Keywords
Computer Science and Digital Science
- A3.1.1. Modeling, representation
- A3.3. Data and knowledge analysis
- A3.3.3. Big data analysis
- A3.4. Machine learning and statistics
- A3.4.1. Supervised learning
- A3.4.5. Bayesian methods
- A3.4.6. Neural networks
- A3.4.7. Kernel methods
- A3.4.8. Deep learning
- A5.3. Image processing and analysis
- A5.3.2. Sparse modeling and image representation
- A5.3.3. Pattern recognition
- A5.3.4. Registration
- A5.4.1. Object recognition
- A5.4.4. 3D and spatio-temporal reconstruction
- A5.4.5. Object tracking and motion analysis
- A5.4.6. Object localization
- A5.9.1. Sampling, acquisition
- A5.9.2. Estimation, modeling
- A5.9.3. Reconstruction, enhancement
- A5.9.5. Sparsity-aware processing
- A5.9.6. Optimization tools
- A6.1.2. Stochastic Modeling
- A6.1.3. Discrete Modeling (multi-agent, people centered)
- A6.1.4. Multiscale modeling
- A6.1.5. Multiphysics modeling
- A6.2.3. Probabilistic methods
- A6.2.4. Statistical methods
- A6.2.6. Optimization
- A6.3. Computation-data interaction
- A6.3.1. Inverse problems
- A6.3.2. Data assimilation
- A6.3.3. Data processing
- A6.3.4. Model reduction
- A6.3.5. Uncertainty Quantification
- A9.2. Machine learning
- A9.3. Signal analysis
Other Research Topics and Application Domains
- B1.1.1. Structural biology
- B1.1.7. Bioinformatics
- B1.1.8. Mathematical biology
- B2.2.3. Cancer
- B2.6. Biological and medical imaging
1 Team members, visitors, external collaborators
Research Scientists
- Charles Kervrann [Team leader, Inria, Senior Researcher, HDR]
- Anaïs Badoual [Inria, Researcher]
- Patrick Bouthemy [Inria, Senior Researcher, HDR]
- Jean Salamero [CNRS, Senior Researcher, Institut Curie, HDR]
Post-Doctoral Fellows
- Emmanuel Moebel [Inria]
- Cesar Augusto Valades-Cruz [CNRS, Institut Curie, until Janv 2023]
PhD Students
- Lisa Balsollier [CNRS]
- Antonin Deschemps [Inria]
- Gwendal Fouche [Inria]
- Sébastien Herbreteau [Inria]
- Etienne Meunier [Inria]
- Quentin Rapilly [Inria, from Dec 2022]
- Claire-Jing Rouchet [INRAe]
- Quentin Tallon [IRSN]
Technical Staff
- Kevin Fournier [Inria, Engineer, CDD (IE), jointly with Hybrid Team]
- Ludovic Leconte [CNRS, Engineer, permanent (IR), Institut Curie]
- Léo Maury [CNRS, Engineer, CDD (IE), Institut Curie]
- Sylvain Prigent [Inria, Engineer, until Sep 2022, CDD (IR)]
Interns and Apprentices
- Constantin Aronssohn [Inria, Intern, from Apr 2022 until Jul 2022]
- Salomé Papereux [Inria, Intern, from May 2022, until Dec 2022]
Administrative Assistant
- Caroline Tanguy [Inria]
External Collaborator
- Frédéric Lavancier [University of Nantes, HDR]
2 Overall objectives
2.1 Glossary
- LLSM (Lattice Light Sheet Microscopy): high resolution Structured Illumination Microscopy that uses Light Sheet Bessel Beam illumination 39.
- TIRF (Total Internal Reflectance): 2D optical microscopy using evanescent waves and total reflectance 35.
- STORM (Stochastic Optical Reconstruction Microscopy): high-resolution microscopy using stochastic photo-activation of fluorophores and adjustment of point spread functions.
- PALM (Photo-Activated Localization Microscopy): high-resolution microscopy using stochastic photo-activation of fluorophores and adjustment of point spread functions 36.
- Cryo-ET (Cryo-Electron Tomography): 3D representation of sub-cellular and molecular objects of 5-20 nanometers, frozen at very low temperatures, from 2D projections using a transmission electron microscope.
2.2 Scientific context and motivations
During the past two decades, biological imaging has undergone a revolution in the development of new microscopy techniques that allow visualization of tissues, cells, proteins and macromolecular structures at all levels of resolution, physiological states, chemical composition and dynamics. Thanks to recent advances in optics, digital sensors and labeling probes (e.g., Colored Fluorescence Protein), one can now visualize sub-cellular components and organelles at the scale of several hundreds of nanometers to a tens nanometers, in live. As a result, fluorescent microscopy and multimodal imaging (fluorophores at various wavelengths) have become the workhorse of modern biology. As a matter of fact, taking into account all the publications in the 10 most relevant journals in fundamental biology (those with highest IF) for the last 2018-2019 years, the ratio of experimental figures based on BioImage data is close to 70% (GBI EoE.V, Singapour, Sep 2019). All the technological advances in microscopy have created new issues and challenges for researchers in quantitative image processing and analysis. Since the digital processing is now part of the imaging loop, image processing may even drive imaging. A brilliant example of this shift in paradigm is super-resolution localization microscopy (PALM, STED), which was awarded the 2014 Nobel Prize in Chemistry.
2.3 Challenges in biological image processing and quantitative microscopy
In most cases, modern microscopy in biology is characterized by a large number of dimensions that fit perfectly with the complexity of biological features: two or three spatial dimensions, at macro to nano-scales, and one temporal dimension, sometimes spectrally defined and often corresponding to one particular biomolecular species. Dynamic microscopy is also characterized by the nature of the observable objects (cells, organelles, single molecules, ...), by the large number of small size and mobile elements (chromosomes, vesicles, ...), by the complexity of the dynamic processes involving many entities or group of entities sometimes interacting, by particular phenomena of coalescence often linked to image resolution problems, finally by the association, dissociation, recomposition or constitution of those entities (such as membrane fusion and budding). Thus, the corpus of data to be considered for any analysis involving multiple image series acquisitions is massive (up to few GigaBytes per hour). Therefore, it becomes necessary to facilitate and rationalize the production of those multidimensional data, to improve post acquisition analysis, and to favor the organization and the interpretation of the information extracted from this data corpus. It motivates innovative methods and concepts for data fusion, image registration, super-resolution, data mining... More importantly, modern microscopy has led to recent breakthroughs, related to the potential interactions between molecules in the cell. A long-term research consists now in inferring the relationships between the dynamics of macromolecules and their functions. Research on computational biology and quantitative bioimaging lies at the core of the activities of SERPICO team.
2.4 Objectives of SERPICO in cell imaging
In order to tackle the aforementioned challenges, the SERPICO team aims to develop innovative approaches and paradigms for image reconstruction, 3D molecule tracking and motion estimation, and biophysical parameter estimation to face the huge data volumes acquired with cutting-edge microscopy set-ups. To this end, applied mathematics, image processing and analysis have to be considered in association with biophysics and biology. To be successful, a sustained synergy between all these scientific domains is necessary. To improve state-of-the-art methods and solve important problems in computational bioimaging, the members of SERPICO especially address the following topics:
- Image restoration/reconstruction motivated by preserving cell integrity (photo-toxicity versus exposure time) and image analysis in multidimensional microscopy;
- Motion analysis and computation of molecule trajectories in live-cell imaging to study molecular interactions in space and time;
- Computational simulation, modeling and estimation of molecule trafficking and interactions at different spatial and temporal scales.
The resulting mathematical models and algorithms will help biologists to decipher molecular processes in fundamental biology and will be exploited for health applications: disease diagnosis, detection of genomic instabilities, deterioration of cell cycle, cancer prevention.
We have successfully developed statistical and variational aggregation methods for image denoising and optical flow, and elaborated powerful methods for image colocalization, diffusion estimation, trajectory estimation-classification, and multimodal registration. An additional issue was the design and distribution of software tools for the biological image analysis and microscopy communities. Finally, the team has focused on the cellular and molecular mechanisms involved in molecule and protein transport and trafficking at the scale of a single cell. Our contributions are detailed in the next sections along three research axes.
2.5 Organization and collaborations
In collaboration with UMR 144 CNRS - Institut Curie (and in cooperation with the “Space Time imaging of Endomembranes and organelles Dynamics” team) and PICT-IBiSA (Cell and Tissue Imaging Facilities), the members of the SERPICO team have participated in several projects (PhD and post-doc supervision, contracts...) in the field of cell biology and microscopy. We have promoted non-parametric methods and developed user-friendly algorithms for processing and analyzing 2D/3D images and videos. The projects of SERPICO were in line with several studies led in the UMR 144 CNRS - Institut Curie Unit. A subset of studies was related to instrumentation in electronic and photonic microscopy (PICT-IBiSA platform) including computational aspects on the reconstruction and enhancement of images related to sub-diffraction light microscopy and multimodal approaches. SERPICO projects relied partially on the advances of these instrumental projects and a positive synergy was established.
3 Research program
3.1 Statistics and algorithms for computational microscopy
Fluorescence microscopy limitations are due to the optical aberrations, the resolution of the microscopy system, and the photon budget available for the biological specimen. Hence, new concepts have been defined to address challenging image restoration and molecule detection problems while preserving the integrity of samples. Accordingly, the main stream regarding denoising, deconvolution, registration and detection algorithms advocates appropriate signal processing framework to improve spatial resolution, while at the same time pushing the illumination to extreme low levels in order to limit photo-damages and phototoxicity. As a consequence, the question of adapting cutting-edge signal denoising and deconvolution, object detection, and image registration methods to 3D fluorescence microscopy imaging has retained the attention of several teams over the world.
In this area, the SERPICO team has developed a strong expertise in key topics in computational imaging including image denoising and deconvolution, object detection and multimodal image registration. Several methods proposed by the team outperformed several state-of-the-art algorithms, and some developments are compatible with “high-throughput microscopy” and the processing of several hundreds of cells. We especially promoted non local, non-parametric and patch-based methods to solve well-known inverse problems or more original reconstruction problems. A recent research direction consists in adapting the deep-learning concept to solve challenging detection and reconstruction problems in microscopy. We have investigated convolution neural networks to detect small macromolecules in 3D noisy electron images with promising results. The next step consists in proposing smart paradigms and architectures to save memory and computations.
More generally, many inverse problems and image processing become intractable with modern 3D microscopy, because very large temporal series of volumes (200 to 1000 images per second for one 3D stack) are acquired for several hours. Novel strategies are needed for 3D image denoising, deconvolution and reconstruction since computation is extremely heavy. Accordingly, we adapted the estimator aggregation approach developed for optical flow computation to meet the requirements of 3D image processing. We investigated regularization-based aggregation energy over super-voxels to reduce complexity, combined to modern optimization algorithms. Finally, we designed parallelized algorithms that fastly process 3D images, perform energy minimization in few seconds per image, and run on low-cost graphics processor boards (GPU).
3.2 From images to motion descriptors and trajectories
Several particle tracking methods for intracellular analysis have been tailored to cope with different types of cellular and subcellular motion down to Brownian single molecule behavior. Many algorithms were carefully evaluated on the particle tracking challenge dataset published in the Nature Methods journal in 2014. Actually, there is no definitive solution to the particle tracking problem, which remains application-dependent in most cases. The work of SERPICO in particle motion analysis is significant in multiple ways, and inserts within a very active international context. One of the remaining key open issues is the tracking of objects with heterogeneous movements in crowded configurations. Moreover, particle tracking methods are not always adapted for motion analysis, especially when the density of moving features hampers the individual extraction of objects of interest undergoing complex motion. Estimating flow fields can be more appropriate to capture the complex dynamics observed in biological sequences. The existing optical flow methods can be classified into two main categories: i/ local methods, imposing a parametric motion model (e.g. local translation) in a given neighborhood; ii/ global methods, estimating the dense motion field by minimizing a global energy functional composed of a data-fidelity term and a regularization term.
The SERPICO team has developed a strong expertise in key topics, especially in object tracking for fluorescence microscopy, optical flow computation and high-level analysis of motion descriptors and trajectories. Several algorithms proposed by the team are very competitive when compared to the state-of-the-art results, and our new paradigms offer promising ways for molecule traffic quantification and analysis. Among the problems that we currently address we can mention: computation of 3D optical flow for large-size images, combination of two frame-based differential methods and sparse sets of trajectories, detection and analysis of unexpected local motion patterns in global coherent collective motion. Development of efficient numerical schemes will be central in the future but visualization methods are also crucial for evaluation and quality assessment.
Another direction of research consists in exploiting deep-learning to 3D optical flow so as to develop efficient numerical schemes that naturally capture complex motion patterns. Investigation in machine learning and statistics are currently conducted in the team in the two first research axes to address a large range of inverse problems in bioimaging. Deep-learning is an appealing approach since expertise of biologists, via iterative annotation of training data, can be included in the design of image analysis schemes.
3.3 Biological and biophysical models and statistics for quantitative bioimaging
A number of stochastic mathematical models were proposed to describe various intracellular trafficking, where molecules and proteins are transported to their destinations via free diffusion, subdiffusion and ballistic motion representing movements along the cytoskeleton networks assisted by molecular motors 31. Accordingly, the study of diffusion and stochastic dynamics has known a growing interest in bio-mathematics, biophysics and cell biology with the popularization of fluorescence dynamical microscopy and super-resolution imaging. In this area, the competing teams mainly studied MSD and fluorescence correlation spectroscopy methods.
In the recent period, the SERPICO team achieved important results for diffusion-related dynamics involved in exocytosis mechanisms. Robustness to noise has been well investigated, but robustness to environmental effects has yet to be effectively achieved. Particular attention has been given to the estimation of particle motion regime changes, but the available results are still limited for analyzing short tracks. The analysis of spatiotemporal molecular interactions from sets of 3D computed trajectories or motion vector fields (e.g., co-alignment) must be investigated to fully quantify specific molecular machineries. We have already made efforts in these directions this year (e.g., for colocalization) but important evaluation on real microscopy image datasets are required to make our preliminary algorithms reliable enough and well adapted to specific transport mechanisms.
Accordingly, we study quantification methods to represent interactions between molecules and trafficking around three lines of research. First, we focus on 3D space-time global and local object-based co-orientation and co-alignment methods, in the line of previous work on colocalization, to quantify interactions between molecular species. In addition, given tracks associated to molecular species, interaction descriptors, dynamics models and stochastic graphical models representing molecular machines are studied in the statistical data assimilation framework. Second, we currently develop approaches to estimate molecular mobility, active transport and motion regime changes from computed trajectories in the Lagrangian and Eulerian settings. We focus on the concept of super-resolution to provide spatially high-resolved maps of diffusion and active transport parameters based on stochastic biophysical models and sparse image representation. Third, we plan to extend the aggregation framework dedicated to optical flow to the problem of diffusion-transport estimation.
4 Application domains
4.1 Modeling and analysis of membrane transport and molecule trafficking at the single cell scale
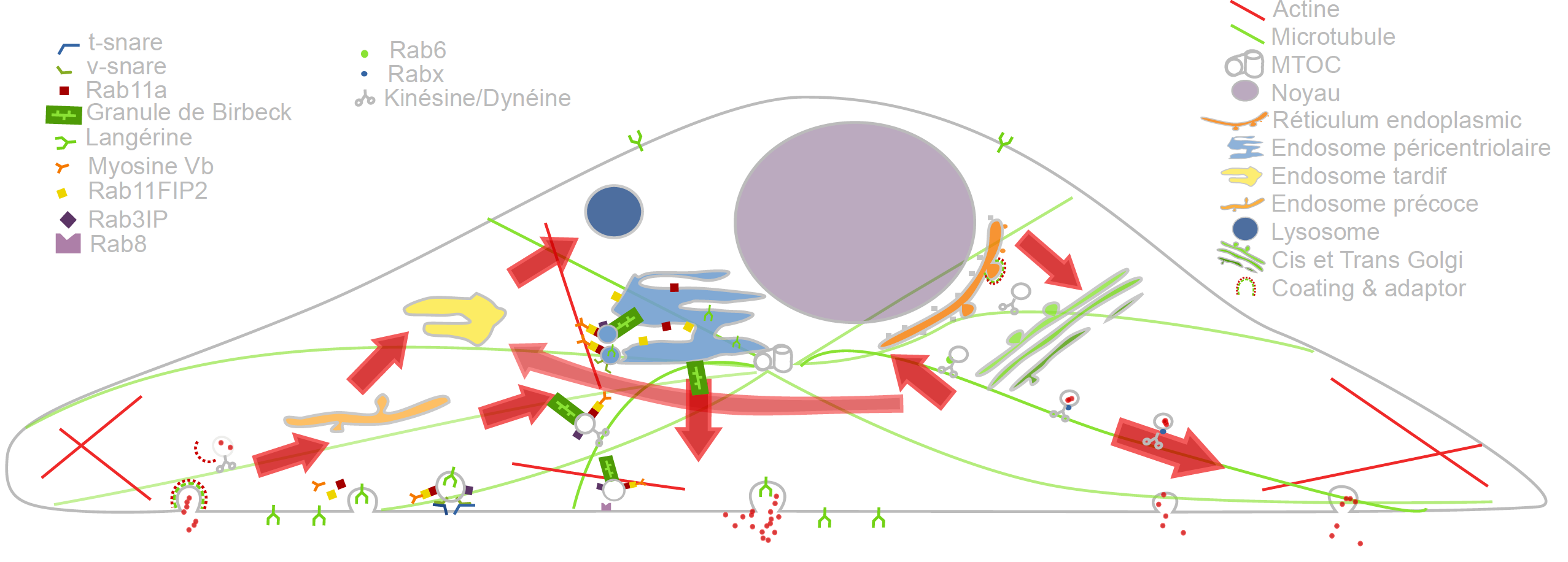
Cargo Langerin Trafficking controlled by Rab11A/Rab11FIP2/MyoVb platform.
In the past recent years, research carried out together with the “Space Time imaging of Endomembranes and organelles Dynamics” team at UMR 144 CNRS - Institut Curie contributed to a better understanding of the intracellular compartmentation, particularly in specialized model cells such as melanocytes and Langerhans cells of the epidermis, of the components and structural events involved in the biogenesis of their specialized organelles: melanosomes and Birbeck granules, respectively and to the understanding on how the dynamics of those structures relate to their physiological functions. These studies have started to highlight: i/ the measurement of multiple sorting and structural events involved in the biogenesis of these organelles; ii/ complexity of the endo-melanosomal network of these highly specialized cells; iii/ complex molecular architecture organizing and coordinating their dynamics; iv/ intracellular transport steps affected in genetic diseases, among which the Hermansky Pudlak syndrome (HPS) or involved in viral infection (HIV and Langerin in Langerhans cells).
In this context, the central aim of SERPICO is to understand how the different machineries of molecular components involved are interconnected and coordinated to generate such specialized structures, an issue that becomes more and more accessible, thanks to improvement in all domains related to live imaging. We need to address the following topics:
- developing new bioimaging approaches to observe and statistically analyze such coordinated dynamics in live material;
- correlating this statistically relevant spatiotemporal organization of protein networks with the biological architectures and at the ultrastructural level;
- modeling intracellular transport of those reference biological complex systems and proposing new experimental plans in an iterative and virtuous circle;
- managing and analyzing the workflow of image data obtained along different multidimensional microscopy modalities.
These studies are essential to unravel the complexity of the endomembrane system, how different machineries evolve together and thus coordinate (e.g. see Fig. 1). They help to decipher cell organization and function at different scales through an integrative workflow of methodological and technological developments. New approaches, such as optogenetics may even help controlling cell functions.
At long term, these studies will shed light on the cellular and molecular mechanisms underlying antigen presentation, viral infection or defense mechanisms, skin pigmentation, the pathogenesis of hereditary genetic disorders (lysosomal diseases, immune disorders) and on the mechanisms underlying cell differentiation and cell transformation. Our methodological goal is also to link dynamics information obtained through diffraction limited light microscopy, at a time regime compatible with live cell imaging and close to biochemical molecular interactions. The overview of ultrastructural organization will be achieved by complementary electron microscopy methods which have also undergone a revolutionary improvement over the last decade. Image visualization and quantitative analysis are of course essential issues in this context.
4.2 Imaging and analysis of cytoskeleton dynamics during cell migration
The ability to migrate in space is among the most fundamental functions of eukaryotic cells and thus is one of the best-studied phenomena in biology. During embryonic development, cell movements result in a massive reorganization of the embryo, from a simple spherical ball of cells into a multi-layered organism; many of the cells at or near the surface of the embryo move to a new, more interior location. Moreover, inadequate or inappropriate migration of immune cells is also critically important for the delivery of protective immune responses to tissues and for wound healing. Finally, cell migration may facilitate the dissemination of tumor cells from primary tumors in blood (extravasation) and eventually the colonization of other organs and the formation of secondary tumors.
