Keywords
Computer Science and Digital Science
- A3.1.1. Modeling, representation
- A3.3. Data and knowledge analysis
- A3.3.3. Big data analysis
- A3.4. Machine learning and statistics
- A3.4.1. Supervised learning
- A3.4.5. Bayesian methods
- A3.4.6. Neural networks
- A3.4.7. Kernel methods
- A3.4.8. Deep learning
- A5.3. Image processing and analysis
- A5.3.2. Sparse modeling and image representation
- A5.3.3. Pattern recognition
- A5.3.4. Registration
- A5.4.1. Object recognition
- A5.4.4. 3D and spatio-temporal reconstruction
- A5.4.5. Object tracking and motion analysis
- A5.4.6. Object localization
- A5.9.1. Sampling, acquisition
- A5.9.2. Estimation, modeling
- A5.9.3. Reconstruction, enhancement
- A5.9.5. Sparsity-aware processing
- A5.9.6. Optimization tools
- A6.1.2. Stochastic Modeling
- A6.1.3. Discrete Modeling (multi-agent, people centered)
- A6.1.4. Multiscale modeling
- A6.1.5. Multiphysics modeling
- A6.2.3. Probabilistic methods
- A6.2.4. Statistical methods
- A6.2.6. Optimization
- A6.3. Computation-data interaction
- A6.3.1. Inverse problems
- A6.3.2. Data assimilation
- A6.3.3. Data processing
- A6.3.4. Model reduction
- A6.3.5. Uncertainty Quantification
- A9.2. Machine learning
- A9.3. Signal analysis
Other Research Topics and Application Domains
- B1.1.1. Structural biology
- B1.1.7. Bioinformatics
- B1.1.8. Mathematical biology
- B2.2.3. Cancer
- B2.6. Biological and medical imaging
1 Team members, visitors, external collaborators
Research Scientists
- Charles Kervrann [Team leader, Inria, Senior Researcher, HDR]
- Anaïs Badoual [Inria, Researcher, from Nov 2021]
- Patrick Bouthemy [Inria, Senior Researcher, HDR]
- Jean Salamero [CNRS, Senior Researcher, UMR 144 CNRS - Institut Curie, HDR]
Post-Doctoral Fellows
- Anaïs Badoual [Inria, until Sep 2021]
- Emmanuel Moebel [Inria]
PhD Students
- Lisa Balsollier [CNRS, from Oct 2021]
- Antonin Deschemps [Inria]
- Gwendal Fouché [Inria, EPC Hybrid, Rennes]
- Sebastien Herbreteau [Inria]
- Yunjiao Lu [INRAE, until June 2021, MaIAGE Unit, Jouy-en-Josas ]
- Etienne Meunier [Inria]
- Claire-Jing Rouchet [INRAE, MICALIS UMR, Jouy-en-Josas ]
- Antoine Salomon [Inria, until Jan 2021]
- Quentin Tallon [IRSN/LRACC, Fontenay-aux-Roses, from Oct 2021]
Technical Staff
- Kevin Fournier [Inria, Engineer, From Dec 2021]
- Ludovic Leconte [CNRS, Engineer, (IE CNRS) UMR 144 CNRS - Institut Curie]
- Leo Maury [CNRS, Engineer, from Oct 2021]
- Sylvain Prigent [Inria, Engineer, (Expert-Engineer)]
- Cesar Augusto Valades-Cruz [Inria, Engineer, (Expert-Engineer)]
Interns and Apprentices
- Lisa Balsollier [Inria, from Apr 2021 until Jun 2021]
- Leo Maury [Inria, Apprentice, until Sep 2021]
Administrative Assistant
- Caroline Tanguy [Inria]
External Collaborator
- Frédéric Lavancier [Univ de Nantes, HDR]
2 Overall objectives
2.1 Glossary
- LLSM (Lattice Light Sheet Microscopy): high resolution Structured Illumination Microscopy that uses Light Sheet Bessel Beam illumination 40.
- TIRF (Total Internal Reflectance): 2D optical microscopy using evanescent waves and total reflectance 37.
- STORM (Stochastic Optical Reconstruction Microscopy): high-resolution microscopy using stochastic photo-activation of fluorophores and adjustment of point spread functions.
- PALM (Photo-Activated Localization Microscopy): high-resolution microscopy using stochastic photo-activation of fluorophores and adjustment of point spread functions 38.
- Cryo-ET (Cryo-Electron Tomography): 3D representation of sub-cellular and molecular objects of 5-20 nanometers, frozen at very low temperatures, from 2D projections using a transmission electron microscope.
2.2 Scientific context and motivations
During the past two decades, biological imaging has undergone a revolution in the development of new microscopy techniques that allow visualization of tissues, cells, proteins and macromolecular structures at all levels of resolution, physiological states, chemical composition and dynamics. Thanks to recent advances in optics, digital sensors and labeling probes (e.g., Colored Fluorescence Protein), one can now visualize sub-cellular components and organelles at the scale of several hundreds of nanometers to a tens nanometers, in live. As a result, fluorescent microscopy and multimodal imaging (fluorophores at various wavelengths) have become the workhorse of modern biology. As a matter of fact, taking into account all the publications in the 10 most relevant journals in fundamental biology (those with highest IF) for the last 2018-2019 years, the ratio of experimental figures based on BioImage data is close to 70% (GBI EoE.V, Singapour, Sep 2019). All the technological advances in microscopy have created new issues and challenges for researchers in quantitative image processing and analysis. Since the digital processing is now part of the imaging loop, image processing may even drive imaging. A brilliant example of this shift in paradigm is super-resolution localization microscopy (PALM, STED), which was awarded the 2014 Nobel Prize in Chemistry.
2.3 Challenges in biological image processing and quantitative microscopy
In most cases, modern microscopy in biology is characterized by a large number of dimensions that fit perfectly with the complexity of biological features: two or three spatial dimensions, at macro to nano-scales, and one temporal dimension, sometimes spectrally defined and often corresponding to one particular biomolecular species. Dynamic microscopy is also characterized by the nature of the observable objects (cells, organelles, single molecules, ...), by the large number of small size and mobile elements (chromosomes, vesicles, ...), by the complexity of the dynamic processes involving many entities or group of entities sometimes interacting, by particular phenomena of coalescence often linked to image resolution problems, finally by the association, dissociation, recomposition or constitution of those entities (such as membrane fusion and budding). Thus, the corpus of data to be considered for any analysis involving multiple image series acquisitions is massive (up to few GigaBytes per hour). Therefore, it becomes necessary to facilitate and rationalize the production of those multidimensional data, to improve post acquisition analysis, and to favor the organization and the interpretation of the information extracted from this data corpus. It motivates innovative methods and concepts for data fusion, image registration, super-resolution, data mining... More importantly, modern microscopy has led to recent breakthroughs, related to the potential interactions between molecules in the cell. A long-term research consists now in inferring the relationships between the dynamics of macromolecules and their functions. Research on computational biology and quantitative bioimaging lies at the core of the activities of SERPICO team.
2.4 Objectives of SERPICO in cell imaging
In order to tackle the aforementioned challenges, the SERPICO team aims to develop innovative approaches and paradigms for image reconstruction, 3D molecule tracking and motion estimation, and biophysical parameter estimation to face the huge data volumes acquired with cutting-edge microscopy set-ups. To this end, applied mathematics, image processing and analysis have to be considered in association with biophysics and biology. To be successful, a sustained synergy between all these scientific domains is necessary. To improve state-of-the-art methods and solve important problems in computational bioimaging, the members of SERPICO especially address the following topics:
- Image restoration/reconstruction motivated by preserving cell integrity (photo-toxicity versus exposure time) and image analysis in multidimensional microscopy;
- Motion analysis and computation of molecule trajectories in live-cell imaging to study molecular interactions in space and time;
- Computational simulation, modeling and estimation of molecule trafficking and interactions at different spatial and temporal scales.
The resulting mathematical models and algorithms will help biologists to decipher molecular processes in fundamental biology and will be exploited for health applications: disease diagnosis, detection of genomic instabilities, deterioration of cell cycle, cancer prevention.
We have successfully developed statistical and variational aggregation methods for image denoising and optical flow, and elaborated powerful methods for image colocalization, diffusion estimation, trajectory estimation-classification, and multimodal registration. An additional issue was the design and distribution of software tools for the biological image analysis and microscopy communities. Finally, the team has focused on the cellular and molecular mechanisms involved in molecule and protein transport and trafficking at the scale of a single cell. Our contributions are detailed in the next sections along three research axes.
2.5 Organization and collaborations
In collaboration with UMR 144 CNRS - Institut Curie (and in cooperation with the “Space Time imaging of Endomembranes and organelles Dynamics” team) and PICT-IBiSA (Cell and Tissue Imaging Facilities), the members of the SERPICO team have participated in several projects (PhD and post-doc supervision, contracts...) in the field of cell biology and microscopy. We have promoted non-parametric methods since prior knowledge cannot be easily taken into account for extracting unattended but desired information from image data. We have also proposed user-friendly algorithms for processing 2D and 3D image sequences. The projects of SERPICO were in line with several studies led in the UMR 144 CNRS - Institut Curie Unit. A subset of studies was related to instrumentation in electronic and photonic microscopy (PICT-IBiSA platform) including computational aspects on the reconstruction and enhancement of images related to sub-diffraction light microscopy and multimodal approaches. SERPICO projects relied partially on the advances of these instrumental projects and a positive synergy was established.
3 Research program
3.1 Statistics and algorithms for computational microscopy
Fluorescence microscopy limitations are due to the optical aberrations, the resolution of the microscopy system, and the photon budget available for the biological specimen. Hence, new concepts have been defined to address challenging image restoration and molecule detection problems while preserving the integrity of samples. Accordingly, the main stream regarding denoising, deconvolution, registration and detection algorithms advocates appropriate signal processing framework to improve spatial resolution, while at the same time pushing the illumination to extreme low levels in order to limit photo-damages and phototoxicity. As a consequence, the question of adapting cutting-edge signal denoising and deconvolution, object detection, and image registration methods to 3D fluorescence microscopy imaging has retained the attention of several teams over the world.
In this area, the SERPICO team has developed a strong expertise in key topics in computational imaging including image denoising and deconvolution, object detection and multimodal image registration. Several algorithms proposed by the team outperformed the state-of-the-art results, and some developments are compatible with “high-throughput microscopy” and the processing of several hundreds of cells. We especially promoted non local, non-parametric and patch-based methods to solve well-known inverse problems or more original reconstruction problems. A recent research direction consists in adapting the deep learning concept to solve challenging detection and reconstruction problems in microscopy. We have investigated convolution neural networks to detect small macromolecules in 3D noisy electron images with promising results. The next step consists in proposing smart paradigms and architectures to save memory and computations.
More generally, many inverse problems and image processing become intractable with modern 3D microscopy, because very large temporal series of volumes (200 to 1000 images per second for one 3D stack) are acquired for several hours. Novel strategies are needed for 3D image denoising, deconvolution and reconstruction since computation is extremely heavy. Accordingly, we will adapt the estimator aggregation approach developed for optical flow computation to meet the requirements of 3D image processing. We plan to investigate regularization-based aggregation energy over super-voxels to reduce complexity, combined to modern optimization algorithms. Finally, we will design parallelized algorithms that fast process 3D images, perform energy minimization in few seconds per image, and run on low-cost graphics processor boards (GPU).
3.2 From images to motion descriptors and trajectories
Several particle tracking methods for intracellular analysis have been tailored to cope with different types of cellular and subcellular motion down to Brownian single molecule behavior. Many algorithms were carefully evaluated on the particle tracking challenge dataset published in the Nature Methods journal in 2014. Actually, there is no definitive solution to the particle tracking problem, which remains application-dependent in most cases. The work of SERPICO in particle motion analysis is significant in multiple ways, and inserts within a very active international context. One of the remaining key open issues is the tracking of objects with heterogeneous movements in crowded configurations. Moreover, particle tracking methods are not always adapted for motion analysis, especially when the density of moving features hampers the individual extraction of objects of interest undergoing complex motion. Estimating flow fields can be more appropriate to capture the complex dynamics observed in biological sequences. The existing optical flow methods can be classified into two main categories: i/ local methods, imposing a parametric motion model (e.g. local translation) in a given neighborhood; ii/ global methods, estimating the dense motion field by minimizing a global energy functional composed of a data-fidelity term and a regularization term.
The SERPICO team has developed a strong expertise in key topics, especially in object tracking for fluorescence microscopy, optical flow computation and high-level analysis of motion descriptors and trajectories. Several algorithms proposed by the team are very competitive when compared to the state-of-the-art results, and our new paradigms offer promising ways for molecule traffic quantification and analysis. Among the problems that we currently address, we can mention: computation of 3D optical flow for large-size images, combination of two frame-based differential methods and sparse sets of trajectories, detection and analysis of unexpected local motion patterns in global coherent collective motion. Development of efficient numerical schemes will be central in the future but visualization methods are also crucial for evaluation and quality assessment. Another direction of research consists in exploiting deep learning to 3D optical flow so as to develop efficient numerical schemes that naturally capture complex motion patterns. Investigation in machine learning and statistics will be actually conducted in the team in the two first research axes to address a large range of inverse problems in bioimaging. Deep learning is an appealing approach since expertise of biologists, via iterative annotation of training data, will be included in the design of image analysis schemes.
3.3 Biological and biophysical models and statistics for quantitative bioimaging
A number of stochastic mathematical models were proposed to describe various intracellular trafficking, where molecules and proteins are transported to their destinations via free diffusion, subdiffusion and ballistic motion representing movements along the cytoskeleton networks assisted by molecular motors. Accordingly, the study of diffusion and stochastic dynamics has known a growing interest in bio-mathematics, biophysics and cell biology with the popularization of fluorescence dynamical microscopy and super-resolution imaging. In this area, the competing teams mainly studied MSD and fluorescence correlation spectroscopy methods.
In the recent period, the SERPICO team achieved important results for diffusion-related dynamics involved in exocytosis mechanisms. Robustness to noise has been well investigated, but robustness to environmental effects has yet to be effectively achieved. Particular attention has been given to the estimation of particle motion regime changes, but the available results are still limited for analyzing short tracks. The analysis of spatiotemporal molecular interactions from sets of 3D computed trajectories or motion vector fields (e.g., co-alignment) must be investigated to fully quantify specific molecular machineries. We have already made efforts in these directions this year (e.g., for colocalization) but important experiments are required to make our preliminary algorithms reliable enough and well adapted to specific transport mechanisms.
Accordingly, we will study quantification methods to represent interactions between molecules and trafficking around three lines of research. First, we will focus on 3D space-time global and local object-based co-orientation and co-alignment methods, in the line of previous work on colocalization, to quantify interactions between molecular species. In addition, given
4 Application domains
4.1 Modeling and analysis of membrane transport and molecule trafficking at the single cell scale
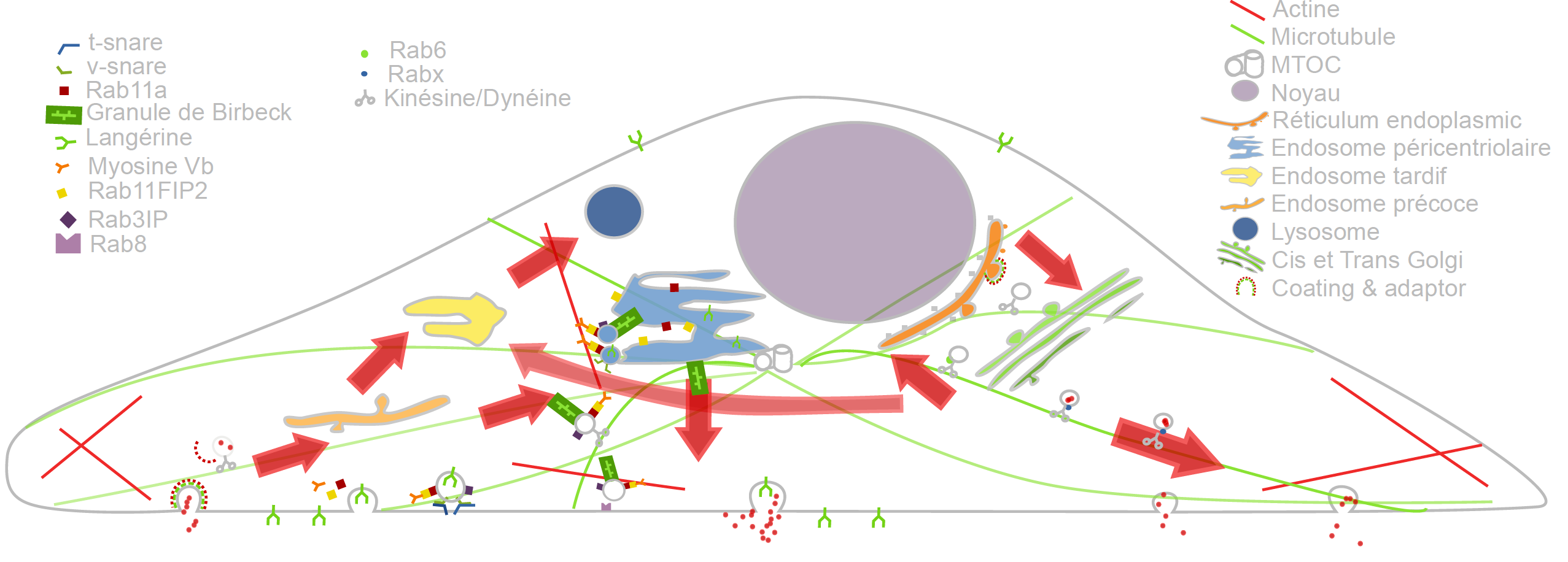
Cargo Langerin Trafficking controlled by Rab11A/Rab11FIP2/MyoVb platform.
In the past recent years, research carried out together with the “Space Time imaging of Endomembranes and organelles Dynamics” team at UMR 144 CNRS - Institut Curie contributed to a better understanding of the intracellular compartmentation, particularly in specialized model cells such as melanocytes and Langerhans cells of the epidermis, of the components and structural events involved in the biogenesis of their specialized organelles: melanosomes and Birbeck granules, respectively and to the understanding on how the dynamics of those structures relate to their physiological functions. These studies have started to highlight: i/ the measurement of multiple sorting and structural events involved in the biogenesis of these organelles; ii/ complexity of the endo-melanosomal network of these highly specialized cells; iii/ complex molecular architecture organizing and coordinating their dynamics; iv/ intracellular transport steps affected in genetic diseases, among which the Hermansky Pudlak syndrome (HPS) or involved in viral infection (HIV and Langerin in Langerhans cells).
In this context, the central aim of SERPICO is to understand how the different machineries of molecular components involved are interconnected and coordinated to generate such specialized structures, an issue that becomes more and more accessible, thanks to improvement in all domains related to live imaging. We need to address the following topics:
- developing new bioimaging approaches to observe and statistically analyze such coordinated dynamics in live material;
- correlating this statistically relevant spatiotemporal organization of protein networks with the biological architectures and at the ultrastructural level;
- modeling intracellular transport of those reference biological complex systems and proposing new experimental plans in an iterative and virtuous circle;
- managing and analyzing the workflow of image data obtained along different multidimensional microscopy modalities.
These studies are essential to unravel the complexity of the endomembrane system, how different machineries evolve together and thus coordinate (e.g. see Fig. 1). They help to decipher cell organization and function at different scales through an integrative workflow of methodological and technological developments. New approaches, such as optogenetics may even help controlling cell functions.
