Keywords
Computer Science and Digital Science
- A5.3.3. Pattern recognition
- A5.4. Computer vision
- A5.4.2. Activity recognition
- A5.4.4. 3D and spatio-temporal reconstruction
- A5.4.5. Object tracking and motion analysis
- A9.1. Knowledge
- A9.2. Machine learning
- A9.3. Signal analysis
- A9.8. Reasoning
Other Research Topics and Application Domains
- B1.2.2. Cognitive science
- B2.1. Well being
- B7.1.1. Pedestrian traffic and crowds
- B8.1. Smart building/home
- B8.4. Security and personal assistance
1 Team members, visitors, external collaborators
Research Scientists
- Francois Bremond [Team leader, Inria, Senior Researcher, HDR]
- Antitza Dantcheva [Inria, Researcher, HDR]
- Esma Ismailova [Ecole Nationale Supérieure des Mines de Saint Etienne, Researcher, until Feb 2021]
- Alexandra Konig [Inria, Starting Research Position]
- Sabine Moisan [Inria, Researcher, HDR]
- Jean-Paul Rigault [Univ Côte d'Azur, Emeritus]
- Monique Thonnat [Inria, Senior Researcher, HDR]
- Susanne Thummler [Univ Côte d'Azur, Researcher, until Aug 2021]
Post-Doctoral Fellows
- Michal Balazia [Univ Côte d'Azur, until Mar 2021]
- Abhijit Das [Inria, until Feb 2021]
- Laura Ferrari [Univ Côte d'Azur]
- Mohsen Tabejamaat [Inria]
- Leonard Torossian [Inria, until Oct 2021]
- Ujjwal Ujjwal [Inria, until Nov 2021]
PhD Students
- Abid Ali [Univ Côte d'Azur]
- David Anghelone [Inria / Thales Group, from Apr 2021]
- Hao Chen [Inria]
- Rui Dai [Univ Côte d'Azur]
- Juan Diego Gonzales Zuniga [Inria, until Jul 2021]
- Mohammed Guermal [Inria]
- Jen Cheng Hou [Inria]
- Indu Joshi [Inria, from Aug 2021]
- Thibaud Lyvonnet [Inria]
- Tomasz Stanczyk [Inria, from Aug 2021]
- Valeriya Strizhkova [Inria]
- Yaohui Wang [Inria]
- Di Yang [Inria]
Technical Staff
- Tanay Agrawal [Inria, Engineer]
- Sebastien Gilabert [Inria, Engineer]
- Snehashis Majhi [Inria, Engineer, from Sep 2021]
- Farhood Negin [Inria, Engineer]
- Duc Minh Tran [Inria, Engineer]
Interns and Apprentices
- Dhruv Agarwal [Inria, until Jul 2021]
- Elias Bou Ghosn [Inria, from Apr 2021 until Sep 2021]
- Benjamin Brou [Inria, from Apr 2021 until Jun 2021]
- Mansi Mittal [Inria, from Aug 2021]
- Vishal Pani [Inria, until Jul 2021]
- Carlotta Sanges [Inria, until Mar 2021]
- Neelabh Sinha [Inria, from Feb 2021 until Jul 2021]
- Rian Touchent [Inria, from May 2021 until Aug 2021]
- Deepak Yadav [Inria, from Sep 2021]
Administrative Assistant
- Sandrine Boute [Inria]
External Collaborators
- Michal Balazia [Univ Côte d'Azur, from Apr 2021]
- Rachid Guerchouche [Univ Côte d'Azur]
2 Overall objectives
2.1 Presentation
The STARS (Spatio-Temporal Activity Recognition Systems) team focuses on the design of cognitive vision systems for Activity Recognition. More precisely, we are interested in the real-time semantic interpretation of dynamic scenes observed by video cameras and other sensors. We study long-term spatio-temporal activities performed by agents such as human beings, animals or vehicles in the physical world. The major issue in semantic interpretation of dynamic scenes is to bridge the gap between the subjective interpretation of data and the objective measures provided by sensors. To address this problem Stars develops new techniques in the field of computer vision, machine learning and cognitive systems for physical object detection, activity understanding, activity learning, vision system design and evaluation. We focus on two principal application domains: visual surveillance and healthcare monitoring.
2.2 Research Themes
Stars is focused on the design of cognitive systems for Activity Recognition. We aim at endowing cognitive systems with perceptual capabilities to reason about an observed environment, to provide a variety of services to people living in this environment while preserving their privacy. In today world, a huge amount of new sensors and new hardware devices are currently available, addressing potentially new needs of the modern society. However the lack of automated processes (with no human interaction) able to extract a meaningful and accurate information (i.e. a correct understanding of the situation) has often generated frustrations among the society and especially among older people. Therefore, Stars objective is to propose novel autonomous systems for the real-time semantic interpretation of dynamic scenes observed by sensors. We study long-term spatio-temporal activities performed by several interacting agents such as human beings, animals and vehicles in the physical world. Such systems also raise fundamental software engineering problems to specify them as well as to adapt them at run time.
We propose new techniques at the frontier between computer vision, knowledge engineering, machine learning and software engineering. The major challenge in semantic interpretation of dynamic scenes is to bridge the gap between the task dependent interpretation of data and the flood of measures provided by sensors. The problems we address range from physical object detection, activity understanding, activity learning to vision system design and evaluation. The two principal classes of human activities we focus on, are assistance to older adults and video analytic.
Typical examples of complex activity are shown in Figure 1 and Figure 2 for a homecare application (See Toyota Smarthome Dataset at ). In this example, the duration of the monitoring of an older person apartment could last several months. The activities involve interactions between the observed person and several pieces of equipment. The application goal is to recognize the everyday activities at home through formal activity models (as shown in Figure 3) and data captured by a network of sensors embedded in the apartment. Here typical services include an objective assessment of the frailty level of the observed person to be able to provide a more personalized care and to monitor the effectiveness of a prescribed therapy. The assessment of the frailty level is performed by an Activity Recognition System which transmits a textual report (containing only meta-data) to the general practitioner who follows the older person. Thanks to the recognized activities, the quality of life of the observed people can thus be improved and their personal information can be preserved.
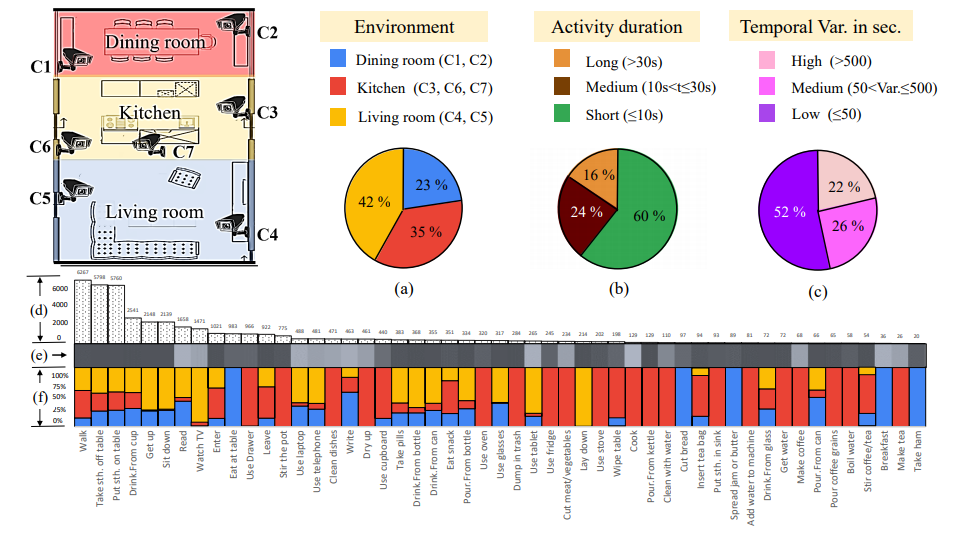
.png)
Activity (PrepareMeal, | |
PhysicalObjects( | (p : Person), (z : Zone), (eq : Equipment)) |
Components( | (s_inside : InsideKitchen(p, z)) |
(s_close : CloseToCountertop(p, eq)) | |
(s_stand : PersonStandingInKitchen(p, z))) | |
Constraints( | (z->Name = Kitchen) |
(eq->Name = Countertop) | |
(s_close->Duration >= 100) | |
(s_stand->Duration >= 100)) | |
Annotation( | AText("prepare meal"))) |
The ultimate goal is for cognitive systems to perceive and understand their environment to be able to provide appropriate services to a potential user. An important step is to propose a computational representation of people activities to adapt these services to them. Up to now, the most effective sensors have been video cameras due to the rich information they can provide on the observed environment. These sensors are currently perceived as intrusive ones. A key issue is to capture the pertinent raw data for adapting the services to the people while preserving their privacy. We plan to study different solutions including of course the local processing of the data without transmission of images and the utilization of new compact sensors developed for interaction (also called RGB-Depth sensors, an example being the Kinect) or networks of small non visual sensors.
2.3 International and Industrial Cooperation
Our work has been applied in the context of more than 10 European projects such as COFRIEND, ADVISOR, SERKET, CARETAKER, VANAHEIM, SUPPORT, DEM@CARE, VICOMO, EIT Health.
We had or have industrial collaborations in several domains: transportation (CCI Airport Toulouse Blagnac, SNCF, Inrets, Alstom, Ratp, Toyota, GTT (Italy), Turin GTT (Italy)), banking (Crédit Agricole Bank Corporation, Eurotelis and Ciel), security (Thales R&T FR, Thales Security Syst, EADS, Sagem, Bertin, Alcatel, Keeneo), multimedia (Thales Communications), civil engineering (Centre Scientifique et Technique du Bâtiment (CSTB)), computer industry (BULL), software industry (AKKA), hardware industry (ST-Microelectronics) and health industry (Philips, Link Care Services, Vistek).
We have international cooperations with research centers such as Reading University (UK), ENSI Tunis (Tunisia), Idiap (Switzerland), Multitel (Belgium), National Cheng Kung University, National Taiwan University (Taiwan), MICA (Vietnam), IPAL, I2R (Singapore), University of Southern California, University of South Florida (USA), Michigan State University (USA), Chinese Academy of Sciences (China), IIIT Delhi (India), Hochschule Darmstadt (Germany), Fraunhofer Institute for Computer Graphics Research IGD (Germany).
2.3.1 Industrial Contracts
-
Toyota: (Action Recognition System):
This project run from the 1st of August 2013 up to 2023. It aimed at detecting critical situations in the daily life of older adults living home alone. The system is intended to work with a Partner Robot (to send real-time information to the robot for assisted living) to better interact with older adults. The funding was 106 Keuros for the 1st period and more for the following years.
- Thales: This contract is a CIFRE PhD grant and runs from September 2018 until September 2021 within the French national initiative SafeCity. The main goal is to analyze faces and events in the invisible spectrum (i.e., low energy infrared waves, as well as ultraviolet waves). In this context models will be developed to efficiently extract identity, as well as event - information. This models will be employed in a school environment, with a goal of pseudo-anonymized identification, as well as event-detection. Expected challenges have to do with limited colorimetry and lower contrasts.
- Kontron: This contract is a CIFRE PhD grant and runs from April 2018 until April 2021 to embed CNN based people tracker within a video-camera.
- ESI: This contract is a CIFRE PhD grant and runs from September 2018 until March 2022 to develop a novel Re-Identification algorithm which can be easily set-up with low interaction.
3 Research program
3.1 Introduction
Stars follows three main research directions: perception for activity recognition, action recognition and semantic activity recognition. These three research directions are organized following the workflow of activity recognition systems: First, the perception and the action recognition directions provide new techniques to extract powerful features, whereas the semantic activity recognition research direction provides new paradigms to match these features with concrete video analytic and healthcare applications.
Transversely, we consider a new research axis in machine learning, combining a priori knowledge and learning techniques, to set up the various models of an activity recognition system. A major objective is to automate model building or model enrichment at the perception level and at the understanding level.
3.2 Perception for Activity Recognition
Participants: François Brémond, Antitza Dantcheva, Sabine Moisan, Monique Thonnat.
Keywords: Activity Recognition, Scene Understanding, Machine Learning, Computer Vision, Cognitive Vision Systems, Software Engineering.
3.2.1 Introduction
Our main goal in perception is to develop vision algorithms able to address the large variety of conditions characterizing real world scenes in terms of sensor conditions, hardware requirements, lighting conditions, physical objects, and application objectives. We have also several issues related to perception which combine machine learning and perception techniques: learning people appearance, parameters for system control and shape statistics.
3.2.2 Appearance Models and People Tracking
An important issue is to detect in real-time physical objects from perceptual features and predefined 3D models. It requires finding a good balance between efficient methods and precise spatio-temporal models. Many improvements and analysis need to be performed in order to tackle the large range of people detection scenarios.
Appearance models. In particular, we study the temporal variation of the features characterizing the appearance of a human. This task could be achieved by clustering potential candidates depending on their position and their reliability. This task can provide any people tracking algorithms with reliable features allowing for instance to (1) better track people or their body parts during occlusion, or to (2) model people appearance for re-identification purposes in mono and multi-camera networks, which is still an open issue. The underlying challenge of the person re-identification problem arises from significant differences in illumination, pose and camera parameters. The re-identification approaches have two aspects: (1) establishing correspondences between body parts and (2) generating signatures that are invariant to different color responses. As we have already several descriptors which are color invariant, we now focus more on aligning two people detection and on finding their corresponding body parts. Having detected body parts, the approach can handle pose variations. Further, different body parts might have different influence on finding the correct match among a whole gallery dataset. Thus, the re-identification approaches have to search for matching strategies. As the results of the re-identification are always given as the ranking list, re-identification focuses on learning to rank. "Learning to rank" is a type of machine learning problem, in which the goal is to automatically construct a ranking model from a training data.
Therefore, we work on information fusion to handle perceptual features coming from various sensors (several cameras covering a large scale area or heterogeneous sensors capturing more or less precise and rich information). New 3D RGB-D sensors are also investigated, to help in getting an accurate segmentation for specific scene conditions.
Long term tracking. For activity recognition we need robust and coherent object tracking over long periods of time (often several hours in video surveillance and several days in healthcare). To guarantee the long term coherence of tracked objects, spatio-temporal reasoning is required. Modeling and managing the uncertainty of these processes is also an open issue. In Stars we propose to add a reasoning layer to a classical Bayesian framework modeling the uncertainty of the tracked objects. This reasoning layer can take into account the a priori knowledge of the scene for outlier elimination and long-term coherency checking.
Controlling system parameters. Another research direction is to manage a library of video processing programs. We are building a perception library by selecting robust algorithms for feature extraction, by insuring they work efficiently with real time constraints and by formalizing their conditions of use within a program supervision model. In the case of video cameras, at least two problems are still open: robust image segmentation and meaningful feature extraction. For these issues, we are developing new learning techniques.
3.3 Action Recognition
Participants: François Brémond, Antitza Dantcheva, Monique Thonnat.
Keywords: Machine Learning, Computer Vision, Cognitive Vision Systems.
3.3.1 Introduction
Due to the recent development of high processing units, such as GPU, this is now possible to extract meaningful features directly from videos (e.g. video volume) to recognize reliably short actions. Action Recognition benefits also greatly from the huge progress made recently in Machine Learning (e.g. Deep Learning), especially for the study of human behavior. For instance, Action Recognition enables to measure objectively the behavior of humans by extracting powerful features characterizing their everyday activities, their emotion, eating habits and lifestyle, by learning models from a large number of data from a variety of sensors, to improve and optimize for example, the quality of life of people suffering from behavior disorders. However, Smart Homes and Partner Robots have been well advertised but remain laboratory prototypes, due to the poor capability of automated systems to perceive and reason about their environment. A hard problem is for an automated system to cope 24/7 with the variety and complexity of the real world. Another challenge is to extract people fine gestures and subtle facial expressions to better analyze behavior disorders, such as anxiety or apathy. Taking advantage of what is currently studied for self-driving cars or smart retails, there is a large avenue to design ambitious approaches for the healthcare domain. In particular, the advance made with Deep Learning algorithms has already enabled to recognize complex activities, such as cooking interactions with instruments, and from this analysis to differentiate healthy people from the ones suffering from dementia.
To address these issues, we propose to tackle several challenges:
3.3.2 Action recognition in the wild
The current Deep Learning techniques are mostly developed to work on few clipped videos, which have been recorded with students performing a limited set of predefined actions in front of a camera with high resolution. However, real life scenarios include actions performed in a spontaneous manner by older people (including people interactions with their environment or with other people), from different viewpoints, with varying framerate, partially occluded by furniture at different locations within an apartment depicted through long untrimmed videos. Therefore, a new dedicated dataset should be collected in a real-world setting to become a public benchmark video dataset and to design novel algorithms for ADL activity recognition. A special attention should be taken to anonymize the videos.
3.3.3 Attention mechanisms for action recognition
Activities of Daily Living (ADL) and video-surveillance activities are different from internet activities (e.g. Sports, Movies, YouTube), as they may have very similar context (e.g. same background kitchen) with high intra-variation (different people performing the same action in different manners), but in the same time low inter-variation, similar ways to perform two different actions (e.g. eating and drinking a glass of water). Consequently, fine-grained actions are badly recognized. So, we will design novel attention mechanisms for action recognition, for the algorithm being able to focus on a discriminative part of the person conducting the action. For instance, we will study attention algorithms, which could focus on the most appropriate body parts (e.g. full body, right hand). In particular, we plan to design a soft mechanism, learning the attention weights directly on the feature map of a 3DconvNet, a powerful convolutional network, which takes as input a batch of videos.
3.3.4 Action detection for untrimmed videos
Many approaches have been proposed to solve the problem of action recognition in short clipped 2D videos, which achieved impressive results with hand-crafted and deep features. However, these approaches cannot address real life situations, where cameras provide online and continuous video streams in applications such as robotics, video surveillance, and smart-homes. Here comes the importance of action detection to help recognizing and localizing each action happening in long videos. Action detection can be defined as the ability to localize starting and ending of each human action happening in the video, in addition to recognizing each action label. There have been few action detection algorithms designed for untrimmed videos, which are based on either sliding window, temporal pooling or frame-based labeling. However, their performance is too low to address real-word datasets. A first task consists in benchmarking the already published approaches to study their limitations on novel untrimmed video datasets, recorded following real-world settings. A second task could be to propose a new mechanism to improve either 1) the temporal pooling directly from the 3DconvNet architecture using for instance Temporal Convolution Networks (TCNs) or 2) frame-based labeling with a clustering technique (e.g. using Fisher Vectors) to discover the sub-activities of interest.
3.3.5 View invariant action recognition
The performance of current approaches strongly relies on the used camera angle: enforcing that the camera angle used in testing is the same (or extremely close to) as the camera angle used in training, is necessary for the approach performs well. On the contrary, the performance drops when a different camera view-point is used. Therefore, we aim at improving the performance of action recognition algorithms by relying on 3D human pose information. For the extraction of the 3D pose information, several open-source algorithms can be used, such as openpose or videopose3D (from CMU or Facebook research, . Also, other algorithms extracting 3d meshes can be used. To generate extra views, Generative Adversial Network (GAN) can be used together with the 3D human pose information to complete the training dataset from the missing view.
3.3.6 Uncertainty and action recognition
Another challenge is to combine the short-term actions recognized by powerful Deep Learning techniques with long-term activities defined by constraint-based descriptions and linked to user interest. To realize this objective, we have to compute the uncertainty (i.e. likelihood or confidence), with which the short-term actions are inferred. This research direction is linked to the next one, to Semantic Activity Recognition.
3.4 Semantic Activity Recognition
Participants: François Brémond, Sabine Moisan, Monique Thonnat.
Keywords: Activity Recognition, Scene Understanding, Computer Vision.
3.4.1 Introduction
Semantic activity recognition is a complex process where information is abstracted through four levels: signal (e.g. pixel, sound), perceptual features, physical objects and activities. The signal and the feature levels are characterized by strong noise, ambiguous, corrupted and missing data. The whole process of scene understanding consists in analyzing this information to bring forth pertinent insight of the scene and its dynamics while handling the low level noise. Moreover, to obtain a semantic abstraction, building activity models is a crucial point. A still open issue consists in determining whether these models should be given a priori or learned. Another challenge consists in organizing this knowledge in order to capitalize experience, share it with others and update it along with experimentation. To face this challenge, tools in knowledge engineering such as machine learning or ontology are needed.
Thus we work along the following research axes: high level understanding (to recognize the activities of physical objects based on high level activity models), learning (how to learn the models needed for activity recognition) and activity recognition and discrete event systems.
3.4.2 High Level Understanding
A challenging research axis is to recognize subjective activities of physical objects (i.e. human beings, animals, vehicles) based on a priori models and objective perceptual measures (e.g. robust and coherent object tracks).
To reach this goal, we have defined original activity recognition algorithms and activity models. Activity recognition algorithms include the computation of spatio-temporal relationships between physical objects. All the possible relationships may correspond to activities of interest and all have to be explored in an efficient way. The variety of these activities, generally called video events, is huge and depends on their spatial and temporal granularity, on the number of physical objects involved in the events, and on the event complexity (number of components constituting the event).
Concerning the modeling of activities, we are working towards two directions: the uncertainty management for representing probability distributions and knowledge acquisition facilities based on ontological engineering techniques. For the first direction, we are investigating classical statistical techniques and logical approaches. For the second direction, we built a language for video event modeling and a visual concept ontology (including color, texture and spatial concepts) to be extended with temporal concepts (motion, trajectories, events ...) and other perceptual concepts (physiological sensor concepts ...).
3.4.3 Learning for Activity Recognition
Given the difficulty of building an activity recognition system with a priori knowledge for a new application, we study how machine learning techniques can automate building or completing models at the perception level and at the understanding level.
At the understanding level, we are learning primitive event detectors. This can be done for example by learning visual concept detectors using SVMs (Support Vector Machines) with perceptual feature samples. An open question is how far can we go in weakly supervised learning for each type of perceptual concept (i.e. leveraging the human annotation task). A second direction is to learn typical composite event models for frequent activities using trajectory clustering or data mining techniques. We name composite event a particular combination of several primitive events.
3.4.4 Activity Recognition and Discrete Event Systems
The previous research axes are unavoidable to cope with the semantic interpretations. However they tend to let aside the pure event driven aspects of scenario recognition. These aspects have been studied for a long time at a theoretical level and led to methods and tools that may bring extra value to activity recognition, the most important being the possibility of formal analysis, verification and validation.
