Keywords
Computer Science and Digital Science
- A3.3.2. Data mining
- A3.3.3. Big data analysis
- A3.4. Machine learning and statistics
- A3.4.1. Supervised learning
- A3.4.2. Unsupervised learning
- A3.4.8. Deep learning
- A4. Security and privacy
- A5.3.3. Pattern recognition
- A5.4.1. Object recognition
- A5.4.3. Content retrieval
- A5.7. Audio modeling and processing
- A5.7.1. Sound
- A5.7.3. Speech
- A5.8. Natural language processing
- A9.2. Machine learning
- A9.3. Signal analysis
- A9.4. Natural language processing
Other Research Topics and Application Domains
- B9. Society and Knowledge
- B9.3. Medias
- B9.6.10. Digital humanities
- B9.10. Privacy
1 Team members, visitors, external collaborators
Research Scientists
- Laurent Amsaleg [Team leader, CNRS, Senior Researcher, HDR]
- Vincent Claveau [CNRS, Researcher, HDR]
- Teddy Furon [INRIA, Senior Researcher, HDR]
- Guillaume Gravier [CNRS, Senior Researcher, HDR]
- Kassem Kallas [INRIA, Starting Research Position, from Mar 2022]
Faculty Members
- Ewa Kijak [UNIV RENNES I, Associate Professor, HDR]
- Simon Malinowski [UNIV RENNES I, Associate Professor]
- Pascale Sébillot [INSA RENNES, Professor, HDR]
Post-Doctoral Fellow
- Gauthier Lyan [CNRS, from May 2022]
PhD Students
- Benoit Bonnet [INRIA]
- Antoine Chaffin [IMATAG, CIFRE]
- Deniz Engin [INRIA, from Jul 2022]
- Deniz Engin [INTERDIGITAL, CIFRE, until May 2022]
- Pierre Fernandez [FACEBOOK, CIFRE, from Mar 2022]
- Louis Hemadou [SAFRAN, CIFRE, from Nov 2022]
- Carolina Jeronimo De Almeida [GOUV BRESIL, from Sep 2022]
- Victor Klotzer [INRIA, from Oct 2022]
- Quentin Le Roux [THALES, CIFRE, from Nov 2022]
- Thibault Maho [INRIA]
- Cyrielle Mallart [UNIV RENNES I (Assistant Professor), ATER]
- Duc-Hau Nguyen [CNRS]
- Samuel Tap [ZAMA SAS, CIFRE]
- Hugo Thomas [UNIV RENNES I, from Oct 2022]
- Karim Tit [THALES, CIFRE]
- Shashanka Venkataramanan [INRIA, from Jan to June and then from December]
Technical Staff
- Maxence Despres [Inria, Engineer, from Nov 2022]
- Nicolas Fouque [CNRS, Engineer, from May 2022]
- Guillaume Le Noé-Bienvenu [CNRS, Engineer, from May 2022]
Administrative Assistant
- Aurélie Patier [UNIV RENNES I]
2 Overall objectives
2.1 Context
Linkmedia is concerned with the processing of extremely large collections of multimedia material. The material we refer to are collections of documents that are created by humans and intended for humans. It is material that is typically created by media players such as TV channels, radios, newspapers, archivists (BBC, INA, ...), as well as the multimedia material that goes through social-networks. It has images, videos and pathology reports for e-health applications, or that is in relation with e-learning which typically includes a fair amount of texts, graphics, images and videos associating in new ways teachers and students. It also includes material in relation with humanities that study societies through the multimedia material that has been produced across the centuries, from early books and paintings to the latest digitally native multimedia artifacts. Some other multimedia material are out of the scope of Linkmedia, such as the ones created by cameras or sensors in the broad areas of video-surveillance or satellite images.
Multimedia collections are rich in contents and potential, that richness being in part within the documents themselves, in part within the relationships between the documents, in part within what humans can discover and understand from the collections before materializing its potential into new applications, new services, new societal discoveries, ... That richness, however, remains today hardly accessible due to the conjunction of several factors originating from the inherent nature of the collections, the complexity of bridging the semantic gap or the current practices and the (limited) technology:
- Multimodal: multimedia collections are composed of very diverse material (images, texts, videos, audio, ...), which require sophisticated approaches at analysis time. Scientific contributions from past decades mostly focused on analyzing each media in isolation one from the other, using modality-specific algorithms. However, revealing the full richness of collections calls for jointly taking into account these multiple modalities, as they are obviously semantically connected. Furthermore, involving resources that are external to collections, such as knowledge bases, can only improve gaining insight into the collections. Knowledge bases form, in a way, another type of modality with specific characteristics that also need to be part of the analysis of media collections. Note that determining what a document is about possibly mobilizes a lot of resources, and this is especially costly and time consuming for audio and video. Multimodality is a great source of richness, but causes major difficulties for the algorithms running analysis;
- Intertwined: documents do not exist in isolation one from the other. There is more knowledge in a collection than carried by the sum of its individual documents and the relationships between documents also carry a lot of meaningful information. (Hyper)Links are a good support for materializing the relationships between documents, between parts of documents, and having analytic processes creating them automatically is challenging. Creating semantically rich typed links, linking elements at very different granularities is very hard to achieve. Furthermore, in addition to being disconnected, there is often no strong structure into each document, which makes even more difficult their analysis;
- Collections are very large: the scale of collections challenges any algorithm that runs analysis tasks, increasing the duration of the analysis processes, impacting quality as more irrelevant multimedia material gets in the way of relevant ones. Overall, scale challenges the complexity of algorithms as well as the quality of the result they produce;
- Hard to visualize: It is very difficult to facilitate humans getting insight on collections of multimedia documents because we hardly know how to display them due to their multimodal nature, or due to their number. We also do not know how to well present the complex relationships linking documents together: granularity matters here, as full documents can be linked with small parts from others. Furthermore, visualizing time-varying relationships is not straightforward. Data visualization for multimedia collections remains quite unexplored.
2.2 Scientific objectives
The ambition of Linkmedia is to propose foundations, methods, techniques and tools to help humans make sense of extremely large collections of multimedia material. Getting useful insight from multimedia is only possible if tools and users interact tightly. Accountability of the analysis processes is paramount in order to allow users understanding their outcome, to understand why some multimedia material was classified this way, why two fragments of documents are now linked. It is key for the acceptance of these tools, or for correcting errors that will exist. Interactions with users, facilitating analytics processes, taking into account the trust in the information and the possible adversarial behaviors are topics Linkmedia addresses.
3 Research program
3.1 Scientific background
Linkmedia is de facto a multidisciplinary research team in order to gather the multiple skills needed to enable humans to gain insight into extremely large collections of multimedia material. It is multimedia data which is at the core of the team and which drives the design of our scientific contributions, backed-up with solid experimental validations. Multimedia data, again, is the rationale for selecting problems, applicative fields and partners.
Our activities therefore include studying the following scientific fields:
- multimedia: content-based analysis; multimodal processing and fusion; multimedia applications;
- computer vision: compact description of images; object and event detection;
- machine learning: deep architectures; structured learning; adversarial learning;
- natural language processing: topic segmentation; information extraction;
- information retrieval: high-dimensional indexing; approximate k-nn search; embeddings;
- data mining: time series mining; knowledge extraction.
3.2 Workplan
Overall, Linkmedia follows two main directions of research that are (i) extracting and representing information from the documents in collections, from the relationships between the documents and from what user build from these documents, and (ii) facilitating the access to documents and to the information that has been elaborated from their processing.
3.3 Research Direction 1: Extracting and Representing Information
Linkmedia follows several research tracks for extracting knowledge from the collections and representing that knowledge to facilitate users acquiring gradual, long term, constructive insights. Automatically processing documents makes it crucial to consider the accountability of the algorithms, as well as understanding when and why algorithms make errors, and possibly invent techniques that compensate or reduce the impact of errors. It also includes dealing with malicious adversaries carefully manipulating the data in order to compromise the whole knowledge extraction effort. In other words, Linkmedia also investigates various aspects related to the security of the algorithms analyzing multimedia material for knowledge extraction and representation.
Knowledge is not solely extracted by algorithms, but also by humans as they gradually get insight. This human knowledge can be materialized in computer-friendly formats, allowing algorithms to use this knowledge. For example, humans can create or update ontologies and knowledge bases that are in relation with a particular collection, they can manually label specific data samples to facilitate their disambiguation, they can manually correct errors, etc. In turn, knowledge provided by humans may help algorithms to then better process the data collections, which provides higher quality knowledge to humans, which in turn can provide some better feedback to the system, and so on. This virtuous cycle where algorithms and humans cooperate in order to make the most of multimedia collections requires specific support and techniques, as detailed below.
Machine Learning for Multimedia Material.
Many approaches are used to extract relevant information from multimedia material, ranging from very low-level to higher-level descriptions (classes, captions, ...). That diversity of information is produced by algorithms that have varying degrees of supervision. Lately, fully supervised approaches based on deep learning proved to outperform most older techniques. This is particularly true for the latest developments of Recurrent Neural Networkds (RNN, such as LSTMs) or convolutional neural network (CNNs) for images that reach excellent performance 48. Linkmedia contributes to advancing the state of the art in computing representations for multimedia material by investigating the topics listed below. Some of them go beyond the very processing of multimedia material as they also question the fundamentals of machine learning procedures when applied to multimedia.
- Learning from few samples/weak supervisions. CNNs and RNNs need large collections of carefully annotated data. They are not fitted for analyzing datasets where few examples per category are available or only cheap image-level labels are provided. Linkmedia investigates low-shot, semi-supervised and weakly supervised learning processes: Augmenting scarce training data by automatically propagating labels 51, or transferring what was learned on few very well annotated samples to allow the precise processing of poorly annotated data 60. Note that this context also applies to the processing of heritage collections (paintings, illuminated manuscripts, ...) that strongly differ from contemporary natural images. Not only annotations are scarce, but the learning processes must cope with material departing from what standard CNNs deal with, as classes such as "planes", "cars", etc, are irrelevant in this case.