At long term, these studies will shed light on the cellular and molecular mechanisms underlying antigen presentation, viral infection or defense mechanisms, skin pigmentation, the pathogenesis of hereditary genetic disorders (lysosomal diseases, immune disorders) and on the mechanisms underlying cell differentiation and cell transformation. Our methodological goal is also to link dynamics information obtained through diffraction limited light microscopy, at a time regime compatible with live cell imaging and close to biochemical molecular interactions. The overview of ultrastructural organization will be achieved by complementary electron microscopy methods which have also undergone a revolutionary improvement over the last decade. Image visualization and quantitative analysis are of course essential issues in this context.
4.2 Imaging and analysis of cytoskeleton dynamics during cell migration
The ability to migrate in space is among the most fundamental functions of eukaryotic cells and thus is one of the best-studied phenomena in biology. During embryonic development, cell movements result in a massive reorganization of the embryo, from a simple spherical ball of cells into a multi-layered organism; many of the cells at or near the surface of the embryo move to a new, more interior location. Moreover, inadequate or inappropriate migration of immune cells is also critically important for the delivery of protective immune responses to tissues and for wound healing. Finally, cell migration may facilitate the dissemination of tumor cells from primary tumors in blood (extravasation) and eventually the colonization of other organs and the formation of secondary tumors.
It has been established that the cytoskeleton, composed of actin filaments, microtubules and intermediate filaments (elongated structures with a diameter of a few dozens of nanometers), is essential for several cell mechanisms, including cell migration, cell division and molecule trafficking:
- i/ the actin filaments promote cell protrusion, adhesion and retraction;
- ii/ the microtubules are the support of molecule traffic and cell polarization;
- iii/ the intermediate filaments are hypothesized to control microtubule organization.
Nevertheless, the mechanical and chemical states of migrating cells under various external conditions remain largely unknown. In the last decade, high-resolution microscopy methods led to the discovery of novel aspects of cell migration. Most approaches and models are limited to migration in 2D, justified by the flatness of the cell-motile mechanisms. However, the mechanical patterns that govern migration in 2D models are often not essential for efficient migration in 3D. Accordingly, recent very challenging 3D models of cells moving on flat surfaces have begun to emerge. The key challenge, however, is to understand how a 3D motile cell crawls through the 3D extracellular matrix. Another issue is of course to measure and understand how membrane protrusion and retraction keep the cell in homeostasis, which of course relate to membrane traffic.
The objective of SERPICO is to develop high-end signal processing and computer vision tools to unfold the dynamical coordination of microtubules, actin filaments and intermediate filaments in 3D, involved in cell migration, cell division and how molecular trafficking is coordinated with cytoskeleton changes in these fundamental cellular functions.
5 Social and environmental responsibility
Application of general recommendations related to transport (train, visioconferences). All conferences were conducted in virtual mode in 2021 because of the pandemic crisis. This has led to a significant reduction of the number of travels, and then a small carbon footprint for the team in 2021.
6 Highlights of the year
- Yunjiao Lu successfully defended her PhD thesis on June 2021.
- Anaïs Badoual has been recruited as CRCN permanent research scientist in 2021.
- The paper entitled "Deep Learning Improves Macromolecule Identification in 3D Cellular Cryo-Electron Tomograms" has been published in Nature Methods journal in October 2021.
7 New software and platforms
7.1 New software
7.1.1 GcoPS
-
Name:
Geo-Co-Positioning System for co-localization of image pairs of fluorescent molecules
-
Keywords:
Photonic imaging, Fluorescence microscopy, Image processing, Statistic analysis
-
Functional Description:
The GcoPS (Geo-Co-Positioning System) software is dedicated to the co-localization of fluorescence image pairs for both conventional and super-resolution microscopy. The procedure is only controlled by a p-value and tests whether the Pearson correlation between two binary images is significantly positive. Colocalization amounts here to quantifying the interaction strength by the area/volume of the intersection between the two binary images viewed as random distributions of geometrical objects. Under mild assumptions, it turns out that the appropriately normalized Pearson correlation follows a standard normal distribution under the null hypothesis if the number of image pixels is large. Unlike previous methods, GcoPS handles 2D and 3D images, variable SNRs and any kind of cell shapes. It is able to co-localize large regions with small dots, as it is the case in TIRF-PALM experiments and to detect negative co-localization. The typical processing time is two milliseconds per image pair in 2D and a few seconds in 3D, with no dependence on the number of objects per image. In addition, the method provides maps to geo-co-localize molecule interactions in specific image regions.
- URL:
- Publication:
-
Contact:
Charles Kervrann
-
Participants:
Thierry Pécot, Frédéric Lavancier, Charles Kervrann, Liu Zengzhen
-
Partners:
Université de Nantes, UMR 144 CNRS - Institut Curie, Hollings Cancer Center at the Medical University of South Carolina, Charleston SC, USA
7.1.2 AiryscanJ
-
Name:
Reconstruction fluorescence microscopy images from confocal array detector
-
Keywords:
Image reconstruction, Fluorescence microscopy, Photonic imaging, Deconvolution, Image analysis
-
Functional Description:
The AiryscanJ software enables to reconstruct a high resolution image from an array of multiple raw images acquired with the Airyscan technology (32 detectors). Airyscanning is a recent technique based on confocal laser scanning microscopy. The AiryscanJ software gathers four reconstruction methods (ISM, IFED, ISFED, ISM-Deconvolution) to compute a high resolution image: 1/ ISM amounts to summing the preliminarily registered raw images. 2/ IFED is the weighted difference between the inner detectors and the outer detector. 3/ ISFED is the weighted difference between the registered outer detectors and the original outer detectors. AiryscanJ automatically estimates the parameter controlling the IFED and ISFED algorithms. 4/ ISM-Deconvolution allows reconstructing a high resolution image by applying a deconvolution algorithm on the ISM image.
- URL:
-
Contact:
Charles Kervrann
-
Participants:
Sylvain Prigent, Stephanie Dutertre, Charles Kervrann
-
Partners:
Université de Rennes 1, CNRS
7.1.3 DeepFinder
-
Name:
Deep learning for macromolecule identification within 3D cellular cryo-electron tomograms
-
Keywords:
Image analysis, Deep learning, Cryo-electron microscopy, Object detection
-
Functional Description:
DeepFinder is a computational approach that uses artificial neural networks to accurately and jointly localize multiple types and/or states of macromolecules in 3D cellular cryo-electron tomograms. DeepFinder leverages deep learning and outperforms the commonly-used template matching method on ideal data. On synthetic image data (SHREC 2019 challenge), DeepFinder is very fast and produces superior detection results when compared to other competitive deep learning methods, especially on small macromolecules. On experimental cryo-ET data depicting ribosomes, the detection results obtained by DeepFinder are consistent with expert annotations. We have got a high overlap of detection (86%) and a similar structure resolution that those determined by subtomogram averaging.
- URL:
-
Contact:
Charles Kervrann
-
Participants:
Emmanuel Moebel, Charles Kervrann
-
Partners:
Max Planck Institute Martinsried, Fondation Fourmentin-Guilbert, Helmholtz Pioneer Campus, Neuherberg, Germany
7.1.4 FlowScope
-
Name:
Optical flow computation for 3D fluorescence microscopy
-
Keywords:
Motion analysis, Fluorescence microscopy, Image analysis, Data visualization
-
Functional Description:
The FLOWSCOPE software is able to estimate 3D motion between two fluorescence microscopy volumes. The underlying variational method amounts to minimizing an energy functional made up of two terms: a data term and a regularization term. The data term is derived from the continuous form of Census signature and the smoothness of the flow field is imposed by a L2 regularization term. The method is implemented for a single core CPU. FLOWSCOPE outputs three separate files corresponding to the motion vector components. The flow fields can be visualized with an appropriate color code named 3PHS. The 3PHS map projects the flow field onto the three orthogonal planes selected by the user. The projections of the vector field is color coded in the Hue (direction) and the Saturation (amplitude) spaces.
- URL:
- Publication:
-
Contact:
Patrick Bouthemy
-
Participants:
Sandeep Manandhar, Patrick Bouthemy, Sylvain Prigent, Leo Maury, Philippe Roudot, Charles Kervrann
-
Partners:
UMR 144 CNRS - Institut Curie, Danuser lab, University of Texas Southwestern, Dallas, USA
7.1.5 DCT2Net
-
Name:
Trained shallow CNN (convolution neural network)-based DCT (Discrete Cosine Transform) denoiser
-
Keywords:
Deep learning, Denoising
-
Functional Description:
DCT2net software, based on the well-known DCT (Discrete Cosine Transform) image denoising algorithm, is dedicated to noise removal from images. The traditional DCT denoiser can be seen as a shallow CNN and thereby its original linear transform can be tuned through gradient descent in a supervised manner, improving considerably its performance. Consequently, DCT2net is a shallow and interpretable convolution network, whose parameters optimization allows to improve very significantly the performances of the traditional DCT denoiser. To deal with the remaining artifacts induced by DCT2net, an original hybrid solution between DCT and DCT2net is proposed, combining the best of what these two methods can offer. Experiments on artificially noisy images show that the two-layer DCT2net method provides results comparable to the BM3D method and is as fast as the DnCNN algorithm composed of more than a dozen of layers.
- URL:
- Publication:
-
Authors:
Sebastien Herbreteau, Charles Kervrann
-
Contact:
Charles Kervrann
7.1.6 SPITFIRE
-
Name:
SParse fIT for Fluorescence Image Restoration
-
Keywords:
Denoising, Fluorescence microscopy
-
Functional Description:
SPITFIR(e) (SParse fIT for Fluorescence Image Restoration) is a very flexible software designed to restore 2D-3D+Time fluorescent images and subtract undesirable out-of-focus background. We assume that the images are sparse and piece-wise smooth, and are corrupted by mixed Poisson-Gaussian noise. The principle resides in the minimization of a convex energy functional that includes a fidelity-to-data term and a Sparse-Hessian Variation regularization term. A fast primal-dual optimization algorithm allows to restore very large images in a few seconds. SPITFIR(e) is nearly parameter-free as the practitioner needs only to specify the amount of desired sparsity (weak, moderate, high). Experimental results in lattice light sheet, stimulated emission depletion, multifocus microscopy, spinning disk confocal, and wide-field microscopy demonstrate the generic ability of the SPITFIR(e) algorithm to efficiently reduce noise and blur, and to subtract undesirable fluorescent background, while avoiding the emergence of deconvolution artifacts.
- URL:
- Publication:
-
Contact:
Charles Kervrann
-
Participants:
Sylvain Prigent, Hoai Nam Nguyen, Ludovic Leconte, Cesar Augusto Valades Cruz, Jean Salamero, Charles Kervrann
-
Partner:
UMR 144 CNRS - Institut Curie
7.1.7 DenseMapping
-
Name:
Dense mapping of diffusion and drift of moving particules
-
Keywords:
Microscopy, Cellular imaging
-
Functional Description:
It is of primary interest for biologists to be able to visualize the dynamics of proteins within the cell. DenseMapping software allows to estimate the diffusion and drift parameters attached to moving biomolecules within cells from 2D/3D individual trajectories. In a first step, each particle track is labeled into three motion categories: confined motion (subdiffusion), Brownian motion (free diffusion), and directed motion (superdiffusion). The long trajectories are also segmented into sub-trajectories according to these three categories. In a second step, two 2D/3D maps (diffusion, drift) are computed from a local analysis of the trajectories. The local spatio-temporal kernel estimators correspond to weighted averages of the trajectory elements. The weights allow to select and aggregate the information in an optimal way.
- URL:
- Publications:
-
Contact:
Charles Kervrann
-
Participants:
Antoine Salomon, Vincent Briane, Cesar Augusto Valades Cruz, Charles Kervrann
-
Partner:
UMR 144 CNRS - Institut Curie
7.2 New platforms
7.2.1 BioimageIT: Open-source framework for integration of image data-management with analysis
Participants: Sylvain Prigent, Cesar Augusto Valades-Cruz, Ludovic Leconte, Léo Maury, Jean Salamero, Charles Kervrann.
-
Func
tional Description: New image acquisition systems generate large numbers of images and large volume images. Such data sets are hard to store, to process and to analyze for one user in a workstation. Many solutions exist for data management (e.g. Omero, OpenImadis), image analysis (e.g., Fiji, Icy, CellProfiler) and statistics (e.g., R). Each of them has its specificities and several bridges have been developed between pieces of software. Nevertheless, in many use-cases, the users need to perform analysis using tools that are available in different pieces of software and different languages. The design of a workflow that brings the data from one tool to another, is a tedious task that may require specific programming skills. Moreover, a dedicated script using a dedicated file system for processed data management is usually developed. To overcome these difficulties, we have developed BioImageIT, an integration platform to facilitate data management and analysis by integrating existing software. In 2021, we created a new desktop application for BioImageIT that integrates all the original BioImageIT functionalities in a single windows application. In this new graphical interface (Fig. 2), scientists can manage their data, design analysis pipelines and run batch processing without coding. Furthermore, we integrated the napari viewer in BioImageIT to interact with multi-dimensional scientific data.
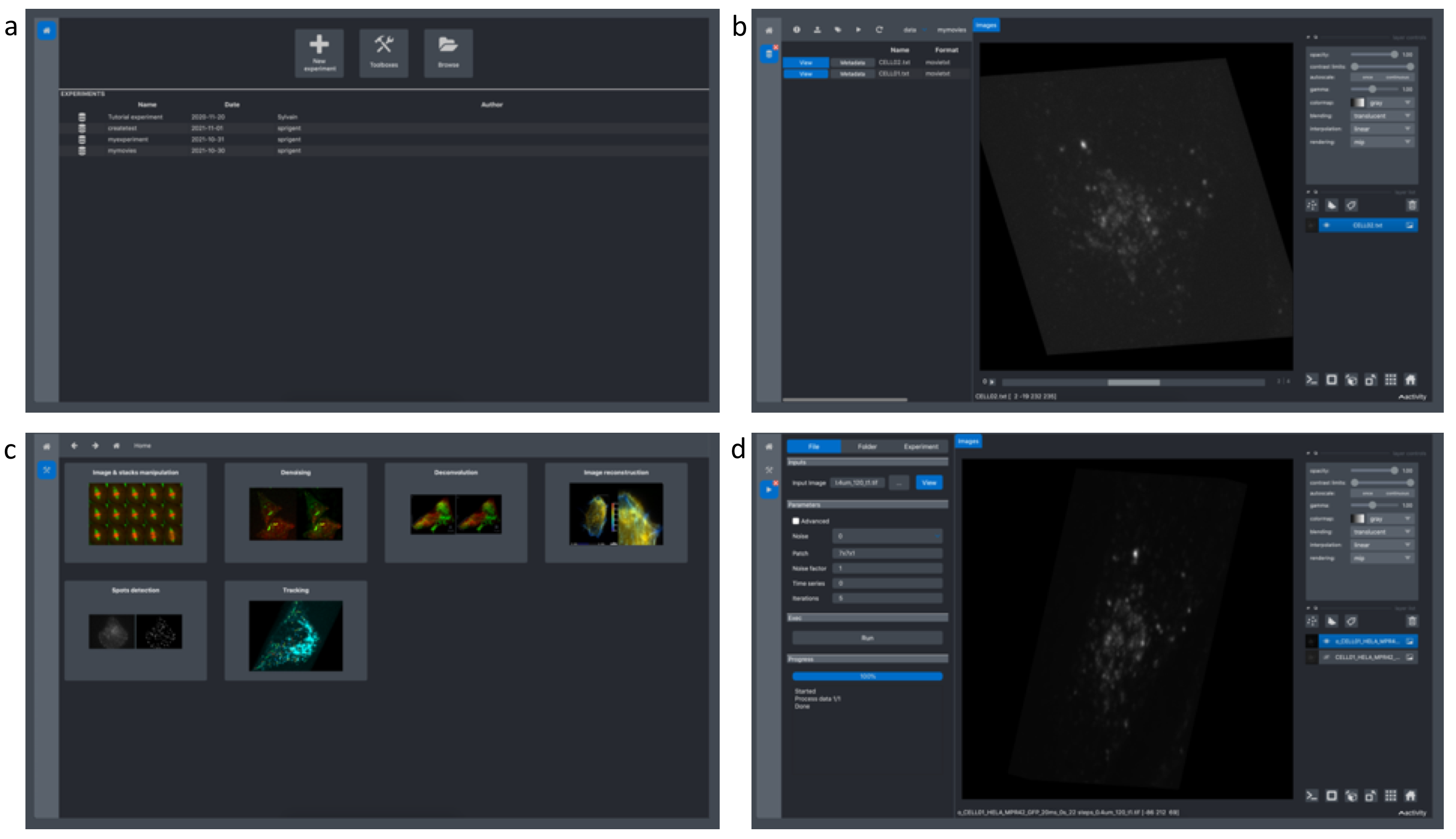
Screen shots of the new single window interface of BioImageIT.a, The first screen allows to select a tool (data management, data analysis, open an existing project). b, The second screen is the data management area to annotate data and visualize data with napari. c, The third screen is the processing toolboxes finder. d, The fourth screen is the processing interface with interactive parameters selection thanks to the integrated viewer, and the batch processing functionality.
Software: BioImageIT interface
Partners: UMR 144 CNRS - Institut, France-BioImaging.
7.2.2 Development of napari plugin for bioimage analysis
Participant: Sylvain Prigent.
-
Func
tional Description:napari is a fast, interactive, multi-dimensional image viewer for Python, especially designed for browsing, annotating, and analyzing large multi-dimensional images. It is built on top of Qt (for the GUI), vispy (for performant GPU-based rendering), and the scientific Python stack (numpy, scipy). We developed the three following napari plugins from previous SERPICO pieces of software:-
–
napari-sdeconv: napari plugin for 2D and 3D image deconvolution.
-
–
napari-stracking: napari plugin for spot detection and tracking in 2D+time and 3D+time images.
-
–
napari-tracks-reader: napari plugin to import in the napari tracks layer the trajectories stored in various file formats (CSV, ICY, TrackMate).
-
–
8 New results
8.1 Methods and algorithms for image restoration and reconstruction
8.1.1 Sparsity promoting regularizer for restoring 2D-3D fluorescence images and videos and background subtraction
Participants: Sylvain Pigent, Ludovic Leconte, Cesar Augusto Valades-Cruz, Jean Salamero, Charles Kervrann.
While fluorescent microscopy imaging has become the spearhead of modern biology as it is able to generate long-term videos depicting 4D nanoscale cell behaviors, it is still limited by the optical aberrations and the photon budget available in the specimen and to some extent to photo-toxicity. A direct consequence is the necessity to develop flexible and "off-road" algorithms in order to recover structural details and improve spatial resolution, which is critical when pushing the illumination to the low levels in order to limit photo-damages. Moreover, as the processing of very large temporal series of images considerably slows down the analysis, special attention must be paid to the feasibility and scalability of the developed restoration algorithms. To address these specifications, we present a very flexible method designed to restore 2D-3D+Time fluorescent images and subtract undesirable out-of-focus background. We assume that the images are sparse and piecewise smooth, and are corrupted by mixed Poisson-Gaussian noise. To recover the unknown image, we consider a novel convex and non-quadratic regularizer (Sparse Hessian Variation) defined as the mixed norms which gathers image intensity and spatial second-order derivatives. The resulting restoration algorithm named SPITFIR(e) (SParse fIT for Fluorescence Image Restoration) utilizes the primal-dual optimization principle for energy minimization and can be used to process large images acquired with varied fluorescence microscopy modalities. It is nearly parameter-free as the practitioner needs only to specify the amount of desired sparsity (weak, moderate, high). Experimental results in lattice light sheet, stimulated emission depletion, multifocus microscopy, spinning disk confocal, and wide-field microscopy demonstrate the generic ability of the SPITFIR(e) algorithm to efficiently reduce noise and blur, and to subtract undesirable fluorescent background, while avoiding the emergence of deconvolution artifacts.