It has been established that the cytoskeleton, composed of actin filaments, microtubules and intermediate filaments (elongated structures with a diameter of a few dozens of nanometers), is essential for several cell mechanisms, including cell migration, cell division and molecule trafficking:
- i/ the actin filaments promote cell protrusion, adhesion and retraction;
- ii/ the microtubules are the support of molecule traffic and cell polarization;
- iii/ the intermediate filaments are hypothesized to control microtubule organization.
Nevertheless, the mechanical and chemical states of migrating cells under various external conditions remain largely unknown. In the last decade, high-resolution microscopy methods led to the discovery of novel aspects of cell migration. Most approaches and models are limited to migration in 2D, justified by the flatness of the cell-motile mechanisms. However, the mechanical patterns that govern migration in 2D models are often not essential for efficient migration in 3D. Accordingly, recent very challenging 3D models of cells moving on flat surfaces have begun to emerge. The key challenge, however, is to understand how a 3D motile cell crawls through the 3D extracellular matrix. Another issue is of course to measure and understand how membrane protrusion and retraction keep the cell in homeostasis, which of course relate to membrane traffic.
The objective of SERPICO is to develop high-end signal processing and computer vision tools to unfold the dynamical coordination of microtubules, actin filaments and intermediate filaments in 3D, involved in cell migration, cell division and how molecular trafficking is coordinated with cytoskeleton changes in these fundamental cellular functions.
5 Social and environmental responsibility
Application of general recommendations related to transport (train, visioconferences). Participation in presential mode to conferences, workshops, and meetings, were limited to events organized in France in 2022. The team essentially published its results via the submission to international journals. This has led to a few travels by train, and then a low carbon footprint for the team in 2022.
6 Highlights of the year
The paper entitled "BioImageIT: Open-source framework for integration of image data management with analysis" has been published in Nature Methods journal (Impact Factor: 50) in October 2022.
7 New software and platforms
7.1 New software
7.1.1 GcoPS
-
Name:
Geo-Co-Positioning System for co-localization of image pairs of fluorescent molecules
-
Keywords:
Photonic imaging, Fluorescence microscopy, Image processing, Statistic analysis
-
Functional Description:
The GcoPS (Geo-Co-Positioning System) software is dedicated to the co-localization of fluorescence image pairs for both conventional and super-resolution microscopy. The procedure is only controlled by a p-value (type I error-rate) and tests whether the Pearson correlation between two binary images is significantly positive. Colocalization amounts here to quantifying the interaction strength by the area/volume of the intersection between the two binary images viewed as random distributions of geometrical objects. Under mild assumptions, it turns out that the appropriately normalized Pearson correlation follows a standard normal distribution under the null hypothesis if the number of image pixels is large. Unlike previous methods, GcoPS handles 2D and 3D images, variable SNRs and any kind of cell shapes. It is able to co-localize large regions with small dots, as it is the case in TIRF-PALM experiments and to detect negative co-localization. The typical processing time is two milliseconds per image pair in 2D and a few seconds in 3D, with no dependence on the number of objects per image. In addition, the method provides maps to geo-co-localize molecule interactions in specific image regions.
- URL:
- Publication:
-
Contact:
Charles Kervrann
-
Participants:
Thierry Pécot, Frédéric Lavancier, Charles Kervrann, Liu Zengzhen
-
Partners:
Université de Nantes, UMR 144 CNRS - Institut Curie, Hollings Cancer Center at the Medical University of South Carolina, Charleston SC, USA
7.1.2 AiryscanJ
-
Name:
Reconstruction of fluorescence microscopy images from confocal array detectors
-
Keywords:
Image reconstruction, Fluorescence microscopy, Photonic imaging, Deconvolution, Image analysis
-
Functional Description:
The AiryscanJ software enables to reconstruct a high resolution image from an array of multiple raw images acquired with the Airyscan technology (32 detectors). Airyscanning is a recent technique based on confocal laser scanning microscopy. The AiryscanJ software gathers four reconstruction methods (ISM, IFED, ISFED, ISM-Deconvolution) to compute a high resolution image: 1/ ISM amounts to summing the preliminarily registered raw images. 2/ IFED is the weighted difference between the inner detectors and the outer detector. 3/ ISFED is the weighted difference between the registered outer detectors and the original outer detectors. AiryscanJ automatically estimates the parameter controlling the IFED and ISFED algorithms. 4/ ISM-Deconvolution allows reconstructing a high resolution image by applying a deconvolution algorithm on the ISM image.
Inter Deposit Digital Number: IDDN.FR.001.490022.000.S.P.2021.000.21000
- URL:
- Publications:
-
Contact:
Charles Kervrann
-
Participants:
Sylvain Prigent, Stephanie Dutertre, Charles Kervrann
-
Partners:
Université de Rennes 1, CNRS
7.1.3 DeepFinder
-
Name:
Deep learning for macromolecule identification within 3D cellular cryo-electron tomograms
-
Keywords:
Image analysis, Deep learning, Cryo-electron microscopy, Object detection
-
Functional Description:
DeepFinder is a computational approach that uses artificial neural networks to accurately and jointly localize multiple types and/or states of macromolecules in 3D cellular cryo-electron tomograms. DeepFinder leverages deep learning and outperforms the commonly-used template matching method on ideal data. On synthetic image data (SHREC 2019, 2020, and 2021 challenges), DeepFinder is very fast and produces superior detection results when compared to other competitive deep learning methods, especially on small macromolecules. On experimental cryo-ET data depicting ribosomes, the detection results obtained by DeepFinder are consistent with expert annotations. We have got a high overlap of detection (86%) and a similar structure resolution that those determined by subtomogram averaging.
Inter Deposit Digital Number: IDDN.FR.001.460030.000.S.P.2021.000.21000
- URL:
- Publication:
-
Contact:
Emmanuel Moebel
-
Participants:
Emmanuel Moebel, Charles Kervrann
-
Partners:
Max Planck Institute Martinsried, Fondation Fourmentin-Guilbert, Helmholtz Pioneer Campus, Neuherberg, Germany
7.1.4 FlowScope
-
Name:
Optical flow computation for 3D fluorescence microscopy
-
Keywords:
Motion analysis, Fluorescence microscopy, Image analysis, Data visualization
-
Functional Description:
The FLOWSCOPE software is able to estimate 3D motion between two fluorescence microscopy volumes. The underlying variational method amounts to minimizing an energy functional made up of two terms: a data term and a regularization term. The data term is derived from the continuous form of Census signature and the smoothness of the flow field is imposed by a L2 regularization term. The method is implemented for a single core CPU. FLOWSCOPE outputs three separate files corresponding to the motion vector components. The flow fields can be visualized with an appropriate color code named 3PHS. The 3PHS map projects the flow field onto the three orthogonal planes selected by the user. The projections of the vector field is color coded in the Hue (direction) and the Saturation (amplitude) spaces.
- URL:
- Publication:
-
Contact:
Patrick Bouthemy
-
Participants:
Sandeep Manandhar, Patrick Bouthemy, Sylvain Prigent, Leo Maury, Philippe Roudot, Charles Kervrann
-
Partners:
UMR 144 CNRS - Institut Curie, Danuser lab, University of Texas Southwestern, Dallas, USA
7.1.5 DCT2Net
-
Name:
Trained shallow CNN (convolution neural network)-based DCT (Discrete Cosine Transform) denoiser
-
Keywords:
Deep learning, Denoising
-
Functional Description:
DCT2net software, based on the well-known DCT (Discrete Cosine Transform) image denoising algorithm, is dedicated to noise removal from images. The traditional DCT denoiser can be seen as a shallow CNN and thereby its original linear transform can be tuned through gradient descent in a supervised manner, improving considerably its performance. Consequently, DCT2net is a shallow and interpretable convolution network, whose parameters optimization allows to improve very significantly the performances of the traditional DCT denoiser. To deal with the remaining artifacts induced by DCT2net, an original hybrid solution between DCT and DCT2net is proposed, combining the best of what these two methods can offer. Experiments on artificially noisy images show that the two-layer DCT2net method provides results comparable to the BM3D method and is as fast as the DnCNN algorithm composed of more than a dozen of layers.
Inter Deposit Digital Number: IDDN.FR.001.460033.000.S.P.2021.000.21000 21
- URL:
- Publication:
-
Authors:
Sebastien Herbreteau, Charles Kervrann
-
Contact:
Charles Kervrann
7.1.6 SPITFIRE
-
Name:
SParse fIT for Fluorescence Image Restoration
-
Keywords:
Denoising, Fluorescence microscopy
-
Functional Description:
SPITFIR(e) (SParse fIT for Fluorescence Image Restoration) is a very flexible software designed to restore 2D-3D+Time fluorescent images and subtract undesirable out-of-focus background. We assume that the images are sparse and piece-wise smooth, and are corrupted by mixed Poisson-Gaussian noise. The principle resides in the minimization of a convex energy functional that includes a fidelity-to-data term and a Sparse-Hessian Variation regularization term. A fast primal-dual optimization algorithm allows to restore very large images in a few seconds. SPITFIR(e) is nearly parameter-free as the practitioner needs only to specify the amount of desired sparsity (weak, moderate, high). Experimental results in lattice light sheet, stimulated emission depletion, multifocus microscopy, spinning disk confocal, and wide-field microscopy demonstrate the generic ability of the SPITFIR(e) algorithm to efficiently reduce noise and blur, and to subtract undesirable fluorescent background, while avoiding the emergence of deconvolution artifacts.
Inter Deposit Digital Number: IDDN.FR.001.460029.000.S.P.2021.000.21000
- URL:
- Publication:
-
Contact:
Charles Kervrann
-
Participants:
Sylvain Prigent, Hoai Nam Nguyen, Ludovic Leconte, Cesar Augusto Valades Cruz, Jean Salamero, Charles Kervrann
-
Partner:
UMR 144 CNRS - Institut Curie
7.1.7 DenseMapping
-
Name:
Dense mapping of diffusion and drift of moving particules
-
Keywords:
Microscopy, Cellular imaging
-
Functional Description:
It is of primary interest for biologists to be able to visualize the dynamics of proteins within the cell. DenseMapping software allows to estimate the diffusion and drift parameters attached to moving biomolecules within cells from 2D/3D individual trajectories. In a first step, each particle track is labeled into three motion categories: confined motion (subdiffusion), Brownian motion (free diffusion), and directed motion (superdiffusion). The long trajectories are also segmented into sub-trajectories according to these three categories. In a second step, two 2D/3D maps (diffusion, drift) are computed from a local analysis of the trajectories. The local spatio-temporal kernel estimators correspond to weighted averages of the trajectory elements. The weights allow to select and aggregate the information in an optimal way.
Inter Deposit Digital Number: IDDN.FR.001.290028.000.S.P.2021.000.21000
- URL:
- Publications:
-
Contact:
Charles Kervrann
-
Participants:
Antoine Salomon, Vincent Briane, Cesar Augusto Valades Cruz, Charles Kervrann
-
Partner:
UMR 144 CNRS - Institut Curie
7.1.8 EM-Flow
-
Name:
Deep optical flow segmentation leveraging Expectation-Maximization
-
Keywords:
Image sequence, Optic-flow, Movement segmentation, Neural networks, Unsupervised learning, Deep learning
-
Functional Description:
: The software takes as input an optical flow field and provides as output an optical flow segmentation map. The inference is done in a single iteration. The method is fully unsupervised, and is able by design to segment multiple motions. It was experimentally validated on four well-known benchmarks, DAVIS2016, SegTrackV2, FBMS59, and MoCA, and performed very well, while being fast at test time. The software includes the trained weights of the network. It also provides the code for using the designed loss in any PyTorch project. The user has to specify the number of masks to segment.
- URL:
- Publication:
-
Contact:
Etienne Meunier
-
Participants:
Etienne Meunier, Patrick Bouthemy, Anais Badoual
7.1.9 BioImage-IT
-
Name:
BioImageIT : Open-source framework for integration of image data management with analysis
-
Keywords:
Image analysis, Fluorescence microscopy, Data management
-
Functional Description:
New image acquisition systems generate large numbers of images and large volume images. Such data sets are hard to store, to process and to analyze for one user in a workstation. Many solutions exist for data management (e.g. Omero), image analysis (e.g., Fiji, Icy, CellProfiler) and statistics (e.g., R). Each of them has its specificities and several bridges have been developed between pieces of software. Nevertheless, in many use-cases, the users need to perform analysis using tools that are available in different pieces of software and different languages. The design of a workflow that brings the data from one tool to another, is a tedious task that may require specific programming skills. Moreover, a dedicated script using a dedicated file system for processed data management is usually developed. To overcome these difficulties, we have developed BioImageIT, an integration platform to facilitate data management and analysis by integrating existing software. We created a desktop application for BioImageIT that integrates all the original BioImageIT functionalities in a unique application window. In this new graphical interface, scientists can manage their data, design analysis pipelines and run batch processing without coding. Furthermore, we integrated the napari viewer in BioImageIT to interact with multi-dimensional data.
- URL:
- Publication:
-
Contact:
Leo Maury
-
Participants:
Sylvain Prigent, Jean Salamero, Charles Kervrann, Leo Maury, Ludovic Leconte, Cesar Augusto Valades Cruz
-
Partner:
UMR 144 CNRS - Institut Curie
7.1.10 STracking
-
Name:
STracking : Open-source Python library for particle tracking and analysis
-
Keywords:
Motion analysis, Target tracking, Fluorescence microscopy, Cellular imaging, Image analysis, Object detection
-
Functional Description:
STracking is an open-source Python library, for combining algorithms into standardized particles tracking pipelines for biological microscopy images. STracking combines particle detection, tracking and analysis methods. A graphical interface is available using two napari plugins: napari-stracking and napari-tracks-reader. These napari plugins can be installed via the napari plugins menu. Unlike previous single particle tracking tools in Python ecosystem, STracking provides a very flexible solution for processing and visualizing the tracks taking advantage of Napari viewer for 3D+time representation. It greatly simplifies the inspection and optimization of single-particle tracking algorithms and thus allows the evaluation of new detection and tracker algorithms, which are constantly being developed.
- URL:
- Publication:
-
Contact:
Charles Kervrann
-
Participants:
Sylvain Prigent, Cesar Augusto Valades Cruz, Ludovic Leconte, Jean Salamero, Charles Kervrann
-
Partner:
UMR 144 CNRS - Institut Curie
7.1.11 Astrocytes Activation Simulator
-
Name:
Astrocytes Activation Simulator : Simulation of calcium events in microscopy images
-
Keywords:
Fluorescence microscopy, Simulator, Image sequence, Neuroimaging
-
Functional Description:
The ”Astrocytes Activation Simulator” software is an open-source plugin developed for Fiji and ImageJ image analysis platform. This simulator generates sequences of 2D/ 3D lattice light sheet microscopy images depicting astrocytic activity. Through the interface, the user specifies the image acquisition parameters and the level of activity of the astrocytes.
- URL:
- Publication:
-
Contact:
Anais Badoual
-
Participant:
Anais Badoual
-
Partners:
Université de Bordeaux, Okinawa Institute of Science and Technology Graduate University (OIST), Japan, Institut Interdisciplinaire de Neurosciences Bordeaux
7.2 New platforms
7.2.1 BioimageIT: Open-source framework for integration of image data-management with analysis
Participants: Sylvain Prigent, Cesar Augusto Valades-Cruz, Ludovic Leconte, Leo Maury, Jean Salamero, Charles Kervrann.
This year, we performed the deployment of BioImageIT (see Fig. 2 for illustration and Section 7.1.9) to the following France-BioImaging facilities: IMAG’IC (Institut Cochin, Paris Centre node), Imagerie-Gif (Île de France Sud node), BIC (Institut Interdisciplinaire des Neurosciences, Bordeaux node), MRI (Montpellier Ressources Imagerie, Montpellier node), Imachem (Collège de France, Paris Centre node), MicroPiCell, Mric and H2P2 (Bretagne-Loire node, Nantes and Rennes). BioImageIT has also been deployed to other facilites such as BIOSIT (University of Rennes 1) and I2B (Institut Biomédical de Bicêtre, Paris). Moreover, we also participated to the following events to promote BioImageIT in France: RTmfm network (online webinar), Hackathon Centuri (Marseille) and "Rencontre Nationale des Ingénieurs en Santé Numérique" (SED, Rennes).
URL:bioimageit.github.io and github.com/bioimageit
Publication: 21
Contact: Léo Maury
Partner: UMR 144 CNRS - Institut Curie
Funding: France-BioImaging, Investissement d'Avenir, ANR INBS-PIA1 2011 and “FBI Next Generation” (ANR program 2020-2024)
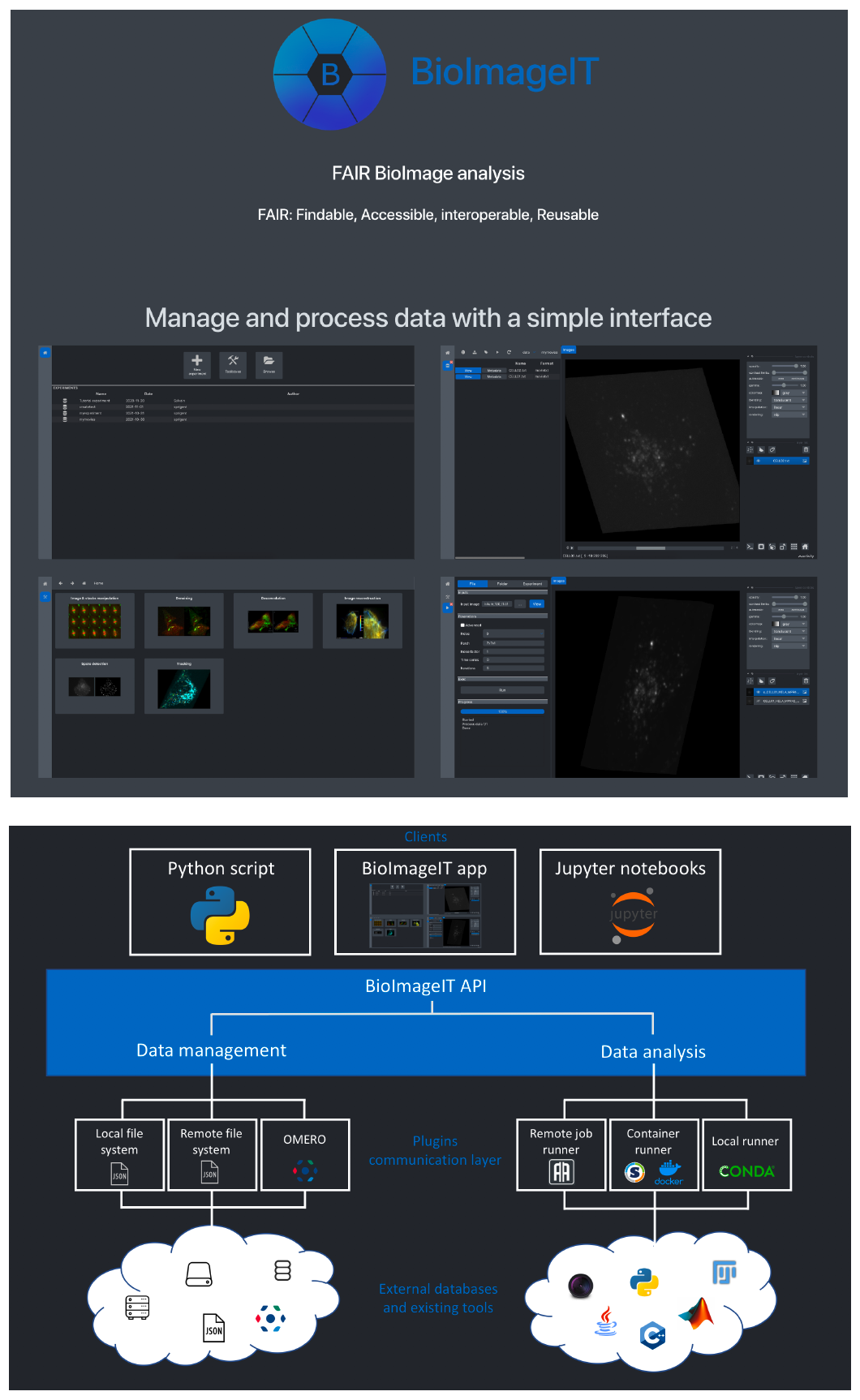
BioImageIT middleware and interface.
8 New results
8.1 Methods and algorithms for solving inverse problems in imaging and microscopy
The main stream regarding image restoration and segmentation methods advocates appropriate information processing and machine learning frameworks to make images intelligible. Over recent years, the Serpico team has promoted sparse and redundant representations, as well as deep-learning to solve the aforementioned problems in imaging and fluorescence microscopy, as well as in cryo-electron tomography. Several algorithms proposed by the team outperformed the state-of-the-art results and have resulted in practitioner-friendly software (see Section 7).
8.1.1 Sparse and redundant representations and deep-learning for image restoration
Convolutional neural networks for image restoration to reveal mitochondrial cristae organization in "intermediate quality" 2D+Time STED microscopy images
Participants: Salomé Papereux, Anaïs Badoual, Ludovic Leconte, Cesar Augusto Valades-Cruz, Jean Salamero.