- Ubiquitous Training. NN (CNNs, LSTMs) are mainstream for producing representations suited for high-quality classification. Their training phase is ubiquitous because the same representations can be used for tasks that go beyond classification, such as retrieval, few-shot, meta- and incremental learning, all boiling down to some form of metric learning. We demonstrated that this ubiquitous training is relatively simpler 51 yet as powerful as ad-hoc strategies fitting specific tasks 65. We study the properties and the limitations of this ubiquitous training by casting metric learning as a classification problem.
- Beyond static learning. Multimedia collections are by nature continuously growing, and ML processes must adapt. It is not conceivable to re-train a full new model at every change, but rather to support continuous training and/or allowing categories to evolve as the time goes by. New classes may be defined from only very few samples, which links this need for dynamicity to the low-shot learning problem discussed here. Furthermore, active learning strategies determining which is the next sample to use to best improve classification must be considered to alleviate the annotation cost and the re-training process 55. Eventually, the learning process may need to manage an extremely large number of classes, up to millions. In this case, there is a unique opportunity of blending the expertise of Linkmedia on large scale indexing and retrieval with deep learning. Base classes can either be "summarized" e.g. as a multi-modal distribution, or their entire training set can be made accessible as an external associative memory 71.
- Learning and lightweight architectures. Multimedia is everywhere, it can be captured and processed on the mobile devices of users. It is necessary to study the design of lightweight ML architectures for mobile and embedded vision applications. Inspired by 75, we study the savings from quantizing hyper-parameters, pruning connections or other approximations, observing the trade-off between the footprint of the learning and the quality of the inference. Once strategy of choice is progressive learning which early aborts when confident enough 56.
- Multimodal embeddings. We pursue pioneering work of Linkmedia on multimodal embedding, i.e., representing multiple modalities or information sources in a single embedded space 69, 68, 70. Two main directions are explored: exploiting adversarial architectures (GANs) for embedding via translation from one modality to another, extending initial work in 70 to highly heterogeneous content; combining and constraining word and RDF graph embeddings to facilitate entity linking and explanation of lexical co-occurrences 45.
- Accountability of ML processes. ML processes achieve excellent results but it is mandatory to verify that accuracy results from having determined an adequate problem representation, and not from being abused by artifacts in the data. Linkmedia designs procedures for at least explaining and possibly interpreting and understanding what the models have learned. We consider heat-maps materializing which input (pixels, words) have the most importance in the decisions 64, Taylor decompositions to observe the individual contributions of each relevance scores or estimating LID 32 as a surrogate for accounting for the smoothness of the space.
- Extracting information. ML is good at extracting features from multimedia material, facilitating subsequent classification, indexing, or mining procedures. Linkmedia designs extraction processes for identifying parts in the images 61, 62, relationships between the various objects that are represented in images 38, learning to localizing objects in images with only weak, image-level supervision 64 or fine-grained semantic information in texts 43. One technique of choice is to rely on generative adversarial networks (GAN) for learning low-level representations. These representations can e.g. be based on the analysis of density 74, shading, albedo, depth, etc.
- Learning representations for time evolving multimedia material. Video and audio are time evolving material, and processing them requests to take their time line into account. In 57, 41 we demonstrated how shapelets can be used to transform time series into time-free high-dimensional vectors, preserving however similarities between time series. Representing time series in a metric space improves clustering, retrieval, indexing, metric learning, semi-supervised learning and many other machine learning related tasks. Research directions include adding localization information to the shapelets, fine-tuning them to best fit the task in which they are used as well as designing hierarchical representations.
Adversarial Machine Learning.
Systems based on ML take more and more decisions on our behalf, and maliciously influencing these decisions by crafting adversarial multimedia material is a potential source of dangers: a small amount of carefully crafted noise imperceptibly added to images corrupts classification and/or recognition. This can naturally impact the insight users get on the multimedia collection they work with, leading to taking erroneous decisions e.g.
This adversarial phenomenon is not particular to deep learning, and can be observed even when using other ML approaches 37. Furthermore, it has been demonstrated that adversarial samples generalize very well across classifiers, architectures, training sets. The reasons explaining why such tiny content modifications succeed in producing severe errors are still not well understood.
We are left with little choice: we must gain a better understanding of the weaknesses of ML processes, and in particular of deep learning. We must understand why attacks are possible as well as discover mechanisms protecting ML against adversarial attacks (with a special emphasis on convolutional neural networks). Some initial contributions have started exploring such research directions, mainly focusing on images and computer vision problems. Very little has been done for understanding adversarial ML from a multimedia perspective 42.
Linkmedia is in a unique position to throw at this problem new perspectives, by experimenting with other modalities, used in isolation one another, as well as experimenting with true multimodal inputs. This is very challenging, and far more complicated and interesting than just observing adversarial ML from a computer vision perspective. No one clearly knows what is at stake with adversarial audio samples, adversarial video sequences, adversarial ASR, adversarial NLP, adversarial OCR, all this being often part of a sophisticated multimedia processing pipeline.
Our ambition is to lead the way for initiating investigations where the full diversity of modalities we are used to work with in multimedia are considered from a perspective of adversarial attacks and defenses, both at learning and test time. In addition to what is described above, and in order to trust the multimedia material we analyze and/or the algorithms that are at play, Linkmedia investigates the following topics:
- Beyond classification. Most contributions in relation with adversarial ML focus on classification tasks. We started investigating the impact of adversarial techniques on more diverse tasks such as retrieval 31. This problem is related to the very nature of euclidean spaces where distances and neighborhoods can all be altered. Designing defensive mechanisms is a natural companion work.
- Detecting false information. We carry-on with earlier pioneering work of Linkmedia on false information detection in social media. Unlike traditional approaches in image forensics 46, we build on our expertise in content-based information retrieval to take advantage of the contextual information available in databases or on the web to identify out-of-context use of text or images which contributed to creating a false information 58.
- Deep fakes. Progress in deep ML and GANs allow systems to generate realistic images and are able to craft audio and video of existing people saying or doing things they never said or did 54. Gaining in sophistication, these machine learning-based "deep fakes" will eventually be almost indistinguishable from real documents, making their detection/rebutting very hard. Linkmedia develops deep learning based counter-measures to identify such modern forgeries. We also carry on with making use of external data in a provenance filtering perspective 63 in order to debunk such deep fakes.
- Distributions, frontiers, smoothness, outliers. Many factors that can possibly explain the adversarial nature of some samples are in relation with their distribution in space which strongly differs from the distribution of natural, genuine, non adversarial samples. We are investigating the use of various information theoretical tools that facilitate observing distributions, how they differ, how far adversarial samples are from benign manifolds, how smooth is the feature space, etc. In addition, we are designing original adversarial attacks and develop detection and curating mechanisms 32.
Multimedia Knowledge Extraction.
Information obtained from collections via computer ran processes is not the only thing that needs to be represented. Humans are in the loop, and they gradually improve their level of understanding of the content and nature of the multimedia collection. Discovering knowledge and getting insight is involving multiple people across a long period of time, and what each understands, concludes and discovers must be recorded and made available to others. Collaboratively inspecting collections is crucial. Ontologies are an often preferred mechanism for modeling what is inside a collection, but this is probably limitative and narrow.
Linkmedia is concerned with making use of existing strategies in relation with ontologies and knowledge bases. In addition, Linkmedia uses mechanisms allowing to materialize the knowledge gradually acquired by humans and that might be subsequently used either by other humans or by computers in order to better and more precisely analyze collections. This line of work is instantiated at the core of the iCODA project Linkmedia coordinates.
We are therefore concerned with:
- Multimedia analysis and ontologies. We develop approaches for linking multimedia content to entities in ontologies for text and images, building on results in multimodal embedding to cast entity linking into a nearest neighbor search problem in a high-dimensional joint embedding of content and entities 68. We also investigate the use of ontological knowledge to facilitate information extraction from content 45.
- Explainability and accountability in information extraction. In relation with ontologies and entity linking, we develop innovative approaches to explain statistical relations found in data, in particular lexical or entity co-occurrences in textual data, for example using embeddings constrained with translation properties of RDF knowledge or path-based explanation within RDF graphs. We also work on confidence measures in entity linking and information extraction, studying how the notions of confidence and information source can be accounted for in knowledge basis and used in human-centric collaborative exploration of collections.
- Dynamic evolution of models for information extraction. In interactive exploration and information extraction, e.g., on cultural or educational material, knowledge progressively evolves as the process goes on, requiring on-the-fly design of new models for content-based information extractors from very few examples, as well as continuous adaptation of the models. Combining in a seamless way low-shot, active and incremental learning techniques is a key issue that we investigate to enable this dynamic mechanisms on selected applications.
3.4 Research Direction 2: Accessing Information
Linkmedia centers its activities on enabling humans to make good use of vast multimedia collections. This material takes all its cultural and economic value, all its artistic wonder when it can be accessed, watched, searched, browsed, visualized, summarized, classified, shared, ... This allows users to fully enjoy the incalculable richness of the collections. It also makes it possible for companies to create business rooted in this multimedia material.
Accessing the multimedia data that is inside a collection is complicated by the various type of data, their volume, their length, etc. But it is even more complicated to access the information that is not materialized in documents, such as the relationships between parts of different documents that however share some similarity. Linkmedia in its first four years of existence established itself as one of the leading teams in the field of multimedia analytics, contributing to the establishment of a dedicated community (refer to the various special sessions we organized with MMM, the iCODA and the LIMAH projects, as well as 52, 53, 49).
Overall, facilitating the access to the multimedia material, to the relevant information and the corresponding knowledge asks for algorithms that efficiently search collections in order to identify the elements of collections or of the acquired knowledge that are matching a query, or that efficiently allow navigating the collections or the acquired knowledge. Navigation is likely facilitated if techniques are able to handle information and knowledge according to hierarchical perspectives, that is, allow to reveal data according to various levels of details. Aggregating or summarizing multimedia elements is not trivial.
