Keywords
Computer Science and Digital Science
- A5.1.3. Haptic interfaces
- A5.1.5. Body-based interfaces
- A5.1.9. User and perceptual studies
- A5.4.2. Activity recognition
- A5.4.5. Object tracking and motion analysis
- A5.4.8. Motion capture
- A5.5.4. Animation
- A5.6. Virtual reality, augmented reality
- A5.6.1. Virtual reality
- A5.6.3. Avatar simulation and embodiment
- A5.6.4. Multisensory feedback and interfaces
- A5.10.3. Planning
- A5.10.5. Robot interaction (with the environment, humans, other robots)
- A5.11.1. Human activity analysis and recognition
- A6. Modeling, simulation and control
Other Research Topics and Application Domains
- B1.2.2. Cognitive science
- B2.5. Handicap and personal assistances
- B2.8. Sports, performance, motor skills
- B5.1. Factory of the future
- B5.8. Learning and training
- B7.1.1. Pedestrian traffic and crowds
- B9.2.2. Cinema, Television
- B9.2.3. Video games
- B9.4. Sports
1 Team members, visitors, external collaborators
Research Scientists
- Franck Multon [Team leader, Inria, Senior Researcher, HDR]
- Adnane Boukhayma [Inria, Researcher]
- Ludovic Hoyet [Inria, Researcher]
- Katja Zibrek [Inria, Starting Research Position]
Faculty Members
- Benoit Bardy [Univ de Montpellier, Professor, from Sep 2021, HDR]
- Nicolas Bideau [Univ Rennes2, Associate Professor]
- Benoit Bideau [Univ Rennes2, Professor, HDR]
- Marc Christie [Univ de Rennes I, Associate Professor]
- Armel Crétual [Univ Rennes, Associate Professor, HDR]
- Georges Dumont [École normale supérieure de Rennes, Professor, HDR]
- Diane Haering [Univ Rennes2, Associate Professor]
- Aline Hufschmitt [Univ de Rennes I, Associate Professor, from Oct 2021]
- Simon Kirchhofer [École normale supérieure de Rennes, Associate Professor, until Aug 2021]
- Richard Kulpa [Univ Rennes2, Associate Professor, HDR]
- Fabrice Lamarche [Univ de Rennes I, Associate Professor]
- Guillaume Nicolas [Univ Renne, Associate Professor]
- Anne-Hélène Olivier [Univ Rennes2, Associate Professor, HDR]
- Charles Pontonnier [École normale supérieure de Rennes, Associate Professor, HDR]
Post-Doctoral Fellows
- Pierre Raimbaud [Inria]
- Divyaksh Subhash Chander [ENS Rennes]
PhD Students
- Vicenzo Abichequer-Sangalli [Inria, co-supervised with Rainbow]
- Jean Basset [Inria, until Aug 2021]
- Jean Baptiste Bordier [Univ de Rennes I]
- Alexandre Bruckert [Univ de Rennes1, From 11/2021]
- Ludovic Burg [Univ de Rennes I]
- Thomas Chatagnon [Inria, co-supervised with Rainbow]
- Adèle Colas [Inria, co-supervised with Rainbow]
- Rebecca Crolan [École normale supérieure de Rennes, from Oct 2021]
- Louise Demestre [École normale supérieure de Rennes]
- Diane Dewez [Inria, co-supervised with Hybrid]
- Olfa Haj Mahmoud [Faurecia, until Dec.2]
- Nils Hareng [Univ Rennes2]
- Alberto Jovane [Inria, co-supervised with Rainbow]
- Qian Li [Inria]
- Claire Livet [École normale supérieure de Rennes]
- Pauline Morin [École normale supérieure de Rennes]
- Lucas Mourot [InterDigital]
- Benjamin Niay [Inria]
- Nicolas Olivier [InterDigital]
- Carole Puil [IFPEK Rennes, until Sep 2022]
- Xiaoyuan Wang [École normale supérieure de Rennes, from Oct 2021]
- Xi Wang [Univ de Rennes I, until Oct 2021]
- Tairan Yin [Inria, co-supervised with Rainbow]
- Mohamed Younes [Inria]
Technical Staff
- Robin Adili [Inria, Engineer]
- Robin Courant [Univ de Rennes1, From 11/2021]
- Ronan Gaugne [Univ de Rennes I, Engineer]
- Ific Goude [Univ de Rennes I, Engineer, from Jun 2021]
- Laurent Guillo [CNRS, Engineer]
- Shubhendu Jena [Inria, Engineer]
- Nena Markovic [Univ de Rennes1, From 12/2021]
- Maé Mavromatis [Univ de Rennes1]
- Anthony Mirabile [Univ de Rennes I, Engineer]
- Adrien Reuzeau [Inria, Engineer]
- Salome Ribault [Inria, Engineer, from Oct 2021]
- Xiaofang Wang [Univ de Reims Champagne-Ardennes, Engineer, until Oct 2021]
Interns and Apprentices
- Francois Bourel [Univ de Rennes I, from Apr 2021 until Oct 2021]
- Vincent Etien [Inria, from Feb 2021 until Jul 2021]
- Quentin Gomelet-Richard [Inria, from Jul 2021 until Nov 2021]
- Capucine Leroux [Polytech Sorbonne, from Apr 2021 until Jul 2021]
- Badr Ouannas [Inria, from Mar 2021 until Aug 2021]
- Amine Ouasfi [Inria, from Mar 2021 until Aug 2021]
- Hugo Pottier [Inria, from Feb 2021 until Jun 2021]
- Arnaud Roger [Inria, from Feb 2021 until Jul 2021]
Administrative Assistant
- Nathalie Denis [Inria]
Visiting Scientist
- Radoslaw Sterna [Jagiellonian University, Krakow, Poland, Jul 2021]
External Collaborator
- Anthony Sorel [Univ Rennes2, until Aug 2021]
2 Overall objectives
2.1 Presentation
MimeTIC is a multidisciplinary team whose aim is to better understand and model human activity in order to simulate realistic autonomous virtual humans: realistic behaviors, realistic motions and realistic interactions with other characters and users. It leads to modeling the complexity of a human body, as well as of his environment where he can pick-up information and he can act on it. A specific focus is dedicated to human physical activity and sports as it raises the highest constraints and complexity when addressing these problems. Thus, MimeTIC is composed of experts in computer science whose research interests are computer animation, behavioral simulation, motion simulation, crowds and interaction between real and virtual humans. MimeTIC also includes experts in sports science, motion analysis, motion sensing, biomechanics and motion control. Hence, the scientific foundations of MimeTIC are motion sciences (biomechanics, motion control, perception-action coupling, motion analysis), computational geometry (modeling of the 3D environment, motion planning, path planning) and design of protocols in immersive environments (use of virtual reality facilities to analyze human activity).
Thanks to these skills, we wish to reach the following objectives: to make virtual humans behave, move and interact in a natural manner in order to increase immersion and improve knowledge on human motion control. In real situations (see Figure 1), people have to deal with their physiological, biomechanical and neurophysiological capabilities in order to reach a complex goal. Hence MimeTIC addresses the problem of modeling the anatomical, biomechanical and physiological properties of human beings. Moreover these characters have to deal with their environment. Firstly they have to perceive this environment and pick-up relevant information. Thus, MimeTIC focuses on the problem of modeling the environment including its geometry and associated semantic information. Second, they have to act on this environment to reach their goals. It leads to cognitive processes, motion planning, joint coordination and force production in order to act on this environment.
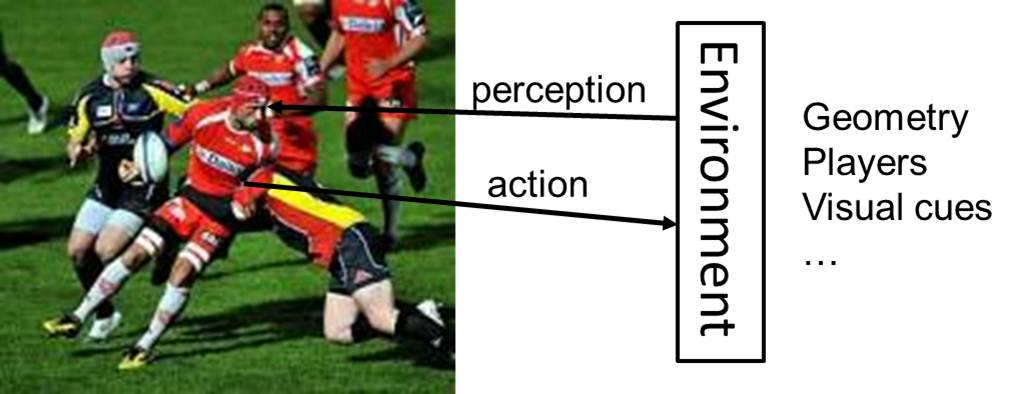
In order to reach the above objectives, MimeTIC has to address three main challenges:
- deal with the intrinsic complexity of human beings, especially when addressing the problem of interactions between people for which it is impossible to predict and model all the possible states of the system,
- make the different components of human activity control (such as the biomechanical and physical, the reactive, cognitive, rational and social layers) interact while each of them is modeled with completely different states and time sampling,
- and measure human activity while balancing between ecological and controllable protocols, and to be able to extract relevant information in wide databases of information.
As opposed to many classical approaches in computer simulation, which mostly propose simulation without trying to understand how real people act, the team promotes a coupling between human activity analysis and synthesis, as shown in Figure 2.
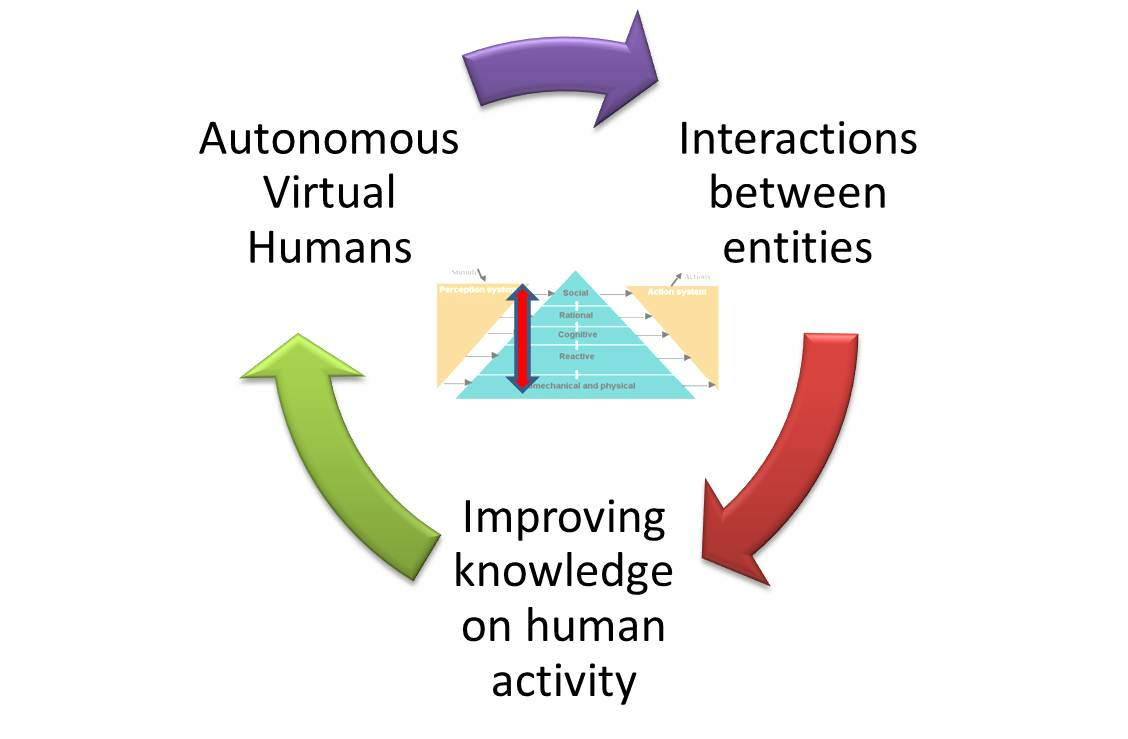
In this research path, improving knowledge on human activity enables us to highlight fundamental assumptions about natural control of human activities. These contributions can be promoted in e.g. biomechanics, motion sciences, neurosciences. According to these assumptions we propose new algorithms for controlling autonomous virtual humans. The virtual humans can perceive their environment and decide of the most natural action to reach a given goal. This work is promoted in computer animation, virtual reality and has some applications in robotics through collaborations. Once autonomous virtual humans have the ability to act as real humans would in the same situation, it is possible to make them interact with others, i.e., autonomous characters (for crowds or group simulations) as well as real users. The key idea here is to analyze to what extent the assumptions proposed at the first stage lead to natural interactions with real users. This process enables the validation of both our assumptions and our models.
Among all the problems and challenges described above, MimeTIC focuses on the following domains of research:
- motion sensing which is a key issue to extract information from raw motion capture systems and thus to propose assumptions on how people control their activity,
- human activity & virtual reality, which is explored through sports application in MimeTIC. This domain enables the design of new methods for analyzing the perception-action coupling in human activity, and to validate whether the autonomous characters lead to natural interactions with users,
- interactions in small and large groups of individuals, to understand and model interactions with lot of individual variability such as in crowds,
- virtual storytelling which enables us to design and simulate complex scenarios involving several humans who have to satisfy numerous complex constraints (such as adapting to the real-time environment in order to play an imposed scenario), and to design the coupling with the camera scenario to provide the user with a real cinematographic experience,
- biomechanics which is essential to offer autonomous virtual humans who can react to physical constraints in order to reach high-level goals, such as maintaining balance in dynamic situations or selecting a natural motor behavior among the whole theoretical solution space for a given task,
- autonomous characters which is a transversal domain that can reuse the results of all the other domains to make these heterogeneous assumptions and models provide the character with natural behaviors and autonomy.
3 Research program
3.1 Biomechanics and Motion Control
Human motion control is a highly complex phenomenon that involves several layered systems, as shown in Figure 3. Each layer of this controller is responsible for dealing with perceptual stimuli in order to decide the actions that should be applied to the human body and his environment. Due to the intrinsic complexity of the information (internal representation of the body and mental state, external representation of the environment) used to perform this task, it is almost impossible to model all the possible states of the system. Even for simple problems, there generally exists an infinity of solutions. For example, from the biomechanical point of view, there are much more actuators (i.e. muscles) than degrees of freedom leading to an infinity of muscle activation patterns for a unique joint rotation. From the reactive point of view there exists an infinity of paths to avoid a given obstacle in navigation tasks. At each layer, the key problem is to understand how people select one solution among these infinite state spaces. Several scientific domains have addressed this problem with specific points of view, such as physiology, biomechanics, neurosciences and psychology.
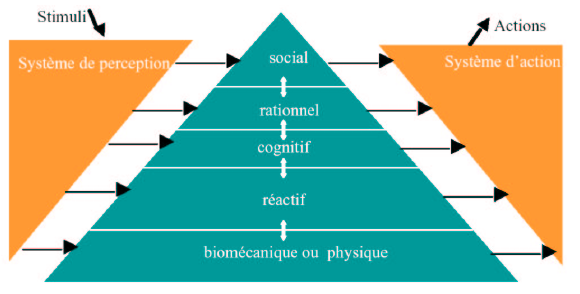
In biomechanics and physiology, researchers have proposed hypotheses based on accurate joint modeling (to identify the real anatomical rotational axes), energy minimization, force and torques minimization, comfort maximization (i.e. avoiding joint limits), and physiological limitations in muscle force production. All these constraints have been used in optimal controllers to simulate natural motions. The main problem is thus to define how these constraints are composed altogether such as searching the weights used to linearly combine these criteria in order to generate a natural motion. Musculoskeletal models are stereotyped examples for which there exists an infinity of muscle activation patterns, especially when dealing with antagonist muscles. An unresolved problem is to define how to use the above criteria to retrieve the actual activation patterns, while optimization approaches still leads to unrealistic ones. It is still an open problem that will require multidisciplinary skills including computer simulation, constraint solving, biomechanics, optimal control, physiology and neuroscience.
In neuroscience, researchers have proposed other theories, such as coordination patterns between joints driven by simplifications of the variables used to control the motion. The key idea is to assume that instead of controlling all the degrees of freedom, people control higher level variables which correspond to combinations of joint angles. In walking, data reduction techniques such as Principal Component Analysis have shown that lower-limb joint angles are generally projected on a unique plane whose angle in the state space is associated with energy expenditure. Although knowledge exists for specific motions, such as locomotion or grasping, this type of approach is still difficult to generalize. The key problem is that many variables are coupled and it is very difficult to objectively study the behavior of a unique variable in various motor tasks. Computer simulation is a promising method to evaluate such type of assumptions as it enables to accurately control all the variables and to check if it leads to natural movements.
Neuroscience also addresses the problem of coupling perception and action by providing control laws based on visual cues (or any other senses), such as determining how the optical flow is used to control direction in navigation tasks, while dealing with collision avoidance or interception. Coupling of the control variables is enhanced in this case as the state of the body is enriched by the large amount of external information that the subject can use. Virtual environments inhabited with autonomous characters whose behavior is driven by motion control assumptions is a promising approach to solve this problem. For example, an interesting problem in this field is to navigate in an environment inhabited with other people. Typically, avoiding static obstacles along with other people moving inside that environment is a combinatory problem that strongly relies on the coupling between perception and action.
One of the main objectives of MimeTIC is to enhance knowledge on human motion control by developing innovative experiments based on computer simulation and immersive environments. To this end, designing experimental protocols is a key point and some of the researchers in MimeTIC have developed this skill in biomechanics and perception-action coupling. Associating these researchers to experts in virtual human simulation, computational geometry and constraints solving enable us to contribute to enhance fundamental knowledge in human motion control.
3.2 Experiments in Virtual Reality
Understanding interactions between humans is challenging because it addresses many complex phenomena including perception, decision-making, cognition and social behaviors. Moreover, all these phenomena are difficult to isolate in real situations, and it is therefore highly complex to understand their individual influence on these human interactions. It is then necessary to find an alternative solution that can standardize the experiments and that allows the modification of only one parameter at a time. Video was first used since the displayed experiment is perfectly repeatable and cut-offs (stop the video at a specific time before its end) allow having temporal information. Nevertheless, the absence of adapted viewpoint and stereoscopic vision does not provide depth information that are very meaningful. Moreover, during video recording sessions, a real human acts in front of a camera and not in front of an opponent. That interaction is then not a real interaction between humans.
Virtual Reality (VR) systems allow full standardization of the experimental situations and the complete control of the virtual environment. It allows to modify only one parameter at a time and observe its influence on the perception of the immersed subject. VR can then be used to understand what information is picked up to make a decision. Moreover, cut-offs can also be used to obtain temporal information about when information is picked up. When the subject can react as in a real situation, his movement (captured in real time) provides information about his reactions to the modified parameter. Not only is the perception studied, but the complete perception-action loop. Perception and action are indeed coupled and influence each other as suggested by Gibson in 1979.
Finally, VR allows the validation of virtual human models. Some models are indeed based on the interaction between the virtual character and the other humans, such as a walking model. In that case, there are two ways to validate it. First, they can be compared to real data (e.g. real trajectories of pedestrians). But such data are not always available and are difficult to get. The alternative solution is then to use VR. The validation of the realism of the model is then done by immersing a real subject into a virtual environment in which a virtual character is controlled by the model. Its evaluation is then deduced from how the immersed subject reacts when interacting with the model and how realistic he feels the virtual character is.