In the internship of S. Papareux supervised by A. Badoual, we developed a CNN-based supervised method for mitochondria cristae image restoration. Biologists suppose that cristae are the contact points between mitochondria and the endosomal pathway. So far existing equipment does not allow the visualization of cristae and their dynamics with both high spatial and temporal resolution. The core idea of this study is to restore cristae in low resolution 2D STED images, allowing the simultaneous visualization of interactions over time through restorations. We implemented this workflow on the basis of the denoising CNN CARE 45 (Weigert et al., 2018), and assessed restoration quality of cristae. We developed a new robust loss SCoP, mixing the mean absolute error (MAE) and the structural similarity index (SSIM). In addition, we revisited the calculation of image quality assessment metrics with the "focus" improvement, which specifically addresses the issue of restoring cristae pixels, making it also suitable for various application pipelines. Finally, our method was combined with a sophisticated yet effective data training. Comparing the performance of our model with denoising and deconvolution methods showed that our approach performs well using real and synthetic datasets (see Fig. 3).
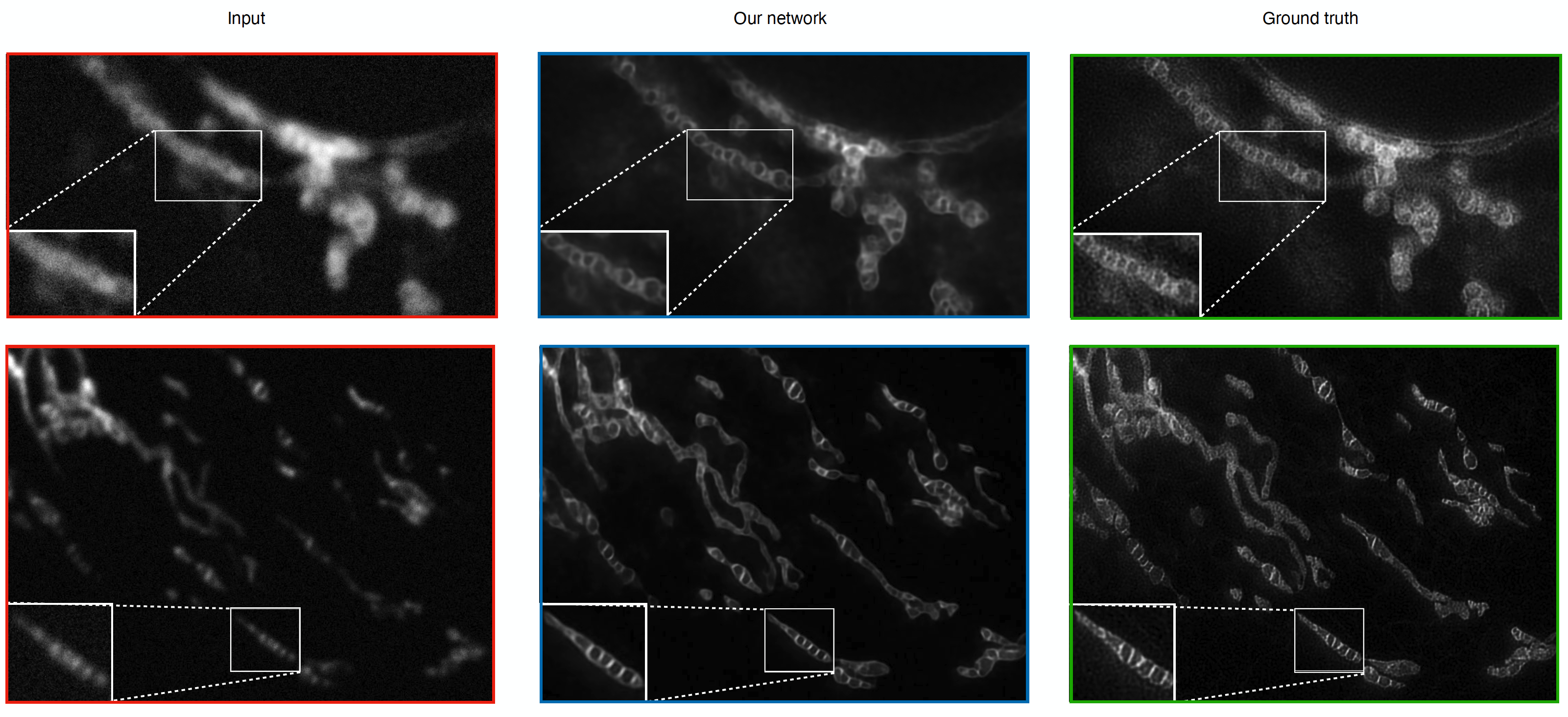
Restoration of mitochondrial cristae in STED images with our CNN-based supervised method. (Left red) Input data. (Middle blue) Results obtained with our method. (Right green) Ground truth data.
Sparse representations for image and video restoration in fluorescence microscopy
Participants: Sylvain Prigent, Ludovic Leconte, Cesar Augusto Valades-Cruz, Jean Salamero, Charles Kervrann.
In fluorescence microscopy, the image quality is limited by out-of-focus blur and high noise. In this area, we adapted a new convex regularization – Sparse Hessian Variation – to preserve image discontinuities and details, and we studied an appropriate fast primal-dual optimization algorithm. Moreover, we formally demonstrated that a quadratic fidelity term combined with any regularization term optimally restored images corrupted by mixed Poisson-Gaussian noise. The resulting deconvolution algorithm SPITFIR(e) 20 is very flexible, fast, easy to control (data-driven estimation of internal parameters by using the minimax principle), and is able to denoise and/or deconvolve multichannel 2D, 2D+time, 3D, and 3D+time fluorescence microscopy images acquired with spinning-disk confocal, wide-field, lattice light sheet, stimulated emission depletion, and multifocus (see Fig. 4) 20 microscopy. Moreover, we developed a SURE-guided (Stein's unbiased risk estimation) method and adapted SPITFIR(e) for super-resolution image reconstruction from confocal multi-array detector microscopes 19, 34.
Software: SPITFIRE (see Section 7.1.6)
Collaborator: B. Hajj (UMR 168 CNRS - Institut Curie)
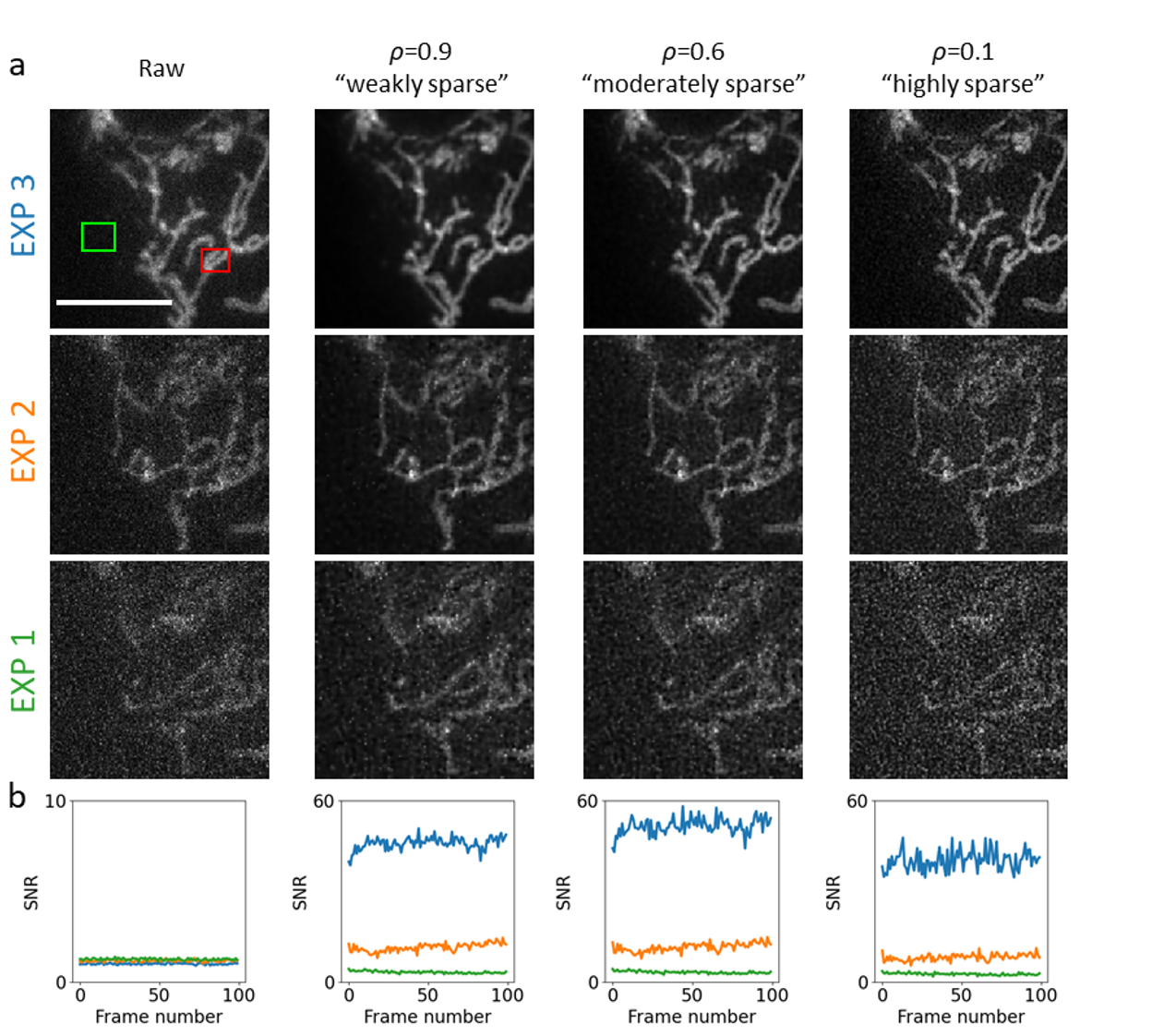
SPITFIR(e) image reconstruction applied to wide-field multifocus microscopy (MFM) with different amounts of sparsity, exposure and illumination values. Data are temporal series (100 time points) of 3D stacks composed of nine planes each depicting mitochondria in U2OS cells transfected with TOM20 (translocase of outer mitochondrial membrane) fused to GFP (GFP-TOM20). Exposure time: 50 ms. The sample is imaged with three different doses of illumination light. MFM allows simultaneous acquisition of a 3D stack of nine images equally spaced with (dz = 330 nm) focal 2D images (pixel size = 120 nm). (a) The image grid displays the deconvolution results on the 6th plane for successive increasing laser power values (EXP 1, 2 and 3). The 3D stacks have been deconvolved with 3D Gaussian PSF model (σxy=1.5\sigma _{xy} = 1.5 pixels and σz=1.5 \sigma _z = 1.5 pixels) and with three different levels of sparsity ("weak", "moderate", and "high"). (b) The plots are SNR values calculated for the 6th plane at each time-point as follows: SNR = Power(signal) / Power(background) = |Ω(xS)|-1∑x∈Ω(xS)I(x)2/|Ω(xB)|-1∑x∈Ω(xB)I(x)2|\Omega (x_S)|^{-1} \sum _{x \in \Omega (x_S)} I(x)^2 / |\Omega _(x_B)|^{-1} \sum _{x \in \Omega (x_B)} I(x)^2 , where Ω(xS)\Omega (x_S) (with size |Ω(xS)||\Omega (x_S)|) and Ω(xB)\Omega (x_B) (with size |Ω(xB)||\Omega (x_B)|) are two ROIs centered at pixels xSx_S (red ROI) and xBx_B (green ROI), respectively. Scale bar: 10 μ\mu m.
Redundant representations for unsupervised image denoising
Participants: Sébastien Herbreteau, Charles Kervrann.
Inspired from recent results in machine learning, we re-visited unsupervised patch-based methods for image restoration. First, we proposed a unified parametric view of non-local two-step denoisers, for which BM3D 40 (Dabov et al., 2007) is a major representative, where quadratic risk minimization is adopted for unsupervised optimization. Leveraging Stein’s unbiased risk estimate (SURE) for the first step and the "internal adaptation", a concept borrowed from deep-learning theory, for the second one, our approach enables to reconcile several algorithms, originally established in different modeling and estimation frameworks (see Fig. 5). Within this paradigm, we derive NL-Ridge 26 that processes all groups of similar patches via plain linear combinations (Fig. 5). In the second step, our closed-form aggregation weights are computed through multivariate ridge regressions. Experiments on artificially noisy images demonstrate that NL-Ridge may outperform well established state-of-the-art unsupervised denoisers such as BM3D 40 (Dabov et al., 2007) and NL-Bayes 38 (Buades et al., 2013), as well as recent unsupervised deep-learning methods, while being conceptually simpler.
Second, in order to further improve the denoising performance, we extended the underlying unified parametric mathematical formulation. This approach involves estimating a very large amount of parameters in an unsupervised manner. Focusing on the parameterized form of NL-Ridge, we proposed a novel scheme to progressively estimate the parameters that best minimize the risk. Our experimental results show that the so-called LIChI algorithm 33 preserves much better structural patterns and textures and generates much less visual artifacts, in particular around the edges, than the single-iterated denoisers, such as BM3D and NL-Ridge. The proposed algorithm compares favorably with WNNM 41 (Weighted Nuclear Norm Minimization, (Gu et al., 2014)), the best unsupervised denoiser, both in terms of quantitative measurement and visual perception quality even on real-world noisy images, while being faster at execution.
Software: NL-ridge (Python), LIChI (Python)
Collaborator: R. Fraisse (AIRBUS Defense and Space SAS)
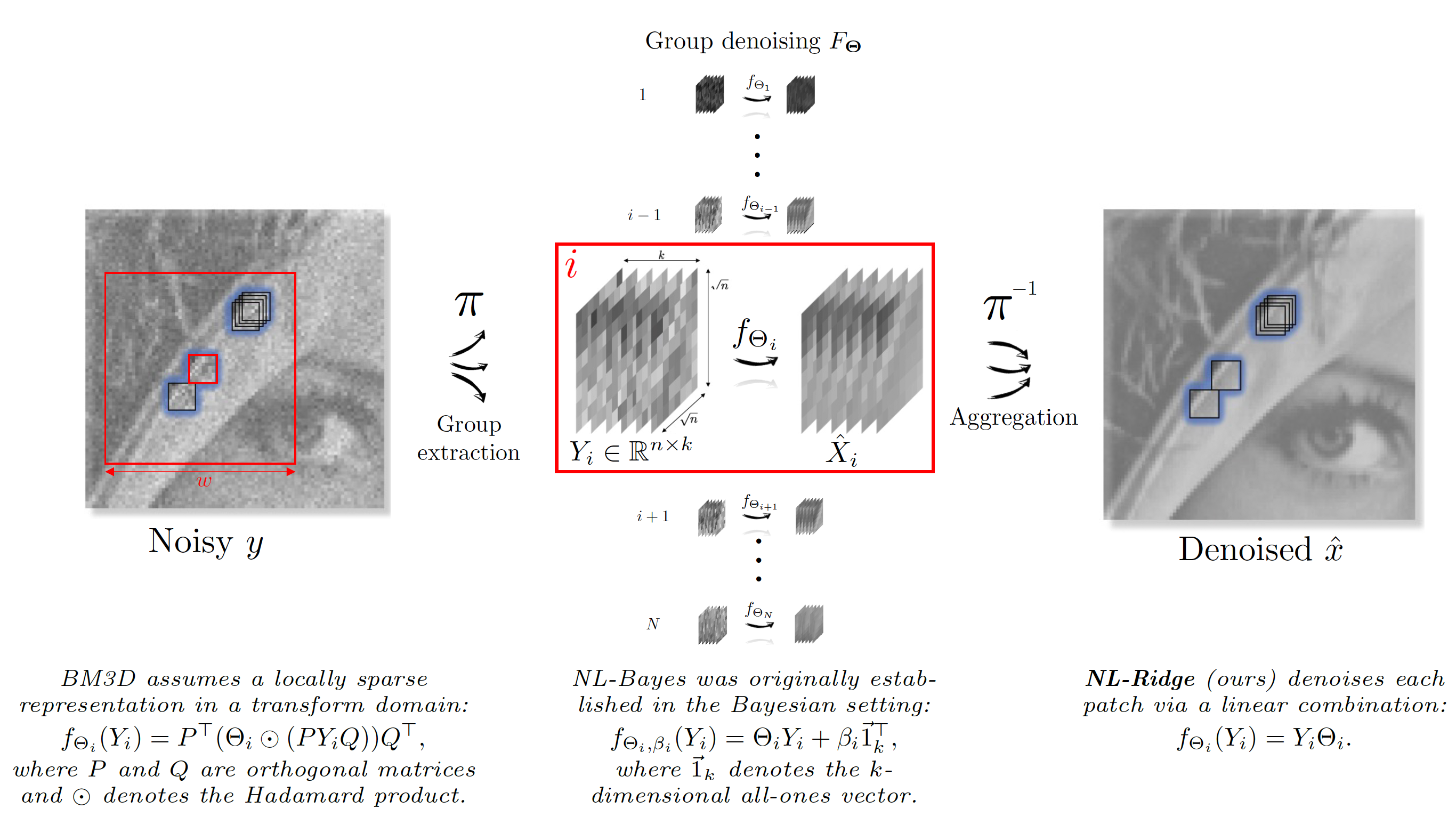
Illustration of the parametric view of non-local denoisers. Examples of parameterized functions fΘif_{\Theta _i}, unequivocally identifying the denoiser, are given whose parameters Θi\Theta _i are eventually selected for each group of patches by "internal adaptation".
8.1.2 Supervised deep-learning for detection, segmentation, and classification in microscopy
Detection and segmentation of chromosomes in 2D microscopy images with deep-learning
Participants: Antonin Deschemps, Emmanuel Moebel, Quentin Tallon, Charles Kervrann.
In the PhD theses of A. Deschemps and Q. Tallon and PostDoc of E. Moebel, we have investigated supervised deep-learning methods to localize chromosomal aberrations in metaphases observed in 2D light (GIEMSA) and fluorescence in situ hybridization (FISH) microscopy images for biological dosimetry, where the proportion of unhealthy chromosomes is directly linked to the dose of ionizing radiation received by the patient. The classification of chromosomes into several categories (monocentric vs dicentric chromosome, fragment of chromosome, translocations, etc.) is challenging because of variable acquisition techniques and unbalanced classes. One serious difficulty comes from the lack of annotation of the data, the rarity of the target class, and the small size of the data set, which limits the performance of supervised methods.
To overcome these difficulties, we developed several strategies that consist in predicting maps of chromosome presence in metaphase images (light GIEMSA microscopy) on one hand, and on the other hand, to combine color attention with several existing architectures (Faster-RCNN, Mask-RCNN, StarDist, etc.) for classification, bounding box regression and instance segmentation of translocations in FISH images (see Fig. 6).
In contrast to the aforementioned methods that jointly perform localization and classification in a single step, we found that dissociating these two tasks significantly improves the detection performance. Thus, we have set up analogical pipelines for both StarDist and Faster-RCNN -type object detection consisting of separate state-of-the-art methods for each task. As a StarDist analogy, Cellpose 44 is used for chromosome localization and ResNet is used for translocation classification. Here, Faster-RCNN is reused without class distinction for localization, and ResNet is used for classification.
Furthermore, as frequently investigated in approaches based on deep-learning, we have explored the effects of filterings and pre-processings applied to our images. Indeed, two problems have been identified related to the homogeneity of our datasets: i) different microscopy slabs lead to different textures and distributions of fluorescence; ii) the quality of chromosome proximity and size can vary from one image to the next. Considering the latter problem, we have manually filtered suspicious proximities and sizes given the segmentation maps produced by the Cellpose algorithm 44. The current goal is to make this step automatic by exploiting shape statistics (centroid coordinates, ellipse and convex hull parameters, etc.). For texture and fluorescence distribution, we used histogram matching techniques based on channel-wise quantile regressions (see Fig. 7). Both solutions, either combined or not, lead to significantly increased performance scores for all classification pipelines. In future work, we envision to improve transferability of a trained model from one dataset to another one, by continuing to investigate domain adaptation techniques.
In the case of light GIEMSA microscopy images, we used deep convolutional neural networks for aberration counting. We showed that standard keypoint regression techniques can be used to build very simple detection models, based on U-Net, using a large, annotated database provided by IRSN. This model reaches significantly higher performance than the competing Metafer system, a software provided by MetaSystem. The false discovery rate of Metafer is known to be high and unpredictable (depending on minute variations in data quality). In our approach, the number of false positives can be decreased by penalizing small irregularities in the prediction with a sparse variation regularization as described in 20. Furthermore, we demonstrated that a simple model aggregation setup may improve performance and offers opportunities for uncertainty modeling. This ensemble can be built using checkpoints of a single training run and provides a simple answer to numerous ambiguous detection cases. The performance of our object detection algorithm with respect to ensemble size and the diversity of its prediction is examined in detail (see Fig. 8). We showed that a careful selection of checkpoints was not needed; the performance spread of the ensemble is quantified with random sampling and is much lower than the spread observed during training.
