be called aWe use Lenia continuous cellular automaton as our artificial "world" 103. We introduce a novel method based on gradient descent and curriculum learning combined within an intrinsically-motivated goal exploration process (IMGEP) to automatically search parameters of the CA rule that can self-organize spatially localized 1 and moving patterns 2 within Lenia. The IMGEP defines an outer exploratory loop (generation of training goal/loss) and an inner optimization loop (goal-conditioned). We use a population-based version of IMGEP 15, 71 but introduce two novel elements compared to previous papers in the IMGEP literature. First, whereas previous work in 8.7.1 and 8.7.2 used a very basic nearest-neighbor goal-achievement strategy, our work relies on gradient descent for the local optimization of the (sensitive) parameters of the complex system, which has shown to be very powerful. To do so we made a differentiable version of the Lenia framework, which is also a contribution of this work. Secondly, we propose to control subparts of the environmental dynamics with functional constraints (through predefined channels and kernels in Lenia) to build a curriculum of tasks; and to integrate this stochasticity in the inner optimization loop. This has shown central to train the system to emerge sensorimotor agents that are robust to stochastic perturbations in the environment. In particular, we focus on modeling obstacles in the environment physics and propose to probe the agent sensorimotor capability as its performance to move forward under a variety of obstacle configurations.
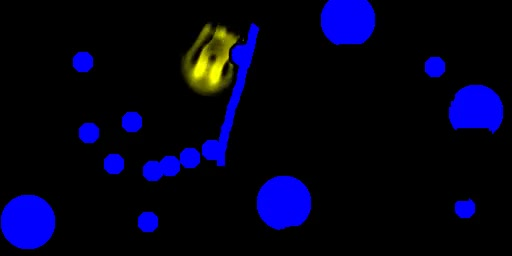
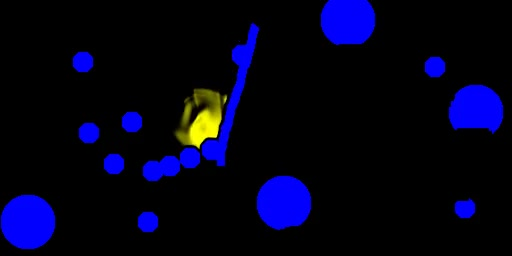
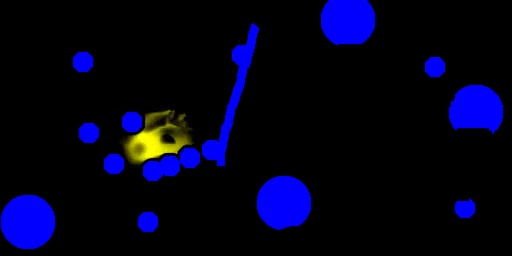
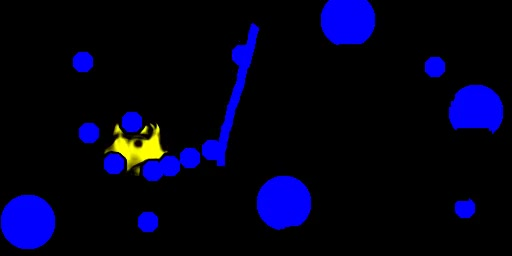
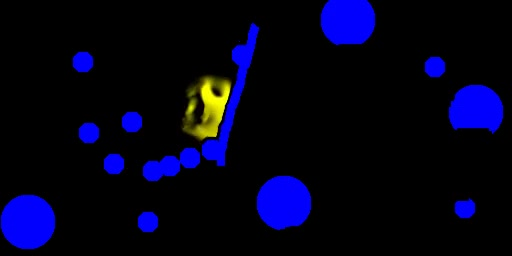
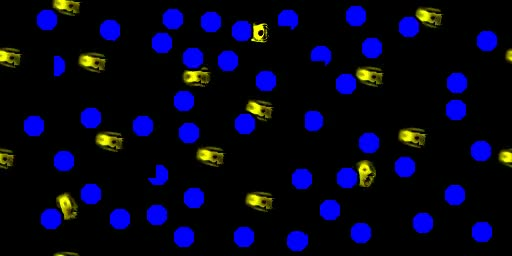
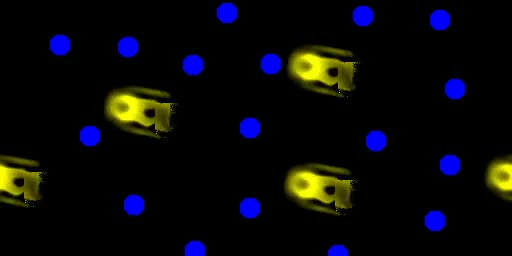
While many complex behaviors have already been observed in Lenia, among which some could qualify as sensorimotor behaviors, they have so far been discovered "by chance" as the result of time-consuming manual search or with simple evolutionary algorithms. Our method provides a more systematic way to automatically learn the CA rules leading to the emergence of basic sensorimotor structures. Moreover, we investigated the (zero-shot) generalization of the discovered sensorimotor agents to several out-of-distribution perturbations that were not encountered during training. Impressively, even though the agents still fail to preserve their integrity in certain configurations, they show very strong robustness to most of the tested variations. The agents are able to navigate in unseen and harder environmental configurations while self-maintaining their individuality (Figure 32, top). Not only the agents are able to recover their individuality when subjected to external perturbations but also when subjected to internal perturbations: they resist variations of the morphogenetic processes such that less frequent cell updates, quite drastic changes of scales as well as changes of initialization (Figure 32, bottom). Furthermore, when tested in a multi-entity initialization and despite having been trained alone, not only the agents are able to preserve their individuality but they show forms of coordinated interactions (attractiveness and reproduction). Our results suggest that, contrary to the (still predominant) mechanistic view on embodiment, biologically-inspired embodiment could pave the way toward agents with strong coherence and generalization to out-of-distribution changes, mimicking the remarkable robustness of living systems to maintain specific functions despite environmental and body perturbations 140. Searching for rules at the cell-level in order to give rise to higher-level cognitive processes at the level of the organism and at the level of the group of organisms opens many exciting opportunities to the development of embodied approaches in AI in general.
The work is not yet published but has been released as a distill-like article which is currently hosted at https://developmentalsystems.org/sensorimotor-lenia/. This article contains an interactive demo in webGL and javascript, as well as many videos and animations of the results. A colab notebook with the source code of the work is publicly available at https://colab.research.google.com/drive/11mYwphZ8I4aur8KuHRR1HEg6ST5TI0RW?usp=sharing.
Collaboration with Bert Chan
In the context of the project 8.7, we have an ongoing collaboration with Bert Chan, a previously independant researcher on Artificial Life and author of the Lenia system 104, 103 and who is now working as a research engineer at Google Brain. During this collaboration, Bert Chan help us design versions of IMGEP usable by scientists (non ML-experts) end-users, which is the aim of project 8.7.4. Having himself created the Lenia system, he is highly-interested to use our algorithms to automatically explore the space of possible emerging structures and provides us valuable insights into end-user habits and concerns. Bert Chan was also involved in the FLOWERS team submission to the Minecraft Open-Endedness challenge discussed in section 8.7.2. Bert Chan also co-supervised with Mayalen Etcheverry the master internship of Gautier Hamon which led to the work described in section 8.7.5.
8.8 Other
8.8.1 EXplainable Neural-Symbolic Learning
Participants: Natalia Díaz Rodríguez [correspondant], David Filliat, Adrien Bennetot.
The latest Deep Learning (DL) models for detection and classification have achieved an unprecedented performance over classical machine learning algorithms. However, DL models are black-box methods hard to debug, interpret, and certify. DL alone cannot provide explanations that can be validated by a non technical audience such as end-users or domain experts. In contrast, symbolic AI systems that convert concepts into rules or symbols-such as knowledge graphs-are easier to explain. However, they present lower generalisation and scaling capabilities. A very important challenge is to fuse DL representations with expert knowledge. One way to address this challenge, as well as the performance-explainability trade-off is by leveraging the best of both streams without obviating domain expert knowledge. In this paper, we tackle such problem by considering the symbolic knowledge is expressed in form of a domain expert knowledge graph. We present the eXplainable Neural-symbolic learning (X-NeSyL) methodology, designed to learn both symbolic and deep representations, together with an explainability metric to assess the level of alignment of machine and human expert explanations. The ultimate objective is to fuse DL representations with expert domain knowledge during the learning process so it serves as a sound basis for explainability. In particular, X-NeSyL methodology involves the concrete use of two notions of explanation, both at inference and training time respectively: 1) EXPLANet: Expert-aligned eXplainable Part-based cLAssifier NETwork Architecture, a compositional convolutional neural network that makes use of symbolic representations, and 2) SHAP-Backprop, an explainable AI-informed training procedure that corrects and guides the DL process to align with such symbolic representations in form of knowledge graphs. We showcase X-NeSyL methodology using MonuMAI dataset for monument facade image classification, and demonstrate that with our approach, it is possible to improve explainability at the same time as performance 35.
8.8.2 Evaluating Robustness over High Level Driving Instruction for Autonomous Driving
Participants: Florence Carton [correspondant], David Filliat [correspondant].
In recent years, we have witnessed increasingly high performance in the field of autonomous end-to-end driving. In particular, more and more research is being done on driving in urban environments, where the car has to follow high level commands to navigate. However, few evaluations are made on the ability of these agents to react in an unexpected situation. Specifically, no evaluations are conducted on the robustness of driving agents in the event of a bad high-level command. We propose here an evaluation method, namely a benchmark that allows to assess the robustness of an agent, and to appreciate its understanding of the environment through its ability to keep a safe behavior, regardless of the instruction 48.
8.8.3 Using Semantic Information to Improve Generalization in Reinforcement Learning
Participants: Florence Carton [correspondant], David Filliat [correspondant].
The problem of generalization of reinforcement learning policies to new environments is seldom addressed but essential in practical applications. We focus on this problem in an autonomous driving context using the CARLA simulator and first show that semantic information is the key to a good generalization for this task. We then explore and compare different ways to exploit semantic information at training time in order to improve generalization in an unseen environment without finetuning, showing that using semantic segmentation as an auxiliary task is the most efficient approach 67.
8.8.4 Machine Learning Optimization of Intervention Strategies for Epidemics
Participants: Cédric Colas [correspondant], Clément Moulin-Frier, Pierre-Yves Oudeyer.