Reference: 34
Software: SPITFIRE
Collaborator: B. Hajj (UMR 168 CNRS - Institut Curie)
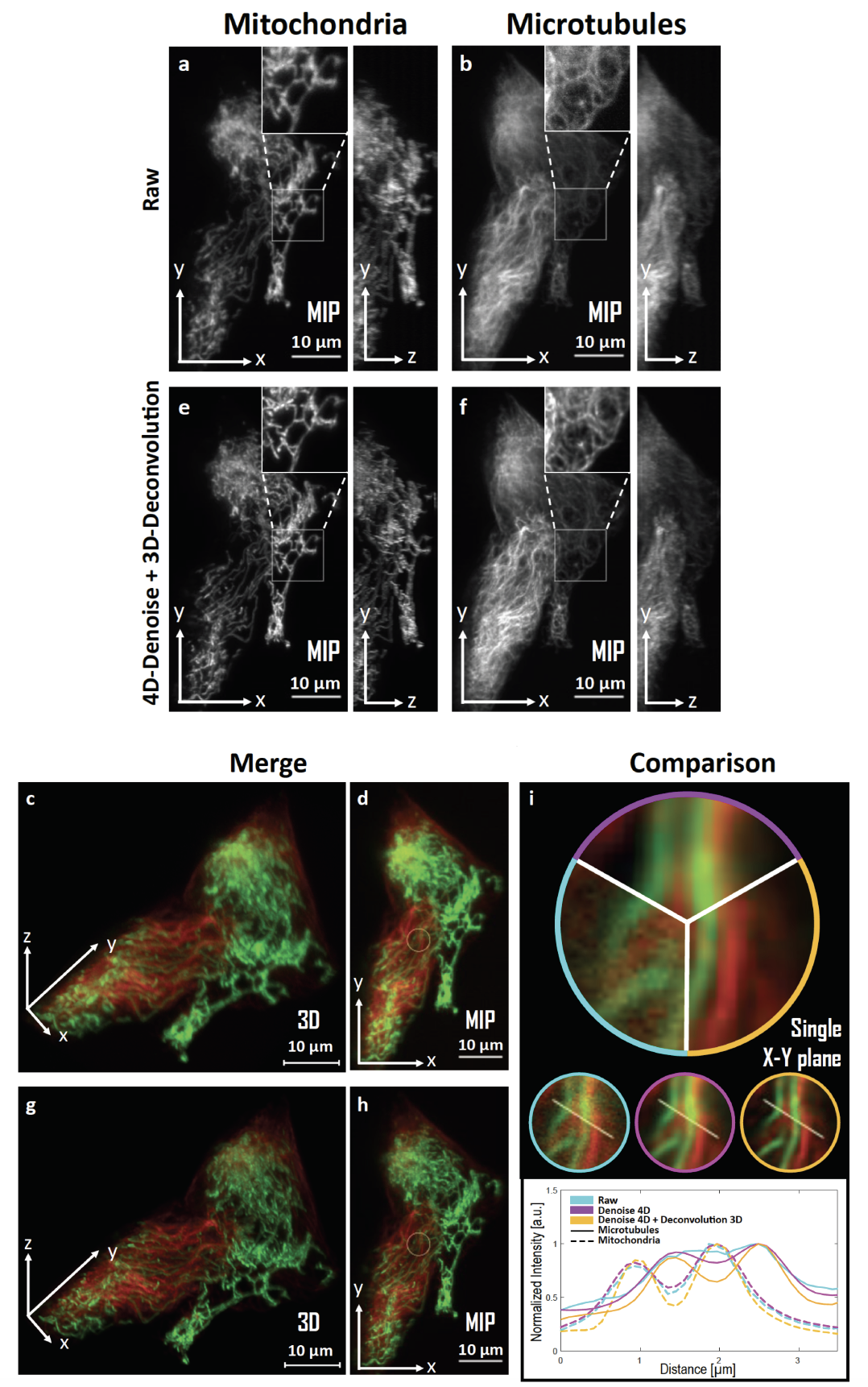
SPITFIR(e) for 4D-denoising, background subtraction, and 3D-deconvolution on LLSM images. 60 planes 3D volumes of live RPE1 cells double stained with PKMR for Mitochondria (a,e) and with Tubulin TrackerTM^{TM} Deep Red for Microtubules (b,f) were acquired within 2.2s2.2s per stack using lattice light-sheet microscopy. MIP of representative raw (see Material and Methods section) images for Mitochondria and Microtubules, respectively, before (a,b) and after (e, f) SPITFIR(e) 4D denoising and 3D deconvolution (3D Gaussian PSF, σxy=1.5\sigma _{xy} =1.5 pixels and σz=1.0\sigma _{z} =1.0 pixel). Insets are zoomed area illustrating SPITFIR(e) improvement in spatial resolution and SNR. 3D angular views and MIP of composite images before (c,d) and after (g,h) SPITFIR(e) treatment (Red for Microtubules; Green for Mitochondria). 4D denoising SPITFIR(e) image improvement shown on a single x-y plane (i) zoomed from the inset indicated in (d, h). Raw image is indicated as a blue lined sector in the upper part and in the left circle, beneath; 4D denoised image is similarly indicated in magenta, while the 4D denoised + 3D deconvolved image is in yellow. Intensity line profiles were measured as indicated in the 3 circles and plotted for each processing step and both Mitochondria and Microtubules at the lower part of (i).
8.1.2 DCT2net: an interpretable shallow CNN for image denoising
Participants: Sébastien Herbreteau, Charles Kervrann.
This work tackles the issue of noise removal from images, focusing on the well-known DCT image denoising algorithm. The latter, stemming from signal processing, has been well studied over the years. Though very simple, it is still used in crucial parts of state-of-the-art "traditional" denoising algorithms such as BM3D. Since a few years however, deep convolutional neural networks (CNN) have outperformed their traditional counterparts, making signal processing methods less attractive. In this paper, we demonstrate that a DCT denoiser can be seen as a shallow CNN and thereby its original linear transform can be tuned through gradient descent in a supervised manner, considerably improving its performance. This gives birth to a fully interpretable CNN called DCT2net. To deal with remaining artifacts induced by DCT2net, an original hybrid solution between DCT and DCT2net is proposed combining the best that these two methods can offer; DCT2net is selected to process non-stationary image patches while DCT is optimal for piecewise smooth patches. Experiments on artificially noisy images demonstrate that two-layer DCT2net provides comparable results to BM3D 41 and is as fast as DnCNN algorithm 46 composed of more than a dozen of layers.
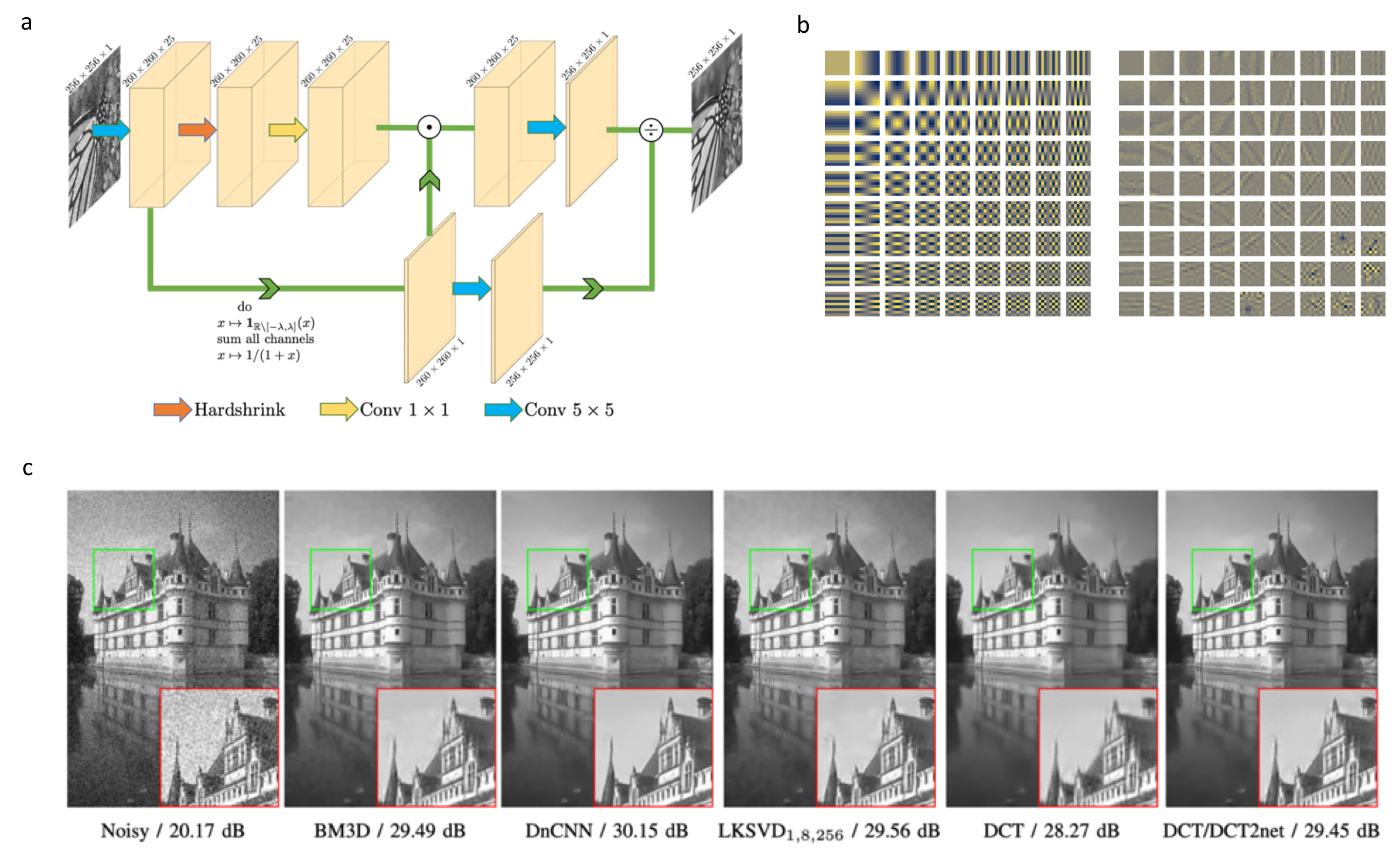
Description DCT2Net method.a, Architecture of DCT2net for a patch size p=5. b, Original DCT basis (left) vs DCT2net learned basis (right). c, Denoising results (with PSNR values) of Castle image from BSD68 dataset corrupted by white Gaussian noise and noise standard deviation σ=25\sigma = 25.
Reference: 30
Software:DCT2Net (see Section 7.1.5)
Collaborator: R. Fraisse (AIRBUS Defense and Space SAS)
8.1.3 Deep learning for image denoising and deconvolution
Participants: Sylvain Prigent, Sébastien Herbreteau, Charles Kervrann.
Image denoising and deconvolution is an ill-posed inverse problem. Many variational models have been proposed to perform deconvolution by introducing priors on the image like smoothness or sparsity 34. In recent years, deep learning methods have shown impressive results in image restoration. Nevertheless, a serious drawback of the deep learning methods is the need to build a representative training dataset of the target application. This is not always possible in the life science imaging field where datasets are not extendible and each experiment performed with different samples and conditions. Accordingly, we studied the limitations of deep network architectures like DnCNN 46 and UNet 45 for image denoising and deconvolution, and we compared the performance to variational approaches. To perform fair comparisons, we implemented the SPITFIR(e) 34 deconvolution algorithm with GPU. From our experimental results, the CNN-based algorithms trained for a target dataset (image contents and noise) clearly outperform variational approaches. Nevertheless, when the test images deviate from the training set, the performance of deep learning-based restoration methods drops down significantly while the variational methods produce stable restoration results.
Software:
-
–
SimgLib: CPU parallel and GPU CUDA implementation of deconvolution algorithms for 2D and 3D images (Richardson-Lucy, Wiener, SPITFIR(e)).
-
–
napari-sdeconv: napari plugin for 2D and 3D image deconvolution.
-
–
sdeep: Python library based on PyTorch that implements image denoising and deconvolution deep neural networks.
Collaborator: R. Fraisse (AIRBUS Defense and Space SAS)
8.1.4 Anomaly detection for residual noise and blur analysis
Participants: Sylvain Prigent, Charles Kervrann.
Denoising and deconvolution results are still difficult to evaluate on real images. In the literature, global metrics like PSNR, MSE or SSIM are often considered as gold standard metrics. Nevertheless, the reconstructed images may contain local artifacts or blurring effects while the global evaluation metrics are still very high. In this study, we investigated several statistical anomaly detection methods to generate restoration quality assessment maps from residual images defined as the difference between the restored (i.e., deblurred and/or denoised) images and ground-truth images. In the absence of anomaly, this map is expected to display a homogeneous noise with no organized structure; a local region that deviates from noise is as an anomaly region. We adapted the following algorithms to localize and to delineate regions with local blur and/or local structural noise: statistical parametric mapping, a-contrario model, Atlas 1, HotSpot 42 (Fig. 5). In future work, we plan to embed these restoration quality assessment maps in loss functions to guide the training of CNN architectures.
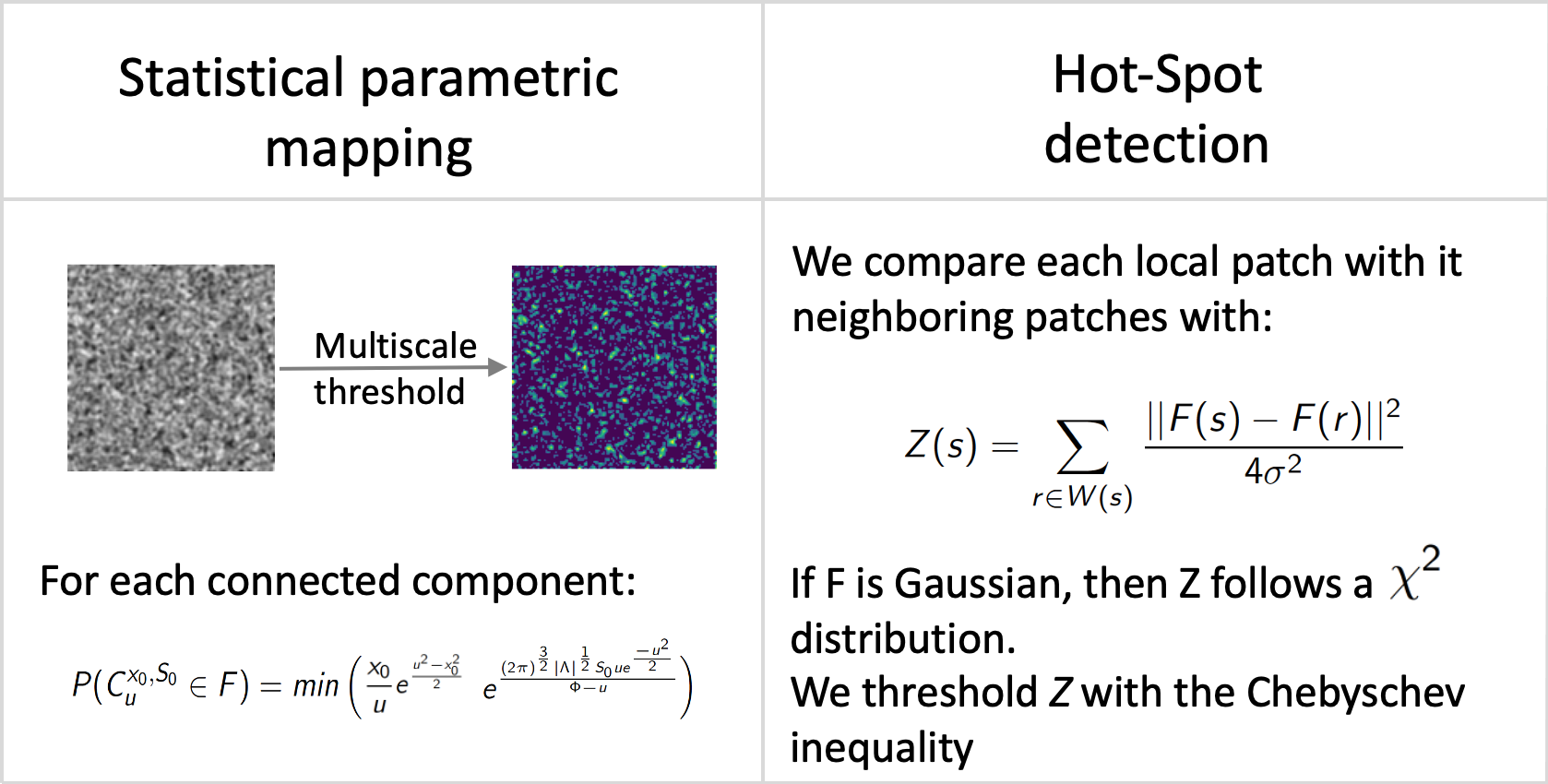
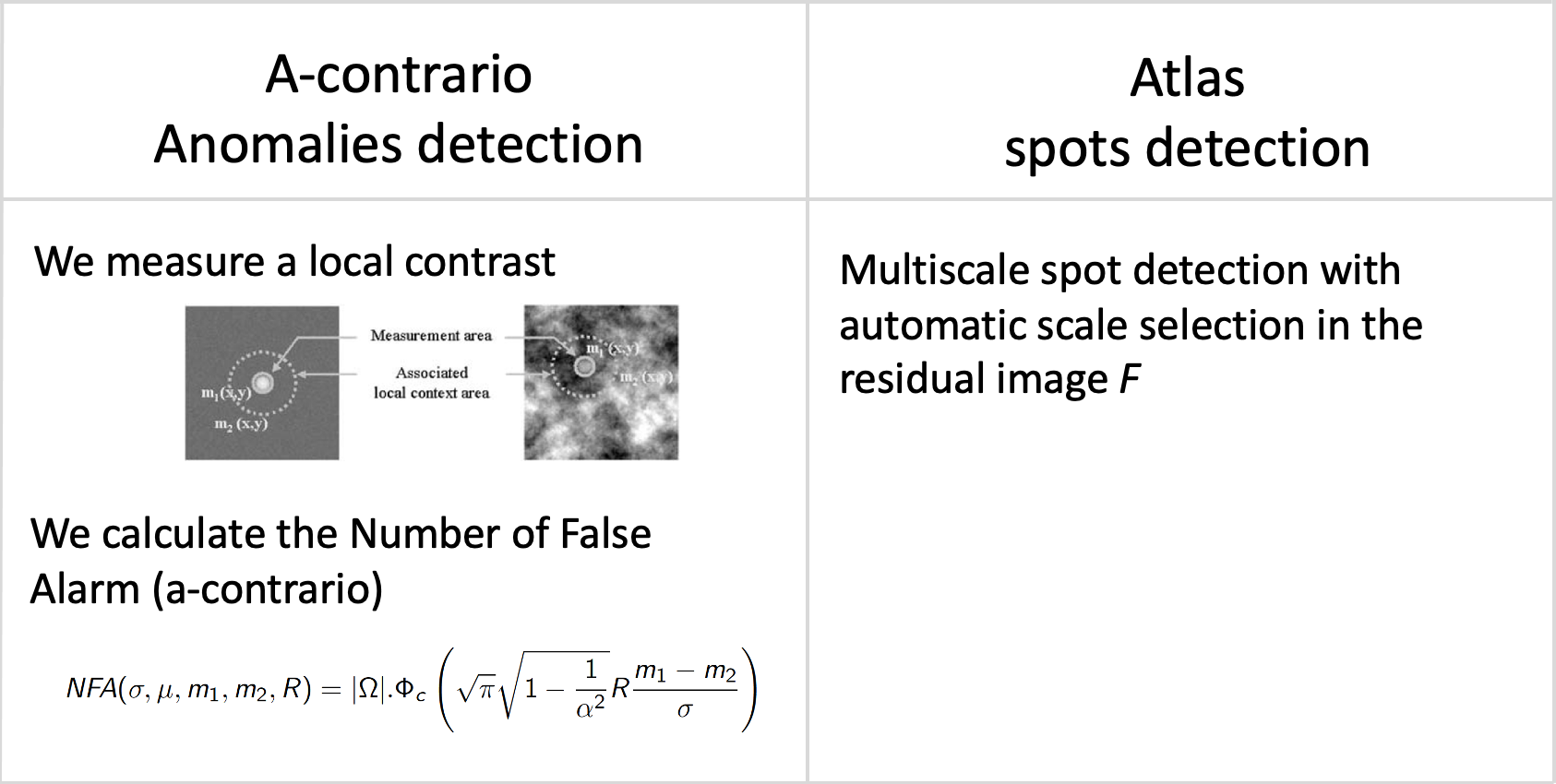
Statistical anomaly detection methods applied to residual images.: Statistical anomaly detection methods applied to residual images.