Collaborator: Mohamed Amine Benadjaoud (IRSN/SERAMED, Fontenay-aux-Roses)
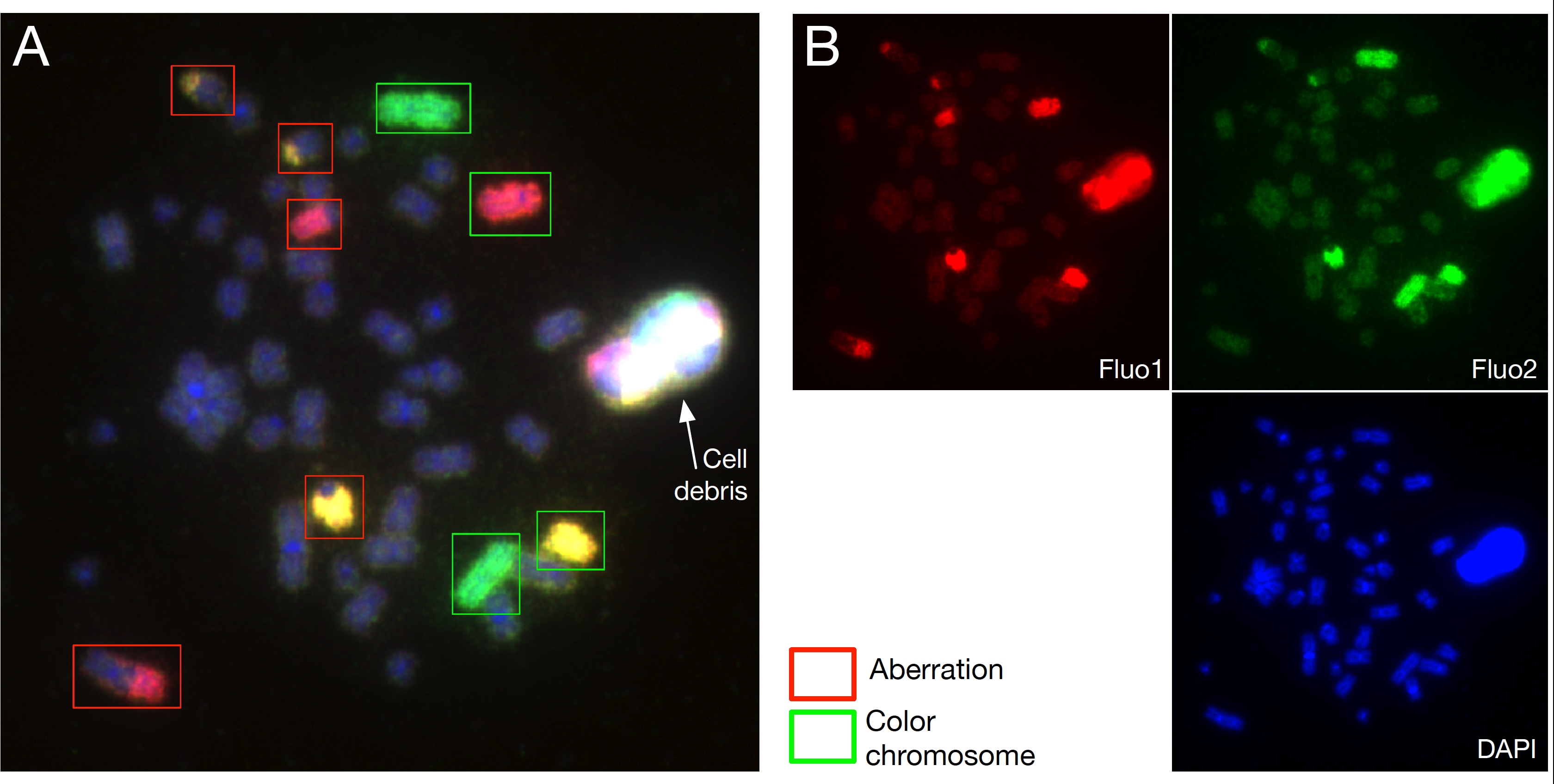
Translocation detection in three-channel FISH images. (A) The green rectangles indicate the location of "normal" chromosomes. The red rectangles correspond to the location of translocated chromosomes. (B) The three separated channels used to localize chromosomes and detect translocations.
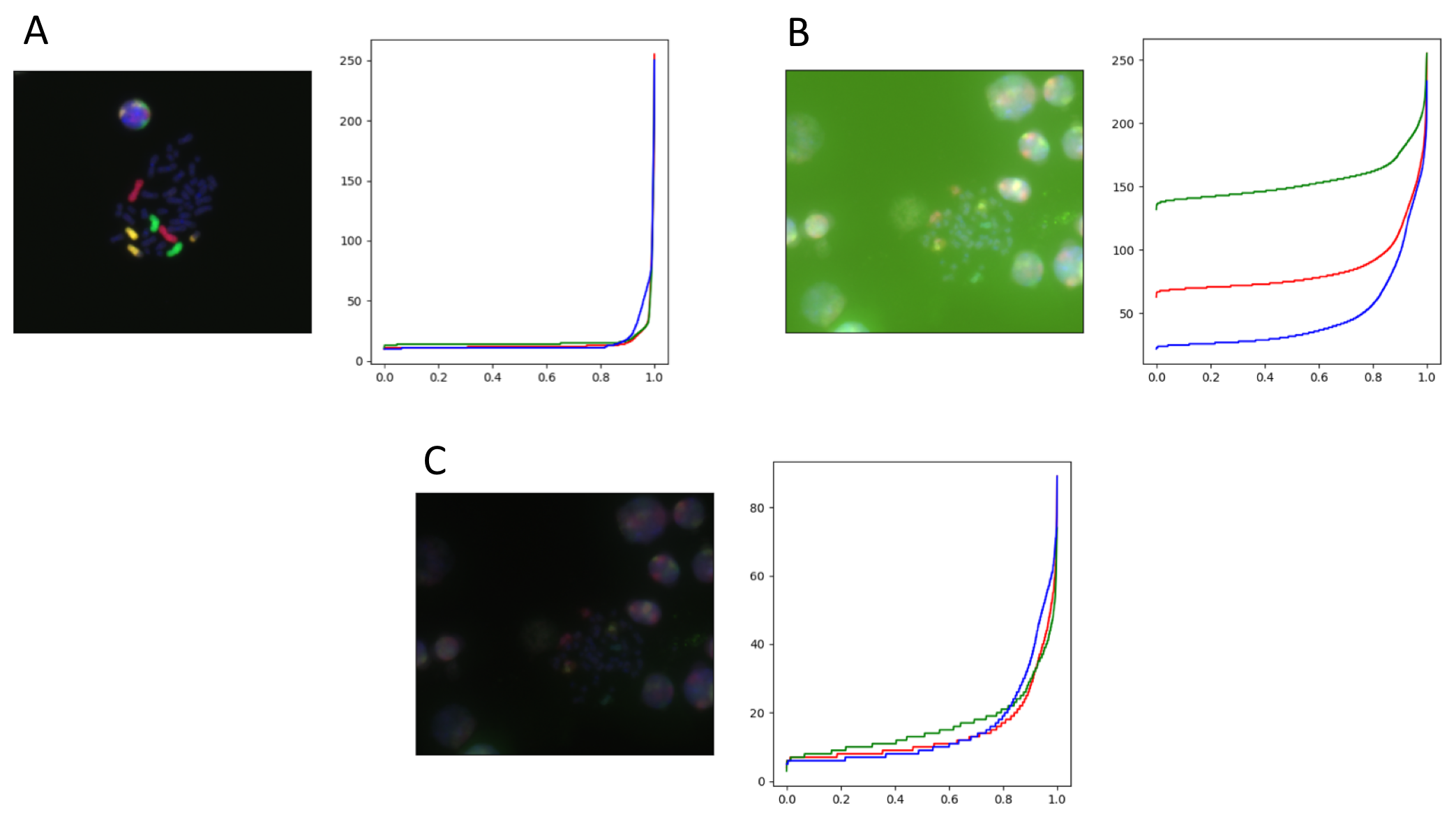
Multi-channel iFISH mages and corresponding 1000-quantiles for each channel. The first image (A) is the template used for quantile regression, and the last two images are an example of texture correction, (B) before and (C) after.
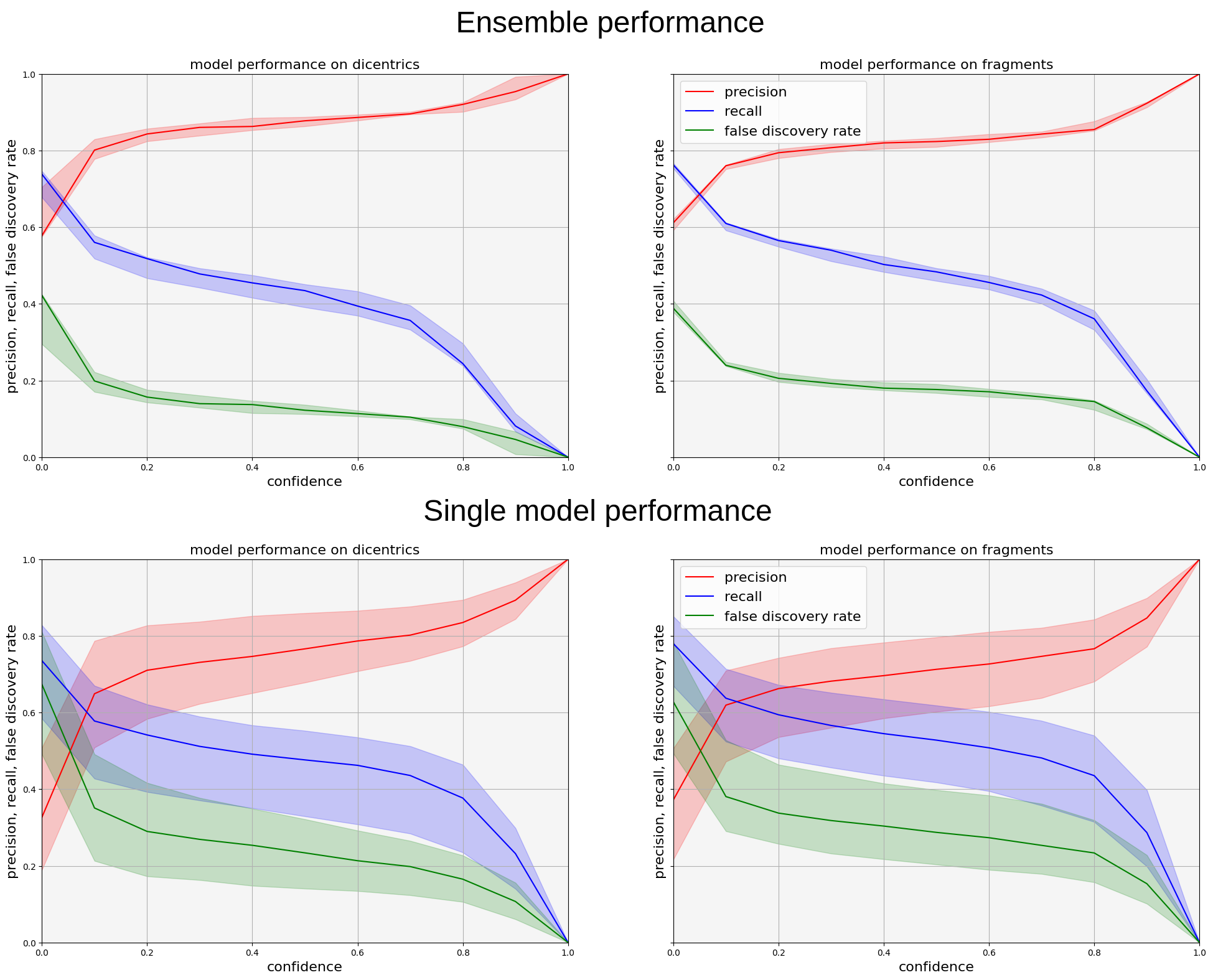
Comparison of model performance in light GIEMSA microscopy. Precision, Recall, and False Discovery Rate curves obtained with model ensemble performance on top and single model performance on the bottom. Shaded are indicates 5% and 95% quantiles of performance, central line is median of performance (computed over 10 repetitions of the ensemble). Model ensemble drastically improves performance and reduces uncertainty.
Detection of macromolecules in 3D cellular cryo-electron tomograms with deep-learning methods
Participants: Emmanuel Moebel, Frédéric Lavancier, Charles Kervrann.
Cryo-ET allows to image cells in situ, with enough resolution to locate macromolecules without sample-altering bio-markers. Developing classification and detection methods is a way of obtaining an efficient counting technique, while being a powerful tool for geo-localization and macromolecule reconstruction with sub-tomogram averaging. In past years, we investigated deep-learning and 3D CNN to accurately and jointly localize multiple macromolecules (e.g., ribosomes, proteasomes, ...) in 3D cell cryo-electron tomography images. Our results demonstrated that the designed 3D CNN Unet-based architecture (DeepFinder) 9 (embedded recently into the Scipion platform 14) outperforms the usual template matching algorithms and most of competitive recent deep-learning methods (as demonstrated in SHREC 20219, SHREC 2020, and SHREC 2021 challenges), and allows to imitate the performance of experts.
This year, we focused on the detection of very small macromolecules in real cellular cryo-electron tomograms, in collaboration with IGBMC Strasbourg. The aim was to apply DeepFinder to detect nucleosomes (200 kDa) in images of neurons in Drosophila embryos (see Fig. 9). Detection performance is now satisfactory, and the current challenge is to study the spatial arrangement of nucleosomes in the nucleus. Moreover, in the internship of C. Aronssohn supervised by E. Moebel, we improved the DeepFinder graphical unit interface via the Napari python package and explored different strategies to segment filaments in 3D images with DeepFinder, in collaboration with University of Oviedo. Meanwhile, we investigated unsupervised deep-learning strategies for macromolecule classification in cryo-electron tomography. Our proof-of-concept strategy 18 consists in learning a clustering-friendly representation, using a simulated dataset (see Fig. 10). Once trained, it can be applied to experimental data for classifying macromolecules according to structural similarity. Finally, we have slighlty modified DeepFinder for analyzing 2D+time fluorescence videos and detecting rare exocytosis events in TIRFM images, in collaboration with Institut Gustave-Roussy and Institute Curie (UMR 144 CNRS).
Software: Stand-alone DeepFinder and Plugin Scipion DeepFinder
Collaborators: B. Engel (Max-Planck Institute of Biochemistry, Martinsried, Germany; Helmholtz Pioneer Campus, Neuherberg, Germany); E. Fourmentin and D. Larivière (Fourmentin-Guilbert Scientific Foundation, Noisy-Le-Grand); C.O Sorzano (Biocomputing Unit, CSIC, Madrid, Spain); A. Martínez-Sánchez (Department of Computer Sciences, University of Oviedo, Spain); M. Eltsov and F. Fatmaoui (IGBMC Strasbourg); D. Levy and A. Bertin (UMR168 CNRS - Institut Curie, Paris); H. Lachuer and A.-S. Macé (UMR 144 CNRS - Institut Curie, Paris) and K. Schauer (Institut Gustave Roussy, Inserm U1279)
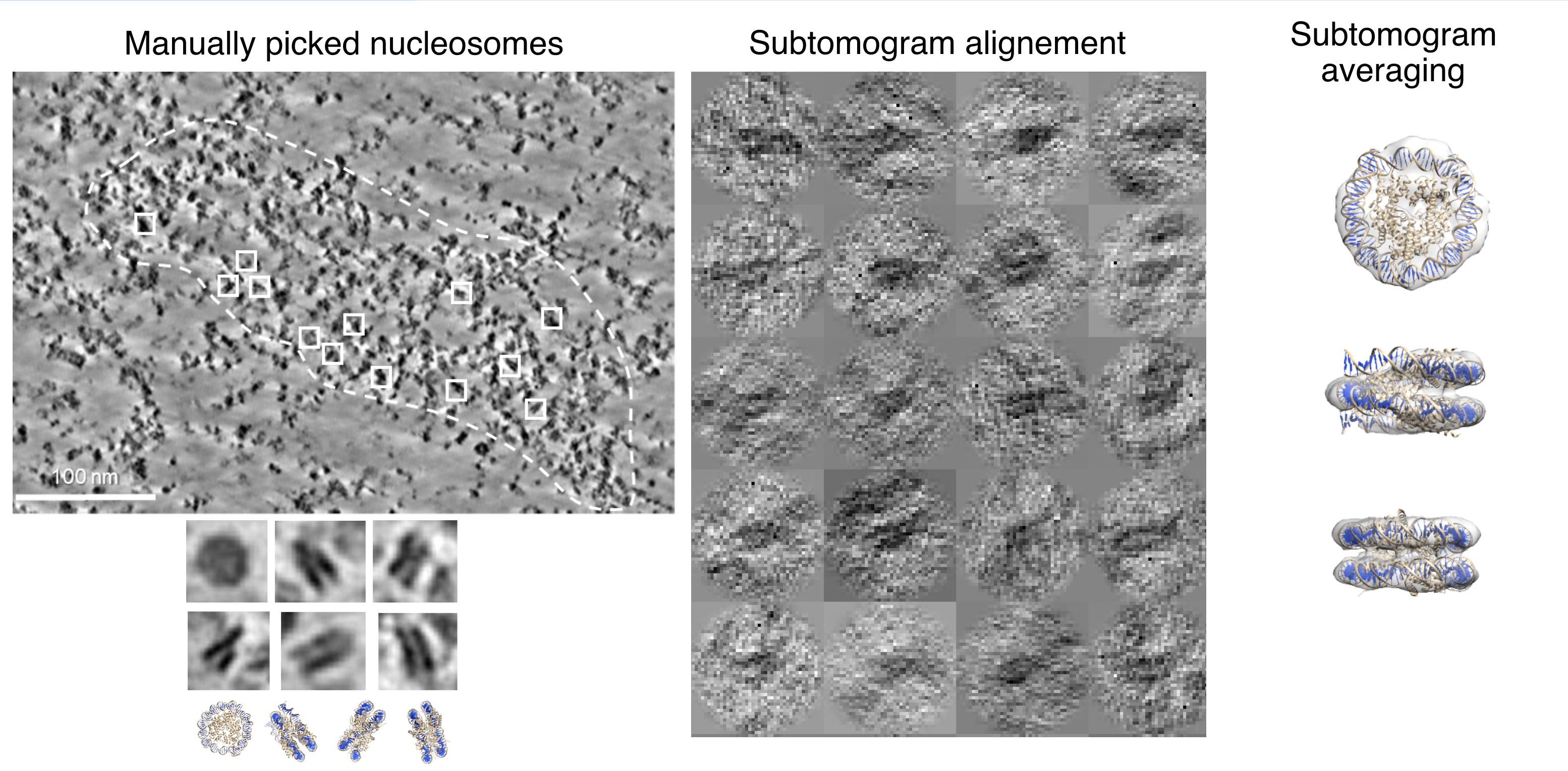
Nucleosome detection in cryo-electron tomography. (Left) localization of nucleosomes in 3D images with DeepFinder. (Right) subtomogram alignment and averaging from the set of detected nucleosomes.
