Keywords
Computer Science and Digital Science
- A5.1.8. 3D User Interfaces
- A5.4. Computer vision
- A5.4.4. 3D and spatio-temporal reconstruction
- A5.4.5. Object tracking and motion analysis
- A5.5.1. Geometrical modeling
- A5.5.4. Animation
- A5.6. Virtual reality, augmented reality
- A6.2.8. Computational geometry and meshes
Other Research Topics and Application Domains
- B2.6.3. Biological Imaging
- B2.8. Sports, performance, motor skills
- B9.2.2. Cinema, Television
- B9.2.3. Video games
- B9.4. Sports
1 Team members, visitors, external collaborators
Research Scientists
- Edmond Boyer [Team leader, Inria, Senior Researcher, HDR]
- Stefanie Wuhrer [Inria, Researcher, HDR]
Faculty Members
- Jean-Sébastien Franco [Institut polytechnique de Grenoble, Associate Professor, until Aug 2021, HDR]
- Sergi Pujades Rocamora [Univ Grenoble Alpes, Associate Professor]
Post-Doctoral Fellow
- Joao Pedro Cova Regateiro [Univ Grenoble Alpes]
PhD Students
- Matthieu Armando [Inria]
- Jean Basset [Inria]
- Nicolas Comte [ANATOSCOPE, Cifre]
- Raphael Dang Nhu [Inria, until May 2021]
- Sanae Dariouche [Univ Grenoble Alpes, until Feb 2021]
- Anilkumar Erappanakoppal Swamy [Naver Labs, from Jul 2021]
- Roman Klokov [Inria]
- Mathieu Marsot [Inria]
- Di Meng [Inria]
- Aymen Merrouche [Inria, from Oct 2021]
- Abdullah Haroon Rasheed [Inria, until Oct 2021]
- Rim Rekik Dit Nekhili [Inria, from Nov 2021]
- Briac Toussaint [Univ Grenoble Alpes, from Oct 2021]
- Nitika Verma [Inria, until Apr 2021]
- Boyao Zhou [Inria]
- Pierre Zins [Inria]
Technical Staff
- Laurence Boissieux [Inria, Engineer]
- Julien Pansiot [Inria, Engineer]
Interns and Apprentices
- Mahmoud Ali [Inria, from Feb 2021 until Aug 2021]
- Yassir Bendou [Inria, from Apr 2021 until Sep 2021]
- Rohit Garg [Inria, from May 2021 until Jul 2021]
- Timothee Launay [Inria, from Mar 2021 until Jul 2021]
- Aymen Merrouche [Inria, from Apr 2021 until Sep 2021]
- Badr Ouannas [Inria, from Mar 2021 until Aug 2021]
- Daria Senshina [Univ Grenoble Alpes, from Feb 2021 until Jun 2021]
- Briac Toussaint [Inria, from Mar 2021 until Aug 2021]
Administrative Assistant
- Nathalie Gillot [Inria]
2 Overall objectives
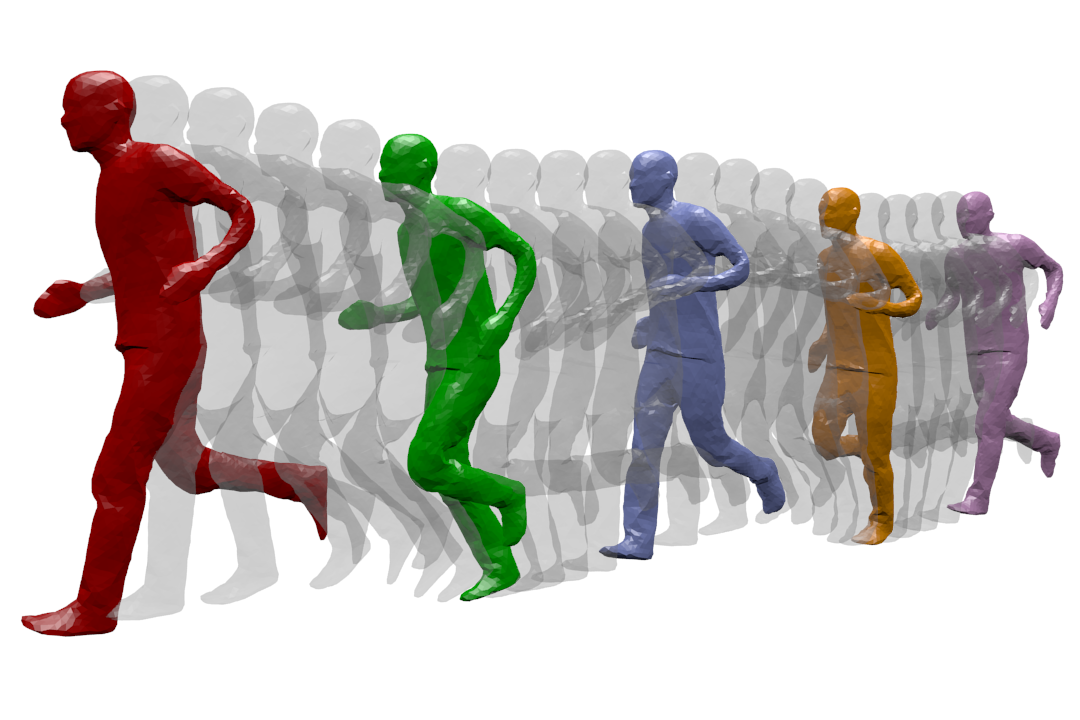
Dynamic Geometry Modeling: a human is captured while running. The different temporal acquisition instances are shown on the same figure.
MORPHEO's ambition is to perceive and interpret shapes that move using multiple camera systems. Departing from standard motion capture systems, based on markers, that provide only sparse information on moving shapes, multiple camera systems allow dense information on both shapes and their motion to be recovered from visual cues. Such ability to perceive shapes in motion brings a rich domain for research investigations on how to model, understand and animate real dynamic shapes, and finds applications, for instance, in gait analysis, bio-metric and bio-mechanical analysis, animation, games and, more insistently in recent years, in the virtual and augmented reality domain. The MORPHEO team particularly focuses on three different axes within the overall theme of 3D dynamic scene vision or 4D vision:
- Shape and appearance models: how to build precise geometric and photometric models of shapes, including human bodies but not limited to, given temporal sequences.
- Dynamic shape vision: how to register and track moving shapes, build pose spaces and animate captured shapes.
- Inside shape vision: how to capture and model inside parts of moving shapes using combined color and X-ray imaging.
The strategy developed by MORPHEO to address the mentioned challenges is based on methodological tools that include in particular learning-based approaches, geometry, Bayesian inference and numerical optimization. The potential of machine learning tools in 4D vision is still to be fully investigated, and Morpheo contributes to exploring this direction.
3 Research program
3.1 Shape and Appearance Modeling
Standard acquisition platforms, including commercial solutions proposed by companies such as Microsoft, 3dMD or 4DViews, now give access to precise 3D models with geometry, e.g. meshes, and appearance information, e.g. textures. Still, state-of-the-art solutions are limited in many respects: They generally consider limited contexts and close setups with typically at most a few meter side lengths. As a result, many dynamic scenes, even a body running sequence, are still challenging situations; They also seldom exploit time redundancy; Additionally, data driven strategies are yet to be fully investigated in the field. The MORPHEO team builds on the Kinovis platform for data acquisition and has addressed these issues with, in particular, contributions on time integration, in order to increase the resolution for both shapes and appearances, on representations, as well as on exploiting machine learning tools when modeling dynamic scenes. Our originality lies, for a large part, in the larger scale of the dynamic scenes we consider as well as in the time super resolution strategy we investigate. Another particularity of our research is a strong experimental foundation with the multiple camera Kinovis platforms.
3.2 Dynamic Shape Vision
Dynamic Shape Vision refers to research themes that consider the motion of dynamic shapes, with e.g. shapes in different poses, or the deformation between different shapes, with e.g. different human bodies. This includes for instance shape tracking and shape registration, which are themes covered by MORPHEO. While progress has been made over the last decade in this domain, challenges remain, in particular due to the required essential task of shape correspondence that is still difficult to perform robustly. Strategies in this domain can be roughly classified into two categories: (i) data driven approaches that learn shape spaces and estimate shapes and their variations through space parameterizations; (ii) model based approaches that use more or less constrained prior models on shape evolutions, e.g. locally rigid structures, to recover correspondences. The MORPHEO team is substantially involved in both categories. The second one leaves more flexibility for shapes that can be modeled, an important feature with the Kinovis platform, while the first one is interesting for modeling classes of shapes that are more likely to evolve in spaces with reasonable dimensions, such as faces and bodies under clothing. The originality of MORPHEO in this axis is to go beyond static shape poses and to consider also the dynamics of shape over several frames when modeling moving shapes, and in particular with shape tracking, animation and, more recently, face registration.