3.3 Computer Animation
Computer animation is the branch of computer science devoted to models for the representation and simulation of the dynamic evolution of virtual environments. A first focus is the animation of virtual characters (behavior and motion). Through a deeper understanding of interactions using VR and through better perceptive, biomechanical and motion control models to simulate the evolution of dynamic systems, the Mimetic team has the ability to build more realistic, efficient and believable animations. Perceptual study also enables us to focus computation time on relevant information (i.e. leading to ensure natural motion from the perceptual points of view) and save time for unperceived details. The underlying challenges are (i) the computational efficiency of the system which needs to run in real-time in many situations, (ii) the capacity of the system to generalise/adapt to new situations for which data were not available, or models were not defined for, and (iii) the variability of the models, i.e. their ability to handle many body morphologies and generate variations in motions that would be specific to each virtual character.
In many cases, however, these challenges cannot be addressed in isolation. Typically character behaviors also depend on the nature and the topology of the environment they are surrounded by. In essence, a character animation system should also rely on smarter representations of the environments, in order to better perceive the environment itself, and take contextualised decisions. Hence the animation of virtual characters in our context often requires to be coupled with models to represent the environment, to reason, and to plan both at a geometric level (can the character reach this location), and at a semantic level (should it use the sidewalk, the stairs, or the road). This represents the second focus. Underlying challenges are the ability to offer a compact -yet precise- representation on which efficient path, motion planning and high-level reasoning can be performed.
Finally, a third scientific focus is digital storytelling. Evolved representations of motions and environments enable realistic animations. It is yet equally important to question how these events should be portrayed, when and under which angle. In essence, this means integrating discourse models into story models, the story representing the sequence of events which occur in a virtual environment, and the discourse representing how this story should be displayed (i.e. which events to show in which order and with which viewpoint). Underlying challenges are pertained to:
- narrative discourse representations,
- projections of the discourse into the geometry, planning camera trajectories and planning cuts between the viewpoints,
- means to interactively control the unfolding of the discourse.
By therefore establishing the foundations to build bridges between the high-level narrative structures, the semantic/geometric planning of motions and events, and low-level character animations, the Mimetic team adopts a principled and all-inclusive approach to the animation of virtual characters.
4 Application domains
4.1 Animation, Autonomous Characters and Digital Storytelling
Computer Animation is one of the main application domains of the research work conducted in the MimeTIC team, in particular in relation to the entertainment and game industries. In these domains, creating virtual characters that are able to replicate real human motions and behaviours still highlights unanswered key challenges, especially as virtual characters are required to populate virtual worlds. For instance, virtual characters are used to replace secondary actors and generate highly populated scenes that would be hard and costly to produce with real actors. This requires to create high quality replicas that appear, move and behave both individually and collectively like real humans. The three key challenges for the MimeTIC team are therefore:
- to create natural animations (i.e., virtual characters that move like real humans),
- to create autonomous characters (i.e., that behave like real humans),
- to orchestrate the virtual characters so as to create interactive stories.
First, our challenge is to create animations of virtual characters that are natural, i.e. moving like a real human real would. This challenge covers several aspects of Character Animation depending on the context of application, e.g., producing visually plausible or physically correct motions, producing natural motion sequences, etc. Our goal is therefore to develop novel methods for animating virtual characters, based on motion capture, data-driven approaches, or learning approaches. However, because of the complexity of human motion (number of degrees of freedom that can be controlled), resulting animations are not necessarily physically, biomechanically, or visually plaisible. For instance, current physics-based approaches produce physically correct motions but not necessarily perceptually plausible ones. All these reasons are why most entertainment industries (gaming and movie production for example) still mainly rely on manual animation. Therefore, research in MimeTIC on character animation is also conducted with the goal of validating the results from objective standpoint (physical, biomechanical) as well as subjective one (visual plausibility).
Second, one of the main challenges in terms of autonomous characters is to provide a unified architecture for the modeling of their behavior. This architecture includes perception, action and decisional parts. This decisional part needs to mix different kinds of models, acting at different time scales and working with different natures of data, ranging from numerical (motion control, reactive behaviors) to symbolic (goal oriented behaviors, reasoning about actions and changes). For instance, autonomous characters play the role of actors that are driven by a scenario in video games and virtual storytelling. Their autonomy allows them to react to unpredictable user interactions and adapt their behavior accordingly. In the field of simulation, autonomous characters are used to simulate the behavior of humans in different kinds of situations. They enable to study new situations and their possible outcomes. In the MimeTIC team, our focus is therefore not to reproduce the human intelligence but to propose an architecture making it possible to model credible behaviors of anthropomorphic virtual actors evolving/moving in real-time in virtual worlds. The latter can represent particular situations studied by psychologists of the behavior or to correspond to an imaginary universe described by a scenario writer. The proposed architecture should mimic all the human intellectual and physical functions.
Finally, interactive digital storytelling, including novel forms of edutainment and serious games, provides access to social and human themes through stories which can take various forms and contains opportunities for massively enhancing the possibilities of interactive entertainment, computer games and digital applications. It provides chances for redefining the experience of narrative through interactive simulations of computer-generated story worlds and opens many challenging questions at the overlap between computational narratives, autonomous behaviours, interactive control, content generation and authoring tools. Of particular interest for the MimeTIC research team, virtual storytelling triggers challenging opportunities in providing effective models for enforcing autonomous behaviours for characters in complex 3D environments. Offering both low-level capacities to characters such as perceiving the environments, interacting with the environment itself and reacting to changes in the topology, on which to build higher-levels such as modelling abstract representations for efficient reasoning, planning paths and activities, modelling cognitive states and behaviours requires the provision of expressive, multi-level and efficient computational models. Furthermore virtual storytelling requires the seamless control of the balance between the autonomy of characters and the unfolding of the story through the narrative discourse. Virtual storytelling also raises challenging questions on the conveyance of a narrative through interactive or automated control of the cinematography (how to stage the characters, the lights and the cameras). For example, estimating visibility of key subjects, or performing motion planning for cameras and lights are central issues for which have not received satisfactory answers in the literature.
4.2 Fidelity of Virtual Reality
VR is a powerful tool for perception-action experiments. VR-based experimental platforms allow exposing a population to fully controlled stimuli that can be repeated from trial to trial with high accuracy. Factors can be isolated and objects manipulations (position, size, orientation, appearance, ..) are easy to perform. Stimuli can be interactive and adapted to participants’ responses. Such interesting features allow researchers to use VR to perform experiments in sports, motion control, perceptual control laws, spatial cognition as well as person-person interactions. However, the interaction loop between users and their environment differs in virtual conditions in comparison with real conditions. When a user interacts in an environment, movement from action and perception are closely related. While moving, the perceptual system (vision, proprioception,..) provides feedback about the users’ own motion and information about the surrounding environment. That allows the user to adapt his/her trajectory to sudden changes in the environment and generate a safe and efficient motion. In virtual conditions, the interaction loop is more complex because it involves several material aspects.
First, the virtual environment is perceived through a numerical display which could affect the available information and thus could potentially introduce a bias. For example, studies observed a distance compression effect in VR, partially explained by the use of a Head Mounted Display with reduced field of view and exerting a weight and torques on the user’s head. Similarly, the perceived velocity in a VR environment differs from the real world velocity, introducing an additional bias. Other factors, such as the image contrast, delays in the displayed motion and the point of view can also influence efficiency in VR. The second point concerns the user’s motion in the virtual world. The user can actually move if the virtual room is big enough or if wearing a head mounted display. Even with a real motion, authors showed that walking speed is decreased, personal space size is modified and navigation in VR is performed with increased gait instability. Although natural locomotion is certainly the most ecological approach, the physical limited size of VR setups prevents from using it most of the time. Locomotion interfaces are therefore required. They are made up of two components, a locomotion metaphor (device) and a transfer function (software), that can also introduce bias in the generated motion. Indeed, the actuating movement of the locomotion metaphor can significantly differ from real walking, and the simulated motion depends on the transfer function applied. Locomotion interfaces cannot usually preserve all the sensory channels involved in locomotion.
When studying human behavior in VR, the aforementioned factors in the interaction loop potentially introduce bias both in the perception and in the generation of motor behavior trajectories. MimeTIC is working on the mandatory step of VR validation to make it usable for capturing and analyzing human motion.
4.3 Motion Sensing of Human Activity
Recording human activity is a key point of many applications and fundamental works. Numerous sensors and systems have been proposed to measure positions, angles or accelerations of the user's body parts. Whatever the system is, one of the main problems is to be able to automatically recognize and analyze the user's performance according to poor and noisy signals. Human activity and motion are subject to variability: intra-variability due to space and time variations of a given motion, but also inter-variability due to different styles and anthropometric dimensions. MimeTIC has addressed the above problems in two main directions.
First, we have studied how to recognize and quantify motions performed by a user when using accurate systems such as Vicon (product of Oxford Metrics), Qualisys, or Optitrack (product of Natural Point) motion capture systems. These systems provide large vectors of accurate information. Due to the size of the state vector (all the degrees of freedom) the challenge is to find the compact information (named features) that enables the automatic system to recognize the performance of the user. Whatever the method used, finding these relevant features that are not sensitive to intra-individual and inter-individual variability is a challenge. Some researchers have proposed to manually edit these features (such as a Boolean value stating if the arm is moving forward or backward) so that the expertise of the designer is directly linked with the success ratio. Many proposals for generic features have been proposed, such as using Laban notation which was introduced to encode dancing motions. Other approaches tend to use machine learning to automatically extract these features. However most of the proposed approaches were used to seek a database for motions, whose properties correspond to the features of the user's performance (named motion retrieval approaches). This does not ensure the retrieval of the exact performance of the user but a set of motions with similar properties.
Second, we wish to find alternatives to the above approach which is based on analyzing accurate and complete knowledge on joint angles and positions. Hence new sensors, such as depth-cameras (Kinect, product of Microsoft) provide us with very noisy joint information but also with the surface of the user. Classical approaches would try to fit a skeleton into the surface in order to compute joint angles which, again, lead to large state vectors. An alternative would be to extract relevant information directly from the raw data, such as the surface provided by depth cameras. The key problem is that the nature of these data may be very different from classical representation of human performance. In MimeTIC, we try to address this problem in some application domains that require picking specific information, such as gait asymmetry or regularity for clinical analysis of human walking.
4.4 Sports
Sport is characterized by complex displacements and motions. One main objective is to understand the determinants of performance through the analysis of the motion itself. In the team, different sports have been studied such as the tennis serve, where the goal was to understand the contribution of each segment of the body in the performance but also the risk of injuries as well as other situation in cycling, swimming, fencing or soccer. Sport motions are dependent on visual information that the athlete can pick up in his environment, including the opponent's actions. Perception is thus fundamental to the performance. Indeed, a sportive action, as unique, complex and often limited in time, requires a selective gathering of information. This perception is often seen as a prerogative for action. It then takes the role of a passive collector of information. However, as mentioned by Gibson in 1979, the perception-action relationship should not be considered sequentially but rather as a coupling: we perceive to act but we must act to perceive. There would thus be laws of coupling between the informational variables available in the environment and the motor responses of a subject. In other words, athletes have the ability to directly perceive the opportunities of action directly from the environment. Whichever school of thought considered, VR offers new perspectives to address these concepts by complementary using real time motion capture of the immersed athlete.
In addition to better understand sports and interactions between athletes, VR can also be used as a training environment as it can provide complementary tools to coaches. It is indeed possible to add visual or auditory information to better train an athlete. The knowledge found in perceptual experiments can be for example used to highlight the body parts that are important to look at, in order to correctly anticipate the opponent's action.
4.5 Ergonomics
The design of workstations nowadays tends to include assessment steps in a Virtual Environment (VE) to evaluate ergonomic features. This approach is more cost-effective and convenient since working directly on the Digital Mock-Up (DMU) in a VE is preferable to constructing a real physical mock-up in a Real Environment (RE). This is substantiated by the fact that a Virtual Reality (VR) set-up can be easily modified, enabling quick adjustments of the workstation design. Indeed, the aim of integrating ergonomics evaluation tools in VEs is to facilitate the design process, enhance the design efficiency, and reduce the costs.
The development of such platforms asks for several improvements in the field of motion analysis and VR. First, interactions have to be as natural as possible to properly mimic the motions performed in real environments. Second, the fidelity of the simulator also needs to be correctly evaluated. Finally, motion analysis tools have to be able to provide in real-time biomechanics quantities usable by ergonomists to analyse and improve the working conditions.
In real working condition, motion analysis and musculoskeletal risk assessment raise also many scientific and technological challenges. Similarly to virtual reality, fidelity of the working process may be affected by the measurement method. Wearing sensors or skin markers, together with the need of frequently calibrating the assessment system may change the way workers perform the tasks. Whatever the measurement is, classical ergonomic assessments generally address one specific parameter, such as posture, or force, or repetitions..., which makes it difficult to design a musculoskeletal risk factor that actually represents this risk. Another key scientific challenge is then to design new indicators that better capture the risk of musculoskeletal disorders. However, this indicator has to deal with the trade-off between accurate biomechanical assessment and the difficulty to get reliable and required information in real working conditions.
4.6 Locomotion and Interactions between walkers
Modeling and simulating locomotion and interactions between walkers is a very active, complex and competitive domain, being investigating by various disciplines such as mathematics, cognitive sciences, physics, computer graphics, rehabilitation etc. Locomotion and interactions between walkers are by definition at the very core of our society since they represent the basic synergies of our daily life. When walking in the street, we should produce a locomotor movement while taking information about our surrounding environment in order to interact with people, move without collision, alone or in a group, intercept, meet or escape to somebody. MimeTIC is an international key contributor in the domain of understanding and simulating locomotion and interactions between walkers. By combining an approach based on Human Movement Sciences and Computer Sciences, the team focuses on locomotor invariants which characterize the generation of locomotor trajectories, conducts challenging experiments focusing on visuo-motor coordination involved during interactions between walkers both using real and virtual set-ups. One main challenge is to consider and model not only the "average" behaviour of healthy younger adult but also extend to specific populations considering the effect of pathology or the effect of age (kids, older adults). As a first example, when patients cannot walk efficiently, in particular those suffering from central nervous system affections, it becomes very useful for practitioners to benefit from an objective evaluation of their capacities. To facilitate such evaluations, we have developed two complementary indices, one based on kinematics and the other one on muscle activations. One major point of our research is that such indices are usually only developed for children whereas adults with these affections are much more numerous. We extend this objective evaluation by using person-person interaction paradigm which allows studying visuo-motor strategies deficit in these specific populations.
Another fundamental question is the adaptation of the walking pattern according to anatomical constraints, such as pathologies in orthopedics, or adaptation to various human and non-human primates in paleoanthropoly. Hence, the question is to predict plausible locomotion according to a given morphology. This question raises fundamental questions about the variables that are regulated to control gait: balance control, minimum energy, minimum jerk...In MimeTIC we develop models and simulators to efficiently test hypothesis on gait control for given morphologies.
5 Highlights of the year
- MimeTIC is leading DIGISPORT, one of the 24 PIA3 EUR projects (among 81 projects submitted). This project aims at creating a graduate school to support multidisciplinary research on sports, with the main Rennes actors in sports sciences, computer sciences, electronics, data sciences, and human and social sciences. The project launch meeting took place on December 10, 2021 with political, financial, sports, academic and socio-professional representatives. It marks the launch of the project, with already 18 funding and thesis accreditations, but also and above all the opening of the DIGISPORT Master's degree, co-accredited by the two Rennes universities and the grandes écoles INSA, ENS, ENSAI, CentraleSupelec.
- A member of MimeTIC is leading one of the 12 PIA PPR projects "Sport Très Haute Performance" whose objective is to optimize the performance of French athletes for the Paris 2024 Olympics. The objective of the REVEA project is to exploit the unique properties of virtual reality to optimize the perceptual-motor and cognitive processes underlying performance. The French Boxing Federation wishes to improve the perceptual-motor anticipation capacities of boxers in opposition situations while reducing impacts and thus the risk of injuries. The French Athletics Federation wishes to improve the perceptual-motor anticipation capacities of athletes in cooperation situations (4x100m relay) without running at high intensity. The French Gymnastics Federation wishes to optimize the movements of its gymnasts by observing their own motor production to avoid increasing the physical training load even more.
5.1 Awards
- Franck Multon received the price "Transition" from the "Trophées Valorisation du Campus d'innovation", University of Rennes, for his involvement in the transfer of KIMEA software to the Moovency start-up company. November 2021.
6 New software and platforms
MimeTIC is strongly involved in the immerStar platform described below.
6.1 New software
6.1.1 AsymGait
-
Name:
Asymmetry index for clinical gait analysis based on depth images
-
Keywords:
Motion analysis, Kinect, Clinical analysis
-
Scientific Description:
The system uses depth images delivered by the Microsoft Kinect to retrieve the gait cycles first. To this end it is based on a analyzing the knees trajectories instead of the feet to obtain more robust gait event detection. Based on these cycles, the system computes a mean gait cycle model to decrease the effect of noise of the system. Asymmetry is then computed at each frame of the gait cycle as the spatial difference between the left and right parts of the body. This information is computed for each frame of the cycle.
-
Functional Description:
AsymGait is a software package that works with Microsoft Kinect data, especially depth images, in order to carry-out clinical gait analysis. First it identifies the main gait events using the depth information (footstrike, toe-off) to isolate gait cycles. Then it computes a continuous asymmetry index within the gait cycle. Asymmetry is viewed as a spatial difference between the two sides of the body.
-
Contact:
Franck Multon
-
Participants:
Edouard Auvinet, Franck Multon
6.1.2 Cinematic Viewpoint Generator
-
Keyword:
3D animation
-
Functional Description:
The software, developed as an API, provides a mean to automatically compute a collection of viewpoints over one or two specified geometric entities, in a given 3D scene, at a given time. These viewpoints satisfy classical cinematographic framing conventions and guidelines including different shot scales (from extreme long shot to extreme close-up), different shot angles (internal, external, parallel, apex), and different screen compositions (thirds,fifths, symmetric of di-symmetric). The viewpoints allow to cover the range of possible framings for the specified entities. The computation of such viewpoints relies on a database of framings that are dynamically adapted to the 3D scene by using a manifold parametric representation and guarantee the visibility of the specified entities. The set of viewpoints is also automatically annotated with cinematographic tags such as shot scales, angles, compositions, relative placement of entities, line of interest.
-
Contact:
Marc Christie
-
Participants:
Christophe Lino, Emmanuel Badier, Marc Christie
-
Partners:
Université d'Udine, Université de Nantes
6.1.3 CusToM
-
Name:
Customizable Toolbox for Musculoskeletal simulation
-
Keywords:
Biomechanics, Dynamic Analysis, Kinematics, Simulation, Mechanical multi-body systems
-
Scientific Description:
The present toolbox aims at performing a motion analysis thanks to an inverse dynamics method.
Before performing motion analysis steps, a musculoskeletal model is generated. Its consists of, first, generating the desire anthropometric model thanks to models libraries. The generated model is then kinematical calibrated by using data of a motion capture. The inverse kinematics step, the inverse dynamics step and the muscle forces estimation step are then successively performed from motion capture and external forces data. Two folders and one script are available on the toolbox root. The Main script collects all the different functions of the motion analysis pipeline. The Functions folder contains all functions used in the toolbox. It is necessary to add this folder and all the subfolders to the Matlab path. The Problems folder is used to contain the different study. The user has to create one subfolder for each new study. Once a new musculoskeletal model is used, a new study is necessary. Different files will be automaticaly generated and saved in this folder. All files located on its root are related to the model and are valuable whatever the motion considered. A new folder will be added for each new motion capture. All files located on a folder are only related to this considered motion.