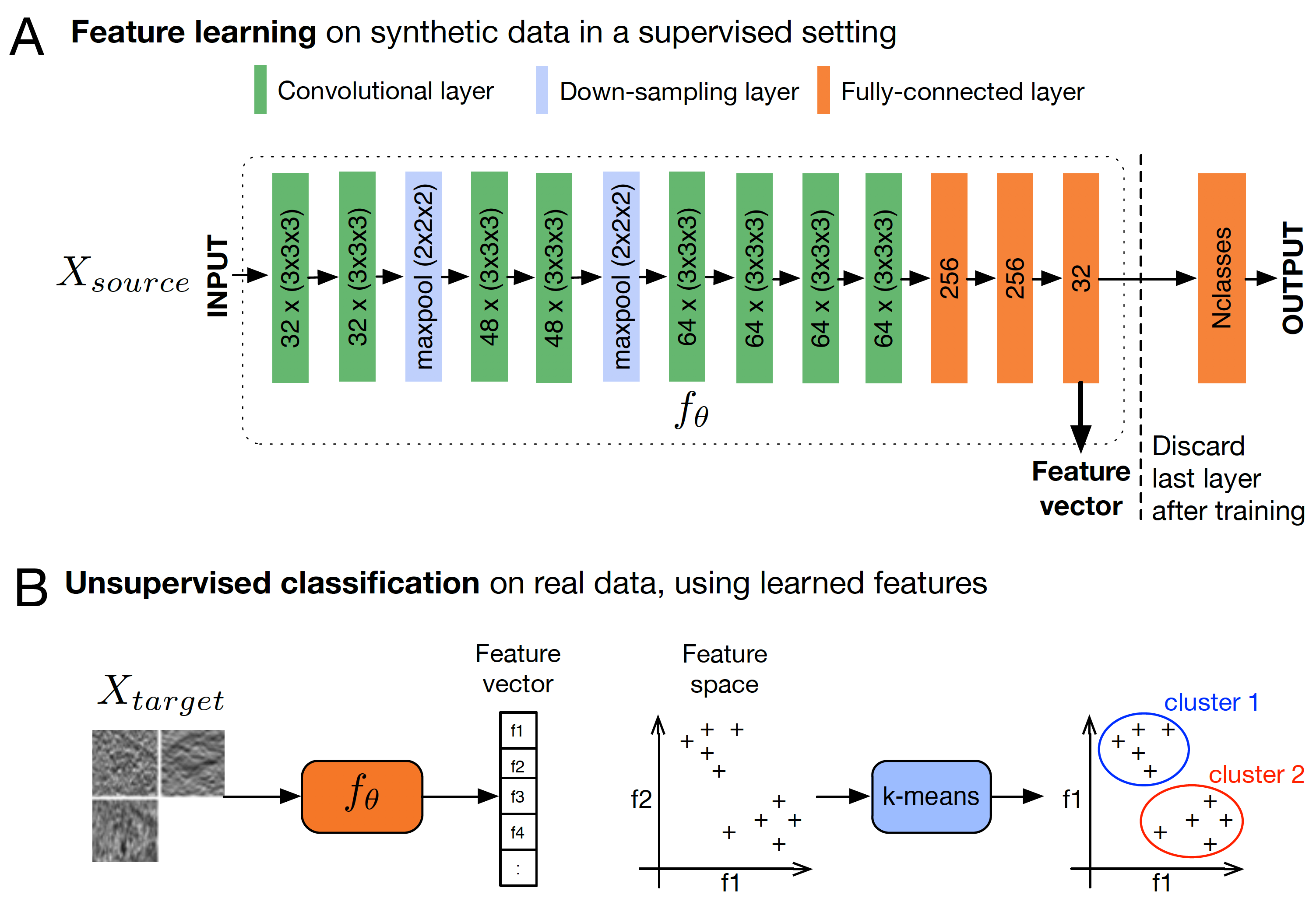
Weakly supervised subtomogram classification. (A) Architecture of the CNN used to learn a feature space that characterizes 3D shapes. The network is trained on a synthetic data-set composed of 13 different macromolecule classes, obtained from the Protein Data Bank (PDB). Once trained, the last layer is discarded and the resulting network that takes as an input volumes, outputs feature vectors of size 32. (B) The previous network is used to compute the feature vectors of experimental subtomograms. Classification is performed by applying k-means clustering given feature vectors. The obtained clusters group the subtomograms by structural similarity.
8.2 Motion computation, dynamics analysis, and visualization in imaging and microscopy
Several particle tracking methods for intracellular analysis have been tailored to cope with different types of cellular and sub-cellular motion down to Brownian single molecule behavior. One remaining key open issues is the tracking of intracellular components with heterogeneous movements in crowded configurations. Meanwhile, particle tracking methods are not always adapted for motion analysis, especially when the density of moving features hampers the individual extraction of objects of interest undergoing complex motion. Estimating flow fields and dense maps can be then more appropriate to capture the complex dynamics observed in biological sequences. There is actually no definitive solution to both particle tracking and motion estimation problems as they remain application-dependent in most cases.
In recent years, we have focused on unsupervised deep-learning-based image motion segmentation and motion saliency, while developing original concepts for 3D optical flow estimation and investigating 3D+time data visualization techniques in fluorescence microscopy. Our new paradigms offer promising ways for quantification and interpretation in cell imaging.
8.2.1 Particle tracking and visualization in 3D+time microscopy
Particle tracking in fluorescence microscopy
Participants: Sylvain Prigent, Ludovic Leconte, Cesar Augusto Valades-Cruz, Jean Salamero, Charles Kervrann.
Analysis of intracellular and extracellular dynamic like vesicles transport involves particle tracking algorithms 11. The design of a particle tracking pipeline is a routine but tedious task. Therefore, particle dynamics analysis is often performed by combining several pieces of software (filtering, detection, tracking, etc.) requiring many manual operations, and thus leading to poorly reproducible results. Given the new segmentation tools based on deep-learning, modularity and interoperability between softwares have become essential in particle tracking algorithms. A good synergy between a particle detector and a tracker is of paramount importance. In addition, a user-friendly interface to control the quality of estimated trajectories is necessary. To address these issues, we developed STracking, a Python library that allows combining algorithms into standardized particle tracking pipelines. STracking combines particle detection, tracking and analysis methods and can be used via a napari plugin. STracking was combined to 2D-TIRFM 35 to characterize the biophysical properties of the Tau protein that regulates microtubule dynamics in neuronal cells, as well as to Lattice Light-Sheet Structured Illumination Microscopy 39 to characterize endosomal pathways in RPE1 cells (see Fig. 11).
Reference: 22
Software: see Section 7.1.10
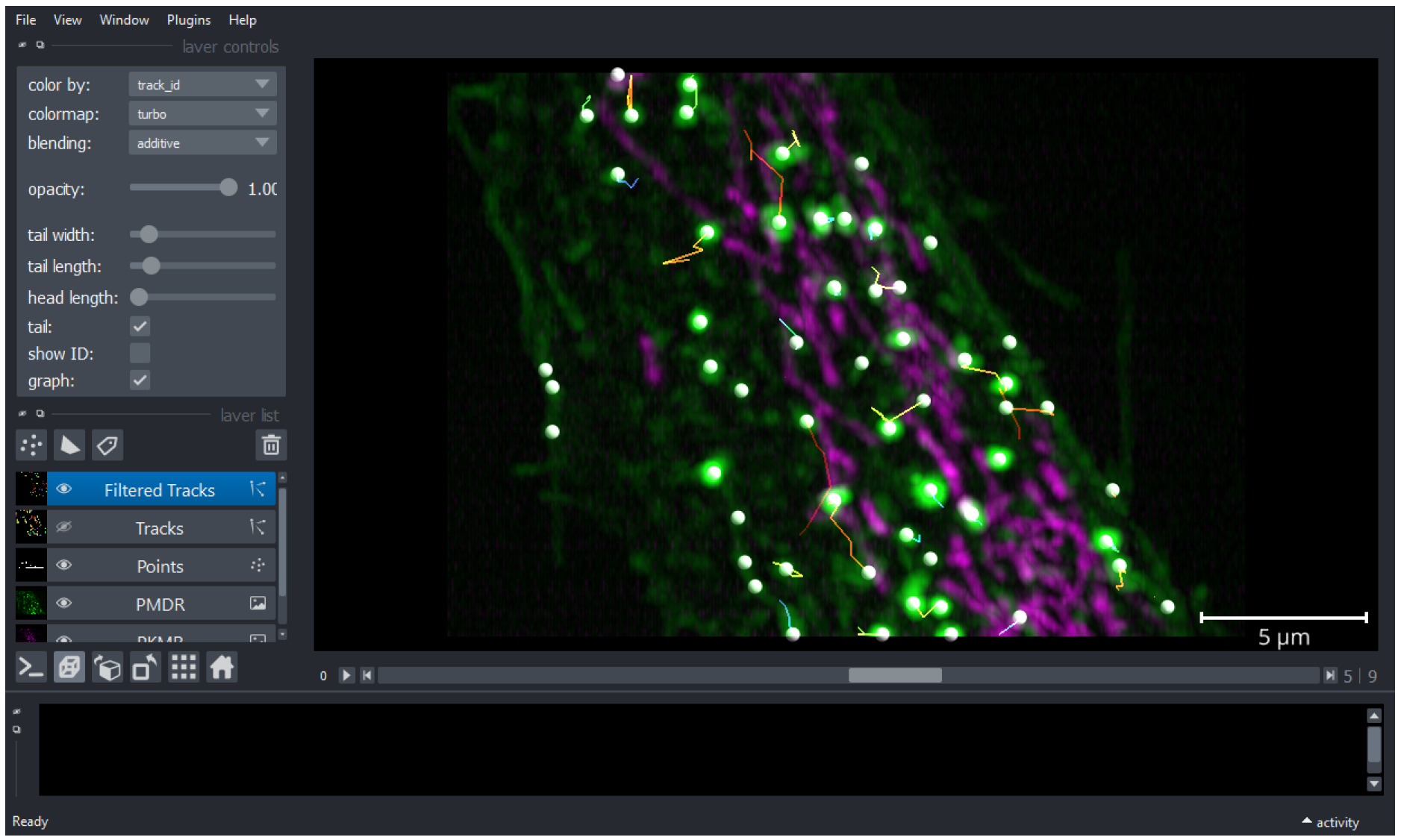
STracking library implemented in its napari plugin. Fifty-five planes 3D volumes of live RPE1 cells double stained with PKMR for Mitochondria (magenta) and with plasma membrane deep red (PMDR) for endosomal pathway (green) were acquired within 4.3 s per stack using Lattice light-sheet Structure Illumination Microscopy (LLS-SIM). STracking is illustrated here with single particle tracking of endosomal pathway (PMDR). 3D data and tracks are rendered using napari viewer.
Spatiotemporal data visualization and immersive interaction
Participants: Gwendal Fouché, Kevin Fournier, Ludovic Leconte, Cesar Augusto Valades-Cruz, Jean Salamero, Charles Kervrann.
The exploration of the 3D+time data is known to be challenging, and the usual methods, such as temporal sliders, handling few time points, can come limited when analyzing dynamics features. As visualization of 3D+time biological processes has proven to work poorly on 2D monitor screens so far, we have investigated Virtual Reality (VR) and methods from the field of "immersive analytics" to better explore multidimensional and multi-valued images, especially in cell and tissue imaging 24.
This year, we have explored the potential of timelines to display temporal series of cellular events, linearly along curved or helicoidal temporal axes 25. This representation is very flexible, producing expressive visualizations that can be adapted to support a narrative or highlight specific temporal features. Timelines are usually represented on 2D displays, and are not suitable for the visualization of 3D temporal data. As such, we proposed a 3D timeline design space for 3D temporal data. We explored different dimensions in the design, in order to produce different visualizations adapted for the analysis of microscopy imaging datasets, as shown in Fig. 12. We experimented 3D timelines for the exploration of CD63 endosomes vs mitochondria dataset, as well as a Phallusia Mammillata embryo development dataset, and gathered the feedback of 20 biology experts regarding the benefits of such visualizations. Finally, we implemented this work on timeline visualizations in the already distributed MorphoNet software 42. This implementation required modifications and enhancements to several parts of MorphoNet, including computing performance. VR interfaces were designed so that users could easily switch between desktop and Virtual Reality environments. Most of the dataset transformation and manipulation from MorphoNet were replicated in VR (see Fig. 13).
Moreover, we addressed the challenge of selecting 3D objects or events appearing in 3D temporal datasets. We addressed in particular the selection of 3D objects that appear only shortly in time. For example, calcium signals in astrocytes (see Fig. 14 (A)) appear over only a few time steps in the hundred-time-steps-long dataset. Traditional methods to select such objects most often consist in executing, at each time step, an exploration of the 3D space, i.e., a 3Dx1D exploration of the dataset. This year, we proposed a method based on a Space-Then-Time interaction process in Virtual Reality, based on three main development axes. First, the user explores a view of the information, condensed into a single 3D view, such as a density map (see Fig. 14(B)). Second, the user selects adequate 3D information in this condensed view related to their required object, for example through a brushing interaction. Third, the user performs a disambiguation in time, choosing between the potentially multiple objects that match the selection. A prototype of this method was partially implemented and is yet to be tested.
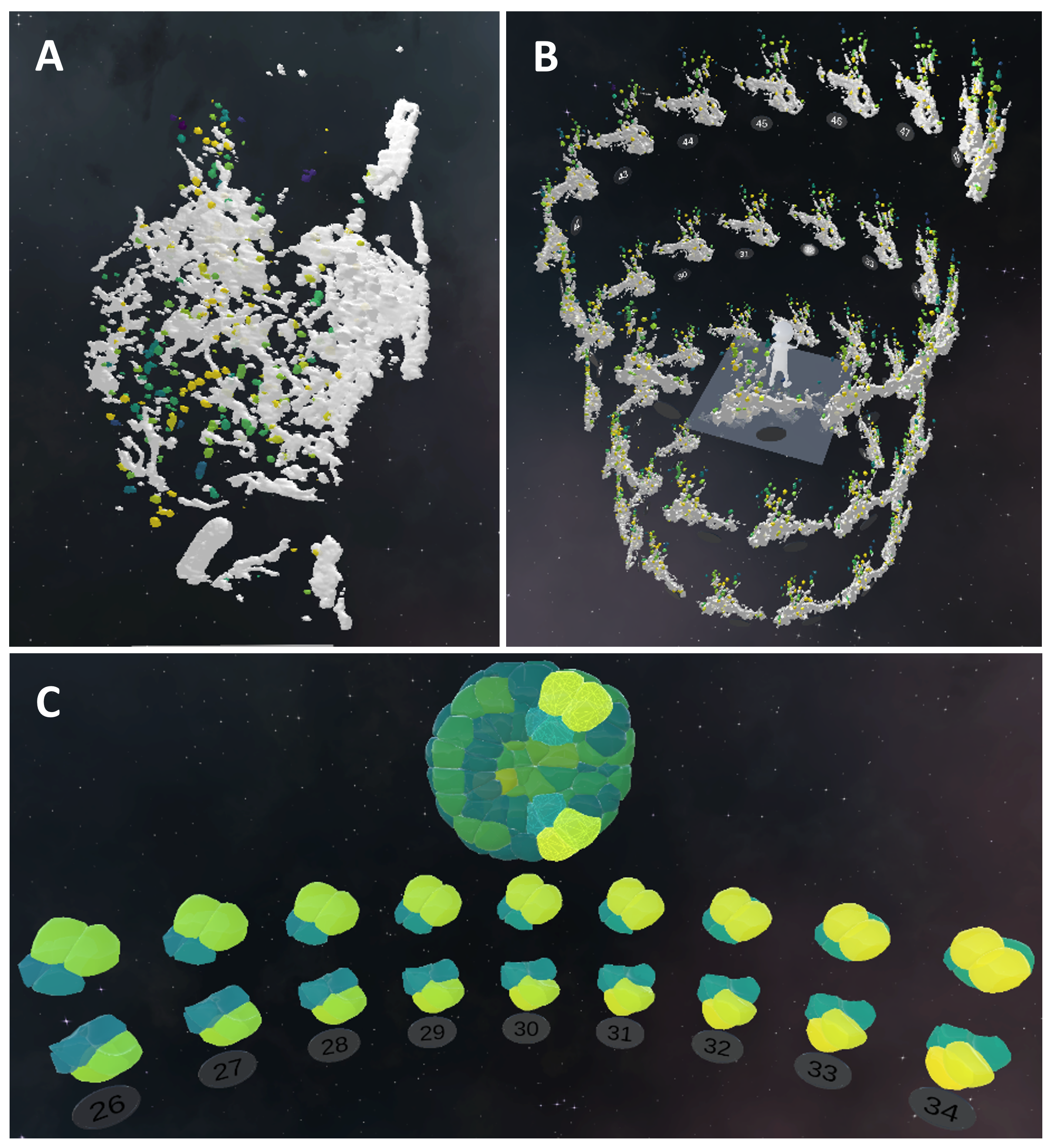
3D Timeline visualization in VR for 3D temporal microscopy images. (A) 3D view of CD63 endosomes (green-yellow) versus mitochondria (grey) in RPE1 cells. (B) Helicoid 3D timeline visualization of the temporal evolution of a selected region of interest in the cell. (C) 3D view of Phallusia Mammillata embryo recording, with a curved 3D timelime visualization of the temporal evolution of the highlighted cells.

Desktop and VR view in MorphoNet. (A) 3D view of Phallusia Mammillata embryo in the desktop environment. (B) 3D view of Phallusia Mammillata in the VR environment. (C) 3D view of Phallusia Mammillata with a helicolidal timeline visualization of the evolution of the embryo.
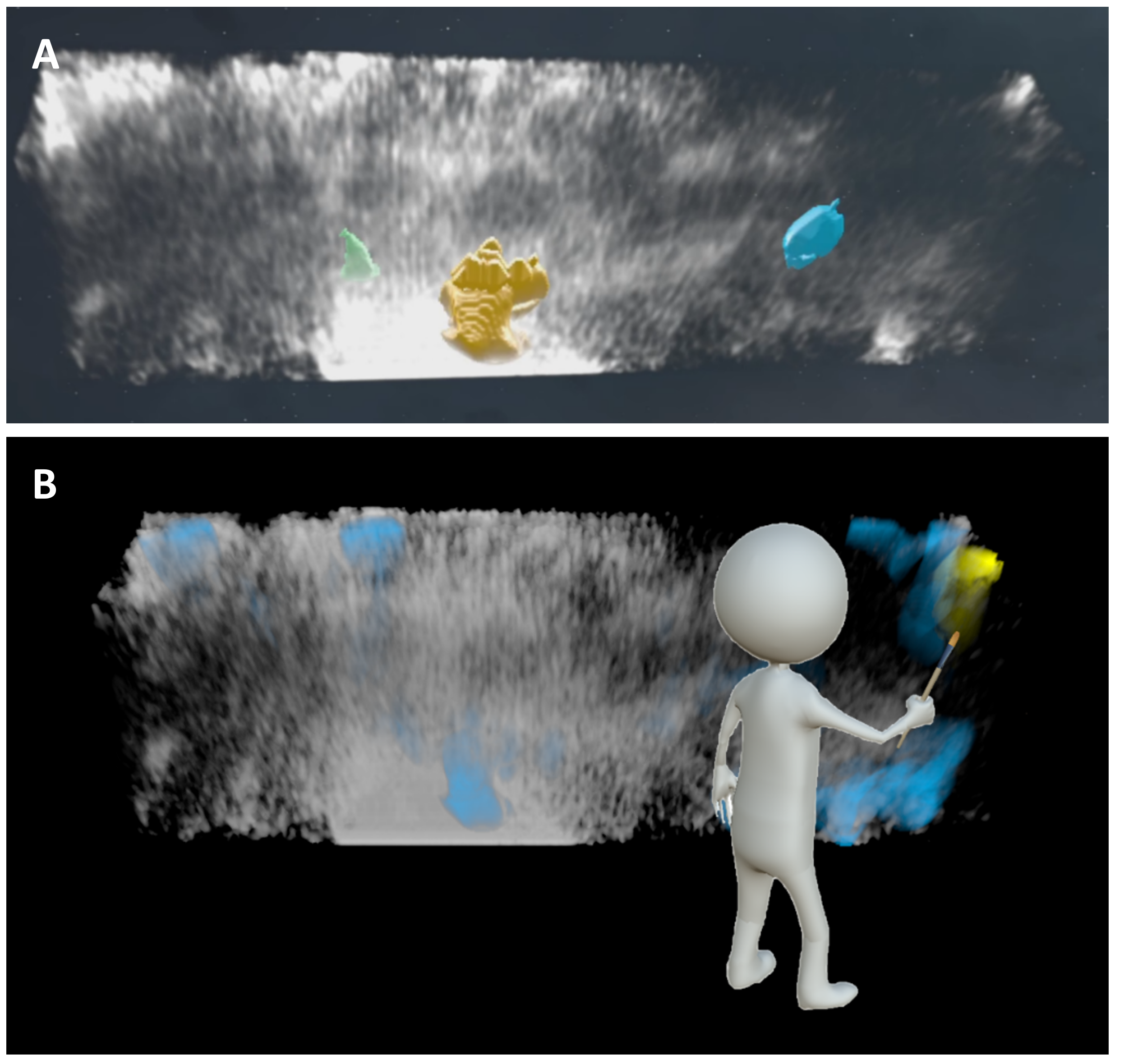
Selection in VR of temporally short elements in 3D temporal microscopy data. (A) Visualization of simulated calcium signals (in color) in a LLSM recording of an astrocyte (grey). (B) The user selects a 3D zone through a brushing interaction in the calcium signal density map (blue) to designate a specific object.
8.2.2 Motion segmentation and saliency detection in videos
We have developed deep-learning-based methodologies for motion analysis in 2D image sequences that will contribute to develop appropriate solutions to problems in 2D/3D cell imaging.
EM-driven unsupervised learning for efficient motion segmentation
Participants: Etienne Meunier, Anaïs Badoual, Patrick Bouthemy.
We have defined a CNN-based fully unsupervised method for motion segmentation from optical flow 17. We assume that the input optical flow can be represented as a piecewise set of parametric motion models, typically, affine or quadratic motion models. The core idea of our work is to leverage the Expectation-Maximization (EM) framework in order to design in a well-founded manner a loss function and a training procedure of our motion segmentation neural network that does not require either ground-truth or manual annotation. However, in contrast to the classical iterative EM, once the network is trained, we can provide a segmentation for any unseen optical flow field in a single inference step and without estimating any motion models. We have investigated different loss functions including robust ones, and proposed a novel efficient data augmentation technique on the optical flow field, applicable to any network taking optical flow as input. In addition, our method is able by design to segment multiple motions. Our motion segmentation network was tested on four benchmarks, DAVIS2016, SegTrackV2, FBMS59, and MoCA, and performed very well, while being very fast at test time (see Fig. 15).