3.3 Inside Shape Vision
Another research axis is concerned with the ability to perceive inside moving shapes. This is a more recent research theme in the MORPHEO team that has gained importance. It was originally the research associated to the Kinovis platform installed in the Grenoble Hospitals. This platform is equipped with two X-ray cameras and ten color cameras, enabling therefore simultaneous vision of inside and outside shapes. We believe this opens a new domain of investigation at the interface between computer vision and medical imaging. Interesting issues in this domain include the links between the outside surface of a shape and its inner parts, especially with the human body. These links are likely to help understanding and modeling human motions. Until now, numerous dynamic shape models, especially in the computer graphic domain, consist of a surface, typically a mesh, bound to a skeletal structure that is never observed in practice but that help anyway parameterizing human motion. Learning more accurate relationships using observations can therefore significantly impact the domain.
3.4 Shape Animation
3D animation is a crucial part of digital media production with numerous applications, in particular in the game and motion picture industry. Recent evolutions in computer animation consider real videos for both the creation and the animation of characters. The advantage of this strategy is twofold: it reduces the creation cost and increases realism by considering only real data. Furthermore, it allows to create new motions, for real characters, by recombining recorded elementary movements. In addition to enable new media contents to be produced, it also allows to automatically extend moving shape datasets with fully controllable new motions. This ability appears to be of great importance with the recent advent of deep learning techniques and the associated need for large learning datasets. In this research direction, we investigate how to create new dynamic scenes using recorded events. More recently, this also includes applying machine learning to datasets of recorded human motions to learn motion spaces that allow to synthesize novel realistic animations.
4 Application domains
4.1 4D modeling
Modeling shapes that evolve over time, analyzing and interpreting their motion has been a subject of increasing interest of many research communities including the computer vision, the computer graphics and the medical imaging communities. Recent evolutions in acquisition technologies including 3D depth cameras (Time-of-Flight and Kinect), multi-camera systems, marker based motion capture systems, ultrasound and CT scanners have made those communities consider capturing the real scene and their dynamics, create 4D spatio-temporal models, analyze and interpret them. A number of applications including dense motion capture, dynamic shape modeling and animation, temporally consistent 3D reconstruction, motion analysis and interpretation have therefore emerged.
4.2 Shape Analysis
Most existing shape analysis tools are local, in the sense that they give local insight about an object's geometry or purpose. The use of both geometry and motion cues makes it possible to recover more global information, in order to get extensive knowledge about a shape. For instance, motion can help to decompose a 3D model of a character into semantically significant parts, such as legs, arms, torso and head. Possible applications of such high-level shape understanding include accurate feature computation, comparison between models to detect defects or medical pathologies, and the design of new biometric models.
4.3 Human Motion Analysis
The recovery of dense motion information enables the combined analysis of shapes and their motions. Typical examples include the estimation of mean shapes given a set of 3D models or the identification of abnormal deformations of a shape given its typical evolutions. The interest arises in several application domains where temporal surface deformations need to be captured and analyzed. It includes human body analyses for which potential applications are anyway numerous and important, from the identification of pathologies to the design of new prostheses.
4.4 Virtual and Augmented Reality
This domain has actually seen new devices emerge that enable now full 3D visualization, for instance the HTC Vive, the Microsoft Hololens and the Magic leap one. These devices create a need for adapted animated 3D contents that can either be generated or captured. We believe that captured 4D models will gain interest in this context since they provide realistic visual information on moving shapes that tend to avoid negative perception effects such as the uncanny valley effect. Furthermore, captured 4D models can be exploited to learn motion models that allow for realistic generation, for instance in the important applicative case of generating realistically moving human avatars. Besides 3D visualization devices, many recent applications also rely on everyday devices, such as mobile phones, to display augmented reality contents with free viewpoint ability. In this case, 3D and 4D contents are also expected.
5 Social and environmental responsibility
5.1 Footprint of research activities
We are concerned by the environmental impact of our research. While we have yet no tools to exactly measure the footprint of our activities, we are anyway evolving our practice on different aspects.
Dissemination strategy: Traditionally, Morpheo's dissemination strategy has been to publish in the top conferences of the field (CVPR, ECCV, ICCV, MICCAI), as well as to give invited talks at various locations all over the world, leading therefore to sometimes long disance work trips. Even if Morpheo's member have never been frequent long distance travelers their practice has changed. As a result of the Covid-19 crisis, our research community has started in 2020 to experiment virtual conferences. These digital meetings have some merits: beyond the obvious advantage of reduced travel (leading to fewer emissions and improved work-life balance), they democratize attendance as attending has a fraction of the previous cost. On the other hand, an important drawback is that digital attendance leads to low-quality exchanges between participants as on-line discussions can be often difficult, which actually tend to reduce the global attendance. Within the team we currently try to increase submissions to top journals in the field directly, hence reducing travels, while still attending some in-person conferences or seminars to allow for networking.
Data management: The data produced by the Morpheo team occupies large memory volumes. The Kinovis platform at Inria Grenoble typically produces around 1,5GB per second when capturing one actor at 50fps. The platform that also captures X-ray images at CHU produces around 1.3GB of data per second at 30fps for video and X-rays. For practical reasons, we reduce the data as much as possible with respect to the targeted application by only keeping e.g. 3D reconstructions or down-sampled spatial or temporal camera images. Yet, acquiring, processing and storing these data is costly in terms of resources. In addition, the data captured by these platforms are personal data with new highly constrained regulations. Our data management therefore needs to consider multiple aspects: data encryption to protect personal information, data backup to allow for reproducibility, and the environmental impact of data storage and processing. For all these aspects, we are constantly checking for new and more satisfactory solutions .
For data processing, we rely heavily on cluster uses, in particular with neural networks which are known to have a heavy carbon footprint. Yet, in our research field, these types of processing methods have been shown to lead to significant performance gains. For this reason, we continue to use neural networks as tools, while attempting to use architectures that allow for faster and more energy efficient training, and simple test cases that can often be trained on local machines (with GPU) to allow for less costly network design.
5.2 Impact of research results
Morpheo's main research topic is not related to sustainability. Yet, some of our research directions could be applied to solve problems relevant to sustainability such as:
- Realistic virtual avatar creation holds the potential to allow for realistic interactions through large geographic distances, leading to more realistic and satisfactory teleconferencing systems. As has been observed during the Covid-19 crisis, such systems can remove the need for traveling, thereby preventing costs and pollution and preserving resources. If avatars were to allow for more realistic interactions than existing teleconferencing systems, there is hope that the need to travel can be reduced even in times without health emergency. Morpheo captures and analyzes humans in 4D, thereby allowing to capture both their shapes and movement patterns, and actively works on human body and body part (in particular face) modeling. These research directions tackle problems that are highly relevant for the creation of digital avatars that do not fall into the uncanny valley (even during movement). In this line of work, Morpheo currently participates in the IPL Avatar project, in particular with the work of the Ph.D. student Jean Basset.
- Modeling and analyzing humans in clothing holds the potential to reduce the rate of returns of online sales in the clothing industry, which are currently high. In particular, highly realistic dynamic clothing models could help a consumer better evaluate the fit and dynamic aspects of the clothing. Morpheo's interest includes the analysis of clothing deformations based on 4D captures. Preliminary results have been achieved in the past (works of the former Ph.D. student Jinlong Yang), and we plan to continue investigating this line of work. To this end, our plans for future work include the capture a large-scale dataset of humans in different clothing items performing different motions in collaboration with NaverLabs Research. No such dataset exists to date, and it would allow the research community to study the dynamic effects of clothing.
Of course, as with any research direction, ours can also be used to generate technologies that are resource-hungry and whose necessity may be questionable, such as inserting animated 3D avatars in scenarios where simple voice communication would suffice, for instance.
6 Highlights of the year
We mention here the Habilitation à Diriger des recherches (HDR) obtained Jean Sebastian Franco. We also mention the starting collaboration with Naver Labs on the acquisition of a 3D human dataset.
7 New software and platforms
Morpheo's softwares are described below.