This project is a collaboration with the SISTM team from Inria Bordeaux. Modelling the dynamics of epidemics helps proposing control strategies based on pharmaceutical and non-pharmaceutical interventions (contact limitation, lock down, vaccination, etc). Hand-designing such strategies is not trivial because of the number of possible interventions and the difficulty to predict long-term effects. This task can be cast as an optimization problem where state-of-the-art machine learning algorithms such as deep reinforcement learning, might bring significant value. However, the specificity of each domain – epidemic modelling or solving optimization problem – requires strong collaborations between researchers from different fields of expertise. This is why we introduce EpidemiOptim, a Python toolbox that facilitates collaborations between researchers in epidemiology and optimization. EpidemiOptim turns epidemiological models and cost functions into optimization problems via a standard interface commonly used by optimization practitioners (OpenAI Gym)—see Figure 33. Reinforcement learning algorithms based on Q-Learning with deep neural networks (dqn) and evolutionary algorithms (nsga-ii) are already implemented. We illustrate the use of EpidemiOptim to find optimal policies for dynamical on-off lock-down control under the optimization of death toll and economic recess using a Susceptible-Exposed-Infectious-Removed (seir) model for COVID-19. Using EpidemiOptim and its interactive visualization platform in Jupyter notebooks, epidemiologists, optimization practitioners and others (e.g. economists) can easily compare epidemiological models, costs functions and optimization algorithms to address important choices to be made by health decision-makers. Trained models can be explored by experts and non-experts via a web interface. This led to a submission at the journal JAIR (under review) 33. This project also led to a web interface where users can interact with trained lock-down intervention strategies, look at their effects on a models of the COVID-19 epidemics and design their own intervention strategy: https://epidemioptim.bordeaux.inria.fr/.
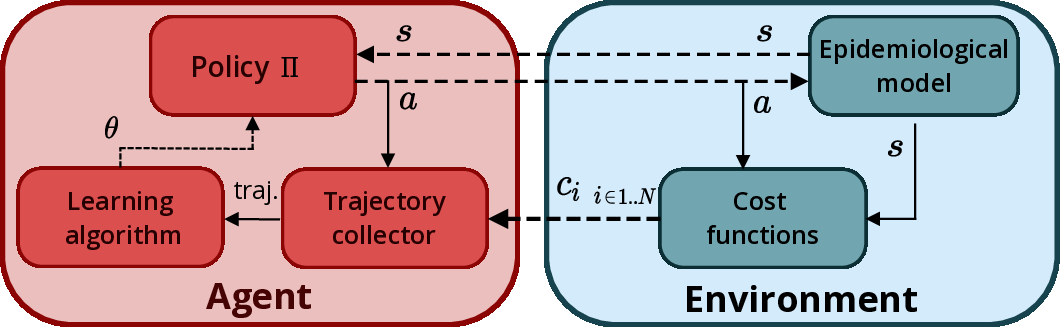
The EpidemiOptim formalization of the epidemic control problem. The optimization problem (left) is built around 1) epidemiological models that predict the evolution of the considered epidemics; 2) pre-defined cost functions that measure the cost of the epidemic propagation as well as the cost of interventions. The learning agent (right) interacts with the environment (the epidemic) via interventions/actions (aa), which triggers new epidemic states (ss) and associated costs (cic_i). The learning algorithm then use this experience to improve the internvention policy θ\theta to as to minimize the expected cumulative cost.
8.8.5 Automatic Curriculum Learning for Language Modeling
Participants: Clément Romac [correspondant], Rémy Portelas, Pierre-Yves Oudeyer.
We showed in recent works how Automatic Curriculum Learning (ACL) could help Deep Reinforcement Learning methods by tayloring a curriculum adapted to learner's capabilities 53, 25. Using ACL can lead to sample efficiency, asymptotic performance boost and help in solving hard tasks.
Parallel to this, recent works in Language Modeling using Transformers (e.g. GPT-2) have starting to get more interested in better understanding convergence and learning dynamics of these models. Trained in a supervised setup, these models are fed with hundred of millions of natural language sequences crawled from the web. The current standard way of training these models (i.e. constructing batches of randomly selected sequences) makes the assumption that all sequences have same interest for the model. However, recent works showed that this does not seem to be the case and that datasets can contain outliers harming training. Additionally, some works also showed that hand-designing a curriculum over sequences (e.g. ordered by their length) could speed up and stabilize training.
Building on this, we propose to experiment how ACL could help taylor such a curriculum in an automated way relying on Learning Progress. Our study has several contributions:
- Propose a standardized and more in-depth comparison of current curriculum learning methods used to train language models
- Introduce the first study of ACL in such a training
- Use ACL to propose deeper insights about training dynamics of Transformer models when doing Language Modeling by analysing generated curricula and Learning Progress estimations
We chose to train GPT-2 on the standard OSCAR dataset and use teacher algorithms to select samples that are shown to the model (see fig. 34).
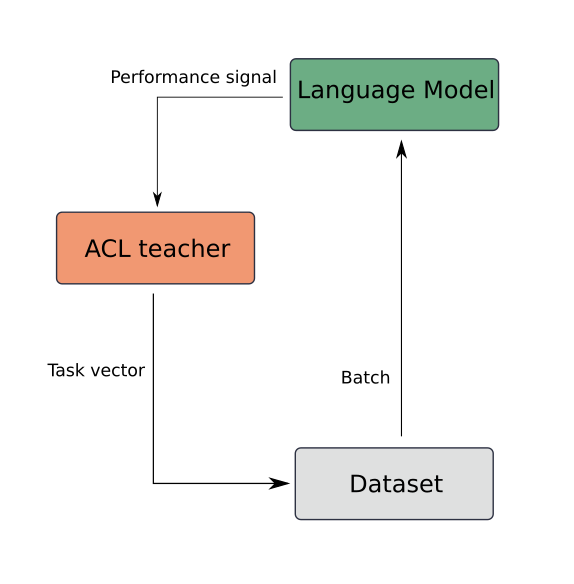
8.8.6 SocialAI: Socio-Cognitive Abilities in Deep Reinforcement Learning Agents
Participants: Grgur Kovač [correspondant], Remy Portelas, Katja Hoffman, Pierre-Yves Oudeyer.
Building embodied autonomous agents capable of participating in social interactions with humans is one of the main challenges in AI. Within the Deep Reinforcement Learning (DRL) field, this objective motivated multiple works on embodied language use. However, current approaches focus on language as a communication tool in very simplified and non-diverse social situations: the "naturalness" of language is reduced to the concept of high vocabulary size and variability. In this project, we argue that aiming towards human-level AI requires a broader set of key social skills: 1) language use in complex and variable social contexts; 2) beyond language, complex embodied communication in multimodal settings within constantly evolving social worlds. We explain how concepts from cognitive sciences could help AI to draw a roadmap towards human-like intelligence, with a focus on its social dimensions. As a first step, we propose to expand current research to a broader set of core social skills. To do this, we present SocialAI, a benchmark to assess the acquisition of social skills of DRL agents using multiple grid-world environments featuring other (scripted) social agents. We then study the limits of a recent SOTA DRL approach when tested on SocialAI and discuss important next steps towards proficient social agents. Videos and code are available at https://sites.google.com/view/socialai.
Based on research in developmental psychology, we identified some, of many possible, core social skills one should consider in aiming to train socially competent artificial agents. Those skills are: Intertwined multimodality (the ability to adapt its multimodal interaction sequence, rather than following a pre-established progression of modalities), Theory Of Mind (the ability of an agent to attribute to others and itself mental states, including beliefs, intents, desires, emotions and knowledge), and learning and using Pragmatic Frames (regular patterns characterizing the unfolding of possible social interactions).
We present a set of environments testing the ability of RL agents to acquire those skills (see figure 35).
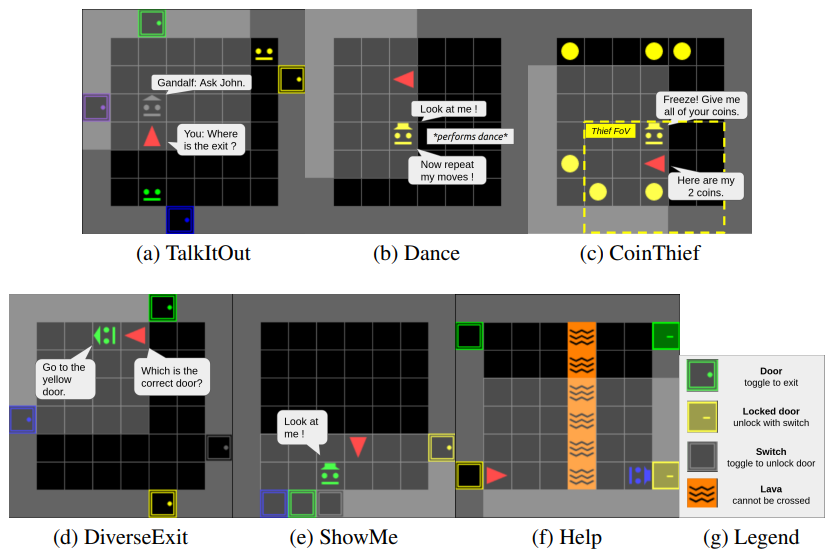
We show that current RL agents fail to solve any of the tasks, as can be seen in table 1. This implies that a lot of progress can be made in following this research directions.
Env \Cond | PPO | PPO + Explo | Unsocial PPO |
TalkItOut | |||
Dance | |||
CoinThief | |||
DiverseExit | |||
ShowMe | |||
Help | |||
SocialEnv | N/A |
8.8.7 Transflower: probabilistic autoregressive dance generation with multimodal attention
Participants: Guillermo Valle-Pérez [correspondant], Gustav Eje Henter, Jonas Beskow, André Holzapfel, Pierre-Yves Oudeyer, Simon Alexanderson.
Supervised learning is emerging as an important technique to solve reinforcement learning (RL) tasks. However, the ways of parametrizing policies typically used, for example for imitation learning, are fundamentally limited in the kinds of probability distributions they can fit. In this work, we developed a new kind of probabilistic autoregressive model combining the benefits of long-range time-dependence modelling of transformers, with the power of normalizing flows to capture probability distributions (see overview of architecture in Fig. 36). We apply this to a challenging motion generation task (dance generation), which is cast as a likelihood maximization problem, equivalent to behavioural cloning.