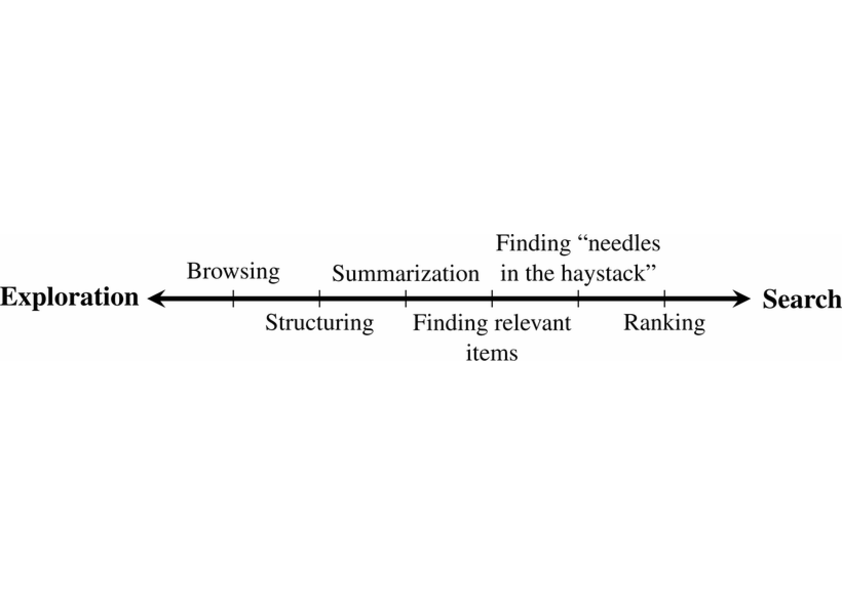
Three topics are therefore in relation with this second research direction. Linkmedia tackles the issues in relation to searching, to navigating and to summarizing multimedia information. Information needs when discovering the content of a multimedia collection can be conveniently mapped to the exploration-search axis, as first proposed by Zahálka and Worring in 73, and illustrated by Figure 1 where expert users typically work near the right end because their tasks involve precise queries probing search engines. In contrast, lay-users start near the exploration end of the axis. Overall, users may alternate searches and explorations by going back and forth along the axis. The underlying model and system must therefore be highly dynamic, support interactions with the users and propose means for easy refinements. Linkmedia contributes to advancing the state of the art in searching operations, in navigating operations (also referred to as browsing), and in summarizing operations.
Searching.
Search engines must run similarity searches very efficiently. High-dimensional indexing techniques therefore play a central role. Yet, recent contributions in ML suggest to revisit indexing in order to adapt to the specific properties of modern features describing contents.
-
Advanced scalable indexing. High-dimensional indexing is one of the foundations of Linkmedia. Modern features extracted from the multimedia material with the most recent ML techniques shall be indexed as well. This, however, poses a series of difficulties due to the dimensionality of these features, their possible sparsity, the complex metrics in use, the task in which they are involved (instance search,
-nn, class prototype identification, manifold search 51, time series retrieval, ...). Furthermore, truly large datasets require involving sketching 35, secondary storage and/or distribution 34, 33, alleviating the explosion of the number of features to consider due to their local nature or other innovative methods 50, all introducing complexities. Last, indexing multimodal embedded spaces poses a new series of challenges. - Improving quality. Scalable indexing techniques are approximate, and what they return typically includes a fair amount of false positives. Linkmedia works on improving the quality of the results returned by indexing techniques. Approaches taking into account neighborhoods 44, manifold structures instead of pure distance based similarities 51 must be extended to cope with advanced indexing in order to enhance quality. This includes feature selection based on intrinsic dimensionality estimation 32.
- Dynamic indexing. Feature collections grow, and it is not an option to fully reindex from scratch an updated collection. This trivially applies to the features directly extracted from the media items, but also to the base class prototypes that can evolve due to the non-static nature of learning processes. Linkmedia will continue investigating what is at stake when designing dynamic indexing strategies.
Navigating.
Navigating a multimedia collection is very central to its understanding. It differs from searching as navigation is not driven by any specific query. Rather, it is mostly driven by the relationships that various documents have one another. Relationships are supported by the links between documents and/or parts of documents. Links rely on semantic similarity, depicting the fact that two documents share information on the same topic. But other aspects than semantics are also at stake, e.g., time with the dates of creation of the documents or geography with mentions or appearance in documents of some geographical landmarks or with geo-tagged data.
In multimedia collections, links can be either implicit or explicit, the latter being much easier to use for navigation. An example of an implicit link can be the name of someone existing in several different news articles; we, as humans, create a mental link between them. In some cases, the computer misses such configurations, leaving such links implicit. Implicit links are subject to human interpretation, hence they are sometimes hard to identify for any automatic analysis process. Implicit links not being materialized, they can therefore hardly be used for navigation or faceted search. Explicit links can typically be seen as hyperlinks, established either by content providers or, more aligned with Linkmedia, automatically determined from content analysis. Entity linking (linking content to an entity referenced in a knowledge base) is a good example of the creation of explicit links. Semantic similarity links, as investigated in the LIMAH project and as considered in the search and hyperlinking task at MediaEval and TRECVid, are also prototypical links that can be made explicit for navigation. Pursuing work, we investigate two main issues:
- Improving multimodal content-based linking. We exploit achievements in entity linking to go beyond lexical or lexico-visual similarity and to provide semantic links that are easy to interpret for humans; carrying on, we work on link characterization, in search of mechanisms addressing link explainability (i.e., what is the nature of the link), for instance using attention models so as to focus on the common parts of two documents or using natural language generation; a final topic that we address is that of linking textual content to external data sources in the field of journalism, e.g., leveraging topic models and cue phrases along with a short description of the external sources.
- Dynamicity and user-adaptation. One difficulty for explicit link creation is that links are often suited for one particular usage but not for another, thus requiring creating new links for each intended use; whereas link creation cannot be done online because of its computational cost, the alternative is to generate (almost) all possible links and provide users with selection mechanisms enabling personalization and user-adaptation in the exploration process; we design such strategies and investigate their impact on exploration tasks in search of a good trade-off between performance (few high-quality links) and genericity.
Summarizing.
Multimedia collections contain far too much information to allow any easy comprehension. It is mandatory to have facilities to aggregate and summarize a large body on information into a compact, concise and meaningful representation facilitating getting insight. Current technology suggests that multimedia content aggregation and story-telling are two complementary ways to provide users with such higher-level views. Yet, very few studies already investigated these issues. Recently, video or image captioning 72, 67 have been seen as a way to summarize visual content, opening the door to state-of-the-art multi-document text summarization 47 with text as a pivot modality. Automatic story-telling has been addressed for highly specific types of content, namely TV series 39 and news 59, 66, but still need a leap forward to be mostly automated, e.g., using constraint-based approaches for summarization 36, 66.
Furthermore, not only the original multimedia material has to be summarized, but the knowledge acquired from its analysis is also to summarize. It is important to be able to produce high-level views of the relationships between documents, emphasizing some structural distinguishing qualities. Graphs establishing such relationships need to be constructed at various level of granularity, providing some support for summarizing structural traits.
Summarizing multimedia information poses several scientific challenges that are:
- Choosing the most relevant multimedia aggregation type: Taking a multimedia collection into account, a same piece of information can be present in several modalities. The issue of selecting the most suitable one to express a given concept has thus to be considered together with the way to mix the various modalities into an acceptable production. Standard summarization algorithms have to be revisited so that they can handle continuous representation spaces, allowing them to benefit from the various modalities 40.
- Expressing user’s preferences: Different users may appreciate quite different forms of multimedia summaries, and convenient ways to express their preferences have to be proposed. We for example focus on the opportunities offered by the constraint-based framework.
- Evaluating multimedia summaries: Finding criteria to characterize what a good summary is remains challenging, e.g., how to measure the global relevance of a multimodal summary and how to compare information between and across two modalities. We tackle this issue particularly via a collaboration with A. Smeaton at DCU, comparing the automatic measures we will develop to human judgments obtained by crowd-sourcing.
- Taking into account structuring and dynamicity: Typed links between multimedia fragments, and hierarchical topical structures of documents obtained via work previously developed within the team are two types of knowledge which have seldom been considered as long as summarization is concerned. Knowing that the event present in a document is causally related to another event described in another document can however modify the ways summarization algorithms have to consider information. Moreover the question of producing coarse-to-fine grain summaries exploiting the topical structure of documents is still an open issue. Summarizing dynamic collections is also challenging and it is one of the questions we consider.
4 Application domains
4.1 Asset management in the entertainment business
Media asset management—archiving, describing and retrieving multimedia content—has turned into a key factor and a huge business for content and service providers. Most content providers, with television channels at the forefront, rely on multimedia asset management systems to annotate, describe, archive and search for content. So do archivists such as the Institut National de l'Audiovisuel, the bibliothèque Nationale de France, the Nederlands Instituut voor Beeld en Geluid or the British Broadcast Corporation, as well as media monitoring companies, such as Yacast in France. Protecting copyrighted content is another aspect of media asset management.
4.2 Multimedia Internet
One of the most visible application domains of linked multimedia content is that of multimedia portals on the Internet. Search engines now offer many features for image and video search. Video sharing sites also feature search engines as well as recommendation capabilities. All news sites provide multimedia content with links between related items. News sites also implement content aggregation, enriching proprietary content with user-generated content and reactions from social networks. Most public search engines and Internet service providers offer news aggregation portals. This also concerns TV on-demand and replay services as well as social TV services and multi-screen applications. Enriching multimedia content, with explicit links targeting either multimedia material or knowledge databases is central here.
4.3 Data journalism
Data journalism forms an application domain where most of the technology developed by Linkmedia can be used. On the one hand, data journalists often need to inspect multiple heterogeneous information sources, some being well structured, some other being fully unstructured. They need to access (possibly their own) archives with either searching or navigational means. To gradually construct insight, they need collaborative multimedia analytics processes as well as elements of trust in the information they use as foundations for their investigations. Trust in the information, watching for adversarial and/or (deep) fake material, accountability are all crucial here.
5 Social and environmental responsibility
5.1 Impact of research results
Social biases in text generation.
Recent advances in the domain of text generation allow realistic text-based interaction with a computer. These systems rely on complex neural architectures that leverage very large amount of training texts collected the Web. The problem is that these texts contains unwanted biases (sexism, racism, harmful language...) that are sometimes even amplified by the training procedure. Curating the training texts once for all is not feasible due to the complexity of defining a priori what is relevant or not at the training time. Our work on controlled generation 9 takes another point of view and tries to impose constraints at the inference time. This work aims at making the text generation respect application-specific conditions with the help of a simple classifier.