We have thus started to specify a formal model to define, analyze, simulate, and prove scenarios. This model deals with both absolute time (to be realistic and efficient in the analysis phase) and logical time (to benefit from well-known mathematical models providing re-usability, easy extension, and verification). Our purpose is to offer a generic tool to express and recognize activities associated with a concrete language to specify activities in the form of a set of scenarios with temporal constraints. The theoretical foundations and the tools being shared with Software Engineering aspects.
The results of the research performed in perception and semantic activity recognition (first and second research directions) produce new techniques for scene understanding and contribute to specify the needs for new software architectures (third research direction).
4 Application domains
4.1 Introduction
While in our research the focus is to develop techniques, models and platforms that are generic and reusable, we also make effort in the development of real applications. The motivation is twofold. The first is to validate the new ideas and approaches we introduce. The second is to demonstrate how to build working systems for real applications of various domains based on the techniques and tools developed. Indeed, Stars focuses on two main domains: video analytic and healthcare monitoring.
Domain: Video Analytics
Our experience in video analytic (also referred to as visual surveillance) is a strong basis which ensures both a precise view of the research topics to develop and a network of industrial partners ranging from end-users, integrators and software editors to provide data, objectives, evaluation and funding.
For instance, the Keeneo start-up was created in July 2005 for the industrialization and exploitation of Orion and Pulsar results in video analytic (VSIP library, which was a previous version of SUP). Keeneo has been bought by Digital Barriers in August 2011 and is now independent from Inria. However, Stars continues to maintain a close cooperation with Keeneo for impact analysis of SUP and for exploitation of new results.
Moreover new challenges are arising from the visual surveillance community. For instance, people detection and tracking in a crowded environment are still open issues despite the high competition on these topics. Also detecting abnormal activities may require to discover rare events from very large video data bases often characterized by noise or incomplete data.
Domain: Healthcare Monitoring
Since 2011, we have initiated a strategic partnership (called CobTek) with Nice hospital (CHU Nice, Prof P. Robert) to start ambitious research activities dedicated to healthcare monitoring and to assistive technologies. These new studies address the analysis of more complex spatio-temporal activities (e.g. complex interactions, long term activities).
4.1.1 Research
To achieve this objective, several topics need to be tackled. These topics can be summarized within two points: finer activity description and longitudinal experimentation. Finer activity description is needed for instance, to discriminate the activities (e.g. sitting, walking, eating) of Alzheimer patients from the ones of healthy older people. It is essential to be able to pre-diagnose dementia and to provide a better and more specialized care. Longer analysis is required when people monitoring aims at measuring the evolution of patient behavioral disorders. Setting up such long experimentation with dementia people has never been tried before but is necessary to have real-world validation. This is one of the challenge of the European FP7 project Dem@Care where several patient homes should be monitored over several months.
For this domain, a goal for Stars is to allow people with dementia to continue living in a self-sufficient manner in their own homes or residential centers, away from a hospital, as well as to allow clinicians and caregivers remotely provide effective care and management. For all this to become possible, comprehensive monitoring of the daily life of the person with dementia is deemed necessary, since caregivers and clinicians will need a comprehensive view of the person's daily activities, behavioral patterns, lifestyle, as well as changes in them, indicating the progression of their condition.
4.1.2 Ethical and Acceptability Issues
The development and ultimate use of novel assistive technologies by a vulnerable user group such as individuals with dementia, and the assessment methodologies planned by Stars are not free of ethical, or even legal concerns, even if many studies have shown how these Information and Communication Technologies (ICT) can be useful and well accepted by older people with or without impairments. Thus one goal of Stars team is to design the right technologies that can provide the appropriate information to the medical carers while preserving people privacy. Moreover, Stars will pay particular attention to ethical, acceptability, legal and privacy concerns that may arise, addressing them in a professional way following the corresponding established EU and national laws and regulations, especially when outside France. Now, Stars can benefit from the support of the COERLE (Comité Opérationnel d'Evaluation des Risques Légaux et Ethiques) to help it to respect ethical policies in its applications.
As presented in 2, Stars aims at designing cognitive vision systems with perceptual capabilities to monitor efficiently people activities. As a matter of fact, vision sensors can be seen as intrusive ones, even if no images are acquired or transmitted (only meta-data describing activities need to be collected). Therefore new communication paradigms and other sensors (e.g. accelerometers, RFID, and new sensors to come in the future) are also envisaged to provide the most appropriate services to the observed people, while preserving their privacy. To better understand ethical issues, Stars members are already involved in several ethical organizations. For instance, F. Brémond has been a member of the ODEGAM - “Commission Ethique et Droit” (a local association in Nice area for ethical issues related to older people) from 2010 to 2011 and a member of the French scientific council for the national seminar on “La maladie d'Alzheimer et les nouvelles technologies - Enjeux éthiques et questions de société” in 2011. This council has in particular proposed a chart and guidelines for conducting researches with dementia patients.
For addressing the acceptability issues, focus groups and HMI (Human Machine Interaction) experts, are consulted on the most adequate range of mechanisms to interact and display information to older people.
5 Social and environmental responsibility
5.1 Footprint of research activities
We have limited our travels by reducing our physical participation to conferences and to international collaborations.
5.2 Impact of research results
We have been involved for many years in promoting public transportation by improving safety onboard and in station. Moreover, we have been working on pedestrian detection for self-driving cars, which will help also reducing the number of individual cars.
6 Highlights of the year
During this period, several novel activity recognition algorithms have been designed for Activities of Daily Living (ADLs) in real-world settings. These algorithms got the best performances on all relevant action datasets. However, most of them were built in more or less artificial settings. Therefore, we have released a new video dataset in real-world settings, which is going to become one of the main benchmarks of the domain: Real-World Activities of Daily Living. We have validated our activity detection algorithms on this new video dataset to foster novel research directions.
7 New software and platforms
Most of team contributions come with a published paper and an associated software, which is publicly available through github.
7.1 New software
7.1.1 SUP
-
Name:
Scene Understanding Platform
-
Keywords:
Activity recognition, 3D, Dynamic scene
-
Functional Description:
SUP is a software platform for perceiving, analyzing and interpreting a 3D dynamic scene observed through a network of sensors. It encompasses algorithms allowing for the modeling of interesting activities for users to enable their recognition in real-world applications requiring high-throughput.
- URL:
-
Contact:
François Brémond
-
Participants:
Etienne Corvée, François Brémond, Hung Nguyen, Vasanth Bathrinarayanan
-
Partners:
CEA, CHU Nice, USC Californie, Université de Hamburg, I2R
7.1.2 VISEVAL
-
Functional Description:
ViSEval is a software dedicated to the evaluation and visualization of video processing algorithm outputs. The evaluation of video processing algorithm results is an important step in video analysis research. In video processing, we identify 4 different tasks to evaluate: detection, classification and tracking of physical objects of interest and event recognition.
- URL:
-
Contact:
François Brémond
-
Participants:
Bernard Boulay, François Brémond
8 New results
8.1 Introduction
This year Stars has proposed new results related to its three main research axes: (i) perception for activity recognition, (ii) action recognition and (iii) semantic activity recognition.
Perception for Activity Recognition
Participants: François Brémond, Antitza Dantcheva, Juan Diego Gonzales zuniga, Farhood Negin, Vishal Pani, Indu Joshi, David Anghelone, Laura M. Ferrari, Neelabh Sinha, Neelabh Sinha, Hao Chen, Yaohui Wang, Valeriya Strizhkova, Mohsen Tabejamaat, Abhijit Das.
The new results for perception for activity recognition are:
- Detection of Tiny Vehicles from Satellite Video (see 8.2)
- TrichTrack: Multi-Object Tracking of Small-Scale Trichogramma Wasps (see 8.3)
- On Generalizable and Interpretable Biometric (see 8.4)
- Sensor-invariant Fingerprint ROI Segmentation Using Recurrent Adversarial Learning (see 8.5)
- Biosignals analysis for multimodal learning (see REFERENCE NOT FOUND: STARS-RA-2021/label/resultats:laurra_1)
- Learning-based approach for wearables design (see REFERENCE NOT FOUND: STARS-RA-2021/label/resultats:laurra_2)
- Computer Vision and Deep Learning applied to Facial Analysis in the invisible spectra (see 8.8)
- Explainable Thermal to Visible Face Recognition using Latent-Guided Generative Adversarial Network (see 8.9)
- Facial Landmark Heatmap Activated Multimodal Gaze Estimation (see 8.10)
- ICE: Inter-instance Contrastive Encoding for Unsupervised Person Re-identification (see 8.11)
- Joint Generative and Contrastive Learning for Unsupervised Person Re-identification (see 8.12)
- Emotion Editing in Head Reenactment Videos using Latent Space Manipulation (see 8.13)
- Learning to Generate Human Video (see 8.14)
- Guided Flow Field Estimation by Generating Independent Patches (see 8.15)
- BVPNet: Video-to-BVP Signal Prediction for Remote Heart Rate Estimation (see 8.16)
- Demystifying Attention Mechanisms for Deepfake Detection (see 8.17)
- Computer Vision for deciphering and generating faces (see 8.18)
Action Recognition
Participants: François Brémond, Antitza Dantcheva, Monique Thonnat, Mohammed Guermal, Tanay Agrawal, Abid Ali, Jen-Cheng Hou, Di Yang, Rui Dai, Snehashis Majhi.
The new results for action recognition are:
- DAM : Dissimilarity Attention Module for Weakly-supervised Video Anomaly Detection (see 8.19)
- Pyramid Dilated Attention Network (see 8.20)
- Class-Temporal Relational Network (see 8.21)
- Learning an Augmented RGB Representation with Cross-Modal Knowledge Distillation for Action Detection (see 8.22)
- VPN++: Rethinking Video-Pose embeddings for understanding Activities of Daily Living (see 8.23)
- Multimodal Personality Recognition using Cross-Attention Transformer and Behaviour Encoding (see 8.24)
- From Multimodal to Unimodal Attention in Transformers using Knowledge Distillation (see 8.25)
- Quantified Analysis for Video Recordings of Seizure (see 8.26)
- A Self-supervised pre-training framework for Vision-based Seizure Classification (see 8.27)
- Video-based Behavior Understanding of Children for Objective Diagnosis of Autism (see 8.28)
- Human activity recognition for interaction scerarios (see 8.29)
- Self-Supervised Video Pose Representation Learning for Occlusion-Robust Action Recognition (see 8.30)
Semantic Activity Recognition
Participants: Sabine Moisan, François Brémond, Monique Thonnat, Jean-Paul Rigault, Alexandra Konig, Rachid Guerchouche, Thibaud L'Yvonnet.
For this research axis, the contributions are:
8.2 Detection of Tiny Vehicles from Satellite Video
Participants: Farhood Negin, François Brémond.
In this work, our goal is to achieve a perceptual understanding of the images/videos captured by satellites or other aerial imaging techniques. This will allow us to evaluate the behavior of various entities in those images and to plan appropriate responses and prevent undesirable actions such as abnormal behaviors 58, 59. To develop such systems, the primary step is the detection of objects of interest in the acquired imagery. Therefore, our first task is to detect objects in satellite images.
8.2.1 Work Description
Objects in the satellite datasets have a very small size (5 to 20 pixels) and the conventional methods have a hard time detecting those objects 70. There is a huge discrepancy between how a model works on small objects compared to large objects. In deep architectures, as the model learns, it forms features from the images passing through it. These features are based on pixels, and pixels in small objects are not so many for the model to learn strong features. Spatiotemporal information is already utilized in other contexts such as temporal segmentation but it is not fairly investigated in object detection in satellite images and there are only a few works using this information 65. In this work, the first goal is to develop a spatiotemporal-based framework to achieve a reliable detection and tracking of tiny objects from the satellite image sequences.
8.2.2 Datasets and Methods
Three datasets will be used for our evaluations (Figure 4): AirBus provided data, CGSTL dataset (Chinese satellite), and WPAFB 2009 (U.S. Air force). CGST dataset is a wide area imagery dataset that covers 3-4 square kilometers where 3 selected areas (
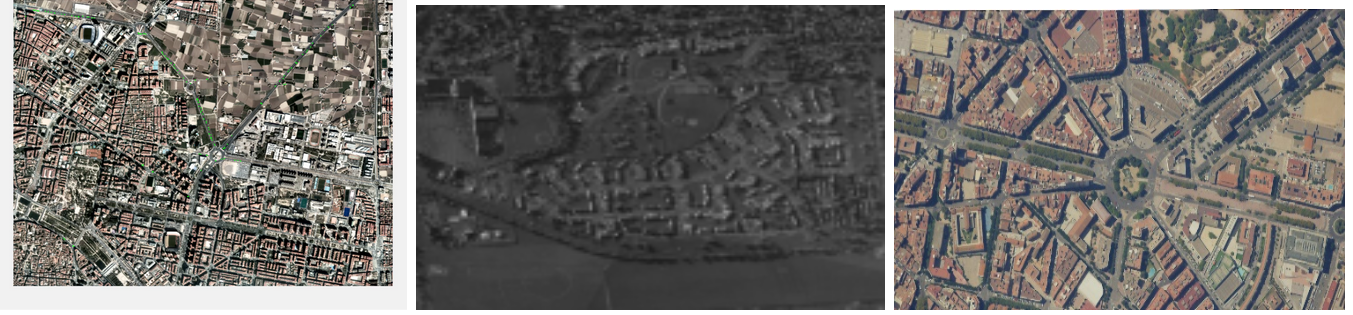
To achieve a baseline the subsequent steps are followed: Image registration (stabilization), background subtraction, filtering. It is necessary to compensate for the camera motion by aligning all the previous frames to the current frame. Therefore, the transformation matrix: denoting transformation from frame t-k to frame t is calculated. For that SIFT feature point detector for interest point detection and SURF for feature extraction are utilized. Then, features matched between the two frames and transformation which is calculated by RANSAC. The stabilized frames then are subsampled at every 5 frames. The foreground objects are detected by frame differentiation and then morphological operations are applied to remove irregular blobs and too small/big detections (results are shown in Figure 5).
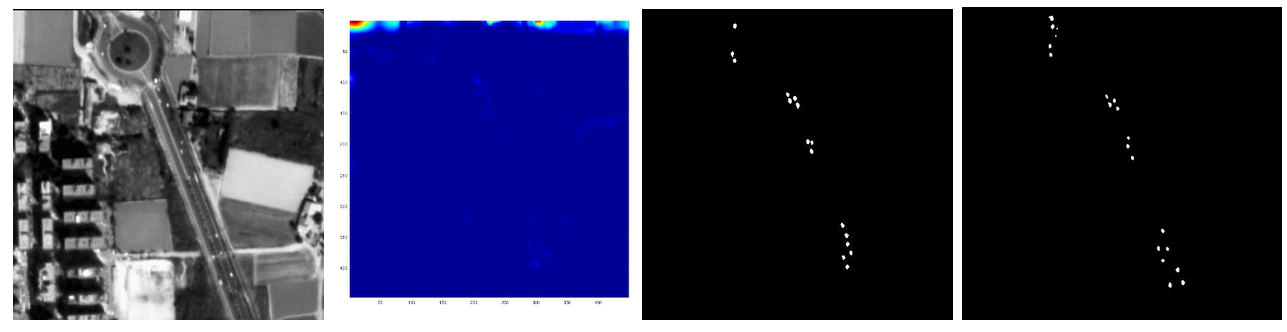
In this work, to produce a baseline, we applied image processing techniques for detection and tracking problems. In this step, the challenges are realized and will be addressed by designing new solutions and leveraging the available methods. One approach to solving the object detection challenge is to have an iterative process for training a deep model. In this approach, a background subtraction algorithm with a higher threshold is used to generate object proposals. Then, a classifier is trained based on the proposals to decide which proposal is a genuine object or merely a noise.
8.3 TrichTrack: Multi-Object Tracking of Small-Scale Trichogramma Wasps
Participants: Vishal Pani, Martin Bernet, Vincent Calcagno, Louise Van Oudenhove, François Brémond.
Trichogramma wasps behaviors are studied extensively due to their effectiveness as biological control agents across the globe. However, to our knowledge, the field of intra/inter-species Trichogramma behavior is yet to be explored thoroughly. To study these behaviors it is crucial to identify and track Trichogramma individuals over a long period in a lab setup. For this, we propose a robust tracking pipeline named TrichTrack 51. Due to the unavailability of labeled data, we train our detector using an iterative weakly supervised method. We also use a weakly supervised method to train a Re-Identification (ReID) network by leveraging noisy tracklet sampling. This enables us to distinguish Trichogramma individuals that are indistinguishable from human eyes. We also develop a two-staged tracking module that filters out the easy association to improve its efficiency. Our method outperforms existing insect trackers on most of the MOTMetrics, specifically on ID switches and fragmentations.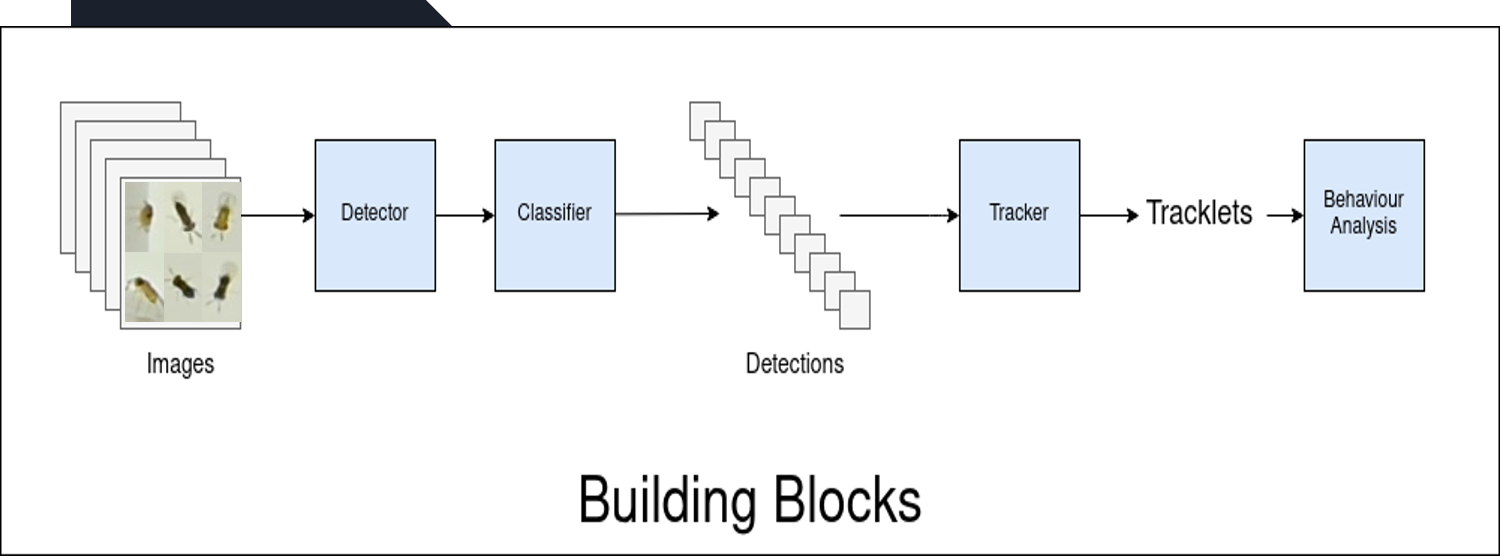
8.4 On Generalizable and Interpretable Biometrics
Participants: Indu Joshi, Antitza Dantcheva.
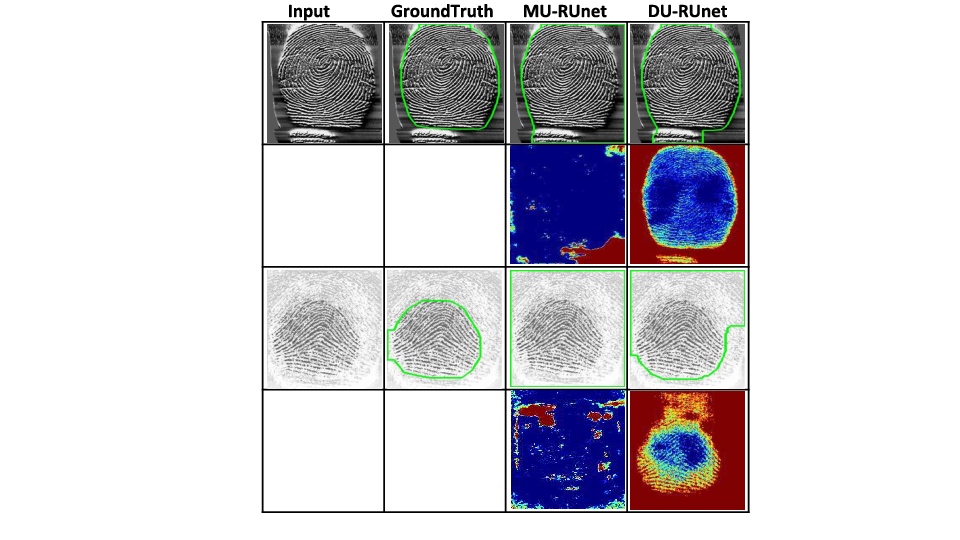
Black box behaviour and poor generalization are major limitation of state-of-the-art biometrics recognition systems. We work towards addressing these limitations in our recent work. We exploit attention mechanisms and adversarial learning to improve generalization ability and uncertainty estimation to impart interpretability to biometrics recognition systems. We describe these contributions in the following sections.
Data Uncertainty Guided Noise Aware Preprocessing We exploit Bayesian framework to estimate data uncertainty in a fingerprint preprocessing model 41. Given the input fingerprint image, data uncertainty estimation requires placing a prior distribution over the output of the model and calculating the variance of noise in model output. Predicted data uncertainty being input dependent, is learned as a function of input image. To obtain both the preprocessed image and its associated uncertainty, the network architecture of the baseline fingerprint preprocessing model is modified. The last layer of the baseline architecture is modified by splitting it into two. One branch predicts the model output (preprocessed image), whereas the other branch predicts the data uncertainty (noise variance). The mapping between input and the preprocessed image is learnt in a supervised manner. However, no labels for uncertainty are used, and the uncertainty values are learnt in an unsupervised manner. Furthermore, The loss function of the baseline architecture is also modified as suggested in 64. Results reveal that predicting data uncertainty helps the model to identify noisy regions in fingerprint images (see Figure 7), due to which higher activations are obtained around foreground fingerprint pixels. As a result, improved segmentation performance on noisy background pixels is obtained. Similar observations are reported for fingerprint enhancement.