-
Functional Description:
Inverse kinematics Inverse dynamics Muscle forces estimation External forces prediction
- Publications:
-
Contact:
Charles Pontonnier
-
Participants:
Antoine Muller, Charles Pontonnier, Georges Dumont, Pierre Puchaud, Anthony Sorel, Claire Livet, Louise Demestre
6.1.4 Directors Lens Motion Builder
-
Keywords:
Previzualisation, Virtual camera, 3D animation
-
Functional Description:
Directors Lens Motion Builder is a software plugin for Autodesk's Motion Builder animation tool. This plugin features a novel workflow to rapidly prototype cinematographic sequences in a 3D scene, and is dedicated to the 3D animation and movie previsualization industries. The workflow integrates the automated computation of viewpoints (using the Cinematic Viewpoint Generator) to interactively explore different framings of the scene, proposes means to interactively control framings in the image space, and proposes a technique to automatically retarget a camera trajectory from one scene to another while enforcing visual properties. The tool also proposes to edit the cinematographic sequence and export the animation. The software can be linked to different virtual camera systems available on the market.
-
Contact:
Marc Christie
-
Participants:
Christophe Lino, Emmanuel Badier, Marc Christie
-
Partner:
Université de Rennes 1
6.1.5 Kimea
-
Name:
Kinect IMprovement for Egronomics Assessment
-
Keywords:
Biomechanics, Motion analysis, Kinect
-
Scientific Description:
Kimea consists in correcting skeleton data delivered by a Microsoft Kinect in an ergonomics purpose. Kimea is able to manage most of the occlultations that can occur in real working situation, on workstations. To this end, Kimea relies on a database of examples/poses organized as a graph, in order to replace unreliable body segments reconstruction by poses that have already been measured on real subject. The potential pose candidates are used in an optimization framework.
-
Functional Description:
Kimea gets Kinect data as input data (skeleton data) and correct most of measurement errors to carry-out ergonomic assessment at workstation.
- Publications:
-
Contact:
Franck Multon
-
Participants:
Franck Multon, Hubert Shum, Pierre Plantard
-
Partner:
Faurecia
6.1.6 Populate
-
Keywords:
Behavior modeling, Agent, Scheduling
-
Scientific Description:
The software provides the following functionalities:
- A high level XML dialect that is dedicated to the description of agents activities in terms of tasks and sub activities that can be combined with different kind of operators: sequential, without order, interlaced. This dialect also enables the description of time and location constraints associated to tasks.
- An XML dialect that enables the description of agent's personal characteristics.
- An informed graph describes the topology of the environment as well as the locations where tasks can be performed. A bridge between TopoPlan and Populate has also been designed. It provides an automatic analysis of an informed 3D environment that is used to generate an informed graph compatible with Populate.
- The generation of a valid task schedule based on the previously mentioned descriptions.
With a good configuration of agents characteristics (based on statistics), we demonstrated that tasks schedules produced by Populate are representative of human ones. In conjunction with TopoPlan, it has been used to populate a district of Paris as well as imaginary cities with several thousands of pedestrians navigating in real time.
-
Functional Description:
Populate is a toolkit dedicated to task scheduling under time and space constraints in the field of behavioral animation. It is currently used to populate virtual cities with pedestrian performing different kind of activities implying travels between different locations. However the generic aspect of the algorithm and underlying representations enable its use in a wide range of applications that need to link activity, time and space. The main scheduling algorithm relies on the following inputs: an informed environment description, an activity an agent needs to perform and individual characteristics of this agent. The algorithm produces a valid task schedule compatible with time and spatial constraints imposed by the activity description and the environment. In this task schedule, time intervals relating to travel and task fulfillment are identified and locations where tasks should be performed are automatically selected.
-
Contact:
Fabrice Lamarche
-
Participants:
Carl-Johan Jorgensen, Fabrice Lamarche
6.1.7 PyNimation
-
Keywords:
Moving bodies, 3D animation, Synthetic human
-
Scientific Description:
PyNimation is a python-based open-source (AGPL) software for editing motion capture data which was initiated because of a lack of open-source software enabling to process different types of motion capture data in a unified way, which typically forces animation pipelines to rely on several commercial software. For instance, motions are captured with a software, retargeted using another one, then edited using a third one, etc. The goal of Pynimation is therefore to bridge the gap in the animation pipeline between motion capture software and final game engines, by handling in a unified way different types of motion capture data, providing standard and novel motion editing solutions, and exporting motion capture data to be compatible with common 3D game engines (e.g., Unity, Unreal). Its goal is also simultaneously to provide support to our research efforts in this area, and it is therefore used, maintained, and extended to progressively include novel motion editing features, as well as to integrate the results of our research projects. At a short term, our goal is to further extend its capabilities and to share it more largely with the animation/research community.
-
Functional Description:
PyNimation is a framework for editing, visualizing and studying skeletal 3D animations, it was more particularly designed to process motion capture data. It stems from the wish to utilize Python’s data science capabilities and ease of use for human motion research.
In its version 1.0, Pynimation offers the following functionalities, which aim to evolve with the development of the tool : - Import / Export of FBX, BVH, and MVNX animation file formats - Access and modification of skeletal joint transformations, as well as a certain number of functionalities to manipulate these transformations - Basic features for human motion animation (under development, but including e.g. different methods of inverse kinematics, editing filters, etc.). - Interactive visualisation in OpenGL for animations and objects, including the possibility to animate skinned meshes
-
Authors:
Ludovic Hoyet, Robin Adili, Benjamin Niay, Alberto Jovane
-
Contact:
Ludovic Hoyet
6.1.8 The Theater
-
Keywords:
3D animation, Interactive Scenarios
-
Functional Description:
The Theater is a software framework to develop interactive scenarios in virtual 3D environements. The framework provides means to author and orchestrate 3D character behaviors and simulate them in real-time. The tools provides a basis to build a range of 3D applications, from simple simulations with reactive behaviors, to complex storytelling applications including narrative mechanisms such as flashbacks.
-
Contact:
Marc Christie
-
Participant:
Marc Christie
6.2 New platforms
6.2.1 Immerstar Platform
Participants: Georges Dumont [contact], Ronan Gaugne, Anthony Sorel, Richard Kulpa.
With the two platforms of virtual reality, Immersia) and Immermove Immermove, grouped under the name Immerstar, the team has access to high level scientific facilities. This equipment benefits the research teams of the center and has allowed them to extend their local, national and international collaborations. The Immerstar platform was granted by a Inria CPER funding for 2015-2019 that enabled important evolutions of the equipment. In 2016, the first technical evolutions have been decided and, in 2017, these evolutions have been implemented. On one side, for Immermove, the addition of a third face to the immersive space, and the extension of the Vicon tracking system have been realized and continued this year with 23 new cameras. And, on the second side, for Immersia, the installation of WQXGA laser projectors with augmented global resolution, of a new tracking system with higher frequency and of new computers for simulation and image generation in 2017. In 2018, a Scale One haptic device has been installed. It allows, as in the CPER proposal, one or two handed haptic feedback in the full space covered by Immersia and possibility of carrying the user. Based on these supports, in 2020, we participated to a PIA3-Equipex+ proposal. This proposal CONTINUUM involves 22 partners, has been succesfully evaluated and will be granted. The CONTINUUM project will create a collaborative research infrastructure of 30 platforms located throughout France, to advance interdisciplinary research based on interaction between computer science and the human and social sciences. Thanks to CONTINUUM, 37 research teams will develop cutting-edge research programs focusing on visualization, immersion, interaction and collaboration, as well as on human perception, cognition and behaviour in virtual/augmented reality, with potential impact on societal issues. CONTINUUM enables a paradigm shift in the way we perceive, interact, and collaborate with complex digital data and digital worlds by putting humans at the center of the data processing workflows. The project will empower scientists, engineers and industry users with a highly interconnected network of high-performance visualization and immersive platforms to observe, manipulate, understand and share digital data, real-time multi-scale simulations, and virtual or augmented experiences. All platforms will feature facilities for remote collaboration with other platforms, as well as mobile equipment that can be lent to users to facilitate onboarding. The kick-off meeting of continuum will be held in 2022, Januray the 14th.
7 New results
7.1 Outline
In 2021, MimeTIC has maintained its activity in motion analysis,modelling and simulation, to support the idea that these approaches are strongly coupled in a motion analysis-synthesis loop. This idea has been applied to the main application domains of MimeTIC:
- Animation, Autonomous Characters and Digital Storytelling,
- Fidelity of Virtual Reality,
- Motion sensing of Human Activity,
- Sports,
- Ergonomics,
- Locomotion and Interactions Between Walkers.
7.2 Animation, Autonomous Characters and Digital Storytelling
MimeTIC main research path consists in associating motion analysis and synthesis to enhance the naturalness in computer animation, with applications in camera control, movie previsualisation, and autonomous virtual character control. Thus, we pushed example-based techniques in order to reach a good trade-off between simulation efficiency and naturalness of the results. In 2021, to achieve this goal, MimeTIC continued to explore the use of perceptual studies and model-based approaches, but also began to investigate deep learning.
7.2.1 Binary Graph Descriptor for Robust Relocalization on Heterogeneous Data
Participants: Marc Christie [contact], Xi Wang.
Motion analysis in open and uncontrolled environments remains a challenging task. We here address the specific issue of robust relocalization of cameras that finds many applications in SLAM system (Simultaneous Localization and Mapping): extracting camera trajectories from movies, robust augmented reality, motion matching, etc. In 2021, we proposed a novel binary graph descriptor to improve loop detection for visual SLAM systems. Our contribution is twofold: i) a graph embedding technique for generating binary descriptors which conserve both spatial and histogram information extracted from images; ii) a generic mean of combining multiple layers of heterogeneous data into the proposed binary graph descriptor, coupled with a matching and geometric checking method. We also introduce an implementation of our descriptor into an incremental Bag-of-Words (iBoW) structure that improves efficiency and scalability, and propose a method to interpret Deep Neural Network (DNN) results. We evaluate our system on synthetic and real datasets across different lighting and seasonal conditions. The proposed method outperforms state-of-the-art loop detection frameworks in terms of relocalization precision and computational performance, as well as displays high robustness against cross-condition datasets. Paper and results are reported in 30.
7.2.2 TT SLAM: Dense Monocular SLAM for Planar Environments
Participants: Marc Christie [contact], Xi Wang.
As an addition to 30, we also report our 2021 work on a novel visual SLAM method with dense planar reconstruction using a monocular camera: TT-SLAM. The method exploits planar template-based trackers (TT) to compute camera poses and reconstructs a multi planar scene representation. Multiple homographies are estimated simultaneously by clustering a set of template trackers supported by superpixelized regions. Compared to RANSAC-based multiple homographies method, data association and keyframe selection issues are handled by the continuous nature of template trackers. A non-linear optimization process is applied to all the homographies to improve the precision in pose estimation. Experiments show that the proposed method outperforms RANSAC-based multiple homographies method as well as other dense method SLAM techniques such as LSD-SLAM or DPPTAM. It competes with keypoint-based techniques like ORB-SLAM while providing dense planar reconstructions of the environment. The work is reported in 41.
7.2.3 Analyzing visual attention in movies
Participants: Marc Christie [contact], Alexandre Bruckert.
In the overarching objective of better understanding movies through their high-level visual characteristics (camera motions, camera angles, scene layouts and mise-en-scene), we have been trying to search for better automated visual saliency techniques. Indeed, the way a film director plays with the spectator gaze is a key stylistic feature. A first work consisted in better estimating this visual saliency by improving existing techniques through well-designed loss functions. Indeed, deep learning techniques are widely used to model human visual saliency, to such a point that state-of-the-art performances are now only attained by deep neural networks. However, one key part of a typical deep learning model is often neglected when it comes to modeling visual saliency: the choice of the loss function.
In this work, we explored some of the most popular loss functions that are used in deep saliency models. We demonstrate that on a fixed network architecture, modifying the loss function can significantly improve (or depreciate) the results, hence emphasizing the importance of the choice of the loss function when designing a model. We also evaluate the relevance of new loss functions for saliency prediction inspired by metrics used in style-transfer tasks. Finally, we show that a linear combination of several well-chosen loss functions leads to significant improvements in performance on different datasets as well as on a different network architecture, thus demonstrating the robustness of a combined metric.
By building on our progressive understanding of saliency in movies, we actually demonstrated that current state of the art visual saliency techniques, trained on non-cinematographic contents, fail to capture the real spectator gaze displacements in a number of situations. Indeed, in the process of making a movie, directors constantly care about where the spectator will look on the screen. Shot composition, framing, camera movements or editing are tools commonly used to direct attention. In order to provide a quantitative analysis of the relationship between those tools and gaze patterns, we propose a new eye-tracking database, containing gaze pattern information on movie sequences, as well as editing annotations. We show how state-of-the-art computational saliency techniques behave on this dataset. In this work, we expose strong links between movie editing and spectators scanpaths, and open several leads on how the knowledge of editing information could improve human visual attention modeling for cinematic content49. The dataset generated and analysed during the current study is available at https://github. com/abruckert/eye_tracking_filmmaking.
7.2.4 High-Level Features for Movie Style Understanding
Participants: Marc Christie [contact], Robin Courant.
After identifying the lack of high-level feature extraction techniques for movies, we proposed a new set of stylistic features (character pose, camera pose, scene depth, focus map, frame layering and camera motion). Automatically analysing such stylistic features in movies is a challenging task, as it requires an in-depth knowledge of cinematography. In the literature, only a handful of methods explore stylistic feature extraction, and they typically focus on limited low-level image and shot features (colour histograms, average shot lengths or shot types, amount of camera motion). However, it only capture a subset of the stylistic features which help to characterise a movie (e.g. black and white vs. coloured, or film editing). To this end, we systematically explore seven high-level features for movie style analysis: character segmentation, pose estimation, depth maps, focus maps, frame layering, camera motion type and camera pose. Our findings show that low-level features remain insufficient for movie style analysis, while high-level features seem promising. These results are reported in 43 and received the best paper award at the ICCV workshop on AI for Creative Video Editing and Understanding.
7.2.5 Camera Keyframing with Style and Control
Participants: Marc Christie [contact], Xi Wang.
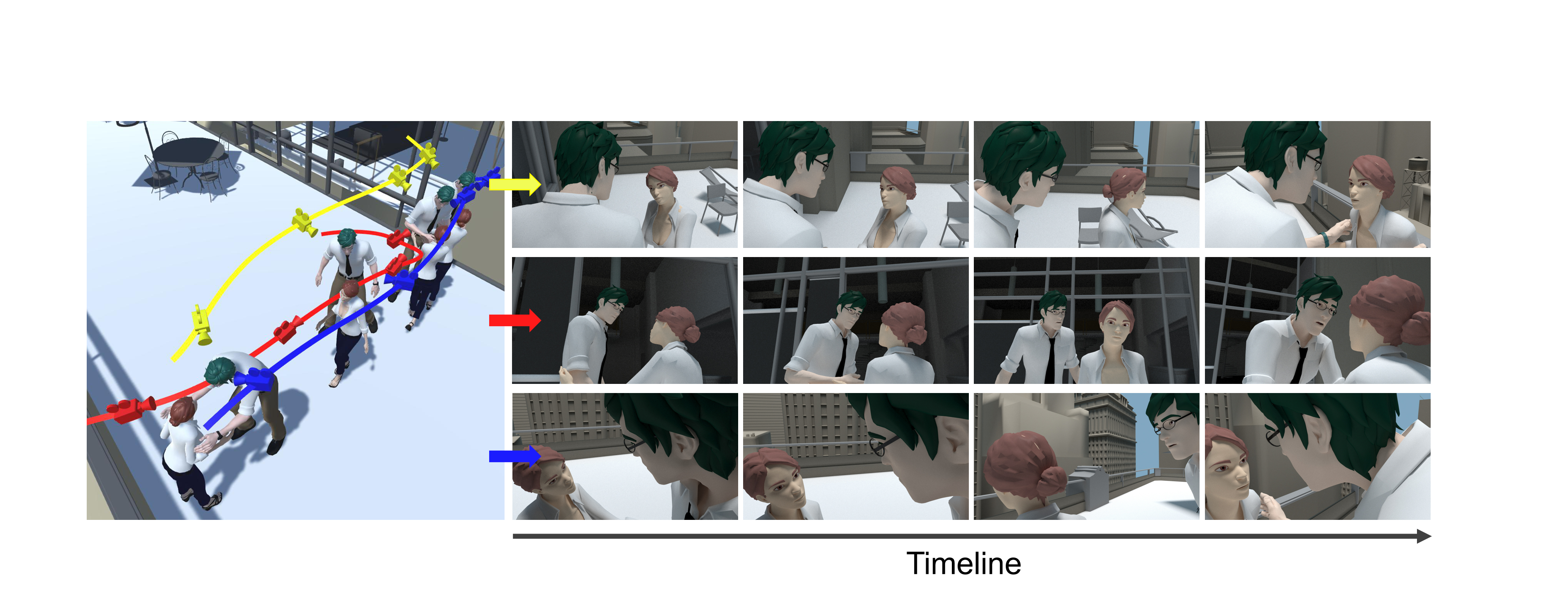
We present a novel technique that enables 3D artists to synthesize camera motions in virtual environments following a camera style, while enforcing user-designed camera keyframes as constraints along the sequence (see figure 4). To solve this constrained motion in-betweening problem, we design and train a camera motion generator from a collection of temporal cinematic features (camera and actor motions) using a conditioning on target keyframes. We further condition the generator with a style code to control how to perform the interpolation between the keyframes. Style codes are generated by training a second network that encodes different camera behaviors in a compact latent space, the camera style space. Camera behaviors are defined as temporal correlations between actor features and camera motions and can be extracted from real or synthetic film clips. We further extend the system by incorporating a fine control of camera speed and direction via a hidden state mapping technique. We evaluate our method on two aspects: i) the capacity to synthesize style-aware camera trajectories with user defined keyframes; and ii) the capacity to ensure that in-between motions still comply with the reference camera style while satisfying the keyframe constraints. As a result, our system is the first style-aware keyframe in-betweening technique for camera control that balances style-driven automation with precise and interactive control of keyframes.
7.2.6 Real-Time Cinematic Tracking of Targets in Dynamic Environments
Participants: Marc Christie [contact], Ludovic Burg.
Building on our previous work on efficient visibility estimation, we proposed a novel camera tracking algorithm for virtual environments. Tracking targets in unknown 3D environments requires to simultaneously ensure a low computational cost, a good degree of reactivity and a high cinematic quality despite sudden changes. In this paper, we draw on the idea of Motion-Predictive Control to propose an efficient real-time camera tracking technique which ensures these properties. Our approach relies on the predicted motion of a target to create and evaluate a very large number of camera motions using hardware ray casting. Our evaluation of camera motions includes a range of cinematic properties such as distance to target, visibility, collision, smoothness and jitter. Experiments are conducted to display the benefits of the approach with relation to prior work. Work is reported in 34.7.2.7 FaceTuneGAN: Face Autoencoder for Convolutional Expression Transfer Using Neural Generative Adversarial Networks
Participants: Franck Multon [contact], Nicolas Olivier.
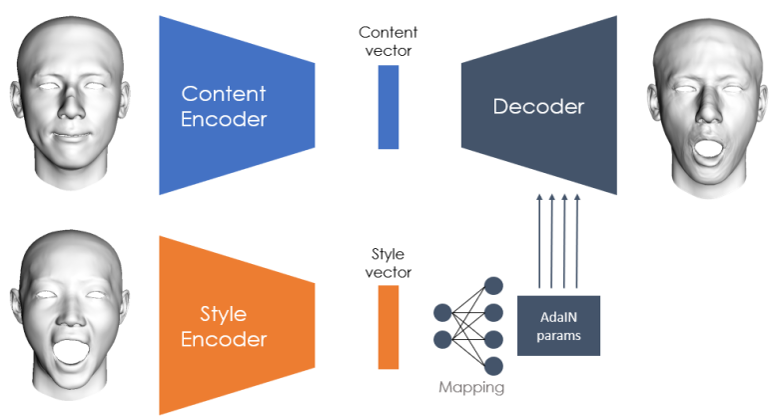
Our network architecture. The encoders extract and compress the features of their input into low-dimensional content and style vectors. A decoder reconstructs a mesh from these compact representations. The style information is passed to the decoder through AdaIN normalization layers.