Software: smetrics (Python library to evaluate quality metrics (e.g., MSE, PSNR, Fourier Ring Correlation, Statistical Parametric Mapping, a-contrario model) for image restoration), Atlas 1, HotSpot 42
Collaborator: R. Fraisse (AIRBUS Defense and Space SAS)
8.2 Methods and algorithms for object segmentation and identification in images
8.2.1 Deep learning for detecting chromosomal aberrations in light (GIEMSA) images
Participants: Antonin Deschemps, Charles Kervrann.
Modern deep learning has shown promising results in medical and biological imaging, mainly for classification and detection tasks. Our goal is to achieve similar results within the context of chromosomal aberration detection in metaphases for biological dosimetry, where the proportion of unhealthy chromosomes (chromosomal aberrations) is directly linked to the dose of ionizing radiation received by the patient. So far, counting these chromosomal aberrations is performed by trained humans, but this manual analysis needs to be rapidly automated to handle large-scale datasets. Here, object counting is generally based on the detection of the objects of interest and the classification into categories (e.g., healthy chromosome, dicentric chromosome, fragment of chromosome ...). U-Net and R-CNN architectures, trained with small datasets, have both shown promising results for chromosome localization in metaphase images. The classification of chromosomes is more challenging. The visual aspect of chromosomes can showcase extreme variations, depending on measurement factors: image capture time with regard to progress within the cell division process, how much GIEMSA coloration "stuck" to the chromosomes during cell division, lighting unevenness depending on the metaphase location on the glass sheet. This visual aspect also depends on biological factors, like amount of irradiation or random folding of chromosomes. It is frequent that objects of different categories may be visually very close to indistinguishable. Furthermore, this problem is statistically "unbalanced": chromosomal aberrations are outnumbered by healthy chromosomes, by a ratio that depends on the irradiation dose. This means that usual resampling strategies may bias the classifier towards a specific dose scenario. Meanwhile, we experimented with a direct counting approach, by predicting a map of chromosome presence in the metaphase image. Again, our unbalanced training dataset is still a problem: while it is easy to count all chromosomes, it is very difficult to count only the chromosomal aberrations within the image. This may be improved by a larger dataset, or more sophisticated training criterions. The on-going work consists in developing an appropriate classification approach to address those unsolved issues.
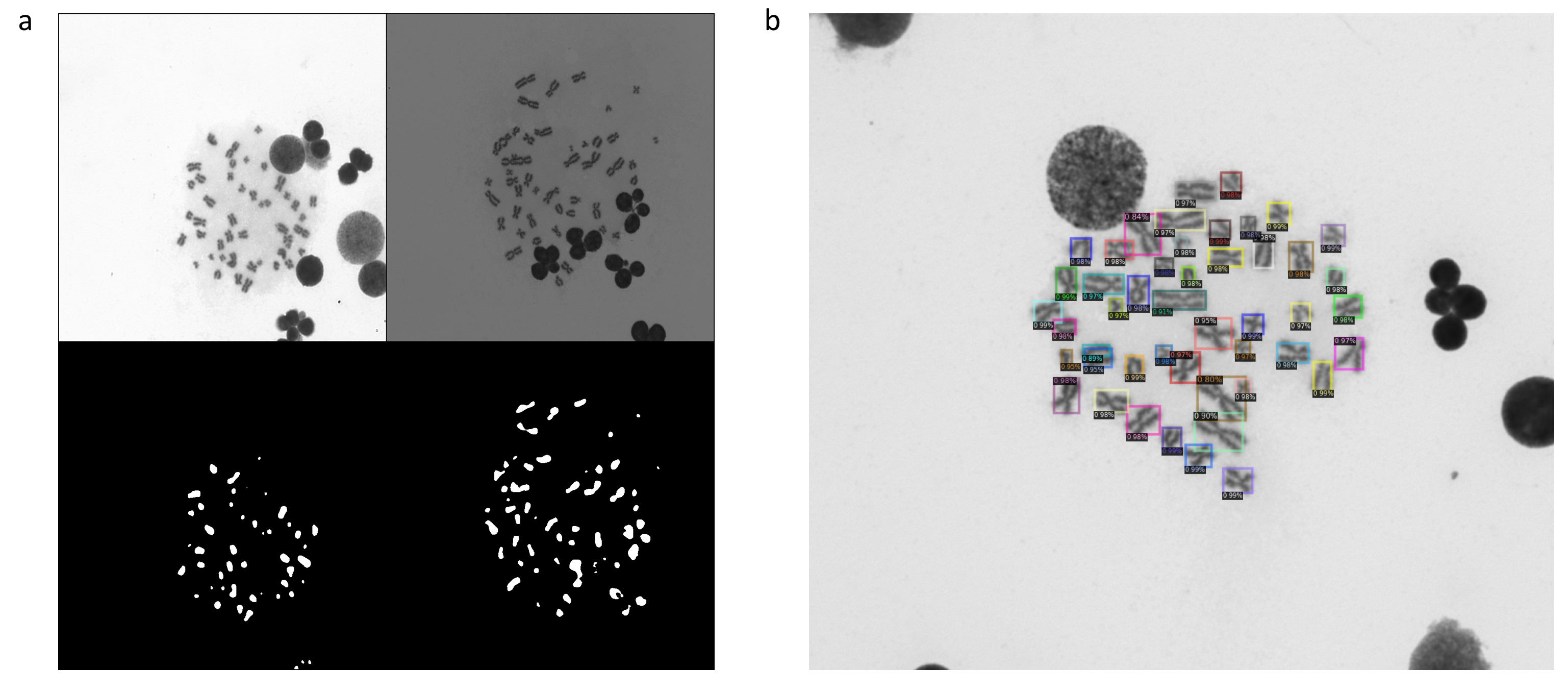
Localization of chromosomes in GIEMSA images.a, A pair of GIEMSA images and the segmentation maps by U-Net. b, The rectangles correspond to the chromosome locations predicted by Faster R-CNN. Each score is a class confidence metric.
Collaborator: Mohamed Amine Benadjaoud (IRSN/LRACC, Fontenay-aux-Roses)
8.2.2 Deep learning for automatic localization and classification of gene translocations in fluorescence (FISH) microscopy images
Participants: Emmanuel Moebel, Quentin Tallon, Charles Kervrann.
Fluorescence in situ hybridization (FISH) allows the detection of specific DNA sequences on chromosomes. In the context of biological dosimetry, this provides observation of translocation aberrations on metaphase chromosomes. Our goal is to automatically detect these aberrations by using deep learning methods (Fig. 7(a)). Here the difficulties of the problem come from the lack of annotation of the data, which limits the performance of supervised machine learning methods. Moreover, the "translocation" class is under-represented (rare event). The challenge is then to develop a method that can take advantage of both the lack of annotations available and the vast amount of unannotated data.
To address this issue, we first evaluated several existing deep learning algorithms such as Faster-RCNN, Mask-RCNN, YOLO, STARDIST.... Then, two concurrent strategies have been explored in parallel. One strategy currently consists in adding to STARDIST self-supervised modules inspired from auto-encoders, GANs, deep-cluster etc. The second strategy aims at creating an unsupervised pipeline for a color attention mechanism on fluorescent chromosomes (Fig. 7(b-c)). In this latter one, clustering algorithms are required for color quantization on relevant channels (red and green) of the image. An outlier detection procedure is also applied in order to improve color separation. The final output of this pipeline is a “color mask” obtained for each image, the main element of our attention mechanism. Cell debris and other non-chromosomal elements are previously filtered thanks to U-Net architectures trained on blue channel segmentation of chromosomes. The color attention is then applied to a custom-modified Mask-RCNN architecture for classification, bounding box regression and instance segmentation of the translocations. Our architecture, with and without color attention (Fig. 7(c)), has shown very promising results on small datasets.
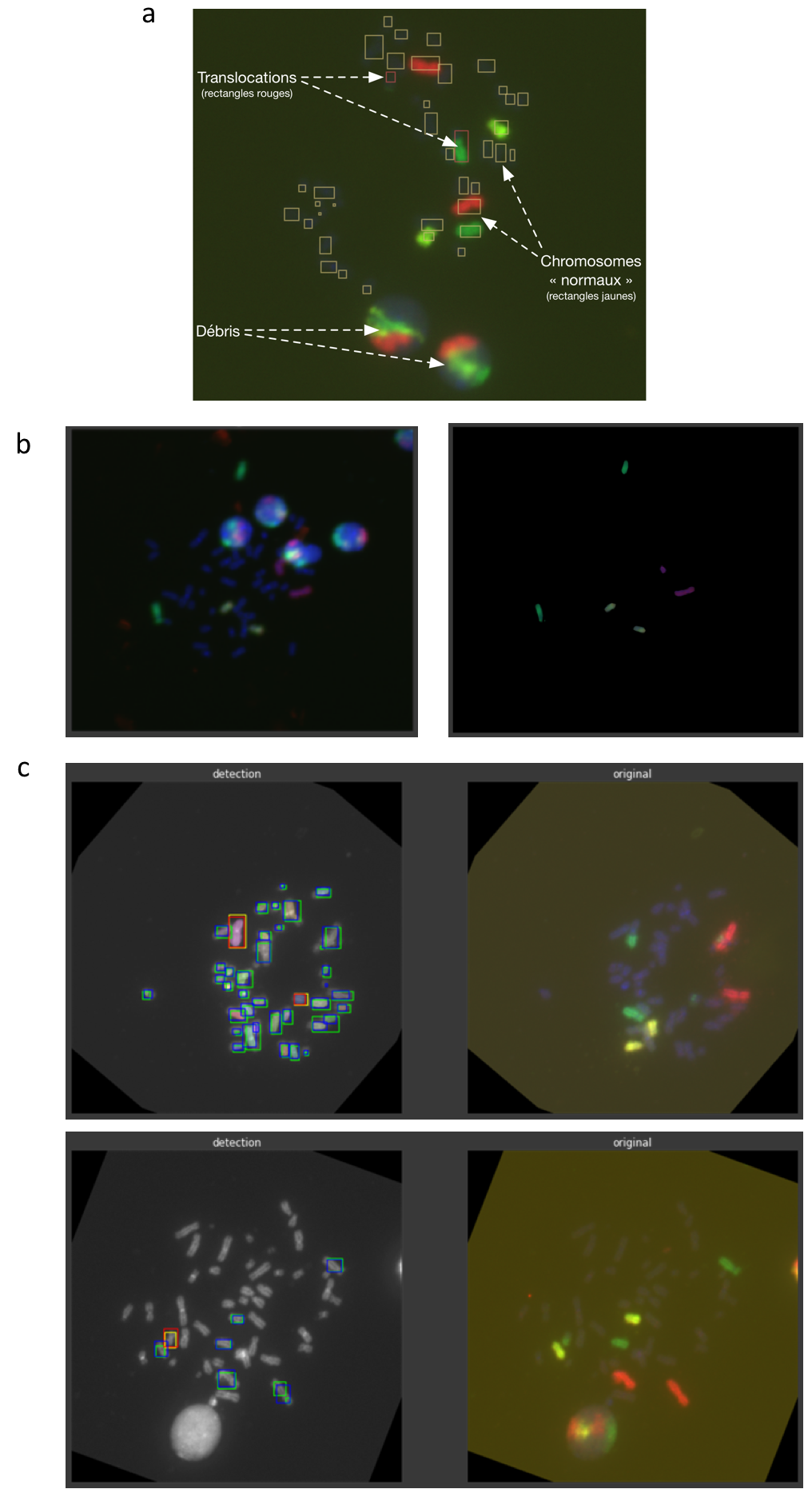
Translocation detection in FISH images.a, FISH image depicting chromosomes. The yellow rectangles indicate the location of "normal" chromosomes. The red rectangles correspond to the location of translocated chromosomes. b, Attention mechanism color mask generation: (left) original FISH metaphase (right) the “color mask” output. c, Output of custom Mask-RCNN for translocation detection, without (top) and with (bottom) color attention mechanism.
Collaborator: Mohamed Amine Benadjaoud (IRSN/LRACC, Fontenay-aux-Roses)
8.2.3 Deep learning for macromolecule identification in 3D cellular cryo-electron tomograms
Participants: Emmanuel Moebel, Charles Kervrann.
Cryogenic electron tomography (cryo-ET) visualizes the 3D spatial distribution of macromolecules at nanometer resolution inside native cells. However, automated identification of macromolecules inside cellular tomograms is challenged by noise and reconstruction artifacts, as well as the presence of many molecular species in the crowded volumes. We present DeepFinder, a computational procedure that uses artificial neural networks to simultaneously localize multiple classes of macromolecules. Once trained, the inference stage of DeepFinder is faster than template matching and performs better than other competitive deep learning methods at identifying macromolecules of various sizes in both synthetic and experimental datasets. On cellular cryo-ET data, DeepFinder localized membrane-bound and cytosolic ribosomes (
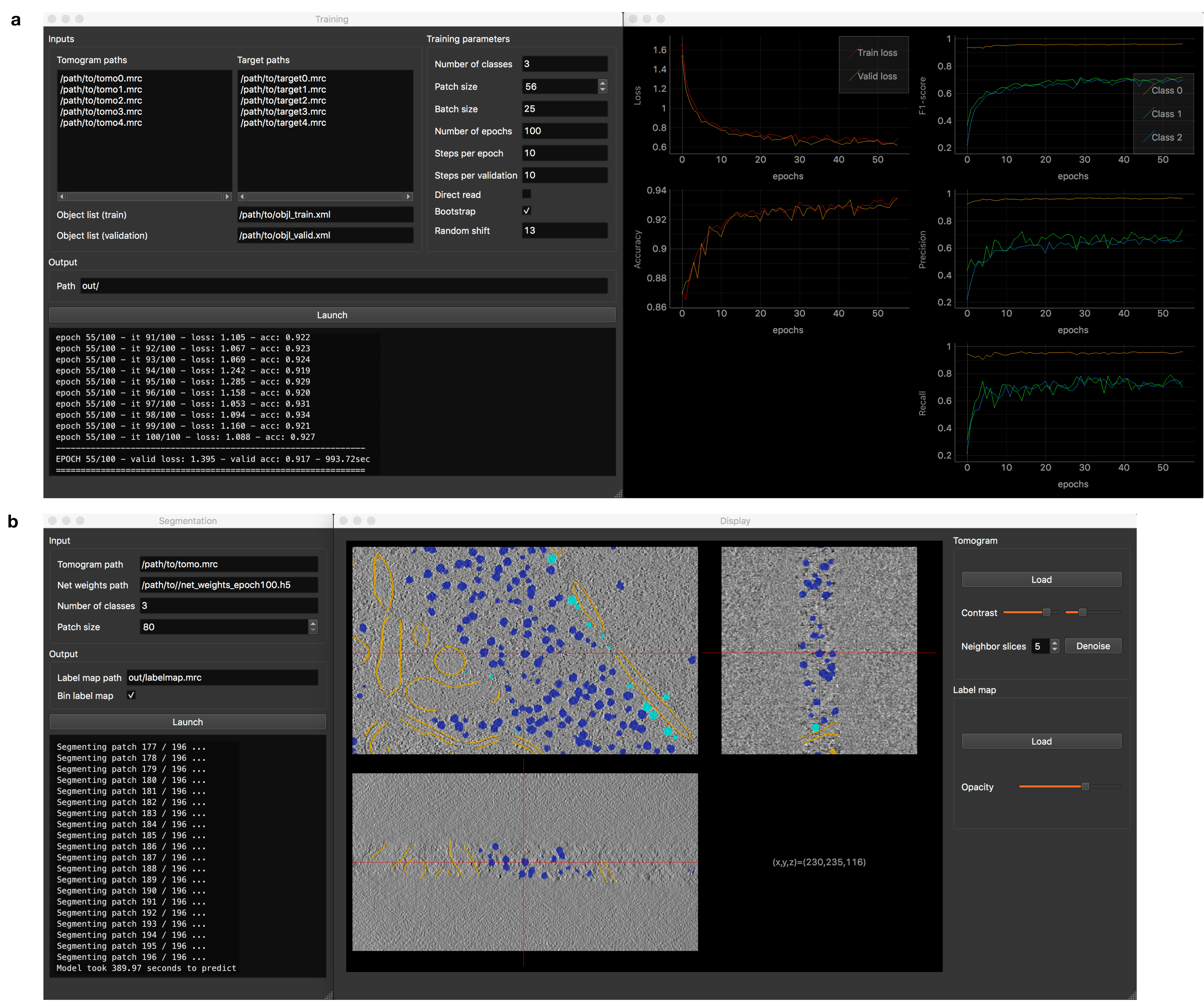
DeepFinder graphical user interface.a, Training interface composed of a first window for parametrizing the procedure and a second window for displaying the training metrics in real-time. b, Segmentation interface which also opens a data visualization tool. This tool allows the user to explore the tomogram with superimposed segmentations. In addition, DeepFinder also incorporates interfaces for tomogram annotation, target generation and clustering (see the documentation (GitLab) of DeepFinder for more information).
Software: Stand-alone DeepFinder and Plugin Scipion DeepFinder
Collaborators: B. Engel (Max-Planck Institute of Biochemistry, Martinsried, Germany; Helmholtz Pioneer Campus, Neuherberg, Germany); E. Fourmentin and D. Larivière (Fourmentin-Guilbert Scientific Foundation, Noisy-Le-Grand); C.O Sorzano (Biocomputing Unit, CSIC, Madrid, Spain); M. Eltsov and F. Fatmaoui (IGBMC Strasbourg); D. Levy and A. Bertin (UMR168 CNRS - Institut Curie, Paris); H. Lachuer (UMR 144 CNRS - Institut Curie, Paris)
8.2.4 Active subdivision surfaces for the semi-automatic segmentation of objects in 3D biological images
Participant: Anaïs Badoual.