8.5 Sensor-invariant Fingerprint ROI Segmentation Using Recurrent Adversarial Learning
Participants: Indu Joshi, Antitza Dantcheva.
A fingerprint region of interest (ROI) segmentation algorithm is designed 42 to separate the foreground fingerprint from the background noise. All the learning based state-of-the-art fingerprint ROI segmentation algorithms proposed in the literature are benchmarked on scenarios when both training and testing databases consist of fingerprint images acquired from the same sensors. However, when testing is conducted on a different sensor, the segmentation performance obtained is often unsatisfactory. As a result, every time a new fingerprint sensor is used for testing, the fingerprint ROI segmentation model needs to be re-trained with the fingerprint image acquired from the new sensor and its corresponding manually marked ROI. Manually marking fingerprint ROI is expensive because firstly, it is time consuming and more importantly, requires domain expertise. In order to save the human effort in generating annotations required by state-of-the-art, we propose a fingerprint ROI segmentation model which aligns the features of fingerprint images derived from the unseen sensor such that they are similar to the ones obtained from the fingerprints whose ground truth ROI masks are available for training. Specifically, we propose a recurrent adversarial learning based feature alignment network that helps the fingerprint ROI segmentation model to learn sensor-invariant features. Consequently, sensor-invariant features learnt by the proposed ROI segmentation model help it to achieve improved segmentation performance on fingerprints acquired from the new sensor. Experiments on publicly available FVC databases demonstrate the efficacy of the proposed work.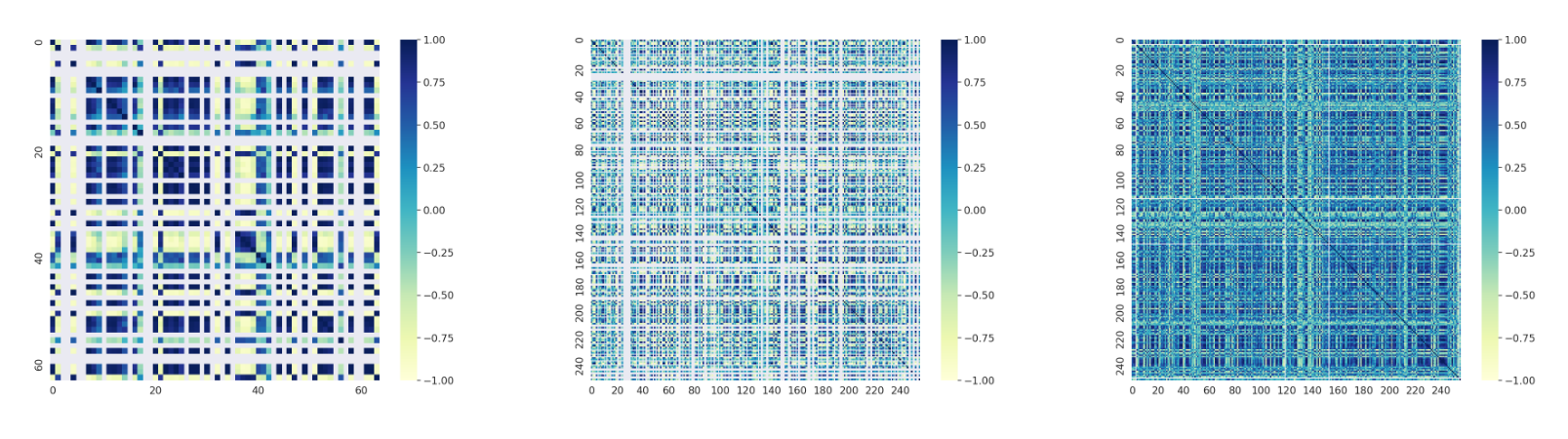
8.6 Biosignals analysis for multimodal learning
Participants: Laura M. Ferrari, François Brémond.
Multimodal machine learning aims at developing models that can process information from multiple input. Recently many fields have started exploiting multimodality as emotions or personality recognition. The idea is to combine salient information from different modalities such as RGB/3D cameras, thermal sensor, audio and biosensors. Despite the proven increased accuracy over singular modality the limits of multimodal analysis are multiple. Regarding the processing of biosignals, little has been reported for the treatment of multiple biosignals, coming from the skin surface. Indeed, while lot of works deal with electroencephalography (EEG) analysis for clinical applications, other source of information as electrodermal activity (EDA) and electrocardiography (ECG) have not been fully exploited nor a multimodal analysis proposed. Moreover multimodal datasets with high quality biosignals are limited in terms of dimension and quantity. In this project we are working to develop a robust pipeline to analyse multiple kinds of biosignals (e.g. EDA, ECG, EEG, EMG etc.). The analysis comprehends a pre-processing step (Figure 9c), with filtering and artefact removal, and then a feature extraction and selection step. Furthermore, we are building a refined dataset with more than 60 participants (Figure 9a). The goal is to combine and compare biosignal with video analysis to infer on emotion recognition, at first. Nevertheless this approach can be extended to health data in order to assist clinicians in their daily activities developing new insights in neuroscience.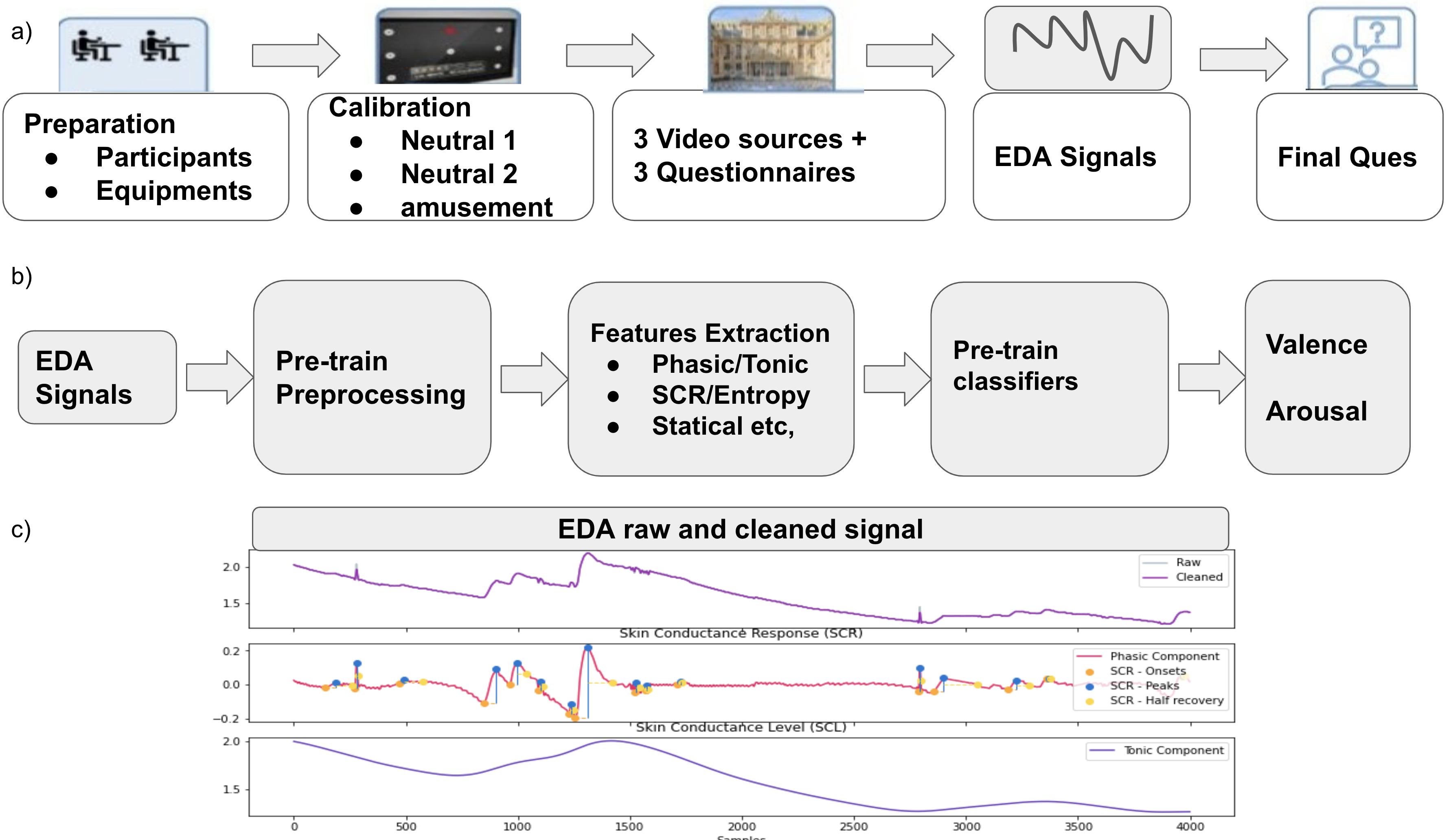
8.7 Learning-based approach for wearables design
Participants: Laura M. Ferrari, François Brémond.
A limiting factor towards the wide use of wearable devices for continuous healthcare monitoring is their cumbersome and obtrusive nature. This is particularly true in electroencephalography (EEG), where numerous electrodes are placed in contact with the scalp to perform brain activity recordings. We propose to identify the optimal wearable EEG electrode set, in terms of minimal number of electrodes, comfortable location and performance, for EEG-based event detection and monitoring 38. By relying on the demonstrated power of autoencoder (AE) networks to learn latent representations from high-dimensional data, our proposed strategy trains an AE architecture in a one-class classification setup with different electrode combinations as input data. Alpha waves detection is the use case through which we demonstrate that the proposed method allows to detect a brain state from an optimal set of electrodes. The so-called wearable configuration, consisting of electrodes in the forehead and behind the ear, is the chosen optimal set, with an average F-score of 0.78 (Figure 10). Comparing this work with the state-of-the-art, it can be related to the more general problem of feature selection. Since this accounts to select the EEG channels achieving the highest performing accuracy, these methods do not consider the required number of electrodes, comfort or discreteness as a selection criteria. However, some methods used in sleep studies have considered comfortable EEG channels, e.g. forehead electrodes, among their pool of features. The main difference with other methods is that the AE network here adopted permits the use of unbalanced training, which is an advantage in view of wearable implementation. Although not directly comparable, this formulation allows our method to achieve a higher accuracy (83%) than that one reported in previous works, in forehead electrodes (76-77%) 60, 62. The proposed method represents a proof-of-concept on how machine learning-based techniques can help the design of realistic wearables for every-day use, which can go beyond EEG applications.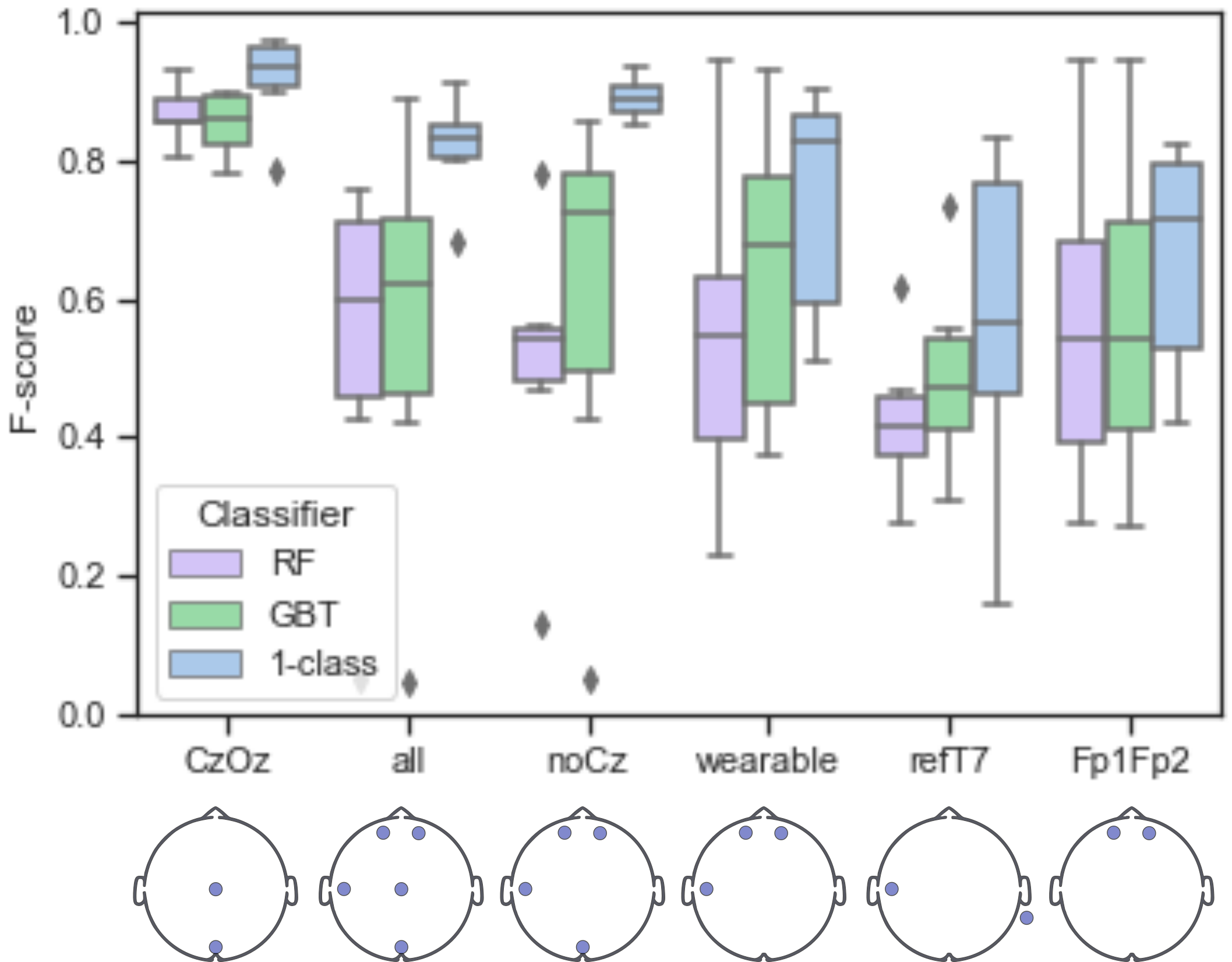
8.8 Computer Vision and Deep Learning applied to Facial Analysis in the invisible spectra
Participants: David Anghelone, Antitza Dantcheva.
Beyond the Visible - A survey on cross-spectral face recognition
This subject is within the framework of the national project SafeCity: Security of Smart Cities.
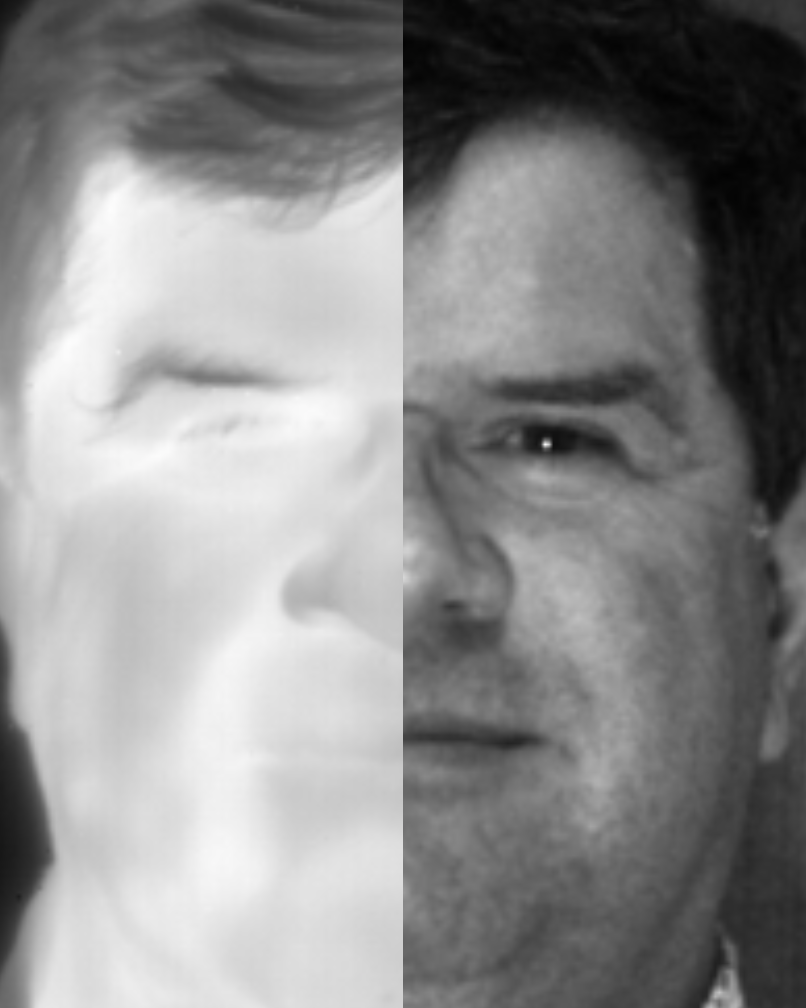
Face recognition has been a highly active area for decades and has witnessed increased interest in the scientific community. In addition, these technologies are being widely deployed, becoming part of our daily life. So far, these systems operate mainly in the visible spectrum as RGB-imagery, due to the ubiquity of advanced sensor technologies. However, limitations encountered in the visible spectrum such as illumination-restriction, variation in poses, noise as well as occlusion significantly degrades the recognition performance. In order to overcome such limitations, recent research has explored face recognition based on spectral bands beyond the visible. In this context, one pertinent scenario has been the matching of facial images that are sensed in different modalities - infrared vs. visible. Challenging in this recognition process has been the significant variation in facial appearance caused by the modality gap, this is depicted on Figure 11. Motivated by this, we conduced a survey on cross-spectral face recognition by providing an overview of recent advance and placing emphasis on deep learning methods.
8.9 Explainable Thermal to Visible Face Recognition using Latent-Guided Generative Adversarial Network
Participants: David Anghelone, Cunjian Chen, Philippe Faure, Arun Ross, Antitza Dantcheva.
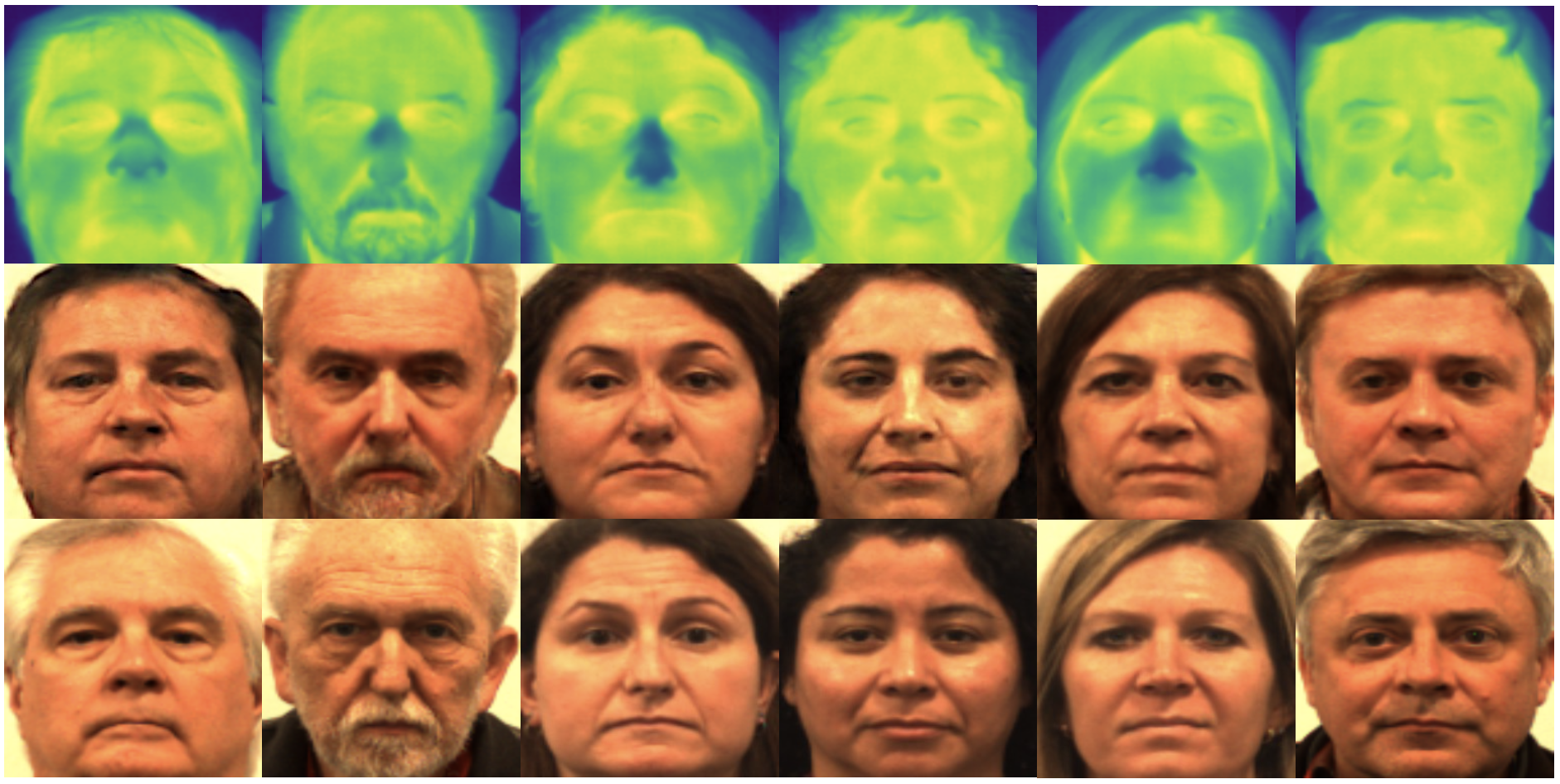
One major challenge in performing thermal-to-visible face image translation is preserving the identity across different spectral bands. Existing work does not effectively disentangle the identity from other confounding factors. We hence proposed LG-GAN 28
a Latent-Guided Generative Adversarial Network to explicitly decompose an input image into identity code that is spectral-invariant and style code that is spectral-dependent. By using such a disentanglement, we were able to analyze the identity preservation by interpreting and visualizing the identity code. We presented extensive face recognition experiments on two challenging Visible-Thermal face datasets.
In particular, LG-GAN increased facial recognition accuracy
8.10 Facial Landmark Heatmap Activated Multimodal Gaze Estimation
Participants: Neelabh Sinha, Michal Balazia, Mansi Mittal.