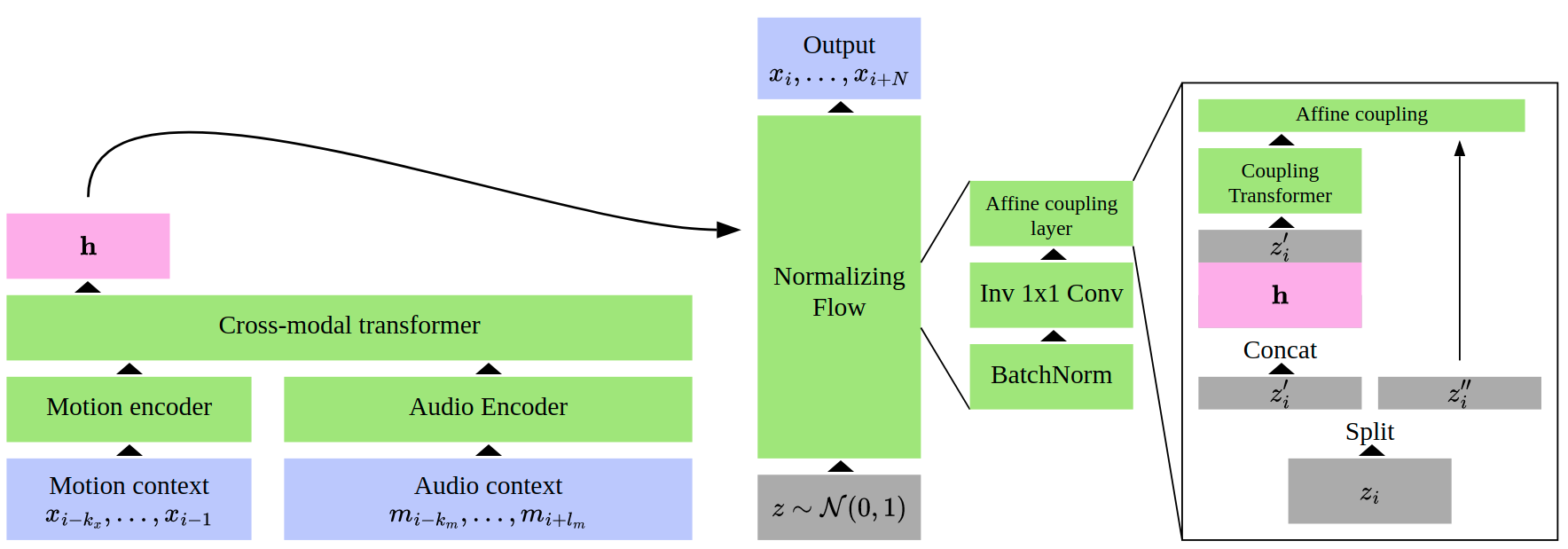
In this work, we also explored the potential of using VR technologies to facilitate gathering data about human movement. We compiled the biggest public dataset to date of music-paired dance motion, including data from remote VR dancers. This allowed us to train the models to produce diverse dance styles and movements, and showed the potential of Transflower to fit large and heterogeneous datasets with a single model.
As we mentioned in the first paragraph, we aim to use this model in other domains, in particular RL domains, like robotics. Furthermore, we plan to extend the VR software to allow for remote data collection, and interactive evaluation of the AI models.
9 Bilateral contracts and grants with industry
9.1 Bilateral contracts with industry
Autonomous Driving Commuter Car (Renault)
Participants: David Filliat [correspondant], Emmanuel Battesti.
We developed planning algorithms for a autonomous electric car for Renault SAS in the continuation of the previous ADCC project. We improved our planning algorithm in order to go toward navigation on open roads, in particular with the ability to reach higher speed than previously possible, deal with more road intersection case (roundabouts), and with multiple lane roads (overtake, insertion...).
9.2 Bilateral grants with industry
Curiosity-driven Learning Algorithms for Exploration of Video Game Environments (Ubisoft)
Participants: Pierre-Yves Oudeyer [correspondant].
Financing of a postdoc grant for a 2 year project with Ubisoft and Région Aquitaine.
Intrinsically Motivated Exploration for Lifelong Deep Reinforcement Learning in the Malmo Environment (Microsoft)
Participants: Pierre-Yves Oudeyer [correspondant], Remy Portelas.
Financing of the PhD grant of Rémy Portelas by Microsoft Research.
Research on lifelong Deep Reinforcement Learning of multiple tasks (Microsoft
Participants: Pierre-Yves Oudeyer [correspondant], Alexander Ten.
Financing of the PhD grant of Alexander Tan
Explainable continual learning for autonomous driving (Segula Technologies)
Participants: Natalia Díaz Rodríguez [correspondant], Adrien Bennetot.
Financing of the CIFRE PhD grant of Adrien Bennetot by Segula Technologies.
Automated Discovery of Self-Organized Structures (Poïetis)
Participants: Pierre-Yves Oudeyer [correspondant], Mayalen Etcheverry.
Financing of the CIFRE PhD grant of Mayalen Etcheverry by Poietis.
Machine learning for adaptive cognitive training (OnePoint)
Participants: Hélène Sauzéon [correspondant], Pierre-Yves Oudeyer, Maxime Adolph.
Financing of the CIFRE PhD grant of Maxime Adolph by Onepoint.
Curiosity-driven interaction system for learning (evidenceB)
Participants: Hélène Sauzéon [correspondant], Pierre-Yves Oudeyer, Rania Abdelghani.
Financing of the CIFRE PhD grant of Rania Adolph by EvidenceB.
Perception Techniques and Sensor Fusion for Level 4 Autonomous Vehicles (Renault)
Participants: David Filliat [correspondant], Vyshakh Palli-Thazha.
Financing of the CIFRE PhD grant of Vyshakh Palli-Thazha by Renault.
Exploration of reinforcement learning algorithms for drone visual perception and control (CEA)
Participants: David Filliat [correspondant], Florence Carton.
Financing of the CIFRE PhD grant of Florence Carton by CEA.
Incremental learning for sensori-motor control (Softbank Robotics)
Participants: David Filliat [correspondant], Hugo Caselles Dupré.
Financing of the CIFRE PhD grant of Hugo Caselles-Dupré by Softbank Robotics.
9.3 Bilateral Grants with Fundation
School+ /ToGather project (FIRAH)
Participants: Hélène Sauzéon [correspondant], Cécile Mazon, Isabeau Saint-supery, Eric Meyer.
Financing of one year-postdoctoral position and the app. development by the International Foundation for Applied Research on Disability (FIRAH). The School+ project consists of a set of educational technologies to promote inclusion for children with Autism Spectrum Disorder (ASD). School+ primary aims at encouraging the acquisition of socio-adaptive behaviours at school while promoting self-determination (intrinsic motivation), and has been created according to the methods of the User-Centered Design (UCD). Requested by the stakeholders (child, parent, teachers, and clinicians) of school inclusion, Flowers team works to the adding of an interactive tool for a collaborative and shared monitoring of school inclusion of each child with ASD. This new app will be assessed in terms of user experience (usability and elicited intrinsic motivation), self-efficacy of each stakeholder and educational benefit for child. This project includes the Academie de Bordeaux –Nouvelle Aquitaine, the CRA (Health Center for ASD in Aquitania), and the ARI association.
10 Partnerships and cooperations
10.1 International initiatives
10.1.1 Associate Teams in the framework of an Inria International Lab or in the framework of an Inria International Program
Without content for this year.
10.1.2 Inria associate team not involved in an IIL or an international program
Without content for this year.
10.1.3 STIC/MATH/CLIMAT AmSud project
Without content for this year.
10.1.4 Participation in other International Programs
Idex mobility program
-
Title
Curiosity-driven learning and personalized (re-)education technologies across the lifespan
-
Partner Institution(s):
- University of Bordeaux, France
- Game Institute, University of Waterloo, Canada
-
Date/Duration:
2019-2021 (20 000€)
-
Additionnal info/keywords:
: Interactive systems, education, curiosity
MITACS mobility program
-
Title
Curiosity-driven spatial learning across the lifespan
-
Partner Institution(s):
- Cognitive Neurocience Lab, University of Waterloo, Canada
-
Date/Duration:
2019-2021 (6 000 dollars CAD)
-
Additionnal info/keywords:
: Intrinsic motivation ; spatial learning
10.2 International research visitors
10.2.1 Visits of international scientists
Other international visits to the team
Paul Barde
-
Status:
PhD
-
Institution of origin:
McGill University, Quebec AI institute (Mila)
-
Country:
Canada
-
Dates:
From January to December 2021
-
Context of the visit:
Paul Barde visited the Flowers team in the context of a collaboration with Tristan Karch. The collaboration also involved Paul's PhD supervisors Christopher Pal and Derek Nowrouzezahrai. Paul and Tristan carried out a project on the emergence of communication in the Architect-Builder problem, a setup where two artificial agents with asymmetrical roles need to communicate in order to solve a collaborative task.
-
Mobility program/type of mobility:
Internship
10.2.2 Visits to international teams
Research stays abroad
: PY Oudeyer was invited at Microsoft Research Lab Montreal and at MILA/University of Montreal starting from september 2012 and until june 2022.
10.3 European initiatives
10.3.1 Horizon Europe
Without content for this year.
10.3.2 FP7 & H2020 projects
VeriDREAM
Participants: David Filliat [correspondant], Natalia Diaz Rodriguez.
The H2020 FET VeriDREAM project (VERtical Innovation in the Domain of Robotics Enabled by Artificial intelligence Mandhods) is a European project with the objective of developing industrial applications following the H2020 DREAM and RobDREAM projects.- Date: 2020 - 2022
- Amount: 204 000€
- Partners: Sorbonne Université, DLR, Magazino, GoodAI, Synesis
- Website: https://www.veridream.eu
10.3.3 Other european programs/initiatives
Without content for this year.
10.4 National initiatives
ANR Chaire Individuelle Deep Curiosity
- PY Oudeyer continued to work on the research program of this Chaire, funding 2 PhDs and 3 postdocs for five years (until 2025).
ANR JCJC ECOCURL
- C. Moulin-Frier obtained an ANR JCJC grant. The project is entitled "ECOCURL: Emergent communication through curiosity-driven multi-agent reinforcement learning". The project starts in Feb 2021 for a duration of 48 months. It will fund a PhD student (36 months) and a Research Engineer (18 months) as well as 4 Master internships (one per year).
Inria Exploratory Action ORIGINS
- Clément Moulin-Frier obtained an Exploratory Action from Inria. The project is entitled "ORIGINS: Grounding artificial intelligence in the origins of human behavior". The project starts in October 2020 for a duration of 24 months. It funds a post-doc position (24 months). Eleni Nisioti has been recruited on this grant.