In this work 52, we present FaceTuneGAN, a new 3D face model representation decomposing and encoding separately facial identity and facial expression. We propose a first adaptation of image-to-image translation networks, that have successfully been used in the 2D domain, to 3D face geometry. Leveraging recently released large face scan databases, a neural network has been trained to decouple factors of variations with a better knowledge of the face, enabling facial expressions transfer and neutralization of expressive faces. Specifically, we design an adversarial architecture adapting the base architecture of FUNIT and using SpiralNet++ for our convolutional and sampling operations. Using two publicly available datasets (FaceScape and CoMA), FaceTuneGAN has a better identity decomposition and face neutralization than state-of-the-art techniques. The architecture is depicted in Figure 5. It also outperforms classical deformation transfer approach by predicting blendshapes closer to ground-truth data and with less of undesired artifacts due to too different facial morphologies between source and target.
7.2.8 Contact Preserving Shape Transfer For Rigging-Free Motion Retargeting
Participants: Franck Multon [contact], Jean Basset, Adnane Boukhayma.
In 2018, we introduced the idea of context graph to capture the relationship between body parts surfaces and enhance the quality of the motion retargetting problem. Hence, it becomes possible to retarget the motion of a source character to a target one while preserving the topological relationship between body parts surfaces. However this approach implies to strictly satisfy distance constraints between body parts, whereas some of them could be relaxed to preserve naturalness. In 2019, we introduced a new paradigm based on transfering the shape instead of encoding the pose constraints to tackle this problem for isolated poses.
In 2020, we extended this approach to handle continuity in motion, and non-human characters. this idea resulted from the collaboration with the Morpheo Inria team in Grenoble, in the context of the IPL AVATAR project. The proposed approaches were based on a nonlinear optimization framework, which involved manually editing the constraints to satisfy, and required a huge amount of computation time.
In 32, to achieve the deformation transfer, we proposed a neural encoder-decoder architecture where only identity information is encoded and where the decoder is conditioned on the pose. We use pose independent representations, such as isometry-invariant shape characteristics, to represent identity features. The model is depicted in Figure 6. Our model uses these features to supervise the prediction of offsets from the deformed pose to the result of the transfer. We show experimentally that our method outperforms state-of-the-art methods both quantitatively and qualitatively, and better generalizes better to poses not seen during training. We also introduce a fine-tuning step that allows to obtain competitive results for extreme identities, and allows to transfer simple clothing.
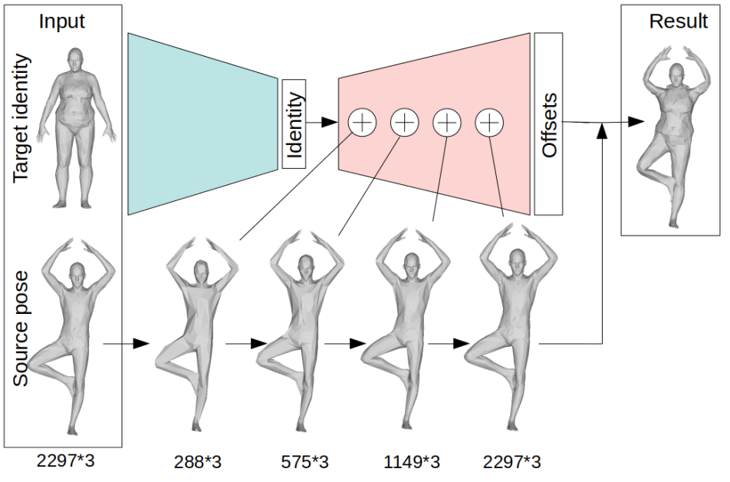
Overview of the proposed approach. The encoder (green) generates an identity code for the target. We feed this code to the decoder (red) along with the source, which is concatenated with the decoder features at all resolution stages. The decoder finally outputs per vertex offsets from the input source towards the identity transfer result.
7.2.9 Perception of Motion Variations in Large-Scale Virtual Human Crowds
Participants: Robin Adili [contact], Ludovic Hoyet [contact], Benjamin Niay, Anne-Hélène Olivier, Katja Zibrek.
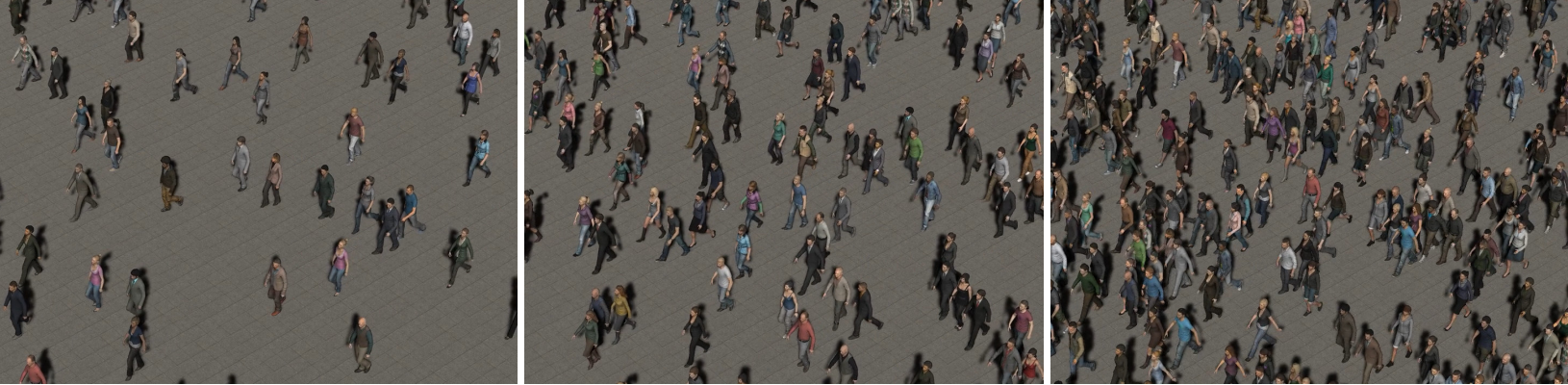
Virtual human crowds are regularly featured in movies and video games. With a large number of virtual characters, each behaving in their own way, spectacular scenes can be produced. The more diverse the characters and their behaviors are, the more realistic the virtual crowd is expected to be perceived. Hence, creating virtual crowds is a trade-off between the cost associated with acquiring more diverse assets, namely more virtual characters with their animations, and achieving better realism. In this work 31, conducted in collaboration with Julien Pettré from the Rainbow team, our focus is on the perceived variety in virtual crowd character motions. We present an experiment exploring whether observers are able to identify virtual crowds including motion clones in the case of large-scale crowds (from 250 to 1000 characters), see figure 7. As it is not possible to acquire individual motions for such numbers of characters, we rely on a state-of-the-art motion variation approach to synthesize unique variations of existing examples for each character in the crowd. Participants then compared pairs of videos, where each character was animated either with a unique motion or using a subset of these motions. Our results show that virtual crowds with more than two motions (one per gender) were perceptually equivalent, regardless of their size. We believe these findings can help create efficient crowd applications, and are an additional step into a broader understanding of the perception of motion variety.
7.2.10 Interaction Fields: Sketching Collective Behaviours.
Participants: Marc Christie [contact], Adèle Colas [contact], Ludovic Hoyet [contact], Anne-Hélène Olivier [contact], Katja Zibrek.
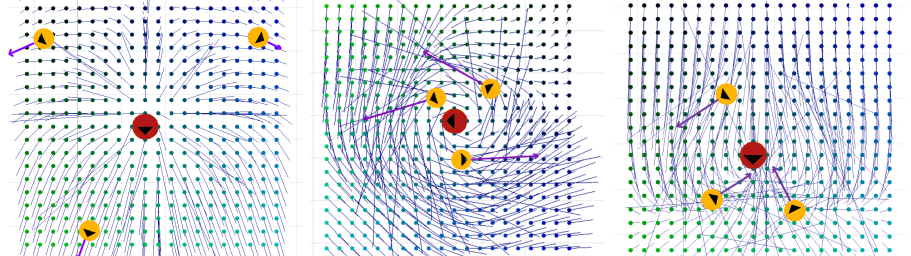
Many applications of computer graphics, such as cinema, video games, virtual reality, training scenarios, therapy, or rehabilitation, involve the design of situations where several virtual humans are engaged. In applications where a user is immersed in the virtual environment, the (collective) behavior of these virtual humans must be realistic to improve the user's sense of presence. While expressive behaviour appears to be a crucial aspect as part of the realism, collective behaviours simulated using typical crowd simulation models (e.g., social forces, potential fields, velocity selection, vision-based) usually lack expressiveness and do not allow to capture more subtle scenarios (e.g., a group of agents hiding from the user or blocking his/her way), which require the ability to simulate complex interactions. As subtle and adaptable collective behaviours are not easily modeled, there is therefore a need for more intuitive ways to design such complex scenarios. In 2020, we proposed a novel approach to sketch such interactions to define collective behaviours, which we called Interaction Fields, in collaboration with Julien Pettré and Claudio Pacchierotti in Rainbow team. Although other sketch-based approaches exist, these usually focus on goal-oriented path planning, and not on modelling social or collective behaviour. In comparison, our approach is based on a user-friendly application enabling users to draw target interactions between agents through intuitive vector fields (Figure 8). This year, we therefore extended this work to include novel features facilitating the design of expressive and collective behaviours, and conducted an experiment evaluating the usability of the approach. By considering more generic and dynamic situations, we demonstrate that we can design diversified and subtle interactions, which so far have mostly focused on predefined static scenarios.
7.2.11 Reactive Virtual Agents: A Viewpoint-Driven Approach for Bodily Nonverbal Communication
Participants: Marc Christie [contact], Ludovic Hoyet [contact], Alberto Jovane [contact], Anne-Hélène Olivier [contact], Pierre Raimbaud, Katja Zibrek.
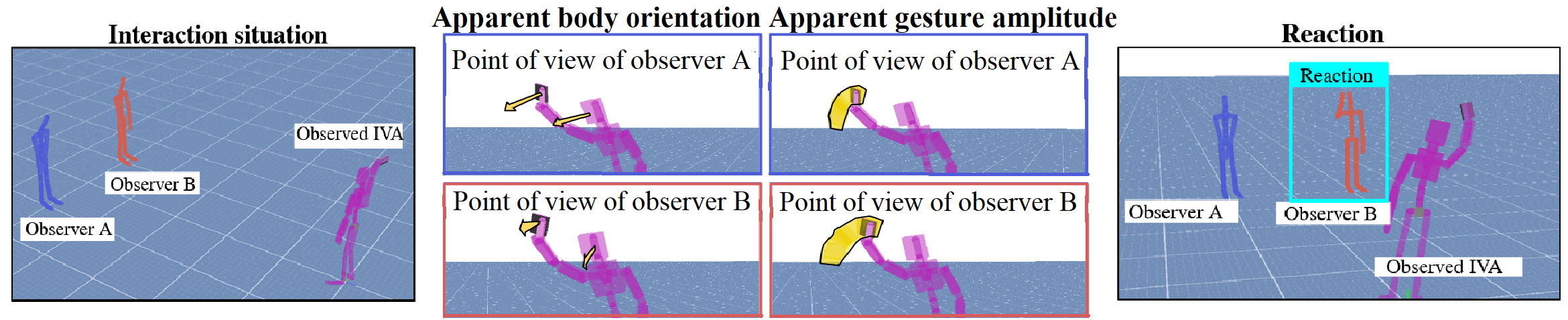
Intelligent Virtual Agents (IVA) should be designed to perform realistic and expressive interactions that convey emotions and express personalities. These are notably expressed by humans through nonverbal behaviours in everyday interactions. Thus, the ability to perform nonverbal communication is paramount for IVA design. Nonverbal communication, notably reflected by proxemics and kinesics, contributes to the social realism of IVA interactions. In this regard, user studies have been conducted to evaluate generative models of IVAs’ expressive facial motions or body motions. These last ones in particular – divided into postures and gestures – appear to be the key to expressivity in nonverbal communication between humans, and with IVAs. Nonetheless, ensuring the expressivity of IVAs' actions is not the only challenge related to bodily nonverbal communication. IVAs should also embed the ability to react to humans or other IVAs through adapted bodily communication.
Relying on the perception-action loop involved during human interactions, this work 39 conducted in collaboration with Julien Pettré and Claudio Pacchierotti from the Rainbow team, introduces a new concept to design IVAs with reactive body motions based on a viewpoint-driven approach. We present a new paradigm for reactive behaviours simulation, based on the visual analysis of body movements, see figure 9. In this short paper, we focus on situations where the poses of all agents are known. However, the same idea can easily be extended to more complex scenarios where only partial information about the agents' poses is available. Our viewport-driven approach first analyses the observed IVA's motions from the viewpoint of the observer IVA, and then adjusts the observer IVA's reactions.
7.3 Fidelity of Virtual Reality
MimeTIC wishes to promote the use of Virtual Reality to analyze and train human motor performance. It raises the fundamental question of the transfer of knowledge and skills acquired in VR to real life. In 2021, we maintain our efforts to enhance the experience of users when interacting physically with a virtual world. We developed an original setup to carry-out experiments aiming at evaluating various haptic feedback rendering techniques. In collaboration with Hybrid team, we put many efforts in better simulating avatars of the users, and analyzed embodiment in various VR conditions, leading to several co-supervised PhD students.
7.3.1 Biofidelity in VR
Participants: Simon Hilt [contact], Georges Dumont [contact], Charles Pontonnier [contact].
Virtual environments (VE) and haptic interfaces (HI) tend to be introduced as virtual prototyping tools to assess ergonomic features of workstations. These approaches are cost-effective and convenient since working directly on the Digital Mock-Up. It thus allows low-cost solutions for studying the ergonomics of workstations upstream of the design chain, thanks to the interaction of an operator and a digital mock-up in a VE. This is preferred solution to constructing a physical mock-up in a Real Environment (RE). However it can be usable only if the ergonomic conclusions made from the VE are similar to the ones you would make in the real world. The focus was put on the evaluation of the impact of visual and haptic renderings in terms of biomechanical fidelity for pick-and-place tasks. We developed an original setup 10, enabling to separate the effect of visual and haptic renderings in the scene. This allows us to investigate individual and combined effects of those modes of immersion and interaction on the biomechanical behavior of the subject during the task. We focused particularly on the mixed effects of the renderings on the biomechanical fidelity of the system to simulate pick-and-place tasks. Fourteen subjects performed time-constrained pick-and-place tasks in RE and VE with a real and a virtual, haptic driven object at three different speeds. Motion of the hand and muscles activation of the upper limb were recorded. A questionnaire assessed subjectively discomfort and immersion. The results revealed significant differences between measured indicators in RE and VE and with real and virtual object. Objective and subjective measures indicated higher muscle activity and higher length of the hand trajectories in VE and with HI. Another important element is that no cross effect between haptic and visual rendering was reported. Theses results confirmed that such systems should be used with caution for ergonomics evaluation, especially when investigating postural and muscle quantities as discomfort indicators. The last of this part lies in an experimental setup easily replicable to asses more systematically the biomechanical fidelity of virtual environments for ergonomics purposes 20. Haptic feedback is a way to interact with the digital Digital Mock-Up but the control of the haptic device may affect the feedback. We proposed to evaluate the biomechanical fidelity of a pick-and-place task interacting with a 6 degrees of freedom HI and a dedicated inertial and viscous friction compensation algorithm. The proposed work shows that when manipulating such low masses in the proposed setup (see Figure 11) the subjective feeling (obtained by a questionnaire) of the user using the HI does not correspond to the muscle activity. Such a result is fundamental to classify what can be transferred from virtual to real at a biomechanical level.
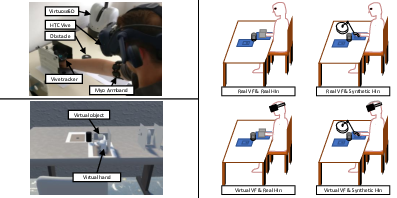
Top left: Experimental setup with two targets for pick-and-place tasks. A cylindrical obstacle 8 cm in diameter and 10 cm high was placed between the targets. The HI (Virtuose6D) was placed close by so that the movement was within its range of accessibility. The subject was equipped with a Vive tracker to record the position and orientation of his hand and with a myo bracelet to record the electromyographic activity of his arm. Finally, the subject was equipped with an HTC Vive Pro helmet during the virtual immersion where the virtual scene was projected. Bottom left: This virtual scene included the same elements as the real scene except for the HI with the addition of a virtual hand collocated thanks to the Vive Tracker. Right: Illustration of the four combinations of immersion/interaction modes.
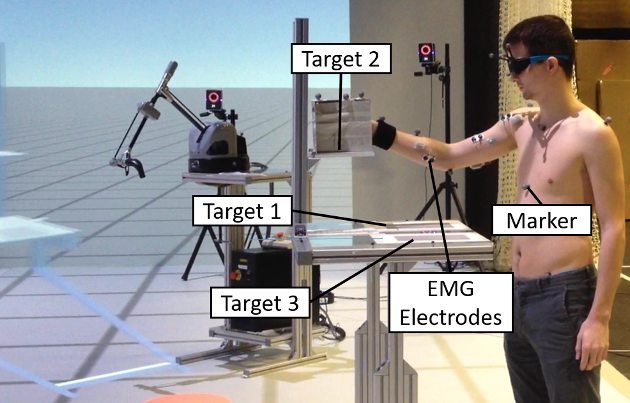
7.3.2 Towards "Avatar-Friendly" 3D Manipulation Techniques: Theoretical Background and Practical Design Guidelines
Participants: Diane Dewez [contact], Ludovic Hoyet [contact].
Avatars, the users' virtual representations, are becoming ubiquitous in virtual reality applications. In this context, the avatar indeed becomes the medium which enables users to manipulate objects in the virtual environment. It also becomes the users' main spatial reference, which can not only alter their interaction with the virtual environment, but also the perception of themselves. In this work 35, conducted in collaboration with Ferran Argelaguet and Anatole Lécuyer from the Hybrid team, we review and analyse the current state-of-the-art for 3D object manipulation and the sense of embodiment. Our analysis is twofold. First, we discuss the impact that the avatar can have on object manipulation. Second, we discuss how the different components of a manipulation technique (i.e. input, control and feedback) can influence the user’s sense of embodiment. Throughout the analysis, we crystallise our discussion with practical guidelines for VR application designers and we propose several research topics towards avatar-friendly manipulation techniques.
7.3.3 Does virtual threat harm VR experience?: Impact of threat occurrence and repeatability on virtual embodiment and threat response
Participants: Rebecca Fribourg [contact], Ludovic Hoyet [contact].

This work 18, conducted in collaboration with Ferran Argelaguet and Anatole Lécuyer from the Hybrid team, extends our previous work to explore the potential impact of threat occurrence and repeatability on users' Sense of Embodiment (SoE) and threat response 12. To that aim, we conducted an experiment in which participants were embodied in a virtual avatar, and performed a task in which a threat towards the virtual body was introduced a first time, then repeated several times through the experiment (in total 5 times). The SoE of participants as well as their subjective response to the threat were assessed through subjective questionnaires before the introduction of the threat, after a first introduction of the threat and at the end of the experiment. A control group did the same experiment with no threat introduced during the task. The main findings of our experiment are that the introduction of a threat does not alter users' SoE but might change their behaviour while performing a task after the threat occurrence. In addition, threat repetitions did not show any effect on users' subjective SoE, or subjective and objective responses to threat. Taken together, our results suggest that embodiment studies should expect potential change in participants behaviour while doing a task after a threat was introduced, but that threat introduction and repetition do not seem to impact the subjective measure of the SoE (subective ratings) nor the objective measure of the SoE (physical reaction to threat).