3D gaze estimation is about predicting the line of sight of a person in 3D space. Person-independent models (Figure 13(a)) lack precision due to anatomical differences of subjects, whereas person-specific calibrated techniques (Figure 13(b)) add strict constraints on scalability. To overcome these issues, we propose a novel technique, Facial Landmark Heatmap Activated Multimodal Gaze Estimation (FLAME), as a way of combining eye anatomical information using eye landmark heatmaps to obtain precise gaze estimation without any person-specific calibration (Figure 13(c)).
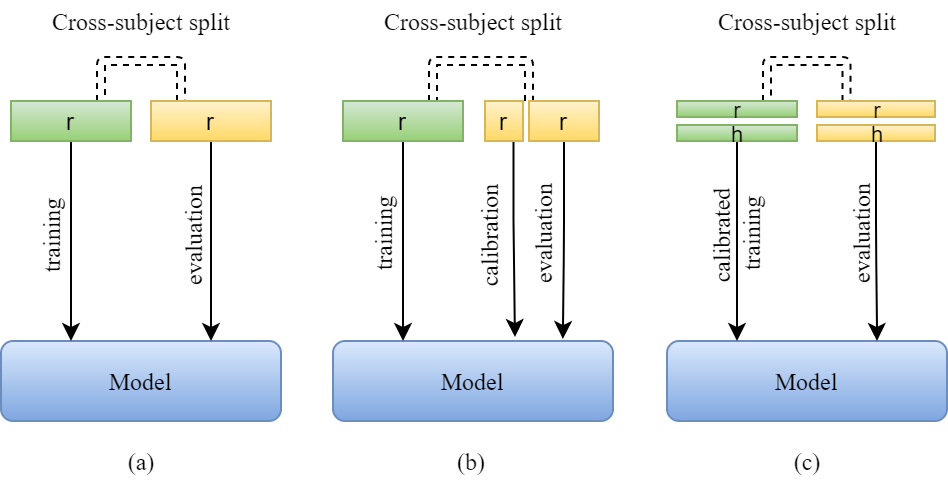
Different types of gaze estimation methods: (a) person-independent technique, (b) person-specific technique, (c) FLAME. Training subjects are green and test subjects are yellow. rr stands for RGB image and hh stands for eye landmark heatmap.
Information exchanged between the RGB stream and the eye landmark heatmap stream is defined by a transfer function based on Multimodal Transfer Module (MMTM). MMTM is a slow modality fusion block used to re-calibrate channel-wise features based on squeeze and excitation mechanism between any two feature maps of arbitrary dimension. To design the transfer function, we use this MMTM block for feature reactivation at the last and second last feature maps, as given in Figure 14. This is because we want the network to learn some initial representation and then, the higher-level features of both streams can be utilized by each other. At the deeper layers, using this transfer function can help both individual unimodal streams to learn better representation by benefiting from the information extracted by the other stream.
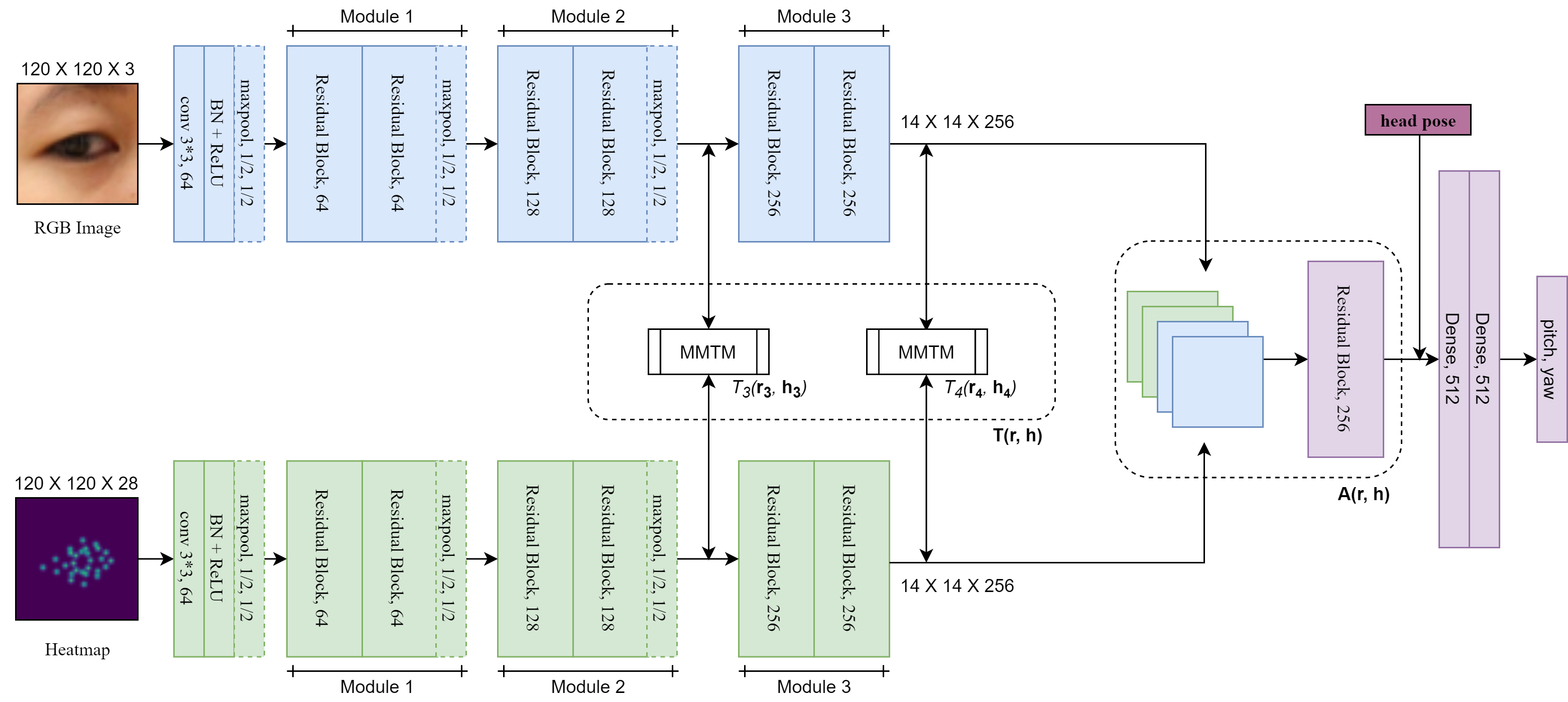
Evaluations reported in our paper 46 demonstrates a competitive performance of about 10% improvement on benchmark datasets ColumbiaGaze and EYEDIAP. To validate our method, we also conduct an ablation study, further proving that incorporating eye anatomical information plays a vital role in accurately predicting gaze.
8.11 ICE: Inter-instance Contrastive Encoding for Unsupervised Person Re-identification
Participants: Hao Chen, Benoit Lagade, François Brémond.
Recent self-supervised contrastive learning provides an effective approach for unsupervised person re-identification (ReID) by learning invariance from different views (transformed versions) of an input. In this work, we incorporate a Generative Adversarial Network (GAN) and a contrastive learning module into one joint training framework. While the GAN provides online data augmentation for contrastive learning, the contrastive module learns view-invariant features for generation, as shown in Figure 15. In this context, we propose a mesh-based view generator. Specifically, mesh projections serve as references towards generating novel views of a person. In addition, we propose a view-invariant loss to facilitate contrastive learning between original and generated views. Deviating from previous GAN-based unsupervised ReID methods involving domain adaptation, we do not rely on a labeled source dataset, which makes our method more flexible. Extensive experimental results show that our method 32 significantly outperforms state-of-the-art methods under both, fully unsupervised and unsupervised domain adaptive settings on several large scale ReID datsets. Source code and models are available under . This work has been published in IEEE/CVF Conference on Computer Vision and Pattern Recognition (CVPR) 2021.

Left: Traditional self-supervised contrastive learning maximizes agreement between representations (f1f_1 and f2f_2) of augmented views from Data Augmentation (DA). Right: Joint generative and contrastive learning maximizes agreement between original and generated views.
8.12 Joint Generative and Contrastive Learning for Unsupervised Person Re-identification
Participants: Hao Chen, Yaohui Wang, Benoit Lagade, Antitza Dantcheva, François Brémond.
Unsupervised person re-identification (ReID) aims at learning discriminative identity features without annotations. Recently, self-supervised contrastive learning has gained increasing attention for its effectiveness in unsupervised representation learning. The main idea of instance contrastive learning is to match a same instance in different augmented views. However, the relationship between different instances has not been fully explored in previous contrastive methods, especially for instance-level contrastive loss. To address this issue, we propose Inter-instance Contrastive Encoding (ICE) 31 that leverages inter-instance pairwise similarity scores to boost previous class-level contrastive ReID methods. We first use pairwise similarity ranking as one-hot hard pseudo labels for hard instance contrast, which aims at reducing intra-class variance. Then, we use similarity scores as soft pseudo labels to enhance the consistency between augmented and original views, which makes our model more robust to augmentation perturbations. Experiments on several large-scale person ReID datasets validate the effectiveness of our proposed unsupervised method ICE, as shown in Figure 16, which is competitive with even supervised methods. Code is made available at github. This work has been published in IEEE/CVF International Conference on Computer Vision (ICCV) 2021.
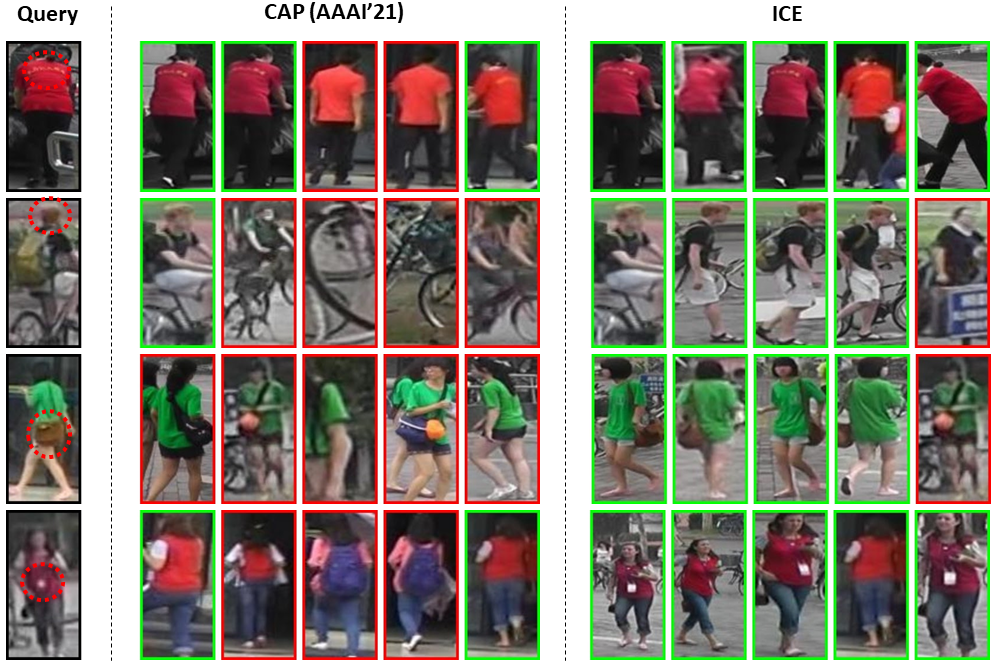
Comparison of top 5 retrieved images on Market1501 between CAP and ICE. Green boxes denote correct results, while red boxes denote false results. Important visual clues are marked with red dashes.
8.13 Emotion Editing in Head Reenactment Videos using Latent Space Manipulation
Participants: Valeriya Strizhkova, François Brémond, Antitza Dantcheva, Yaohui Wang, David Anghelone, Di Yang.
Video generation greatly benefits from integrating facial expressions, as they are highly pertinent in social interaction and hence increase realism in generated talking head videos. Motivated by this, we propose a method for editing emotions in head reenactment videos that is streamlined to modify the latent space of a pre-trained neural head reenactment system 47. Specifically, our method seeks to disentangle emotions from the latent pose and identity representation. The proposed learning process is based on cycle consistency and image reconstruction losses. Our results suggest that despite its simplicity, such learning successfully decomposes emotion from pose and identity. Our method reproduces facial mimics of a person from a driving video, as well as allows for emotion editing in the reenactment video. We compare our method to the state-of-art for altering emotions in reenactment videos, producing more realistic results that the state-of-art.
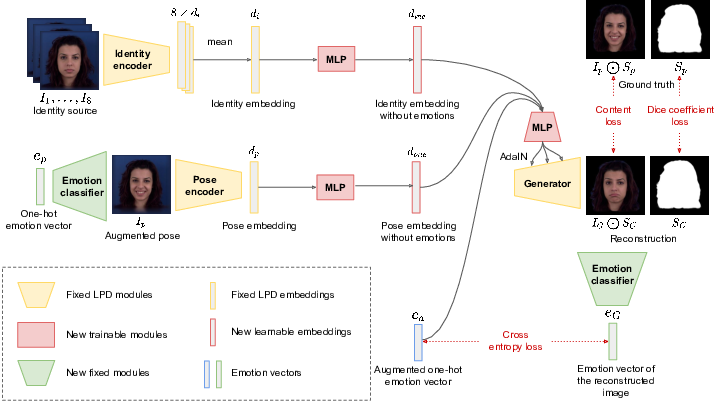
8.14 Learning to Generate Human Video
Participants: Yaohui Wang, Antitza Dantcheva, François Brémond.
Generative Adversarial Networks (GANs) have witnessed increasing attention due to their abilities to model complex visual data distributions, which allow them to generate and translate realistic images. While realistic video generation is the natural sequel, it is substantially more challenging w.r.t. complexity and computation, associated to the simultaneous modeling of appearance, as well as motion. Specifically, in inferring and modeling the distribution of human videos, generative models face three main challenges: (a) generating uncertain motion and retaining of human appearance, (b) modeling spatio-temporal consistency, as well as (c) understanding of latent representation.
In this thesis 56, we propose three novel approaches towards generating high-visual quality videos and interpreting latent space in video generative models. We firstly introduce a method, which learns to conditionally generate videos based on single input images. Our proposed model allows for controllable video generation by providing various motion categories. Secondly, we present a model, which is able to produce videos from noise vectors by disentangling the latent space into appearance and motion. We demonstrate that both factors can be manipulated in both, conditional and unconditional manners. Thirdly, we introduce an unconditional video generative model that allows for interpretation of the latent space. We place emphasis on the interpretation and manipulation of motion. We show that our proposed method is able to discover semantically meaningful motion representations, which in turn allow for control in generated results. Finally, we describe a novel approach to combine generative modeling with contrastive learning for unsupervised person re-identification. Specifically, we leverage generated data as data augmentation and show that such data can boost re-identification accuracy.
8.15 Guided Flow Field Estimation by Generating Independent Patches
Participants: Mohsen Tabejamaat, Farhood Negin, François Brémond.
Guided flow field estimation is a novel strategy for high fidelity generation of fashion images. Current strategies propose to warp the samples using an offline pre-training fashion, where an additional network is considered for extracting the trajectory of warping flows so as to be further used as a prior to the main generative process. While interesting, pushing the network in a pseudo-Siamese way leads to a huge number of parameters, ending up to a significantly reduced generalization ability of the network. To address the issue, we propose the flow maps to adaptively learn from the estimations of the output sample rather than the fix keypoints at the input of the network (Figure 17), published in BMVC 2021 52. Finding a solution that enables for a fine trade off between the quantity of the parameters and the quality of samples was at the forefront of our project definition.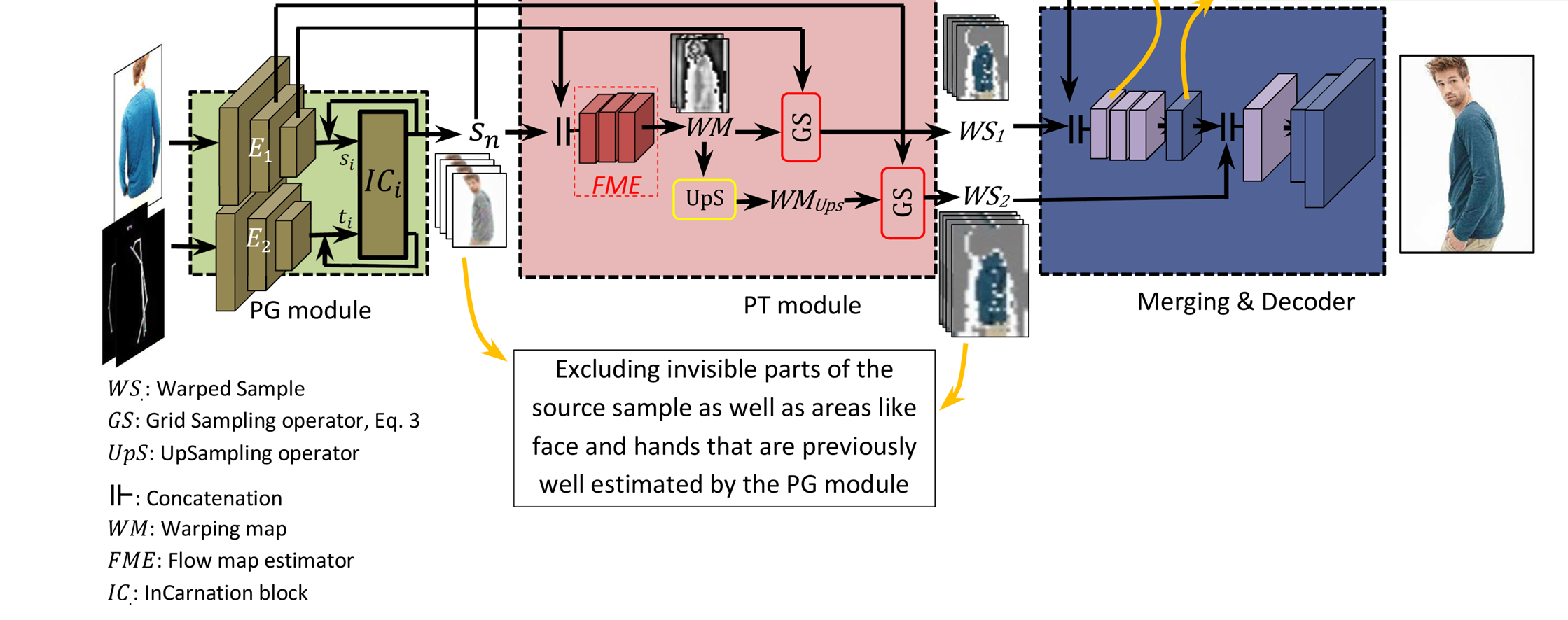
We also proposed a patch generation module which helps the transfer function be specialized on specific tasks. We proposed the target patches to be estimated from the same locations in the source sample but through two distinct functions that act as individual experts on the source and target samples.
8.16 BVPNet: Video-to-BVP Signal Prediction for Remote Heart Rate Estimation
Participants: Abhijit Das, Antitza Dantcheva.
We propose a new method for remote photoplethysmography (rPPG) based heart rate (HR) estimation. In particular, our proposed method BVPNet 37 is streamlined to predict the blood volume pulse (BVP) signals from face videos. Towards this, we firstly define ROIs based on facial landmarks and then extract the raw temporal signal from each ROI. Then the extracted signals are pre-processed via first-order difference and Butterworth filter and combined to form a Spatial-Temporal map (STMap). We then propose to revise U-Net, in order to predict BVP signals from the STMap. BVPNet takes into account both temporal and frequency domain losses in order to learn better than conventional models. Our experimental results suggest that our BVPNet outperforms the state-of-the-art methods on two publicly available datasets (MMSE-HR and VIPL-HR).
8.17 Demystifying Attention Mechanisms for Deepfake Detection
Participants: Abhijit Das, Ritaban Roy, Indu Joshi, Srijan Das, Antitza Dantcheva.
Manipulated images and videos, i.e., deepfakes have become increasingly realistic due to the tremendous progress of deep learning methods. However, such manipulation has triggered social concerns, necessitating the introduction of robust and reliable methods for deepfake detection. In this works 53, 36, we explore a set of attention mechanisms and adapt them for the task of deepfake detection. Generally, attention mechanisms in videos modulate the representation learned by a convolutional neural network (CNN) by focusing on the salient regions across space-time. In our scenario, we aim at learning discriminative features to take into account the temporal evolution of faces to spot manipulations. To this end, we address the two research questions `How to use attention mechanisms?', and `What type of attention is effective for the task of deepfake detection?' Towards answering these questions, we provide a detailed study and experiments on videos tampered by four manipulation techniques, as included in the FaceForensics++ dataset. We investigate three scenarios, where the networks are trained to detect (a) all manipulated videos, (b) each manipulation technique individually, as well as (c) the veracity of videos pertaining to manipulation techniques not included in the train set.
8.18 Computer Vision for deciphering and generating faces
Participants: Antitza Dantcheva.
The main volume of the HDR 54 is in computer vision, and it aims to holistically decipher information enciphered in human faces.Motivation originates from the emerging importance of automated face-analysis in our evolving society, be it for security or health applications, as well as from the practicality of such systems. Specifically, we have placed emphasis on learning representations of human faces concerning two main domains of application: security and healthcare. While seemingly different, these applications share the core processing-competence, which has proven to be beneficial as it has brought to the fore cross-fertilization of ideas across areas. With respect to security, we have designed algorithms, which extract soft biometrics attributes such as gender, age, ethnicity, height and weight. We have aimed at mitigating bias, when estimating such attributes. Prior, we have established the impact of facial cosmetics on automated face analysis systems and have then focused on the design of methods that reduce such impact and ensure for makeup-robust face recognition.
Results related to healthcare deal with facial behavioral analysis, as well as apathy analysis of Alzheimer's disease patients. In our current work with the STARS team of Inria and the Cognition Behaviour Technology (CoBTeK) lab of the Université Côte d'Azur, we have developed a series of spatio-temporal methods for facial behavior, emotion and expression recognition.
Most recently, we have additionally focused on Generative Adversarial Networks (GANs), which have witnessed increasing attention due to their abilities to model complex visual data distributions. We have proposed a number of novel approaches towards conditional and unconditional generation of realistic videos and have additionally aimed at disentangling the latent space into appearance and motion, as well as interpreting it.
8.19 DAM : Dissimilarity Attention Module for Weakly-supervised Video Anomaly Detection
Participants: Snehashis Majhi, Srijan Das, François Brémond.