Inria Exploratory Action AIDE
- Didier Roy is collaborator of the Inria Exploratory Action AIDE "Artificial Intelligence Devoted to Education", ported by Frédéric Alexandre (Inria Mnemosyne Project-Team), Margarida Romero (LINE Lab) and Thierry Viéville (Inria Mnemosyne Project-Team, LINE Lab). The aim of this Exploratory Action consists to explore to what extent approaches or methods from cognitive neuroscience, linked to machine learning and knowledge representation, could help to better formalize human learning as studied in educational sciences. AIDE is a four year project started middle 2020 until 2024. https://team.inria.fr/mnemosyne/aide/
Poppy Station structure:
- Poppy Station Project : D. Roy continued to support the development of the Poppy station NGO aiming to perpetuate the Poppy robot ecosystem and Poppy Education project by creating an external structure from outside Inria, with various partners in the field of education (La Ligue de l’Enseignement, HESAM Université, IFÉ-ENS Lyon, MOBOTS – EPFL, Génération Robots, Pollen Robotics, KONEXInc, Mobsya, CERN Microclub, LINE Lab (Université Nice), Stripes, Canopé Martinique, Rights Tech Women, Editions Nathan). Poppy Station, which includes the Poppy robot ecosystem (hardware, software, community) from the beginning, is a place of excellence to build future educational robots and to design pedagogical activities to teach computer science, robotics and Artificial Intelligence. Poppy Station participates in various national and international projects, prototypes new educational robots and provides training in educational robotics and AI. Web: https://www.poppy-station.org
- Partners of Poppy Station : Inria, La Ligue de l’Enseignement, HESAM Université, IFÉ-ENS Lyon, MOBOTS – EPFL, Génération Robots, Pollen Robotics, KONEXInc, Mobsya, CERN Microclub, LINE Lab (Université Nice), Stripes, Canopé Martinique, Rights Tech Women, Editions Nathan.
10.4.1 Adaptiv'Math
- Adaptiv'Math
- Program: PIA
- Duration: 2019 - 2020
- Coordinator: EvidenceB
- Partners:
- EvidenceB
- Nathan
- APMEP
- LIP6
- INRIA
- ISOGRAD
- Daesign
- Schoolab
- BlueFrog
The solution Adaptiv'Math comes from an innovation partnership for the development of a pedagogical assistant based on artificial intelligence. This partnership is realized in the context of a call for projects from the Ministry of Education to develop a pedagogical plateform to propose and manage mathematical activities intended for teachers and students of cycle 2. The role of Flowers team is to work on the AI of the proposed solution to personalize the pedagogical content to each student. This contribution is based on the work done during the Kidlearn Project and the thesis of Benjamin Clement 108, in which algorithms have been developed to manage and personalize sequence of pedagogical activities. One of the main goal of the team here is to transfer technologies developed in the team in a project with the perspective of industrial scaling.
10.5 Regional initiatives
10.6 SNPEA
(RNA and Inria)
- Associated Researcher: Hélène Sauzéon
- Date: 2020 - 2025
- Amount: 150 000€
- Participants: Pierre-Yves Oudeyer, Masataka Sawayama
- Description: The project's objective is twofold: 1) to adapt and develop new algorithms in machine learning to the field of attention training and 2) to evaluate with the help of experimental methods from psychology whether automated personalization according to progress generates more responders in elderly and young people with attentional disorders.
10.7 Evaluation TousEnsemble/ ToGatherAssessment project
(RNA)
- Associated Researcher: Hélène Sauzéon
- Date: 2021 - 2026
- Amount: 57 000€
- Participants: Cécile Mazon; Eric Meyer; Isabeau Saint-Supery; Christelle Maillart (Univ. Liège); Bordeaux Academy of National French education; Centre Ressources Autisme d'Aquitaine; ARI Association.
- Description: This project is the continuation of the Togather app. project. To validate the effectiveness of this new tool, a controlled study (control group vs. equipped group) is planned over 2 to 3 quarters with 60 students (ASD and/or ID). In addition, a study of applicability to the Walloon context will be conducted.
11 Dissemination
11.1 Promoting scientific activities
11.1.1 Scientific events: organisation
Member of the organizing committees
- Clément Moulin-Frier has co-organized the 1st and 2nd SMILES (Sensorimotor Interaction, Language and Embodiment of Symbols) workshop at ICDL 2020 and 2021. https://sites.google.com/view/smiles-workshop/.
- Hélène Sauzéon and Cécile Mazon were co-organizer of the CREATE Workshop on “Designing Technology for older adults”, Sep. 2021, Bordeaux. https://www.inria.fr/fr/technologies-personnes-agees-vieillesse-dependance.
- David Filliat is co-animating the Working group on Machine Learning and Robotics for the french GDR Robotique.
11.1.2 Scientific events: selection
Member of the conference program committees
- PY. Oudeyer has been in the conference program committee of ICML and AAAI.
- H. Sauzéon has been member of the scientific committee of “Journées d’étude du vieillissement cognitif” (Lyon 2021).
- D. Roy was member of the scientific committee for DIDAPRO Colloquium.
- D. Roy was member of the scientific committee for LUDOVIA Switzerland Conference.
- C. Moulin-Frier was associate editor for the ICDL conference.
Reviewer
- David Filliat was reviewer for the IROS, ICLR conferences.
- Clément Moulin-Frier has reviewed for the ICRA, NeurIPS and ICDL conferences.
- Cédric Colas reviewed for the ICML, ICLR and NeurIPS conferences.
- Rémy Portelas reviewed for the IJCAI and NeurIPS conferences.
- PY Oudeyer was a reviewer for AAAI, ICML.
- Didier Roy was a reviewer for PRUNE Conference (Poitiers).
- Didier Roy was a reviewer for RNRE (IFE ENS Lyon).
11.1.3 Journal
Member of the editorial boards
- PY. Oudeyer was associate editor of IEEE Transactions on CDS and Frontiers in Neurorobotics.
- Clément Moulin-Frier is co-editing a Research Topics in Frontiers: Emergent Behavior in Animal-inspired Robotics. https://www.frontiersin.org/research-topics/13627/emergent-behavior-in-animal-inspired-robotics.
- Hélène Sauzéon was associate editor for the special issue on Serious Games in Neurodevelopmental Disorders) for Frontiers in Psychiatry, section Child and Adolescent Psychiatry.
- S.M. Nguyen was guest editor for the special issue on continual unsupervised sensorimotor learning for IEEE Transactions on CDS.
- S.M. Nguyen was associate editor for IEEE Transactions on CDS.
Reviewer - reviewing activities
- David Filliat was reviewer for IEEE Transaction on Robotics, IEEE Transactions on Cognitive and Developmental Systems, Robotics and Automation Letters.
- PY Oudeyer has been a reviewer for the journal Child Development.
- N. Diaz Rodriguez was reviewer for: Transactions on Emerging Telecommunications Technologies, Knowledge-Based Systems, Robotics and Autonomous Systems, IEEE Robotics & Automation Magazine, IEEE Transactions on Cognitive and Developmental Systems.
- PY Oudeyer was reviewer for Journal of Artificial Intelligence Research, Journal of the Royal Society Interface, Cognitive Science, Child Development Perspectives, Nature Scientific Reports, Frontiers in Psychology, Handbook of Computational Psychology, Motivation and Emotion.
- Clément Moulin-Frier reviewed articles for Journal of Artificial Intelligence Research (JAIR) and Frontiers in Psychology.
- Hélène Sauzéon reviewed journal papers for Frontiers in psychiatry, Gerontechnology Journal and Gérontologie et société (Journal de la CNSA).
- Natalia Díaz Rodríguez reviewed at Frontiers in Robotics and AI, Transactions on Emerging Telecommunications Technologies, IEEE Robotics & Automation Magazine, Neurocomputing, Robotics and Autonomous Systems.
- Cédric Colas reviewed an article for the Robotics Science and Systems (RSS) conference.
- Mayalen Etcheverry has reviewed for the Applied Intelligence (APIN) journal.
- Rémy Portelas has reviewed for IEEE Robotics and Automation Letters (RA-L), KI – Künstliche Intelligenz journal, IJCAI survey track, NeurIPS Benchmark and Dataset track.
- Cecile Mazon reviewed an article for Computers in Human Behaviors (2020) and for Behavior Research and Therapy (2021), and is currently reviewing a paper for the International Journal of Evaluation and Research in Education.
- Laetitia Teodorescu has reviewed for CogSci 2021.
11.1.4 Invited talks
- Didier Roy gave an invited talk at Adaptiv’math project webinar on on Flowers researches and AI for education - 2021.
- Didier Roy gave an invited talk at Class’code AI webinar. on AI for education and education to AI - 2021.
- Didier Roy gave an invited talk at EPFL learning sciences conference, on Flowers researches and Poppy Station structure - 2019, 2020, 2021.
- Didier Roy gave an invited talk at COPIRELEM Colloquium 2021, on learning personalization with ZPDES Algorithm, Adaptiv’math.
- Didier Roy gave a keynote talk on Flowers researches and AI for education, at TablUcation 2021 Conference, organized by Institut de formation de l’Éducation nationale (IFEN) and Ministère de l’Éducation nationale, de l’Enfance et de la Jeunesse of Luxemburg.
- Clément Moulin-Frier gave invited talks at the Teratec workshop (2021), at the symposium “Preprogrammed: Innateness in Neuroscience and AI” (2021), at the “Brains@Bay” meetup (2021), and at Deepmind (2021).
- SM Nguyen gave invited talks for the network Robotics research in Nouvelle-Aquitaine, and at Idiap.
- Cécile Mazon gave a (remote) invited talk about Educational technologies for children with autism at the 8th “Colloque International en Education” (Apr. 2021, Montréal, Canada).
- Hélène Sauzéon gave a (remote) invited talk about Learning progress based approach for designing ITS, at the 10th EIAH (June. 2021, Fribourg, Switzerland).
- PY Oudeyer gave an IEEE CIS Distinguised Speaker presentation on Developmental AI for the IEEE CIS chapter in India (Feb 21).
- PY Oudeyer gave an invited talk at ELLIS workshop on Meta-Learning (March 21).
- PY Oudeyer gave an invited talk at the Affective Brain Lab, University College London (April 21).
- PY Oudeyer gave an invited talk at the Self-Supervised Learning workshop, ICLR 2021 (April 21).