Software: The software EM-Flow, implementing the method, is publicly available (see Section 7.1.8).
Collaborator: R. Fraisse (AIRBUS Defense and Space SAS)
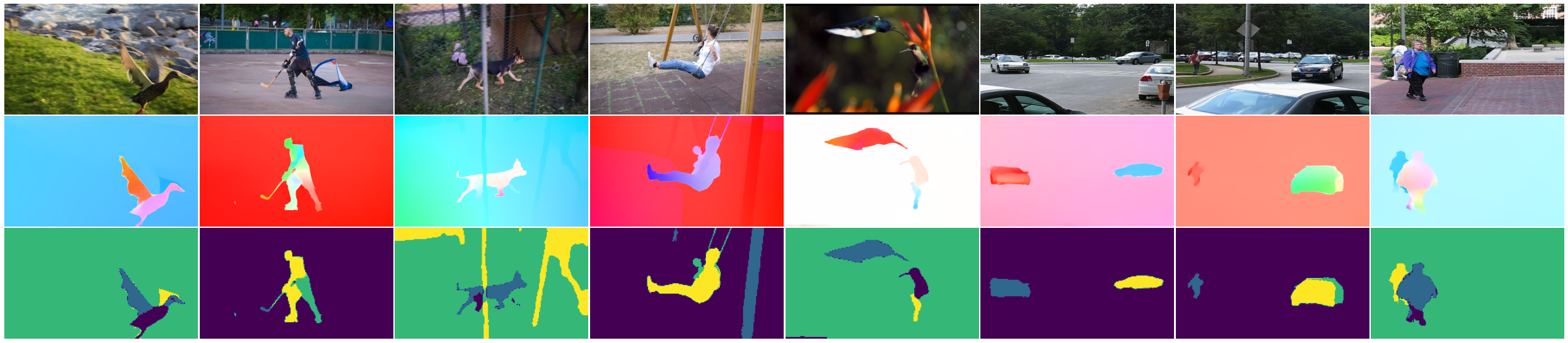
Results obtained with the EM-driven unsupervised learning method for four masks (K=4K=4) regarding multiple motion segmentation. First row: one image of the video. Second row: input optical flow displayed with the HSV color code. Third row: motion segmentation maps with four masks, one color per mask (the four masks may be not present if not necessary). We adopt the same color code for all segmentation maps (dark blue: mask 1, light blue: mask 2, green: mask 3, yellow: mask 4). Examples are drawn from DAVIS2016, FBMS59 and SegTrackV2 datasets.
Motion saliency detection based on an adversarial approach
Participants: Etienne Meunier, Patrick Bouthemy.
Continuing our previously published work on motion saliency detection, we designed an alternative approach based on an adversarial framework for training a convolutional neural network (CNN) to segment areas presenting salient motion in videos (see Fig. 16). This CNN exploits the predictions given by a frame-based classification network to learn to locate salient motions on input optical flow fields. The quality of the segmentation mask given by the CNN is measured by the probability produced by the classification network after inpainting the optical flow of the segmented region. This criterion, along with a regularization term measuring the coherence of motion in the segmented region, is used as loss function to train the CNN in a weakly supervised manner –no annotated segmentation masks are required–. In addition, we introduced a method based on constrained optimization to balance the different terms of the loss dynamically without introducing hyper parameters. This technique shows promising results for detecting abnormal motion in a crowd.
Collaborator: R. Fraisse (AIRBUS Defense and Space SAS)
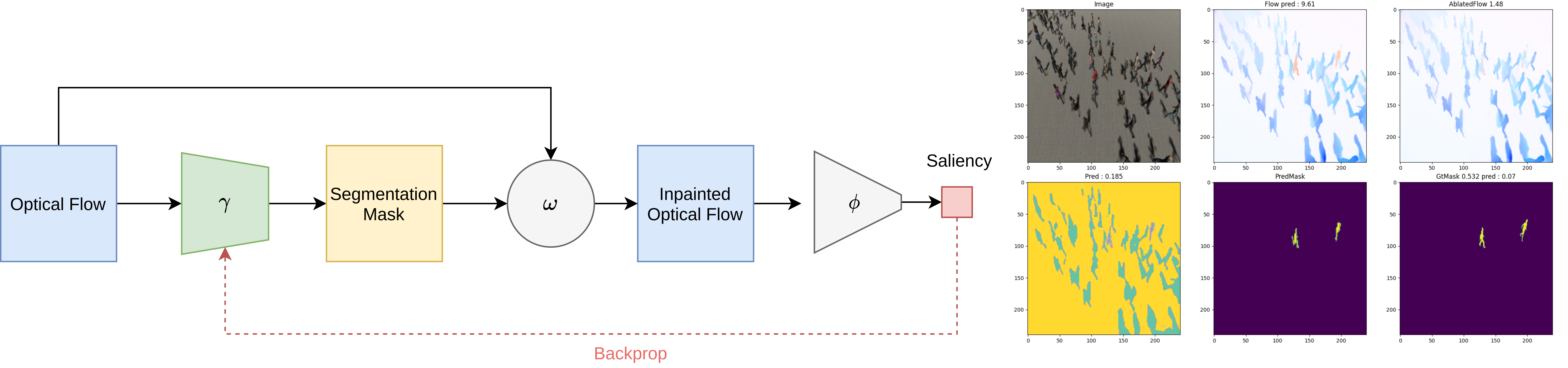
Motion saliency detection based on an adversarial approach. (Left) Descriptive diagram in which γ\gamma is the segmentation network we train, ω\omega is the differentiable flow inpainting operation and ϕ\phi is the saliency classifier. (Right) Preliminary results obtained on simulated crowd images. From left to right, top row: RGB image, input optical flow, ablated flow. Bottom row: Segmentation (in blue: region to be inpainted, in green: inpainting support), Segmented anomalies, GT anomalies. The predicted classification scores are shown above "Input optical flow" and "Ablated Flow".
Unsupervised space-time network for temporally-consistent segmentation of multiple motions
Participants: Etienne Meunier, Patrick Bouthemy.
Motion segmentation is one of the main tasks in computer vision and is relevant for many applications. Optical flow is the input generally used to segment every frame of a video sequence into regions of coherent motion. Temporal consistency is a key feature of motion segmentation, but it is often neglected. We have proposed an original unsupervised spatio-temporal framework for motion segmentation from optical flow that fully investigates the temporal dimension of the problem. More specifically, we have defined a 3D network for multiple motion segmentation that takes as input a sub-volume of successive optical flows and delivers accordingly a sub-volume of coherent segmentation maps. Our network is trained in a fully unsupervised way, and the loss function combines a flow reconstruction term involving spatio-temporal parametric motion models, and a regularization term enforcing temporal consistency on the masks. We have specified an easy temporal linkage of the predicted segments. Besides, we have designed a flexible and efficient way of coding U-nets. We carried out experiments on several benchmarks with convincing quantitative results, while not using appearance and not training with any ground-truth data. A paper has been submitted to a top-tier computer vision conference.
Collaborator: R. Fraisse (AIRBUS Defense and Space SAS)
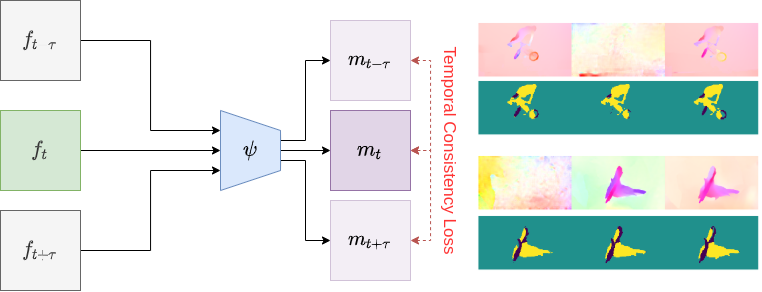
Unsupervised space-time network for temporally-consistent segmentation of multiple motions. (Left) Principle diagram of our space-time multiple motion segmentation network ψ\psi , taking as input a space-time sub-volume composed of three flow fields sampled every τ\tau time instants and delivering a sub-volume of three coherent segmentation maps. (Right) Results obtained with our method on two cases of amodal segmentation, in fact corresponding to a repeated image in the video file mimicking a stop (first row contains optical flow input, second row displays motion segments).
8.3 Modeling, simulation and estimation of spatiotemporal biological mechanisms and processes
Understanding the molecular interactions and intracellular transport mechanisms in space and time is central in fundamental cell biology to characterize cellular functions, and crucial to the treatment and cure of human disease. In this context, the modeling, classification, and estimation of diffusion processes has known a growing interest in statistics, biophysics, and biology with the popularization of fluorescence dynamical microscopy and super-resolution imaging.
In this research axis, we considered non-conventional spatiotemporal modeling, simulation and estimation frameworks and focused our activity on diffusion estimation and particle track analysis to address questions in cell biology that are related to cancer research (e.g., dynamics and interactions of proteins in exocytosis and endocytosis), neurobiology (e.g., astrocytic calcium signals) and antibiotic design (e.g., protein dynamics analysis over cell membrane of bacteria). While dedicated to target projects, our methodologies and concepts have been designed to adapt to a large range of biological studies.
Reinforcing interdisciplinary collaborations to unravel the astrocyte "Calcium Code"
Participant: Anaïs Badoual.
Calcium signals in astrocytes contribute to the modulation of neuronal communication as well as neurovascular coupling. Those signals display a striking spatiotemporal diversity. Elucidating their mechanisms and physiological roles is a major challenge and requires interdisciplinary approaches. In this project, we advocated for more collaborations across fields and disciplines working on astrocyte function. As early-career scientists with different backgrounds and expertise, we presented the major challenges faced in our fields: data acquisition, analysis and modeling of astrocyte calcium activity. We further highlighted the insights gained from those different approaches, aiming at bridging the gap between them to crack the complex astrocyte ‘Calcium Code’. Finally, we proposed strategies to promote fruitful interdisciplinary collaborative projects to unravel astrocyte function in health and disease.
Reference: 12
Collaborators: A. Denizot (Computational Neuroscience Unit, Okinawa Institute of Science and Technology, Onna, Japan); A. Covalo (INSERM, U1215 NeuroCentre Magendie, Bordeaux)
Birth and deaths processes for molecular dynamics analysis
Participants: Lisa Balsollier, Frédéric Lavancier, Ludovic Leconte, Cesar Augusto Valades-Cruz, Jean Salamero, Charles Kervrann.
Investigation of dynamic processes in cell biology very often relies on the observation in two dimensions of 3D biological processes. Consequently, the data are partial, and statistical methods and models are required to recover the parameters describing the dynamical processes. In the thesis of L. Balsollier (start in Oct 2021), we currently explore the birth-death-move processes that are stochastic processes describing the spatiotemporal evolution of a system of moving particles (possibly in interaction), and where births of new particles along with deaths of existing particles can occur over time. We studied the maximum likelihood estimator of a (possibly multidimensional) parameter involved in this dynamics. We proved, under some assumptions, that the underlying statistical model is locally asympotic normal (LAN), a theoretical property that ensures the asymptotic optimality of the maximum likelihood procedure. In particular, the consistency and asymptotic normality of this estimator follow when the observation time grows to infinity, with an explicit expression of the asymptotic variance. Simulations implemented in Python confirmed our theoretical findings. An example of the estimation of the range parameter involved in the birth density kernel of a new particle given an existing point pattern is shown in Fig. 18. Moreover, we currently investigate this modeling framework to decipher and quantify 3D spatiotemporal interactions of proteins involved in exocytosis (e.g., Rab11/TfR-Langerin) in living cells physically constrained or not on micro-patterns, and using multi-Angle TIRF microscopy 37 (Boulanger et al., 2014).
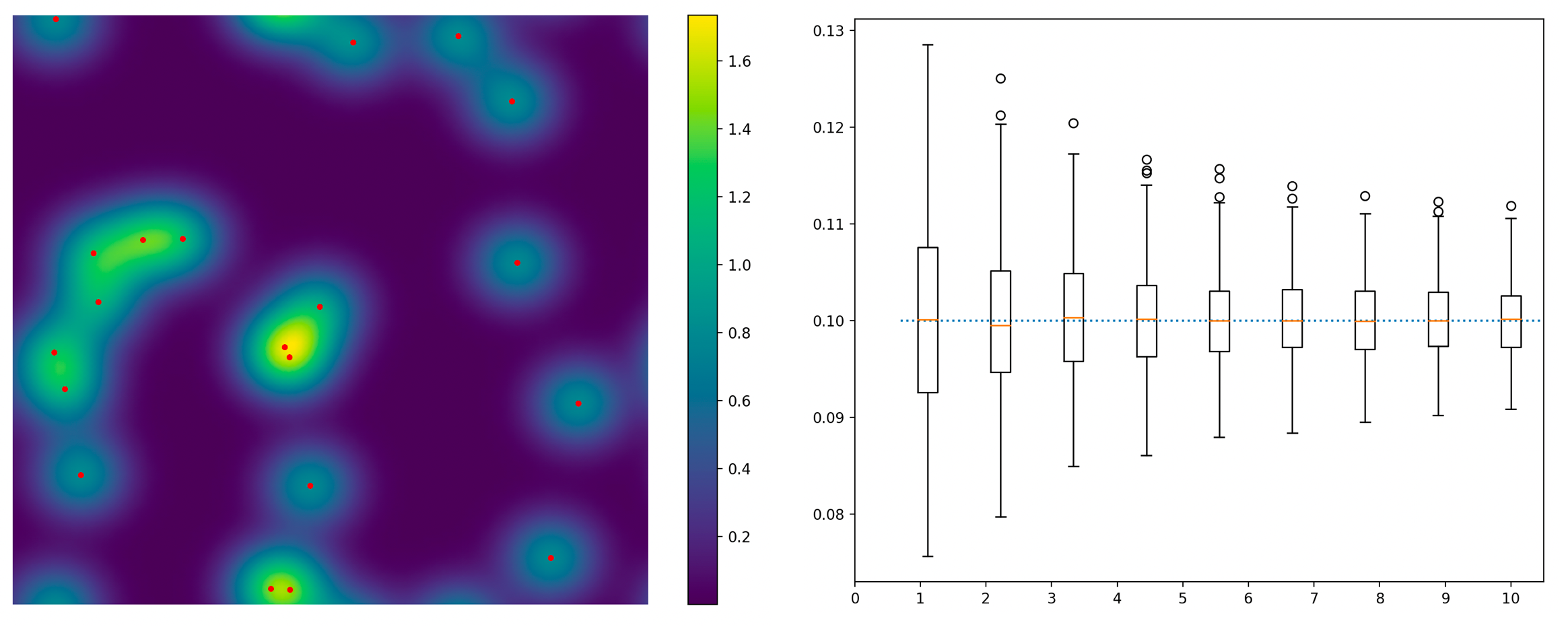
Estimation of the range parameter involved in the birth density kernel of a new particle given an existing point pattern. (Left) conditional probability density function for the birth of a new particle, given the red points that correspond to a frame of a birth-death-move sequence. (Right) Estimation of the range parameter of the latter density (encoding the size of the bright spots on the left-hand plot) over 300 replications of a birth-death-move sequence, when the sequence length increases from a factor 1 to a factor 10.
Hybrid Lagrangian-Eulerian approaches for molecular mobility estimation
Participants: Claire-Jing Rouchet, Cesar Augusto Valades-Cruz, Charles Kervrann.
Last year, we focused on the local estimation diffusion and drift from particle tracks. As starting point, we assumed that particle motion is governed by the Langevin equation. Given tracks and track segments labelled as 'Brownian', 'subdiffusive' or 'superdiffuse' 4, 5, we have proposed a hybrid Lagrangian-Eulerian method (DenseMapping) 43 to estimate both drift and diffusion in local neighborhoods centered on trajectory points. Unlike existing methods, the estimation of two or three-dimensional drift vector and the diffusion coefficient are performed on trajectory-sliding kernels, calculated at coordinates corresponding exactly to the coordinates given by the preliminary particle tracking. Also, trajectory classification allows one to calculate drift and diffusion coefficient maps separately on each class of motion with the most appropriate diffusion models: confined motion, Brownian motion, and directed motion.
The so-called DenseMapping method has been tested in several biological studies such as dynamics of transcription factors in the nucleus, biomolecules trafficking of endoplasmic reticulum luminal proteins, and virus dynamics analysis. In the PhD of C.-J. Rouchet, we currently study the spatiotemporal organization of cell wall elongation machineries in Bacillus subtilis and focus on the dynamics of MreB proteins over 3D cylindrical shape-based cell membrane of bacteria. Several components of the pipeline were optimized, ranging from cell preparation, acquisition with TIRM microscopy, and pre-processing (image filtering, particle tracking) before applying the DenseMapping approach to Halo-tagged MreB proteins (see Fig. 19).
Collaborators: R. Carballido-López and C. Billaudeau (INRAE, MICALIS UMR, Jouy-en-Josas)
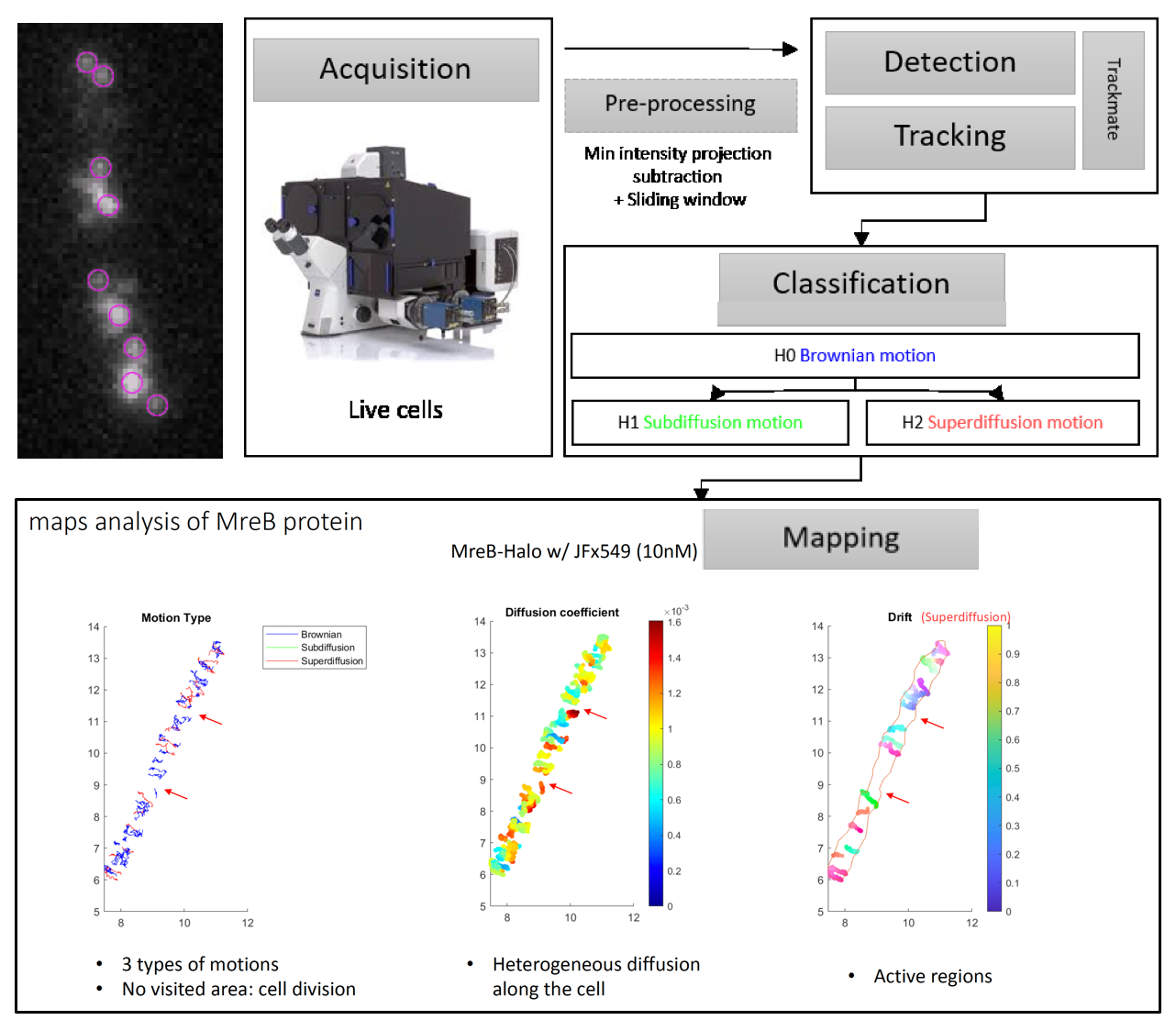
Characterization of micro-domain in bacterial membrane and interplay with the PGEM elements involved in the cell wall synthesis. The DenseMapping approach is applied to Halo-tagged MreB tracks estimated from 2D-TIRM videos. The localization of diffusive or directed MreB does not follow a specific pattern inside the cell. Areas without track in the cell are the localization of its septum. MreB diffusion is slightly heterogeneous between the middle of the cell and the poles but do not correlate with the type of motion.