Video anomaly detection under weak supervision is complicated due to the difficulties in identifying the anomaly and normal instances during training, hence, resulting in non-optimal margin of separation. In this paper, we propose a framework consisting of Dissimilarity Attention Module (DAM) 44 to discriminate the anomaly instances from normal ones both at feature level and score level. In order to decide instances to be normal or anomaly, DAM takes local spatio-temporal (i.e. clips within a video) dissimilarities into account rather than the global temporal context of a video 45. This allows the framework to detect anomalies in real-time (i.e. online) scenarios without the need of extra window buffer time. Further more, we adopt two-variants of DAM for learning the dissimilarities between successive video clips. The proposed framework along with DAM is validated on two large scale anomaly detection datasets i.e. UCF-Crime and ShanghaiTech, outperforming the online state-of-the-art approaches by 1.5
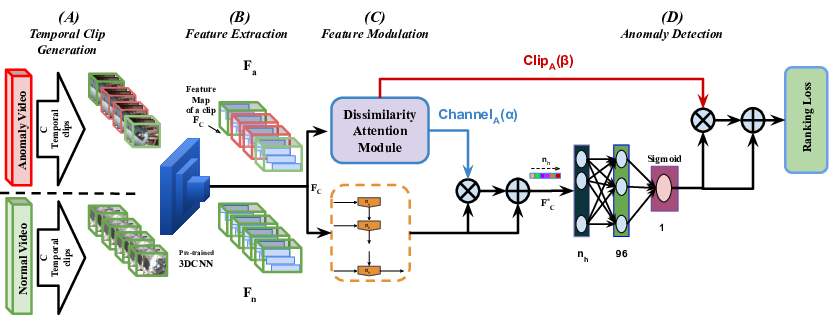
8.20 Pyramid Dilated Attention Network
Participants: Rui Dai, Srijan Das, François Brémond.
Handling long and complex temporal information is an important challenge for action detection tasks. This challenge is further aggravated by densely distributed actions in untrimmed videos. Previous action detection methods fail in selecting the key temporal information in long videos. To this end, we introduce the Dilated Attention Layer (DAL) 35, see Fig. 20. Compared to previous temporal convolution layer, DAL allocates attentional weights to local frames in the kernel, which enables it to learn better local representation across time. Furthermore, we introduce Pyramid Dilated Attention Network (PDAN) which is built upon DAL, see Fig. 21. With the help of multiple DALs with different dilation rates, PDAN can model short-term and long-term temporal relations simultaneously by focusing on local segments at the level of low and high temporal receptive fields. This property enables PDAN to handle complex temporal relations between different action instances in long untrimmed videos. To corroborate the effectiveness and robustness of our method, we evaluate it on three densely annotated, multi-label datasets: MultiTHUMOS, Charades and Toyota Smarthome Untrimmed (TSU) dataset. PDAN is able to outperform previous state-of-the-art methods on all these datasets. This work was published in the Winter Conference on Applications of Computer Vision 2021 (WACV 2021).
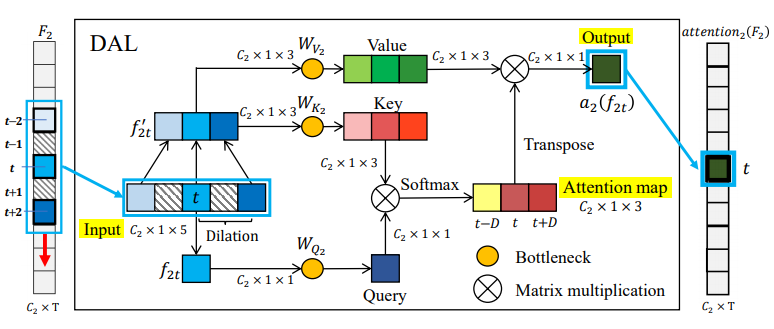
Dilated Attention Layer (DAL). In this figure, we present a computation flow inside the kernel at time step tt for layer ii=2 (kernel size KS is 3, dilation rate D is 2). Afterwards, DAL processes one step forward following the red arrow at time t+1t+1.
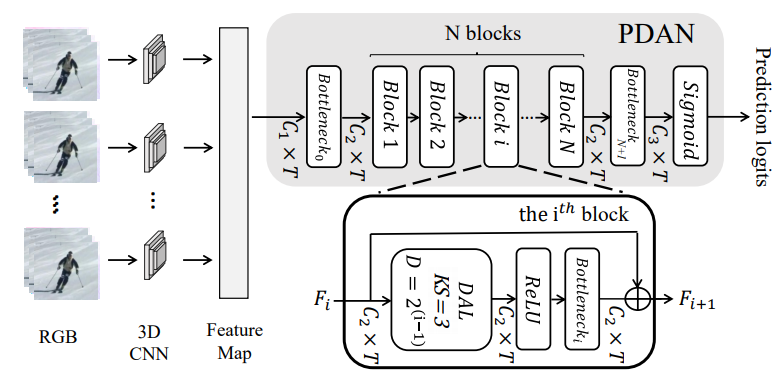
8.21 Class-Temporal Relational Network
Participants: Rui Dai, Srijan Das, François Brémond.
Action detection is an essential and challenging task, especially for densely labelled datasets of untrimmed videos. There are many real-world challenges in those datasets, such as composite action, co-occurring action, and high temporal variation of instance duration. For handling these challenges, we propose to explore both the class and temporal relations of detected actions. We introduce an end-to-end network 33 (see Fig. 22): Class-Temporal Relational Network (CTRN). It contains three key components: (1) The Representation Transform Module filters the class-specific features from the mixed representations to build graph-structured data. (2) The Class-Temporal Module models the class and temporal relations in a sequential manner. (3) G-classifier leverages the privileged knowledge of the snippet-wise co-occurring action pairs to further improve the co-occurring action detection. We evaluate CTRN on three challenging densely labelled datasets and achieve state-of-the-art performance, reflecting the effectiveness and robustness of our method. This work is accepted in The British Machine Vision Conference 2021 (BMVC 2021) 33 as an oral presentation.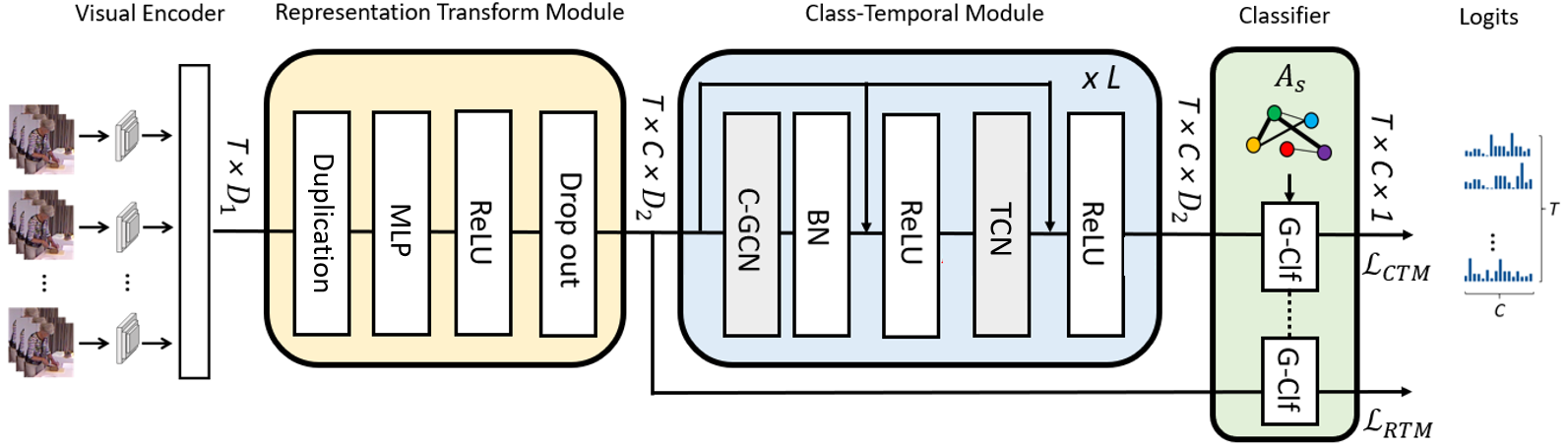
8.22 Learning an Augmented RGB Representation with Cross-Modal Knowledge Distillation for Action Detection
Participants: Rui Dai, Srijan Das, François Brémond.
In video understanding, most cross-modal knowledge distillation (KD) methods are tailored for classification tasks, focusing on the discriminative representation of the trimmed videos. However, action detection requires not only categorizing actions, but also localizing them in untrimmed videos. Therefore, transferring knowledge pertaining to temporal relations is critical for this task which is missing in the previous cross-modal KD frameworks. To this end, we aim at learning an augmented RGB representation for action detection, taking advantage of additional modalities at training time through KD. We propose a KD framework 34 consisting of two levels of distillation (see Fig. 23). On one hand, atomic-level distillation encourages the RGB student to learn the sub-representation of the actions from the teacher in a contrastive manner. On the other hand, sequence-level distillation encourages the student to learn the temporal knowledge from the teacher, which consists of transferring the Global Contextual Relations and the action Boundary Saliency. The result is an Augmented-RGB stream that can achieve competitive performance as the two-stream network while using only RGB at inference time. Extensive experimental analysis shows that our proposed distillation framework is generic and outperforms other popular cross-modal distillation methods in the action detection task. This work was published in the International Conference on Computer Vision 2021 (ICCV 2021) 34.
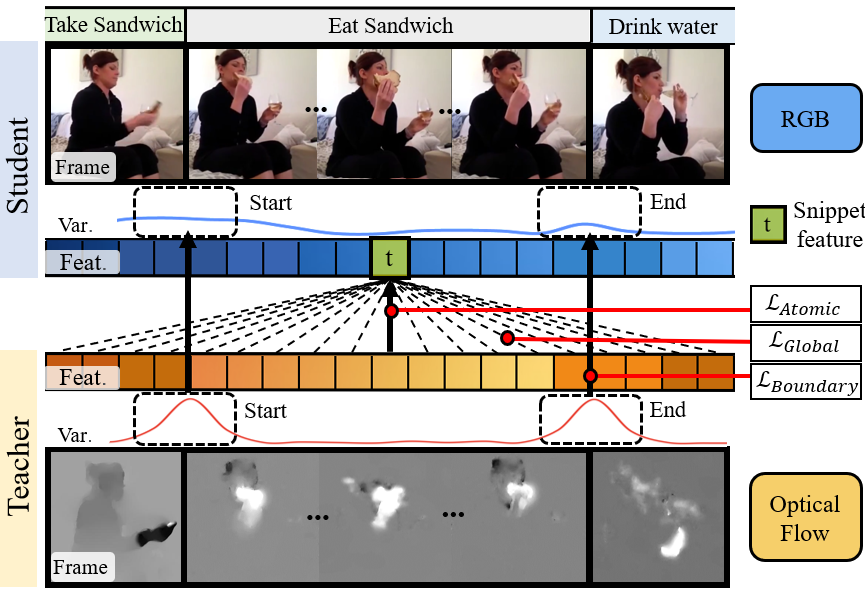
Proposed cross-modal distillation framework for action detection. Our distillation framework is composed of three loss terms corresponding to different types of knowledge to transfer across modalities. ℒAtomic\mathcal {L}_{Atomic}: Atomic KD loss; ℒGlobal\mathcal {L}_{Global}: Global Contextual Relation loss; ℒBoundary\mathcal {L}_{Boundary}: Boundary Saliency loss.
8.23 VPN++: Rethinking Video-Pose embeddings for understanding Activities of Daily Living
Participants: Rui Dai, Srijan Das, François Brémond.
Many attempts have been made towards combining RGB and 3D poses for the recognition of Activities of Daily Living (ADL). ADL may look very similar and often necessitate to model fine-grained details to distinguish them. Because the recent 3D ConvNets are too rigid to capture the subtle visual patterns across an action, this research direction is dominated by methods combining RGB and 3D Poses. But the cost of computing 3D poses from RGB stream is high in the absence of appropriate sensors. This limits the usage of aforementioned approaches in real-world applications requiring low latency. Then, how to best take advantage of 3D Poses for recognizing ADL? To this end, we propose an extension of a pose driven attention mechanism: Video-Pose Network (VPN) 17, exploring two distinct directions. One is to transfer the Pose knowledge into RGB through a feature-level distillation and the other towards mimicking pose driven attention through an attention-level distillation. Finally, these two approaches are integrated into a single model, we call VPN++ (see Fig. 24). We show that VPN++ is not only effective but also provides a high speed up and high resilience to noisy Poses. VPN++, with or without 3D Poses, outperforms the representative baselines on 4 public datasets. This work is accepted in Transactions on Pattern Analysis and Machine Intelligence (TPAMI) 17.
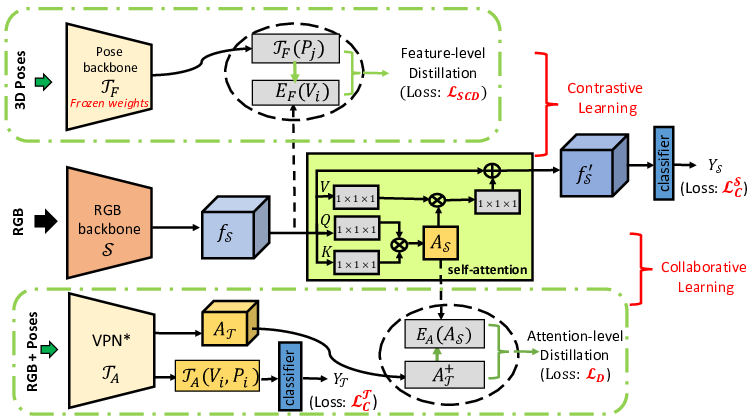
8.24 Multimodal Personality Recognition using Cross-Attention Transformer and Behaviour Encoding
Participants: Tanay Agrawal, Dhruv Agrawal, Neelabh Sinha, François Brémond.
Personality computing and affective computing have gained recent interest in many research areas. The datasets for the task generally have multiple modalities like video, audio, language and bio-signals. We propose a flexible model for the task which exploits all available data. The task involves complex relations and, to avoid using a large model for video processing specifically, we propose the use of behaviour encoding which boosts performance with minimal change to the model. Cross-attention using transformers has become popular in recent times and is utilised for fusion of different modalities. Since long term relations may exist, breaking the input into chunks is not desirable, thus the proposed model processes the entire input together.
Our approach uses face crops of the target person and relates it to body language, surroundings and speech using a transformer based architecture. Short-term temporal relations are processed in this way and longer temporal relations are established using LSTM. For transcript analysis, short term temporal relations are not very meaningful so the features for the entire input sequences are extracted using BERT. Late fusion is then finally used for inferring the OCEAN personality traits. Figure 25 shows the overview of the entire architecture.
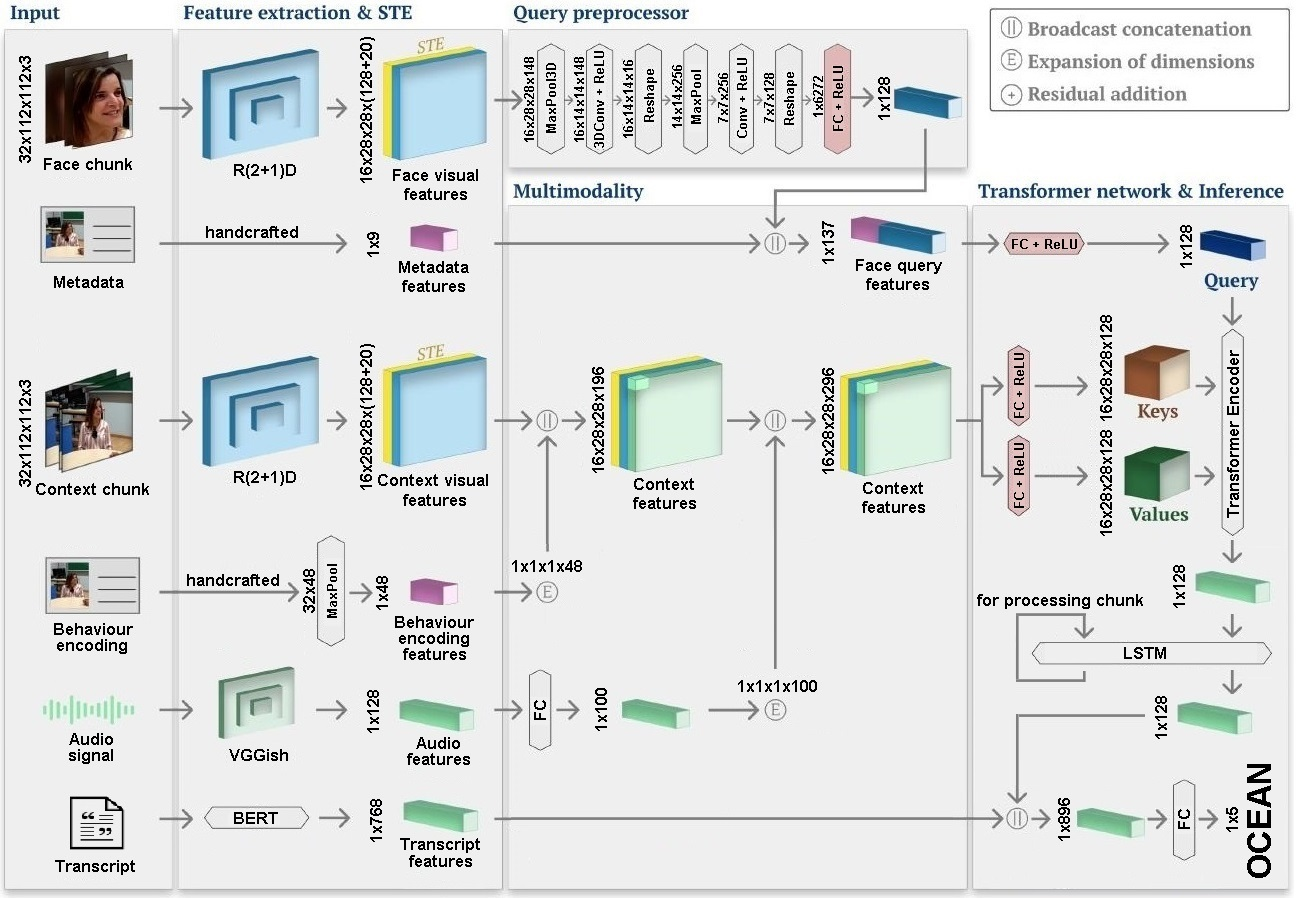
In our paper 26 we show that a model for personality recognition will benefit from more modalities and data as input. We further show the effectiveness of all the inputs in the data through ablation studies. We also give our opinion on the trends shown in the ablation studies. Owing to the interdisciplinary nature of the project, there are numerous additions that will further improve performance. From intuition, there are some which might improve performance by a higher margin than others. Using better backbones for feature extraction would be interesting. We use the same ones as in the baseline we choose but there are existing models with better performance for similar tasks that can be utilised. Transformers have been shown to perform better than LSTMs.
8.25 From Multimodal to Unimodal Attention in Transformers using Knowledge Distillation
Participants: Dhruv Agarwal, Tanay Agrawal, Laura M. Ferrari, François Brémond.
Multimodal Deep Learning has garnered much interest and transformers have triggered novel approaches, thanks to the cross-attention mechanism. Here we propose an approach 25 to deal with two key existing challenges: the high computational resource demanded and the issue of missing modalities. We introduce for the first time the concept of knowledge distillation in transformers to use only one modality at inference time. We report a full study analyzing multiple student-teacher configurations, levels at which distillation is applied, and different methodologies. With the best configuration, we improved the state-of-the-art accuracy by 3% , we reduced the number of parameters by 2.5 times and the inference time by 22%. Such performance-computation trade off can be exploited in many applications and we aim at opening a new research area where the deployment of complex models with limited resources is demanded. Figure 26 shows the proposed framework for the approach. This work has been published in the 17th IEEE International Conference on Advanced Video and Signal-based Surveillance (AVSS 2021) 25.

8.26 Quantified Analysis for Video Recordings of Seizure
Participants: Jen-Cheng Hou, Monique Thonnat.
We finish a doctoral thesis regarding quantified analysis for video recordings of seizure 55. Epilepsy is a neurological disorder caused by abnormal neuron activity in the brain. Around 1% of the population worldwide is affected by it. Numerous motor manifestations (including convulsions, tonic, clonic, hyperkinetic changes) can be observed and are a source of major disability for patients. The motivation of this research is to develop methods based on recent machine learning techniques to provide objective analysis for clinical seizure videos.
In this thesis, we propose three main contributions towards automated vision-based seizure analysis. In the first contribution, we explore some hyperkinetic epileptic seizures by analyzing the head movement trajectories of the patients. The results provide a basis for studying the correlation between the spectrum of EEG and the head movement frequency. Nevertheless, epilepsy is not the only cause that gives rise to a seizure event. For example, psychogenic non-epileptic seizures (PNES) are one of them. They are events resembling an epileptic seizure (ES), but without the characteristic electrical discharges associated with epilepsy. How to distinguish them is important for accurate diagnosis and follow-up treatments. The clinical signs or semiology are evaluated by neurologists, but the subjective interpretation is liable for inter-observer variability. Hence, there is an urgent need to build an automated system to analyze seizure videos with the latest computer vision progress. In this research, we propose two other contributions for classifying ES and PNES solely based on the videos. Our second contribution utilizes multi-stream information from appearance and key-points for both the bodies and faces of the patients. In addition by introducing the knowledge distillation mechanism, the performance of the F1-score and the accuracy are 0.85 and 0.82. Furthermore, based on this approach, we conduct a side experiment for distinguishing ES with emotion/non-emotion and dystonia/non-dystonia based on the face and body streams in the method. The LOSO validation gives satisfactory results, indicating our model can capture effective spatio-temporal features for face and body for seizure analysis.
In our third contribution, we propose a two-step model which is first pre-trained on large contextual videos then this model is fine-tuned for seizure type classification. This part is detailed in the following section.
8.27 A Self-supervised pre-training framework for Vision-based Seizure Classification
Participants: Jen-Cheng Hou, Monique Thonnat.