- PY Oudeyer participated to a panel discussion at the Self-Supervised Learning workshop and at the Never-Ending Learning workshop at ICLR 2021 (April 21).
- PY Oudeyer gave an invited talk at the workshop on Learning to Learn, ICRA 2021 (May 21).
- PY Oudeyer gave an invited talk at the Journées Scientifiques Inria (June 21).
- PY Oudeyer was invited to give the inaugural talk of the Developing Minds seminar series, https://sites.google.com/view/developing-minds-series (Oct 21).
- PY Oudeyer gave an invited talk at MILA, University of Montreal (tea talk), https://sites.google.com/lisa.iro.umontreal.ca/tea-talks/fall-2021#h.wvsuh0updb8n.
- PY Oudeyer gave an invited talk at the Ecological Theory in RL workshop at Neurips (Dec 21).
- PY Oudeyer gave an invited talk at the december meeting of CIFAR Learning in Machines and Brain program (Dec 21).
11.1.5 Leadership within the scientific community
Flowers’ team members have been highly active withing the scientific community, including the organisation of events, editing journals, reviewing or giving invited talks.
11.1.6 Scientific expertise
- PY Oudeyer was a reviewer for Idex institute of University of Strasbourg (Feb 21).
- PY Oudeyer was a member of the jury selecting grants for PhDs in AI in the context of Plan IA at University of Bordeaux (April 21).
- PY Oudeyer was interviewed as an expert for the preparation of the national PEPR project on educational technologies (March 21).
- PY Oudeyer was interviewed as an expert by a working group of Senat on neurotechnologies (Nov 21).
- PY Oudeyer was a member of the advisory board of the BigScience project, https://bigscience.huggingface.co.
- PY Oudeyer was a reviewer for Agence Nationale de la Recherche (ANR).
- PY Oudeyer was a reviewer for Fondation Sciences Patrimoine.
- D. Filliat has been a member of the ANR ASTRID evaluation committee (2018-2021).
- Hélène Sauzéon was a reviewer for the ANR call on International and european and scientific networks.
- Hélène Sauzéon was in 2021 a member of HCERES committee for the assessment of LP3C - Laboratoire de psychologie : cognition, comportement, communication.
- Pierre-Yves Oudeyer and Hélène Sauzéon participated to several Selection committees for permanent positions as researcher (e.g., inria) or assistant professor at the university (2 committee organization for Assistant Professors positions at the Univ. of Bordeaux) , and for young and senior non permanent researchers position at inria (SRP and ARP).
- David Filliat is a member of the recruitment committee of the Computer Science department at Ecole Polytechnique (since 2018) and participated in the recruitment committee for a professor position at INRAE.
- Sao Mai Nguyen participated to a Selection committee an assistant professor at the university (ENSIBS-Matmeca).
11.1.7 Research administration
- PY Oudeyer has been member of piloting committees of consortium projects Adaptiv’Maths and Perseverons (eFran) on educational technologies.
- D.Roy has been member of scientific committee of consortium project Perseverons (eFran) on educational technologies.
- D.Roy has been member of project committee of consortium project Adaptiv’Maths on educational technologies.
- Hélène Sauzéon is the head of HACS team (BPH Lab, Inserm-UB), and thus member of directory committee of the Public Health Department of Univ. of Bordeaux.
- Helène Sauzéon is member of the directory committee of the « centre d’excellence BIND », and she manages the Industrial Innovation and transfer sub-committee.
- Hélène Sauzéon and Cécile Mazon are members of directory committee of LILLAB (https://www.lillabneurodev.fr/) which is a living and learning lab funded by the “délégation interministérielle à la stratégie nationale à l’autisme et troubles neurodéveloppementaux” and aiming the dissemination of knowledge in connection with the 3 centers of excellence for autism and Neurodevelopmental syndromes; since 2020.
- Hélène Sauzéon is member of directory committee of IFHR (https://ifr-handicap.inserm.fr/) which is a national institute on disability funded by Inserm aiming the researcher networking and dissemination of knowledge on multidisciplinary research on disability; since 2018.
- David Filliat was director of the Computer Science and System Engineering laboratory at ENSTA Paris (2018-2021).
- David Filliat is the scientific director of the Interdisciplinary Center for Defense and Security at IP Paris (since 2021).
11.2 Teaching - Supervision - Juries
11.2.1 Teaching
Teaching Responsibilities:
- Hélène Sauzéon is director of the curriculum in Technology, Ergonomics, Cognition and handicap (First and Second years of master degree in cognitive Science - University of Bordeaux) since sept. 2021.
- Hélène Sauzéon is in charge of the "Autonomy & Digital" axis of the Bordeaux instanciation of the PIA3 project (2018-22) Aspie-Friendly (P. Monthubert, Univ. Toulouse) aiming to develop digital tools to prepare, support the university inclusion of students with ASD.
- David Filliat is in charge since 2012 of the "Robotics and autonomous systems" third year speciality at ENSTA Paris.
- Sao Mai is in charge of the "Robot Learning" third year course at ENSTA Paris.
- Clément Moulin-Frier is responsible professor of the "System Design, Integration and Control" course at the University Pompeu Fabra in Barcelona, Spain.
- Cécile Mazon is responsible of the second year of the curriculum in Technology, Ergonomics, Cognition and Handicap (Cognitive Sciences - University of Bordeaux) since sept. 2021.
Teaching Involvement in Computer / Engineer science or in cognitive science:
- Université de Bordeaux: first year introductory course on programming 64h, sep. 2020 to jan. 2021 (Rémy Portelas and Tristan Karch)
- Bachelor: Introductory course on user experience, 5h, (Isabeau Saint-Supery)
- ENSC: basics of AI, 18h, sep. 2020 to jan. 2021 (Maxime Adolphe)
- ENSC: Introductory course on web development, 36h (Maxime Adolphe)
- ENSC/ENSEIRB: Deep generative models, 3h. option IA (Maxime Adolphe)
- ENSC/ENSEIRB: Reproducibility in deep learning, 6h. option IA (Maxime Adolphe)
- ENSC/ENSEIRB Presentation of developmental artificial intelligence and the Flowers Lab, 2h, Option Robot (Laetitia Teodorescu)
- BS & Master: Cognitive Science, Univ. of Bordeaux- , 96h, Hélène Sauzéon
- BS & Master: Cognitive Science, Univ. of Bordeaux- , 128h, Cécile Mazon
- University of Côtes d’Azur -International Master of SMART-EDTECH, Co-creativity, Digital technologies for educational innovation. 2h (H. Sauzéon)
- Master: Navigation for Robotics, 21 h, M2, ENSTA Paris, David Filliat
- Master: Navigation for Robotics, 24 h, M2 DataAI, IP Paris - Paris, David Filliat
- 2nd year : Deep Learning, 12h, IMT Atlantique (Sao Mai Nguyen).
- Master: Project Management, 12h, Isabeau Saint-Supery
- Master UPF-Barcelona: Robotics and AI, 10h (Clément Moulin-Frier)
- Master : PY Oudeyer taught a course (5h) on Developmental Machine Learning at CogMaster, University Paris-Sorbonne (Jan 21)
- Industry : PY Oudeyer gave a course (1h) on Developmental AI at the AI4Industry event, Bordeaux (Jan 21)
- Academic : PY Oudeyer gave an invited tutorial (1h) at the CoRL robot learning conference (Nov 21)
11.2.2 Supervision
- PhD in progress : Rémy Portelas, "Teacher algorithms for curriculum learning in Deep RL", beg. in sept. 2018 (supervisors: PY Oudeyer and K Hoffmann)
- PhD in progress : Mehdi Zadem, "Continuously Learning Complex Tasks via Symbolic Analysis", beg in June 2021 (supervisors : SM Nguyen and Sergio Mover and Alexandre Chapoutot and Sylvie Putot)
- PhD defended: Cédric Colas, "Intrinsically Motivated Deep RL", beg. in sept. 2017 (supervisors: PY Oudeyer and O Sigaud)
- PhD in progress: Tristan Karch, "Language acquisition in curiosity-driven Deep RL", beg. in sept. 2019 (supervisors: PY Oudeyer and C Moulin-Frier)
- PhD in progress: Alexander Ten, "Models of human curiosity-driven learning and exploration", beg. in sept. 2018 (supervisors: PY. Oudeyer and J. Gottlieb)
- PhD in progress: Laetitia Teodorescu, "Graph Neural Networks in Curiosity-driven Exploring Agents", beg. in sept. 2020 (supervisors: PY. Oudeyer and K. Hoffman)
- PhD in progress: Maxime Adolphe, "Adaptive personalization in attention training systems", beg. in sept. 2020 (supervisors: H. Sauzéon and PY. Oudeyer)
- PhD in progress: Rania Abdelgani, "Fostering curiosity and meta-cognitive skills in educational technologies", beg. in dec. 2020 (supervisors: H. Sauzéon and PY. Oudeyer.
- PhD in progress: Julius Taylor, "Emergent communication through curiosity-driven multi-agent reinforcement learning", beg. in nov. 2020 (supervisor: C Moulin-Frier and PY Oudeyer)
- PhD in progress: Mayalen Etcheverry, "Automated Discovery of Self-Organized Structures", beg. in sept. 2020 (supervisor: PY Oudeyer)
- PhD in progress: Adrien Bennetot, "Explainable continual learning for autonomous driving", Sorbonne University and ENSTA Paris (supervised by N Díaz Rodríguez & R Chatila).
- PhD in progress: Vyshakh Palli Thazha, "Data fusion for autonomous vehicles.", supervised by D. Fillait and J. Ibanez Guzman.
- PhD in progress: Isabeau Saint-Supery, "Designing and Assessing a new interactive tool fostering stakeholders' cooperation for school inclusion", supervised by H. Sauzéon and C. Mazon.
- Master Thesis Defended: Emma Tison, "The effect of curiosity-based encoding on spatial learning in children : A study of uncertainty effect." (supervised by H. Sauzéon and PY Oudeyer)
- Master Thesis Defended: Gautier Hamon, "Learning Sensorimotor Capabilities in Cellular Automata" (supervised by M Etcheverry, B Chan, C Moulin Frier and PY Oudeyer)
- PhD defended: Florence Carton, "Exploration of reinforcement learning algorithms for autonomous vehicle visual perception and control", supervisors: D Filliat, J. Rabarisoa and Q. C. Pham
- PhD defended: Timothée Lesort, "Continual Learning : Tackling Catastrophic Forgetting in Deep Neural Networks with Replay Processes", supervisors: D Filliat, J.F Goudou and A. Stoian
- PhD defended: Hugo Caselles-Dupré "On the role of Actions and Machine Learning in Artificial Agent Perception", supervisors: D Filliat, and M. Garcia-Ortiz
11.2.3 Juries
- H. Sauzéon has been member of two Thesis Juries in psychology.