7.1 New software
7.1.1 Lucy Viewer
-
Keywords:
Data visualization, 4D, Multi-Cameras
-
Scientific Description:
Lucy Viewer is an interactive viewing software for 4D models, i.e, dynamic three-dimensional scenes that evolve over time. Each 4D model is a sequence of meshes with associated texture information, in terms of images captured from multiple cameras at each frame. Such data is available from the 4D repository website hosted by INRIA Grenoble.
With Lucy Viewer, the user can use the mouse to zoom in onto the 4D models, zoom out, rotate, translate and view from an arbitrary angle as the 4D sequence is being played. The texture information is read from the images at each frame in the sequence and applied onto the meshes. This helps the user visualize the 3D scene in a realistic manner. The user can also freeze the motion at a particular frame and inspect a mesh in detail. Lucy Viewer lets the user to also select a subset of cameras from which to apply texture information onto the meshes. The supported formats are meshes in .OFF format and associated images in .PNG or .JPG format.
-
Functional Description:
Lucy Viewer is an interactive viewing software for 4D models, i.e, dynamic three-dimensional scenes that evolve over time. Each 4D model is a sequence of meshes with associated texture information, in terms of images captured from multiple cameras at each frame.
- URL:
-
Contact:
Edmond Boyer
-
Participants:
Edmond Boyer, Jean Franco, Matthieu Armando, Eymeric Amselem
7.1.2 Shape Tracking
-
Functional Description:
We are developing a software suite to track shapes over temporal sequences. The motivation is to provide temporally coherent 4D Models, i.e. 3D models and their evolutions over time , as required by motion related applications such as motion analysis. This software takes as input a temporal sequence of 3D models in addition to a template and estimate the template deformations over the sequence that fit the observed 3D models.
-
Contact:
Edmond Boyer
7.1.3 QuickCSG V2
-
Keywords:
3D modeling, CAD, 3D reconstruction, Geometric algorithms
-
Scientific Description:
See the technical report "QuickCSG: Arbitrary and Faster Boolean Combinations of N Solids", Douze, Franco, Raffin.
The extension of the algorithm to self-intersecting meshes is described in "QuickCSG with self-intersections", a document inside the package.
-
Functional Description:
QuickCSG is a library and command-line application that computes Boolean operations between polyhedra. The basic algorithm is described in the research report "QuickCSG: Arbitrary and Faster Boolean Combinations of N Solids", Douze, Franco, Raffin. The input and output polyhedra are defined as indexed meshes. In version 2, that was developed in the context of a software transfer contract, the meshes can be self-intersecting, in which case the inside and outside are defined by the non-zero winding rule. The operation can be any arbitrary Boolean function, including one that is defined as a CSG tree. The focus of QuickCSG is speed. Robustness to degeneracies is obtained by carefully applied random perturbations.
- URL:
-
Authors:
Matthys Douze, Jean Franco, Bruno Raffin
-
Contact:
Jean Franco
7.1.4 CVTGenerator
-
Name:
CVTGenerator
-
Keywords:
Mesh, Centroidal Voronoi tessellation, Implicit surface
-
Functional Description:
CVTGenerator is a program to build Centroidal Voronoi Tessellations of any 3D meshes and implicit surfaces.
- URL:
-
Contact:
Li WANG
-
Partner:
INP Grenoble
8 New results
8.1 Volume Sweeping: Learning Photoconsistency for Multi-View Shape Reconstruction
Participants: Vincent Leroy, Jean-Sébastien Franco, Edmond Boyer.
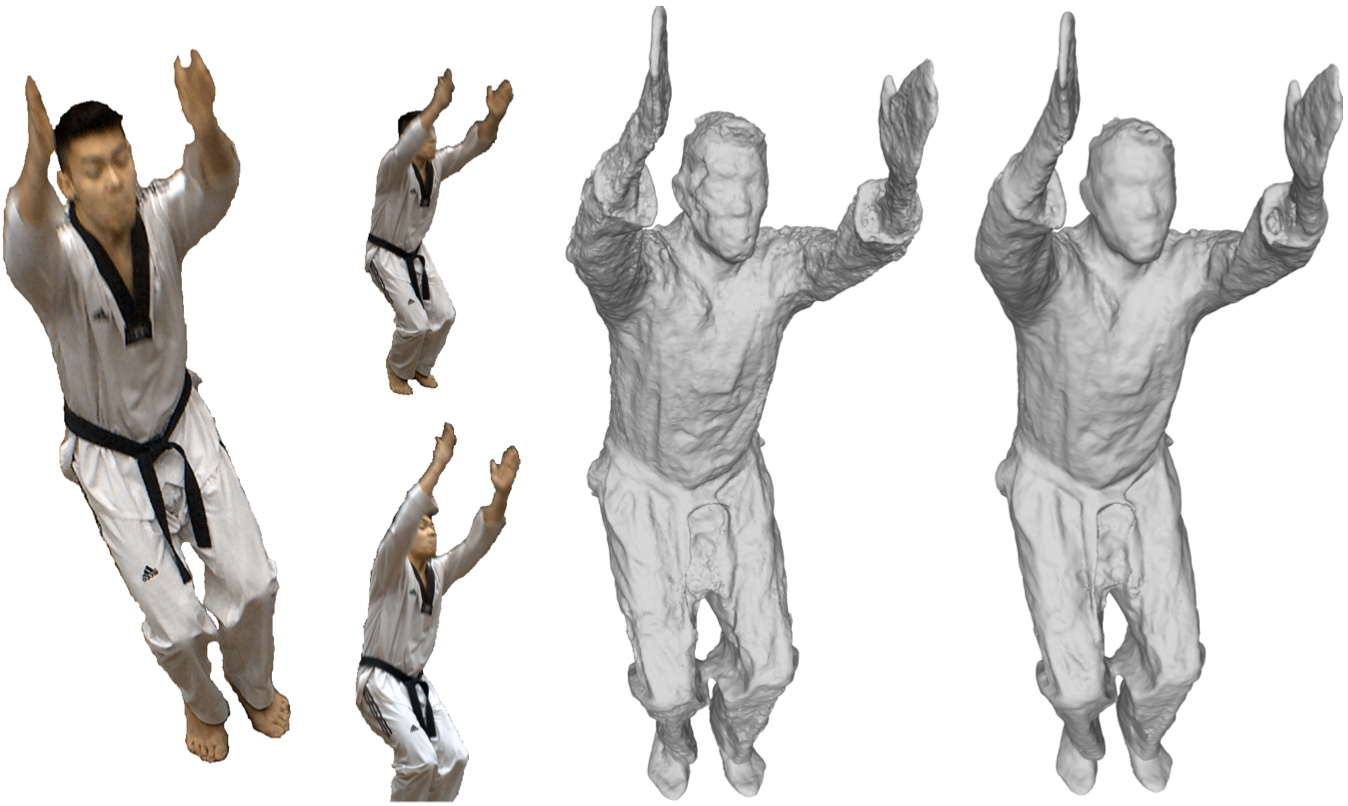
The figure shows: Left) 3 input images, (middle) plane based classifier, (right) volumetric classifier. The face is highly occluded in many views (left) making the reconstruction noisy and inaccurate when using a planar support whereas the volume counterpart yields smoother and more accurate details.
We propose a full study and methodology for multi-view stereo reconstruction with performance capture data. Multi-view 3D reconstruction has largely been studied with general, high resolution and high texture content inputs, where classic low-level feature extraction and matching are generally successful. However in performance capture scenarios, texture content is limited by wider angle shots resulting in smaller subject projection areas, and intrinsically low image content of casual clothing. We present a dedicated pipeline, based on a per-camera depth map sweeping strategy, analyzing in particular how recent deep network advances allow to replace classic multi-view photoconsistency functions with one that is learned. We show that learning based on a volumetric receptive field around a 3D depth candidate improves over using per-view 2D windows, giving the photoconsistency inference more visibility over local 3D correlations in viewpoint color aggregation. Despite being trained on a standard dataset of scanned static objects, the proposed method is shown to generalize and significantly outperform existing approaches on performance capture data, while achieving competitive results on recent benchmarks.
This work was published at International Journal of Computer Vision, one of the top journals in computer vision 4.
8.2 A Visual Approach to Measure Cloth-Body and Cloth-Cloth Friction
Participants: Haroon Rasheed , Stefanie Wuhrer, Jean-Sébastien Franco.