6 Highlights of the year
- Ewa Kijak defended her Habilitation à Diriger des Recherches.
- Teddy Furon has been appointed Directeur de Recherche.
6.1 Awards
- In September, Kassem Kallas has been elevated to the grade of Senior Member of IEEE.
7 New results
7.1 Extracting and Representing Information
7.1.1 RoSe: A RObust and SEcure Black-Box DNN Watermarking
Participants: Kassem Kallas, Teddy Furon.
Protecting the Intellectual Property rights of DNN models is of primary importance prior to their deployment. So far, the proposed methods either necessitate changes to internal model parameters or the machine learning pipeline, or they fail to meet both the security and robustness requirements. In contrast, 15 proposes a lightweight, robust, and secure black-box DNN watermarking protocol that takes advantage of cryptographic one-way functions as well as the injection of in-task key imagelabel pairs during the training process. These pairs are later used to prove DNN model ownership during testing. The main feature is that the value of the proof and its security are measurable. The extensive experiments watermarking image classification models for various datasets as well as exposing them to a variety of attacks, show that it provides protection while maintaining an adequate level of security and robustness.7.1.2 Randomized Smoothing under Attack: How Good is it in Pratice?
Participants: Thibault Maho, Teddy Furon, Erwan Le Merrer [WIDE].
Randomized smoothing is a recent and celebrated solution to certify the robustness of any classifier. While it indeed provides a theoretical robustness against adversarial attacks, the dimensionality of current classifiers necessarily imposes Monte Carlo approaches for its application in practice. This paper questions the effectiveness of randomized smoothing as a defense, against state of the art black-box attacks 18. This is a novel perspective, as previous research works considered the certification as an unquestionable guarantee. We first formally highlight the mismatch between a theoretical certification and the practice of attacks on classifiers. We then perform attacks on randomized smoothing as a defense. Our main observation is that there is a major mismatch in the settings of the RS for obtaining high certified robustness or when defeating black box attacks while preserving the classifier accuracy.7.1.3 Traitor tracing
Participant: Teddy Furon.
27 presents the problem of tracing traitors and the most efficient solution known, Tardos codes. Tardos codes are an application overlay to the watermarking transmission layer: an identifier is generated for each user and then watermarked into a confidential document to be shared. The chapter focuses on the modeling of collusion when several traitors mix their copies. Thanks to this model, mathematics (statistics, information theory) gives us the fundamental limits of this tool.7.1.4 FBI: Fingerprinting models with Benign Inputs
Participants: Thibault Maho, Teddy Furon, Erwan Le Merrer [WIDE].
Les avancées récentes dans le domaine des empreintes de réseaux profonds détectent des instances de modèles placées dans une boîte noire. Les entrées utilisées en tant qu'empreintes sont spécifiquement conçues pour chaque modèle à vérifier. Bien qu'efficace dans un tel scénario, il en résulte néanmoins un manque de garantie après une simple modification (e.g. réentraînement, quantification) d'un modèle. Cet article (voir 17) s'attaque aux défis de proposer i) des empreintes qui résistent aux modifications significatives des modèles, en généralisant la notion de familles de modèles et leurs variantes, ii) une extension de la tâche d'empreinte à des scénarios où l'on souhaite un modèle précis (précédemment appelé tâche de detection), mais aussi d'identifier la famille de modèles qui se trouve dans la boîte noire (tâche d'identification). Nous atteignons ces deux objectifs en démontrant que des entrées authentiques (non modifiées) sont un matériau suffisant pour les deux tâches. Nous utilisons la théorie de l'information pour la tâche d'identification et un algorithme glouton pour la tâche de détection. Les deux approches sont validées expérimentalement sur un ensemble inédit de plus de 1 000 réseaux.7.1.5 Watermarking Images in Self-Supervised Latent Spaces
Participants: Pierre Fernandez [Meta IA], Alexandre Sablayrolles [Meta IA], Teddy Furon, Hervé Jégou [Meta IA], Matthijs Douze [Meta IA].
We revisit watermarking techniques based on pre-trained deep networks, in the light of self-supervised approaches. We present in 12, 11 a way to embed both marks and binary messages into their latent spaces, leveraging data augmentation at marking time. Our method can operate at any resolution and creates watermarks robust to a broad range of transformations (rotations, crops, JPEG, contrast, etc). It significantly outperforms the previous zero-bit methods, and its performance on multi-bit watermarking is on par with state-of-the-art encoder-decoder architectures trained end-to-end for watermarking.7.1.6 Adversarial images with downscaling
Participants: Teddy Furon, Benoît Bonnet, Patrick Bas [CRIStAL - Centre de Recherche en Informatique, Signal et Automatique de Lille - UMR 9189].
Most works on adversarial attacks consider small images whose size already fits the model. In this work, we explore attacking large images on classifiers with different input sizes 13. Downscaling is a necessary first step to adapt the size of the image to the model that might reform the adversarial signal. This paper studies the possibility of forging adversarial images through different interpolation methods, the distortion of their adversarial signal, and the transferability over other downscaling methods. This paper finally explores attacking an ensemble model which gathers different resizing interpolations to increase the transferability of the attack against a set downscaling kernels.7.1.7 Temporal Relation Extraction in Clinical Texts
Participants: Yohan Bonescki Gumiel [PUCPR - Pontifícia Universidade Católica do Paraná, Brazil], Lucas Emanuel Silva Oliveira [PUCPR - Pontifícia Universidade Católica do Paraná, Brazil], Vincent Claveau, Natalia Grabar [STL - Savoirs, Textes, Langage (STL) - UMR 8163], Emerson Cabrera Paraiso [PUCPR - Pontifícia Universidade Católica do Paraná, Brazil], Claudia Moro [PUCPR - Pontifícia Universidade Católica do Paraná, Brazil], Deborah Ribeiro Carvalho [PUCPR - Pontifícia Universidade Católica do Paraná, Brazil].
Unstructured data in electronic health records, represented by clinical texts, are a vast source of healthcare information because they describe a patient's journey, including clinical findings, procedures, and information about the continuity of care. The publication of several studies on temporal relation extraction from clinical texts during the last decade and the realization of multiple shared tasks highlight the importance of this research theme. Therefore, we propose in 5 a review of temporal relation extraction in clinical texts. We analyzed 105 articles and verified that relations between events and document creation time, a coarse temporality type, were addressed with traditional machine learning–based models with few recent initiatives to push the state-of-the-art with deep learning–based models. For temporal relations between entities (event and temporal expressions) in the document, factors such as dataset imbalance because of candidate pair generation and task complexity directly affect the system's performance. The state-of-the-art resides on attention-based models, with contextualized word representations being fine-tuned for temporal relation extraction. However, further experiments and advances in the research topic are required until real-time clinical domain applications are released. Furthermore, most of the publications mainly reside on the same dataset, hindering the need for new annotation projects that provide datasets for different medical specialties, clinical text types, and even languages.7.1.8 Deep Neural Network Attacks and Defense: The Case of Image Classification
Participants: Hanwei Zhang [LIS Marseille], Teddy Furon, Laurent Amsaleg, Yannis Avrithis [IARAI].
Machine learning using deep neural networks applied to image recognition works extremely well. However, it is possible to modify the images very slightly and intentionally, with modifications almost invisible to the eye, to deceive the classification system into misclassifying such content into the incorrect visual category. Deep neural networks have made it possible to automatically recognize the visual content of images. White box attacks consider the scenario where the attacker knows everything about the classifier network. 28 provides a quick overview of techniques used to produce adversarial images. It schematically distinguishes three families of defenses: reactive techniques, proactive techniques and obfuscation techniques. Another viable view distinguishes whether the defense is an add-on module connected to the network, or whether the defense is an integral part of the network resulting in a radical transformation of the classifier.7.1.9 AlignMixup: Improving Representations By Interpolating Aligned Features
Participants: Shashanka Venkataramanan, Ewa Kijak, Laurent Amsaleg, Yannis Avrithis [IARAI].
Mixup is a powerful data augmentation method that interpolates between two or more examples in the input or feature space and between the corresponding target labels. However, how to best interpolate images is not well defined. Recent mixup methods overlay or cut-and-paste two or more objects into one image, which needs care in selecting regions. Mixup has also been connected to autoencoders, because often autoencoders generate an image that continuously deforms into another. However, such images are typically of low quality. In this work, we revisit mixup from the deformation perspective and introduce AlignMixup, where we geometrically align two images in the feature space 21. The correspondences allow us to interpolate between two sets of features, while keeping the locations of one set. Interestingly, this retains mostly the geometry or pose of one image and the appearance or texture of the other. We also show that an autoencoder can still improve representation learning under mixup, without the classifier ever seeing decoded images. AlignMixup outperforms state-of-the-art mixup methods on five different benchmarks.7.1.10 It Takes Two to Tango: Mixup for Deep Metric Learning
Participants: Shashanka Venkataramanan, Bill Psomas [National Technical University of Athens], Ewa Kijak, Laurent Amsaleg, Konstantinos Karantzalos [National Technical University of Athens], Yannis Avrithis [IARAI].
Metric learning involves learning a discriminative representation such that embeddings of similar classes are encouraged to be close, while embeddings of dissimilar classes are pushed far apart. State-of-the-art methods focus mostly on sophisticated loss functions or mining strategies. On the one hand, metric learning losses consider two or more examples at a time. On the other hand, modern data augmentation methods for classification consider two or more examples at a time. The combination of the two ideas is under-studied. In this work, we aim to bridge this gap and improve representations using mixup, which is a powerful data augmentation approach interpolating two or more examples and corresponding target labels at a time 22. This task is challenging because unlike classification, the loss functions used in metric learning are not additive over examples, so the idea of interpolating target labels is not straightforward. To the best of our knowledge, we are the first to investigate mixing both examples and target labels for deep metric learning. We develop a generalized formulation that encompasses existing metric learning loss functions and modify it to accommodate for mixup, introducing Metric Mix, or Metrix. We also introduce a new metric - utilization to demonstrate that by mixing examples during training, we are exploring areas of the embedding space beyond the training classes, thereby improving representations. To validate the effect of improved representations, we show that mixing inputs, intermediate representations or embeddings along with target labels significantly outperforms state-of-the-art metric learning methods on four benchmark deep metric learning datasets.7.1.11 Confronting Active Learning for Relation Extraction to a Real-life Scenario on French Newspaper Data
Participants: Cyrielle Mallart, Michel Le Nouy [Sipa Ouest-France, Rennes], Guillaume Gravier, Pascale Sébillot.