- H. Sauzéon and C. Mazon are permanent members of jury of Master degree in cognitive science at the university of Bordeaux.
- Clément Moulin-Frier was member of the PhD jury of Sock-Chin Low (UPF Barcelona), of the "comité de suivi de thèse" de Marc-Antoine Georges (GIPSA-Lab, Grenoble), and of the Master thesis jury of Azim Maninani (UPF Barcelona).
- PY Oudeyer was a member of Thomas Moerland's PhD jury, "The intersection of planning and learning", University of Amsterdam.
- PY Oudeyer was a reviewer of Shoko Ota's PhD thesis, "Intrinsic Motivation in Creative Activity", OIST, Japan.
- PY Oudeyer was a reviewer of Benoit Choffin's PhD thesis, "Algorithmes d'espacement adaptatif de l'apprentissage pour l'optimisation de la maitrise à long terme de composantes de connaissance", University Paris-Saclay.
- PY Oudeyer was a member of Alexandre Zenon's HdR jury, University of Bordeaux.
- PY Oudeyer was a member of Marin Toromanoff's PhD thesis, "Apprentissage par renforcement du contrôle d’un véhicule autonome à partir de la vision", University PSL, Paris.
- PY Oudeyer was a member of Nicolas Lair's PhD jury, "Langage et Apprentissage en Interaction pour des Assistants Numériques Autonomes Une Approche Développementale", University of Franche-Comté.
- PY Oudeyer was a member of comités de suvi of R. Rashidi (Inria), E. Segas (Univ. Bordeaux), E. Menager (Inria), M. Josserand (Univ. Lyon).
- PY Oudeyer was a member of Alexander Pashevich's PhD thesis, "Robots that can see: Learning visually guided behavior", University of Grenoble.
11.3 Popularization
11.3.1 Internal or external Inria responsibilities
See the subsection 11.1.7.
11.3.2 Articles and contents
- Didier Roy and PY Oudeyer published an illustrated educational book on artificial intelligence and robotics for 7-8 years old children, Nathan, see https://www.nathan.fr/catalogue/fiche-produit.asp?ean13=9782092593295 and https://www.lemonde.fr/blog/binaire/2020/12/. This book has been selected for the final of the international Roberval prize 2021.
- Didier Roy was one of the authors of the Inria White Paper "Education and Digital, Challenges and Issues" https://hal.inria.fr/hal-03051329.
- H. Sauzéon co-wrote with P. Guitton, in 2020 a popularisation paper - Aïana, le lecteur de Mooc qui offre une accessibilité sur-mesure. La collection numérique de l’AMUE – Accessibilité numérique universitaire, N° 9, 42-43.
- Mayalen Etcheverry wrote a blogpost for the Minecraft Open-Endedness Challenge holded at GECCO 2021. https://mayalene.github.io/evocraftsearch/.
- Helene Sauzéon animated a Lilllab webinar on Curiosity and self-determination driven technologies for children with cognitive impairments, October, 21rst,2021, https://www.lillabneurodev.fr/events/webinaires-lillab/.
- Paul Germon (former intern supervised by Rémy portelas and Clément Romac) created an interactive website allowing to play and educate on generalization abilities of Deep RL methods: https://developmentalsystems.org/Interactive_DeepRL_Demo/.
- Clément Moulin-Frier was interviewed for an article in La Tribune in 2021: https://objectifaquitaine.latribune.fr/business/2021-07-19/intelligence-artificielle-et-epidemiologie-deux-clefs-pour-la-sante-publique-889192.html.
- Cécile Mazon gave a talk on technologies for special education and children with ASD at the webinar organized by the LilLab (Living and Learning Lab for Neurodevelopment, Oct. 2021). https://www.lillabneurodev.fr/events/webinaires-lillab/.
11.3.3 Education
See the subsection 11.2.
11.3.4 Interventions
- Members of the Flowers team participated to many interviews and documentaries for the press, the radio and television.
- Didier Roy has written an article for the journal of Palais de la Découverte: “A brief history of robotics, from Electric Dog to Poppy”.
- Didier Roy. R2T2 Richter event, remote robotics programming, in caribbean islands, in collaboration with EPFL.
- Hélène Sauzéon participated to several talks targeted disability-related professionals, students or industries, organised by Aspriefriendly PAI program or INSHE (Paris).
- PY Oudeyer, B. Clément, L. Teodorescu and D. Roy (2021) made interventions as part of the "Le Procès du robot" animation at Cap Sciences. The goal was to present in layman’s terms the research done at the lab for an audience of junior high school students and to foster discussion among them around an imagined scenario, about the legal responsibility of a domestic robot having caused a minor accient in a home. The web page of the intervention can be found there: https://www.cap-sciences.net/vous-etes/espace-enseignants/proces-robot.
- Pierre-Yves Oudeyer made several popular science interventions in Ecole Primaire AygueMarine (Ayguemorteles-Graves), College de Cadillac (of which he is "parrain scientifique" in the context of "Maison des sciences"), in particular for the CHICHE action.
- PY Oudeyer, H. Sauzéon, C. Moulin-Frier, D. Roy, M. Etcheverry, M. Adolphe and A. Ten received high-school student girl interns during one week in the lab: several activities were organized to foster discussions and motivations around numeric sciences.
- H. Sauzéon participated to the “1 scientifique, 1 classe : CHICHE!” action (Two classrooms of high school).
- Paul Germon (former intern), Rémy Portelas and Clément Romac, presented the interactive website on Deep RL generalization (https://developmentalsystems.org/Interactive_DeepRL_Demo/) during Le Village des Sciences at Cap Sciences (2021).
- Tristan Karch gave an invited talk on Vygotskian Autotelic Artificial Agents at the Minds at Play! workshop of CogSci 2021.
- Tristan Karch presented Vygotskian Autotelic Artificial Agents to the Cognitive Machine Learning Team at ENS/INRIA.
- Hélène Sauzéon and Didier Roy gave an interview for an Inria podcast : ”À quoi sert la recherche... en éducation numérique ?” (2021).
- Didier Roy gave an interview for EU-RATE : European Robotics Access To Everybody (2021).
- Didier Roy gave a keynote talk at Eidos64 Forum: AI for personalized learning: the kidlearn project and the ZPDES algorithm.
- Didier Roy was interviewed by JM Fourgous, Mayor of Elancourt, and his Office, on Educational technologies and advices for their projects.
- Didier Roy was jury member of hackathon “Digital technology for inclusive education” INSHEA (2021).
- Didier Roy was jury member of hackathon “AI with Thymio robot” MOBSYA, ROTECO, EPFL (2021)
- Didier Roy was jury member of Serge Hocquenghem Prize (2021).
- Didier Roy was expert and instructor for “Fondation Main à la Pâte” (from 2015).
- Cécile Mazon and Isabeau Saint-Supery animated 2 workshop sessions on the participatory design methodology for creating a collaborative website for stakeholders of school inclusion, INSHEA (Oct. 2021).
- Rania Abdelghani will give a talk for the third “European Advanced Educational Technology Conference” at the Imperial College, University of Cambridge, UK (March. 2022).
- Gautier Hamon, Mayalen Etcheverry and Bert Chan gave an invited talk for the Levin Lab at Tufts University Boston about the project "Learning Sensorimotor Agency in Cellular Automata" (November 2021).
- Clément Romac gave a talk on "Des algorithmes professeurs pour favoriser la généralisation dans l’apprentissage par renforcement profond" at the “IA en Nouvelle Aquitaine” event part of the “Réseau Régional de Recherche en Intelligence Artificielle” (R3IA) network (July 2021).
- Paul Germon, Clément Romac and Rémy Portelas created a web demonstration on Deep RL and generalization, available at http://developmentalsystems.org/TeachMyAgent/. They presented this demonstration during the 2021's edition of the "Village des Sciences" at the science fair CapSciences Bordeaux.
- H. Sauzéon participated as women researcher to the "AI and data science : where are the Women?" webinar organized by Digital Aquitaine b (March,10th, 2021). https://app.livestorm.co/digital-aquitaine/ia-data-science-ou-sont-les-femmes?type=detailed.
- PY Oudeyer gave a popular science presentation at Lycée Lac-Odyssée, Nouvelle Aquitaine (Jan 21).
- PY Oudeyer made 2 educational interventions introducing computer science and AI at primary school Ayguemarine, Ayguemorte-les-Graves.
- PY Oudeyer gave a population science presentation at Lycée Magendie, in the program Chiche (May 21).