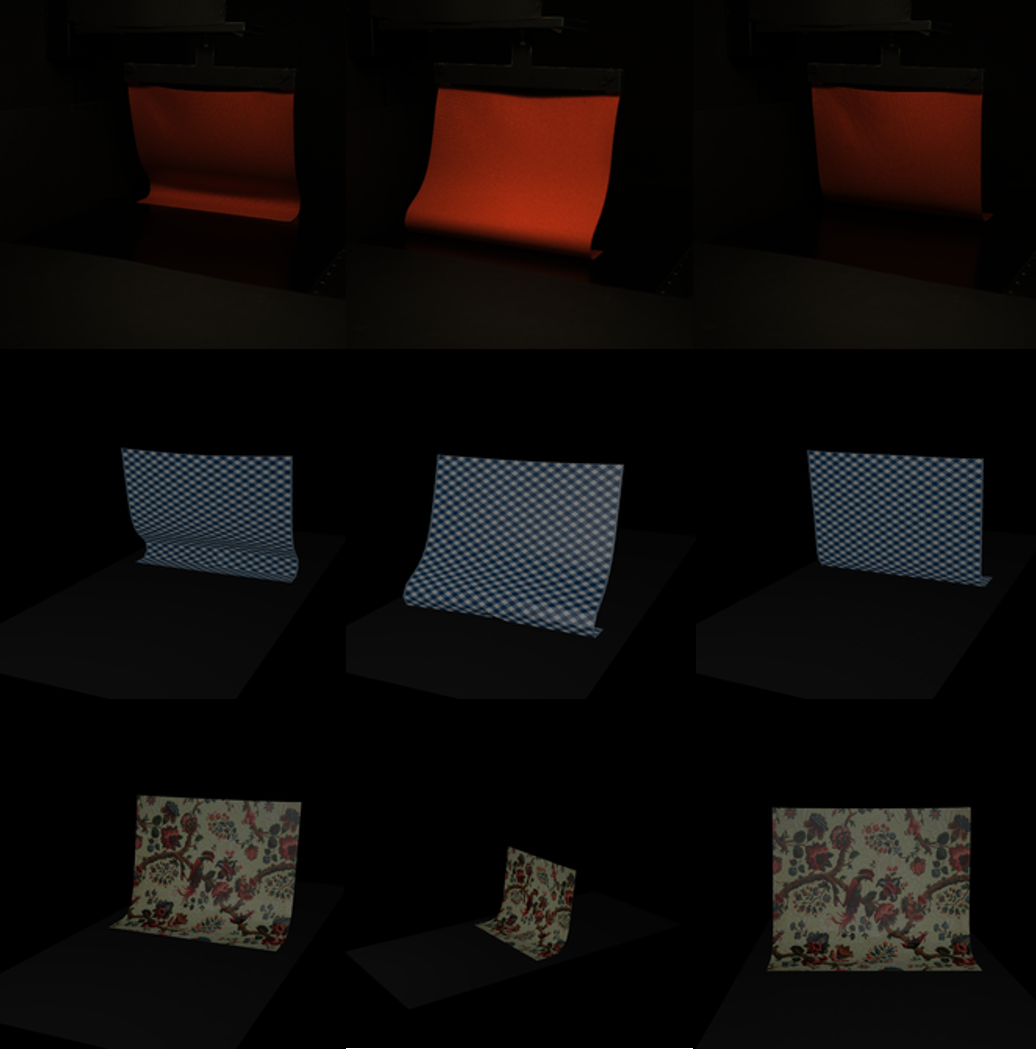
The figure shows Dataset Examples: First and second row show corresponding frames from real and synthetic data respectively. Third row shows 3 viewpoints rendered in the simulated dataset.
Measuring contact friction in soft-bodies usually requires a specialised physics bench and a tedious acquisition protocol. This makes the prospect of a purely non-invasive, video-based measurement technique particularly attractive. Previous works have shown that such a video-based estimation is feasible for material parameters using deep learning, but this has never been applied to the friction estimation problem which results in even more subtle visual variations. Because acquiring a large dataset for this problem is impractical, generating it from simulation is the obvious alternative. However, this requires the use of a frictional contact simulator whose results are not only visually plausible, but physically-correct enough to match observations made at the macroscopic scale. In this paper, which is an extended version of our former work [31], we propose to our knowledge the first non-invasive measurement network and adjoining synthetic training dataset for estimating cloth friction at contact, for both cloth-hard body and cloth-cloth contacts. To this end we build a protocol for validating and calibrating a state-of-the-art frictional contact simulator, in order to produce a reliable dataset. We furthermore show that without our careful calibration procedure, the training fails to provide accurate estimation results on real data. We present extensive results on a large acquired test set of several hundred real video sequences of cloth in friction, which validates the proposed protocol and its accuracy.
This result was published in IEEE Transactions on Pattern Analysis and Machine Intelligenge 5.
8.3 A pose-independent method for accurate and precise body composition from 3D optical scans
Participants: Sergi Pujades.
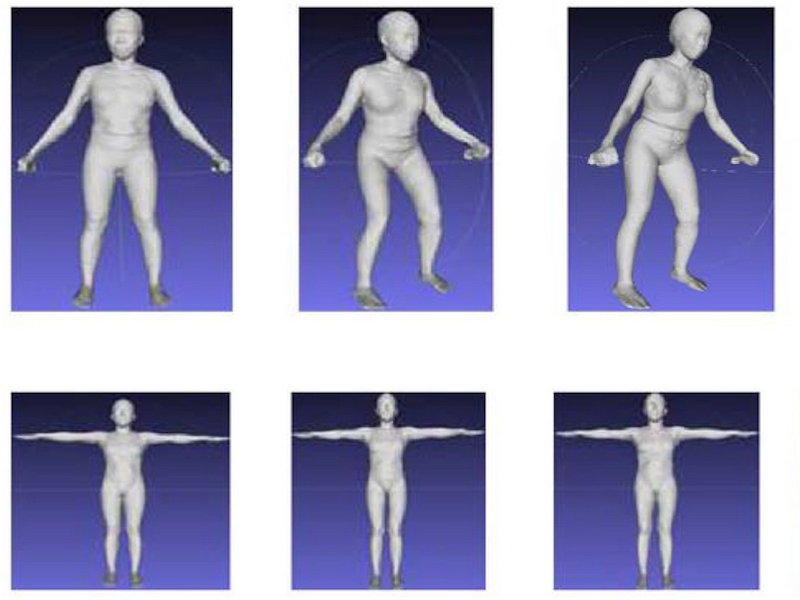
The figure shows that deriving body model composition from raw scans has the problem that, while the pose of the subject is standardized, small pose differences bias the estimates. Using a mathematically pose-normalized version of the scan allows to improve the body composition from 3D optical scans.
The aim of this study was to investigate whether digitally re-posing three-dimensional optical (3DO) whole-body scans to a standardized pose would improve body composition accuracy and precision regardless of the initial pose. Methods: Healthy adults (n = 540), stratified by sex, BMI, and age, completed whole-body 3DO and dual-energy X-ray absorptiometry (DXA) scans in the Shape Up! Adults study. The 3DO mesh vertices were represented with standardized templates and a low-dimensional space by principal component analysis (stratified by sex). The total sample was split into a training (80%) and test (20%) set for both males and females. Stepwise linear regression was used to build prediction models for body composition and anthropometry outputs using 3DO principal components (PCs). Results: The analysis included 472 participants after exclusions. After re-posing, three PCs described 95% of the shape variance in the male and female training sets. 3DO body composition accuracy compared with DXA was as follows: fat mass R2 = 0.91 male, 0.94 female; fat-free mass R2 = 0.95 male, 0.92 female; visceral fat mass R2 = 0.77 male, 0.79 female. Conclusions: Re-posed 3DO body shape PCs produced more accurate and precise body composition models that may be used in clinical or nonclinical settings when DXA is unavailable or when frequent ionizing radiation exposure is unwanted.
This result was published in the Obesity Wiley Journal. 6.
8.4 Spatio-Temporal Human Shape Completion With Implicit Function Networks
Participants: Boyao Zhou, Jean-Sébastien Franco, Edmond Boyer.