We developed a new family of active surfaces for the semi-automatic segmentation of volumetric objects in 3D biological and biomedical images. We represent our deformable model by a subdivision surface encoded by a small set of control points and generated through a geometric refinement process. The subdivision operator confers important properties to the surface such as smoothness, reproduction of desirable shapes and interpolation of the control points. We deform the subdivision surface through the minimization of suitable gradient-based and region-based energy terms that we have designed for that purpose. In addition, we provide an easy way to combine these energies with convolutional neural networks. Our active subdivision surface satisfies the property of multiresolution, which allows us to adopt a coarse-to- fine optimization strategy (see Fig. 9(A)). This speeds up the computations and decreases its dependence on initialization compared to single resolution active surfaces. Performance evaluations on both synthetic and real biomedical data show that our active subdivision surface is robust in the presence of noise and outperforms current state-of-the-art methods. We have applied our proposed method to a variety of problems that involve synthetic data and real biomedical and biological images (see Fig. 9(B)).
Reference: 11
Collaborators: M. Unser (EPFL, Lausanne, Switzerland); L. Romani (Dipartimento di Matematica, Alma Mater Studiorum Università di Bologna, Italy)
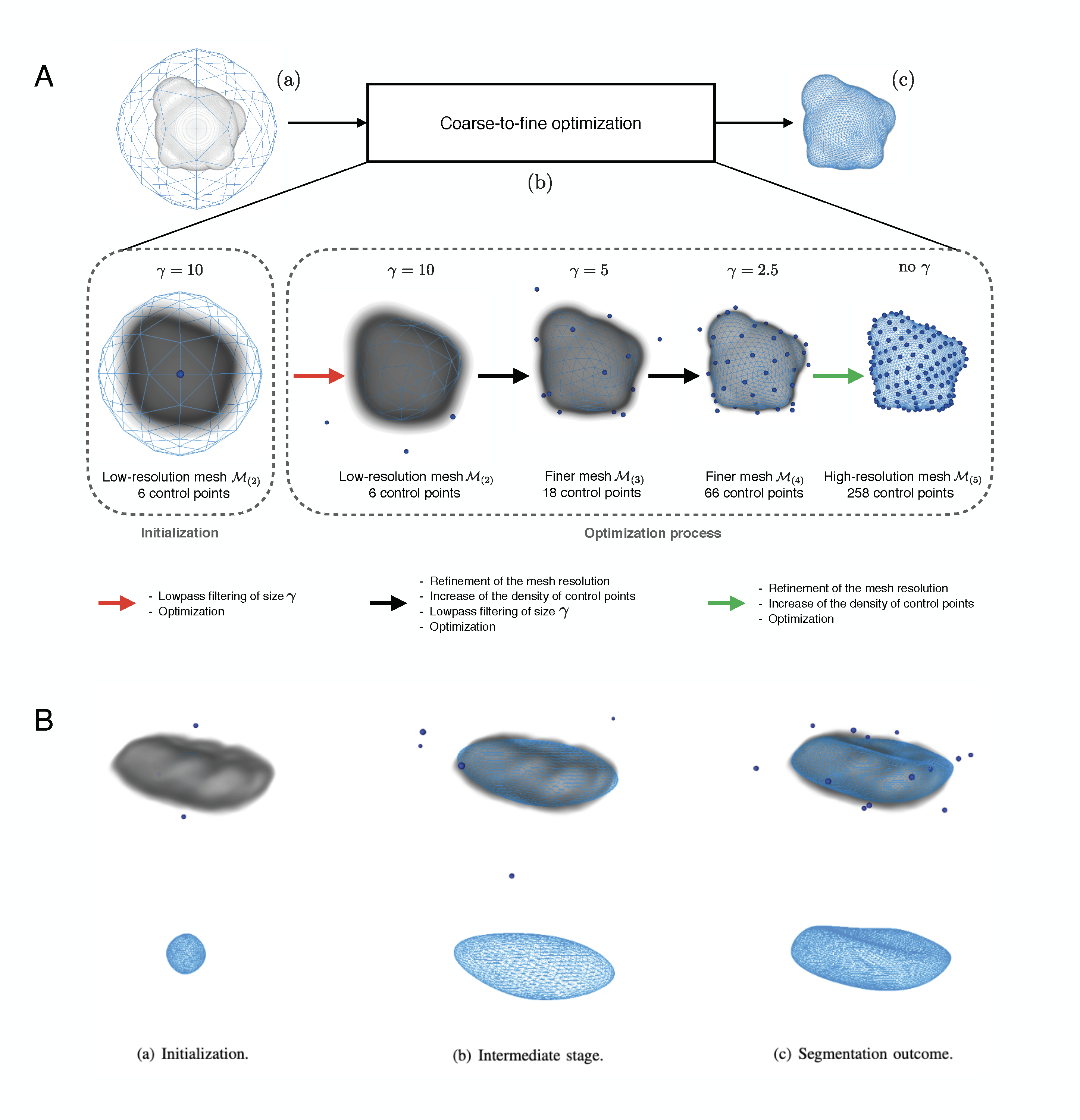
Active sufaces for 3D object segmentation in biomedical imaging. A, Flowchart of the proposed active subdivision surface. Blue dots: control points; Blue mesh: subdivision surface. (a) Segmentation inputs: the volume ff to segment and a subdivision surface represented by the triangular low-resolution mesh M(k)M_{(k)} and encoded by N0N_0 control points. For the illustration, we choose k=2k = 2 and N0=6N_0 = 6. (b) Coarse-to-fine optimization: first, we apply a low pass filter to f to obtain a volume where the object of interest contains few details only. We fit the coarse mesh M(2)M_{(2)} on this smoothed volume through the minimization of an energy functional that consists of a combination of gradient-based and region-based terms. Then, we use the outcome of this first optimization as the initialization at the next resolution level. We refine the mesh and the number of control points, optimizing it on a smoothed version of ff where the object of interest has more details than at the previous step. We continue until we reach the finest resolution level that corresponds to the original volume ff. (c) Segmentation outcome. B, Segmentation of the nucleus of the neuron of a rat in a 3D microscopic volume with the active subdivision surface. Blue dots: control points.
8.3 Models and methods for deciphering biological mechanisms and processes observed in light microscopy
8.3.1 Immersive interaction and visualization of endosomes and mitochondria colocalization
Participants: Gwendal Fouché, Ludovic Leconte, Cesar Augusto Valades-Cruz, Jean Salamero, Charles Kervrann.
Visualization of 3D+time intracellular events and biological processes has proven to work poorly on 2D monitor screens so far. Methods from the field of Immersive Analytics could be beneficial for biological imaging, where large time-varying datasets are more and more present, and have emerging constraints from the growing spatiotemporal resolutions. In this context, we have proposed a VR tool called MorphonetVR. This approach allowed an immersive 3D+time visualization of CD63 endosome tracks in the whole cell (see Fig. 10) and to localize interactions with the mitochondrial network. Another objective was to partially annotate co-localization events between CD63 endosomes and mitochondria, over time and in the whole cell. These results were presented to general public at the “Fête de la Science” (October 2021, Institut Curie, Paris).
Collaborators: F. Argelaguet (EPC HYBRID, Inria Rennes); E. Faure (Laboratory of Computer Science, Robotics and Microelectronics of Montpellier)
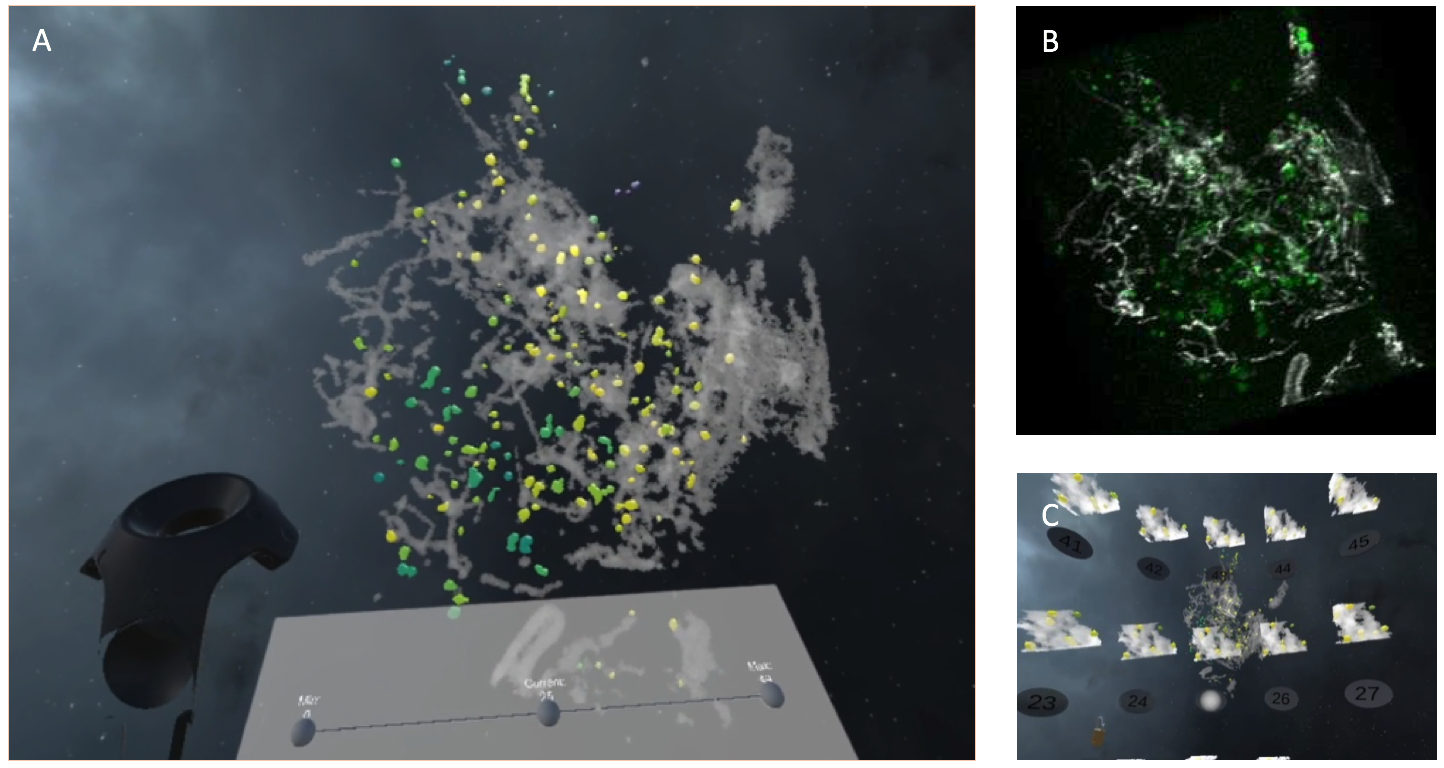
Visualization of CD63 endosomes (green - yellow) vs mitochondria (gray) in RPE1 cells.A, CD63 (green-yellow) and mitochondria (gray) visualization using MorphonetVR. Endosomes color represents distance to Mitochondria (green-far and yellow-close). B, CD63 endosomes(green) and mitochondria (gray) rendered in napari. C, Time evolution of 3D selected region.
8.3.2 Advocating for interdisciplinary collaborations to unravel the astrocyte calcium code
Participant: Anaïs Badoual.
Calcium signals in astrocytes contribute to the modulation of neuronal communication as well as neurovascular coupling. Those signals display a striking spatio-temporal diversity. Elucidating their mechanisms and physiological roles is a major challenge and requires interdisciplinary approaches (see Fig. 11). In this project, we advocated for more collaborations across fields and disciplines working on astrocyte function. As early-career scientists with different backgrounds and expertise, we presented the major challenges faced in our fields: data acquisition, analysis and modeling of astrocyte calcium activity. We further highlighted the insights gained from those different approaches, aiming at bridging the gap between them to crack the complex astrocyte ‘Calcium Code’. Finally, we proposed strategies to promote fruitful interdisciplinary collaborative projects to unravel astrocyte function in health and disease.
Reference: 29
Collaborators: A. Denizot (Computational Neuroscience Unit, Okinawa Institute of Science and Technology, Onna, Japan); A. Covalo (INSERM, U1215 NeuroCentre Magendie, Bordeaux)
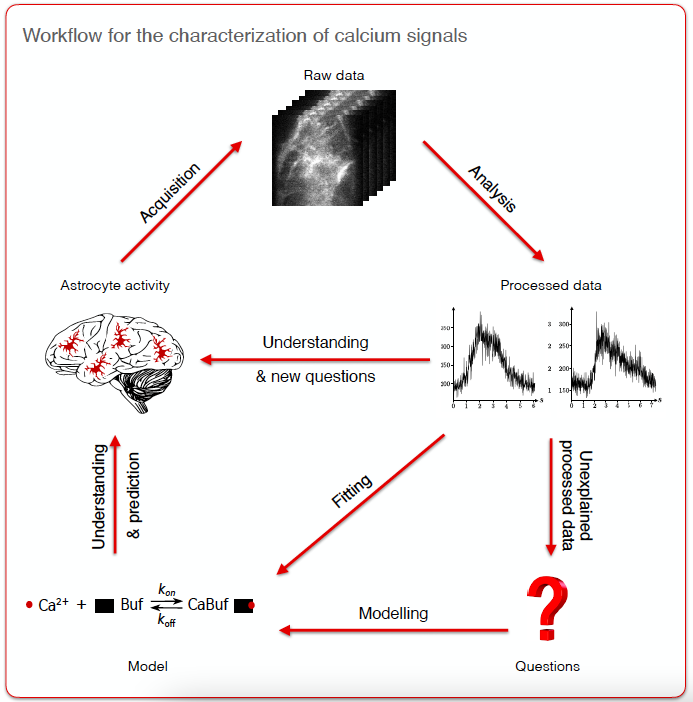
Workflow for the characterization of calcium signals involving the fields of acquisition, analysis and modeling. The raw data acquired by experimentalists include calcium images, structural images or omics data. Raw data processing by analysts results in dynamical (e.g., duration, trajectory, frequency) and structural characterization (e.g., protein localization, cell morphology) of astrocytes as well as the quantification of protein expression levels.
8.3.3 Deciphering the role of membrane microdomains in bacterial morphogenesis
Participants: Claire-Jing Rouchet, Charles Kervrann.
Characterisation of the membrane organisation of eukaryotic cells has revealed different lipid and/or protein domains that are essential for certain cellular functions. Recent studies have shown that bacterial membranes also exhibit lateral heterogeneity and can form specific lipid domains: regions of increased fluidity (RIFs) and functional membrane microdomains (FMMs), which differ in their lipid composition. They can regulate essential functions such as DNA segregation and replication, cell division, morphogenesis and motility (see Fig. 12). In the case of the rod-shaped model bacteria B. subtilis, rapid cell growth in the exponential phase requires fine control of bacterial wall synthesis, which is essential for maintaining cell shape. This mechanism is in part provided by the peptidoglycan elongation machinery (PGEM) with which the RIF domains appear to interact. By combining molecular biology, fluorescent microscopy (super-resolution microscopy (spt-PALM), spot-variation fluorescence correlation spectroscopy (svFCS)), and DenseMapping software 43 analysis, our aim is to understand the role of spatiotemporal dynamics of the membrane domains and proteins involved in PGEM.
Collaborators: R. Carballido-López and C. Billaudeau (INRAE, MICALIS UMR, Jouy-en-Josas)
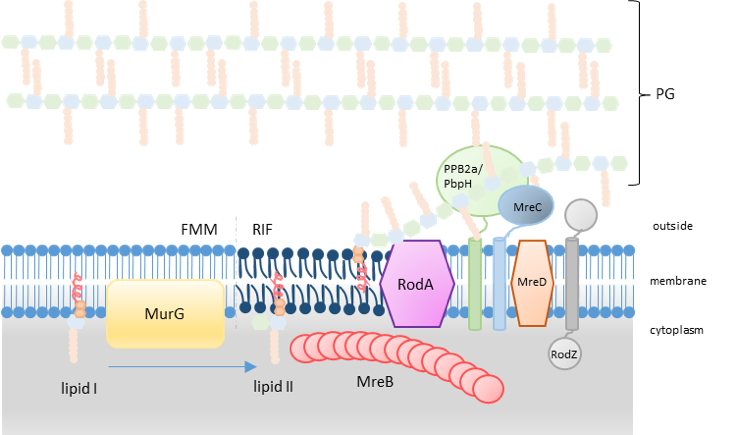
Representation of the PG synthesis with the hypothetic localisation of RIF in the PGEM system. (inspired by (Scheffers and Tol, 2015 ) and (Angeles and Scheffers, 2021 )
8.3.4 Tracking and size estimation of virus and extracellular vesicles in interferometric light microscopy
Participants: Cesar Augusto Valades-Cruz, Charles Kervrann.
In collaboration with a biotechnology startup, we have developed several models and image processing algorithms to improve the tracking and size estimation of virus and extracellular vesicles observed with the VideoDrop microscopy set-up. First, we proposed several strategies to estimate the distribution of nanoparticle sizes, including the DenseMapping method 43539. Second, the Atlas software 1 was implemented in Matlab to detect nanobeads, extracellular vesicles, gold nano-particles and viruses in image sequences. Evaluation of performance in comparison with current detectors were performed on large-scale datasets. Third, we evaluated the potential of HotSpot 42, implemented in Matlab, to detect beads of 2, 4 and 6
Software: DenseMapping 43, Atlas 1, HotSpot 42
Collaborator: Matthieu Greffet (Myriade)
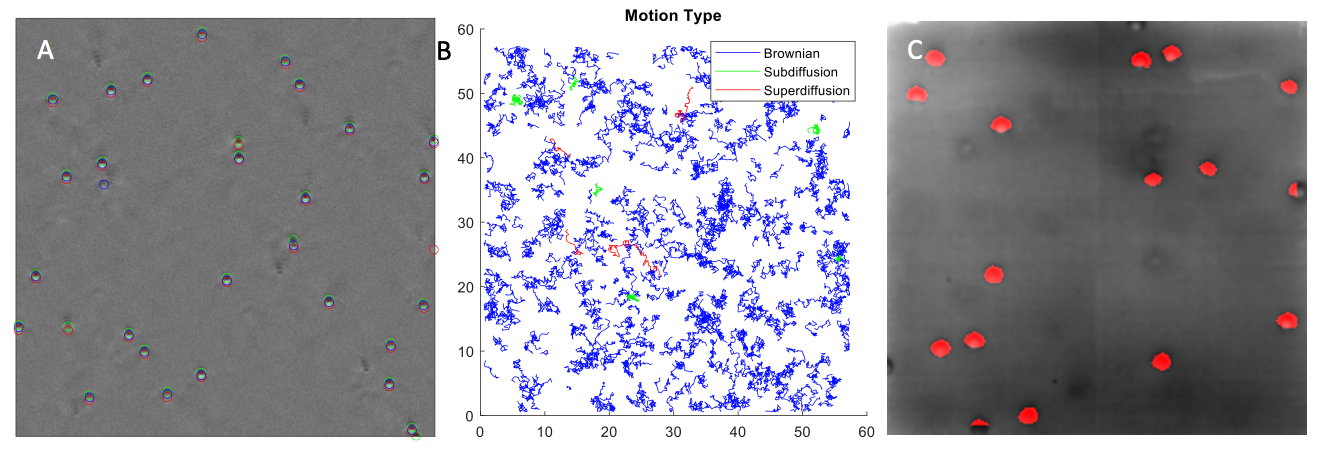
Evaluation of algorithms on VideoDrop images depicting nanoparticles. A, Detection (Atlas sotware) of beads with size of 200 nm. B, Tracking and classification (Dense-Mapping software) of beads with size of 100 nm. C, Detection (HotSpot software) of beads with size of 2 μ\mu m.