7.3.4 May I sit next to you? The effect of motion quality of virtual agents on the proximity in virtual reality
Participants: Vincent Etien [contact], Ludovic Hoyet [contact], Katja Zibrek [contact].
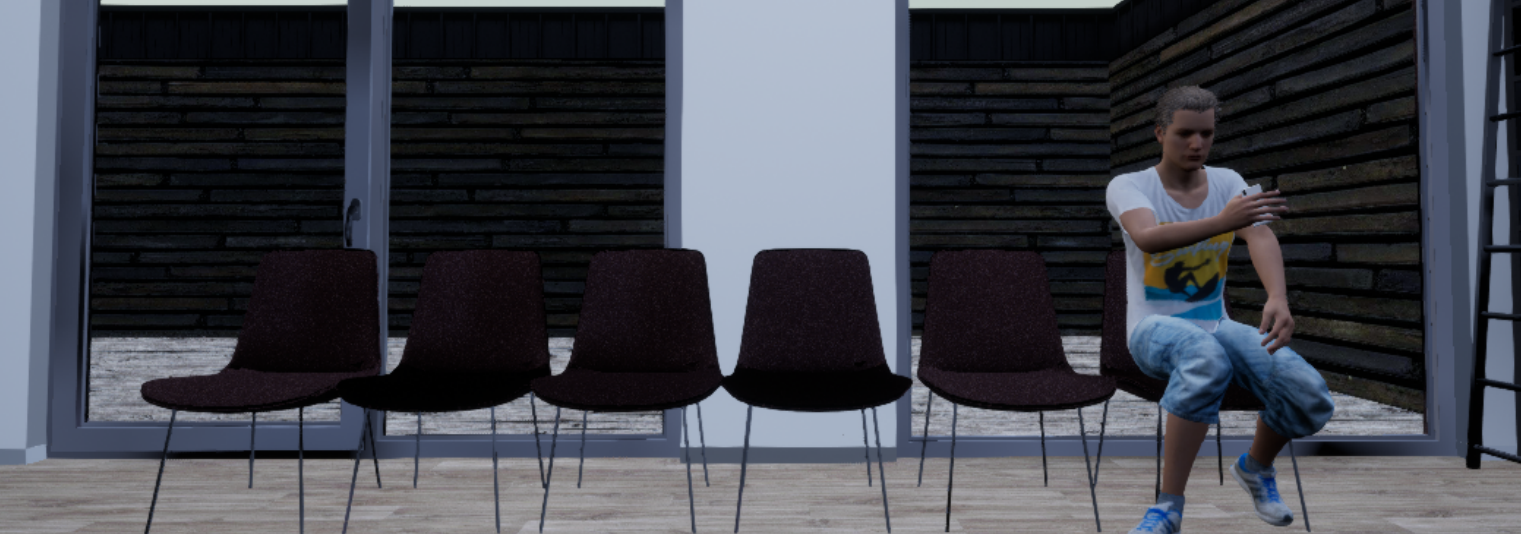
Proximity is a useful measure when investigating the perception of virtual agents in virtual reality. The measure can be used to assess the level of comfort people feel with virtual agents and is a sign of social presence with the agent. In this work, conducted in collaboration with Radoslaw Sterna from Jagiellonian University (Krakow, Poland), we investigated if the motion quality of agents impacts the proximity distance towards them. We created three levels of motion quality, by manipulating different body and facial animation techniques. We also introduced a new way of measuring proximity by asking the participants, embodied in a virtual waiting room, to sit on a chair as close as possible to the virtual agent (see Figure 13. We also asked them to recognise the emotional expression of the agent, rate their uncanniness traits (appeal, eeriness, familiarity), and assess their level of motion realism. Emotion recognition and motion realism detection showed that participants could recognise emotions and the type of motion quality as predicted. Our main result is that motion quality as well as eeriness are related to proximity.
7.3.5 Exploring behaviour towards avatars and agents in immersive virtual environments with mixed-agency interactions
Participants: Ludovic Hoyet [contact], Anne-Hélène Olivier [contact], Katja Zibrek.
Immersive virtual environments (IVEs) in which multiple users navigate by walking and interact with each other in natural ways are perfectly suited for team applications from training to recreation. At the same time, they can solve scheduling conflicts by employing virtual agents in place of missing team members or additional participants of a scenario. While this idea has been long discussed in IVEs research, there are no prior publications on social interactions in systems with multiple embodied users and agents. This work 38, conducted in collaboration with Julien Pettré from the Rainbow team, presents an experiment at a work-in-progress stage that addresses the impact of perceived agency and control of a virtual character in a collaborative scenario, with two embodied users and one virtual agent. Our future study will investigate whether users treat avatars and agents differently within a mixed-agency scenario, analysing several behavioural metrics and self-report of participants.
7.3.6 Understanding, Modeling and Simulating Unintended Positional Drift during Repetitive Steering Navigation Tasks in Virtual Reality
Participants: Anne-Hélène Olivier [contact], Hugo Brument.
This work was performed in collaboration with Ferran Argelaguet and Maud Marchal from Hybrid and Rainbow teams 12.
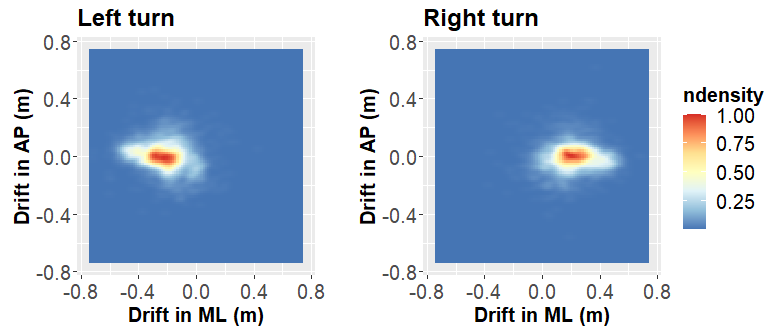
Virtual steering techniques enable users to navigate in larger Virtual Environments (VEs) than the physical workspace available. Even though these techniques do not require physical movement of the users (e.g. using a joystick and the head orientation to steer towards a virtual direction), recent work observed that users might unintentionally move in the physical workspace while navigating, resulting in Unintended Positional Drift (UPD). This phenomenon can be a safety issue since users may unintentionally reach the physical boundaries of the workspace while using a steering technique. In this context, as a necessary first step to improve the design of navigation techniques minimizing the UPD, we aim here analyzing and modeling the UPD during a virtual navigation task. In particular, we characterize and analyze the UPD for a dataset containing the positions and orientations of eighteen users performing a virtual slalom task using virtual steering techniques (cf Figure 14). Participants wore a head-mounted display and had to follow three different sinusoidal-like trajectories (with low, medium and high curvature) using a torso-steering navigation technique. We analyzed the performed motions and proposed two UPD models: the first based on a linear regression analysis and the second based on a Gaussian Mixture Model (GMM) analysis. Then, we assessed both models through a simulation-based evaluation where we reproduced the same navigation task using virtual agents. Our results indicate the feasibility of using simulation-based evaluations to study UPD. We conclude with a discussion of potential applications of the results in order to gain a better understanding of UPD during steering and therefore improve the design of navigation techniques by compensating for UPD.
7.3.7 Studying the Influence of Translational and Rotational Motion on the Perception of Rotation Gains in Virtual Environments
Participants: Anne-Hélène Olivier [contact], Hugo Brument.
This work was performed in collaboration with Ferran Argelaguet and Maud Marchal from Hybrid and Rainbow teams 33.
Rotation gains in Virtual Reality (VR) enable the exploration of wider Virtual Environments (VEs) compared to the workspace users have in VR setups. The perception of these gains has been consequently explored through multiple experimental conditions in order to improve redirected navigation techniques. While most of the studies consider rotations, in which participants can rotate to the place they desire but without translational motion, we have no information about the potential impact of the translational and rotational motions on the perception of rotation gains. In this paper, we estimated the influence of these motions and compared the perceptual thresholds of rotations gains through a user study (n = 14), in which participants had to perform virtual rotation tasks at a constant rotation speed. Participants had to determine whether their virtual rotation speed was faster or slower than their real one. We varied the translational optical flow (static or forward motion), the rotational speed (20, 30, or 40 deg/s), and the rotational gain (from 0.5 to 1.5). The main results are that the rotation gains are less perceivable at lower rotation speeds and that translational motion makes detection more difficult at lower rotation speeds. Furthermore, we provides insights into the user’s gaze and body motions behaviour when exposed to rotation gains. These results contribute to the understanding of the perception of rotation gains in VEs and they are discussed to improve the implementation of rotation gains in redirection techniques.
7.4 Motion Sensing of Human Activity
MimeTIC has a long experience in motion analysis in laboratory condition. In the MimeTIC project, we proposed to explore how these approaches could be transferred to ecological situations, with a lack of control on the experimental conditions. In 2021, we began to explore the use of deep learning techniques to capture human performance based on simple RGB or depth images. We also continued exploring how customizing complex musculoskeletal models with simple calibration processes.
7.4.1 Monocular Human Shape and Pose with Dense Mesh-borne Local Image Features
Participants: Adnane Boukhayma [contact], Franck Multon, Shubhendu Jena.
We propose to improve on graph convolution based approaches for human shape and pose estimation from monocular input, using pixel-aligned local image features. Given a single input color image, existing graph convolutional network (GCN) based techniques for human shape and pose estimation use a single convolutional neural network (CNN) generated global image feature appended to all mesh vertices equally to initialize the GCN stage, which transforms a template T-posed mesh into the target pose. In contrast, we propose for the first time the idea of using local image features per vertex. Figure 15 depicts the architecture of such a new solution. These features are sampled from the CNN image feature maps by utilizing pixel-to-mesh correspondences generated with DensePose. Our quantitative and qualitative results on standard benchmarks show that using local features improves on global ones and leads to competitive performances with respect to the state-of-the-art. This work was published at the IEEE International Conference on Automatic Face and Gesture Recognition (FG 2021) 50.
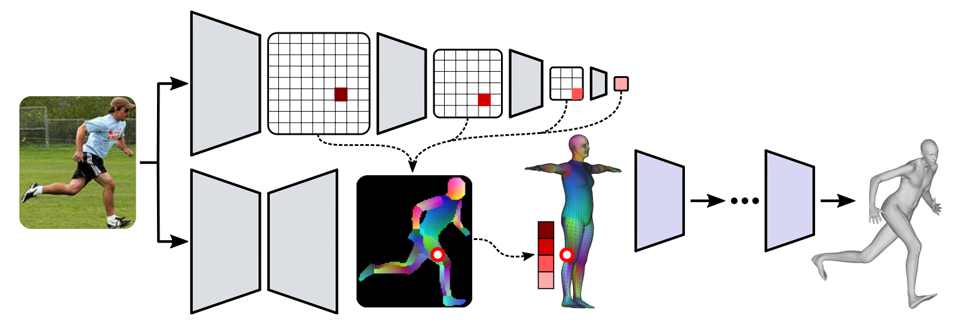
7.4.2 Dual Mesh Convolutional Networks for Human Shape Correspondence
Participants: Adnane Boukhayma [contact].
Convolutional neural networks have been extremely successful for 2D images and are readily extended to handle 3D voxel data. Meshes are a more common 3D shape representation that quantize the shape surface instead of the ambient space as with voxels, hence giving access to surface properties such as normals or appearances. The formulation of deep neural networks on meshes is, however, more complex since they are irregular data structures where the number of neighbors varies across vertices. While graph convolutional networks have previously been proposed over mesh vertex data, we explore how these networks can be extended to the dual face-based representation of triangular meshes, where nodes represent triangular faces in place of vertices. In comparison to the primal vertex mesh, its face dual offers several advantages, including, importantly, that the dual mesh is regular in the sense that each triangular face has exactly three neighbors. Moreover, the dual mesh suggests the use of a number of input features that are naturally defined over faces, such as surface normals and face areas. We evaluate the dual approach on the shape correspondence task on the FAUST human shape dataset and other versions of it with varying mesh topology. While applying generic graph convolutions to the dual mesh shows already improvements over primal mesh inputs, our experiments demonstrate that building additionally convolutional models that explicitly leverage the neighborhood size regularity of dual meshes enables learning shape representations that perform on par or better than previous approaches in terms of correspondence accuracy and mean geodesic error, while being more robust to topological changes in the meshes between training and testing shapes. This work was published at the IEEE International Conference on 3D Vision (3DV 2021) and accepted as an oral presentation 40. It was a collaboration with Nivika Verma and Edmond boyer from the Morpheo team in Inria Grenoble, and Jakob Verbeek from Facebook Research.
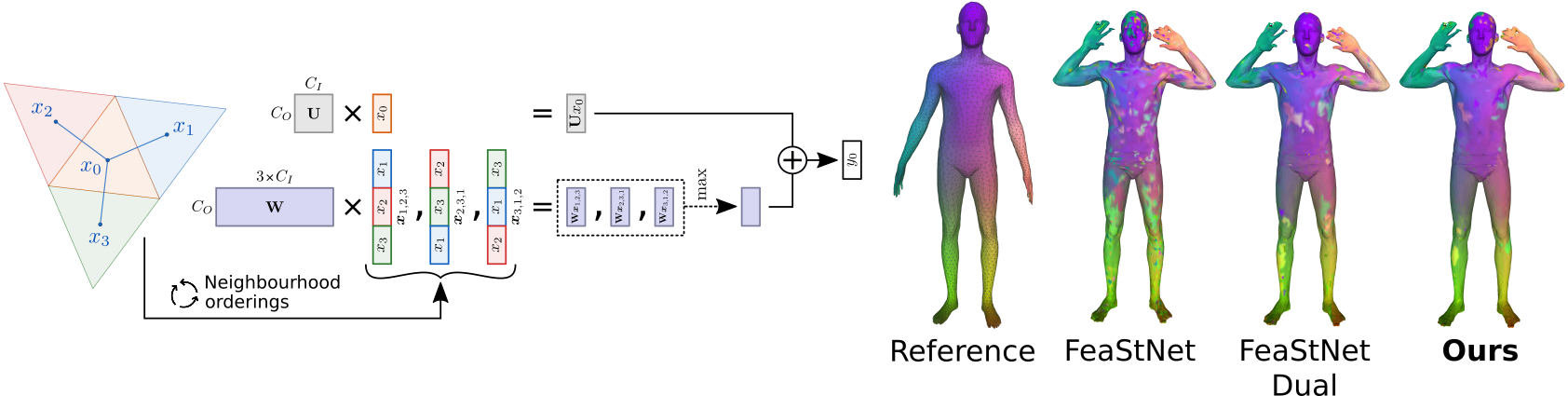
7.4.3 Muscle path modeling for simplified musculoskeletal analysis
Participants: Claire Livet [contact], Georges Dumont [contact], Charles Pontonnier [contact].
The current study 21 aims at proposing an automatic method to design and adjust simplified muscle paths of a musculoskeletal model. These muscle paths are composed of straight-lines described by a limited set of fixed active via points and an optimization routine is developed to place these via points on the model in order to fit moment arms and musculotendon lengths input data. The method has been applied to a forearm musculoskeletal model extracted from the literature, using theoretical input data as an example. Results showed that for 75% of the muscle set, the relative root mean square error between literature theoretical data and the results from optimized muscle path was under 29.23% for moment arms and of 1.09% for musculotendon lengths. These results confirm the ability of the method to automatically generate computationally efficient muscle paths for musculoskeletal simulations. Using only via points lowers computational expense compared to paths exhibiting wrapping objects. A proper balance between computational time and anatomical realism should be found to help those models being interpreted by practitioners.
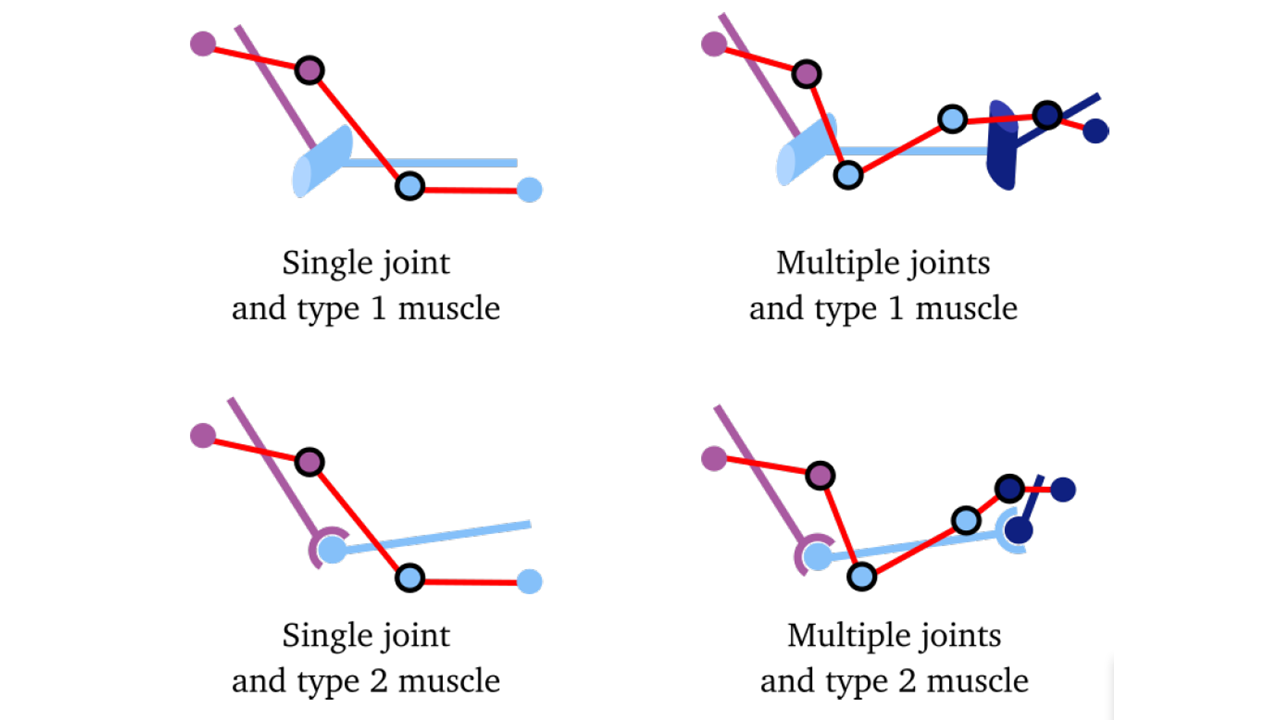
Muscle types taken into account in the muscle path modeling method developed in
7.4.4 Musculosekeletal analysis library developments
Participants: Claire Livet [contact], Georges Dumont [contact], Charles Pontonnier [contact].
Our team develops since a few years a musculoskeletal analysis toolbox (CusToM, that serves at a basis for many different PhD works now. We continue to enhance the capabilities of this toolbox by integrating PhD works. This year, we worked specifically on the development of closed loop models such as the kinematics of the shoulder complex 22 and the musculoskeletal modeling of the forearm 46. We also presented CusToM at the national conference Sciences 2024 to promote its usage in sport analysis 42.
7.4.5 pressure insoles assessment for external forces prediction
Participants: Pauline Morin [contact], Georges Dumont [contact], Charles Pontonnier [contact].