We propose a Transformer-based 68 self-supervised pre-training framework for learning features suitable for the downstream task, i.e. classifying epileptic seizures (ES) and psychogenic non-epileptic seizures (PNES) videos. In our previous work, a multi-stream deep learning approach 40 for ES/PNES classification has been proposed. Nevertheless, instead of training on a limited number of clinical labeled videos, we wonder if we can use the unlabeled clinical data for facilitating the model training. Inspired by BERT 61, our proposed paradigm aligns with the research direction of self-supervised pre-training that takes advantage of large unannotated data and learns useful representations from it for downstream tasks. This may be especially favored for medical applications where data annotations are usually costly. In our work, a Transformer-based model is pre-trained on a large volume of contextual videos with denoising pre-training objectives. The contextual videos cover the daily behaviors of patients in the Video-SEEG/Video-EEG monitoring unit, and they are easier to access and collect. By simply fine-tuning the pre-trained model with a minimum model modification, the experimental classification results can compete with methods from other state-of-the-art works for similar tasks. To our knowledge, this is the first deep learning work exploiting large unlabeled data for facilitating vision-based seizure analysis. We hope our study can inspire the research community regarding seizure video analysis to rethink how we can benefit from large unannotated data. This work has been submitted for peer review.
Pre-training and fine-tuning the model Inspired by BART 66, another Transformer-based SSL model for NLP, which corrupts input text with an arbitrary noising function and makes Transformer to reconstruct the original text, we include this concept of denoising objective into our model in the pre-training phase, as shown in Fig. 27. For each contextual video, we perturb the frame ordering and randomly mask out some frames, resulting in a corrupted version of the original video frames. The pre-training objective is to regress the Transformer output of each frame in the corrupted input to the visual features of the original one. After pre-training, we add a fully-connected layer on top of the pre-trained Transformer for classification, as shown in Fig. 28. Then fine-tune the whole model on the target dataset, which contains seizure videos for seizure type classification with the standard cross-entropy loss. The task is a binary classification which aims to distinguish epileptic seizures (ES) from psychogenic non-epileptic seizures (PNES).
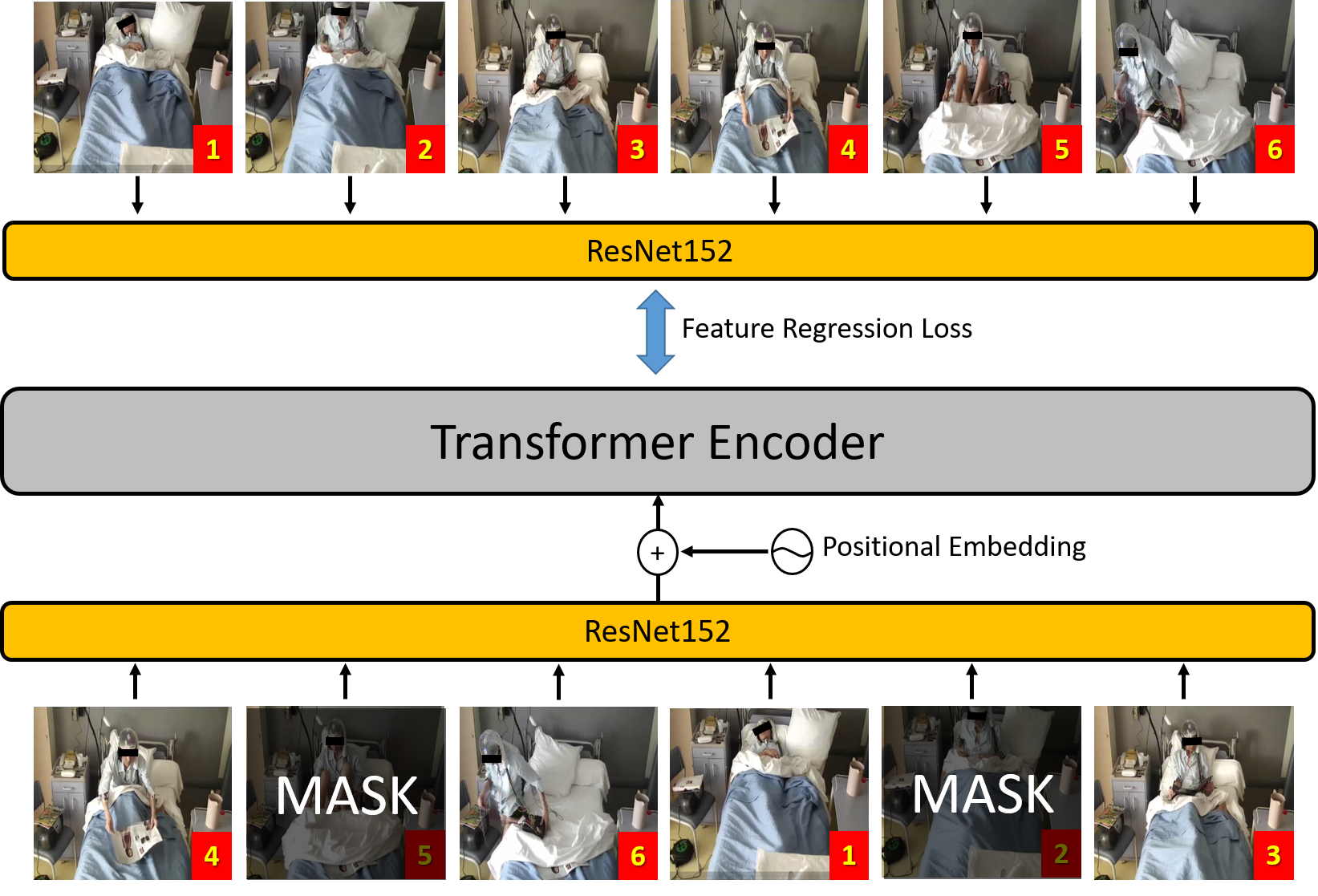
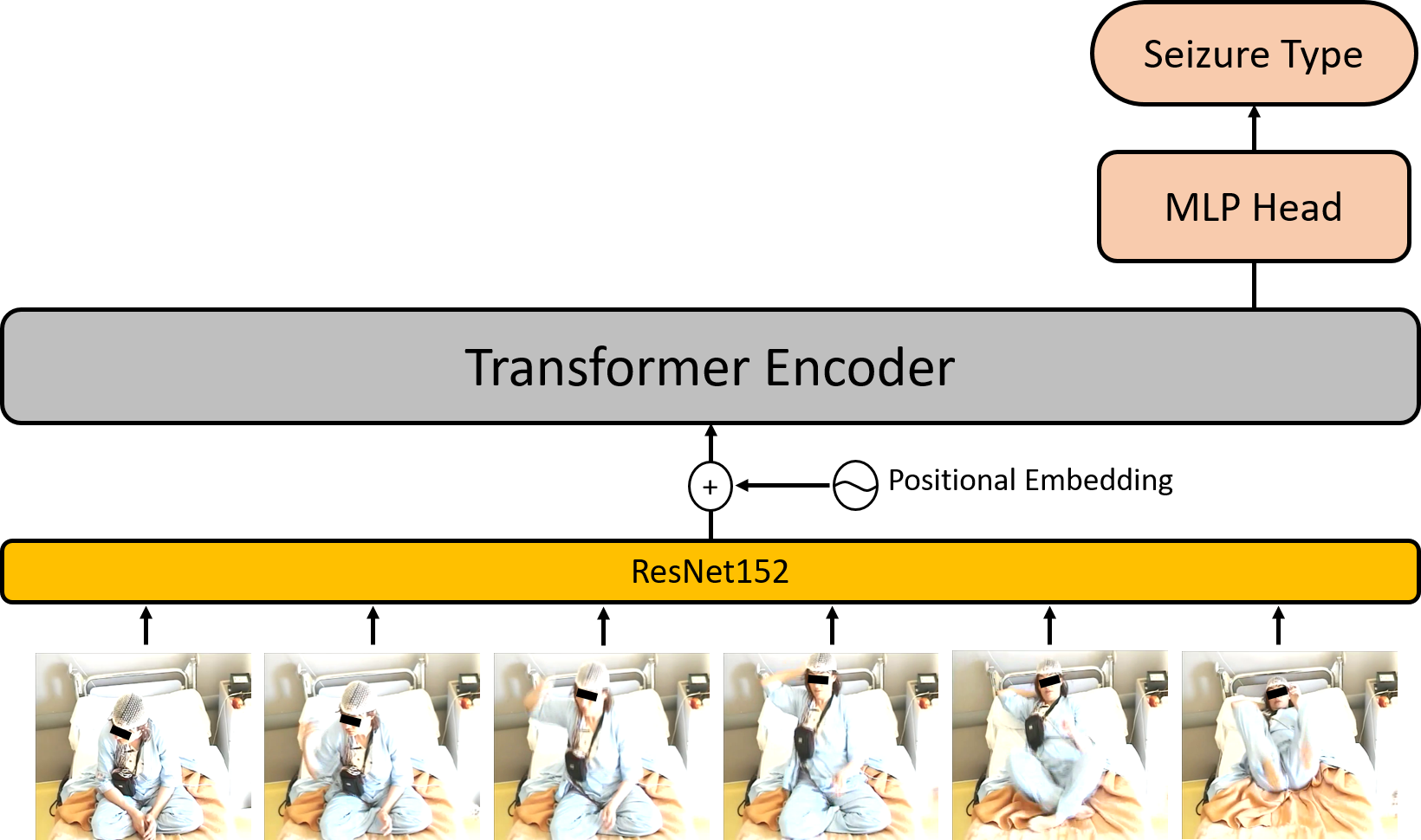
Experimentation The target dataset for classification contains 283 trimmed seizure videos, and among them, 235 videos belong to ES, and 48 videos are PNES. A total of 81 patients are involved, in which the ES and PNES class has 52 and 29 patients, respectively. The length of seizure videos ranges from 7 seconds to 150 seconds. We perform a leave-one-subject-out (LOSO) validation for evaluation. The F1-score and the accuracy are 0.82 and 0.75, respectively. As shown in Table 1, our results are comparable to other state-of-the-art seizure classification tasks given different class targets. This indicates our proposed Transformer-based pre-training approach can learn robust and generalizable features for the downstream task. The video-wise confusion matrix is shown in Table 2.
Method | Classes | Performance |
A.-Aristizaba et al. (2018) 57 | MTLE |
Average accuracy: |
Maia et al. (2019) 67 | TLE |
Accuracy: 0.83 |
Karácsony et al. (2020) 63 | TLE |
F1-score: 0.84 |
Hou et al. (2021) 40 | ES |
F1-score: 0.76 |
Ours | ES |
F1-score: 0.82 |
|
||
ES |
|
|
PNES |
||
(true) | ||
ES |
181 | 54 |
(true) | ||
PNES |
15 | 33 |
8.28 Video-based Behavior Understanding of Children for Objective Diagnosis of Autism
Participants: Abid Ali, Farhood Negin, Sebastien Gilbert, François Brémond, Susanne Thümmler, Monique Thonnat.
One of the major diagnostic criteria for Autism Spectrum Disorder (ASD) is the recognition of stereotyped behaviors. However, it primarily relies on parental interviews and clinical observations, which result in a prolonged diagnosis cycle preventing ASD children from timely treatment. To help clinicians speed up the diagnosis process, we propose a computer-vision-based solution. First, we collected and annotated a novel dataset for action recognition tasks in videos of children with ASD in an uncontrolled environment. Second, we propose a multi-modality fusion network based on 3D CNNs. In the first stage of our method, we pre-process the RGB videos to get the ROI (child) using Yolov5 and DeepSORT algorithms. For optical flow extraction, we use the RAFT algorithm. In the second stage, we perform extensive experiments on different deep learning frameworks to propose a baseline. In the last stage, a multi-modality-based late fusion network is proposed to classify and evaluate performance of ASD children. The results revealed that the multi-modality fusion network achieves the best accuracy as compared to other methods. The baseline results also demonstrate the potential of an action-recognition-based system to assist clinicians in a reliable, accurate, and timely diagnosis of ASD disorder.Prior to action recognition, we pre-process our data, firstly detecting each person followed by tracking in the videos. A modified I3D network based on RGB and optical flow has been used to classify actions in video clips as explained in Fig 29. More details are in 27.
We are creating our own dataset called Activis. The Activis dataset consists of 60 children recorded during child assessment sessions with presence of clinicians at the hospital. The actions in the dataset were divided in five categories (388 videos in total): arm-flapping, clapping, to-taste, jump-up and others. For further details see 27.
We evaluate our algorithm in K-fold cross validation manner. The value of K was kept 5 in all experiments. We conduct experiments on two datasets (Activis and SSBD). The results are given in details in 27.
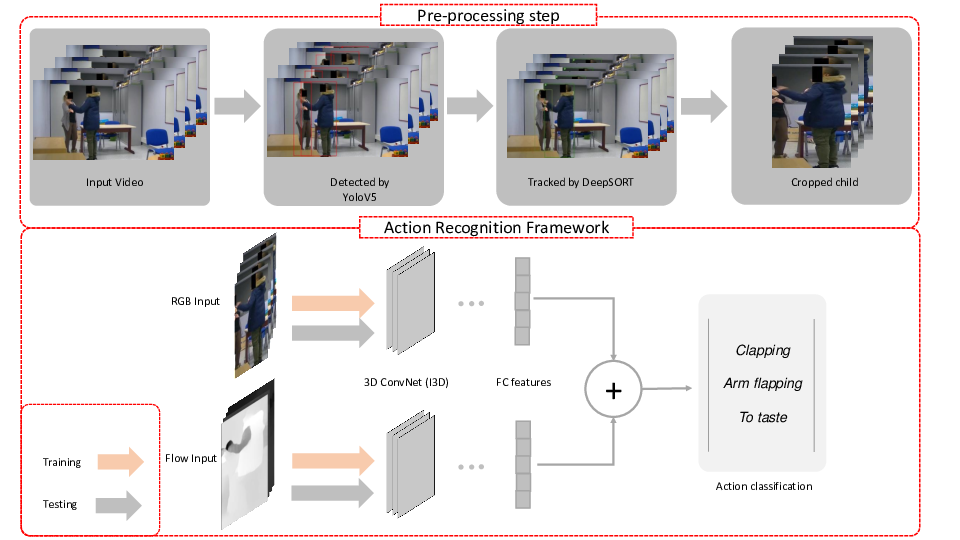
8.29 Human activity recognition for interaction scerarios
Participants: Mohammad Guermal, François Brémond.
To improve the performance of 3D-CNNs for recognizing Human Object Interactions, we propose to add a set of additional modules on top of these structures. As mentioned earlier, we want to have specific semantics for different objects present in the scene. Traditional CNNs fail at doing so as they extract full scene features. To leverage such semantics, we present the object representation filter. This module can extract class-specific features from the mixed representation and finally maps this representation to graph-like data. To process these semantics, we add Object Relation Reasoning module based on graph convolutions to leverage the temporal as well as the spatial relations between different objects in a sequential manner. Our intuition behind graph convolutions is the fact that its architecture is very descriptive of the problem of interactions. We can easily model the scene by building a graph where nodes represent objects while edges represent their relations. Finally, we can build a model that starts from a global scene representation and map it to class-specific semantics to leverage the relation (interaction) between these classes. Such a module is more representative and more adequate to HOIs actions.Below in Figure 30 we have the overall framework.
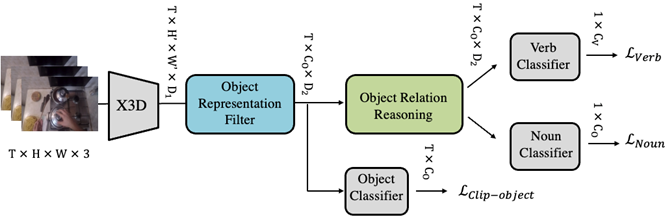
8.30 Self-Supervised Video Pose Representation Learning for Occlusion-Robust Action Recognition
Participants: Di Yang, Yaohui Wang, Antitza Dantcheva, Lorenzo Garattoni, Gianpiero Francesca, François Brémond.
Action recognition based on human pose has witnessed increasing attention due to its robustness to changes in appearances, environments, and view-points. Despite associated progress, one remaining challenge has to do with occlusion in real-world videos that hinders the visibility of all joints. Such occlusion impedes representation of such scenes by models that have been trained on full-body pose data, obtained in laboratory conditions with specific sensors. To address this, as a first contribution, we introduce OR-VPE 49, a novel video pose embedding network that is streamlined to learn an occlusion-robust representation for pose sequences in videos. In order to enable our embedding network to handle partially visible joints, we propose to incorporate a sub-graph data augmentation mechanism during training, which simulates occlusions, into a video pose encoder based on Graph Convolutional Networks (GCNs). As a second contribution, we apply a contrastive learning module to train the video pose representation in a self-supervised manner without the necessity of action annotations. This is achieved by minimizing the mutual information of the same pose sequence pruned into different spatio-temporal sub-graphs. Experimental analyses show that compared to training the same encoder from scratch, our proposed OR-VPE, with pre-training on a large-scale dataset, NTU-RGB+D 120, improves the performance of the downstream action classification on Toyota Smarthome, N-UCLA and Penn Action datasets.
Action recognition based on skeleton data has recently witnessed increasing attention and progress. State-of-the-art approaches adopting Graph Convolutional networks (GCNs) can effectively extract features on human skeletons relying on the pre-defined human topology. Despite associated progress, GCN-based methods have difficulties to generalize across domains, especially with different human topological structures. In this context, we introduce UNIK 50, a novel skeleton-based action recognition method that is not only effective to learn spatio-temporal features on human skeleton sequences but also able to generalize across datasets. This is achieved by learning an optimal dependency matrix from the uniform distribution based on a multi-head attention mechanism. Subsequently, to study the cross-domain generalizability of skeleton-based action recognition in real-world videos, we re-evaluate state-of-the-art approaches as well as the proposed UNIK in light of a novel Posetics dataset. This dataset is created from Kinetics-400 videos by estimating, refining and filtering poses. We provide an analysis on how much performance improves on smaller benchmark datasets after pre-training on Posetics for the action classification task. Experimental results show that the proposed UNIK, with pre-training on Posetics, generalizes well and outperforms state-of-the-art when transferred onto four target action classification datasets: Toyota Smarthome, Penn Action, NTU-RGB+D 60 and NTU-RGB+D 120.
Results
The quantitative evaluation results of Work1 (see Tab. 4) demonstrate the effectiveness of the proposed OR-VPE. Results in Tab. 3 demonstrate the effectiveness of the proposed method UNIK and pre-training dataset Posetics in Work2.
Methods | Pre-training | Smarthome (J) | Penn Action (J) | *NTU-60 (J+B) | *NTU-120 (J+B) |
CS (%) CV1(%) CV2 (%) | Top-1 Acc. (%) | CS (%) CV (%) | CS (%) CSet (%) | ||
2s-AGCN | Scratch | 55.7 21.6 53.3 | 89.5 | 84.2 93.0 | 78.2 82.9 |
MS-G3D | Scratch | 55.9 17.4 56.7 | 88.5 | 86.0 94.1 | 80.2 86.1 |
UNIK (Ours) | Scratch | 58.9 21.9 58.7 | 90.1 | 85.1 93.6 | 79.1 83.5 |
2s-AGCN | Posetics | 58.8 32.2 57.9 | 96.4 | 85.8 93.4 | 79.7 85.0 |
MS-G3D | Posetics | 59.1 26.6 60.1 | 92.2 | 86.2 94.1 | 80.6 86.4 |
UNIK (Ours) | Posetics | 62.1 33.4 63.6 | 97.2 | 86.8 94.4 | 80.8 86.5 |
Methods | Toyota Smarthome | Penn Action | N-UCLA |
#Params CS(%) CV1(%) CV2(%) | #Params Top-1 Accuracy (%) | #Params CV (%) | |
Baseline (Linear classification w/o Embedding) | 7.97K 23.1 15.8 19.3 | 3.85K 28.5 | 2.57K 35.6 |
Linear classification with Self-supervised Embedding (Ours) | 7.97K 42.7 18.1 32.4 | 3.85K 78.5 | 2.57K 56.7 |
Baseline (AGCNs w/o Embedding) | 3.45M 55.7 21.6 53.3 | 3.45M 77.2 | 3.45M 78.2 |
Fine-tuned with Self-supervised Embedding (Ours) | |||
with temporal SubG-DA only | 3.45M 55.8 22.1 54.4 | 3.45M 78.8 | 3.45M 78.9 |
with spatial-temporal SubG-DA | 3.45M 56.3 24.6 59.0 | 3.45M 93.3 | 3.45M 84.5 |
Fine-tuned with Supervised Embedding | 3.45M 58.2 27.3 58.5 | 3.45M 90.7 | 3.45M 87.6 |
8.31 Activity Modeling for Medical Serious Games
Participants: Elisabetta De Maria, Thibaud L'Yvonnet, Sabine Moisan, Jean-Paul Rigault.
We study the ability of formal methods to analyze the behavior of patients during neurocognitive tests. We focus on the use of serious games for cognitive training of senior patients with minor neurocognitive disorders. Serious games offer a framework in which the expected behavior is well identified and it is possible to rely on different sensors (bio-metric and external) while playing the game. Thus, the set of human activities associated with these games is constrained compared to usual daily human activity. Therefore modeling and evaluation are feasible.
This year we continue the modeling of medical serious games using a formal approach based on Discrete Time Markov Chains (DTMCs) to model human activities of different populations playing clinical serious games. Important properties of these models can be automatically verified thanks to model checking. We modeled 3 serious games targeting different cognitive functions (episodic memoric, inhibitory control, visual attention) 22, 43. This approach should allow both a better understanding of the variety of behaviors and a better prediction of patient diagnosis based on their behavior.
In parallel, we designed and conducted a 13 months clinical protocol to collect data on the use of the selected games by patients with mild Neuro-Cognitive Disorders (mild NCD) and patients with Subjective Cognitive Disorders (SCD). We also completed a model of the inhibitory control loop in the brain, using DTMCs.
8.31.1 Clinical Experimentation Protocol
In order to validate our formal models in a clinical experimentation, we
proposed a protocol involving patients coming to the Institut Claude
Pompidou (ICP) in Nice. In this protocol, both mild neurocognitive
disorder (mild NCD) patients and subjective cognitive decline (SCD)
ones played the three serious games previously modeled.
The objective of the protocol was to collect real data to validate the hypothesis that models can
differentiate mild NCD patients apart from control subjects, based on
their behavior and performance.
The main constraint was to go through several ethical validation
processes. The CPP committee (Committee for the protection of
people) finally accepted our proposal mid May 2020.
We recruited 50 volunteers including 30 mild NCD subjects and 20 SCD ones.
The protocol lasted 13 months, with 10 months of inclusion of patients and 3 months of result analysis.
All the data (including scores, answers, and response times) as well
as video recordings (focused only on the hand gestures above the
screen, for privacy) were collected and anonymized.