8.3.5 Parametric estimation of birth-death-move processes for protein dynamics analysis
Participants: Lisa Balsollier, Frédéric Lavancier.
Birth-death-move processes are stochastic processes describing the spatio-temporal evolution of a system of particles that move in space over time (possibly in interaction), and where births of new particles along with deaths of existing particles can occur over time. In the first part of the internship of Lisa Balsolier, we obtained the general likelihood function of this process (assuming that it depends on some parameters). In a second part, we studied the maximum likelihood estimator of a parameter involved in the birth and death intensity functions of the process. These functions rule the arrival times of births and deaths in the dynamics. We proved, under some assumptions, the consistency and asymptotic normality of this estimator, when the observation time grows to infinity, with an explicit expression of the asymptotic variance. Some simulations implemented in Python confirmed our theoretical findings.
8.3.6 Study of the correlation of cellular death pathways and location of Hepatocytes in cells
Participant: Léo Maury.
Hepatocytes are the chief functional cells of the liver. Our goal was to determine if there is a link between the location of a hepatocyte and its death pathway. We studies four types of cellular death: apoptosis, necrosis, pyroptosis, and ferroptosis. Our data was composed of 1,415 hepatic cells and gene expression profile (27,297 genes, in UMI), the most likely location between nine liver layers per cell (probabilities determined from expression of six landmark genes), and a list of 333 genes involved in death pathways. We first used genes with known locations within the hepatic lobule (Glul, Cyp2e1, Ass1, Asl, Alb and Cyp2f2). These genes were used as reference and visualized with principal component analysis (Fig. 14a). The color gradient displays that the expression of the landmark genes correlates well with the position of cells in the liver. Furthermore, we applied random forest technique to predict the layer with landmark genes expression (low error rate of 15.69%). The same approach was applied to genes involved in each of the four death pathways. The results of the PCA (Fig. 14b) indicates that the hepatocytes impacted by these cell deaths are relatively well distributed between the different layers. However, random forest shows an accumulation of liver cells in layers #3, #5, #6 and #7 (Fig. 14c). If there had been a correlation, we would have observed layers specific to each type of cellular death. Note that the prediction errors are very high (between 63 % and 80 %) so far, suggesting that cell death pathways would not be correlated to hepatocyte locations in the cell.
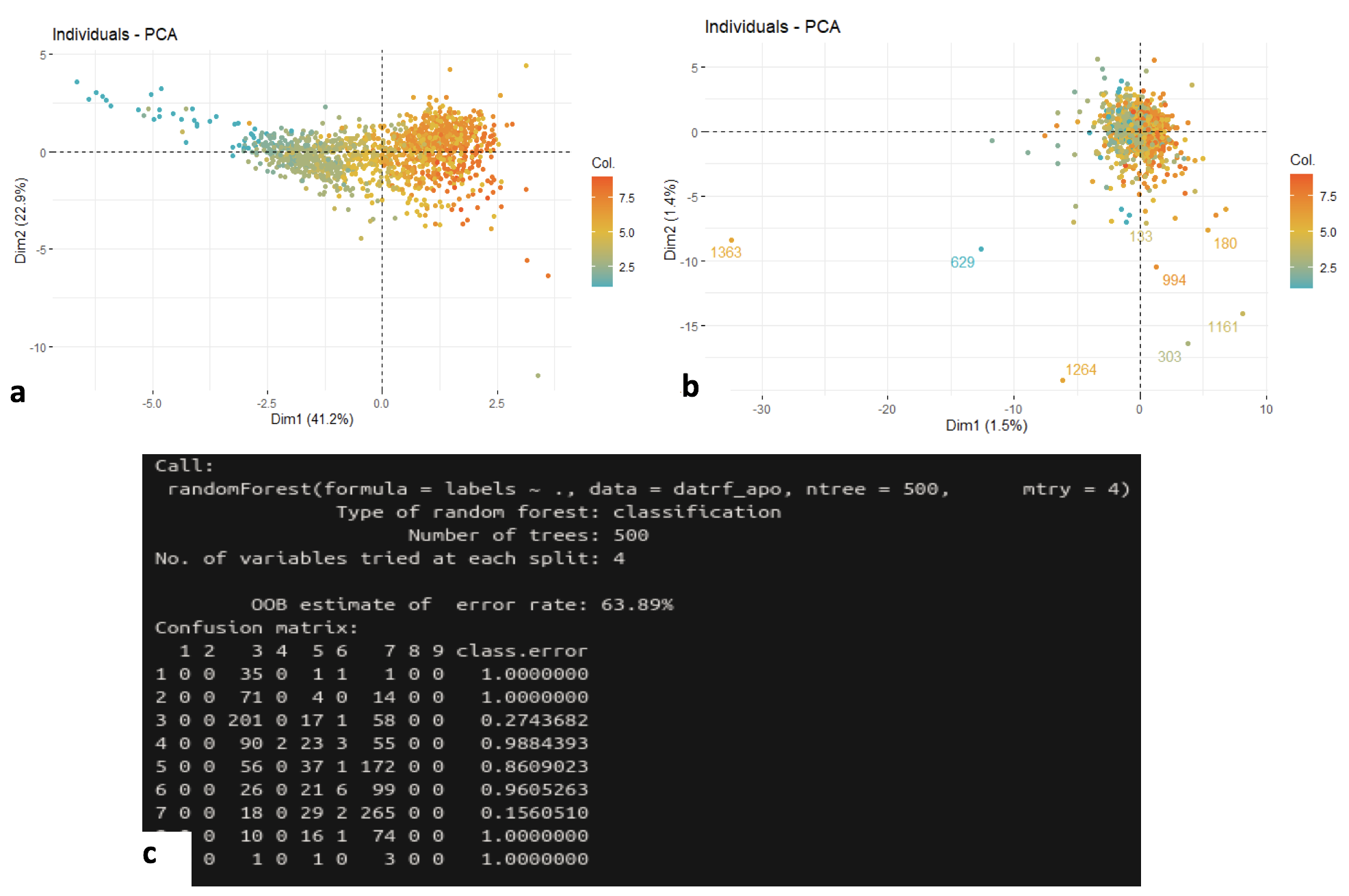
Analysis of the correlation of cellular death pathways and locations of Hepatocytes in cells.a, Principal component analysis on 1,415 cells (1 dot = 1 cell) according to the expression profiles of landmark genes (position of the dots). High color magnitude encodes the most likely layer of the liver per cell (Table S2, blue: layer #1, red: layer #9). b, PCA on 1,415 cells (1 dot to denote 1 cell) according to the expression profiles of apoptosis genes (dot position). High color magnitude encodes the most likely layer of the liver per cell (Table S2, blue: layer #1; red: layer #9). Same results for necrosis, pyrroptosis and ferroptosis. c, Prediction of layers using random forest applied to apoptosis genes. Same results for necrosis, pyrroptosis and ferroptosis.
Collaborators: Y. Le Cunff (EPC Dyliss, Inria Rennes), J. Le Seyec and A. Hamon (2IFEF, IRSET, Rennes)
8.4 Machine learning for motion estimation and segmentation
8.4.1 Estimation of salient motion maps from the interpretation of a frame-based classification network
Participants: Etienne Meunier, Patrick Bouthemy.
We have introduced a new paradigm to compute salient motions in video frames based on optical flow only 22. Motion saliency (MS) is an important issue in dynamic scene analysis, whatever the application domain (e.g., live cell microscopy, remote sensing, or autonomous navigation). MS aims to highlight local motions departing from their surrounding context, thus prone to reveal significant dynamic events. We formulated MS as a meta-task that can be instantiated for different tasks usually handled independently. To support this claim, we have addressed two important general problems with our MS paradigm: segmentation of independently moving objects, and detection of anomalous motion, in videos acquired with a mobile camera. We estimate MS from the interpretation of a frame-based motion saliency classification network with optical flow (OF) as input. Our paradigm can accommodate any given form of motion saliency by simply training the frame-based classification network on the corresponding task. Our MS estimation is unsupervised, as it does not require any ground-truth saliency maps for training. In addition, we have designed an original two-step network interpretation scheme leveraging the LRP method. It supplies the binary salient motion segmentation. Finally, we can recover the valued motion saliency map using a parametric flow inpainting algorithm. Experimental results on real videos and comparison with existing methods demonstrated the performance of our method.
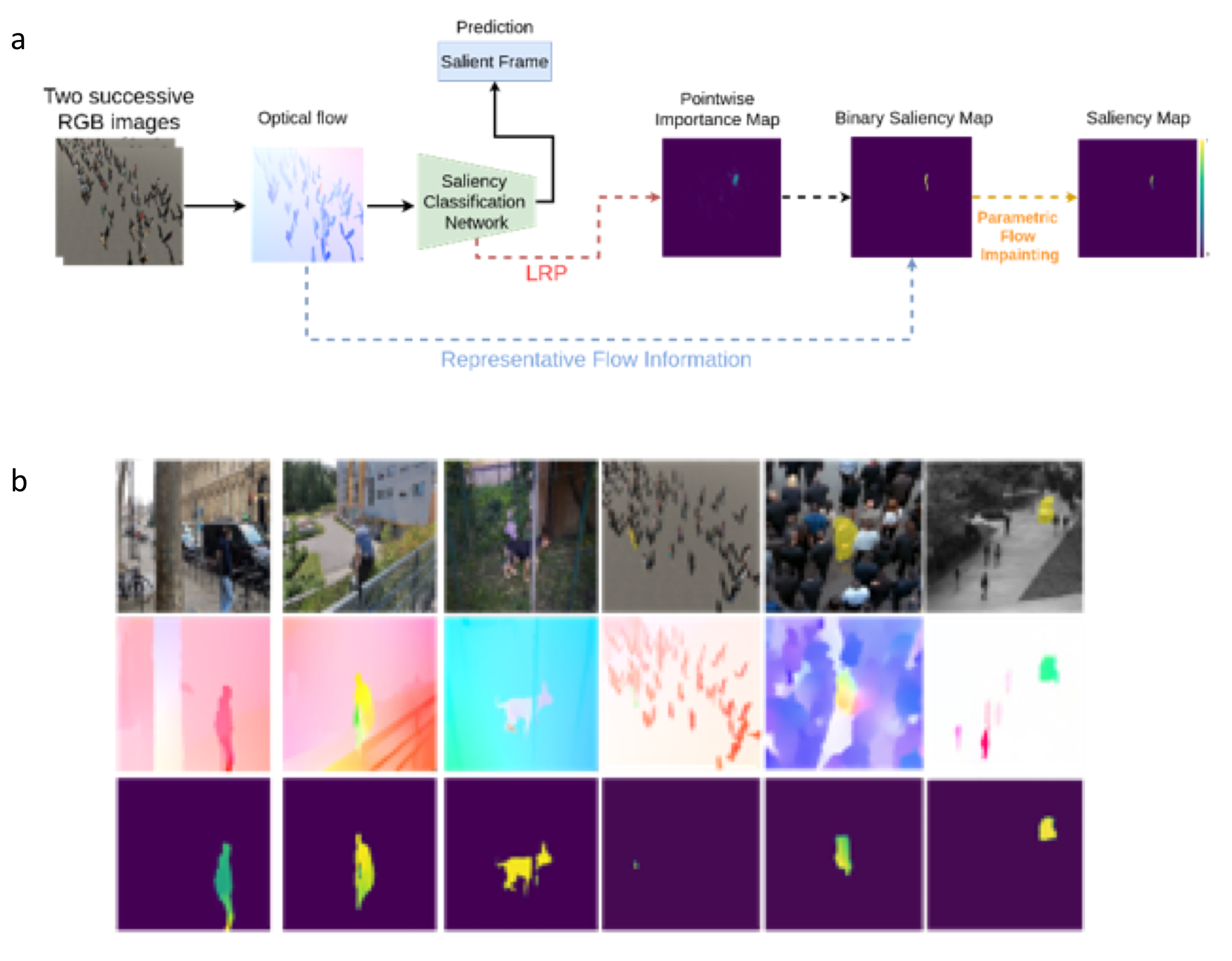
Estimation of salient motion maps.a, Overall workflow of our motion saliency framework. b, Six different examples, the three first ones correspond to the first task (independent motion segmentation), the three last ones to the second task (anomalous motion detection). From top to bottom, One image of the video (with the ground-truth superimposed in yellow for the last three ones), the optical flow taken as input of the network and displayed with the HSV code, the motion saliency maps computed with our method (with highest saliency in yellow).
Collaborator: R. Fraisse (AIRBUS Defense and Space SAS)
8.4.2 Deep EM-driven motion segmentation from optical flow
Participants: Etienne Meunier, Anaïs Badoual, Patrick Bouthemy.
We have defined a fully unsupervised CNN-based method to segment optical flow fields into coherent motion regions. We assume that the input optical flow can be represented as a piecewise set of polynomial models, typically affine or quadratic motion models, each of them characterizing one motion segment (a segment is either a region, i.e., a connected component, or a layer that is not necessarily connected). The core idea of our work is to leverage the Expectation-Maximization (EM) framework. The latter enables us to design in a well-founded manner the loss function and the training procedure of our motion-segmentation neural network. However, in contrast to the classical EM, our network, once trained, can provide a segmentation for any unseen optical flow field in a single inference step, with no dependence on initialization and without estimating any parametric motion models. Different loss functions have been investigated including robust ones. We have also defined a novel data augmentation scheme dedicated to optical flow that has a noticeable impact on the network performance. We tested our motion segmentation network on the DAVIS2016 dataset, and our method outperforms comparable unsupervised methods. In addition, it can run at 125fps making it usable for real-time applications.
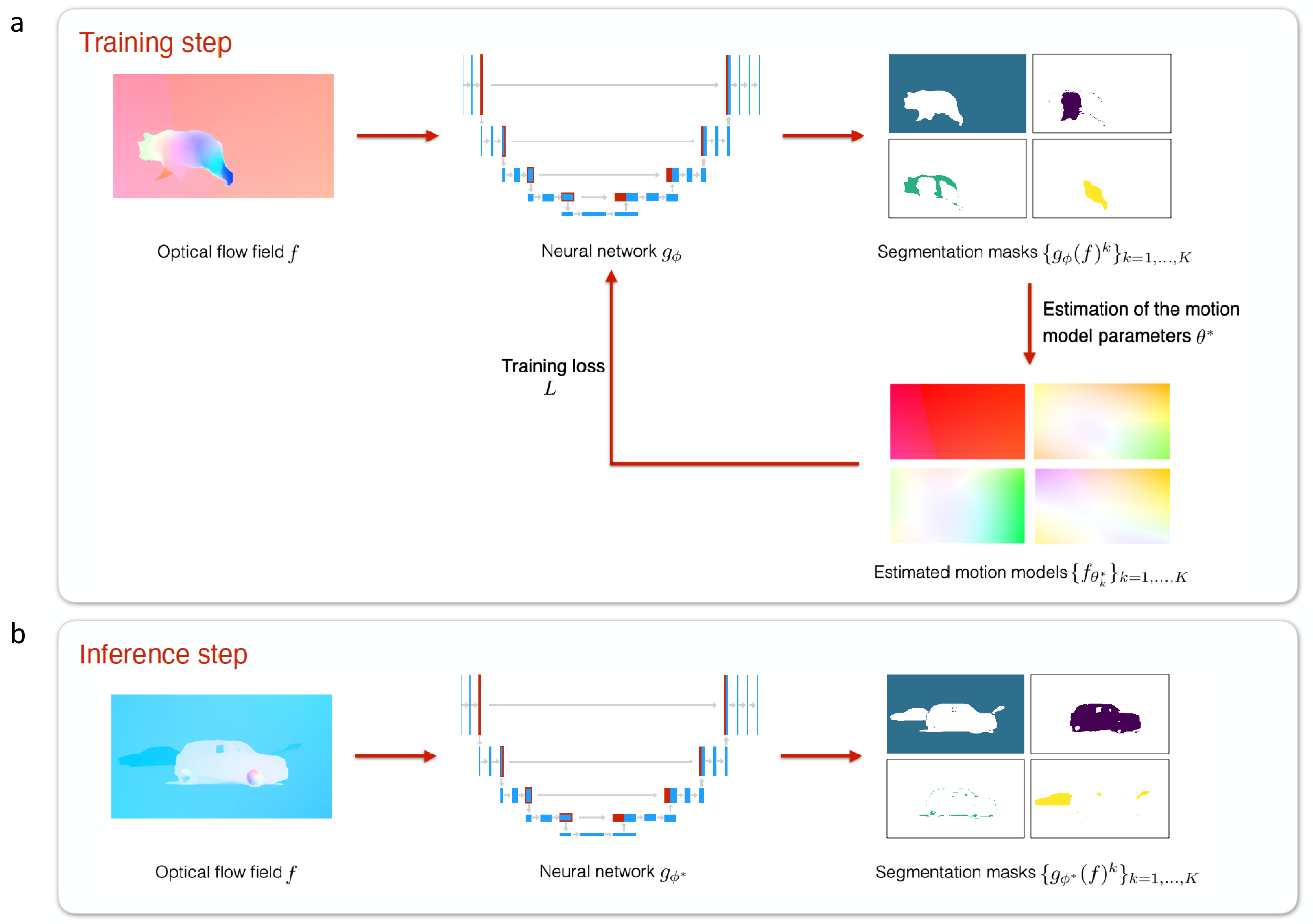
Flowchart of the proposed CNN method for the training (a) and inference (b) steps. Training step: First, we segment the optical flow field ff with the neural network gϕg_\phi . Then, we get the optimal parametric motion models {fθk*}k=1,⋯,K\lbrace f_{\theta _k^*}\rbrace _{k=1,\dots ,K} within each probabilistic segmentation masks {gϕ(f)k}k=1,⋯,K\lbrace g_\phi (f)^k\rbrace _{k=1,\dots ,K}. Finally, we update the parameters ϕ\phi of the neural network. This training step is performed iteratively over each batch ℬ\mathcal {B} (of size 1 in this illustration). Inference step: We directly apply the trained network gϕ*g_{\phi ^*} to any new unseen optical flow field ff to obtain the probabilistic segmentation masks {gϕ*(f)k}k=1,⋯,K\lbrace g_{\phi ^*}(f)^k\rbrace _{k=1,\dots ,K}.
Reference: 32
Collaborator: R. Fraisse (AIRBUS Defense and Space SAS)
9 Bilateral contracts and grants with industry
9.1 Bilateral contracts with industry
9.1.1 Contract with GATACA Systems: Super-resolution microscopy and in live cell imaging
Participants: Jean Salamero, Ludovic Leconte, Cesar Augusto Valades-Cruz, Charles Kervrann.
Funding: GATACA Systems company
Duration: (2017 – 2023)
Collaborator: C. Gueudry (GATACA Systems)
The objective of the project is to transfer innovations in Multi-Angle (MA) TIRFM (using Azymuthal TIRFM from Ilas2/3) and development of polarization Multi-Angle TIRFM (Polar MA-TIRFM).