9 Bilateral contracts and grants with industry
9.1 Bilateral contracts with industry
9.1.1 Contract with GATACA Systems: Super-resolution microscopy and in live cell imaging
Participants: Jean Salamero, Ludovic Leconte, Cesar Augusto Valades-Cruz, Charles Kervrann.
Funding: GATACA Systems company
Duration: (2017 – 2023)
Collaborator: C. Gueudry (GATACA Systems)
The last objective of the project (2022-2023) is to transfer innovations in High-Resolution Multi-Angle (MA) TIRFM (using Azymuthal TIRFM from Ilas2/3) and development of Polar MA-TIRFM (Polarization Multi-Angle TIRFM). This project has been reinforced by a call “Outreach”, launched by the National Research Infrastructure “France BioImaging” (POLARIMAGING, project, coordinated by S. Brasselet Institut Fresnel, Marseille), and the IDEX PSL-Labex Cell(n)Scale “groundbreaking call ” (POMIMO, project, coordinated by J. Salamero, Institut Curie SERPICO/STED).
9.1.2 Contract with CryoCapCell SA: 3D LIVE CLEM (Correlative Light and Electron Microscopy) to decipher fates and functions of exosomes in vivo
Participant: Jean Salamero.
Funding: DIM–ELICIT Empowering LIfe sCiences with Innovative Technologies (Région Ile de France)
Duration: (2018 – 2022)
Collaborators: G. Van Niel (coordinator, Institute of Psychiatry and Neuroscience of Paris), G. Raposo (UMR 144 CNRS - Institut Curie, PSL Research), X. Heiligenstein (CryoCapCell SA).
The objective of the project is to link dynamic biogenesis of intracellular membrane compartments with their ultrastructures. It combines fast high resolution photonic imaging (MA-TIRFM) and fast high pressure freezing for 3D cryoEM. It requires adapted registration methods in 3D, in order to navigate through the multiple scales.
9.2 Bilateral grants with industry
9.2.1 Contract with IRSN: DeepSuN – Localization of chromosomal aberrations induced by nuclear radiation dose excess
Participants: Antonin Deschemps, Charles Kervrann.
Funding: IRSN (Institut de Radioprotection et de Sureté Nucléaire) and Région-Bretagne
Duration: 36 months (Oct 2020 – Sep 2023)
Collaborator: M. Benadjaoud (IRSN/SERAMED, Fontenay-aux-Roses)
This project funded by the IRSN (Institut de Radioprotection et de Sureté Nucléaire) and Région-Bretagne concerns the PhD thesis (co-funding) carried out by Antonin Deschemps. The goal is to develop statistical and deep-learning methods for localizing and classifying chromosomal aberrations observed in 2D microscopy images (blood test) and estimating radiation dose following a postulated nuclear reactor accident. See details in Section 10.3.2 (ANR INCREASED).
9.2.2 Contract with AID: Localization and classification of gene translocation in FISH microscopy images
Participants: Quentin Tallon, Charles Kervrann.
Funding: AID (Agence de l'Innovation de Defense)
Duration: 36 months (Oct 2021 – Sep 2024)
Collaborator: M. Benadjaoud (IRSN/SERAMED, Fontenay-aux-Roses)
This project funded by the AID (Ministry of Defense) concerns the PhD thesis carried out by Quentin Tallon. The goal is to develop supervised and unsupervised machine learning methods and algorithms for detection and classification of gene translocation between chromosomes observed in FISH (Fluorescence in situ hybridization) microscopy images. See details in Section 10.3.2 (ANR INCREASED).
9.2.3 Contract with AIRBUS Defense and Space SAS: LION Chaine Image Elargie (LiChIE)
Participants: Sébastien Herbreteau, Etienne Meunier, Anaïs Badoual, Sylvain Prigent, Emmanuel Moebel, Patrick Bouthemy, Charles Kervrann.
Funding: Bpifrance / Projets Structurants pour la Compétitivité (PSPC)
Duration: 65 months (Aug 2019 – Dec 2024)
Collaborators: M. Ortner and R. Fraisse (AIRBUS Defense and Space SAS)
This project funded by Bpifrance concerns the PhD theses carried out by Sébastien Herbreteau and Etienne Meunier, the 6-month postdoc position of Anaïs Badoual, and the engineer positions of Sylvain Prigent (2021-2022) and Emmanuel Moebel (2022-2023). The goal is to develop statistical and deep-learning methods for image restoration in night conditions and motion saliency detection in image sequences, respectively. The resulting algorithms will be embedded in hardware platforms for a next generation of observation satellites.
10 Partnerships and cooperations
10.1 International initiatives
10.1.1 Participation in other International Programs
Global BioImaging (GBI)
Participant: Jean Salamero.
Since 2019, GBI is funded in part by a grant from the Chan Zuckerberg Initiative DAF, an advised fund of the Silicon Valley Community Foundation. J. Salamero is member of the Management Board of "Global Bioimaging" (GBI)(INFRASUPP-6-2014 - International Cooperation for Research Infrastructure) since 2014. He is co-leader of the WP "Training courses for facility staff" (Task leader Rainer Pepperkok, EMBL), and since 2019, member of the WG "Societal Impact of Imaging Infrastructures".
African BioImaging Consortium (ABIC) and AMI (African Microscopy Inititative)
Participant: Jean Salamero.
Partnership (since October 2021, for France BioImaging (FBI), J. Salamero) with ABIC (African BioImaging Consortium), a new Pan-African Consortium in BioImaging co-funded by the Chan Zuckerberg Initiative DAF, an advised fund of the Silicon Valley Community Foundation, and with AMI since 2022 funded by Chan Zuckerberg Initiative and Bill and Melinda Gates Foundation (BMGF). France-BioImaging is an international partner involved in training, pitch program and managing imaging core facilities.
Informal international partners
Participants: Anaïs Badoual, Charles Kervrann, Jean Salamero, Ludovic Leconte, Emmanuel Moebel, Cesar Augusto Valades-Cruz.
-
–
Collaboration with Biocomputing Unit, CSIC Madrid, Spain: Integration of DeepFinder software into the Scipion platform dedicated to cryo-electron microscopy image analysis (Plugin DeepFinder in Scipion). (with E. Moebel and C. Kervrann)
-
–
Collaboration with EPFL, Biomedical Imaging Group, Lausanne, Switzerland: Dissemination and teaching of deep-learning for bioimage analysis (with A. Badoual):
-
>"Traitement et Analyse d’Images en Microscopie", book in preparation (with D. Sage)
-
>Courses through different events (ANF DeepScopie 2022, BioImage Analysis course at Gothenburg University 2022, Mifobio 2023 in preparation) (with D. Sage)
-
>
-
–
Collaboration with the Cambridge Advanced Imaging Centre, Cambridge, UK: Dense mapping for transcription factors analysis in single molecule localization microscopy. (with C. Kervrann)
-
–
Collaboration with Computational Neuroscience Unit, Okinawa Institute of Science and Technology Graduate University (OIST), Japan: Generation of synthetic datasets of astrocytic calcium activity to support the development of learning based analysis tools; Advocating for interdisciplinary collaborations to unravel the astrocyte 'Calcium Code'. (with A. Badoual)
-
–
Collaboration with the PKU University, Institute of Molecular Medicine, Beijing, People Republic of China: Development of robust mitochondria fluorescent probes and validation in LLSM, STED and LiveSR microscopy for Live cells. (with C.A. Valades-Cruz, L. Leconte, and J. Salamero)
10.1.2 Visits to international teams
Research stays abroad in the frame of the French-Canadan MITACS Globalink program
Participants: Antonin Deschemps, Charles Kervrann.
Funding: MITACS Globalink
Duration: 36 months (2020 – 2022)
Collaborator: Q. Fang (McMaster University, Engineering Physics, Hamilton Toronto, Canada)
In this project, we have initiated a new collaborative partnership that integrates the expertise of advanced optical imaging instrumentation (McMaster) and image sequence analysis (Inria) towards new dynamic imaging technologies. We focus on optofluidics imaging flow cytometers for point-of-care infectious diseases diagnosis as well as high throughput protein-protein interaction measurements in live cell imaging. A key novelty of this project is, instead of relying static 2D images and morphological features, we acquire 3D videos depicting particles in flows and use motion analysis to perform detection and classification. Such research is expected to have direct impact in drug discovery and anti-viral therapy.
10.2 European initiatives
10.2.1 Other european programs/initiatives
ESFRI initiative program: EuroBioImaging
Participant: Jean Salamero.
Coordinator: J. Eriksson (Turku University, Finland)
Funding: Member states of the European Union
Partners: 18 European countries in 2022 (+1 observer)
As a member of the National Research Infrastructures (RI) France BioImaging, SERPICO is involved in the ESFRI Euro-BioImaging project, and now in the ERIC EuroBioImaging (since November 2019), one of the landmarks of biomedical science Research Infrastructures in the roadmap of the European Strategic Forum on Research Infrastructures (ESFRI 2018). The mission of Euro-BioImaging is to provide access, service and training to state-of-the-art imaging technologies and foster the cooperation and networking at the European level including multidisciplinary scientists, industry, regional, national and European authorities.
J. Salamero is a special advisor of the MESRI and CNRS French Representatives in the ERIC-Euro-BioImaging board.
10.3 National initiatives
10.3.1 France-BioImaging project
Participants: Jean Salamero, Charles Kervrann, Léo Maury, Sylvain Prigent, Cesar Augusto Valades-Cruz, Ludovic Leconte.
Duration: 2011 – 2024
Funding: Investissement d'Avenir, ANR INBS-PIA1 2011 and “FBI Next Generation” (ANR program 2020-2024)
Coordinator: E. Bertrand (UMR 9002 CNRS)
Partners: CNRS, Aix-Marseille Université, Collège de France, Ecole Normale Supérieure, Ecole Polytechnique, Inria, Institut Curie, Institut Pasteur, Inserm, Université de Bordeaux, Université de Montpellier, Université de Nantes, Université de Paris, Université de Rennes 1.
SERPICO is a member of the French initiative, the so-called “France-BioImaging” (FBI) National Research Infrastructure which gathers several outstanding cellular imaging centers (microscopy, spectroscopy, probe engineering and signal processing). FBI is on the French Roadmap of Research Infrastructure. The mission of FBI is to build a distributed coordinated French infrastructure for photonic and electronic cellular bioimaging, dedicated to innovation, training and technology transfer. High-computing capacities are needed to exhaustively analyze image flows.
SERPICO is co-head of the IPDM (Image Processing and Data Management) node of the FBI network composed of 7 nodes. In this context, we address the following scientific problems: i/ exhaustive analysis of bioimaging data sets; ii/ deciphering of key steps of biological mechanisms at organ, tissular, cellular and molecular levels through the systematic use of time-lapse 3D microscopy and image processing methods; iii/ storage and indexing of extracted and associated data and metadata through an intelligent data management system. SERPICO recruited R&D engineers to disseminate image processing software, to build the Mobyle@serpico web portal and to manage the IGRIDA-SERPICO cluster opened for end-users and dedicated to large scale computing and data sets processing.
Two projects involving the Serpico Team were selected to FBI open calls in 2021 and 2022:
-
–
"BioImageIT Dissemination" project, 18 months (2021-2023), coordinated by J. Salamero, with the particpation of S. Prigent C.-A. Valades-Cruz, L. Leconte, and C.Kervrann. This project concerns the engineer position of L. Maury.
-
–
POLARIMAGING project, 18 months (2022-2024), coordinated by S. Brasselet (Institut Fresnel, Marseille), with the participation of J. Salamero, C.-A. Valades-Cruz, L. Leconte, and C.Kervrann.
10.3.2 ANR INCREASED project: Artificial intelligence for the detection of chromosomal aberrations in dosimetry
Participants: Antonin Deschemps, Quentin Tallon, Emmanuel Moebel, Charles Kervrann.
Duration: 36 months (Oct 2020 – Sept 2023)
Funding: ANR (Agence Nationale de la Recherche) ASTRID
Coordinator: Gaetan Gruel (Institut de Radioprotection et de Sureté Nucléaire (IRSN/LRAcc), Fontenay-aux-Roses)
Partners: IRSN/LRAcc and IRSN/SERAMED, SERPICO Team, IRBA (Institut de Recherche Biomédicale des Armées)
During insidious scenarios, when the assessment of ionizing radiation exposure condition is difficult or impossible, the quantification, on samples taken from the victims, of radio-induced chemical or biological effects is more suitable for an individualized dose reconstruction compared to theoretical calculation or Monte-Carlo simulations in regard to their great uncertainties. The INCREASED project aims to adapt the most powerful algorithms of modern artificial intelligence to the context of automatic detection of chromosomal aberrations in dosimetry. This project proposes to revisit the semi-automatic or automatic detection methods currently used for the recognition of dicentrics in GIEMSA imagery in the light of the most recent advances in artificial intelligence and deep-learning. These modern methods, which demonstrated their indisputable superiority in other areas of computer vision, will be deployed on GIEMSA images for an exhaustive multi-class count not only of dicentric chromosomes but also of ring-centric aberrations, acentric fragments, and even tricentrics. Furthermore, the INCREASED initiative is also quite innovative in dosimetry based on FISH imaging. To date, this type of dosimetric reconstruction is based on manual counting of non-exhaustive and very simplified annotations of the different possible forms of translocations. This protocol is therefore as heavy as imprecise. The INCREASED project addresses two major improvements: i/ design of a universal, rigorous and exhaustive scoring of the different forms of observable FISH translocations (3 colors). ii/ development of modern artificial intelligence algorithms able to detect and translate co-locations/co-neighborhoods into different categories of translocations. This project concerns the post-doc position of Emmanuel Moebel in 2021.
10.3.3 Labex Cell(n)Scale PoMIMO – Polarization Microscopy for Imaging of Membrane Organization
Participants: Jean Salamero, Charles Kervrann, Cesar Augusto Valades-Cruz, Ludovic Leconte.
Duration: 36 months (2022 – 2024).
Funding: Labex Cell(n)Scale
Coordinator: J. Salamero and C. Kervrann.
Partners: U1143/UMR 3666 (Institut Curie), UMR 168 (Institut Curie), GATACA Systems company.
The main objective is to study the molecular organization of cargo proteins and membrane lipids by adding information on their dipole orientations. To do so, we develop innovative polarization modalities adapted to available microscopic setups and appropriate computational methods. We plan to use chemical engineering of lipid and protein-based fluorescent labels to make them rigid for polarization imaging. We expect to create a new imaging approach that will not only provide novel information about endocytosis, but will have the potential to be applied to all topics where the spatial and molecular organization of lipids/proteins defines both the structure and function of these assemblies (i.e., mitochondria cristae, MAMs....) in reconstituted systems and in living cells.
10.3.4 Inria DEFI NAVISCOPE: image-guided NAvigation and VISualization of large data sets in live cell imaging and microsCOPy
Participants: Gwendal Fouché, Kévin Fournier, Cesar Augusto Valades-Cruz, Ludovic Leconte, Anaïs Badoual, Jean Salamero, Charles Kervrann.
Duration: 60 months (2018 – 2022)
Funding: Inria
Coordinator: C. Kervrann
Partners: AVIZ (Inria Saclay), BEAGLE (Inria Lyon), HYBRID(Inria Rennes), MORPHEME (Inria (Sophia-Antipolis), MOSAIC (Inria Lyon), PARIETAL (Inria Saclay), SERPICO (Inria Rennes), MaIAGE INRAe Unit (Jouy-en-Josas), UMR 144 CNRS and UMR 168 CNRS - Institut Curie (Paris), UMR 3571 CNRS - Institut Pasteur (Paris)
Microscopy image exploration is routinely performed on 2D screens, which limits human capacities to grasp volumetric complex biological dynamics. With the massive production of multidimensional images (3D + time, multi-channels) and derived images (e.g., restored images, segmentation maps, and object tracks), scientists need performant visualization and navigation methods to better handle information in videos.
In the "Naviscope" DEFI project, our objective was then to develop cutting-edge visualization and navigation methods and techniques to better explore temporal series of multi-valued volumetric images, with a strong focus on live cell imaging and microscopy application domains. Naviscope, built upon the strength of scientific visualization and artificial intelligence methods, aimed to provide systems capable to assist the scientist in obtaining a better understanding of massive amounts of information. The resulting technologies must be capable of have of selecting and displaying the most informative regions in videos, guiding the observer attention, and interacting with the image contents. The teams involved in the consortium addressed the three following problems:
-
–
Development of novel visualization methods capable of displaying heterogeneous dynamics with appropriate spatiotemporal representations and abstractions.
-
–
Design of novel machine learning strategies to localize regions of interest, make corrections on derived data (segmentation maps), and enrich annotations in datasets.
-
–
Conception of effective navigation and interaction methods for the exploration and analysis of sparse sets of localized intra-cellular events and cell processes (migration, division, etc.).
Moreover, another challenge was to gather up the software developed in several teams to provide, as much as possible, a unique original and usable tool for users in biological imaging, and potentially in medical imaging, and worked to ensure that these communities take ownership of this tool.
This project concerns the PhD thesis carried out by Gwendal Fouché and the engineer position of Kevin Fournier in 2022.
10.3.5 CNRS Project 80|Prime ModSpatioTempCell: Spatiotemporal stochastic models for the comprehension of membrane trafficking at the single cell scale
Participants: Lisa Balsollier, Frédéric Lavancier, Jean Salamero, Charles Kervrann.
Duration: 36 months (Oct 2021 – March 2023).
Funding: CNRS
Coordinator: F. Lavancier.
Partners: SERPICO Team, UMR 144 CNRS - Institut Curie, Laboratoire de Mathématiques Jean-Leray Nantes, UMR 6629 CNRS .
Endocytosis and exocytosis mechanisms involve multiple molecular components that interact through space and time according to a complex process. In this project, we plan to analyze the spatiotemporal activity of some of these proteins thanks to a tailored mathematical model, fitted to sequences of real data acquired by fluorescence microscopy. The mathematical model at hand characterizes the dynamics of a system of interacting particles with possible appearance and disappearance of some particles through time, in accordance with the observed dynamics of proteins in cell membrane trafficking. This project concerns the PhD thesis carried out by Lisa Balsollier (since October 2021).
10.3.6 INRAE DIGITBIO - BovMovie2Pred: Bovine early development and sustainability, exploration and prediction
Participant: Patrick Bouthemy.
Duration: 12 months (2021 – 2022).
Funding: INRAE
Coordinator: Alain Trubuil (INRAe, MaIAGE Unit, Jouy-en-Josas)
Partners: INRAE (MaIAGE Unit, Jouy-en-Josas, MIA Unit Paris), UMR BREED (Ecole Nationale Vétérinaire d’Alfort), SERPICO Team
The current performance of in vitro fertilization and embryo transfer in cattle is around 30% of viable births. The selection of fertilized embryos is based on a classification at D7 after fertilization. The objective of the consortium is to optimize the selection of embryos as early as possible by exploiting the morphokinetics history, from fertilization to the day of selection. For that, we have gathered methodological competences around embryonic development, microscopy video analysis, machine learning and deep-learning. We have built a challenge in the context of the DATA-IA RAMP platform that is accessible here. It is open to any partners and especially (Master 2) students, to provide researchers in developmental biology with state-of-the-art classification software, requiring as little expert annotation of videos as possible. In addition, within a 3-month Master 1 internship (M. Meignin), we have designed a preliminary deep-learning solution for an early classification of embryo videos (at D3), concatenating a convolutional neural network and a recurrent one. In addition, a segmentation method to delineate the embryo in the image has been defined and experimentally validated.
10.3.7 INRAE - MreB: Role of membrane microdomains in bacterial morphogenesis
Participant: Charles Kervrann.
Duration: 36 months (Oct 2020 – Sep 2023)
Funding: INRAE
Coordinator: Carballido-Lopez (INRAE UMR MICALIS, Jouy-en-Josas)
Partners: INRAE UMR MICALIS (Jouy-en-Josas), SERPICO Team
This project concerns the PhD thesis carried out by Claire-Jing Rochet (since October 2020). The goal is to understand how dynamic molecular interactions are regulated in time and in space to form functional machineries that establish long-range orders and cellular functions. To this end we combine cutting-edge high-resolution fluorescence microscopy and spectroscopy techniques with powerful genetic, biophysical, biochemical and systems biology approaches available in model bacteria, in particular the rod-shaped Gram-positive bacterium Bacillus subtilis, to determine mechanistic details underlying basic cellular and developmental processes: the molecules, macromolecular assemblies, cellular events and physiological adaptations involved. Understanding how bacterial cells grow has important implications for the identification of new antimicrobial targets and for the development of synthetic biology tools for bioproduction and biotechnology purposes.
10.3.8 PIA3 project Shared digital spaces for life sciences - MuDIS4LS: Mutualised Digital Spaces for FAIR data in Life and Health Science
Participant: Jean Salamero.