This clinical protocol allowed us to compare the consistency of the
model predictions with
experimental results and to tune the formal models according to real
data. It validated the selected serious games as suitable
tools to discriminate between mild NCD patients and SCD ones.
The selected games were also able to display correlations with classical neurocognitive
(pen and paper) tests.
The collected data were analyzed with classical statistical
tools (Mann-Whitney U test,
Answers | Right answers | Wrong answers | Time | ||
3*MMSE | coeff. corr | 0.412 | 0.447 | -0.171 | -0.417 |
p-value |
|
|
|
|
|
Sample sizes | 50 | ||||
3*DSS | coeff. corr | 0.619 | 0.623 | -0.222 | -0.624 |
p-value |
|
|
|
|
|
Sample sizes | 50 |
These results were used to redesign and manually calibrate (i.e., adjust DTMC probabilities) the model of the game targeting visual attention (Code game) so that the formal model can reproduce behaviors observed in clinical conditions.
8.31.2 Inhibitory Control Model
We chose to model the inhibitory control cognitive function because it is a routinely assessed function in elder patients and a big part of its mechanisms and potential source of dysfunctions is located in a rather small region of the brain named basal ganglia. We proposed a discrete probabilistic model of the neural network governing the inhibitory control function and we studied some of its dynamic properties. We modeled the different structures implied in the inhibitory control loop thanks to a probabilistic discrete Markov chain. To implement this model we proposed a new generalization of the LI&F third generation artificial neuron, that we named neuron box, allowing to observe rather complex behaviors. The choice of boxes to represent basal ganglia structures makes it possible to have a behavior relatively close to a small network of neurons, without requiring a lot of computing power. The model was able to reproduce known biological behaviors from the literature and to state the importance of some known brain connections. We also adapted this model to represent a behavior compliant with Parkinson disease.
As future work, we have to redesign and calibrate the Inhibitory Control game model similarly to the Code game one to better match the observed results. Once this calibration done, the representation of the relationships between the basal ganglia model and the game model will be possible. To do so, a new model has to be created to merge the calibrated game model and the basal ganglia one.
8.32 ALCOTRA E-Santé Silver Economy Project
Participants: Rachid Guerchouche, Monique Thonnat, Alexandra König, François Brémond.
Inria (via STARS team) was involved in the E-Santé Silver Economy project (2019-2022) as delegate of the Métropole Nice Côte d’Azur. E-Santé Silver Economy is a France-Italy cross-border project, which aims to bring together players in the health and medico-social sectors, senior-users and companies offering solutions to prevent autonomy-loss and promote home-care in a sustainable manner. Inria is involved in the different phases of the project, starting from elderly needs analysis through Focus Groups, to technology experimentations within livings-Labs, and ending by experimentations “in the filed” through a clinical study in Nice and Digne-les-Bains regions. In 2021, the main work concerned the Focus Groups and the Living-Labs. Five Focus Groups (3 in France and 2 in Italy were organized) to study the seniors needs in both ALCOTRA regions. Two Living-Labs were then organized, one in Nice and the other in Digne-les-Bains. In addition to understanding the global needs of elderly population, one of the main interests of the STARS team was to verify whether the technologies developed by the team respond to the expressed needs. From the results of Focus Groups, loss of autonomy is expressed as one of the main fears of elderly, especially when this leads to leave their home and be obliged to go into nursing-homes or specialized clinics. STARS through its research work is particularly interested in the activity recognition, and one of its applications is Activity Daily Living (ADL) monitoring applied to elderly in order to delay their loss of autonomy and prevent physical and cognitive declines. From the results of Living-Labs, two technologies from STARS were demonstrated: SUP for camera based tele-surveillance and activity monitoring and the telemedicine tool for cognitive disorders diagnostic and screening developed in the context of DeepSpA project (2019-2020). During the Living-Labs, we asked the participants (around 25) about their opinion about many technologies, including the two STARS technologies. For both technologies (SUP and telemedicine), almost all the subjects expressed their interest, and found a utility to use both systems. Some concerns were expressed regarding the cost of the technologies and about some privacy issues. Inria organized the first peer-review of the project regarding the Focus-Groups, a second peer-review is expected to be organized in 2022 about the Living-Labs. 2022 will be mainly dedicated to the experimentations “in the field” through a clinical study.
8.33 MePheSTO – Digital Phenotyping for Psychiatric Disorders from Social Interaction
Participants: Alexandra König, Tanay Agrawal, François Brémond.
This work is part of the Inria-DFKI joint project “MePheSTO – Digital Phenotyping for Psychiatric Disorders from Social Interaction”. MePheSTO is an interdisciplinary research project that aims to develop a methodology based on artificial intelligence methods for the identification and classification of objective, and thus measurable, digital phenotypes of psychiatric disorders. MePheSTO has a solid foundation of clinically motivated scenarios and use-cases (four in total) synthesized jointly with clinical partners. Important to MePheSTO is the creation of a multimodal corpus including speech, video, and biosensors of social patient-clinician interactions, which serves as the basis for deriving methods, models and knowledge on psychiatric symptoms. A set of novel multimodal digital biomarkers derived from the interaction data will be identified and formalized derived from the interaction data corpus allowing reliable phenotyping of the target psychiatric disorders.
Methods/models for the extraction of those biomarkers from multimodal data will be developed allowing the forecasting of patient’s status (e.g. relapse prediction). Feasibility of computer-supported diagnostics and patient monitoring for the set of target psychiatric disorders will be demonstrated within the defined use cases (e.g. face-to-face interview with real-time feedback, telemedical interview, etc.). The approach and developed methods will be validated in at least 2 countries/languages (France and Germany). Important project outcomes include technical tools 18 and organizational methods for the management of medical data that implement both ELSI and GDPR requirements, demonstration scenarios covering patients' journeys including early detection, diagnosis support, relapse prediction, therapy support, an annotated corpus, Ph.D. theses, and publications. A foundation for continuity of the cooperation should be assured through developing and submitting new competitive research and innovation project proposals. MePheSTO builds a joint DFKI-Inria workforce – the foundation for future R&D and innovation projects.
9 Bilateral contracts and grants with industry
Stars team has currently several experiences in technological transfer towards industries, which have permitted to exploit research result.
9.1 Bilateral contracts with industry
9.1.1 Toyota
Toyota is working with Stars on action recognition software to be integrated on their robot platform. This project aims at detecting critical situations in the daily life of older adults alone at home. This will require not only recognition of ADLs but also an evaluation of the way and timing in which they are being carried out. The system we want to develop is intended to help them and their relatives to feel more comfortable because they know that potential dangerous situations will be detected and reported to caregivers if necessary. The system is intended to work with a Partner Robot - HSR - (to send real-time information to the robot) to better interact with the older adult.
9.1.2 Thales
Thales and Inria jointly explore facial analysis in the invisible spectrum. Among the different spectra low energy infrared waves, as well as ultraviolet waves will be studied. In this context following tasks will be included: 1. We are designing a model to extract biometric features from the acquired data. Analysis of the data related to contours, shape, etc. will be performed. Current methodology cannot be adopted, since colorimetry in the invisible spectrum is more restricted with less diffuse variations and is less nuanced. Then facial recognition will be performed in the invisible spectrum. Expected challenges have to do with limited colorimetry and lower contrasts. In addition to the first milestone (face recognition in the invisible spectrum), there are two other major milestones: 2. Implementation of such a face recognition system, to be tested at the passage of the access portal to a school. 3. Pseudo-anonymized identification within a school (outdoor courtyards, interior buildings). Combining biometrics in the invisible spectra and anonymisation within an established group requires removing certain additional barriers that are specific to biometrics but also the use of statistical methods associated with biometrics. This pseudo-anonymized identification must also incorporate elements of information provided by the proposed electronic school IDs.
9.1.3 Kontron
Kontron has a collaboration with Stars, which runs from April 2018 until July 2021 to embed CNN based people tracker within a video-camera. Their system uses Intel VPU modules, such as Myriad X (MA2485), based on OpenVino library.
9.1.4 European System Integration
The company ESI (European System Integration) has a collaboration with Stars, which runs from September 2018 until March 2022 to develop a novel Re-Identification algorithm which can be easily set-up with low interaction for videosurveillance applications. ESI provides software solutions for remote monitoring stations, remote assistance, video surveillance, and call centers. It was created in 1999 and ESI is a leader in the French remote monitoring market. Nowadays, ensuring the safety of goods and people is a major problem. For this reason, surveillance technologies are attracting growing interest and their objectives are constantly evolving: it is now a question of automating surveillance systems and helping video surveillance operators in order to limit interventions and staff. One of the current difficulties is the human processing of video, as the multiplication of video streams makes it difficult to understand meaningful events. It is therefore necessary to give video surveillance operators suitable tools to assist them with tasks that can be automated. The integration of video analytics modules will allow surveillance technologies to gain in efficiency and precision. In recent times, deep learning techniques have been made possible by the advent of GPU processors, which offer significant processing possibilities. This leads to the development of automatic video processing.
9.1.5 Fantastic Sourcing
Fantastic Sourcing is a French SME specialized in micro-electronics, it develops e-health technologies. Fantastic Sourcing is
collaborating with Stars through the UCA Solitaria project, by providing their Nodeus system. Nodeus is a
IoT (Internet of Things) system for home support for the elderly, which consists of a set of small sensors
(without video cameras) to collect precious data on the habits of isolated people. Solitaria project performs
a multi-sensor activity analysis for monitoring and safety of older and isolated people. With the increase
of the ageing population in Europe and in the rest of the world, keeping elderly people at home, in their
usual environment, as long as possible, becomes a priority and a challenge of modern society. A system for
monitoring activities and alerting in case of danger, in permanent connection with a device (an application on a
phone, a surveillance system ...) to warn relatives (family, neighbours, friends ...) of isolated people still living
in their natural environment could save lives and avoid incidents that cause or worsen the loss of autonomy. In
this R
9.1.6 Nively - WITA SRL
Nively is a French SME specialized in e-health technologies, it develops position and activity monitoring of activities of daily living platforms based on video technology. Nively’s mission is to use technological tools to put people back at the center of their interests, with their emotions, identity and behavior. Nively is collaborating with Stars through the UCA Solitaria project, by providing their MentorAge system. This software allows the monitoring of elderly people in nursing homes in order to detect all the abnormal events in the lives of residents (falls, runaways, strolls, etc.). Nively’s technology is based on RGBD video sensors (Kinects type) and a software platform for event detection and data visualization. Nively is also in charge of Software distribution for the ANR Activis project. This project is based on an objective quantification of the atypical behaviors on which the diagnosis of autism is based, with medical (diagnostic assistance and evaluation of therapeutic programs) and computer scientific (by allowing a more objective description of atypical behaviors in autism) objectives. This quantification requires video analysis of the behavior of people with autism. In particular, we propose to explore the issues related to the analysis of ocular movement, gestures and posture to characterize the behavior of a child with autism. Thus, Nively will add autistic behavior analysis software to its product range.
9.1.7 ARECO
ARECO is a French SME specialized in the field of nebulization and misting technologies. It manufactures and sells nebulization devices and dynamic display processes. In this study, an algorithm will be designed for the analysis of customer behaviors when approaching the Fruits & Vegetables department. The analysis should be done on an offline video tape in the evening to store the analyzed interactions. The algorithm will be able to extract the recognized actions and the corresponding time information from a video and store them in a separate file.
9.2 Bilateral grants with industry
9.2.1 LiChIE Project
The LiChIE project (Lion Chaine Image Elargie) is conducted in collaboration with AirBus and BPI to found nine topics including six on the theme of In-flight imagery and three on the robotics theme for the assembly of satellites. The two topics involving STARS are :
- Mohammed Guermal's PhD thesis on Visual Understanding of Activities for an improved collaboration between humans and robots. He began on December 1, 2020.
- Farhood Negin post-doctoral studies on detection and tracking of vehicles from satellite videos and abnormal activity detection. He started in Oct 2020 for 2 years.
10 Partnerships and cooperations
10.1 European initiatives
10.1.1 FP7 and H2020 Projects
BIM2TWIN
-
Title:
BIM2TWIN: Optimal Construction Management
-
Duration:
November 2020 - November 2024
-
Coordinator:
CSTB
-
Partners:
Centre Scientifique Et Technique Du Batiment; Technion - Israel Institute Of Technology; The Chancellor Masters and Scholars of The University Of Cambridge; Technische Universitaet Muenchen.
-
Inria contact:
Pierre Alliez and Francois Bremond
-
Summary:
BIM2TWIN aims to build a Digital Building Twin (DBT) platform for construction management that implements lean principles to reduce operational waste of all kinds, shortening schedules, reducing costs, enhancing quality and safety and reducing carbon footprint. BIM2TWIN proposes a comprehensive, holistic approach. It consists of a (DBT) platform that provides full situational awareness and an extensible set of construction management applications. It supports a closed loop Plan-Do-Check-Act mode of construction. Its key features are:
- Grounded conceptual analysis of data, information and knowledge in the context of DBTs, which underpins a robust system architecture;
- A common platform for data acquisition and complex event processing to interpret multiple monitored data streams from construction site and supply chain to establish real-time project status in a Project Status Model (PSM);
- Exposure of the PSM to a suite of construction management applications through an easily accessible application programming interface (API) and directly to users through a visual information dashboard.]
Applications include monitoring of schedule, quantities, budget, quality, safety, and environmental impact. PSM representation based on property graph semanticaly linked to the Building Information Model (BIM) and all project management data. The property graph enables flexible, scalable storage of raw monitoring data in different formats, as well as storage of interpreted information. It enables smooth transition from construction to operation.
BIM2TWIN is a broad, multidisciplinary consortium with hand-picked partners who together provide an optimal combination of knowledge, expertise and experience in a variety of monitoring technologies, artificial intelligence, computer vision, information schema and graph databases, construction management, equipment automation and occupational safety. The DBT platform will be experimented on 3 demo sites (SP, FR, FI).
HEROES
-
Title:
Novel Strategies to Fight Child Sexual Exploitation and Human Trafficking Crimes and Protect their Victims.
-
Duration:
December 2021 - November 2024
-
Coordinator:
Universidad Complutense de Madrid
-
Partners:
Universidade de Brasília - UnB, International Center for Missing and Exploited Children, Secretaria de Inteligencia Estratégica de Estado - Presidencia
-
Inria contact:
François Brémond
-
Summary:
Trafficking of human beings (THB) and child sexual abuse and exploitation (CSA/CSE) are two big problems in our society. Inadvertently, new information and communication technologies (ICTs) have provided a space for these problems to develop and take new forms, made worse by the lockdown caused by the COVID-19 pandemic. At the same time, technical and legal tools available to stakeholders that prevent, investigate, and assist victims – such as law enforcement agencies (LEAs), prosecutors, judges, and civil society organisations (CSOs) – fail to keep up with the pace at which criminals use new technologies to continue their abhorrent acts. Furthermore, assistance to victims of THB and CSA/CSE is often limited by the lack of coordination among these stakeholders. In this sense, there is a clear and vital need for joint work methodologies and the development of new strategies for approaching and assisting victims. In addition, due to the cross-border nature of these crimes, harmonisation of legal frameworks from each of the affected countries is necessary for creating bridges of communication and coordination among all those stakeholders to help victims and reduce the occurrence of these horrendous crimes. To address these challenges, the HEROES project comes up with an ambitious, interdisciplinary, international, and victim-centred approach. The HEROES project is structured as a comprehensive solution that encompasses three main components: Prevention, Investigation and Victim Assistance. Through these components, our solution aims to establish a coordinated contribution with LEAs by developing an appropriate, victim-centred approach that is capable of addressing specific needs and providing protection. The HEROES project’s main objective is to use technology to improve the way in which help and support can be provided to victims of THB and CSA/CSE.
10.2 Collaborations in European programs, except FP7 and H2020
10.2.1 MePheSTO
Title:
MePheSTO: Digital Phenotyping 4 Psychiatric Disorders from Social Interaction
Duration:
September 2020 - August 2023
Coordinator:
Inria-DFKI joint project
Partners:
François Brémond (Inria-STARS team), Maxime Amblard (Inria-SEMAGRAMME team), Jan Alexandersson (DFKI-COS, Saarbruecken), Johannes Tröger (DFKI-COS, Saarbruecken).
Inria contact:
Maxime Amblard and Francois Bremond
Summary:
MePheSTO is an interdisciplinary research project that envisions a scientifically sound methodology based on artificial intelligence methods for the identification and classification of objective, and thus measurable, digital phenotypes of psychiatric disorders. MePheSTO has a solid foundation of clinically motivated scenarios and use-cases synthesized jointly with clinical partners. Important to MePheSTO is the creation of a multimodal corpus including speech, video, and biosensors of social patient-clinician interactions, which serves as the basis for deriving methods, models and knowledge. Important project outcomes include technical tools and organizational methods for the management of medical data that implement both ELSI and GDPR requirements, demonstration scenarios covering patients journeys including early detection, diagnosis support, relapse prediction, therapy support, an annotated corpus, Ph.D. theses, and publications. MePheSTO builds a joint DFKI-Inria workforce the foundation for future R D and innovation projects.
10.2.2 DeepSpa
Title:
DeepSpa: Deep Speech Analysis
Duration:
January 2019 - June 2021.
Coordinator:
Inria
Partners:
Inria: technical partner and project coordinator, University of Maastricht: clinical partner, Jansen and Jansen: pharma partner and business champion, Association Innovation Alzheimer: subgranted clinical partner, Ki-element: subgranted technical partner.
Inria contact:
Alexandra König (STARS)
Summary:
The DeepSpa is a EIT Health project, which aims to deliver telecommunication based neurocognitive assessment tools for early screening, early diagnostic and follow-up of cognitive disorders, mainly in elderly. The target is also clinical trials addressing Alzheimer’s and other neurodegenerative diseases. By combining AI in speech recognition and video analysis for facial expression recognition, the proposed tools allow remote cognitive and psychological testing, thereby saving time and money.
10.2.3 E-Santé Silver Economy - Alcotra
Title:
E-Santé
Duration:
February 2020 - June 2022.
Coordinator:
Nice Metropole
Partners:
Nice Metropole; Inria (Stars); CoBTek; Nice hospital; University of Genova; University of Torino; Liguria Region; Liguria Digitale; Provence Alpes Agglomération; University of Côte d'Azur.
Inria contact:
François Brémond (STARS)
Summary:
E-Santé Silver Economy is a Alcotra project, which performs a multi-sensor activity analysis for the monitoring and safety of older and isolated people. The E-Health (E-Santé in French and E-Sanità in Italian) / Silver Economy project is a collaborative project within the framework of the European cross-border cooperation program between France and Italy Interreg ALCOTRA. The aim is to increase innovation projects (in particular clusters, poles and companies) - and to develop innovative services at cross-border level. The E-Health / Silver Economy project tackles the problems of frailty among elderly, more particularly in rural and isolated areas; as well as access to innovations for the ALCOTRA regions, where there is an imbalance in terms of innovation and access to public services between urban and rural areas. The majority of the population, services and economic activities are concentrated in cities. The aims of the project are therefore: to experiment innovative e-health tools and to increase the accessibility of isolated people to care (screening, diagnosis and follow-up); in order to keep elderly people at their own houses as long as possible, by proposing solutions to delay the decrease in their mental, cognitive and physical capacities.
10.3 National initiatives
10.3.1 ANR
ENVISION
:
Title:
ENVISION: Computer Vision for automated holistic analysis of humans
Duration:
2017 - 2021.
Coordinator:
Inria
Inria contact:
Antitza Dantcheva
Summary:
The main objective of ENVISION is to develop the computer vision and theoretical foundations of efficient biometric systems that analyze appearance and dynamics of both face and body, towards recognition of identity, gender, age, as well as mental and social states of humans in the presence of operational randomness and data uncertainty. Such dynamics - which will include facial expressions, visual focus of attention, hand and body movement, and others, constitute a new class of tools that have the potential to allow for successful holistic analysis of humans, beneficial in two key settings: (a) biometric identification in the presence of difficult operational settings that cause traditional traits to fail, (b) early detection of frailty symptoms for health care.
10.3.2 RESPECT
Title:
RESPECT: Computer Vision for automated holistic analysis of humans
Duration:
2018 - 2022.
Coordinator:
Hochschule Darmstadt
Partners:
Inria, Hochschule Darmstadt, EURECOM.
Inria contact:
Antitza Dantcheva
Summary:
In spite of the numerous advantages of biometric recognition systems over traditional authentication systems based on PINs or passwords, these systems are vulnerable to external attacks and can leak data. Presentations attacks (PAs) – impostors who manipulate biometric samples to masquerade as other people – pose serious threats to security. Privacy concerns involve the use of personal and sensitive biometric information, as classified by the GDPR, for purposes other than those intended. Multi-biometric systems, explored extensively as a means of improving recognition reliability, also offer potential to improve PA detection (PAD) generalisation. Multi-biometric systems offer natural protection against spoofing since an impostor is less likely to succeed in fooling multiple systems simultaneously. For the same reason, previously unseen PAs are less likely to fool multi-biometric systems protected by PAD. RESPECT, a Franco-German collaborative project, explores the potential of using multi-biometrics as a means to defend against diverse PAs and improve generalisation while still preserving privacy. Central to this idea is the use of (i) biometric characteristics that can be captured easily and reliably using ubiquitous smart devices and, (ii) biometric characteristics which facilitate computationally manageable privacy preserving, homomorphic encryption. The research focuses on characteristics readily captured with consumer-grade microphones and video cameras, specifically face, iris and voice. Further advances beyond the current state of the art involve the consideration of dynamic characteristics, namely utterance verification and lip dynamics. The core research objective is to determine which combination of biometrics characteristics gives the best biometric authentication reliability and PAD generalisation while remaining compatible with computationally efficient privacy preserving biometric template protection schemes.
10.3.3 ACTIVIS
Title:
ACTIVIS: Video-based analysis of autism behavior
Duration:
2020 - 2023.
Coordinator:
Aix-Marseille Université - LIS
Partners:
Inria, Aix-Marseille Université - LIS, Hôpitaux Pédiatriques Nice CHU-Lenval - CoBTeK, Nively
Inria contact:
François Brémond
Summary:
The ACTIVIS project is an ANR project (CES19: Technologies pour la santé) started in January 2020 and will end in December 2023 (48 months). This project is based on an objective quantification of the atypical behaviors on which the diagnosis of autism is based, with medical (diagnostic assistance and evaluation of therapeutic programs) and computer scientific (by allowing a more objective description of atypical behaviors in autism) objectives. This quantification requires video analysis of the behavior of people with autism. In particular, we propose to explore the issues related to the analysis of ocular movement, gestures and posture to characterize the behavior of a child with autism.