With recent deep learning advances in natural language processing, tasks such as relation extraction have been solved on benchmark data with near-perfect accuracy. However, in a realistic scenario, such as in a French newspaper company mostly dedicated to local information, relations are of varied, highly specific types, with virtually no data annotated for relations, and many entities co-occur in a sentence without being related. We question the use of supervised state-of-the-art models in such a context, where resources such as time, computing power and human annotators are limited. To adapt to these constraints, we experiment with an active-learning based relation extraction pipeline, consisting of a binary LSTM-based model for detecting the relations that do exist, and a state-of-the-art model for relation classification 19. We compare several classification models of different depths, from simplistic word embedding averaging, to graph neural networks and Bert-based models, as well as several active learning query strategies, including a proposal for a balanced uncertainty-based strategy, in order to find the most cost-efficient yet accurate approach in our newspaper company’s use case. Our findings highlight the unsuitability of deep models in this data-scarce scenario, as well as the need to further develop data-driven active learning strategies.7.1.12 Statistical study of word embeddings in French transformer models
Participants: Loïc Fosse, Duc-Hau Nguyen, Pascale Sébillot, Guillaume Gravier.
In this line of work, we studied the statistical properties of word embeddings in transformer models for the French language 25. We applied variance analysis, intra-sentence cosine similarity and effective rank at various depth of pre-trained French transformers, namely FlauBERT and CamemBERT. We also studied the impact of fine-tuning the models on a text classification task. We evidenced that the two pre-trained models exhibit very different behavior. Both however tend to generate anisotropic word embeddings that concentrate on a cone for a given sentence. This behavior confirms observations previously reported for the Enlgish language. Fine-tuning the models for text classification modifies the behavior of the models, in particular on the final layers, and strongly reinforces convergence of the word embeddings in a cone within each sentence. The effective dimension of the final embedding is significantly reduced. We also highlighted the relationship between convergence of the word embeddings and performance on text classification, with the direction of the cone being class-dependant. This relationship however remains difficult to fully apprenhend.7.1.13 Improving the plausibility of attention weights in natural language inference
Participants: Duc-Hau Nguyen, Guillaume Gravier, Pascale Sébillot.
We studied the plausibility of an attention mechanism for a sentence inference task (entailment), i.e., its ability to provide a human plausible explanation of the relationship between two sentences 26. Based on the Explanation-Augmented Standford Natural Language Inference corpus, it has been shown that attention weights are implausible in practice and tend not to focus on important tokens. We study here different approaches to make attention weights more plausible, relying on masks from morphosyntactic analysis or on regularization to force parsimony. We show that these strategies significantly improve the plausibility of attention weights and outperform saliency map approaches.7.1.14 Graph-based image gradients aggregated with random forests
Participants: Raquel Almeida, Ewa Kijak, Simon Malinowski, Silvio J.R. Guimarães [PUC Minas, Brésil] .
Gradient methods subject images to a series of operations to enhance some characteristics and facilitate image analysis, usually the contours of large objects. In 4, we argue that a gradient must show other characteristics, such as minor components and large uniform regions, particularly for the image segmentation task where subjective concepts such as region coherence and similarity are hard to interpret from the pixel information. We propose a graph-based image gradient method that uses edge-weighted graphs aggregated with Random Forest (RF) to create descriptive gradients. We evaluated the proposals on the edge and segmentation tasks, analyzing the gradient characteristics that most impacted the final segmentation. The experiments indicated that sharp thick contours are crucial, whereas fuzzy maps yielded the worst results even when created from deep methods with more precise edge maps. Also, we analyzed how uniform regions and small details impacted the final segmentation. Statistical analysis on the segmentation task demonstrated that the gradients created by the proposed are significantly better than most of the best edge maps methods and validated our original choices of attributes.7.1.15 AIP: Adversarial Interaction Priors for Multi-Agent Physics-based Character Control
Participants: Mohamed Younes [MIMETIC], Ewa Kijak, Richard Kulpa [MiMETIC, M2S], Simon Malinowski, Franck Multon [MIMETIC] .
We address the problem of controlling and simulating interactions between multiple physics-based characters, using short unlabeled motion clips 30. We propose Adversarial Interaction Priors (AIP), a multi-agents generative adversarial imitation learning (MAGAIL) approach, which extends recent deep reinforcement learning (RL) works aiming at imitating single character example motions. The main contribution of this work is to extend the idea of motion imitation of a single character to interaction imitation between multiple characters. Our method uses a control policy for each character to imitate interactive behaviors provided by short example motion clips, and associates a discriminator for each character, which is trained on actor-specific interactive motion clips. The discriminator returns interaction rewards that measure the similarity between generated behaviors and demonstrated ones in the reference motion clips. The policies and discriminators are trained in a multi-agent adversarial reinforcement learning procedure, to improve the quality of the behaviors generated by each agent. The initial results show the effectiveness of our method on the interactive task of shadowboxing between two fighters.7.1.16 Impact of Data Cleansing for Urban Bus Commercial Speed Prediction
Participants: Gauthier Lyan, David Gross-Amblard [Druid], Jean-Marc Jézéquel [Diverse], Simon Malinowski.
Les systèmes d'information pour les transports publics (SITP) sont largement répandus et utilisés par les services de bus publics dans nombre de villes à travers le monde. Ces systèmes recueillent des informations sur les trajets, les arrêts de bus, la vitesse des bus, la fréquentation, etc. Ces données massives constituent une source d'information intéressante pour les outils prédictifs d'apprentissage automatique. Cependant, elles souffrent le plus souvent de déficiences qualitatives, dues à des ensembles de données multiples aux structures multiples, à des infrastructures différentes utilisant des technologies incompatibles, à des erreurs humaines ou à des défaillances matérielles. Dans cet article (6), nous examinons l'impact du nettoyage des données sur une tâche classique d'apprentissage automatique : la prédiction de la vitesse commerciale des bus urbains. Nous montrons que des règles de gestion et de qualité simples et spécifiques au transport peuvent améliorer considérablement la qualité des données, alors que des règles plus sophistiquées tendent à offrir peu d'améliorations tout en ayant un coût de calcul élevé.7.1.17 Reasoning over Time into Models with DataTime
Participants: Gauthier Lyan, Jean-Marc Jézéquel [Diverse], David Gross-Amblard [Druid], Romain Lefeuvre [Diverse], Benoit Combemale [Diverse].
Models at runtime have been initially investigated for adaptive systems. Models are used as a reflective layer of the current state of the system to support the implementation of a feedback loop. More recently, models at runtime have also been identified as key for supporting the development of full-fledged digital twins. However, this use of models at runtime raises new challenges, such as the ability to seamlessly interact with the past, present and future states of the system. In 7, we propose a framework called DataTime to implement models at runtime which capture the state of the system according to the dimensions of both time and space, here modeled as a directed graph where both nodes and edges bear local states (ie. values of properties of interest). DataTime offers a unifying interface to query the past, present and future (predicted) states of the system. This unifying interface provides i) an optimized structure of the time series that capture the past states of the system, possibly evolving over time, ii) the ability to get the last available value provided by the system's sensors, and iii) a continuous micro-learning over graph edges of a predictive model to make it possible to query future states, either locally or more globally, thanks to a composition law. The framework has been developed and evaluated in the context of the Intelligent Public Transportation Systems of the city of Rennes (France). This experimentation has demonstrated how DataTime can be used for managing data from the past, the present and the future, and facilitate the development of digital twins.7.1.18 Prédiction du niveau de nappes phréatiques : comparaison d'approches locale, globale et hybride
Participants: Lola Beuchée, Thomas Guyet [LIRIS, LBBE], Simon Malinowski.
Cet article (8) présente l'exploration d'une méthode autorégressive de prévision d'une série temporelle pour répondre au défi de la prédiction du niveau de nappes phréatiques. Une méthode autorégressive estime une valeur future d'une série temporelle par régression à partir des valeurs historiques de la série. Plusieurs méthodes de régression peuvent alors être employées. Dans cet article, on présente des expérimentations visant à identifier la meilleure configuration pour prédire de manière précise le niveau de nappes phréatiques. On compare pour cela différents prédicteurs, l'apprentissage de modèle par série ou par groupe de séries, et l'utilisation de données exogènes. Des expérimentations intensives ont été menées et nous permettent de conclure sur le choix de la méthode que nous utiliserons pour répondre au défi.7.1.19 Temporal Disaggregation of the Cumulative Grass Growth
Participants: Thomas Guyet [LIRIS, LBBE], Laurent Spillemaecker [ENSAI], Simon Malinowski, Anne-Isabelle Graux [PEGASE - Physiologie, Environnement et Génétique pour l'Animal et les Systèmes d'Elevage].
Information on the grass growth over a year is essential for some models simulating the use of this resource to feed animals on pasture or at barn with hay or grass silage. Unfortunately, this information is rarely available. The challenge is to reconstruct grass growth from two sources of information: usual daily climate data (rainfall, radiation, etc.) and cumulative growth over the year. We have to be able to capture the effect of seasonal climatic events which are known to distort the growth curve within the year. In 14, 20, we formulate this challenge as a problem of disaggregating the cumulative growth into a time series. To address this problem, our method applies time series forecasting using climate information and grass growth from previous time steps. Several alternatives of the method are proposed and compared experimentally using a database generated from a grassland process-based model. The results show that our method can accurately reconstruct the time series, independently of the use of the cumulative growth information.7.2 Accessing Information
7.2.1 PPL-MCTS: Constrained Textual Generation Through Discriminator-Guided MCTS Decoding
Participants: Antoine Chaffin [IMATAG], Vincent Claveau, Ewa Kijak.