Duration: (2021- )
Funding: ANR (ESR/Equipex+ PIA)
Coordinator: INBS Institut Français de Bioinformatique (IFB)
The national project MuDIS4LS brings together 39 teams from 14 organizations, 4 national data centers, 7 regional data centers, and 6 national data-producing infrastructures. Jean Salamero particitates to the IS-1 program on behalf of France BioImaging (design and implementation of a "BioImaging Structure DMP" on behalf of FBI, with the INBS EMBRC-FR and IFB, see tutorial).
10.4 Regional initiatives
10.4.1 Deep-SuN: Localization of chromosomal aberrations induced by nuclear radiation dose excess
Participants: Antonin Deschemps, Charles Kervrann.
Participants: Antonin Deschemps, Charles Kervrann.
Funding: IRSN (Institut de Radioprotection et Sureté Nucléaire) and Région-Bretagne
Duration: 36 months (Oct 2020 – Sep 2023)
This project concerns the PhD thesis carried out by Antonin Deschemps, in collaboration with IRSN/SERAMED (see Section 9.2.1).
11 Dissemination
Participants: Charles Kervrann, Jean Salamero, Patrick Bouthemy, Anaïs Badoual, Sylvain Prigent, Ludovic Leconte, Cesar Augusto Valades-Cruz, Emmanuel Moebel, Léo Maury, Frédéric Lavancier, Sébastien Herbreteau, Gwendal Fouché, Etienne Meunier, Lisa Balsollier, Quentin Tallon.
11.1 Promoting scientific activities
11.1.1 Scientific events: organization/participation
Member of the organizing committees
-
–
Jean Salamero was:
-
>organizer of the meetings of the "Club des INBS (National Research Infrastructures in Biology and Health)”, every 1 to 2 months, since December 2020 (all by online conferences, except the 15th of December in Institut Curie, Paris, France);
-
>co-organizer of the meeting “Rencontre des INBS et de leur tutelles », Auditorium de Paris Santé Campus, Paris, France, June 2022;
-
>co-organizer and round table chair of the “digital spaces (VR/AR) for biology and medicine”, Insitut Curie, Paris, France, July 2022.
-
>
-
–
Patrick Bouthemny was organizer of CominLabs days, Rennes, France, March 2022 (held virtually) and October 2022.
-
–
Frédéric Lavancier is co-organizer of the online "seminar on spatial and spatiotemporal point processes and beyond” (OSSP).
Participation to events
-
–
Jean Salamero participated to:
-
>GBI EoE VII. SDGs in BioImaging with the WG Impact, Montevideo, Uruguay, online meeting, September 2022;
-
>First international AMI symposium and Inauguration of the ABIC (first open imaging facility in Africa), Cape Town University, South Africa, October 2022;
-
>"Les RDV Carnot", the "village des infras" (presentation of the "France-BioImaging"activities), Paris, France, October 2022.
-
>
11.1.2 Scientific events: selection
Member of the conference program committees
-
–
Charles Kervrann is member of scientific committee of IABM’2023 (Colloque Français d’Intelligence Artificielle en Imagerie Biomédicale).
-
–
Patrick Bouthemy is Associate Editor for the Intl ISBI’2023 conference and member of scientific committee of IABM’2023 (Colloque Français d’Intelligence Artificielle en Imagerie Biomédicale).
Reviewer
-
–
Anaïs Badoual, Etienne Meunier, Emmanuel Moebel, and Sébastien Herbreteau served as reviewers for IEEE ISBI’2023.
-
–
Frédéric Lavancier was reviewer for GRETSI'22.
11.1.3 Journal
Member of the editorial boards
-
–
Charles Kervrann is Associate Editor for the "Transactions on Image Processing" and "Biological Imaging" journals since 2021. He is Guest Associate Editor for a Special Issue of "Journal of Selected Topics in Quantum Electronics" (to appear in 2023).
Reviewer - reviewing activities
-
–
Charles Kervrann and Emmanuel Moebel were reviewers for "Nature Communications".
-
–
Jean Salamero was reviewer for "Nature Methods", "Nature Communications", "Bioinformatics", and "Traffic".
-
–
Anaïs Badoual was reviewer for "Biological Imaging", "PLoS Computational Biology", "Journal of Computational and Applied Mathematics","Scientific Reports", and "SIAM Journal on Imaging Sciences".
-
–
Frédéric Lavancier was reviewer for "Spatial Statistics" and "Journal of the Royal Statistical Society: Series C".
-
–
Cesar Augusto Valades-Cruz was reviewer for "Journal of Physical Chemistry Letters".
-
–
E. Moebel was reviewer for "IEEE/ACM Transactions on Computational Biology and Bioinformatics", and "Nature Communications".
11.1.4 Invited talks
-
–
Charles Kervrann
-
>"Statistical and artificial intelligence methods for live-cell fluorescence imaging", Young Scientists Network (YSN, GdR ImaBio) Conference, Lyon, France, July 2022; Meeting "France-BioImaging / Montpellier Node", University of Montpellier, France, October 2022.
-
>"Statistical and AI methods for quantification in live-cell fluorescence imaging : promises and perspectives", Meeting BioSensors (CNRS-GdR ImaBio), Rennes, France, November 2022.
-
>
-
–
Jean Salamero:
-
>"Presentation of FBI activities" at the ABIC consortium africanbioimaging.org to the Dakar Institute of Technology, UCAD (Université Cheik Anta Diop, Dakar, Senegal), IRD office ( Mauritanie-Senegal), VPs research and International affair of Aix Marseille University, Bureau joint CNRS/IRD/CIRAD Pretoria, South Africa, and Hassan II university-Casablanca, Morocco, from January to December 2022.
-
>“Past and Future challenges in Research Infrastructure in bioimaging", France-BioImaging Annual National Meeting, Nantes, France, December 2022.
-
>
-
–
Anaïs Badoual:
-
>“Simulation of astrocytic calcium signals in microscopy images to support the development of a learning-based analysis tool”, (Invited Talk), France-BioImaging Annual National Meeting, Nantes, France, December 2022.
-
>"Snakes: segmentation of microscopy images", RTmfm Webinar series, March 2022.
-
>
-
–
Frédéric Lavancier:
-
>"Birth-death-move processes: basic properties and estimation of their intensity functions”, OSSP online seminar, January 2022; “Point processes” day, FRUMAM, Marseille, France, December 2022.
-
>"Modélisation et inférence des processus ponctuels spatiaux", Crash Course, “Point processes” day, FRUMAM, Marseille, France, December 2022.
-
>
-
–
Sylvain Prigent:
-
>"BioImageIT: Integration of data management with analysis", RTmfm Webinar series, January 2022.
-
>
-
–
Léo Maury:
-
>"BioImageIT: Integration of data management with analysis", France-BioImaging Annual National Meeting, Nantes, France, December 2022.
-
>"BioImageIT: Integration of data management with analysis", UMR 144 CNRS Institut Curie Seminar, Paris, France, November 2022.
-
>
-
–
Emmanuel Moebel:
-
>"How collaboration between biologists and computer scientists allows to uncover new mechanisms of the cell", (with F. Fatmaoui , IGBMC Strasbourg) Meeting INRAE DIGIT-BIO "L'IA en Sciences du Vivant", Paris, France, April 2022.
-
>"Finding is believing: AI reveals the presence of macromolecules in 3D cellular cryogenic electron tomograms", Symposium AVIESAN/ITMO PMN and TS: "A walk through the use of AI in experimental biology and bio-medicine", Paris, France, April 2022.
-
>
-
–
Quentin Tallon:
-
>"Artificial Intelligence for the automatic detection of chromosomal translocations. Application to retrospective dosimetry based on FISH imaging", IMRIS (International Military Radiation and Innovation Symposium), Paris, France, November 2022.
-
>
11.1.5 Leadership within the scientific community
-
–
Patrick Bouthemy is member of the board of AFRIF (Association Française pour la Reconnaissance et l’Interprétation des Formes) and of the jury for the AFRIF thesis prize.
-
–
Jean Salamero is leader of the Africa-France FBI project (calls and training activities starting beginning 2023), special advisor of the MESR and CNRS French Representatives in the ERIC-Euro- BioImaging Management board (2020-2022), and Mission Officer appointed by the Executive Board of FBI, 2020-2022:
-
>in charge of activities and reorganization of the FBI IdF Nodes (IdF-SUD and Paris-Centre): preparation for and integration of the Genethon and IGR imaging platforms (IBiSA accredited end of 2021) into the IdF-SUD node of FBI. In 2022-2023, ongoing integration of the MIMA2 Inrae imaging platform (Jouy-en-Josas) into the IdF SUD Node.
-
>in charge of activities and inter-Research Infrastructures animation in Biology and Health": animation of the "Club des INBS" (internal meetings every 1-2 months), organization of symposia related to diverse topics in research infrastructure activities.
-
>
-
–
Frédéric Lavancier is member (leadership) of the board of the CNRS GdR GeoSto (3477 - Géométrie stochastique).
11.1.6 Scientific expertise
-
–
Charles Kervrann was member of the selection committee for the recruitment of Inria junior researchers (CRCN) in 2022.
-
–
Jean Salamero reviewed 2 projects for NOW (The Netherlands, National Roadmap of Research Infrastructures 2021-2022), 2 projects for the Danish Ministry of Higher Education and Science for their National Roadmap of Research Infrastructures, and 6 projects BINA funding program ("Professional Development (PD) program BINA"). He is France-BioImaging delegate to the Working Group "Research Infrastructure and Industry" of the MESR-DGRI.
-
–
Patrick Bouthemy was member of the Scientific Advisory Board (SAB) of the Interdisciplinary Center for Scientific Computing (IWR, University of Heidelberg, Germany, May 2022). He was head of the selection committee for a Professor position at INSA Rennes in 2022. He is member of the thesis selection committee for the national Excellence Future & Ruptures Program of IMT (2020-2022).
-
–
Frédéric Lavancier was reviewer for ANR (France) and the Czech Science Foundation.
11.1.7 Research administration
-
–
Charles Kervrann:
-
>Member of the executive board of the project committee "Bureau du Comité des Projets" of the Inria Rennes - Bretagne Atlantique centre since 2010.
-
>Co-head of the "BioImage Informatics" node (ANR France-BioImaging project, National Research Infrastructure" for Biology and Health) since 2011.
-
>
-
–
Jean Salamero:
-
>Member of the Advisory Board of EMBL Core Facilities.
-
>Member of the Qlife “Institut Convergences” since 2018.
-
>Member of the Steering Committee of the DIM-ELICIT program (Région Ile de France) (2016-2022).
-
>
-
–
Patrick Bouthemy:
-
>Head of Excellence Lab (Labex) CominLabs(until Jul 2022).
-
>Member of the Selection and Validation Committee of the Images & Réseaux competitivity cluster.
-
>Head of the ANR evaluation committee for the 2022 call for proposals regarding the Artificial Intelligence field.
-
>Head of the evaluation committee of the ANR - JST CREST call for proposals on Trusted quality AI systems (2021 and 2022 calls)
-
>Member of the steering committee of the medical imaging Neurinfo platform.
-
>
-
–
Frédéric Lavancier:
-
>Head of the CNRS Project 80|Prime " ModSpatioTempCell : Spatio-temporal stochastic models for the comprehension of membrane trafficking at the single cell scale".
-
>
11.2 Teaching - Supervision - Juries
11.2.1 Teaching
-
–
Charles Kervrann:
-
>Master 2: "From Bioimage Processing to BioImage Informatics", 3 hours (4.5 hours TD), coordinator of the module (30 hours / equiv 45 hours TD), Master 2 Research IRIV, Telecom-Physique Strasbourg and University of Strasbourg.
-
>Engineer Degree (3rd year) and Master 2 Statistics and Mathematics: Statistical Models and Image Analysis", 27.5 hours (41.25 hours TD), Ecole Nationale de la Statistique et de l'Analyse de l'Information (ENSAI), Bruz.
-
>
-
–
Anaïs Badoual:
-
>Engineer Degree (3rd year): "Statistical Models and Image Analysis", 15 hours (TP / Practical courses), Ecole Nationale de la Statistique et de l'Analyse de l'Information (ENSAI), Bruz.
-
>Master degree: "Analysis of Image Sequences", 8 hours (12 hours TD), Master 2 Research SISEA, ISTIC & University of Rennes 1.
-
>Master degree: "Object Tracking in microscopy", 1.75 hours (3.5 hours TD), Master 2 Research IRIV, Telecom-Physique Strasbourg.
-
>PhD degree: "BioImage Analysis", 6.5 hours (TD), Gothenburg University, Sweden.
-
>
-
–
Frédéric Lavancier:
-
>Master degree : "Linear and Logistic Regression Models" 64 hours (96 hours TD), University of Nantes.
-
>Master degree: "High Dimensional Statistics", 56 hours (84 Hours TD), University of Nantes.
-
>
-
–
Sébastien Herbeteau:
-
>Licence (L2) degree: "Linear algebra", 18 hours TD, INSA Rennes.
-
>Licence (L2) degree: "Introduction to probabilities theory", 24 hours TD, INSA Rennes.
-
>
-
–
Gwendal Fouché:
-
>Master (M2) degree: "Virtual Reality", 3 hours TD, Ecole Polytechnique, Palaiseau.
-
>Master (M1) degree: "Virtual and augmented Reality", 9 hours TD, CentralSupélec, Rennes.
-
>
-
–
Etienne Meunier:
-
>Master degree: "Machine Learning", 22 hours TP, EFREI, Paris.
-
>
-
–
Lisa Balsollier:
-
>Licence (L2) degree : "Probabilities", 24 hours TD, University of Rennes 1.
-
>Licence (L2) degree : "Statistics", 24 hours TD, University of Rennes 1.
-
>Master degree : "Probabilities and statistics (for "agrégation interne"), 3 hours (4,5 hours TD) and 11,5 hours TD, University of Rennes 1.
-
>
-
–
Sylvain Prigent and Anaïs Badoual:
-
>ANF DeepScopie: "Fundamentals and Practice of Deep-Learning for Microscopy", 3 sessions of 4 hours (practice), 2022.
-
>
-
–
Cesar Augusto Valades-Cruz, Jean Salamero, Ludovic Leconte, Léo Maury:
-
>Atelier Inserm "Light-Sheet Fluorescence Microscopy (LSFM)", University of Bordeaux, 19-22 May 2022, Theoretical Phase; 21-23 June 2022, Institut Curie, Paris, Practical Phase.
-
>
-
–
Ludovic Leconte:
-
>EMBO Practical Course "Light-Sheet Microscopy", 15-26 August, Brno, Czech Republic.
-
>
11.2.2 Supervision
-
–
Sébastien Herbreteau (PhD in progress): "Deep-Learning for image restoration in night conditions" (started in october 2020, supervised by C. Kervrann).
-
–
Antonin Deschemps (PhD in progress): "Statistical learning for localization and classification of chromosomal aberrations induced by nuclear radiation dose excess" (started in October 2020, supervised by C. Kervrann and M.A. Benadjaoud (IRSN/SERAMED, Fontenay-aux-Roses)).
-
–
Etienne Meunier (PhD in progress): "Deep-Learning for saliency detection in image sequences" (started in September 2020, supervised by P. Bouthemy).
-
–
Claire-Jing Rouchet (PhD in progress): "Role of membrane microdomains in bacterial morphogenesis" (started in October 2020, supervised by R. Carballido-Lopez, C. Billaudeau (INRAE MICALIS Unit, Jouy-en-Josas), and C. Kervrann).
-
–
Quentin Tallon (PhD in progress): "Statistical learning for localization and classification of chromosomal aberrations in FISH microscopy images" (started in october 2021, supervised by C. Kervrann and M.A. Benadjaoud (IRSN/SERAMED, Fontenay-aux-Roses)).
-
–
Lisa Balsollier (PhD in progress): "Spatiotemporal stochastic models for the comprehension of membrane trafficking at the single cell scale" (started in october 2021, supervised by F. Lavancier, C. Kervrann, and J. Salamero).
-
–
Quentin Rapilly (PhD in progress): "Hybrid CNN-Snake algorithms for quantitative analysis of 3D+time live-cell images" (started in December 2022, supervised by A. Badoual and C. Kervrann).
-
–
Salomé Papareux (Master 2), May-December 2022, supervised by A. Badoual.
-
–
Constantin Aronssohn (Master 1), April-July 2022, supervised by E. Moebel.
11.2.3 Juries
-
–
Charles Kervrann:
-
>President of the PhD thesis committee of M. Harastani (Sorbonne University, supervised by S. Jonic).
-
>Reviewer for the PhD theses of D. Blanc (University of Montpellier, supervised by J. Salmon and B. Charlier), X. Galindo Herández (University of Bordeaux, supervised by F. Levet), and F. Simon (Paris City University, supervised by S. Teeffelen).
-
>
-
–
Patrick Bouthemy:
-
>President of HdR committee of E. Caruyer (University of Rennes 1).
-
>Reviewer of the HdR of S. Ghalila-Sevestre (Paris City University).
-
>Reviewer of the PhD thesis of A. Laugros (University of Grenoble-Alpes, supervised by A. Caplier).
-
>
-
–
Frédréric Lavancier:
-
>Reviewer of the PhD thesis of A. Brochard (ENS Paris, supervised by B. Blaszczyszyn and S. Mallat).
-
>
11.3 Popularization
11.3.1 Interventions
Ludovic Leconte, Cesar Augusto Valades-Cruz, and Jean Salamero organized the "Digital Spaces for Research and Medicine" meeting at Institut Curie, July 7th, and the "Virtual Reality" workshop in the frame of the 2022 "Fête de la Science", Institut Curie, Paris, France, October 7, 2022.
12 Scientific production
12.1 Major publications
- 1 articleAdaptive spot detection with optimal scale selection in fluorescence microscopy images.IEEE Transactions on Image Processing2411November 2015, 16
- 2 articleAn extended model of vesicle fusion at the plasma membrane to estimate protein lateral diffusion from TIRF microscopy images.BMC Bioinformatics1812017, 352
- 3 articlePatch-based nonlocal functional for denoising fluorescence microscopy image sequences.IEEE Transactions on Medical Imaging292Feb 2010, 442-453
- 4 articleStatistical analysis of particle trajectories in living cells.Physical Review E 976June 2018, 1-20
- 5 articleA sequential algorithm to detect diffusion switching along intracellular particle trajectories.BioinformaticsJune 2019, 1-13
- 6 article Fast live simultaneous multiwavelength four-dimensional optical microscopy.Proc Natl Acad Sci USA10737Sep 2010, 16016-16022
- 7 articleMotion Textures: Modeling, Classification, and Segmentation Using Mixed-State.SIAM Journal on Imaging Sciences64December 2013, 2484-2520
- 8 inproceedingsPEWA: Patch-based Exponentially Weighted Aggregation for image denoising.NIPS - Neural Information Processing SystemsNeural Information Processing Systems FoundationMontreal, CanadaDecember 2014
- 9 articleDeep Learning Improves Macromolecule Identification in 3D Cellular Cryo-Electron Tomograms.Nature Methods1811October 2021, 1386-1394
- 10 articleCounting-based particle flux estimation for traffic analysis in live cell imaging.IEEE Journal of Selected Topics in Signal ProcessingSeptember 2015
- 11 articlePiecewise-stationary motion modeling and iterative smoothing to track heterogeneous particle motions in dense environments.IEEE Transactions on Image Processing2611June 2017, 5395 - 5410
12.2 Publications of the year
International journals
International peer-reviewed conferences
Conferences without proceedings
Scientific book chapters
Reports & preprints
12.3 Cited publications
- 35 articleTotal Internal Reflection Fluorescent Microscopy in cell biology.Traffic22004, 4658--4668
- 36 articleImaging intracelluar fluorescent proteins at nanometer resolution.Science3132006, 1642--1645
- 37 articleFast high-resolution 3D total internal reflection fluorescence microscopy by incidence angle scanning and azimuthal averaging.Proc Natl Acad Sci U S A111482014, 17164--17169
- 38 articleA Non-local Bayesian image denoising algorithm.SIAM J. Imaging Sciences632013, 1665--1688
- 39 articleLattice light-sheet microscopy: Imaging molecules to embryos at high spatiotemporal resolution.Science34662082014, 1257998
- 40 articleImage denoising by sparse 3-d transform-domain collaborative filtering.IEEE Trans. Image Processing1682007, 2080--2095
- 41 inproceedingsWeighted nuclear norm minimization with application to image denoising.IEEE Conference on Computer Vision and Pattern Recognition (CVPR)Columbus, OH, USA,June 2014, 2862-2869
- 42 articleMorphoNet: an interactive online morphological browser to explore complex multi-scale data.Nature Communications1028122019, 1-8
- 43 inproceedingsDense mapping of intracellular diffusion and drift from single-particle tracking data analysis.ICASSP 2020 - IEEE International Conference on Acoustics, Speech and Signal ProcessingBarcelona, SpainIEEEMay 2020, 1-5
- 44 articleCellpose: a generalist algorithm for cellular segmentation.Nature Methods182021, 100--106
- 45 articleContent-aware image restoration: pushing the limits of fluorescence microscopy.Nature Methods122018, 1090-1097