10.4 Regional initiatives
10.4.1 FairVision - video monitoring for soccer games
Title:
FairVision
Duration:
September 2021 - January 2022.
Coordinator:
Inria
Partners:
Inria (Stars): technical partner and project coordinator; FairVision.
Inria contact:
François Brémond (STARS)
Summary:
In this project, we were collaborating with the start-up company Fair Vision, which focuses on monitoring amateur soccer matches. The topic involved mastering of player and ball tracking in soccer videos. We were given input videos from the company, which included stadium recordings of soccer matches, as well as corresponding annotation files with detections of the players and the ball per each frame. We approached ball tracking as a single object tracking (SOT) problem.For this, we tested visual trackers and prepared an adapted version of the CSRT tracker (Discri-minative Correlation Filter Tracker with Channel and Spatial Reliability) to enhance tracking of the ball, especially when ball detections were missing. Player tracking was approached as multi object tracking (MOT) problem. We tried several state-of-the-art MOT algorithms, e.g., FairMOT, TransTrack, ByteTrack, through applying them on the given input videos. After studying and analyzing the algorithm limitations, we started developing new version of the ByteTrack algorithm,aiming for the reduction of identity switches and enhancement of long term tracking. This work is continued in 2022.
10.4.2 MASCOT - Machine-learning et analyse des mouvements collectifs chez les trichogrammes
Title:
MASCOT
Duration:
March 2021 - September 2021.
Coordinator:
Idex UCA
Partners:
INRAE: technical partner and project coordinator; Inria (Stars).
Inria contact:
François Brémond (STARS)
Summary:
The smallest insects in the world (<0,5mm), barely visible by eye, are sometimes qualified as “intelligent dust”. This diverse group of insects mostly contains egg parasitoids, i.e. parasitic species that lay their eggs inside the eggs of other insect species. They complete development inside the host egg, causing its death. They thus have great applied interest in agriculture: many are biocontrol agents (BCAs), that are released to protect crops from pest attacks. They also have high scientific interest, qualifying as the smallest insects in the world, featuring extreme morphological and neurological miniaturization. One of the best-known examples is insects in genus Trichogramma, produced and used at industrial scale as an alternative to chemicals in different cropping systems, from maize fields to tomato-producing greenhouses. Institut Sophia Agrobiotech has a long expertise in the study of BCAs in general and Trichogramma in particular. In recent years, the experimental study of behavior and movement of insects in the laboratory has greatly benefited from the development of video-analysis and automated video-tracking techniques, in particular Multi-Object Tracking (MOT). This opens new perspectives for the quantitative phenotypic characterization of biocontrol agents. The Unit is presently setting-up a new facility and an experimental platform dedicated to these approaches. This project consists in using Artificial Intelligence to improve the performance of more classical tracking technologies. A major shortcoming of current tracking methods is to correctly identify individuals while they are interacting in a group (identity preservation), even without the use of marking techniques. This lack prevents the study of individual differences and personalities in realistic group contexts. AI methods enable us to lift this shortcoming, however no tool is yet available to the biological community that would allow experimenters to benefit from these technologies. To make this happen, we have initiated this year a new collaboration to automatically analyse video scenes of Trichogramma.
11 Dissemination
11.1 Promoting scientific activities
11.1.1 Scientific events: organisation
Member of the organizing committees
- François Brémond served in the organizing committee of ICIAP’21, to be organized in Lecce in 2022 by the Italian Association for Research in Computer Vision, Pattern Recognition and Machine Learning.
- Antitza Dantcheva co-organized the Special Session on “Applications in Healthcare and Health Monitoring” at the IEEE Conference on Automatic Face and Gesture Recognition (FG), December 2021.
- Antitza Dantcheva co-organized the 2nd Remote Physiological Signal Sensing (RePSS) challenge/workshop in conjunction with ICCV'21.
11.1.2 Scientific events: selection
- Antitza Dantcheva defended her HDR entitled "Computer Vision for deciphering and generating faces" on 10/09/2021.
Member of the conference program committees
- Monique Thonnat was senior program committee member of the conference IJCAI 2021.
- Monique Thonnat was program committee member of the conference ICPRAM 2022.
Reviewer
- François Brémond was a reviewer for AVSS 2021, MAPR 2021, JdCHE 2020-21, VJCS 2021, ICCV 2021, CVPR 2021&2022, NeurIPS 2021, ICLR 2022, WACV 2022.
11.1.3 Journal
Member of the editorial boards
- François Brémond was an editorial boards member of the Journal, Frontiers in Computer Science.
Reviewer - reviewing activities
- François Brémond was a reviewer for Medical Image Analysis Journal and IEEE Access Journal.
11.1.4 Invited talks
- François Brémond gave lectures at thematic schools of Computer Vision (2h): PhD summer school in AI: part of the fourth edition of the International Summer School On Artificial Intelligence: from Deep Learning to Data Analytics in Udine – Italy from June 28th to July 2nd 2021.
Scientific expertise
- Monique Thonnat was member of the recruitment of Assistant/Associate Professor in Deep Learning for Computer Vision Telecom Paris June 2021.
- Monique Thonnat is member of the scientific board of ENPC, Ecole Nationale des Ponts et Chaussées since June 2008.
11.2 Teaching - Supervision - Juries
11.2.1 Teaching
- François Brémond organized and lectured the Master MSc Data Science and Artificial Intelligence (Computer Vision and Deep Learning) 30h class at Université Côte d'Azur in 2020 and 2021. Web-site
- Antitza Dantcheva taught 2 classes at Polytech Nice Sophia - Univ Côte d'Azur (Applied Artificial Intelligence, Master 2) in Oct.2021.
- Rui Dai taught two lectures for MSc. Data Science and Artificial Intelligence, UCA.
- Farhood Negin taught one lecture for MSc. Data Science and Artificial Intelligence, UCA.
- Hao Chen taught two lectures for MSc. Data Science and Artificial Intelligence, UCA.
- Valeriya Strizhkova taught one lecture for MSc. Data Science and Artificial Intelligence, UCA and one research project for DSAI.
11.2.2 Supervision
- Monique Thonnat was Phd supervisor of Jen-Cheng Hou "Quantified Analysis for Seizure Videos", defended on 13rd of December 2021.
- Antitza Dantcheva and François Brémond were PhD supervisors of Yaohui Wang "Learning to Generate Human Videos", defended on 30th of September 2021.
11.2.3 Juries
François Brémond was a jury member for the Mi-term PhD defense of
- Anam Zahra, Max Planck Institute for Evolutionary Anthropology, 24 March 2021
- Florent Jousse, Université Côte d’Azur, 18 May 2021
François Brémond was a jury member for the PhD defense of
- Renato Baptista, Université Du Luxembourg, 13th January 2021
- Claire Labit Bonis, Univ. Paul Sabatier, 21 June 2021
- Lucas Pascal, Eurecom, 8 November 2021
- Melissa Sanabria, Université Côte d’Azur, 3 December 2021
Monique Thonnat was reviewer for the HDR defense of Valeria Manera in Neurosciences at UCA, 10th of June 2021.
François Brémond was a jury member for the HDR defense of
- Maria Zuluaga, Eurecom, 19 March 2021
- Lionel Robinault, Université Lumière Lyon 2, 9 June 2021
- Valeria Manera, Université Côte d’Azur, 10 June 2021
- Carlo Bertoncelli, Université Côte d’Azur, 21 October 2021
11.3 Popularization
Articles and contents
- Antitza Dantcheva was interviewed on the topic of deepfakes for the Data Analytics Post, see article.
- François Brémond was interviewed on the topic of Activity Recognition for the Data Analytics Post, see article.
Education
Interventions
- Monique Thonnat and Antitza Dantcheva participated in a meeting with the Conseil National du Numérique 14/12/2021.
- François Brémond gave a Conference on "Troubles du spectre de l’autisme : les projets de recherche liés à l’autisme " - Intranet Inria.
- Antitza Dantcheva gave a talk on "Generating and Deciphering Faces" at In'Tro Inria on 31/05/21 YouTube Link.
- François Brémond gave a presentation at Inria-JST Meeting, at a meeting with Allistene, at a workshop IA et Santé at a meeting with Caisse des Dépôts et Consignations.
12 Scientific production
12.1 Major publications
- 1 inproceedingsLearning to match appearances by correlations in a covariance metric space.European Conference on Computer VisionSpringer2012, 806--820
- 2 articleExploiting Feature Correlations by Brownian Statistics for People Detection and Recognition.IEEE transactions on systems, man, and cybernetics2016
- 3 inproceedingsVideo Covariance Matrix Logarithm for Human Action Recognition in Videos.IJCAI 2015 - 24th International Joint Conference on Artificial Intelligence (IJCAI)Buenos Aires, ArgentinaJuly 2015
- 4 articleSemantic Event Fusion of Different Visual Modality Concepts for Activity Recognition.IEEE Transactions on Pattern Analysis and Machine Intelligence382016, 1598 - 1611
- 5 articleGender estimation based on smile-dynamics.IEEE Transactions on Information Forensics and Security2016, 11
- 6 inproceedingsToyota Smarthome: Real-World Activities of Daily Living.ICCV 2019 -17th International Conference on Computer VisionSeoul, South KoreaOctober 2019
- 7 inproceedingsVPN: Learning Video-Pose Embedding for Activities of Daily Living.ECCV 2020 - 16th European Conference on Computer VisionGlasgow (Virtual), United KingdomAugust 2020
- 8 inproceedingsGesture Recognition by Learning Local Motion Signatures.CVPR 2010 : IEEE Conference on Computer Vision and Pattern RecognitionSan Franscico, CA, United StatesIEEE Computer Society PressJune 2010
- 9 articleRecognizing Gestures by Learning Local Motion Signatures of HOG Descriptors.IEEE Transactions on Pattern Analysis and Machine Intelligence2012
- 10 inproceedingsKnowledge Representation for Program Reuse.European Conference on Artificial Intelligence (ECAI)Lyon, FranceJuly 2002, 240-244
- 11 articleBlocks, a Component Framework with Checking Facilities for Knowledge-Based Systems.Informatica, Special Issue on Component Based Software Development254November 2001, 501-507
- 12 articleCompilation Modulaire d'un Langage Synchrone.Revue des sciences et technologies de l'information, série Théorie et Science Informatique430June 2011, 441-471URL: http://hal.inria.fr/inria-00524499/en
- 13 article What Can Program Supervision Do for Software Re-use? IEE Proceedings - Software Special Issue on Knowledge Modelling for Software Components Reuse 147 5 2000
- 14 inproceedingsAutomatic Video Interpretation: A Novel Algorithm based for Temporal Scenario Recognition.The Eighteenth International Joint Conference on Artificial Intelligence (IJCAI'03)Acapulco, Mexico9-15 September 2003
- 15 inproceedingsG3AN: Disentangling Appearance and Motion for Video Generation.CVPR 2020 - IEEE Conference on Computer Vision and Pattern RecognitionSeattle / Virtual, United StatesJune 2020
12.2 Publications of the year
International journals
- 16 articleHigh-order sliding mode control of a doubly salient permanent magnet machine driving marine current turbine.Journal of Ocean Engineering and Science61March 2021, 12-20
- 17 articleVPN++: Rethinking Video-Pose embeddings for understanding Activities of Daily Living.IEEE Transactions on Pattern Analysis and Machine IntelligenceDecember 2021
- 18 articleMultimodal MRI cerebral correlates of verbal fluency switching and its impairment in women with depression.Neuroimage-Clinical332022, 1-11
- 19 articleCapacitive Coupling of Conducting Polymer Tattoo Electrodes with the Skin.Advanced Materials Interfaces815July 2021, 2100352
- 20 articleMeasuring neuropsychiatric symptoms in patients with early cognitive decline using speech analysis.European Psychiatry6412021
- 21 articleMeasuring Stress in Health Professionals Over the Phone Using Automatic Speech Analysis During the COVID-19 Pandemic: Observational Pilot Study.Journal of Medical Internet Research2342021, e24191
- 22 articleProbabilistic Model Checking for Human Activity Recognition in Medical Serious Games.Science of Computer Programming206June 2021, 102629
- 23 articleLanguage Impairment in Alzheimer’s Disease—Robust and Explainable Evidence for AD-Related Deterioration of Spontaneous Speech Through Multilingual Machine Learning.Frontiers in Aging Neuroscience1311May 2021, 6108
- 24 articlePilot Study to Assess the Feasibility of a Mobile Unit for Remote Cognitive Screening of Isolated Elderly in Rural Areas.International Journal of Environmental Research and Public Health1811June 2021, 6108
International peer-reviewed conferences
- 25 inproceedingsFrom Multimodal to Unimodal Attention in Transformers using Knowledge Distillation.AVSS 2021 - 17th IEEE International Conference on Advanced Video and Signal-based SurveillanceVirtual, United StatesNovember 2021
- 26 inproceedingsMultimodal Personality Recognition using Cross-Attention Transformer and Behaviour Encoding.VISAPP 2022 - 17th International Conference on Computer Vision Theory and ApplicationsVirtual, FranceFebruary 2022
- 27 inproceedingsVideo-based Behavior Understanding of Children for Objective Diagnosis of Autism.VISAPP 2022 - 17th International Conference on Computer Vision Theory and ApplicationsOnline, FranceFebruary 2022
- 28 inproceedingsExplainable Thermal to Visible Face Recognition Using Latent-Guided Generative Adversarial Network.FG 2021 - IEEE International Conference on Automatic Face and Gesture RecognitionJodhpur, IndiaDecember 2021
- 29 inproceedingsHow Unique Is a Face: An Investigative Study.ICPR 2020 - 25th International Conference on Pattern RecognitionMilan / Virtual, ItalyJanuary 2021
- 30 inproceedingsEnhancing Diversity in Teacher-Student Networks via Asymmetric branches for Unsupervised Person Re-identification.WACV 2021 – IEEE Winter Conference on Applications of Computer VisionWaikoloa / Virtual, United StatesJanuary 2021
- 31 inproceedingsICE: Inter-instance Contrastive Encoding for Unsupervised Person Re-identification.IEEE/CVF International Conference on Computer Vision (ICCV)Virtual, CanadaOctober 2021
- 32 inproceedingsJoint Generative and Contrastive Learning for Unsupervised Person Re-identification.IEEE/CVF Conference on Computer Vision and Pattern Recognition (CVPR)Virtual, United StatesJune 2021
- 33 inproceedingsCTRN: Class Temporal Relational Network For Action Detection.BMVC 2021 - The British Machine Vision ConferenceVirtual, United KingdomNovember 2021
- 34 inproceedingsLearning an Augmented RGB Representation with Cross-Modal Knowledge Distillation for Action Detection.ICCV 2021 - IEEE/CVF International Conference on Computer VisionMontreal, CanadaOctober 2021
- 35 inproceedingsPDAN: Pyramid Dilated Attention Network for Action Detection.WACV 2021 - Winter Conference on Applications of Computer Vision 2021Waikoloa / Virtual, United StatesJanuary 2021
- 36 inproceedingsDemystifying Attention Mechanisms for Deepfake Detection.FG 2021 - IEEE International Conference on Automatic Face and Gesture Recognitionvirtual, IndiaDecember 2021
- 37 inproceedingsBVPNet: Video-to-BVP Signal Prediction for Remote Heart Rate Estimation.FG 2021 - IEEE International Conference on Automatic Face and Gesture RecognitionJodhpur (virtual), IndiaDecember 2021
- 38 inproceedingsOne-class autoencoder approach for optimal electrode set-up identification in wearable EEG event monitoring.EMBC 2021 - 43rd Annual International Conference of the IEEE Engineering in Medicine and Biology SocietyVirtuel, FranceOctober 2021
- 39 inproceedingsDeTracker: A Joint Detection and Tracking Framework.VISAPP 2022 - 17th International Conference on Computer Vision Theory and Applicationsonline, FranceFebruary 2022
- 40 inproceedingsA Multi-Stream Approach for Seizure Classification with Knowledge Distillation.AVSS 2021 - 17th IEEE International Conference on Advanced Video and Signal-based SurveillanceVirtual, United StatesNovember 2021
- 41 inproceedingsData Uncertainty Guided Noise-aware Preprocessing Of Fingerprints.IJCNN 2021 - International Joint Conference on Neural Networks2021 International Joint Conference on Neural Networks (IJCNN)Shenzhen (online), ChinaJuly 2021
- 42 inproceedingsSensor-invariant Fingerprint ROI Segmentation Using Recurrent Adversarial Learning.IJCNN 2021 - International Joint Conference on Neural NetworksVIRTUAL, ChinaJuly 2021
- 43 inproceedingsProbabilistic Model Checking for Activity Recognition in Medical Serious Games.SEH 2021 - 3rd ICSE Workshop on Software Engineering for HealthcareMadrid, SpainJune 2021
- 44 inproceedingsDAM : Dissimilarity Attention Module for Weakly-supervised Video Anomaly Detection.AVSS 2021 - 17th IEEE International Conference on Advanced Video and Signal-based Surveillanceonline, United StatesNovember 2021
- 45 inproceedingsWeakly-supervised Joint Anomaly Detection and Classification.FG 2021 - IEEE International Conference on Automatic Face and Gesture RecognitionJodhpur, IndiaDecember 2021
- 46 inproceedingsFLAME: Facial Landmark Heatmap Activated Multimodal Gaze Estimation.AVSS 2021 - 17th IEEE International Conference on Advanced Video and Signal-based SurveillanceVirtual, United StatesNovember 2021
- 47 inproceedingsEmotion Editing in Head Reenactment Videos using Latent Space Manipulation.2021 16th IEEE International Conference on Automatic Face and Gesture Recognition (FG 2021)FG 2021 - IEEE International Conference on Automatic Face and Gesture RecognitionJodhpur, IndiaDecember 2021
- 48 inproceedingsSelective Spatio-Temporal Aggregation Based Pose Refinement System: Towards Understanding Human Activities in Real-World Videos.IEEE/CVF Winter Conference on Applications of Computer Vision (WACV)Virtual, United StatesJanuary 2021
- 49 inproceedingsSelf-Supervised Video Pose Representation Learning for Occlusion-Robust Action Recognition.FG 2021 - IEEE International Conference on Automatic Face and Gesture RecognitionJodhpur (Virtual), IndiaDecember 2021
- 50 inproceedingsUNIK: A Unified Framework for Real-world Skeleton-based Action Recognition.BMVC 2021 - The British Machine Vision ConferenceVirtual, United KingdomNovember 2021
Conferences without proceedings
- 51 inproceedingsTrichTrack: Multi-Object Tracking of Small-Scale Trichogramma Wasps.AVSS 2021 - 17th IEEE International Conference on Advanced Video and Signal-based SurveillanceVirtual, United StatesNovember 2021
- 52 inproceedingsGuided Flow Field Estimation by Generating Independent Patches.32nd British Machine Vision Conference (BMVC 2021)Virtual, United KingdomNovember 2021
Scientific book chapters
- 53 inbook3D CNN Architectures and Attention Mechanisms for Deepfake Detection.Handbook of Digital Face Manipulation and Detection2022
Doctoral dissertations and habilitation theses
- 54 thesisComputer vision for deciphering and generating faces.Université Côte d'AzurSeptember 2021
- 55 thesisQuantified analysis for video recordings of seizure.Université Côte d'AzurDecember 2021
- 56 thesisLearning to Generate Human Videos.Inria - Sophia Antipolis; Université Cote d'AzurSeptember 2021
12.3 Cited publications
- 57 articleA hierarchical multimodal system for motion analysis in patients with~epilepsy.Epilepsy & Behavior87October 2018, 46--58
- 58 inproceedingsGanomaly: Semi-supervised anomaly detection via adversarial training.Asian conference on computer visionSpringer2018, 622--637
- 59 inproceedingsMissing data reconstruction and anomaly detection in crop development using agronomic indicators derived from multispectral satellite images.2017 IEEE International Geoscience and Remote Sensing Symposium (IGARSS)IEEE2017, 5081--5084
- 60 articleA deep learning architecture for temporal sleep stage classification using multivariate and multimodal time series.IEEE Transactions on Neural Systems and Rehabilitation Engineering2642018, 758--769
- 61 inproceedingsBERT: Pre-training of Deep Bidirectional Transformers for Language Understanding.Proceedings of the 2019 Conference of the North American Chapter of the Association for Computational Linguistics: Human Language Technologies, Volume 1 (Long and Short Papers)Minneapolis, MinnesotaAssociation for Computational LinguisticsJune 2019, 4171--4186URL: https://www.aclweb.org/anthology/N19-1423
- 62 inproceedingsA hierarchical classification system for sleep stage scoring via forehead EEG signals.2013 IEEE Symposium on Computational Intelligence, Cognitive Algorithms, Mind, and Brain (CCMB)IEEE2013, 1--5
- 63 inproceedingsA Deep Learning Architecture for Epileptic Seizure Classification Based on Object and Action Recognition.ICASSP 2020 - 2020 IEEE International Conference on Acoustics, Speech and Signal Processing (ICASSP)IEEEMay 2020
- 64 article What uncertainties do we need in bayesian deep learning for computer vision? arXiv preprint arXiv:1703.04977 2017
- 65 inproceedingsClusternet: Detecting small objects in large scenes by exploiting spatio-temporal information.Proceedings of the IEEE Conference on Computer Vision and Pattern Recognition2018, 4003--4012
- 66 inproceedingsBART: Denoising Sequence-to-Sequence Pre-training for Natural Language Generation, Translation, and Comprehension.Proceedings of the 58th Annual Meeting of the Association for Computational LinguisticsOnlineAssociation for Computational LinguisticsJuly 2020, 7871--7880URL: https://www.aclweb.org/anthology/2020.acl-main.703
- 67 inproceedingsEpileptic seizure classification using the NeuroMov database.2019 IEEE 6th Portuguese Meeting on Bioengineering (ENBENG)IEEEFebruary 2019, URL: https://doi.org/10.1109/enbeng.2019.8692465
- 68 inproceedingsAttention is All you Need.Advances in Neural Information Processing Systems30Curran Associates, Inc.2017, URL: https://proceedings.neurips.cc/paper/2017/file/3f5ee243547dee91fbd053c1c4a845aa-Paper.pdf
- 69 inproceedingsCamera-aware Proxies for Unsupervised Person Re-Identification.AAAI2021
- 70 inproceedingsDetecting and tracking small moving objects in wide area motion imagery (wami) using convolutional neural networks (cnns).2019 22th International Conference on Information Fusion (FUSION)IEEE2019, 1--8