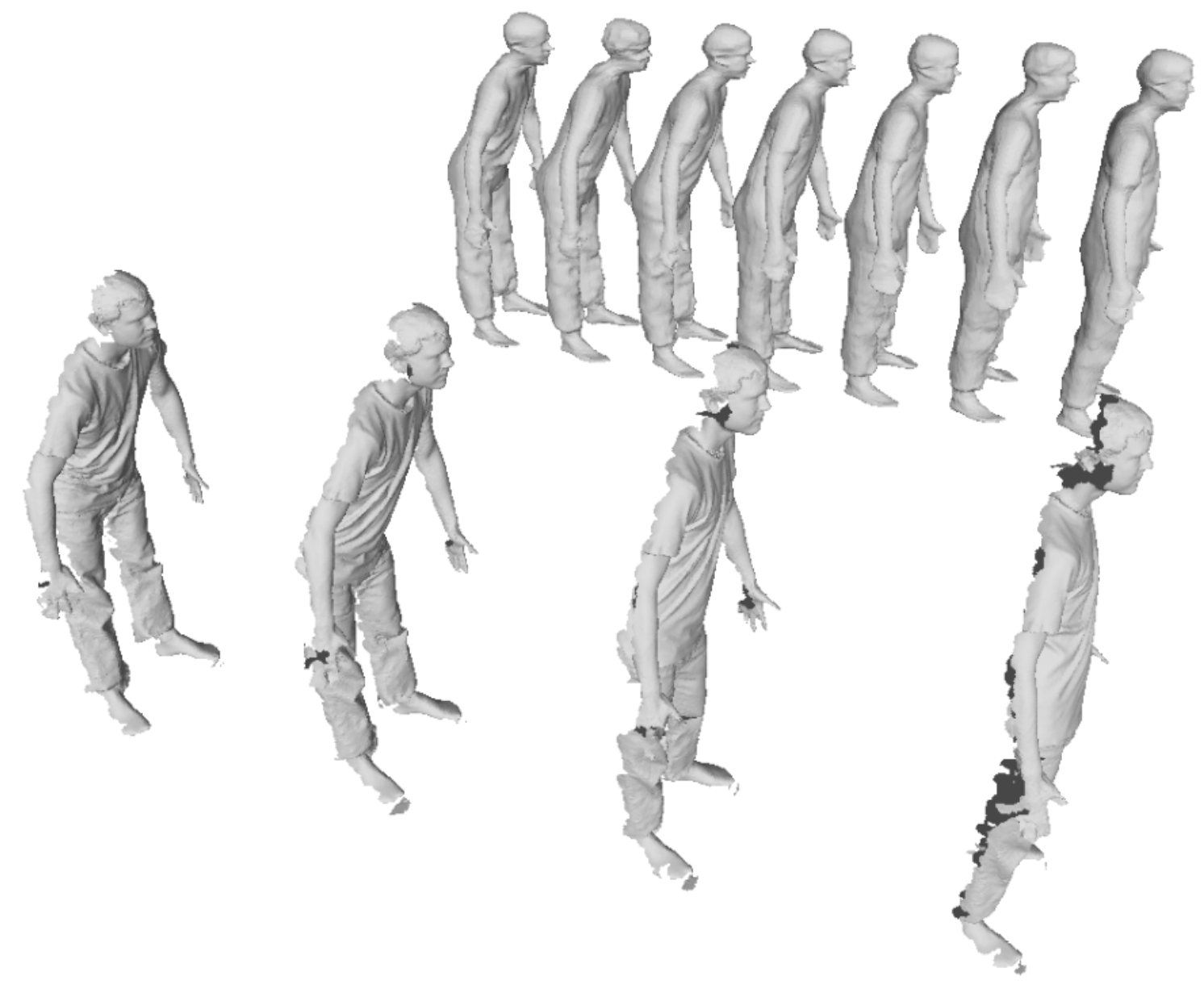
Teaser figure showing reconstructed movement completion for an example human
We address the problem of inferring a human shape from partial observations, such as depth images, in temporal sequences. Deep Neural Networks (DNN) have been shown successful to estimate detailed shapes on a frame-by-frame basis but consider yet little or no temporal information over frame sequences for detailed shape estimation. Recently, networks that implicitly encode shape occupancy using MLP layers have shown very promising results for such single-frame shape inference, with the advantage of reducing the dimensionality of the problem and providing continuously encoded results. In this work we propose to generalize implicit encoding to spatio-temporal shape inference with spatio-temporal implicit function networks or STIF-Nets, where temporal redundancy and continuity is expected to improve the shape and motion quality. To validate these added benefits, we collect and train with motion data from CAPE for dressed humans, and DFAUST for body shapes with no clothing. We show our model's ability to estimate shapes for a set of input frames, and interpolate between them. Our results show that our method outperforms existing state of the art methods, in particular the single-frame methods for detailed shape estimation.
This result was published in the 3D Vision conference 9.
8.5 Data-Driven 3D Reconstruction of Dressed Humans From Sparse Views
Participants: Pierre Zin, Stefanie Wuhrer, Edmond Boyer.
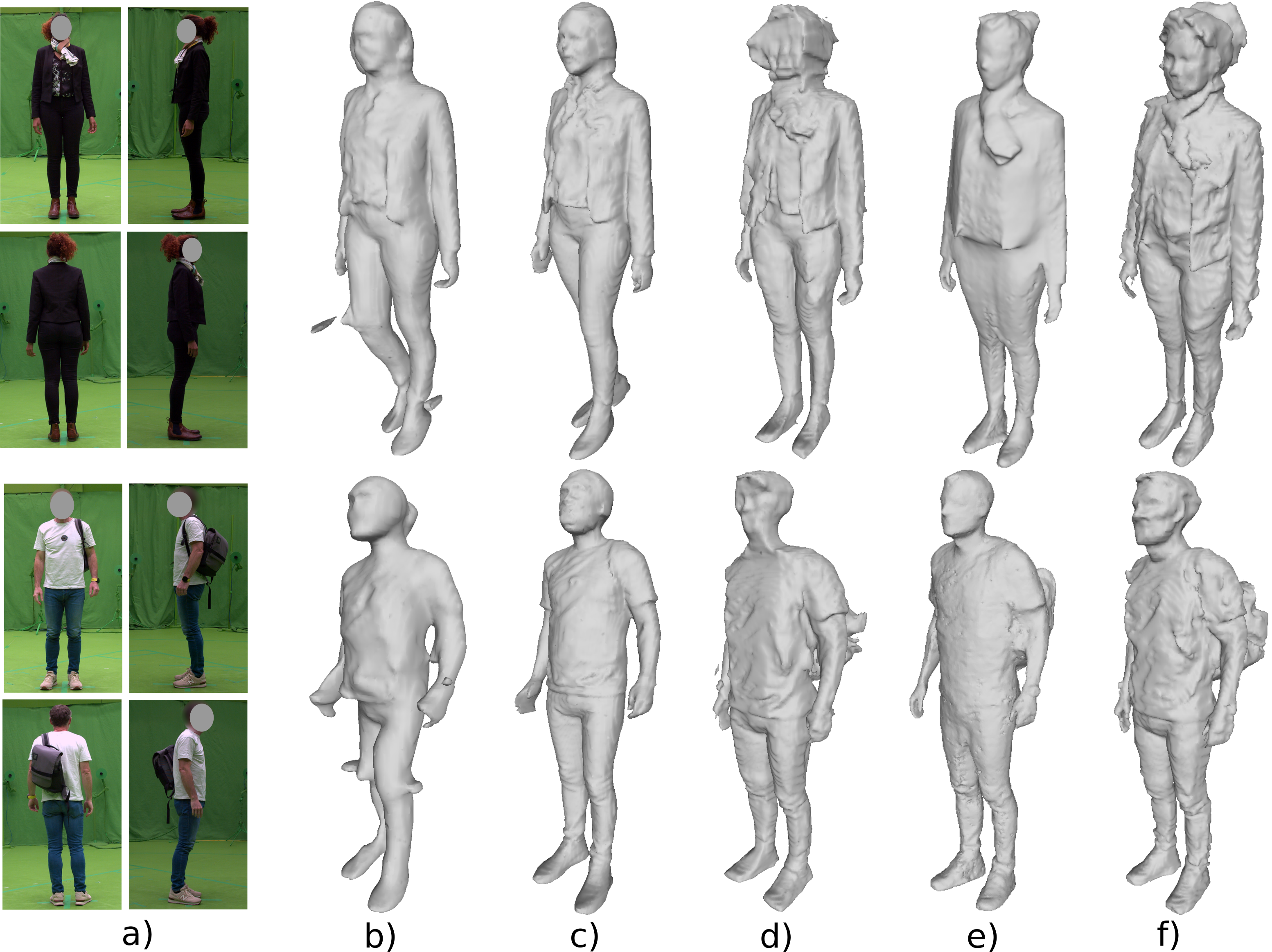
The figure shows reconstruction results: a) Real scene cropped images. b) PIFu [Saito et al. 2019] and c) PIFuHD [Saito et al. 2020] with a single frontal view. d) PIFu with 4 views. e) Multi-view stereo [Leroy et al. 2018] reconstruction with 60 views. f) Our method with 4 views.