Large language models (LM) based on Transformers allow to generate plausible long texts. In 9, 23, we explore how this generation can be further controlled at decoding time to satisfy certain constraints (e.g. being non-toxic, conveying certain emotions, using a specific writing style, etc.) without fine-tuning the LM.Precisely, we formalize constrained generation as a tree exploration process guided by a discriminator that indicates how well the associated sequence respects the constraint. This approach, in addition to being easier and cheaper to train than fine-tuning the LM, allows to apply the constraint more finely and dynamically.We propose several original methods to search this generation tree, notably the Monte Carlo Tree Search (MCTS) which provides theoretical guarantees on the search efficiency, but also simpler methods based on re-ranking a pool of diverse sequences using the discriminator scores. These methods are evaluated, with automatic and human-based metrics, on two types of constraints and languages: review polarity and emotion control in French and English. We show that discriminator-guided MCTS decoding achieves state-of-the-art results without having to tune the language model, in both tasks and languages. We also demonstrate that other proposed decoding methods based on re-ranking can be really effective when diversity among the generated propositions is encouraged.7.2.2 Which Discriminator for Cooperative Text Generation?
Participants: Antoine Chaffin [IMATAG], Thomas Scialom [reciTAL], Sylvain Lamprier [MLIA-ISIR], Jacopo Staiano [reciTAL], Benjamin Piwowarski [MLIA-ISIR], Ewa Kijak, Vincent Claveau.
Language models generate texts by successively predicting probability distributions for next tokens given past ones. A growing field of interest tries to leverage external information in the decoding process so that the generated texts have desired properties, such as being more natural, non toxic, faithful, or having a specific writing style. A solution is to use a classifier at each generation step, resulting in a cooperative environment where the classifier guides the decoding of the language model distribution towards relevant texts for the task at hand. In 10, 24, we examine three families of (transformer-based) discriminators for this specific task of cooperative decoding: bidirectional, left-to-right and generative ones. We evaluate the pros and cons of these different types of discriminators for cooperative generation, exploring respective accuracy on classification tasks along with their impact on the resulting sample quality and computational performances. We also provide the code of a batched implementation of the powerful cooperative decoding strategy used for our experiments, the Monte Carlo Tree Search, working with each discriminator for Natural Language Generation.7.2.3 Generative Cooperative Networks for Natural Language Generation
Participants: Sylvain Lamprier [MLIA-ISIR], Thomas Scialom [reciTAL], Antoine Chaffin [IMATAG], Vincent Claveau, Ewa Kijak, Jacopo Staiano [reciTAL], Benjamin Piwowarski [MLIA-ISIR].
Generative Adversarial Networks (GANs) have known a tremendous success for many continuous generation tasks, especially in the field of image generation. However, for discrete outputs such as language, optimizing GANs remains an open problem with many instabilities, as no gradient can be properly back-propagated from the discriminator output to the generator parameters. An alternative is to learn the generator network via reinforcement learning, using the discriminator signal as a reward, but such a technique suffers from moving rewards and vanishing gradient problems. Finally, it often falls short compared to direct maximum-likelihood approaches. We introduce Generative Cooperative Networks, in which the discriminator architecture is cooperatively used along with the generation policy to output samples of realistic texts for the task at hand 16. We give theoretical guarantees of convergence for our approach, and study various efficient decoding schemes to empirically achieve state-of-the-art results in two main NLG tasks.8 Bilateral contracts and grants with industry
8.1 Bilateral contracts with industry
CIFRE PhD: Incremental dynamic construction of knowledge bases from text mining
Participants: Guillaume Gravier, Cyrielle Mallart, Pascale Sébillot.
Duration: 3 years, started in Dec. 2018 Partner: Ouest France
In the context of a newspaper, the thesis explores the combination of text mining and knowledge representation techniques to assist the extraction, interpretation and validation of valuable pieces of information from the journal’s content so as to incrementally build a full-scale knowledge base. This thesis is in close relation with the iCODA Inria Project Lab, with direct contribution to the project’s results. Cyrielle Mallart defended her PhD thesis on Nov 23, 2022.CIFRE PhD: Few shot learning for object recognition in aerial images
Participants: Yannis Avrithis, Yann Lifchitz.
Duration: 3 years, started in March 2018 Partner: Safran Tech
This is a CIFRE PhD thesis project aiming to study architectures and learning techniques most suitable for object recognition from few samples and to validate these approaches on multiple recognition tasks and use-cases related to aerial images.CIFRE PhD: Deep Learning and Homomorphic encryption
Participants: Teddy Furon, Samuel Tap.
Duration: 3 years, started in December 2020 Partner: ZAMA.ia
This is a CIFRE PhD thesis project aiming to study inference and training of neural networks in the encrypted domain. This means that inputs (test or training data) are encrypted to protect confidentiality.CIFRE PhD: Robustness of machine learning against uncertainties
Participants: Teddy Furon, Mathias Rousset, Karim Tit.
Duration: 3 years, started in December 2020 Partner: THALES La Ruche
This is a CIFRE PhD thesis project aiming to study the robustness of machine learning algorithm facing uncertainties in the acquisition chain of the data.CIFRE PhD: Semantic multimodal question answering (MQA) in domestic environments
Participants: Yannis Avrithis, Teddy Furon, Deniz Engin.
Duration: 3 years, started in September 2020 Partner: InterDigital
This is a CIFRE PhD thesis project aiming at designing novel question answering methods based on deep learning to facilitate living conditions in home environments. It investigates moving from image understanding towards multimodal context understanding in video of long duration. This may allow answering questions based on what has happened in the past.CIFRE PhD: Multimodal detection of fake news
Participants: Vincent Claveau, Ewa Kijak, Antoine Chaffin.
Duration: 3 years, started in November 2020 Partner: IMATAG
This is a CIFRE PhD thesis project aiming at designing multimodal models able to detect fake news, like repurposing techniques, based on joint analysis of visual and textual modalities.CIFRE PhD: Certification of Deep Neural Networks
Participants: Teddy Furon, Kassem Kallas, Quentin Le Roux.
Duration: 3 years, started in November 2022 Partner:THALES
This is a CIFRE PhD thesis project aiming at assessing the security of already trained Deep Neural Networks, especially in the context of face recognition.CIFRE PhD: Watermarking and deep learning
Participants: Teddy Furon, Pierre Fernandez.
Duration: 3 years, started in May 2022 Partner: META AI
This is a CIFRE PhD thesis project aiming at watermarking deep learning models analyzing or generating images or at using deep learning to watermark images.CIFRE PhD: Domain generalization exploiting synthetic data
Participants: Ewa Kijak, Louis Hemadou.
Duration: 3 years, started in Nov. 2022 Partner: SAFRAN
This is a CIFRE PhD thesis project aiming at exploiting synthetic data to be able to perform transfer learning in presence of very few or inexistent real data in the context of image detection or classification tasks.Telegramme-CNRS bilateral contract: NLP for computational journalism
Participants: Vincent Claveau, Nicolas Fouqué.
Duration: 2 years, started in Jan 2022
The project aims developing a wide range of text-mining and classification tools with the French press group Le Télégramme. In particular, we aim at discovering cues of success in the already published news articles and then exploit them to propose new angles of coverage of newsworthy events to the journalists.9 Partnerships and cooperations
9.1 International initiatives
9.1.1 Associate Teams in the framework of an Inria International Lab or in the framework of an Inria International Program
LOGIC
-
Title:
Learning on graph-based hierarchical methods for image and multimedia data
-
Duration:
2020 ->
-
Coordinator:
Silvio Jamil Guimaraes (sjamil@pucminas.br)
-
Partners:
- Pontifícia Universidade Católica de Minas Gerais Belo Horizonte (Brésil)
-
Inria contact:
Simon Malinowski
-
Summary:
The main goal of this project is related to learning graph-based hierarchical methods to be applied on image and multimedia data. Regarding image data, we aim at advancing in the state-of-the-art on hierarchy of partitions taking into account aspects of efficiency, quality, and interactivity, as well as the use of hierarchical information to help the information extraction process. Research on graph-based multimedia label/information propagation will be developed within this project along two main lines of research : construction of multimedia graphs where links should depict semantic proximity between documents or fragments of documents; how different graph structures can be used to propagate information (usually tags or labels) from one document to another and across modalities
9.1.2 Participation in other International Programs
CAPES COFECUB-CNRS Hierarchical graph-based analysis of image, video, and multimedia data
Participants: Guillaume Gravier, Ewa Kijak, Raquel Pereira de Almeida.
-
Title:
Hierarchical graph-based analysis of image, video, and multimedia data
-
Partner Institution(s):
- Insitut National Polytechnique de Grenoble, France
- Laboratoire d'Informatique Gaspard-Monge, France
- PUC Minas, Brésil
- Universidade Estadual de Campinas, Brésil
- Universidade Federal Minas Gerais, Brésil
-
Date/Duration:
2018–2022
9.2 National initiatives
Chaire Security of AI for Defense Applications (SAIDA)
Participants: Teddy Furon, Laurent Amsaleg, Erwan Le Merrer [WIDE], Mathias Rousset [SIMSMART], Benoit Bonnet, Thibault Maho, Patrick Bas [CRIStAL - Centre de Recherche en Informatique, Signal et Automatique de Lille - UMR 9189], Samuel Tap, Karim Tit.
Duration: 4 years, started Sept 2020 ANR-20-CHIA-0011-01
SAIDA targets the AID "Fiabilité de l’intelligence artificielle, vulnérabilités et contre-mesures" chair. It aims at establishing the fundamental principles for designing reliable and secure AI systems: a reliable AI maintains its good performance even under uncertainties; a secure AI resists attacks in hostile environments. Reliability and security are challenged at training and at test time. SAIDA therefore studies core issues in relation with poisoning training data, stealing the parameters of the model or inferring sensitive training from information leaks. Additionally, SAIDA targets uncovering the fundamentals of attacks and defenses engaging AI at test time. Three converging research directions make SAIDA: 1) theoretical investigations grounded in statistics and applied mathematics to discover the underpinnings of reliability and security, 2) connects adversarial sampling and Information Forensics and Security, 3) protecting the training data and the AI system. SAIDA thus combines theoretical investigations with more applied and heuristic studies to guarantee the applicability of the findings as well as the ability to cope with real world settings.