In motion analysis studies, classical inverse dynamics methods require knowledge of the ground reaction forces and moments (GRF&M) to compute internal forces. Force platforms are considered as the gold standard to measure GRF&M applied to the feet but they reduce the ecological aspect of the experimental conditions by limiting the analysis area. Estimating external forces from motion data and dynamic equations circumvents this limitation at the expense of accuracy. In such an estimation method, the inverse dynamics problem is undetermined since contact is modelled by multiple points representing the potential ground-foot contact area. We have investigated the potential of pressure insoles to detect contact in an external force estimation method. We have tested two contact detection methods: one is based on kinematic thresholds and the other is based on pressure insole data. Pressure insoles have shown real potential for detecting contact and they represent a new data source about force distribution. Such an approach may be of interest to enlarge the in-situ motion analysis 24. We proposed a pilot study questioning the implication of the internal forces (considering joint torques) in external forces prediction during a fencing lunge. This motion showed two easily identifiable phases (static and dynamic), and we may assume that joint torques were minimized during the static phase to let the fencer being relaxed before the assault. Any other motion may present specificities to be considered to find the best combination between internal and external forces to be minimized in the prediction. In conclusion, minimizing joint torques and external forces in a motion-based external forces prediction method seems relevant for static phases of motions 37.7.5 Sports
MimeTIC promotes the idea of coupling motion analysis and synthesis in various domains, especially sports. More specifically, we have a long experience and international leadership in using Virtual Reality for analyzing and training sports performance. In 2021, we continued to explore how enhancing the use of VR to design original training system. More specifically we addressed the problem of early motion recognition to make a virtual opponent react to the user's action before it ends. We also performed biomechanical analysis to better understand the physical interaction between a subject and its environment.
7.5.1 Early Motion Recognition
Participants: Richard Kulpa [contact], William Mocaer.
In order to produce sports training tools in virtual reality integrating strong interactions between the real and virtual athlete, it is necessary to have the earliest possible recognition of the gesture of the immersed athlete. This allows to react as soon as possible, well before the end of the action. To begin with, we propose OLT-C3D (Online Long-Term Convolutional 3D), a new architecture based on a 3D Convolutional Neural Network (3D CNN) inspired by recent spatio-temporal Convolutional Neural Networks in computer vision field, to address the complex task of early recognition of 2D handwritten gestures in real time (47). . The input signal of the gesture is converted to an image sequence along time with the trajectory history. The image sequence is passed to our 3D CNN OLT-C3D which gives a prediction at each new frame. OLT-C3D is coupled with an integrated temporal reject system to postpone the decision in time if more information is needed. Moreover, our system is end-to-end trainable, OLT-C3D and the temporal reject system are jointly trained to optimize the earliness of the decision. Our approach achieves superior performances on two complementary and freely available datasets: ILGDB and MTGSetB.
7.5.2 Diving board characterization for diving analysis
Participants: Georges Dumont [contact], Charles Pontonnier, Guillaume Nicolas, Nicolas Bideau, Louise Demestre, Pauline Morin.
In this study we developed several approaches aimed at understanding the interaction between a diver and its diving board during olympic diving. Such developments may be really useful to enhance the athlete's performance by pointing specifics actions (impulse, arm motion, leg/arm synchronization) to both the trainer and to the athlete. We created a finite element based diving board model and used experimental data to characterize it. The external force applied to the diving board was computed thanks to an external forces prediction method minimizing the dynamics residuals on the diver during the jump. Results were published in several conferences 15, 44, 45 and are currently submitted in a journal.7.6 Ergonomics
Ergonomics has become an important application domain in MimeTIC: being able to capture, analyze, and model human performance at work. In this domain, key challenge consists in using limited equipment to capture the physical activity of workers in real conditions. Hence, in 2021, we have proposed to explore machine learning approaches to predict discomfort that would have been reported when performing picking and placing tasks. We also developed a new approach to assess the impact of using exoskeletons on biomechanical variables.
7.6.1 Exoskeleton biomechanical impact assessment
Participants: Charles Pontonnier [contact], Divyaksh Subhash Chander, Simon Kirchhofer.
The interaction of an exoskeleton with the worker raises many issues such as joint misalignment, force transfer, control design… To properly detect such issues, is a keystone to assist the user efficiently. We developed an experimental methodology to detect any action of a wrist exoskeleton prototype, by investigating the synchronicity of action of both the user and the device during controlled tasks. Additionally, we explored the impact of the exoskeleton on range of motions and muscle activity during the execution of those tasks. The example was made on a radio ulnar deviation task and a wrist exoskeleton, showing particularly that the exoskeleton did well in detection but was not able to provide a relevant assistance due to his design 26.7.6.2 Posture Assessment and Subjective Scale Agreement in Picking Tasks with Low Masses
Participants: Franck Multon [contact], Charles Pontonnier, Georges Dumont, Olfa Haj Mahmoud.
This work aims at analyzing the relationship between postural assessment and perceived discomfort for picking tasks with low mass (up to 3kg), involving a wide range of positions/postures. In 2020, we analyzed the agreement of the different postural scores (mean value, integral value, root mean square value, weighted average time at each RULA level and the % of time per RULA level) with the subjective assessments. The statistical analysis showed no correlation between subjective and postural scores.
Based on this result, in 2021, we extended this approach by considering more variables as input (not limited to posture assessment) and replacing linear statistical relationship by non linear machine learning methods 19.
Hence, a neural networks approach has been proposed to handle various inputs such as postural, anthropometric and environmental variables in order to estimate self-reported discomfort in picking tasks. An input reduction method has been proposed, reducing the input variables to the minimum data required to estimate self-reported discomfort with similar accuracy as the neural network fed with all variables. To this end, eleven subjects carried-out picking tasks with various masses (0, 1, 3 kg) and imposed duration (5, 10 or 15 seconds). Continuous REBA score, anthropometric and environmental data were computed, and subjects’ discomfort were collected. The data set of this work consisted in the computed continuous REBA score, anthropometric, environmental data and collected subjects’ discomfort. The results showed that the correlation between the estimated and experimental tested data was equal to 0.775 when using all the 14 available variables. After data reduction, only 6 variables were left, with a very close performance when predicting discomfort. This method has the potential to support ergonomists in workstation designing processes, by adding discomfort prediction to virtual manikins’ behaviors in simulation tools.
7.7 Locomotion and Interactions between Walkers
MimeTIC is a leader in the study and modeling of walkers' visuomotor strategies. This implies to understand how humans generate their walking trajectories within an environment. In 2021, we proposed a new simulation approach to compute plausible cyclic and symmetrical gaits based on anatomical description only. Regarding interactions between pedestrians, we started exploring the question of physical contacts in dense crowds. In the frame of the BEAR Associate team, we continued our research on visuomotor coordination in specific populations including older adults and previously concussed athletes. We also used VR as a mean to better understand the factors affecting interactions between walkers but also between a walker and autonomous vehicles.
7.7.1 Modeling physical interactions in human dense crowds: study of individual response to controlled external pushes
Participants: Charles Pontonnier [contact], Ludovic Hoyet, Anne-Hélène Olivier, Thomas Chatagnon.
This work is performed in collaboration with Julien Pettré from the Rainbow team, in the frame of the European project CrowdDNA and the PhD of Thomas Chatagnon.
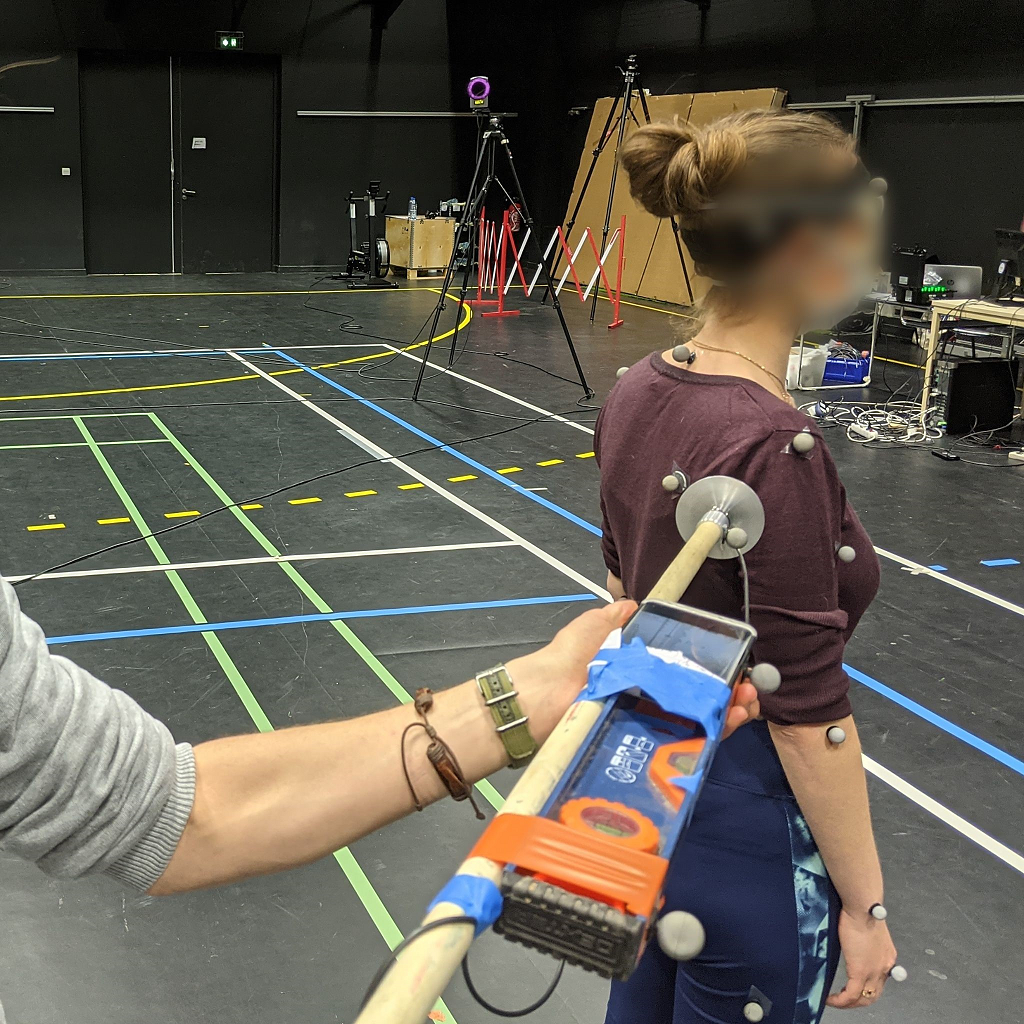
Dense crowds are complex environments in which obtaining meaningful metrics to understand and predict general behavior can be really challenging. Modelling such phenomena requires to consider local interactions such as local contacts and resulting motion. Dense crowds are generally found in cultural, social or religious events (concerts, pilgrimages, giant sales...) in which many stimuli (pushes) may come from many directions on individuals, resulting in large motions of the crowd that can have tragic outcomes. At the same time, individuals may be focused on many other cognitive distractions (e.g. music, video) that may affect their response to these stimuli.
Following works on push recovery and on reaction to external perturbations, we designed this year a pilot study (Figure 18) aiming at relating the response of a subject to external perturbations for several situations, including potential distractions (by controlling the availability of some of their sensory inputs) 13.
7.7.2 Visuomotor coordinations and person-specific factors during a collision avoidance task
Participants: Anne-Hélène Olivier [contact], Armel Crétual.
This research is performed in close collaboration with Wilfrid Laurier University (Canada) in the frame of Inria Bear Associate team. We have conducted experiments to understand the effect of person-specific factors on visuomotor coordination during a collision avoidance locomotor task. Collision avoidance between two walkers requires a mutual adaptation based on visual information in order to be successful.
We first focused on age-related changes 28. Indeed these changes to visuomotor processing, kinesthetic input, and intersegmental dynamics increases the risk of collision and falls in older adults. However, few studies examine behavioural strategies in older adults during collision avoidance tasks with another pedestrian. Is there a difference between older adults’ and young adults’ collision avoidance behaviours with another pedestrian? Seventeen older adults and seventeen young adults walked at a comfortable walking speed along a 12.6 m pathway while avoiding another walker. Trials were randomized equally to include 20 interactions with the same age group and 21 interactions with the opposite age group. Minimum predicted distance (mpd) was used to characterize collision avoidance behaviours between older adults and young adults. Our results showed that older adults had riskier avoidance behaviours, passing closer to the other pedestrian compared to when two young adults were on a collision course. Whenever an older adult was on a collision course with a young adult, the young adult contributed more to the avoidance regardless of passing order. The results from the current study highlight age-related effects during a collision avoidance task in older adults resulting in risky behaviour and a potential collision. Future studies should further investigate age-related visuomotor deficits during collision avoidance tasks in cluttered environments using virtual reality in order to tease out factors that contribute most to avoidance behaviours in older adults.
Second, we considered the effect of a concussion on these visuomotor coordinations 29. Individuals who have sustained a concussion often display associated balance control deficits and visuomotor impairments despite being cleared by a physician to return to sport. Such visuomotor impairments can be highlighted in collision avoidance tasks that involves a mutual adaptation between two walkers. However, studies have yet to challenge athletes with a previous concussion during an everyday collision avoidance task, following return to sport. We then wonder whether athletes with a previous concussion display associated behavioural changes during a 90 degree collision avoidance task with an approaching pedestrian. To this end, we conducted an experiment involving thirteen athletes (ATH; 9 females) and 13 athletes with a previous concussion (CONC; 9 females, concussion <6 months). They were asked to walke at a comfortable walking speed along a 12.6 m pathway while avoiding another athlete on a 90degree collision course. Each participant randomly interacted with individuals from the same group 20 times and interacted with individuals from the opposite group 21 times. Minimum predicted distance (mpd) was used to examine collision avoidance behaviours between ATH and CONC groups. Our results showed that the overall progression of mpd(t) did not differ between groups. During the collision avoidance task, previously concussed athletes contributed less when passing second compared to their peers. When two previously concussed athletes were on a collision course, there was a greater amount of variability resulting in inappropriate adaptive behaviours. Although successful at avoiding a collision with an approaching athlete, previously concussed athletes exhibit behavioural changes manifesting in riskier behaviours. The current findings suggest that previously concussed athletes possess behavioural changes even after being cleared to returned to sport, which may increase their risk of a subsequent injury when playing.
7.7.3 Interactions between walkers in VR
Participants: Anne-Hélène Olivier [contact], Armel Crétual, Ludovic Hoyet, Benjamin Niay.
A first axis of this work concerns the effect of walkers’ path on avoidance behavior 23. Navigating crowded community spaces requires interactions with pedestrians that follow rectilinear and curvilinear trajectories. In the case of rectilinear trajectories, it has been shown that the perceived action opportunities of the walkers might be afforded based on a future distance of closest approach. However, little is known about collision avoidance behaviours when avoiding walkers that follow curvilinear trajectories. Twenty-two participants were immersed in a virtual environment and avoided a virtual human (VH) that followed either a rectilinear path or a curvilinear path with a 5 m or 10 m radius curve at various distances of closest approach. Compared to a rectilinear path (control condition), the curvilinear path with a 5 m radius yielded more collisions when the VH approached from behind the participant and more inversions when the VH approached from in-front. During each trial, the evolution of the future distance of closest approach showed similarities between rectilinear paths and curvilinear paths with a 10 m radius curve. Overall, with few collisions and few inversions of crossing order, we can conclude that participants were capable of predicting future distance of closest approach of virtual walkers that followed curvilinear trajectories. The task was solved with similar avoidance adaptations to those observed for rectilinear interactions. These findings should inform future endeavors to further understand collision avoidance strategies and the role of—for example—non-constant velocities.
In the continuity of this work, a second axis has focused on the influence of gait patterns 11. Indeed, gait patterns provide a rich source of person-specific information such as age, sex, identity, and vulnerability. However, it is unknown to what extent person-specific gait information can affect collision avoidance behaviours with an approaching “person”. We sought to determine whether young adults’ spatiotemporal avoidance behaviours were affected by changes to a virtual agent’s gait parameters (i.e., speed or trunk sway). In a virtual environment (FOVE head-mounted display; 70Hz), young adults (n=21) walked along an 18m pathway towards a goal while avoiding an approaching virtual agent. The agent’s walking speed and trunk sway magnitude were a multiples of each participant’s average speed or sway: fast (x1.5m/s), normal (matched), or slow (x0.8m/s); large (2x), normal (matched), or small (0x) respectively. The agent was non-reactive and walked straight forward at a constant speed. Participants’ kinematics were recorded (Qualisys; 120Hz) to examine avoidance behaviours of initiation of path deviation and medial-lateral clearance at the time of crossing. Statistical analysis revealed that participants initiated a path deviation (i.e., estimate of time-to-contact, TTC) significantly earlier when the agent was walking fast (M=3.93s, SD=.56) as opposed to normal (M=4.31s, SD=.33) and slow (M=4.41s, SD=.44) walking speeds. However, the agent’s trunk sway magnitudes did not affect participants’ initiation of path deviation or medial-lateral clearance at crossing. Participants appear not to use temporal information to initiate an avoidance, but rather a point in space (i.e., TTC was affected by approach speed) due to awareness that the agent was non-reactive and always approaching. The agent’s sway magnitude did not affect medial-lateral avoidance behaviours most likely because there was little observable difference between. Conceivably, the study’s environmental conditions may underrepresent people’s behaviours in real-world. Future work is needed to understand the perception of an approaching person’s gait characteristics on collision avoidance.

Illustration of the experiment in the simulator room to study the interaction between a pedestrian and self-driving and conventional cars.
Finally, in a third axis, we consider the interaction between pedestrians and autonomous vehicles 16. Self-driving vehicles are gradually becoming a reality. But the consequences of introducing such automated vehicles (AVs) into current road traffic cannot be clearly foreseen yet, especially for pedestrian safety. The present study used virtual reality to examine the pedestrians' crossing behavior in front of AVs as compared to conventional cars (CVs) (Figure 19). Thirty young (ages 21-39) and 30 older (ages 68-81) adults participated in a simulated street-crossing experiment allowing for a real walk across an experimental two-way street. Participants had to cross (or not cross) in mixed traffic conditions where highly perceptible AVs always stopped to let them cross, while CVs did not brake to give them the right of way. Available time gap (from 1 to 5 s), approach speed (30 or 50 km/h), and the lane in which the cars were approaching (near and/or far lane of the two-way street) were varied. The results revealed a significantly higher propensity to cross the street, at shorter gaps, when AVs gave way to participants in the near lane while CVs were approaching in the far lane, leading to more collisions in this condition than in the others. These risky decisions were observed for both young and older participants, but much more so for the older ones. The results also indicated hesitation to cross in front of an AV in both lanes of the two-way street, with later initiations and longer crossing times, especially for the young participants and when the AVs were approaching at a short distance and braked suddenly. This study highlights the potential risks for pedestrians of introducing AVs into current road traffic, complicating the street-crossing task for young and older people alike. Future studies should look further into the role of repeated practice and trust in AVs.
8 Bilateral contracts and grants with industry
8.1 Bilateral contracts with industry
Cifre Faurecia - Monitoring of gestual efficiency at work
Participant: Franck Multon [contact], Georges Dumont, Charles Pontonnier, Olfa Haj Mahmoud.
This Cifre contract has started in September 2018 for three years and is funding the PhD thesis of Olfa Haj Mamhoud. It consists in designing new methods based on depth cameras to monitor the activity of workers in production lines, computes the potential risk of musculoskeletal disorders, and efficiency compared to reference workers. It raises several fundamental questions, such as adapting previous methods to assess the risk of musculoskeletal disorders, as they generally rely on static poses whereas the worker is performing motion. Based on previous works in the team (previous Cifre PhD thesis of Pierre Plantard) we will provide 30Hz motion capture of the worker, that will enable us to evaluate various time-dependent assessment methods.