Recently, data-driven single-view reconstruction methods have shown great progress in modeling 3D dressed humans. However, such methods suffer heavily from depth ambiguities and occlusions inherent to single view inputs. In this paper, we tackle this problem by considering a small set of input views and investigate the best strategy to suitably exploit information from these views. We propose a data-driven end-to-end approach that reconstructs an implicit 3D representation of dressed humans from sparse camera views. Specifically, we introduce three key components: first a spatially consistent reconstruction that allows for arbitrary placement of the person in the input views using a perspective camera model; second an attention-based fusion layer that learns to aggregate visual information from several viewpoints; and third a mechanism that encodes local 3D patterns under the multi-view context. In the experiments, we show the proposed approach outperforms the state of the art on standard data both quantitatively and qualitatively. To demonstrate the spatially consistent reconstruction, we apply our approach to dynamic scenes. Additionally, we apply our method on real data acquired with a multi-camera platform and demonstrate our approach can obtain results comparable to multi-view stereo with dramatically less views.
This work was published in the 3D Vision conference 10.
8.6 Neural Human Deformation Transfer
Participants: Jean Basset, Stefanie Wuhrer, Edmond Boyer.
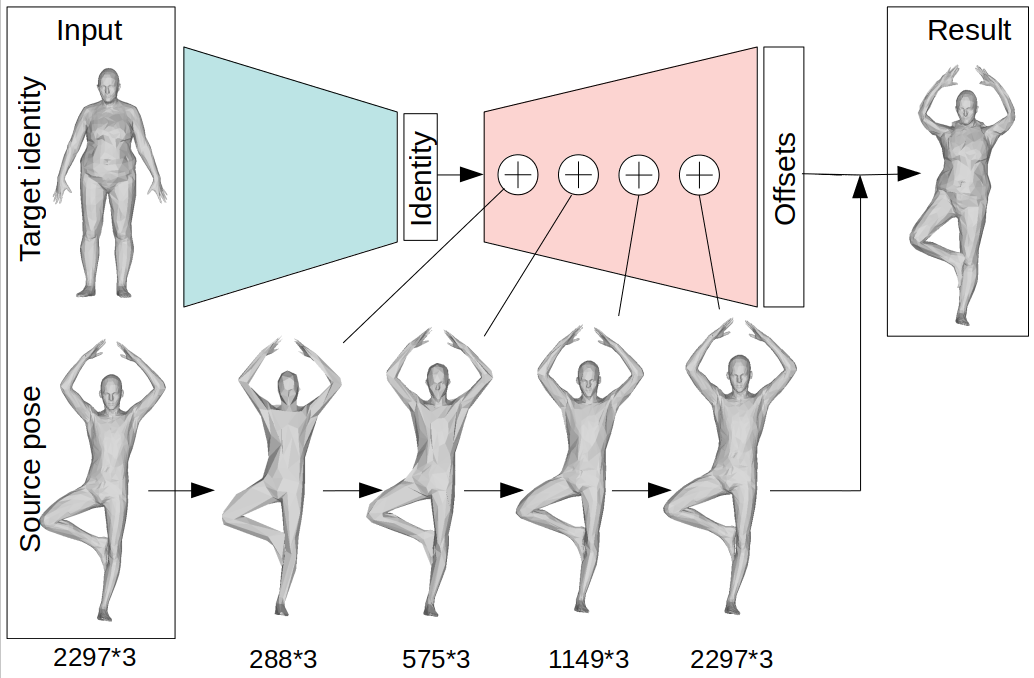
The figure shows an overview of the proposed approach. The encoder (green) generates an identity code for the target. We feed this code to the decoder (red) along with the source, which is concatenated with the decoder features at all resolution stages. The decoder finally outputs per vertex offsets from the input source towards the identity transfer result.
We consider the problem of human deformation transfer, where the goal is to retarget poses between different characters. Traditional methods that tackle this problem assume a human pose model to be available and transfer poses between characters using this model. In this work, we take a different approach and transform the identity of a character into a new identity without modifying the character's pose. This offers the advantage of not having to define equivalences between 3D human poses, which is not straightforward as poses tend to change depending on the identity of the character performing them, and as their meaning is highly contextual. To achieve the deformation transfer, we propose a neural encoder-decoder architecture where only identity information is encoded and where the decoder is conditioned on the pose. We use pose independent representations, such as isometryinvariant shape characteristics, to represent identity features. Our model uses these features to supervise the prediction of offsets from the deformed pose to the result of the transfer. We show experimentally that our method outperforms state-of-the-art methods both quantitatively and qualitatively, and generalises better to poses not seen during training. We also introduce a fine-tuning step that allows to obtain competitive results for extreme identities, and allows to transfer simple clothing.
This work was published in the 3D Vision conference 7.
8.7 Dual Mesh Convolutional Networks for Human Shape Correspondence
Participants: Nitika Verma, Edmond Boyer.
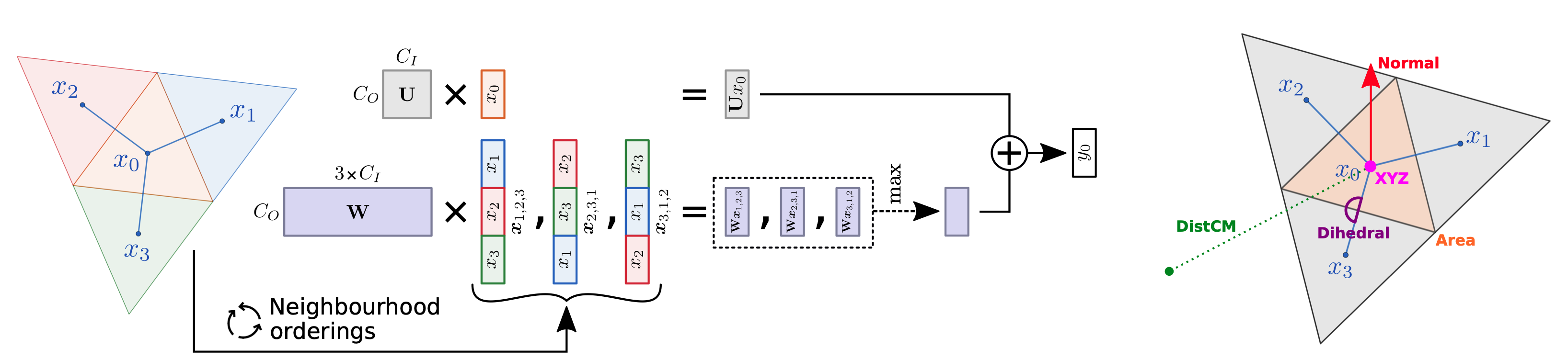
The figure shows: Left: Illustration of the Dual layer we propose for dual meshes. Right:Illustration of the triangular primal mesh M (in black) and the corresponding dual mesh D (in blue) with vertices x0 , x1 , x2 , x3 . Note that the central vertex x0 of D has exactly three neighbors. We also illustrate the input features on the dual mesh that we consider in this work.
Convolutional networks have been extremely successful for regular data structures such as 2D images and 3D voxel grids. The transposition to meshes is, however, not straightforward due to their irregular structure. We explore how the dual, face-based representation of triangular meshes can be leveraged as a data structure for graph convolutional networks. In the dual mesh, each node (face) has a fixed number of neighbors, which makes the networks less susceptible to overfitting on the mesh topology, and also allows the use of input features that are naturally defined over faces, such as surface normals and face areas. We evaluate the dual approach on the shape correspondence task on the Faust human shape dataset and variants of it with different mesh topologies. Our experiments show that results of graph convolutional networks improve when defined over the dual rather than primal mesh. Moreover, our models that explicitly leverage the neighborhood regularity of dual meshes allow improving results further while being more robust to changes in the mesh topology.
This work was published in the 3D Vision conference 8.
9 Bilateral contracts and grants with industry
9.1 Bilateral contracts with industry
Participants: Matthieu Armando, Boyao Zhou, Pierre Zin, Stefanie Wuhrer, Jean-Sébastien Franco, Edmond Boyer.
- The Morpheo INRIA team and Microsoft research set up a collaboration on the capture and modelling of moving shapes using multiple videos. Two PhD works are part of this collaboration with the objective to make contributions on 4D Modeling. The PhDs take place at Inria Grenoble Rhône-Alpes and involve visits and stays at Microsoft in Cambridge (UK) and Zurich (CH). The collaboration is part of the Microsoft Research - Inria Joint Centre. One of the PhD (Matthieu Armendo) has finished in 2021 and the toher PhD (Boyao Zhou) is pursuing his work.