ANR Archival: Multimodal machine comprehension of language for new intelligent interfaces of scientific and cultural mediation
Participants: Laurent Amsaleg, Guillaume Gravier, Guillaume Le Noé-Bienvenu, Duc Hau Nguyen, Pascale Sébillot.
Duration: 3.5 year, started in Dec. 2019
The multidisciplinary and multi-actor ARCHIVAL project aims at yielding collaborations between researchers from the fields of Information and Communication Sciences as well as Computer Sciences around archive value enhancing and knowledge sharing for arts, culture and heritage. The project is structured around the following questionings: What part can machine comprehension methods play towards the reinterpretation of thematic archive collections? How can content mediation interfaces exploit results generated by current AI approaches?
ARCHIVAL teams will explore heterogeneous document collection structuration in order to explicitly reveal implicit links, to explain the nature of these links and to promote them in an intelligible way towards ergonomic mediation interfaces that will guarantee a successful appropriation of contents. A corpus has been delimited from the FMSH “self-management” collection, recently awarded as Collex, which will be completed from the large Canal-U academic audiovisual portal. The analysis and enhancement of this collection is of particular interest for Humanities and Social Sciences in a context where it becomes a necessity to structurally reconsider new models of socioeconomic development (democratic autonomy, social and solidarity-based economy, alternative development,…).
ANR MEERQAT: MultimEdia Entity Representation and Question Answering Tasks
Participants: Laurent Amsaleg, Yannis Avrithis, Ewa Kijak, Shashanka Venkataramanan.
Duration: 3.5 year, started in April 2020 Partners: Inria project-teams Linkmedia, CEA LIST, LIMSI, IRIT.
The overall goal of the project is to tackle the problem of ambiguities of visual and textual content by learning then combining their representations. As a final use case, we propose to solve a Multimedia Question Answering task, that requires to rely on three different sources of information to answer a (textual) question with regard to visual data as well as an external knowledge base containing millions of unique entities, each being represetd by textual and visual content as well as some links to other entities. An important work will deal with the representation of entities into a common tri-modal space, in which one should determine the content to associate to an entity to adequately represent it. The challenge consists in defining a representation that is compact (for performance) while still expressive enough to reflect the potential links between the entity and a variety of others.
MinArm: EVE3
Participants: Teddy Furon.
Duration: 3 year, started in April 2019 Partners: MinArm, CRIStAL Lille, LIRMM, Univ. Troyes, Univ. Paris Saclay
Teaching and technology survey on steganography and steganalysis in the real world.AID-CNRS: FakeNews
Participants: Vincent Claveau, Ewa Kijak, Gauthier Lyan.
Duration: 2 years, started mid-2021
This AID funded project aims at building tools and concepts to help detect Fake News (incl. deepfake) in social networks. It relies on NLP and multimodal analysis to leverage textual and visual clues of manipulation.ASTRID: HybrInfox
Participants: Vincent Claveau, Guillaume Gravier.
Duration: 20 months, started Jan. 2022
This ANR-AID funded project aims at building exploring how hybridation of symbolic and deep learning NLP tools. These hybrid tools are expected to be used to detect some types of disinformation; in particular, these NLP tools target vagueness (non precise) or subjective (opinion rather than factual) discourses.10 Dissemination
Participants: Laurent Amsaleg, Vincent Claveau, Teddy Furon, Guillaume Gravier, Ewa Kijak, Simon Malinowski, Pascale Sébillot.
10.1 Promoting scientific activities
10.1.1 Scientific events: organisation
General chair, scientific chair
- Vincent Claveau and the whole LinkMedia team organized a thematic day of the CNRS GdR TAL: details on the event website
- Vincent Claveau organized a thematic call for project on "NLP for defence" on behalf of AID and CNRS.
10.1.2 Scientific events: selection
Member of the conference program committees
- Laurent Amsaleg was a PC member of: ACM International Conference on Multimedia, ACM International Conference on Multimedia Retrieval, Multimedia Modeling, Content-Based Multimedia Indexing, IEEE International Conference on Multimedia & Expo, International Conference on Similarity Search and Applications. Laurent Amsaleg was area chair for ACM Multimedia 2022.
- Guillaume Gravier was a PC member of: ACM International Conference on Multimedia Retrieval, IEEE International Symposium on Multimedia
- Vincent Claveau was a PC member of: CIRCLE, LREC, TALN, AAAI 2022
- Ewa Kijak was a PC member of: : International Conference on Content-Based Multimedia Indexing
- Pascale Sébillot was a PC member of: 13th Language Resources and Evaluation Conference, 29th Conference Traitement Automatique des Langues Naturelles
- Teddy Furon was a reviewer for: IEEE ICASSP, NeurIPS, AISTAT, ICLR.
10.1.3 Journal
Member of the editorial boards
- Pascale Sébillot is editor of the Journal Traitement Automatique des Langues (TAL)
- Vincent Claveau is an editorial board member of the journal TAL (Traitement Automatique des Langues)
- Pascale Sébillot is member of the editorial board of the Journal Traitement Automatique des Langues (TAL)
Reviewer - reviewing activities
- Teddy Furon was a reviewer for: IEEE Transactions on Dependable and Secure Computing, IEEE Transactions on Pattern Analysis and Machine Intelligence, IEEE Transactions on Information Forensics and Security.
- Vincent Claveau was a reviewer for: IEEE Transactions of Affective Computing
10.1.4 Invited talks
- Teddy Furon was an invited speaker at Conference on Artificial Intelligence for Defense, during the European Cyberweek, and Journées de la Statistiques Rennaises (JSTAR).
10.1.5 Leadership within the scientific community
- Laurent Amsaleg is a member of the Steering Committee of ACM Multimedia for the 2020-2023 term
- Guillaume Gravier is a member of the scientific board of the GDR Traitement automatique des langues
- Guillaume Gravier is a referent on AI within Allistene representing the CPU
- Pascale Sébillot is a member of the board of the GDR Traitement automatique des langues
10.1.6 Scientific expertise
- Teddy Furon was a scientific expert for the call for projects ANR ASTRID.
- Ewa Kijak was a scientific expert for the call for projects ANR AAPG.
- Ewa Kijak was member of the selection committees in charge of recruiting an associate professor for INSA, ISTIC and Centrale-Supelec Paris
- Vincent Claveau was a reviewer for the Prize Paul Caseau (EDF – Académie des Technologies).
- Vincent Claveau was member of the selection committee in charge of recruiting an associate professor for Centrale-Supelec Paris
- Vincent Claveau was a reviewer for: the Emergence call for project of the MSH Paris-Saclay; the call for project of the LabEx CIMI ; the CIFRE PhD selection, ANRT.
10.1.7 Research administration
- Guillaume Gravier is director of IRISA (UMR 6074)
- Pascale Sébillot is deputy director of IRISA (UMR 6074)
- Guillaume Gravier is the scientific leader of the AI for Semantic Data Analytics (PNRIA) doctoral program for the Rennes site
- Guillaume Gravier is a member of the executive board of CREACH Labs, a general partnership agreement between the Brittany region, MINARM and higher education and research (ESR) institutions
- Guillaume Gravier is a member of the board of directors of the Images & Networks competitiveness cluster
- Teddy Furon est membre de la commission du personnel et président de la commission des délégations Inria.
- Pascale Sébillot is deputy director of the Scientific Advisory Committee of IRISA (UMR 6074)
- Pascale Sébillot was a member of the board of the MathSTIC doctoral school until August 2022
10.2 Teaching - Supervision - Juries
10.2.1 Teaching
- Licence: Pascale Sébillot, Natural Language Processing, 6h, L3, INSA Rennes, France
- Master: Laurent Amsaleg, Bases de données avancées, 25h, M2, INSA Rennes, France
- Master: Teddy Furon, Rare Event Simulations, 40h, INSA Rennes, France
- Master: Pascale Sébillot, Natural Language Processing, 6h, M1, INSA Rennes, France
- Engineering school: Vincent Claveau, Machine Learning, 18h, 3rd year, INSA Rennes, France
- Engineering school: Vincent Claveau, Natural Language Processing, 12h, ESIR, Univ. Rennes, France
- Licence: Guillaume Gravier, Base de données, 26h, L2, INSA Rennes
- Licence: Guillaume Gravier, Natural language processing, 12h, L3, INSA Rennes
- Licence: Guillaume Gravier, Markov models, 6h, L3, INSA Rennes
- Master: Guillaume Gravier, Natural Language Processing, 6h, M1, INSA Rennes
- Master: Guillaume Gravier, Natural Language Processing, 33h, M2, ENSAI
- Ewa Kijak is head of the Image engineering track (M1-M2) of ESIR, Univ. Rennes
- Master: Ewa Kijak, Supervised machine learning, 15h, M2R, Univ. Rennes
- Master: Ewa Kijak, Image retrieval, 12h, M2, ESIR
- Master: Ewa Kijak, Image classification, 27h, M1, ESIR
- Master: Ewa Kijak, Image processing, 45h, M1, ESIR, Univ. Rennes
- Master: Simon Malinowski, Basics of Data Analytics for Data Science, 24h, EIT Data Science Master 1, Rennes
- Master: Simon Malinowski, Prediction Methods, 30h, M1 MIAGE and Data Science EIT Master 1, Rennes
- Master: Simon Malinowski, Statisical Data Mining, 24h, M2 MIAGE, ISTIC, Rennes
- Master: Simon Malinowski, Symbolic Data Mining, 12h, M2 MIAGE, ISTIC, Rennes
- Simon Malinowski is responsible for the Master 2 MIAGE parcours Classique
- Simon Malinowski is responsible for the M2 studies within the DataScience track of the EIT-digital master school
10.2.2 Supervision
- PhD in progress: Shashanka Venkataramanan, Metric learning for instance- and category-level visual representations. Started in Dec. 2020. Yannis Avrithis, Ewa Kijak & Laurent Amsaleg
- PhD in progress: Deniz Engin, Video query answering in domestic environments. Started in Sept. 2020. Teddy Furon, Yannis Avrithis, Laurent Amsaleg
- PhD in progress: Raquel Almeida, Learning hierarchichal models for multimedia data. Started Jan. 2019, Ewa Kijak & Simon Malinowski & Laurent Amsaleg
- PhD in progress: Louis Hemadou, Domain generalization exploiting synthetic data. Université de Rennes, Started Nov. 2022. Ewa Kijak (with Frederic Jurie, GREYC, Caen)
- PhD in progress: Antoine Chaffin, Multimodal indexing and generation. Université de Rennes, Started Oct. 2020. Vincent Claveau, Ewa Kijak
- PhD in progress: Mohamed Younes, Learning and simulating strategies in sports for VR training. Started Dec. 2020. Ewa Kijak, Simon Malinowski (with Franck Multon and Richard Kulpa, Mimetic team)
- PhD in progress: Duc Hau Nguyen, Generation and justification of semantic links with attention mechanisms. Université de Rennes, Started Sept. 2020. Pascale Sébillot, Guillaume Gravier
- PhD in progress: Hugo Thomas, Zero-shot and few shot relation extraction in press archives. Université de Rennes. Started Oct. 2022. Pascale Sébillot, Guillaume Gravier
- PhD in progress: Paul Estano, Dynamic-Precision Training of Deep Neural Network Models on the Edge. Started Feb. 2022. Guillaume Gravier (with TARAN and DANTE project-teams, Silviu-Joan Filip and Elisa Riccietti)
- PhD in progress: Benoit Bonnet, Understanding, taming, and defending from adversarial examples. Started Nov. 2019. Teddy Furon (with Patrick Bas, CNRS CRIsTAL, Lille)
- PhD in progress: Thibault Maho, Black-box attacks against deep learning algorithms. Started Feb. 2022. Teddy Furon (with Erwan Le Merrer, team project WIDE)
- PhD in progress: Karim Tit, Robustness assessment of deep neural networks. Started Feb. 2021. Teddy Furon (with Mathias Rousset, team-project SIMSMART)
- PhD in progress: Samuel Tap, Learning in the encrypted domain. Started Mar. 2021. Teddy Furon.