9.1.2 Contract with CryoCapCell SA: 3D LIVE CLEM (Correlative Light and Electron Microscopy) to decipher fates and functions of exosomes in vivo
Participant: Jean Salamero.
Funding: DIM–ELICIT Empowering LIfe sCiences with Innovative Technologies (Région Ile de France)
Duration: (2018 – 2022)
Collaborators: G. Van Niel (coordinator, Institute of Psychiatry and Neuroscience of Paris), G. Raposo (UMR 144 CNRS - Institut Curie, PSL Research), X. Heiligenstein (CryoCapCell SA).
The objective of the project is to link dynamic biogenesis of intracellular membrane compartments with their ultrastructures. It combines fast high resolution photonic imaging (MA-TIRFM) and fast high pressure freezing for 3D cryoEM. It requires adapted registration methods in 3D, in order to navigate through the multiple scales.
9.1.3 Contract with MYRIADE company
Participants: Cesar Augusto Valades-Cruz, Charles Kervrann.
Funding: Myriade
Duration: 12 months (Jan 2021 – Dec 2021)
Collaborators: M. Greffet (Myriade)
This project concerns the research engineer position of Cesar Augusto Valades-Cruz. The goal is to develop statistical methods and algorithms for detecting beads, vesicles, and viruses, and estimating the concentration and the size distribution of nanoparticles in interferometric light microscopy image sequences.
9.2 Bilateral grants with industry
9.2.1 Contract with IRSN: DeepSuN – Localization of chromosomal aberrations induced by nuclear radiation dose excess
Participants: Antonin Deschemps, Charles Kervrann.
Funding: IRSN (Institut de Radioprotection et de Sureté Nucléaire) and Région-Bretagne
Duration: 36 months (Oct 2020 – Sep 2023)
Collaborator: M. Benadjaoud (IRSN/LRACC, Fontenay-aux-Roses)
This project funded by the IRSN (Institut de Radioprotection et de Sureté Nucléaire) and Région-Betagne concerns the PhD thesis (co-funding) carried out by Antonin Deschemps. The goal is to develop statistical and deep learning and methods for localizing and classifying chromosomal aberrations observed in 2D microscopy images (blood test) and estimating radiation dose following a postulated nuclear reactor accident. See details in Section 10.3.3 (ANR INCREASED).
9.2.2 Contract with AID: Localization and classification of gene translocation in FISH microscopy images
Participants: Quentin Tallon, Charles Kervrann.
Funding: AID (Agence de l'Innovation de Defense)
Duration: 36 months (Oct 2021 – Sep 2024)
Collaborator: M. Benadjaoud (IRSN/LRACC, Fontenay-aux-Roses)
This project funded by the AID (Ministry of Defense) concerns the PhD thesis carried out by Quentin Tallon. The goal is to develop supervised and unsupervised machine learning methods and algorithms for detection and classification of gene translocation between chromosomes observed in FISH (Fluorescence in situ hybridization) microscopy images. See details in Section 10.3.3 (ANR INCREASED).
9.2.3 Contract with AIRBUS Defense and Space SAS: LION Chaine Image Elargie (LiChIE)
Participants: Sébastien Herbreteau, Etienne Meunier, Anaïs Badoual, Sylvain Prigent, Patrick Bouthemy, Charles Kervrann.
Funding: Bpifrance / Projets Structurants pour la Compétitivité (PSPC)
Duration: 54 months (Aug 2019 – Jan 2024)
Collaborators: M. Ortner and R. Fraisse (AIRBUS Defense and Space SAS)
This project funded by Bpifrance concerns the PhD theses carried out by Sébastien Herbreteau and Etienne Meunier, the 6-month postdoc position of Anaïs Badoual, and the engineer position of Sylvain Prigent in 2021. The goal is to develop statistical and deep learning and methods for image restoration in night conditions and motion saliency detection in image sequences, respectively. The resulting algorithms will be embedded in hardware platforms for a next generation of observation satellites.
10 Partnerships and cooperations
10.1 International initiatives
10.1.1 Global BioImaging (GBI)
Participant: Jean Salamero.
J. Salamero is a member of the management board of "Global Bioimaging" (GBI) (INFRASUPP-6-2014 - International Cooperation for Research Infrastructure) since 2014. Since 2019, GBI is funded in part by a grant from the Chan Zuckerberg Initiative DAF, an advised fund of the Silicon Valley Community Foundation. J. Salamero is co-leader of the WP "Training courses for facility staff" (Task leader Rainer Pepperkok, EMBL), and since 2019, member of the WG "Societal Impact of Imaging Infrastructures".
10.1.2 African BioImaging Consortium (ABIC)
Participant: Jean Salamero.
Partnership (since October 2021, for France BioImaging (FBI), J. Salamero) with ABIC which is a new Pan-African Consortium in BioImaging co-funded by the Chan Zuckerberg Initiative DAF, an advised fund of the Silicon Valley Community Foundation and by the Bill and Melinda Gates Foundation. France-BioImaging is an international partner involved in training and managing Imaging core facilities.
10.1.3 Informal international partners
-
–
Collaboration with Max-Planck Institute of Biochemistry (Martinsried, Germany), Helmholtz Pioneer Campus (Neuherberg, Germany) and Fourmentin-Guilbert Scientific Foundation (Noisy-Le-Grand, France): Detection and segmentation of macromolecules in cryo-electron tomography. (with E. Moebel and C. Kervrann)
-
–
Collaboration with Biocomputing Unit, CSIC (Madrid, Spain): Integration of DeepFinder software into the Scipion platform dedicated to cryo-electron microscopy image analysis (Plugin DeepFinder in Scipion). (with E. Moebel and C. Kervrann)
-
–
Collaboration with the Cambridge Advanced Imaging Centre, Cambridge, UK: Dense mapping for transcription factors analysis in single molecule localization microscopy. (with A. Salomon and C. Kervrann)
-
–
Collaboration with Computational Neuroscience Unit, Okinawa Institute of Science and Technology Graduate University (OIST), Japan: Generation of synthetic datasets of astrocytic calcium activity to support the development of learning based analysis tools; Advocating for interdisciplinary collaborations to unravel the astrocyte 'Calcium Code'. (with A. Badoual)
-
–
Collaboration with the PKU University, Institute of Molecular Medicine, Beijing, People Republic of China:
-
3D reconstitution of the biogenesis of Endoplasmic Reticulum-Plasma Membrane Contact Sites-ER-PM MSCs upon Ca2+ store depletion or replenishment (collaboration funded by a contract between PSL and PKU for exchange of students).
-
Development and application of new mitochondrial fluorescence probes for advanced live imaging (LLSM, STED microscopy).
(with C.A. Valades-Cruz, L. Leconte, and J. Salamero)
-
10.2 European initiatives
10.2.1 Other european programs/initiatives
ESFRI initiative program: EuroBioImaging
Participant: Jean Salamero.
Coordinator: J. Eriksson (Turku University, Finland)
Funding: Member states of the European Union
Partners: 16 European countries in 2021
As a member of the National Research Infrastructures (RI) France BioImaging, SERPICO is involved in the ESFRI Euro-BioImaging project, and now in the ERIC EuroBioImaging (since November 2019), one of the landmarks of biomedical science Research Infrastructures in the roadmap of the European Strategic Forum on Research Infrastructures (ESFRI 2018). The mission of Euro-BioImaging is to provide access, service and training to state-of-the-art imaging technologies and foster the cooperation and networking at the European level including multidisciplinary scientists, industry, regional, national and European authorities.
J. Salamero is a special advisor of the MESRI and CNRS French Representatives in the ERIC-Euro-BioImaging board.
10.3 National initiatives
10.3.1 France-BioImaging project
Participants: Léo Maury, Sylvain Prigent, Cesar Augusto Valades-Cruz, Ludovic Leconte, Jean Salamero, Charles Kervrann.
Duration: 2011 – 2024
Funding: Investissement d'Avenir, ANR INBS-PIA1 2011 and “FBI Next Generation” (ANR program 2020-2024)
Coordinator: E. Bertrand (UMR 9002 CNRS)
Partners: CNRS, Aix-Marseille Université, Collège de France, Ecole Normale Supérieure, Ecole Polytechnique, Inria, Institut Curie, Institut Pasteur, Inserm, Université de Bordeaux, Université de Montpellier, Université de Nantes, Université de Paris, Université de Rennes 1.
SERPICO is a member of the French initiative, the so-called “France-BioImaging” (FBI) National Research Infrastructure which gathers several outstanding cellular imaging centers (microscopy, spectroscopy, probe engineering and signal processing). FBI is on the French Roadmap of Research Infrastructure. The mission of FBI is to build a distributed coordinated French infrastructure for photonic and electronic cellular bioimaging, dedicated to innovation, training and technology transfer. High-computing capacities are needed to exhaustively analyse image flows.
SERPICO is co-head of the IPDM (Image Processing and Data Management) node of the FBI network composed of 7 nodes. In this context, we address the following scientific problems: i/ exhaustive analysis of bioimaging data sets; ii/ deciphering of key steps of biological mechanisms at organ, tissular, cellular and molecular levels through the systematic use of time-lapse 3D microscopy and image processing methods; iii/ storage and indexing of extracted and associated data and metadata through an intelligent data management system. SERPICO recruited R&D engineers to disseminate image processing software, to build the Mobyle@serpico web portal and to manage the IGRIDA-SERPICO cluster opened for end-users and dedicated to large scale computing and data sets processing. This project concerns the engineer position of Léo Maury in 2021.
10.3.2 ANR DALLISH project: Data Assimilation and Lattice LIght SHeet imaging for endocytosis / exocytosis pathway modeling in the whole cell
Participants: Antoine Salomon, Cesar Augusto Valades-Cruz, Yunjiao Lu, Ludovic Leconte, Patrick Bouthemy, Frédéric Lavancier, Jean Salamero, Charles Kervrann.
Duration: 48+6 months (Oct 2016 – March 2021).
Funding: ANR (Agence Nationale de la Recherche) PRC (Collaborative Research Project)
Coordinator: C. Kervrann.
Partners: Inria SERPICO and BEAGLE Teams, INRAE MaIAGE Unit Jouy-en-Josas, Institut Curie (UMR 144 CNRS and U1143 INSERM / UMR 3666 CNRS), Paris.
Cutting-edge Light Lattice Sheet microscopy represents the novel generation of 3D fluorescence microscopes dedicated to single cell analysis, generating extraordinarily high resolved and sharp, but huge 3D images and videos. One single live cell experiment in one single biological condition can result into up to one terabyte of data. The goal of the project is to develop new paradigms and computational strategies for image reconstruction and 3D molecule motion estimation and tracking. Furthermore, establishing correspondences between image-based measurements and features, stochastic motion models, and underlying biological and biophysical information remains a challenging task. In a larger perspective, the quantitative description of image data corresponding to protein transport will be a prerequisite for understanding the functioning of a cell in normal and pathological situations including cancer, viral infection and neurodegenerative diseases. This project concerns the PhD thesis carried out by Antoine Salomon in 2021.
10.3.3 ANR INCREASED project: Artificial intelligence for the detection of chromosomal aberrations in dosimetry
Participants: Antonin Deschemps, Quentin Tallon, Emmanuel Moebel, Charles Kervrann.
Duration: 36 months (Oct 2020 – Sept 2023)
Funding: ANR (Agence Nationale de la Recherche) ASTRID
Coordinator: Gaetan Gruel (Institut de Radioprotection et de Sureté Nucléaire (IRSN/LRACC), Fontenay-aux-Roses)
Partners: IRSN/LRACC, SERPICO Team, IRBA (Institut de Recherche Biomédicale des Armées)
During insidious scenarios, when the assessment of ionizing radiation exposure condition is difficult or impossible, the quantification, on samples taken from the victims, of radio-induced chemical or biological effects is more suitable for an individualized dose reconstruction compared to theoretical calculation or Monte-Carlo simulations in regard to their great uncertainties. The INCREASED project aims to adapt the most powerful algorithms of modern artificial intelligence to the context of automatic detection of chromosomal aberrations in dosimetry. This project proposes to revisit the semi-automatic or automatic detection methods currently used for the recognition of dicentrics in GIEMSA imagery in the light of the most recent advances in artificial intelligence and deep learning. These modern methods, which demonstrated their indisputable superiority in other areas of computer vision, will be deployed on GIEMSA images for an exhaustive multi-class count not only of dicentric chromosomes but also of ring-centric aberrations, acentric fragments, and even tricentrics. Furthermore, the INCREASED initiative is also quite innovative in dosimetry based on FISH imaging. To date, this type of dosimetric reconstruction is based on manual counting of non-exhaustive and very simplified annotations of the different possible forms of translocations. This protocol is therefore as heavy as imprecise. The INCREASED project adresses two major improvements: i/ desgn of a universal, rigorous and exhaustive scoring of the different forms of observable FISH translocations (3 colors). ii/ development of modern artificial intelligence algorithms able to detect and translate co-locations/co-neighborhoods into different categories of translocations. This project concerns the post-doc position of Emmanuel Moebel in 2021.
10.3.4 Inria DEFI NAVISCOPE: image-guided NAvigation and VISualization of large data sets in live cell imaging and microCOPy
Participants: Gwendal Fouché, Kévin Fournier, Cesar Augusto Valades-Cruz, Ludovic Leconte, Anaïs Badoual, Jean Salamero, Charles Kervrann.
Duration: 60 months (2018 – 2022)
Funding: Inria
Coordinator: C. Kervrann
Partners: AVIZ (Inria Saclay), BEAGLE (Inria Lyon), HYBRID(Inria Rennes), MORPHEME (Inria (Sophia-Antipolis), MOSAIC (Inria Lyon), PARIETAL (Inria Saclay), SERPICO (Inria Rennes), MaIAGE INRAe Unit (Jouy-en-Josas), UMR 144 CNRS and UMR 168 CNRS - Institut Curie (Paris), Institut Pasteur (Paris)
In the frame of the "Naviscope" IPL project, our objective is to develop original and cutting-edge visualization and navigation methods to assist scientists, enabling semi-automatic analysis, manipulation, and investigation of temporal series of multi-valued volumetric images, with a strong focus on live cell imaging and microscopy application domains. Naviscope, built upon the strength of scientific visualization and machine learning methods, will provide systems capable to assist the scientist to obtain a better understanding of massive amounts of information. Such systems will be able to recognize and highlight the most informative regions of the dataset by reducing the amount of information displayed and guiding the observer attention. We address the three following challenges and issues:
-
–
Novel machine learning methods able to detect the main regions of interest, and automatic quantification of sparse sets of molecular interactions and cell processes during navigation to save memory and computational resources.
-
–
Novel visualization methods able to encode 3D motion and deformation vectors and dynamics features with color and texture-based and non-sub-resolved representations, abstractions, and discretization, as used to display 2D motion and deformation vectors and patterns.
-
–
Effective machine learning-driven navigation and interaction techniques for complex functional 3D+Time data enabling the analysis of sparse sets of localized intra-cellular events and cell processes (migration, division, etc.).
Meanwhile, we address the technological challenge of gathering up the software developed in each team to provide a unique original tool for users in biological imaging, and potentially in medical imaging. This project concerns the PhD thesis carried out by Gwendal Fouché and the engineer position of Kevin Fournier in 2021.
10.3.5 CNRS Project 80|Prime ModSpatioTempCell: Spatiotemporal stochastic models for the comprehension of membrane trafficking at the single cell scale
Participants: Lisa Balsolier, Frédéric Lavancier, Jean Salamero, Charles Kervrann.
Duration: 36 months (Oct 2021 – March 2023).
Funding: CNRS
Coordinator: F. Lavancier.
Partners: SERPICO Team, UMR 144 CNRS - Institut Curie, Laboratoire de Mathématiques Jean-Leray Nantes, UMR 6629 CNRS .
Endocytosis and exocytosis mechanisms involve multiple molecular components that interact through space and time according to a complex process. In this project, we plan to analyze the spatiotemporal activity of some of these proteins thanks to a tailored mathematical model, fitted to sequences of real data acquired by fluorescence microscopy. The mathematical model at hand characterizes the dynamics of a system of interacting particles with possible appearance and disappearance of some particles through time, in accordance with the observed dynamics of proteins in cell membrane trafficking. This project concerns the PhD thesis carried out by Lisa Balsollier (since October 2021).
10.3.6 INRAE DIGITBIO BovMovie2Pred project: Bovine early development and sustainability, exploration and prediction
Participant: Patrick Bouthemy.
Duration: 12 months (2021 – 2022).
Funding: INRAE
Coordinator: Alain Trubuil (INRAe, MaIAGE Unit, Jouy-en-Josas)
Partners: INRAE (MaIAGE Unit, Jouy-en-Josas, MIA Unit Paris), UMR BREED (Ecole Nationale Vétérinaire d’Alfort), SERPICO Team
The current performance of in vitro fertilization and embryo transfer in cattle is around 30% of viable births. The selection of fertilized embryos is based on a classification at day 7 after fertilization. The objective of the consortium is to optimize the selection of embryos as early as possible by exploiting the morphokinetic history, from fertilization to the day of selection. For that, we want to gather methodological competencies around embryonic development, microscopy video analysis, machine learning and deep learning. We will organize one or more challenges in the context of the DATA-IA RAMP platform. This should allow us to involve other partners and students, to provide researchers in developmental biology with a state-of-the-art classification software, requiring as little expert annotation of videos as possible.
10.3.7 INRAE MreB project: Role of membrane microdomains in bacterial morphogenesis
Participant: Charles Kervrann.
Duration: 36 months (Oct 2020 – Sep 2023)
Funding: INRAE
Coordinator: Carballido-Lopez (INRAE UMR MICALIS, Jouy-en-Josas)
Partners: INRAE UMR MICALIS (Jouy-en-Josas), SERPICO Team
This project concerns the PhD thesis carried out by Claire-Jing Rochet (since October 2020). The goal is to understand how dynamic molecular interactions are regulated in time and in space to form functional machineries that establish long-range orders and cellular functions. To this end we combine cutting-edge high-resolution fluorescence microscopy and spectroscopy techniques with powerful genetic, biophysical, biochemical and systems biology approaches available in model bacteria, in particular the rod-shaped Gram-positive bacterium Bacillus subtilis, to determine mechanistic details underlying basic cellular and developmental processes: the molecules, macromolecular assemblies, cellular events and physiological adaptations involved. Understanding how bacterial cells grow has important implications for the identification of new antimicrobial targets and for the development of synthetic biology tools for bioproduction and biotechnology purposes.
10.3.8 MuDIS4LS project: Mutualised Digital Spaces for FAIR data in Life and Health Science
Participant: Jean Salamero.
Duration: (2021- )
Funding: ANR (ESR/Equipex+ PIA)
Coordinator: INBS Institut Français de Bioinformatique (IFB)
The national project MuDIS4LS brings together 39 teams from 14 organisations, 4 national data centers, 7 regional data centers, and 6 national data-producing infrastructures. Jean Salamero particitates to the IS-1 program on behalf of France BioImaging (design and implementation of a "BioImaging Structure DMP" on behalf of FBI, with the INBS EMBRC-FR and IFB, see tutorial).
10.4 Regional initiatives
10.4.1 Deep-SuN – Localization of chromosomal aberrations induced by nuclear radiation dose excess
Participants: Antonin Deschemps, Charles Kervrann.
Participants: Antonin Deschemps, Charles Kervrann.
Funding: IRSN (Institut de Radioprotection et Sureté Nucléaire) and Région-Bretagne
Duration: 36 months (Oct 2020 – Sep 2023)
This project concerns the PhD thesis carried out by Antonin Deschemps, in collaboration with IRSN/LRACC. See Sections 10.3.3 and 8.2.1 for more information.