- The Morpheo INRIA team has another collaboration with Facebook reality lab in San Francisco. The collaboration involves one PhD who is currently at the INRIA Grenoble Rhône-Alpes working on the estimation of shape and appearance from a single image. The collaboration started in 2019 and is ongoing with the PhD of Pierre Zins.
9.2 Bilateral grants with industry
Participants: Sergi Pujades Rocamora, Edmond Boyer.
- The Morpheo INRIA team also collaborates with the local start-up Anatoscope created by former researchers at INRIA Grenoble. The collaboration involves one PhD (Nicolas Comte) who shares his time between Morpheo and Anatoscope offices. He is working on new classifications methods of the scoliose disease that take the motion of the patient into account in addition to their morphology. The collaboration started in 2020.
10 Partnerships and cooperations
Participants: Sergi Pujades, Stefanie Wuhrer, Julien Pansiot, Edmond Boyer.
10.1 International initiatives
10.1.1 Participation in other International Programs
ShapeUp Keiki!
- NIH ShapeUp Keiki!
-
Partner Institution(s):
- University of Hawai'i, Cancer Center, USA
- Pennington Biomedical Research Center, USA
- University of California San Francisco, USA
- University of Washington, USA
- Inria Grenoble - Rhone Alpes, France
- Date/Duration: 5 years
-
Additionnal info:
The purpose of the Shape Up! Study is to explore and develop ways to measure health and body composition from 2D and 3D images. These new technologies are developed to use shape information on the outside to predict measures of health on the inside, such as body fat percentage and lean muscle mass, which can then provide useful and detailed information about various health and wellness risks. Participants provide the data needed for researchers to continue this study, which will create the largest and most powerful description of optical body shape and its association and relation to body composition, metabolic markers, function, and dietary intake. The ShapeUp Keiki study focuses on the age range of newborns to 5 year old, introducing additional challenges in the 3D capture and shape modeling of their shape.
10.2 National initiatives
10.2.1 ANR
ANR JCJC SEMBA – Shape, Motion and Body composition to Anatomy.
Existing medical imaging techniques, such as Computed Tomography (CT), Dual Energy X-Ray Absorption (DEXA) and Magnetic Resonance Imaging (MRI), allow to observe internal tissues (such as adipose, muscle, and bone tissues) of in-vivo patients. However, these imaging modalities involve heavy and expensive equipment as well as time consuming procedures. External dynamic measurements can be acquired with optical scanning equipment, e.g. cameras or depth sensors. These allow high spatial and temporal resolution acquisitions of the surface of living moving bodies. The main research question of SEMBA is: "can the internal observations be inferred from the dynamic external ones only?". SEMBA’s first hypothesis is that the quantity and distribution of adipose, muscle and bone tissues determine the shape of the surface of a person. However, two subjects with a similar shape may have different quantities and distributions of these tissues. Quantifying adipose, bone and muscle tissue from only a static observation of the surface of the human might be ambiguous. SEMBA's second hypothesis is that the shape deformations observed while the body performs highly dynamic motions will help disambiguating the amount and distribution of the different tissues. The dynamics contain key information that is not present in the static shape. SEMBA’s first objective is to learn statistical anatomic models with accurate distributions of adipose, muscle, and bone tissue. These models are going to be learned by leveraging medical dataset containing MRI and DEXA images. SEMBA's second objective will be to develop computational models to obtain a subject-specific anatomic model with an accurate distribution of adipose, muscle, and bone tissue from external dynamic measurements only.
ANR JCJC 3DMOVE - Learning to synthesize 3D dynamic human motion.
It is now possible to capture time-varying 3D point clouds at high spatial and temporal resolution. This allows for high-quality acquisitions of human bodies and faces in motion. However, tools to process and analyze these data robustly and automatically are missing. Such tools are critical to learning generative models of human motion, which can be leveraged to create plausible synthetic human motion sequences. This has the potential to influence virtual reality applications such as virtual change rooms or crowd simulations. Developing such tools is challenging due to the high variability in human shape and motion and due to significant geometric and topological acquisition noise present in state-of-the-art acquisitions. The main objective of 3DMOVE is to automatically compute high-quality generative models from a database of raw dense 3D motion sequences for human bodies and faces. To achieve this objective, 3DMOVE will leverage recently developed deep learning techniques. The project also involves developing tools to assess the quality of the generated motions using perceptual studies. This project currently involves two Ph.D. students: Mathieu Marsot hired in November 2019 and Rim Rekik-Nkhili hired November 2021.
ANR Human4D - Acquisition, Analysis and Synthesis of Human Body Shape in Motion
Human4D is concerned with the problem of 4D human shape modeling. Reconstructing, characterizing, and understanding the shape and motion of individuals or groups of people have many important applications, such as ergonomic design of products, rapid reconstruction of realistic human models for virtual worlds, and an early detection of abnormality in predictive clinical analysis. Human4D aims at contributing to this evolution with objectives that can profoundly improve the reconstruction, transmission, and reuse of digital human data, by unleashing the power of recent deep learning techniques and extending it to 4D human shape modeling. It involves 4 academic partners: The laboratory ICube at Strasbourg which is leading the project, the laboratory Cristal at Lille, the laboratory LIRIS at Lyon and the INRIA Morpheo team.
ANR Equipex+ CONTINUUM - Collaborative continuum from digital to human
The CONTINUUM project will create a collaborative research infrastructure of 30 platforms located throughout France, including Inria Grenoble's Kinovis, to advance interdisciplinary research based on interaction between computer science and the human and social sciences. Thanks to CONTINUUM, 37 research teams will develop cutting-edge research programs focusing on visualization, immersion, interaction and collaboration, as well as on human perception, cognition and behaviour in virtual/augmented reality, with potential impact on societal issues. CONTINUUM enables a paradigm shift in the way we perceive, interact, and collaborate with complex digital data and digital worlds by putting humans at the center of the data processing workflows. The project will empower scientists, engineers and industry users with a highly interconnected network of high-performance visualization and immersive platforms to observe, manipulate, understand and share digital data, real-time multi-scale simulations, and virtual or augmented experiences. All platforms will feature facilities for remote collaboration with other platforms, as well as mobile equipment that can be lent to users to facilitate onboarding.
10.2.2 Competitivity Clusters
FUI24 SPINE-PDCA
The goal of the SPINE-PDCA project is to develop a unique medical platform that will streamline the medical procedure and achieve all the steps of a minimally invasive surgery intervention with great precision through a complete integration of two complementary systems for pre-operative planning (EOS platform from EOS IMAGING) and imaging/intra-operative navigation (SGV3D system from SURGIVISIO). Innovative low-dose tracking and reconstruction algorithms will be developed by Inria, and collaboration with two hospitals (APHP Trousseau and CHU Grenoble) will ensure clinical feasibility. The medical need is particularly strong in the field of spinal deformity surgery which can, in case of incorrect positioning of the implants, result in serious musculoskeletal injury, a high repeat rate (10 to 40% of implants are poorly positioned in spine surgery) and important care costs. In paediatric surgery (e. g. idiopathic scoliosis), the rate of exposure to X-rays is an additional major consideration in choosing the surgical approach to engage. For these interventions, advanced linkage between planning, navigation and postoperative verification is essential to ensure accurate patient assessment, appropriate surgical procedure and outcome consistent with clinical objectives. The project has effectively started in October 2018 with Di Meng's recruitment as a PhD candidate.
MIAI Chair Data Driven 3D Vision
Edmond Boyer obtained a chair in the new Multidisciplinary Institute in Artificial Intelligence (MIAI) of Grenoble Alpes University. The chair entitled Data Driven 3D Vision is for 4 years and aims at investigating deep learning for 3D artificial vision in order to break some of the limitations in this domain. Applications are especially related to humans and to the ability to capture and analyze their shapes, appearances and motions, for upcoming new media devices, sport and medical applications. A post doc, Joao Regateiro, was recruited in 2020 within the chair.
11 Dissemination
Participants: Edmond Boyer, Jean-Sébastien Franco, Sergi Pujades, Stefanie Wuhrer.
11.1 Promoting scientific activities
- Edmond Boyer is a member of the board of the European Computer Vision Association (ECVA).
- Edmond Boyer is a member of the steering committee of the 3DV conference.