We will also explore how to estimate joint forces based and torques on such noisy and low-sampling motion data. We will then define a new assessment method based on these forces and torques.
The Cifre contracts funds the PhD salary and 10K€ per year for the supervision and management of the PhD thesis.
Cifre InterDigitial - Adaptive Avatar Customization for Immersive Experiences
Participant: Franck Multon [contact], Ludovic Hoyet, Nicolas Olivier.
This Cifre contract has started in February 2019 for three years and is funding the PhD thesis of Nicolas Olivier. The aim of the project is to design stylized avatars of users in immersive environment and digital arts such as videogames or cinema.
To this end, we will design a pipeline from motion and shape capture of the user to the simulation of the 3D real-time and stylized avatar. It will take hairs, eyes, face, body shape and motion into account. The key idea is to stylized both appaearance and motion to make avatar better correspond to the style of the movie of immersive experience. We will carry-out perceptual studies to better understand the expectation of the users when controlling stylized avatars, to maximize embodiment. The Cifre contracts funds the PhD salary and 15K€ per year for the supervision and management of the PhD thesis. This contract is also in collaboration with Hybrid team.
Cifre InterDigitial - Learning-Based Human Character Animation Synthesis for Content Production
Participant: Ludovic Hoyet [contact], Lucas Mourot.
The overall objective of the PhD thesis of Lucas Mourot, which started in June 2020, is to adapt and improve the state-of-art on video animation and human motion modelling to develop a semi-automated framework for human animation synthesis that brings real benefits to artists in the movie and advertising industry. In particular, one objective is to leverage the use of novel learning-based approaches, in order to propose skeleton-based animation representations, as well as editing tools, in order to improve the resolution and accuracy of the produced animations, so that automatically synthetized animations might become usable in an interactive way by animation artists.
8.2 Bilateral grants with industry
Collaboration with company SolidAnim (Bordeaux, France)
Participant: Marc Christie [contact], Xi Wang.
This contract started in November 2019 for three years. Its purpose is to explore novel means of performing depth detection for augmented reality applied to the film and broadcast industries. The grant serves to fund the PhD of Xi Wang. (160kE)
Collaboration Unreal company (Unreal Megagrant)
Participant: Marc Christie [contact].
This contract started in September 2020 for two years. The objective is to explore means of designing novel VR manipulators for character animation tasks in Unreal Engine. (70kE)
9 Partnerships and cooperations
9.1 International initiatives
9.1.1 Inria associate team not involved in an IIL or an international program
BEAR
Participant: Anne-Hélène Olivier [contact].
-
Title:
from BEhavioral Analysis to modeling and simulation of interactions between walkeRs
-
Duration:
2019 ->
-
Coordinator:
Michael Cinelli (mcinelli@wlu.ca)
-
Partners:
- Wilfrid Laurier University
-
Inria contact:
Anne-Hélène Olivier
-
Summary:
BEAR project (from BEhavioral Analysis to modeling and simulation of interactions between walkeRs) is a collaborative project between France (Inria Rennes) and Canada (Wilfrid Laurier University and Waterloo University), dedicated to the simulation of human behavior during interactions between pedestrians. In this context, the project aims at providing more realistic models and simulation by considering the strong coupling between pedestrians’ visual perception and their locomotor adaptations.
9.2 European initiatives
9.2.1 FP7 & H2020 projects
INVICTUS
Participant: Marc Christie [contact], Adnane Boukhayma.
-
Title:
Innovative Volumetric Capture and Editing Tools for Ubiquitous Storytelling
-
Duration:
2020 - 2022
-
Coordinator:
University of Rennes 1
-
Partners:
- HHI, FRAUNHOFER GESELLSCHAFT ZUR FOERDERUNG DER ANGEWANDTEN FORSCHUNG E.V. (Germany)
- UBISOFT MOTION PICTURES (France)
- INTERDIGITAL (France)
- UNIVERSITE DE RENNES I (France)
-
Inria contact:
Marc Christie
-
Summary:
The world of animation, the art of making inanimate objects appear to move, has come a long way over the hundred or so years since the first animated films were produced. In the digital age, avatars have become ubiquitous. These numerical representations of real human forms burst on the scene in modern video games and are now used in feature films as well as virtual reality and augmented reality entertainment. Given the huge market for avatar-based digital entertainment, the EU-funded INVICTUS project is developing digital design tools based on volumetric capture to help authors create and edit avatars and their associated story components (decors and layouts) by reducing manual labour, speeding up development and spurring innovation.
CLIPE
Participant: Ludovic Hoyet, Katja Zibrek, Anne-Hélène Olivier.
-
Title:
Creating Lively Interactive Populated Environments
-
Duration:
2020 - 2024
-
Coordinator:
University of Cyprus
-
Partners:
- University of Cyprus (CY)
- Universitat Politecnica de Catalunya (ES)
- INRIA (FR)
- University College London (UK)
- Trinity College Dublin (IE)
- Max Planck Institute for Intelligent Systems (DE)
- KTH Royal Institute of Technology, Stockholm (SE)
- Ecole Polytechnique (FR)
- Silversky3d (CY)
-
Inria contact:
Julien Pettré, team Rainbow
-
Summary:
CLIPE is an Innovative Training Network (ITN) funded by the Marie Skłodowska-Curie program of the European Commission. The primary objective of CLIPE is to train a generation of innovators and researchers in the field of virtual characters simulation and animation. Advances in technology are pushing towards making VR/AR worlds a daily experience. Whilst virtual characters are an important component of these worlds, bringing them to life and giving them interaction and communication abilities requires highly specialized programming combined with artistic skills, and considerable investments: millions spent on countless coders and designers to develop video-games is a typical example. The research objective of CLIPE is to design the next-generation of VR-ready characters. CLIPE is addressing the most important current aspects of the problem, making the characters capable of: behaving more naturally; interacting with real users sharing a virtual experience with them; being more intuitively and extensively controllable for virtual worlds designers. To meet our objectives, the CLIPE consortium gathers some of the main European actors in the field of VR/AR, computer graphics, computer animation, psychology and perception. CLIPE also extends its partnership to key industrial actors of populated virtual worlds, giving students the ability to explore new application fields and start collaborations beyond academia. This work is performed in collaboration with Julien Pettré from Rainbow team. Website
CrowdDNA
Participant: Ludovic Hoyet, Charles Pontonnier, Anne-Hélène Olivier.
-
Title:
CrowdDNA
-
Duration:
2020 - 2024
-
Coordinator:
Inria
-
Partners:
- Inria (Fr)
- ONHYS (FR)
- University of Leeds (UK)
- Crowd Dynamics (UK)
- Universidad Rey Juan Carlos (ES)
- Forschungszentrum Jülich (DE)
- Universität Ulm (DE)
-
Inria contact:
Julien Pettré, team Rainbow
-
Summary:
This project aims to enable a new generation of “crowd technologies”, i.e., a system that can prevent deaths, minimize discomfort and maximize efficiency in the management of crowds. It performs an analysis of crowd behaviour to estimate the characteristics essential to understand its current state and predict its evolution. CrowdDNA is particularly concerned with the dangers and discomforts associated with very high-density crowds such as those that occur at cultural or sporting events or public transport systems. The main idea behind CrowdDNA is that analysis of new kind of macroscopic features of a crowd – such as the apparent motion field that can be efficiently measured in real mass events – can reveal valuable information about its internal structure, provide a precise estimate of a crowd state at the microscopic level, and more importantly, predict its potential to generate dangerous crowd movements. This way of understanding low-level states from high-level observations is similar to that humans can tell a lot about the physical properties of a given object just by looking at it, without touching. CrowdDNA challenges the existing paradigms which rely on simulation technologies to analyse and predict crowds, and also require complex estimations of many features such as density, counting or individual features to calibrate simulations. This vision raises one main scientific challenge, which can be summarized as the need for a deep understanding of the numerical relations between the local – microscopic – scale of crowd behaviours (e.g., contact and pushes at the limb scale) and the global – macroscopic – scale, i.e. the entire crowd. This work is performed in collaboration with Julien Pettré from Rainbow team.
PRESENT
Participant: Ludovic Hoyet, Anne-Hélène Olivier, Katja Zibrek.
-
Title:
Photoreal REaltime Sentient ENTity
-
Duration:
2019 - 2023
-
Coordinator:
Universitat Pompeu Fabra
-
Partners:
- Framestore (UK)
- Brainstorm (ES)
- Cubic Motion (UK)
- InfoCert (IT)
- Universitat Pompeu Fabra (ES)
- Universität Augsburg (DE)
- Inria (FR)
- CREW (BE)
-
Inria contact:
Julien Pettré, team Rainbow
-
Summary:
PRESENT is a three-year Research and Innovation project to create virtual digital companions –– embodied agents –– that look entirely naturalistic, demonstrate emotional sensitivity, can establish meaningful dialogue, add sense to the experience, and act as trustworthy guardians and guides in the interfaces for AR, VR and more traditional forms of media. There is no higher quality interaction than the human experience when we use all our senses together with language and cognition to understand our surroundings and –– above all -— to interact with other people. We interact with today’s ‘Intelligent Personal Assistants’ primarily by voice; communication is episodic, based on a request-response model. The user does not see the assistant, which does not take advantage of visual and emotional clues or evolve over time. However, advances in the real-time creation of photorealistic computer generated characters, coupled with emotion recognition and behaviour, and natural language technologies, allow us to envisage virtual agents that are realistic in both looks and behaviour; that can interact with users through vision, sound, touch and movement as they navigate rich and complex environments; converse in a natural manner; respond to moods and emotional states; and evolve in response to user behaviour. PRESENT will create and demonstrate a set of practical tools, a pipeline and APIs for creating realistic embodied agents and incorporating them in interfaces for a wide range of applications in entertainment, media and advertising. This work is performed in collaboration with Julien Pettré from Rainbow team. Website
SCHEDAR
Participant: Franck Multon [contact], Richard Kulpa, Xiaofang Wang.
-
Title:
Safeguarding the Cultural HEritage of Dance through Augmented Reality
-
Duration:
June 2018- June 2022
-
Coordinator:
Cyprus University
-
Partners:
- RISE, UNIVERSITY OF CYPRUS, (Cyprus)
- ALGOLYSIS LTD (Cyprus)
- UNIVERSITY OF WARWICK (UK)
- CRESTIC, UNIVERSITE DE REIMS CHAMPAGNE ARDENNES (France)
- M2S/MIMETIC, UNIVERSITE RENNES2 (France)
-
Inria contact:
Franck MULTON
-
Summary:
Dance is an integral part of any culture. Through its choreography and costumes dance imparts richness and uniqueness to that culture. Over the last decade, technological developments have been exploited to record, curate, remediate, provide access, preserve and protect tangible CH. However, intangible assets, such as dance, has largely been excluded from this previous work. Recent computing advances have enabled the accurate 3D digitization of human motion. Such systems provide a new means for capturing, preserving and subsequently re-creating ICH which goes far beyond traditional written or imaging approaches. However, 3D motion data is expensive to create and maintain, encompassed semantic information is difficult to extract and formulate, and current software tools to search and visualize this data are too complex for most end-users. SCHEDAR will provide novel solutions to the three key challenges of archiving, re-using and re-purposing, and ultimately disseminating ICH motion data. In addition, we will devise a comprehensive set of new guidelines, a framework and software tools for leveraging existing ICH motion databases. Data acquisition will be undertaken holistically; encompassing data related to the performance, the performer, the kind of the dance, the hidden/untold story, etc. Innovative use of state-of-the-art multisensory Augmented Reality technology will enable direct interaction with the dance, providing new experiences and training in traditional dance which is key to ensure this rich culture asset is preserved for future generations. MimeTIC is responsible for WP3 "Dance Data Enhancement".
-
9.3 National initiatives
- -
9.3.1 ANR
-
ANR PRC CAPACITIES
- -
Participants: Charles Pontonnier [contact] -, Georges Dumont, Diane Haering, Claire Livet.
- CAPACITIES is a 42-month ANR JCJC project (2020-2024) - This project is led by Christophe Sauret, from INI/CERAH. The project objective is to build a series of biomechanical indices characterizing the biomechanical difficulty for a wide range of urban environmental situations. These indices will rely on different biomechanical parameters such as proximity to joint limits, forces applied on the handrims, mechanical work, muscle and articular stresses, etc. The definition of a more comprehensive index, called Comprehensive BioMechanical (CBM) cost, including several of the previous indices, will also be a challenging objective. The results of this project would then be used in the first place in VALMOBILE application to assist MWC users in selecting optimal route in Valenciennes agglomeration (project founded by the French National Agency for Urban Renewal and the North Department of France). The MimeTIC team is involved on the musculoskeletal simulation issues and the biomechanical costs definition. The funding for the team is about 80kE.ANR JCJC Per2
Participants: Ludovic Hoyet [contact], Benjamin Niay, Anne-Hélène Olivier, Richard Kulpa, Franck Multon.
Per2 is a 42-month ANR JCJC project (2018-2022) entitled Perception-based Human Motion Personalisation (Budget: 280kE;Website)The objective of this project is to focus on how viewers perceive motion variations to automatically produce natural motion personalisation accounting for inter-individual variations. In short, our goal is to automate the creation of motion variations to represent given individuals according to their own characteristics, and to produce natural variations that are perceived and identified as such by users. Challenges addressed in this project consist in (i) understanding and quantifying what makes motions of individuals perceptually different, (ii) synthesising motion variations based on these identified relevant perceptual features, according to given individual characteristics, and (iii) leveraging even further the synthesis of motion variations and to explore their creation for interactive large-scale scenarios where both performance and realism are critical.
This work is performed in collaboration with Julien Pettré from Rainbow team.
9.3.2 Défi Avatar
Participants: Jean Basset [contact], Diane Dewez [contact], Rebecca Fribourg [contact], Ludovic Hoyet [contact], Franck Multon.
This project aims at design avatars (i.e., the user’s representation in virtual environments) that are better embodied, more interactive and more social, through improving all the pipeline related to avatars, from acquisition and simulation, to designing novel interaction paradigms and multi-sensory feedback. It involves 6 Inria teams (GraphDeco, Hybrid, Loki, MimeTIC, Morpheo, Potioc), Prof. Mel Slater (Uni. Barcelona), and 2 industrial partners (InterDigitak and Faurecia).
9.4 Regional initiatives
9.4.1 KIMEA Cloud Project
Participants: Franck Multon [contact], Adnane Boukhayma, Shubhendu Jena.
The Region Bretagne with “Image & Réseaux” national clusters have funded the project “Kimea Cloud” (Sept 2020-June 2022). This project leaded by Moovency (start-up company created in 2018 based on a PhD from MimeTIC) aims at proposing a new system to assess painful movement at work using ligth devices, such as a simple camera. Quortex (www.quortex.io/) company provides its expertise in delivering video content on Internet to make the assessment be remote and efficient for the future customers. MimeTIC provides a robust 3D posture tracking system based on the video stream.
9.4.2 EXOSCARNE Project
Participants: Charles Pontonnier [contact], Simon Kirchhofer.
This project (2020-2023), funded by the Brittany region and endorsed by both competitive pools Valorial and EMC2, aim at designing, prototyping and commercialing a wrist exoskeleton able to help industrial butchers in their cutting and deboning tasks. It is a partnership between a R&D company called Lab4i, the mimetic team and the industrial butchery cooperl. Our role in the consortium is the development of a virtual prototyping tool based on musculoskeletal modeling to simulate the action of the exoskeleton on the wrist, and to characterize the impact of the real prototype on the action of the user thanks to full scale experimentations involving motion, force and muscle activity sensing. The project funding is about 130kE for the team
9.4.3 ADT PyToM
Participants: Charles Pontonnier [contact], Laurent Guillo, Georges Dumont, Salomé Ribault.
This project (2021-2023), funded by inria, aims at developing a Python version of our musculoskeletal library called CusToM and currently developed in Matlab. The project is also developing additional motion data entries (vision, depth cameras) in the library to enhance the usability of the analysis tools.
10 Dissemination
10.1 Promoting scientific activities
10.1.1 Scientific events: organisation
General chair, scientific chair
- Ludovic Hoyet was Co-Conference Chair for the 2021 ACM Siggraph Conference on Motion, Interaction and Games (virtual)
- Anne-Hélène Olivier was Co-Conference Chair for the 2021 IEEE International Symposium on Mixed and Augmented Reality (ISMAR, virtual) and co-program Chair for the 2021 Symposium on Applied Perception (SAP, Virtual).
10.1.2 Scientific events: selection
Chair of conference program committees
- Ludovic Hoyet was Co-Program Chair for the 2021 Workshop on Affects, Compagnons Artificiels et Interactions (WACAI, France)
- Anne-Hélène Olivier and Katja Zibrek were co-organizers of the 6th Edition of the Virtual Humans and Crowds in Immersive Environments Workshop, held during IEEE VR 2021 Conference (virtual).
Member of the conference program committees
- Ludovic Hoyet: ACM Siggraph Asia 2021, IEEE VR 2021 Journal Paper Track, ACM SIGGRAPH Symposium on Interactive 3D Graphics and Games 2021, ACM Symposium on Applied Perception 2021, Modeling and Animating Realistic Crowds and Humans - AIVR Workshops 2021, Virtual Humans and Crowds in Immersive Environments - IEEE VR Workshops 2021
- Anne-Hélène Olivier: EuroXR 2021, Modeling and Animating Realistic Crowds and Humans - AIVR Workshops 2021
- Marc Christie, ACM Multimedia
- Franck Multon, ACM Motion Interaction in Games
Reviewer
- Ludovic Hoyet: Eurographics 2021
- Charles Pontonnier: IEEE VR 2021, ISMAR 2021, congrès de la société de biomécanique 2021, Ubiquitous Robots 2021
- Anne-Hélène Olivier: CrowdNav Workshop 2021, Humanoids 2021, ACM on Human-Computer Interaction ISS, IEEE VR2021 Journal and Conference tracks and doctoral consortium
- Marc Christie: ACM Siggraph 2021, ACM Siggraph Asia 2021, ACM Multimedia, EG STAR 2021, CASA 2021
- Franck Multon: IEEE VR2021, MIG2021,
10.1.3 Journal
Member of the editorial boards
- Franck Multon is associated editor of the journal Computer Animation and Virtual Worlds (Wiley)
- Franck Multon is associated editor of the Journal Presence (MIT Press)
Reviewer
- Ludovic Hoyet: ACM Transactions on Graphics, IEEE Transactions on Games
- Charles Pontonnier : mdpi international journal of environemental research and public health, mdpi sensors
- Anne-Hélène Olivier: Behaviour and Information Technology, Human Movement Science, Physica A, Computer & Graphics
- Marc Christie: TVCG
- Franck Multon: Physical and Engineering Sciences in Medicine, Virtual Reality journal, Applied Sciences, Journal of King Saud University - Computer and Information Sciences, Frontiers in Bioengineering,
10.1.4 Invited talks
- Marc Christie : Keynote talk : "Towards Computational Cinematography: what's left and what right?", ICCV Workshop on AI for Creative Video Editing and Understanding, CVEU (virtual)
- Charles Pontonnier : "La simulation musculo-squelettique pour le prototypage des exosquelettes", Colloque le soldat augmenté: Les nouvelles technologies au service de l’augmentation des performances du combattant, organisé par le CREC saint-cyr, Paris
- Anne-Hélène Olivier: "Interactions between pedestrians: from real to virtual studies", EuroXR 2021 (virtual)
- Anne-Hélène Olivier: "An interdisciplinary approach between Human Movement Sciences and Digital Sciences to understand collision avoidance behaviour between pedestrians", Rehab Week 2021 (virtual).