11 Dissemination
Participants: Charles Kervrann, Patrick Bouthemy, Anaïs Badoual, Frédéric Lavancier, Ludovic Leconte, Sylvain Prigent, Emmanuel Moebel, Cesar Augusto Valades-Cruz, Sébastien Herbreteau.
11.1 Promoting scientific activities
11.1.1 Scientific events: organization
Member of the organizing committees
-
–
Charles Kervrann and Jean Salamero were members of the program and organization committee of the international "BioImage Informatics" conference, Institut Pasteur, on-line, November 29-December 1, 2021.
-
–
Jean Salamero was:
-
co-organizer of the first meeting (online) and workshop "Imaging Africa" (in collaboration with GBI, HHMI Janelia AIC, Zewail City of Science and Technology, Institut Pasteur, Dakar, University of Ghana, WACCBIP, Stellenbosch University, University of Cape Town), January 13-17, 2021;
-
organizer of the “Meetings of the INBS (National Research Infrastructures in Biology and Health) Club”, every 1 to 2 months, since December 2020;
-
co-organizer of the symposium "Research Infrastructures and Health Industry", with the ENRIITC network, the MESRI -DGRI and I2BC, October 2021 ("Les RDV Carnot", Lyon, October 17-18: participation in the "village des infras");
-
president of the organizing committee of the first Symposium of the CLUB des INBS: "Data from National Infrastructures in Biology and Health: stakes and perspectives", Sorbonne University, Paris, December 16-17, 2021.
-
-
–
Frédéric Lavancier is co-organizer of the online "seminar on spatial and spatiotemporal point processes and beyond” (OSSP).
11.1.2 Scientific events: selection
Reviewer
-
–
Anaïs Badoual was reviewer for IEEE ISBI’2021.
11.1.3 Journal
Member of the editorial boards
-
–
Charles Kervrann is Associate Editor for the "Transactions on Image Processing" and "Biological Imaging" journals since 2021.
Reviewer - reviewing activities
-
–
Charles Kervrann and Emmanuel Moebel were reviewers for Nature Communications.
-
–
Jean Salamero was reviewer for Nature Methods, Bioinformatics, and Journal of Cell Science.
-
–
Anaïs Badaoual was reviewer for Journal of Computational and Applied Mathematics.
-
–
Frédéric Lavancier was reviewer for Electronic Journal of Statistics, Scandinavian Journal of Statistics, Spatial Statistics, Journal of the Royal Statistical Society: Series C, and Stat, ESAIM: P&S.
-
–
Sylvain Prigent was reviewer for IEEE Transactions on Image Processing and Nature Scientific Reports.
-
–
Cesar Augusto Valades-Cruz was reviewer for Progress in Biophysics and Molecular Biology, PLOS Computational Biology and Journal of Physical Chemistry Letters.
11.1.4 Invited/Selected talks
-
–
Jean Salamero:
-
"Presentation of the ABIC consortium", symposium on "Innovation-Education-Sciences", invited speaker on behalf of France bioimaging and of the INBSs Club " Initiatives of infrastructures in biology and health in France and towards Africa ", New Africa-France-AFRICA 2021 summit, Montpellier, October 4, 2021.
-
-
–
Anaïs Badoual:
-
“Active subdivision surfaces for the segmentation of biomedical volumes” (Invited Talk), online Seminars on Numerical Approximation and Applications (OSNA2), April 28, 2021.
-
“Generation of synthetic datasets of astrocytic calcium activity to support the development of learning based analysis tools” (Invited Talk), Workshop proposal for the Virtual Conference of the European Society for Neurochemistry, May 25-26, 2021.
-
“Simulation of astrocytic calcium signals in microscopy images based on kinetic models” (Selected Talk), online Symposium on “Spatiotemporal Encoding and Decoding in Cell Signaling” organized by the Multi-Organization Thematic, Cell Biology, Development and Evolution (ITMO - BCDE), March 18, 2021.
-
-
–
Frédéric Lavancier:
-
"Birth-death-move processes: basic properties and estimation of their intensity functions”, conference Set Estimation, virtual meeting, Luminy, France, March 8, 2021.
-
"Birth-death-move processes: basic properties and estimation of their intensity functions”, Laboratoire de Mathématiques Jean-Leray Seminar, Nantes, April 20, 2021.
-
"Birth-death-move processes: basic properties and estimation of their intensity functions”, Toulouse Schools of Economics Seminar, Toulouse, October 7, 2021
-
-
–
Sylvain Prigent:
-
"BioImageIT: Integration of data management with analysis", online Curie seminar, May 10, 2021.
-
-
–
Emmanuel Moebel:
-
"DeepFinder", online I2PC Seminar series on ImageProcessing, Spain, June 3, 2021.
-
-
–
Sébastien Herbreteau:
-
"Image denoising: from traditional methods to neural networks", IRMAR Seminar (Statistics Team), October 29, 2021.
-
11.1.5 Leadership within the scientific community
-
–
Patrick Bouthemy is member of the board of AFRIF (Association Française pour la Reconnaissance et l’Interprétation des Formes).
-
–
Jean Salamero is Mission Officer appointed by the Executive Board of FBI, in charge of:
-
"activities and reorganization of the FBI IdF-SUD and Paris-Centre Nodes (preparation for and integration of the Genethon and IGR imaging platforms (IBiSA accredited in 2021) into the IdF-SUD node of FBI; integration of the IMAGI'C platform of the Cochin Institute and the Collège de France part of IMACHEM into FBI Paris-Centre);
-
"activities and inter-Research Infrastructures animation in Biology and Health" (creation and animation of the "Club des INBS”, 2020-2021).
-
-
–
Frédéric Lavancier is member of the board of the CNRS GdR GeoSto (3477 - Géométrie stochastique).
11.1.6 Scientific expertise
-
–
Jean Salamero regular reviewer for ANR, ITMO Cancer, Inca, ARC, Conseil Régional (Ile de France), ERC, FNS (Switzerland), NOW (The Netherands).
-
–
Frédéric Lavancier was reviewer for ANR, FNRS (Belgium) and IRGA (Initiatives de Recherche à Grenoble Alpes).
11.1.7 Research administration
-
–
Charles Kervrann:
-
Member of the executive board of the project committee "Bureau du Comité des Projets" of the Inria Rennes - Bretagne Atlantique centre since 2010.
-
Co-head of the "BioImage Informatics" node (ANR France-BioImaging project, National Research Infrastructure" for Biology and Health) since 2011.
-
-
–
Patrick Bouthemy:
-
Head of Excellence Lab (Labex) CominLabs since April 2014.
-
Deputy member of the board of directors and member of the Selection and Validation Committee of the Images & Réseaux competitivity cluster.
-
Deputy member of the board of directors of the IRT b<>com.
-
Head of the ANR evaluation committee for the 2021 call for proposals regarding the Artificial Intelligence field.
-
Member of the steering committee of the medical imaging Neurinfo platform.
-
-
–
Jean Salamero:
-
Member of the Advisory Committee for the creation of the National Biomedical Imaging Center (NBIC@Beijing, Republic of China) since 2017.
-
Member of the Advisory Board of EMBL Core Facilities.
-
Member of the Steering Committee of the DIM-ELICIT program (Région Ile de France) since 2016.
-
Member of the Qlife “Institut Convergences” since 2018.
-
11.2 Teaching - Supervision - Juries
11.2.1 Teaching
-
–
Charles Kervrann:
-
Master 2: "From Bioimage Processing to BioImage Informatics", 3 hours (4.5 hours TD), coordinator of the module (30 hours / equiv 45 hours TD), Master 2 Research IRIV, Telecom-Physique Strasbourg and University of Strasbourg.
-
Master 2: "Analysis of Image Sequence", 6 hours (9 hours TD), Master 2 Research SISEA, University of Rennes 1.
-
Engineer Degree (3rd year) and Master 2 Statistics and Mathematics: Statistical Models and Image Analysis", 27.5 hours (41.25 hours TD), Ecole Nationale de la Statistique et de l'Analyse de l'Information (ENSAI), Bruz.
-
-
–
Anaïs Badoual:
-
Engineer Degree (3rd year): "Statistical Models and Image Analysis", 15 hours (TP / Practical courses), Ecole Nationale de la Statistique et de l'Analyse de l'Information (ENSAI), Bruz.
-
PhD degree: "BioImage Analysis", 6 hours (TD), Gothenburg University, Sweden.
-
Interdisciplinary School Mifobio CNRS: "Practice Deep Learning Without Coding", November 5-12 2021, 4 sessions of 2.5 hours (lectures, practice).
-
-
–
Frédéric Lavancier:
-
Master degree : "Linear and Logistic Regression Models" 64 hours (96 hours TD), University of Nantes.
-
Master degree: "High Dimensional Statistics, 56 hours (84 Hours TD), University of Nantes.
-
Master degree: "Machine Learning", 15 hours (22.5 hours TD), University of Nantes.
-
-
–
Sylvain Prigent and Ludovic Leconte:
-
Interdisciplinary School Mifobio CNRS: "BioImageIT: Integration of Data Management with Analysis ", November 5-12 2021, 3 sessions of 2.5 hours (lectures, practice).
-
-
–
Jean Salamero, Ludovic Leconte, and Cesar Augusto Valades-Cruz:
-
France BioImaging Light-Sheet Fluorescence Microscopy (LSFM) Online Training 2021, February 15 - March 5, 2021 (lecture videos).
-
11.2.2 Supervision
-
–
Yunjiao Lu (PhD thesis defended in June 2021): "Intracellular dynamics and super-resolution imaging: analysis of bacteria wall at the molecular scale" (supervised by C. Kervrann, R. Carballido-Lopez, and A. Trubuil (INRAE, Jouy-en-Josas)).
-
–
Antoine Salomon (PhD in progress): "Statistical aggregation for image analysis in fluorescence microscopy and super-resolution" (started in November 2017, supervised by C. Kervrann).
-
–
Gwendal Fouché (PhD in progress): "Immersive interaction and visualization of temporal 3D data" (started in October 2019, supervised by C. Kervrann, F. Argelaguet (EPC HYBRID, Inria, Rennes), and E. Faure (LIRMM, Montpellier)).
-
–
Sébastien Herbreteau (PhD in progress): "Deep Learning for image restoration in night conditions" (started in october 2020, supervised by C. Kervrann).
-
–
Antonin Deschemps (PhD in progress): "Statistical learning for localization and classification of chromosomal aberrations induced by nuclear radiation dose excess" (started in October 2020, supervised by C. Kervrann and M.A. Benadjaoud (IRSN, Fontenay-aux-Roses)).
-
–
Etienne Meunier (PhD in progress): "Deep Learning for saliency detection in image sequences" (started in September 2020, supervised by P. Bouthemy).
-
–
Claire-Jing Rouchet (PhD in progress): "Role of membrane microdomains in bacterial morphogenesis" (started in October 2020, supervised by R. Carballido-Lopez, C. Billaudeau (INRAE MICALIS Unit, Jouy-en-Josas), and C. Kervrann).
-
–
Quentin Tallon (PhD in progress): "Statistical learning for localization and classification of chromosomal aberrations in FISH microscopy images" (started in october 2021, supervised by C. Kervrann and M.A. Benadjaoud (IRSN/LRACC, Fontenay-aux-Roses)).
-
–
Lisa Balsolier (PhD in progress): "Spatiotemporal stochastic models for the comprehension of membrane trafficking at the single cell scale" (started in october 2021, supervised by F. Lavancier, C. Kervrann, and J. Salamero).
11.2.3 Juries
-
–
Charles Kervrann:
-
President of the PhD thesis committee of A. Pirovano (Institut Polytechnique de Paris, Telecom Paris, supervised by I. Bloch and S. Ladjal).
-
-
–
Patrick Bouthemy:
-
President of the HdR committee of P. Maurel (University of Rennes 1).
-
President of PhD thesis committees of M. Fleury (University of Rennes 1, supervised by A. Lecuyer and C. Barillot), and H. Zhang (University of Rennes 1, supervised by E. Fromont and S. Lefèvre).
-
-
–
Frédréric Lavancier:
-
Member of the PhD thesis committee of M. Raizi (University of Avignon, supervised by F. Bonneu and E. Gabriel).
-
12 Scientific production
12.1 Major publications
- 1 articleAdaptive spot detection with optimal scale selection in fluorescence microscopy images.IEEE Transactions on Image Processing2411November 2015, 16
- 2 articleAn extended model of vesicle fusion at the plasma membrane to estimate protein lateral diffusion from TIRF microscopy images.BMC Bioinformatics1812017, 352
- 3 articleA patch-based method for repetitive and transient event detection in fluorescence imaging.PLoS ONE510Oct 2010
- 4 articlePatch-based nonlocal functional for denoising fluorescence microscopy image sequences.IEEE Transactions on Medical Imaging292Feb 2010, 442-453
- 5 articleStatistical analysis of particle trajectories in living cells.Physical Review E 976June 2018, 1-20
- 6 article Fast live simultaneous multiwavelength four-dimensional optical microscopy.Proc Natl Acad Sci USA10737Sep 2010, 16016-16022
- 7 articleMotion Textures: Modeling, Classification, and Segmentation Using Mixed-State.SIAM Journal on Imaging Sciences64December 2013, 2484-2520
- 8 inproceedingsPEWA: Patch-based Exponentially Weighted Aggregation for image denoising.NIPS - Neural Information Processing SystemsNeural Information Processing Systems FoundationMontreal, CanadaDecember 2014
- 9 articleBackground Fluorescence Estimation and Vesicle Segmentation in Live Cell Imaging with Conditional Random Fields.IEEE Transactions on Image Processing242February 2015, 14
- 10 articleCounting-based particle flux estimation for traffic analysis in live cell imaging.IEEE Journal of Selected Topics in Signal ProcessingSeptember 2015
12.2 Publications of the year
International journals
- 11 articleActive Subdivision Surfaces for the Semiautomatic Segmentation of Biomedical Volumes.IEEE Transactions on Image Processing302021, 5739-5753
- 12 articleThe coordination of spindle-positioning forces during the asymmetric division of the C. elegans zygote.EMBO Reports225April 2021, e50770
- 13 articleLoss of light colour preference after chronic embryonic stress in rainbow trout fry: a novel and potential indicator of fish welfare?Applied Animal Behaviour Science239April 2021, 105335
- 14 articleQuantitative dSTORM super-resolution microscopy localizes Aurora kinase A/AURKA in the mitochondrial matrix.Biology of the CellAugust 2021
- 15 articleThe final twist in endocytic membrane scission.Nature Cell Biology238August 2021, 812-813
- 16 articleAdaptive estimating function inference for non-stationary determinantal point processes.Scandinavian Journal of Statistics481March 2021, 87-107
- 17 articleProbabilistic reconstruction of truncated particle trajectories on a closed surface.Multiscale Modeling and Simulation: A SIAM Interdisciplinary Journal191January 2021, 87-112
- 18 articleTrajectory saliency detection using consistency-oriented latent codes from a recurrent auto-encoder.IEEE Transactions on Circuits and Systems for Video Technology2021
- 19 articleDeep Learning Improves Macromolecule Identification in 3D Cellular Cryo-Electron Tomograms.Nature Methods1811October 2021, 1386-1394
- 20 article4polar-STORM polarized super-resolution imaging of actin filament organization in cells.Nature Communications13January 2022
International peer-reviewed conferences
- 21 inproceedingsSimulation of Astrocytic Calcium Dynamics in Lattice Light Sheet Microscopy Images.ISBI 2021 - IEEE International Symposium on Biomedical ImagingNice, FranceIEEEApril 2021, 1-5
- 22 inproceedingsUnsupervised computation of salient motion maps from the interpretation of a frame-based classification network.BMVC 2021 - 32nd British Machine Vision Conferencevirtual conference, United KingdomNovember 2021, 1-12
National peer-reviewed Conferences
- 23 inproceedingsLocalisation de mouvements saillants dans des cartes de flot optique par l'interprétation d'un réseau de classification.ORASIS 2021 - 18ème édition des journées francophones des jeunes chercheurs en vision par ordinateurSaint Ferréol, FranceSeptember 2021, 1-8
Conferences without proceedings
- 24 inproceedingsBioImageIT: Integration of image data-management with analysis.BioImage Informatics 2021 (poster)Paris, FranceNovember 2021, 1
- 25 inproceedingsDeep Learning Improves Macromolecule Identification in 3D Cellular Cryo-Electron Tomograms.BioImage Informatics 2021 (selected talk)Paris, FranceNovember 2021
- 26 inproceedingsSPITFIR(e): a supermaneuverable image restoration algorithm for 2D, 3D and 3D+Time microscopy images.BioImage Informatics 2021 (poster)Paris, FranceNovember 2021
Doctoral dissertations and habilitation theses
- 27 thesisIntracellular dynamics and super-resolution imaging: the bacterial wall probed at the molecular scale.Université de Rennes 1June 2021
Reports & preprints
- 28 miscLocal temporal image correlation spectroscopy and Bayesian simulation technique for sparse estimation of diffusion in fluorescence imaging.January 2022
- 29 miscAdvocating for interdisciplinary collaborations to unravel the astrocyte "Calcium Code".January 2022
- 30 miscDCT2net: an interpretable shallow CNN for image denoising.January 2022
- 31 miscBiomolecule Trafficking and Network Tomography-based Simulations.January 2022
- 32 miscEM-driven unsupervised learning for efficient motion segmentation.January 2022
- 33 miscA comparison of high resolution image reconstruction methods from confocal array detector with a new deconvolution algorithm.January 2022
- 34 miscSPITFIR(e): A supermaneuverable algorithm for restoring 2D-3D fluorescence images and videos, and background subtraction.January 2022
- 35 miscBioImageIT: Open-source framework for integration of image data-management with analysis.December 2021
12.3 Cited publications
- 36 articleThe Cell Wall of Bacillus subtilis.Curr Issues Mol Biol412011, 539--596
- 37 articleTotal Internal Reflection Fluorescent Microscopy in cell biology.Traffic22004, 4658--4668
- 38 articleImaging intracelluar fluorescent proteins at nanometer resolution.Science3132006, 1642--1645
- 39 articleA sequential algorithm to detect diffusion switching along intracellular particle trajectories.BioinformaticsJune 2019, 1-13
- 40 articleLattice light-sheet microscopy: Imaging molecules to embryos at high spatiotemporal resolution.Science34662082014, 1257998
- 41 articleImage denoising by sparse 3-d transform-domain collaborative filtering.IEEE Trans. Image Processing1682007, 2080--2095
- 42 inproceedingsPatch-based Markov models for event detection in fluorescence bioimaging.Int. Conf. on Medical Image Computing and Computer Assisted Intervention (MICCAI'08)2New York City, USASeptember 2008, 95--103
- 43 inproceedingsDense mapping of intracellular diffusion and drift from single-particle tracking data analysis.ICASSP 2020 - IEEE International Conference on Acoustics, Speech and Signal ProcessingBarcelona, SpainIEEEMay 2020, 1-5
- 44 article LipidII: Just Another Brick in the Wall ? PLoS Pathogens 11 2015
- 45 articleContent-aware image restoration: pushing the limits of fluorescence microscopy.Nature Methods122018, 1090-1097
- 46 articleBeyond a gaussian denoiser: residual learning of deep CNN for image denoising.IEEE Transactions on Image Processing2672017, 3142--3155