11.1.1 Scientific events: selection
Chair of conference program committees
- Edmond Boyer was chairing the best price committee for the conference 3DV 2021.
Member of the conference program committees
- Edmond Boyer was area chair for the conferences BMVC 2021 and 3DV 2021.
Reviewer
- Computer Vision and Pattern Recognition
- ICCV
- MICCAI
- S. Wuhrer reviewed for 3DV, CVPR and ICCV
- J.S. Franco reviewed for 3DV, CVPR and ICCV
11.1.2 Journal
Member of the editorial boards
- Computer and Graphics
- J.S. Franco served as associate editor for International Journal of Computer Vision.
- Edmond Boyer served as associate editor for International Journal of Computer Vision
Reviewer - reviewing activities
- Computer and Graphics
- S. Wuhrer reviewed for International Journal of Computer Vision and Transactions on Pattern Analysis and Machine Intelligence
- J.S. Franco reviewed for International Journal of Computer Vision and Transactions on Pattern Analysis and Machine Intelligence
11.1.3 Scientific expertise
- Sergi Pujades is a member of the Labex Persival committee.
- Stefanie Wuhrer was part of the CRCN / ISFP hiring committee.
- Edmond Boyer was consulting for the company Yokai.
11.1.4 Research administration
- Edmond Boyer is auditor for the European Computer Vision Association.
- Edmond Boyer is a member of the scientific committee at the INRIA Grenoble.
11.2 Teaching - Supervision - Juries
11.2.1 Teaching
- Licence : Sergi Pujades, Découverte des Mathématiques appliquées, 36H EqTD, L1, Université Grenoble Alpes, France.
- Licence : Sergi Pujades, Projet Logiciel, 39.75H EqTD, L2, Université Grenoble Alpes, France.
- Master : Sergi Pujades, Numerical Geometry, 15H EqTD, M1, Université Grenoble Alpes, France
- Master: Sergi Pujades, Introduction to Visual Computing, 15h EqTD, M1 MoSig, Université Grenoble Alpes.
- Master: Sergi Pujades, 3D Graphics, 11.25h EqTD, M1 Ensimag 2nd year.
- Master: Jean-Sébastien Franco, Introduction to Computer Graphics, 45h, Ensimag 2nd year, Grenoble INP.
- Master: Jean-Sébastien Franco, Introduction to Computer Vision, 48h, Ensimag 3rd year, Grenoble INP.
- Master: Jean-Sébastien Franco, Introduction to Visual Computing, 15h, M1 Mosig, Université Grenoble Alpes.
- License: Jean-Sébastien Franco, Basis of Imperative Programming (Python), 33h, Ensimag 1st year, Grenoble INP.
- Master: Jean-Sébastien Franco, Internship supervision, 15h, Ensimag 2nd and 3rd year, Grenoble INP.
- Master: Edmond Boyer, 3D Modeling, 27h, M2R Mosig GVR, Grenoble INP.
- Master: Edmond Boyer, Introduction to Visual Computing, 42h, M1 MoSig, Université Grenoble Alpes.
- Master: Edmond Boyer, Introduction to Computer Vision, 4h30, Ensimag 3rd year, Grenoble INP.
- Master: Stefanie Wuhrer, 3D Graphics, 7.5h, M1 MoSig and MSIAM, Université Grenoble Alpes.
11.2.2 Supervision
- Master: J.S. Franco, Supervision of the 2nd year program (>300 students), 36h, Ensimag 2nd year, Grenoble INP.
- Ph.D. in progress: Jean Basset, Pose transfer for 3D body models, since 01.09.2018, supervised by F. Multon (Université Rennes), E. Boyer, S. Wuhrer
- Ph.D. in progress: Boyao Zhou. Augmenting User Self-Representation in VR Environments, since 01.01.2019. Supervised by J.S. Franco, E. Boyer.
- Ph.D. in progress: Briac Toussaint. High precision non rigid-surface alignment for 3D performance capture, since 01.10.2021. Supervised by J.S. Franco.
- Ph.D. in progress: Anilkumar Swamy. Deep Neural Networks for End-to-end Human 3D Shape Retrieval in Single Images. Since 15.07.2021, supervised by J.S. Franco, Gregory Rogez.
- Ph.D. in progress: Mathieu Marsot, Generative modeling of 3D human motion, since 01.11.2019, supervised by J.S. Franco, S. Wuhrer
- Ph.D. in progress: Rim Rekik Nkhili, Human motion generation and evaluation, since 01.11.2021, supervised by A.-H. Olivier (Université Rennes), S. Wuhrer
- Ph.D. in progress: Pierre Zins, Learning to infer human motion, since 01.10.2019, supervised by E. Boyer, T. Tung (Facebook), S. Wuhrer
- Ph.D. in progress: Aymen Merrouche, Learning non-rigid surface matching, since 01.10.2021, supervised by E. Boyer
- Ph.D.: Raphaël Dang-Nhu, Generating and evaluating motion, since 01.10.2020, until May 2021, supervised by A.-H. Olivier (Université Rennes), S. Wuhrer
- Ph.D. defended: Abdullah-Haroon Rasheed, Inverse Dynamic Modeling of Cloth - Deep Learning using Physics based Simulations, since 01.11.2017, Defended 09.12.2021, supervised by F. Bertails-Descoubes (ELAN team), J.S. Franco, S. Wuhrer
- Ph.D. defended: Mathieu Armando, Processing Mesh Surface Signals for Appearance Modeling, since 01.11.2017, Defended 26.10.2021, supervised by J.S. Franco, E. Boyer.
11.2.3 Juries
- S. Wuhrer was reviewer for the Ph.D. of Riccardo Marin, University of Verona, Jun. 2021.
- S. Wuhrer was reviewer for the Ph.D. of Marie-Julie Rakotosaona, École Polytechnique, Dec. 2021.
- E. Boyer was president of the PhD committee of Beatrix-Emôke Fülop-Balog, University of Lyon, Dec. 2021.
11.3 Popularization
11.3.1 Interventions
- Edmond Boyer organized (October 2021) a seminar for the Inria students at the early stage of a PhD that aims at informing and clarifying on aspects of the PhD thesis execution.
12 Scientific production
12.1 Major publications
- 1 articleVolume Sweeping: Learning Photoconsistency for Multi-View Shape Reconstruction.International Journal of Computer VisionSeptember 2020
- 2 articleA Visual Approach to Measure Cloth-Body and Cloth-Cloth Friction.IEEE Transactions on Pattern Analysis and Machine IntelligenceJuly 2021
- 3 inproceedingsData-Driven 3D Reconstruction of Dressed Humans From Sparse Views.3DV 2021 - International Conference on 3D VisionLondon, United KingdomDecember 2021
12.2 Publications of the year
International journals
- 4 articleVolume Sweeping: Learning Photoconsistency for Multi-View Shape Reconstruction.International Journal of Computer Vision129February 2021, 284-299
- 5 articleA Visual Approach to Measure Cloth-Body and Cloth-Cloth Friction.IEEE Transactions on Pattern Analysis and Machine IntelligenceJuly 2021
- 6 articleA pose‐independent method for accurate and precise body composition from 3D optical scans.Obesity2911November 2021, 1835-1847
International peer-reviewed conferences
- 7 inproceedingsNeural Human Deformation Transfer.3DV 2021 - 9th International Conference on 3D VisionLondres (on line event), United KingdomDecember 2021, 1-12
- 8 inproceedingsDual Mesh Convolutional Networks for Human Shape Correspondence.3DV 2021 - International Conference on 3D VisionSurrey, United KingdomDecember 2021, 1-10
- 9 inproceedingsSpatio-Temporal Human Shape Completion With Implicit Function Networks.International Conference on 3D VisionOnline, United KingdomDecember 2021
- 10 inproceedingsData-Driven 3D Reconstruction of Dressed Humans From Sparse Views.3DV 2021 - International Conference on 3D VisionLondon, United KingdomDecember 2021
Scientific book chapters
- 11 inbookSeaview Version 5: A Multiplatform Software for Multiple Sequence Alignment, Molecular Phylogenetic Analyses, and Tree Reconciliation.2231Multiple Sequence AlignmentMethods in Molecular BiologyHumana2021, 241-260
Doctoral dissertations and habilitation theses
- 12 thesisInverse Dynamic Modeling of Cloth - Deep Learning using Physics based Simulations.Inria; Universite Grenoble AlpesDecember 2021
Reports & preprints
- 13 miscMulti-frame sequence generator of 4D human body motion.June 2021