12 Scientific production
12.1 Major publications
- 1 inproceedingsGrounding Language to Autonomously-Acquired Skills via Goal Generation.ICLR 2021 - Ninth International Conference on Learning RepresentationVienna / Virtual, AustriaMay 2021
- 2 inproceedingsPedagogical Agents for Fostering Question-Asking Skills in Children.CHI '20 - CHI Conference on Human Factors in Computing SystemsHonolulu / Virtual, United StatesApril 2020
- 3 articleActive Learning of Inverse Models with Intrinsically Motivated Goal Exploration in Robots.Robotics and Autonomous Systems611January 2013, 69-73
- 4 inproceedingsS-TRIGGER: Continual State Representation Learning via Self-Triggered Generative Replay.IJCNN 2021 - International Joint Conference on Neural NetworksShenzhen / Virtual, ChinaIEEEJuly 2021, 1-7
- 5 inproceedingsSymmetry-Based Disentangled Representation Learning requires Interaction with Environments.NeurIPS 2019Vancouver, CanadaDecember 2019
- 6 articleTowards Truly Accessible MOOCs for Persons with Cognitive Impairments: a Field Study.Human-Computer Interaction2021
- 7 inproceedingsCURIOUS: Intrinsically Motivated Modular Multi-Goal Reinforcement Learning.International Conference on Machine LearningLong Beach, FranceJune 2019
- 8 articleEpidemiOptim: a Toolbox for the Optimization of Control Policies in Epidemiological Models.Journal of Artificial Intelligence ResearchJuly 2021
- 9 inproceedingsLanguage as a Cognitive Tool to Imagine Goals in Curiosity-Driven Exploration.NeurIPS 2020 - 34th Conference on Neural Information Processing SystemsContains main article and supplementariesVancouver / Virtual, CanadaDecember 2020
- 10 inproceedingsGEP-PG: Decoupling Exploration and Exploitation in Deep Reinforcement Learning Algorithms.International Conference on Machine Learning (ICML)Stockholm, SwedenJuly 2018
- 11 articleExploring to learn visual saliency: The RL-IAC approach.Robotics and Autonomous Systems112February 2019, 244-259
- 12 articleIntrinsically Motivated Open-Ended Multi-Task Learning Using Transfer Learning to Discover Task Hierarchy.Applied Sciences113February 2021, 975
- 13 articleIntelligent Behavior Depends on the Ecological Niche.KI - Künstliche IntelligenzJanuary 2021
- 14 inproceedingsHierarchically Organized Latent Modules for Exploratory Search in Morphogenetic Systems.NeurIPS 2020 - 34th Conference on Neural Information Processing SystemsVancouver / Virtual, CanadaDecember 2020
- 15 unpublishedIntrinsically Motivated Goal Exploration Processes with Automatic Curriculum Learning.November 2017, working paper or preprint
- 16 inproceedingsA Unified Model of Speech and Tool Use Early Development.39th Annual Conference of the Cognitive Science Society (CogSci 2017)Proceedings of the 39th Annual Conference of the Cognitive Science SocietyLondon, United KingdomJuly 2017
- 17 articleTowards a neuroscience of active sampling and curiosity.Nature Reviews Neuroscience1912December 2018, 758-770
- 18 inproceedingsGrounding Spatio-Temporal Language with Transformers.NeurIPS 2021 - 35th Conference on Neural Information Processing SystemsVirtuel, FranceDecember 2021
- 19 inproceedingsCuriosity Driven Exploration of Learned Disentangled Goal Spaces.CoRL 2018 - Conference on Robot LearningZürich, SwitzerlandOctober 2018
- 20 articleState Representation Learning for Control: An Overview.Neural Networks108December 2018, 379-392
- 21 articleActive Navigation in Virtual Environments Benefits Spatial Memory in Older Adults.Brain Sciences92019
- 22 articleEmergent Jaw Predominance in Vocal Development through Stochastic Optimization.IEEE Transactions on Cognitive and Developmental Systems992017, 1-12
- 23 inproceedingsGrounding an Ecological Theory of Artificial Intelligence in Human Evolution.NeurIPS 2021 - Conference on Neural Information Processing Systems / Workshop: Ecological Theory of Reinforcement Learningvirtual event, FranceDecember 2021
- 24 inproceedingsUnsupervised Learning of Goal Spaces for Intrinsically Motivated Goal Exploration.ICLR2018 - 6th International Conference on Learning RepresentationsVancouver, CanadaApril 2018
- 25 inproceedingsTeacher algorithms for curriculum learning of Deep RL in continuously parameterized environments.CoRL 2019 - Conference on Robot Learninghttps://arxiv.org/abs/1910.07224Osaka, JapanOctober 2019
- 26 inproceedingsAutomatic Curriculum Learning For Deep RL: A Short Survey.IJCAI 2020 - International Joint Conference on Artificial IntelligenceKyoto / Virtuelle, JapanJanuary 2021
- 27 inproceedingsIntrinsically Motivated Discovery of Diverse Patterns in Self-Organizing Systems.International Conference on Learning Representations (ICLR)Source code and videos athttps://automated-discovery.github.io/Addis Ababa, EthiopiaApril 2020
- 28 inproceedingsTeachMyAgent: a Benchmark for Automatic Curriculum Learning in Deep RL.Proceedings of the 38th International Conference on MachineLearning, PMLR 139, 2021.ICML 2021 - Thirty-eighth International Conference on Machine Learning139Proceedings of the 38th International Conference on Machine LearningVienna / Virtual, AustriaJuly 2021, 9052--9063
- 29 articleHumans monitor learning progress in curiosity-driven exploration.Nature Communications121December 2021
- 30 inproceedingsTransflower: probabilistic autoregressive dance generation with multimodal attention.SIGGRAPH Asia 2021 - 14th ACM SIGGRAPH Conference and Exhibition on Computer Graphics and Interactive TechniquesTokyo, JapanDecember 2021
12.2 Publications of the year
International journals
International peer-reviewed conferences
National peer-reviewed Conferences
Conferences without proceedings
Scientific books
Scientific book chapters
Doctoral dissertations and habilitation theses
Reports & preprints
Other scientific publications
12.3 Other
Scientific popularization
12.4 Cited publications
- 88 articleValue-driven attentional capture.Proceedings of the National Academy of Sciences108252011, 10367--10371
- 89 inproceedingsTowards measuring states of epistemic curiosity through electroencephalographic signals.IEEE SMC 2020 - IEEE International conference on Systems, Man and CyberneticsToronto / Virtual, CanadaOctober 2020
- 90 articleA Survey of Robot Learning from Demonstration.Robotics and Autonomous Systems5752009, 469--483
- 91 articlePurposive Behavior Acquisition On A Real Robot By Vision-Based Reinforcement Learning.Machine Learning231996, 279-303
- 92 inproceedingsEmergent Tool Use From Multi-Agent Autocurricula.tex.ids: Baker2019 arXiv: 1909.075282020, URL: https://openreview.net/forum?id=SkxpxJBKwS
- 93 article Novelty or surprise? Frontiers in psychology 4 2013
- 94 inproceedingsIntrinsically Motivated Learning of Hierarchical Collections of Skills.Proceedings of the 3rd International Conference on Development and Learning (ICDL 2004)Salk Institute, San Diego2004
- 95 miscRelational inductive biases, deep learning, and graph networks.2018
- 96 bookConflict, Arousal and Curiosity.McGraw-Hill1960
- 97 bookThe Coordination and Regulation of Movements.Preliminary but descriptive evidence that in some tasks the activity of the number of degrees of freedom is initially reduced and subsequently increasedPergamon1967
- 98 inbookHuman Behavioural Ecology.eLSAmerican Cancer Society2012, URL: https://onlinelibrary.wiley.com/doi/abs/10.1002/9780470015902.a0003671.pub2
- 99 bookDesigning sociable robots.The MIT Press2004
- 100 inproceedingsAlternative essences of intelligence.Proceedings of 15th National Conference on Artificial Intelligence (AAAI-98)AAAI Press1998, 961--968
- 101 miscMONet: Unsupervised Scene Decomposition and Representation.2019
- 102 bookDevelopmental robotics: From babies to robots.MIT press2015
- 103 proceedingsLenia and Expanded Universe.ALIFE 2020: The 2020 Conference on Artificial LifeALIFE 2021: The 2021 Conference on Artificial Life07 2020, 221-229URL: https://doi.org/10.1162/isal_a_00297
- 104 articleLenia-biology of artificial life.Complex Systems2832019, 251-286
- 105 articleDesigning accessible MOOCs to expand educational opportunities for persons with cognitive impairments.Behaviour and Information Technologyhttps://www.tandfonline.com/doi/full/10.1080/0144929X.2020.1742381March 2020
- 106 articleOnline e-learning and cognitive disabilities: A systematic review.Computers and Education130March 2019, 152-167
- 107 bookMindware: An Introduction to the Philosophy of Cognitive Science.Oxford University Press2001
- 108 phdthesisAdaptive Personalization of Pedagogical Sequences using Machine Learning.Université de BordeauxDecember 2018
- 109 articleMulti-Armed Bandits for Intelligent Tutoring Systems.Journal of Educational Data Mining (JEDM)72June 2015, 20--48
- 110 articleActive learning with statistical models.Journal of artificial intelligence research41996, 129--145
- 111 bookCognitive Linguistics.Cambridge Textbooks in LinguisticsCambridge University Press2004
- 112 bookFlow-the psychology of optimal experience.Harper Perennial1991
- 113 articleReward, motivation and reinforcement learning.Neuron362002, 285--298
- 114 bookIntrinsic Motivation and Self-Determination in Human Behavior.Plenum Press1985
- 115 inproceedingsDécouverte et exploitation de la hiérarchie des tâches par motivation intrinsèque.Réunion "Apprentissage et Robotique"Visioconférence, Francehttp://www.gdr-isis.fr/index.php?page=reunion&idreunion=424June 2020
- 116 articleA computer science and robotics integration model for primary school: evaluation of a large-scale in-service K-4 teacher-training program.Education and Information TechnologiesNovember 2020
- 117 articleLearning and development in neural networks: The importance of starting small.Cognition481993, 71--99
- 118 miscIntrinsically Motivated Discovery of Diverse Patterns in Self-Organizing Systems.Self-organisation occurs in many physical, chemical and biological systems, as well as in artificial systems like the Game of Life. Yet, these systems are still full of mysteries and we are far from fully grasping what structures can self-organize, how to represent and classify them, and how to predict their evolution. In this blog post, we present our recent paper which formulates the problem of automated discovery of diverse self-organized patterns in such systems. Using a continuous Game of Life as a testbed, we show how intrinsically-motivated goal exploration processes, initially developed for learning of inverse models in robotics, can efficiently be transposed to this novel application area.March 2020
- 119 articleIndividual differences in the attribution of incentive salience to reward-related cues: Implications for addiction.Neuropharmacology562009, 139--148
- 120 articleEnriching behavioral ecology with reinforcement learning methods.Behavioural Processes1612019, 94--100URL: http://www.sciencedirect.com/science/article/pii/S0376635717303637
- 121 articleSocial complexity as a proximate and ultimate factor in communicative complexity.Philosophical Transactions of the Royal Society B: Biological Sciences3671597July 2012, 1785--1801URL: https://royalsocietypublishing.org/doi/10.1098/rstb.2011.0213