- Anne-Hélène Olivier: "Coordinations visuo-motrices durant la locomotion". Journées GDR IGRV (virtual).
- Franck Multon: "Les capteurs dans le sport problématiques et solutions", invité aux Journées capteurs du GDR CNRS Sport, Mars 2021 (virtual).
10.1.5 Scientific expertise
- Charles Pontonnier : expert pour l'ANR 2021, expert pour l'UNIGE
- Richard Kulpa : expert pour MITACS Canada
- Marc Christie : membre du Comité d'Evaluation 38 de l'ANR
10.1.6 Research administration
- Ludovic Hoyet is the coordinator of the Inria Research Challenge Avatar
- Franck Multon is responsible for the coordination of national Inria actions in Sports
- Franck Multon is the scientific representative of Inria in Sciences2024 group and scientific Committee
- Franck Multon is the scientific representative of Inria in the EUR Digisport steering committe and scientific committee
- Franck Multon is member of the Brittany commission of deontology
- Armel Crétual is the elected head of the Sports Sciences departement (STAPS) in University Rennes2
- Benoit Bideau is the head of the M2S Laboratory
- Benoit Bideau is vice-president of University Rennes2, in charge of the valorisation
- Benoit Bideau is the leader of the EUR DIGISPORT project
- Charles Pontonnier is the deputy director of the Mechatronics teaching and research department of ENS Rennes
- Charles Pontonnier is member of the EUR digisport pedagogical comitee
- Anne-Hélène Olivier is a co-director of the master program in adapted physical activity at the University of Rennes 2
- Richard Kulpa is the co-leader of the EUR DIGISPORT project
- Richard Kulpa is the scientific head of the EUR DIGISPORT project
- Marc Christie is the Project Coordinator of the H2020 ICT-55 project INVICTUS
10.2 Teaching - Supervision - Juries
- Master : Franck Multon, co-leader of the IEAP Master (1 and 2) "Ingénierie et Ergonomie de l'Activité Physique", STAPS, University Rennes2, France
- Master : Franck Multon, "Santé et Performance au Travail : étude de cas", leader of the module, 30H, Master 1 M2S, University Rennes2, France
- Master : Franck Multon, "Analyse Biomécanique de la Performance Motrice", leader of the module, 30H, Master 1 M2S, University Rennes2, France
- Master: Charles Pontonnier, leader of the first year of master "Ingénierie des systèmes complexes", mechatronics, Ecole normale supérieur de Rennes, France
- Master: Charles Pontonnier, Responsible of the internships of students (L3 and M1 "Ingénierie des systèmes complexes"), 15H, Ecole Normale Supérieure de Rennes, France
- Master: Charles Pontonnier, "Numerical simulation of polyarticulated systems", leader of the module, 22H, M1 Mechatronics, Ecole Normale Supérieure de Rennes, France
- Master: Charles Pontonnier, Research projects, 20H, Ecole Normale Supérieure de Rennes, France
- Master : Georges Dumont, Responsible of the second year of the master Engineering of complex systems, École Normale Supérieure de Rennes and Rennes 1 University, France
- Master : Georges Dumont, Mechanical simulation in Virtual reality, 36H, Master Engineering of complex systems and Mechatronics, Rennes 1 University and École Normale Supérieure de Rennes, France
- Master : Georges Dumont, Mechanics of deformable systems, 40H, Master, École Normale Supérieure de Rennes, France
- Master : Georges Dumont, oral preparation to agregation competitive exam, 20H, Master, École Normale Supérieure de Rennes, France
- Master : Georges Dumont, Vibrations in Mechanics, 10H, Master, École Normale Supérieure de Rennes, France
- Master : Georges Dumont, Finite Element method, 12H, Master, École Normale Supérieure de Rennes, France
- Master : Ludovic Hoyet, Computer Graphics, 12h, Ecole Normale Supérieure de Rennes, France
- Master : Ludovic Hoyet, Motion for Animation and Robotics, 6h, Université Rennes 1, France
- Master : Ludovic Hoyet, Motion Analysis and Gesture Recognition, 12h, INSA Rennes, France
- Master : Ludovic Hoyet, Réalité Virtuelle pour l'Analyse Ergonomique, Master Ingénierie et Ergonomie des Activités Physique, 21h, University Rennes 2, France
- Master : Anne-Hélène Olivier, co-leader of the APPCM Master (1 and 2) "Activités Physiques et Pathologies Chroniques et Motrices", STAPS, University Rennes2, France
- Master : Anne-Hélène Olivier, "Biostatstiques", 21H, Master 2 APPCM/IEAP, University Rennes2, France
- Master : Anne-Hélène Olivier, "Evaluation fonctionnelle des pathologies motrices", 3H Master 2 APPCM, University Rennes2, France
- Master : Anne-Hélène Olivier, "Maladie neurodégénératives : aspects biomécaniques", 2H Master 1 APPCM, University Rennes2, France
- Master : Anne-Hélène Olivier, "Biostatstiques", 7H, Master 1 EOPS, University Rennes2, France
- Master : Anne-Hélène Olivier, "Méthodologie", 10H, Master 1 IEAP/APPCM, University Rennes2, France
- Master : Anne-Hélène Olivier, "Contrôle moteur : Boucle perceptivo-motrice", 3H, Master 1IEAP, Université Rennes 2, France
- Master: Fabrice Lamarche, "Compilation pour l'image numérique", 29h, Master 1, ESIR, University of Rennes 1, France
- Master: Fabrice Lamarche, "Synthèse d'images", 12h, Master 1, ESIR, University of Rennes 1, France
- Master: Fabrice Lamarche, "Synthèse d'images avancée", 28h, Master 1, ESIR, University of Rennes 1, France
- Master: Fabrice Lamarche, "Modélisation Animation Rendu", 36h, Master 2, ISTIC, University of Rennes 1, France
- Master: Fabrice Lamarche, "Jeux vidéo", 26h, Master 2, ESIR, University of Rennes 1, France
- Master: Fabrice Lamarche, "Motion for Animation and Robotics", 9h, Master 2 SIF, ISTIC, University of Rennes 1, France.
- Master : Armel Crétual, "Méthodologie", leader of the module, 20H, Master 1 M2S, University Rennes2, France
- Master : Armel Crétual, "Biostatstiques", leader of the module, 30H, Master 2 M2S, University Rennes2, France
- Master : Richard Kulpa, "Boucle analyse-modélisation-simulation du mouvement", 27h, leader of the module, Master 2, Université Rennes 2, France
- Master : Richard Kulpa, "Méthodes numériques d'analyse du geste", 27h, leader of the module, Master 2, Université Rennes 2, France
- Master : Richard Kulpa, "Cinématique inverse", 3h, leader of the module, Master 2, Université Rennes 2, France
- Master: Marc Christie, Head of Master 2 Ingénierie Logicielle (45 students)
- Master: Marc Christie, "Multimedia Mobile", Master 2, leader of the module, 32h (IL) + 32h (Miage), Computer Science, University of Rennes 1, France
- Master: Marc Christie, "Projet Industriel Transverse", Master 2, 32h, leader of the module, Computer Science, University of Rennes 1, France
- Master: Marc Christie, "Modelistion Animation Rendu", Master 2, 16h, leader of the module, Computer Science, University of Rennes 1, France
- Master: Marc Christie, "Web Engineering", Master 1, 16h, leader of the module, Computer Science, University of Rennes 1, France
- Master: Marc Christie, "Advanced Computer Graphics", Master 1, 10h, leader of the module, Computer Science, ENS, France
- Master: Marc Christie, "Motion for Animation and Robotics", Master 2 SIF, Computer Science, France
- Licence : Franck Multon, "Ergonomie du poste de travail", Licence STAPS L2 & L3, University Rennes2, France
- Licence: Charles Pontonnier, "Lagrangian Mechanics" , leader of the module, 22H, M2 Mechatronics, Ecole Normale Supérieure de Rennes, France
- Licence: Charles Pontonnier, "Serial Robotics", leader of the module, 24H, L3 Mechatronics, Ecole Normale Supérieure de Rennes, France
- Licence : Anne-Hélène Olivier, "Analyse cinématique du mouvement", 100H , Licence 1, University Rennes 2, France
- Licence : Anne-Hélène Olivier, "Anatomie fonctionnelle", 7H , Licence 1, University Rennes 2, France
- Licence : Anne-Hélène Olivier, "Effort et efficience", 12H , Licence 2, University Rennes 2, France
- Licence : Anne-Hélène Olivier, "Locomotion et handicap", 12H , Licence 3, University Rennes 2, France
- Licence : Anne-Hélène Olivier, "Biomécanique spécifique aux APA", 8H , Licence 3, University Rennes 2, France
- Licence : Anne-Hélène Olivier, "Biomécanique du viellissement", 12H , Licence 3, University Rennes 2, France
- Licence: Fabrice Lamarche, "Initiation à l'algorithmique et à la programmation", 56h, License 3, ESIR, University of Rennes 1, France
- License: Fabrice Lamarche, "Programmation en C++", 46h, License 3, ESIR, University of Rennes 1, France
- Licence: Fabrice Lamarche, "IMA", 24h, License 3, ENS Rennes, ISTIC, University of Rennes 1, France
- Licence : Armel Crétual, "Analyse cinématique du mouvement", 100H, Licence 1, University Rennes 2, France
- Licence : Richard Kulpa, "Biomécanique (dynamique en translation et rotation)", 48h, Licence 2, Université Rennes 2, France
- Licence : Richard Kulpa, "Méthodes numériques d'analyse du geste", 48h, Licence 3, Université Rennes 2, France
- Licence : Richard Kulpa, "Statistiques et informatique", 15h, Licence 3, Université Rennes 2, France
10.2.1 Supervision
- PhD in progress (beginning Oct 2018, Defended December 2021) Hugo Brument, Toward user-adapted interactions techniques based on human locomotion laws for navigating in virtual environments, Anne-Hélène Olivier, Ferran Argelaguet (Hybrid team), Maud Marchal (Rainbow team).
- PhD (beginning Oct. 2018, defended Nov. 2021): Diane Dewez, Avatar-Based Interaction in Virtual Reality, Ferran Argelaguet (Hybrid team), Ludovic Hoyet, Anatole Lécuyer (Hybrid team).
- PhD (beginning September 2018, defended December 2021): Olfa Haj Mamhoud, Monitoring de l’efficience gestuelle d’opérateurs sur postes de travail, University Rennes 2, Franck Multon, Georges Dumont, Charles Pontonnier
- PhD in progress (beginning January 2019): Nils Hareng, simulation of plausible bipedal locomotion of human and non-human primate, University Rennes2, Franck Multon, & Bruno Watier (CNRS LAAS in Toulouse)
- PhD in progress (beginning January 2019): Nicolas Olivier, Adaptive Avatar Customization for Immersive Experience, Cifre InterDigital, Franck Multon, Ferran Arguelaget (Hybrid team), Quentin Avril (InterDigital), Fabien Danieau (InterDigital)
- PhD in progress (beginning September 2017): Lyse Leclerc, Intérêts dans les activités physiques du rétablissement de la fonction inertielle des membres supérieurs en cas d’amputation ou d’atrophie, Armel Crétual, Diane Haering
- PhD in progress (beginning September 2018) : Jean Basset, Learning Morphologically Plausible Pose Transfer, Inria, Edmond Boyer (Morpheo Inria Grenoble), Franck Multon
- PhD in progress (beginning December 2020): Mohamed Younes, Learning and simulating strategies in sports for VR training, University Rennes1, Franck Multon, Richard Kulpa, Ewa Kijak, Simon Malinowski
- PhD in progress (beginning September 2019) : Claire Livet, Dynamique contrainte pour l'analyse musculo-squelettique en temps rapide : vers des méthodes alternatives d'estimation des forces musculaires mises en jeu dans le mouvement humain, Ecole normale supérieure, Georges Dumont, Charles Pontonnier
- PhD in progress (beginning september 2019) : Louise Demestre, simulation MUsculo-squelettique et Structure Elastique pour le Sport (MUSES), Ecole normale supérieure, Georges Dumont, Charles Pontonnier, Nicolas Bideau, Guillaume Nicolas
- PhD in progress (beginning Oct 2018): Benjamin Niay, A framework for synthezing personalised human motions from motion capture data and perceptual information, Ludovic Hoyet, Anne-Hélène Olivier, Julien Pettré (Rainbow team).
- PhD in progress (beginning Sept 2019): Alberto Jovane, Modélisation de mouvements réactifs et comportements non verbaux pour la création d’acteurs digitaux pour la réalité virtuelle, Marc Christie, Ludovic Hoyet, Claudio Pacchierotti (Rainbow team), Julien Pettré (Rainbow team).
- PhD in progress (beginning Nov 2019): Adèle Colas, Modélisation de comportements collectifs réactifs et expressifs pour la réalité virtuelle, Ludovic Hoyet, Anne-Hélène Olivier, Claudio Pacchierotti (Rainbow team), Julien Pettré (Rainbow team).
- PhD in progress (beginning Sep 2018): Carole Puil, Impact d’une stimulation plantaire orthétique sur la posture d’individus sains et posturalement déficients au cours de la station debout, et lors de la marche, Armel Crétual, Anne-Hélène Olivier
- PhD in progress: Annabelle Limballe, Anticipation dans les sports de combat : la réalité virtuelle comme solution innovante d’entraînement, Sep. 2019, Richard Kulpa & Simon Bennett & Benoit Bideau
- PhD in progress: Alexandre Vu, Evaluation de l’influence des feedbacks sur la capacité d’apprentissage dans le cadre d’interactions complexes entre joueurs et influence de ces feedbacks en fonction de l’activité sportive , Sep. 2019, Richard Kulpa & Benoit Bideau & Anthony Sorel
- PhD in progress: William Mocaer, Réseaux de Neurones à Convolution Spatio-Temporelle pour l'analyse et la reconnaissance précoce d’actions et de gestes, Sep. 2019, Eric Anquetil & Richard Kulpa
- PhD in progress (beginning September 2020): Pauline Morin, Adaptation des méthodes prédiction des efforts d’interaction pour l’analyse biomécanique du mouvement en milieu écologique, Ecole normale supérieure de Rennes, direction Georges Dumont et Charles Pontonnier
- PhD in progress (beginning June 2020): Lucas Mourot, Learning-Based Human Character Animation Synthesis for Content Production, Pierre Hellier (InterDigital), Ludovic Hoyet, Franck Multon, François Le Clerc (InterDigital).
- PhD in progress (beginning September 2020): Agathe Bilhaut, Stratégies perceptivo-motrices durant la locomotion des patients douloureux chroniques : nouvelles méthodes d’analyse et de suivi, Armel Crétual, Anne-Hélène Olivier, Mathieu Ménard (Institut Ostéopathie Rennes, M2S)
- PhD in progress (beginning October 2020): Emilie Leblong, Prise en compte des interactions sociales dans un simulateur de conduite de fauteuil roulant électrique en réalité virtuelle : favoriser l’apprentissage pour une mobilité inclusive, Anne-Hélène Olivier, Marie Babel (Rainbow team)
- PhD in progress (beginning November 2020): Thomas Chatagnon, Micro-to-macro energy-based interaction models for dense crowds behavioral simulations, Ecole normale supérieure de Rennes, Ludovic Hoyet, Anne-Hélène Olivier,Charles Pontonnier, Julien Pettré (Rainbow team).
- PhD in progress (beginning November 2020): Vicenzo Abichequer-Sangalli, Humains virtuels expressifs et réactifs pour la réalité virtuelle, Marc Christie, Ludovic Hoyet, Carol O'Sullivan (TCD, Ireland), Julien Pettré (Rainbow team).
- PhD in progress (beginning November 2020): Tairan Yin, Création de scènes peuplées dynamiques pour la réalité virtuelle, Marc Christie, Marie-Paule Cani (LIX), Ludovic Hoyet, Julien Pettré (Rainbow team).
- PhD in progress (beginning October 2020): Qian Li, Neural novel view synthesis of dynamic people from monocular videos, Adnane Boukhayma, Franck Multon.
- PhD in progress (beginning October 2021): Maé Mavromatis, Towards “Avatar-Friendly” Characterization of Virtual Reality Interaction Methods, Ferran Argelaguet (Hybrid team), Ludovic Hoyet, Anatole Lécuyer (Hybrid team).
- PhD in progress (beginning October 2021): Rebecca Crolan, Prediction of low back load during gymnastics landings for the prevention and follow-up of athlete injuries, Charles Pontonnier, Diane Haering, Matthieu Ménard (M2S Lab).
- PhD in progress (beginning November 2021): Rim Rekik, Learning and evaluating 3D human motion synthesis, Anne-Hélène Olivier, Stefanie Wuhrer (Morpheo team)
-
10.2.2 Juries
- -
- PhD defense: Univ. Claude Bernard Lyon1, Mingming Zhao, "Monitoring de la posture du conducteur", november 2021, Franck Multon, reviewer
- PhD defense: Univ. Montpellier, Carmela Calabrese, "Analysis of synchronization and leadership emergence in human group interaction", March 2021, Franck Multon, examiner
- HDR defense: Univ. Lille, Hazem Wannous, "Towards Understanding Human Behavior by Time-Series Analysis of 3D Motion", March 2021, Franck Multon, president
- PhD defense: université Paris Saclay, Benjamin Treussart, "Étude et conception d’un système de pilotage intuitif d’exosquelette pour l’assistance au port de charges", march 2021, Charles Pontonnier, reviewer
- PhD defense: Politecnico di Torino, Divyaksh Subhash Chander, "Modelling the Physical Human-Exoskeleton Interface", september 2021, Charles Pontonnier, examiner
- PhD defense: Institut Supérieur de l'Aéronautique et de l'Espace Toulouse, Rebai Soret, "Paradigme de Posner du laboratoire au monde réel : orientation de l’attention en espace avant et arrière ", November 2021, Anne-Hélène Olivier, reviewer.
11 Scientific production
11.1 Major publications
- 1 inproceedingsNeural Human Deformation Transfer.3DV 2021 - 9th International Conference on 3D VisionLondres (on line event), United KingdomDecember 2021, 1-12
- 2 articleAvatar and Sense of Embodiment: Studying the Relative Preference Between Appearance, Control and Point of View.IEEE Transactions on Visualization and Computer Graphics265May 2020, 2062-2072
- 3 articleA neural networks approach to determine factors associated with self-reported discomfort in picking tasks.Human Factors2021, 1-24
- 4 articleBiomechanical fidelity of simulated pick-and-place tasks: impact of visual and haptic renderings.IEEE Transactions on Haptics (ToH)2021, 8
- 5 articleExample-driven virtual cinematography by learning camera behaviors.ACM Transactions on Graphics394July 2020
- 6 articleAn automatic and simplified approach to muscle path modelling.Journal of Biomechanical Engineering2021, 1-10
- 7 articleCusToM: a Matlab toolbox for musculoskeletal simulation.Journal of Open Source Software433January 2019, 1-3
- 8 inproceedingsDual Mesh Convolutional Networks for Human Shape Correspondence.3DV 2021 - International Conference on 3D VisionSurrey, United KingdomDecember 2021, 1-10
- 9 articleBinary Graph Descriptor for Robust Relocalization on Heterogeneous Data.IEEE Robotics and Automation Letters2022
- 10 articleThe effect of gender and attractiveness of motion on proximity in virtual reality.ACM Transactions on Applied Perception174November 2020, 1-15
11.2 Publications of the year
International journals
International peer-reviewed conferences
National peer-reviewed Conferences
Conferences without proceedings
Doctoral dissertations and habilitation theses
Reports & preprints
11.3 Other
Scientific popularization