- 122 articleModeling the formation of social conventions from embodied real-time interactions.PLoS ONE156June 2020, e0234434
- 123 articleInformation-seeking, curiosity, and attention: computational and neural mechanisms.Trends in Cognitive Sciences1711November 2013, 585-93
- 124 articleA curious formulation robot enables the discovery of a novel protocell behavior.Science advances652020, eaay4237
- 125 inproceedingsSoft Actor-Critic: Off-Policy Maximum Entropy Deep Reinforcement Learning with a Stochastic Actor.Proceedings of the 35th International Conference on Machine Learning80Proceedings of Machine Learning ResearchStockholmsmässan, Stockholm SwedenPMLR10--15 Jul 2018, 1861--1870URL: http://proceedings.mlr.press/v80/haarnoja18b.html
- 126 articleThe symbol grounding problem.Physica D401990, 335--346
- 127 bookActive learning in neural networks.Heidelberg, Germany, GermanyPhysica-Verlag GmbH2002, 137--169
- 128 bookArtificial Intelligence: the very idea.Cambridge, MA, USAThe MIT Press1985
- 129 articleMesolimbocortical and nigrostriatal dopamine responses to salient non-reward events.Neuroscience9642000, 651-656
- 130 inproceedingsNovelty and reinforcement learning in the value system of developmental robots.Proceedings of the 2nd international workshop on Epigenetic Robotics : Modeling cognitive development in robotic systemsLund University Cognitive Studies 942002, 47--55
- 131 inproceedingsPerception and human interaction for developmental learning of objects and affordances.Proc. of the 12th IEEE-RAS International Conference on Humanoid Robots - HUMANOIDSforthcomingJapan2012, URL: http://hal.inria.fr/hal-00755297
- 132 articleHuman-level performance in 3D multiplayer games with population-based reinforcement learning.Science3646443Publisher: American Association for the Advancement of Science2019, 859--865
- 133 bookDevelopmental Cognitive Neuroscience.Blackwell publishing2005
- 134 bookDevelopmental cognitive neuroscience.Wiley-Blackwell2011
- 135 inproceedingsCLEVR: A Diagnostic Dataset for Compositional Language and Elementary Visual Reasoning.2017 IEEE Conference on Computer Vision and Pattern Recognition (CVPR)2017, 1988-1997
- 136 articleIn search of the neural circuits of intrinsic motivation.Frontiers in neuroscience12007, 17
- 137 articleThe psychology and neuroscience of curiosity.Neuron (in press)2015
- 138 articleAuto-encoding variational bayes.arXiv preprint arXiv:1312.61142013
- 139 miscContrastive Learning of Structured World Models.2020
- 140 articleBiological robustness.Nature Reviews Genetics5112004, 826--837
- 141 inproceedingsCombining manual feedback with subsequent MDP reward signals for reinforcement learning.Proceedings of the 9th International Conference on Autonomous Agents and Multiagent Systems (AAMAS'10)Toronto, Canada2010, 5--12
- 142 miscLearning not to learn: Nature versus nurture in silico.2020
- 143 articleAutocurricula and the emergence of innovation from social interaction: A manifesto for multi-agent intelligence research.arXiv preprint arXiv:1903.007422019
- 144 techreportYoung children calibrate effort based on the trajectory of their performance.PsyArXivMay 2021, URL: https://osf.io/hc62q
- 145 articleThe psychology of curiosity: A review and reinterpretation.Psychological bulletin11611994, 75
- 146 inproceedingsSimultaneous Acquisition of Task and Feedback Models.Development and Learning (ICDL), 2011 IEEE International Conference onGermany2011, 1 - 7URL: http://hal.inria.fr/hal-00636166/en
- 147 inproceedingsExploration in Model-based Reinforcement Learning by Empirically Estimating Learning Progress.Neural Information Processing Systems (NIPS)Lake Tahoe, United StatesDecember 2012, URL: http://hal.inria.fr/hal-00755248
- 148 inproceedingsThe Strategic Student Approach for Life-Long Exploration and Learning.2012, 8
- 149 articleDevelopmental Robotics: A Survey.Connection Science1542003, 151-190
- 150 inproceedingsDevelopmental Approach for Interactive Object Discovery.Neural Networks (IJCNN), The 2012 International Joint Conference onAustraliaJune 2012, 1-7
- 151 inproceedingsAn Emergent Framework for Self-Motivation in Developmental Robotics.Proceedings of the 3rd International Conference on Development and Learning (ICDL 2004)Salk Institute, San Diego2004
- 152 inproceedingsRobot Self-Initiative and Personalization by Learning through Repeated Interactions.6th ACM/IEEE International Conference on Human-RobotSwitzerland2011, URL: http://hal.inria.fr/hal-00636164/en
- 153 articleEffectiveness and usability of technology-based interventions for children and adolescents with ASD: A systematic review of reliability, consistency, generalization and durability related to the effects of intervention.Computers in Human Behavior93April 2019
- 154 articleA Region of Proximal Learning model of study time allocation.Journal of Memory and Language524May 2005, 463--477URL: https://linkinghub.elsevier.com/retrieve/pii/S0749596X04001330
- 155 bookTheories of developmental psychology.New York: Worth2001
- 156 bookTheories of developmental psychology.Worth2004
- 157 incollectionFunctions and mechanisms of intrinsic motivations.Intrinsically Motivated Learning in Natural and Artificial SystemsSpringer2013, 49--72
- 158 articleHuman-level control through deep reinforcement learning.Nature5187540February 2015, 529--533URL: http://www.nature.com/articles/nature14236
- 159 inproceedingsBootstrapping Intrinsically Motivated Learning with Human Demonstrations.IEEE International Conference on Development and LearningFrankfurt, Germany2011, URL: http://hal.inria.fr/hal-00645986/en
- 160 inproceedingsConstraining the Size Growth of the Task Space with Socially Guided Intrinsic Motivation using Demonstrations..IJCAI Workshop on Agents Learning Interactively from Human Teachers (ALIHT)Barcelona, Spain2011, URL: http://hal.inria.fr/hal-00645995/en
- 161 incollection L'auto-organisation dans l'évolution de la parole.Parole et Musique: Aux origines du dialogue humain, Colloque annuel du Collège de FranceOdile Jacob2009, 83-112URL: http://hal.inria.fr/inria-00446908/en/
- 162 incollectionDevelopmental Robotics.Encyclopedia of the Sciences of LearningSpringer Reference SeriesSpringer2011, URL: http://hal.inria.fr/hal-00652123/en
- 163 articleIntrinsic Motivation Systems for Autonomous Mental Development.IEEE Transactions on Evolutionary Computation1122007, 265--286
- 164 inproceedingsIntelligent adaptive curiosity: a source of self-development.Proceedings of the 4th International Workshop on Epigenetic Robotics117Lund University Cognitive Studies2004, 127--130
- 165 articleWhat is intrinsic motivation? A typology of computational approaches.Frontiers in Neurorobotics112007
- 166 incollectionSur les interactions entre la robotique et les sciences de l'esprit et du comportement.Informatique et Sciences Cognitives : influences ou confluences ?Presses Universitaires de France2009, URL: http://hal.inria.fr/inria-00420309/en/
- 167 inproceedingsThe dynamics of idealized attention in complex learning environments.IEEE International Conference on Development and Learning and on Epigenetic Robotics2015
- 168 articleArtificial intelligence exploration of unstable protocells leads to predictable properties and discovery of collective behavior.Proceedings of the National Academy of Sciences2018, 201711089
- 169 articleInfants tailor their attention to maximize learning.Science Advances639September 2020, eabb5053URL: https://advances.sciencemag.org/lookup/doi/10.1126/sciadv.abb5053
- 170 inbookImitation and Social Learning in Robots, Humans and Animals: Behavioural, Social and Communicative Dimensions.K.Kerstin DautenhahnC.C. NehanivCambridge University Press2004, How to build an imitator?
- 171 articleCurious eyes: Individual differences in personality predict eye movement behavior in scene-viewing.Cognition12212012, 86--90
- 172 article Which is the best intrinsic motivation signal for learning multiple skills? Frontiers in neurorobotics 7 2013
- 173 inproceedingsLearning motor dependent Crutchfield's information distance to anticipate changes in the topology of sensory body maps.IEEE International Conference on Learning and DevelopmentChine Shangai2009, URL: http://hal.inria.fr/inria-00420186/en/
- 174 articleEvolving internal reinforcers for an intrinsically motivated reinforcement-learning robot. IEEE 6th International Conference on Development and Learning, 2007. ICDL 2007.July 2007, 282-287URL: http://dx.doi.org/10.1109/DEVLRN.2007.4354052
- 175 inproceedingsCurious Model-Building Control Systems.Proceedings of the International Joint Conference on Neural Networks, Singapore2IEEE press1991, 1458--1463
- 176 articleA neural substrate of prediction and reward.Science2751997, 1593-1599
- 177 articleMastering the game of Go with deep neural networks and tree search.nature52975872016, 484--489
- 178 articleA general reinforcement learning algorithm that masters chess, shogi, and Go through self-play.Science36264192018, 1140--1144URL: https://science.sciencemag.org/content/362/6419/1140
- 179 articleMetacognitive Control and Optimal Learning.Cognitive Science2006, 16
- 180 inproceedingsExploiting regularity without development.Proceedings of the AAAI Fall Symposium on Developmental SystemsAAAI Press Menlo Park, CA2006, 37
- 181 bookL.Luc SteelsR.Rodney BrooksThe Artificial Life Route to Artificial Intelligence: Building Embodied, Situated Agents.Hillsdale, NJ, USAL. Erlbaum Associates Inc.1995
- 182 inproceedingsToddlers Always Get the Last Word: Recency biases in early verbal behavior.Proceedings of the 37th Annual Meeting of the Cognitive Science Society2015
- 183 bookA dynamic systems approach to the development of cognition and action.Cambridge, MAMIT Press1994
- 184 articleTeachable robots: Understanding human teaching behavior to build more effective robot learners.Artificial Intelligence Journal1722008, 716-737
- 185 articleJudgments of learning and improvement.Memory & Cognition392February 2011, 204--216URL: http://link.springer.com/10.3758/s13421-010-0019-2
- 186 articleMetacognitive Judgments of Improvement are Uncorrelated with Learning Rate.2011, 6
- 187 articleComputing machinery and intelligence.Mind591950, 433-460
- 188 articleMedia multitasking and memory: Differences in working memory and long-term memory.Psychonomic bulletin & review2015, 1--8
- 189 inproceedingsHow An Automated Gesture Imitation Game Can Improve Social Interactions With Teenagers With ASD.IEEE ICRA Workshop on Social Robotics for Neurodevelopmental DisordersParis, FranceJune 2020
- 190 bookThe embodied mind : Cognitive science and human experience.Cambridge, MAMIT Press1991
- 191 inproceedingsStudying the joint role of partial observability and channel reliability in emergent communication.1st SMILES (Sensorimotor Interaction, Language and Embodiment of Symbols) workshop, ICDL 2020Valparaiso / Virtual, Chilehttps://sites.google.com/view/smiles-workshop/November 2020
- 192 miscPaired Open-Ended Trailblazer (POET): Endlessly Generating Increasingly Complex and Diverse Learning Environments and Their Solutions.2019
- 193 articleAutonomous mental development by robots and animals.Science2912001, 599-600