- PhD in progress: Victor Kötzler, Membership Inference Attack. Started Feb. 2022. Teddy Furon (with Yufei Han, team-project CIDRE).
- PhD in progress: Quentin Le Roux, Security Assessment of pre-trained neural networks. Started Nov. 2022. Teddy Furon.
- PhD: Cyrielle Mallart, Dynamic and incremental construction of knowlege graphs with text mining. INSA Rennes. Defended Nov. 2022.
10.2.3 Juries
- Laurent Amsaleg was reviewer for the PhD of Zhengyu Zhao, Rethinking Realism: Advancing the Transferability and Imperceptibility of Adversarial Images, Radboud University, February.
- Teddy Furon was reviewer for the PhD of Quentin Giboulot (UTT Troyes), and of Amine Hmani (Telecom ParisTech).
- Teddy Furon was reviewer for the HDR of Adrien Chan Hon Tong (Onera).
- Vincent Claveau was a reviewer for the PhD of Miriam Benballa (LITIS, Normandie Univ.)
- Vincent Claveau was an examiner for the PhD of Eliott Maitre (IRIT, Univ. Toulouse 3)
- Pascale Sébillot was reviewer for the PhD of Quentin Portes Université Toulouse III, June 2022
- Pascale Sébillot was examiner for the PhD of Étienne Simon Sorbonne université, July 2022
- Pascale Sébillot was president for the PhD of Betty Fabre Université de Rennes 1, September
- Pascale Sébillot was examiner for the PhD of Luis-Gil Moreno-Jiménez Avignon université, November
10.3 Popularization
10.3.1 Education
- Teddy Furon presented 7 classes Chiche! in Lycées Chateaubriand (Rennes) and Jean Bodin (Les Ponts de Cé).
10.3.2 Interventions
- Vincent Claveau was interviewed by BiKiNi, TV Rennes, Cercle de la Presse, France Bleu Armorique
- Vincent Claveau participated in roundtable discussions: i-expo, Paris and ERRIE, Univ. Rennes
11 Scientific production
11.1 Major publications
- 1 articleGenerating Adversarial Images in Quantized Domains.IEEE Transactions on Information Forensics and Security2022
- 2 inproceedingsPPL-MCTS: Constrained Textual Generation Through Discriminator-Guided Decoding.CtrlGen 2021 - Workshop on Controllable Generative Modeling in Language and Vision at NeurIPS 2021Proceedings of the CtrlGen workshopvirtual, United StatesDecember 2021, 1-19
- 3 inproceedingsAlignMixup: Improving Representations By Interpolating Aligned Features.CVPR 2022 - IEEE/CVF Conference on Computer Vision and Pattern RecognitionNew Orleans, United StatesIEEEJune 2022, 1-13
11.2 Publications of the year
International journals
- 4 articleGraph-based image gradients aggregated with random forests.Pattern Recognition Letters2022
- 5 articleTemporal Relation Extraction in Clinical Texts.ACM Computing Surveys547September 2022, 1-36
- 6 articleImpact of Data Cleansing for Urban Bus Commercial Speed Prediction.SN Computer Science3822022, 1-11
- 7 articleReasoning over Time into Models with DataTime.Software and Systems ModelingDecember 2022, 1-25
International peer-reviewed conferences
- 8 inproceedingsPrédiction du niveau de nappes phréatiques : comparaison d'approches locale, globale et hybride.EGC 2022 - Conférence francophone sur l'Extraction et la Gestion des ConnaissancesBlois, FranceJanuary 2022
- 9 inproceedingsPPL-MCTS: Constrained Textual Generation Through Discriminator-Guided MCTS Decoding.NAACL 2022 - Conference of the North American Chapter of the Association for Computational Linguistics: Human Language TechnologiesHybrid: Seattle, Washington + Online, United StatesJuly 2022, 1-15
- 10 inproceedingsWhich Discriminator for Cooperative Text Generation?SIGIR 2022 - 45th International ACM SIGIR Conference on Research and Development in Information RetrievalMadrid, SpainACMJuly 2022, 2360-2365
- 11 inproceedingsTatouage Numérique d'Images dans l'Espace Latent de Réseaux Auto-Supervisés.GRETSI 2022 - Colloque Francophone de Traitement du Signal et des ImagesNancy, FranceSeptember 2022, 1-4
- 12 inproceedingsWatermarking Images in Self-Supervised Latent Spaces.ICASSP 2022 - IEEE International Conference on Acoustics, Speech and Signal ProcessingSingapore, SingaporeIEEEMay 2022, 1-5
- 13 inproceedingsAdversarial images with downscaling.ICIP 2022 - 29th IEEE International Conference on Image ProcessingBordeaux, FranceIEEEOctober 2022, 1-5
- 14 inproceedingsTemporal Disaggregation of the Cumulative Grass Growth.Lecture Notes in Computer Science. LNCS 13364. Part IIICPRAI 2022 - 3rd International Conference on Pattern Recognition and Artificial Intelligence13364Pattern Recognition and Artificial IntelligenceParis, FranceSpringer International PublishingMay 2022, 383-394
- 15 inproceedingsRoSe: A RObust and SEcure Black-Box DNN Watermarking.WIFS 2022 - IEEE 14th International Workshop on Information Forensics and SecurityShanghai, ChinaIEEEDecember 2022, 1-5
- 16 inproceedingsGenerative Cooperative Networks for Natural Language Generation.Proceedings of Machine Learning ResearchICML 2022 - 39th International Conference on Machine Learning162International Conference on Machine Learning, 17-23 July 2022, Baltimore, Maryland, USABaltimore, MD, United StatesPMLR2022, 11891--11905
- 17 inproceedingsFBI: Fingerprinting models with Benign Inputs.CAID 2022 - Conference on Artificial Intelligence for DefenseActes de la 4ème Conference on Artificial Intelligence for Defense (CAID 2022)Rennes, FranceNovember 2022
- 18 inproceedingsRandomized Smoothing under Attack: How Good is it in Pratice?ICASSP 2022 - IEEE International Conference on Acoustics, Speech and Signal ProcessingSingapore, SingaporeIEEEMay 2022, 1-5
- 19 inproceedingsConfronting Active Learning for Relation Extraction to a Real-life Scenario on French Newspaper Data.InterNLP 2022 - 2nd Workshop on Interactive Learning for Natural Language ProcessingProceedings of 2nd Workshop on Interactive Learning for Natural Language Processing, InterNLP@NeurIPS 2022New Orleans, United StatesDecember 2022, 1-7
- 20 inproceedingsDésagrégation temporelle du cumul annuel de croissance de l'herbe.EGC 2022 - Conférence francophone sur l'Extraction et gestion des connaissancesRNTI-E-38EGC 2022Blois, France2022, 27-38
- 21 inproceedingsAlignMixup: Improving Representations By Interpolating Aligned Features.CVPR 2022 - IEEE/CVF Conference on Computer Vision and Pattern RecognitionNew Orleans, United StatesIEEEJune 2022, 1-13
- 22 inproceedingsIt Takes Two to Tango: Mixup for Deep Metric Learning.ICLR 2022 - 10th International Conference on Learning RepresentationsVirtual, FranceApril 2022, 1-21
National peer-reviewed Conferences
- 23 inproceedingsDiscriminator-guided decoding with Monte Carlo Tree Search for constrained text generation.Actes de la 29e Conférence sur le Traitement Automatique des Langues Naturelles. Volume 1 : conférence principaleTALN 2022 - 29e conférence sur le Traitement Automatique des Langues NaturellesAvignon, FranceATALA2022, 27-41
- 24 inproceedingsChoosing The Right Teammate For Cooperative Text Generation.Actes de la 29e Conférence sur le Traitement Automatique des Langues Naturelles. Volume 1 : conférence principaleTALN 2022 - 29e conférence sur le Traitement Automatique des Langues NaturellesAvignon, FranceATALA2022, 12-26
- 25 inproceedingsUne étude statistique des plongements dans les modèles transformers pour le français.Actes de la 29e Conférence sur le Traitement Automatique des Langues Naturelles. Volume 1 : conférence principaleTraitement Automatique des Langues Naturelles (TALN 2022)Avignon, FranceATALA2022, 247-256
- 26 inproceedingsFiltrage et régularisation pour améliorer la plausibilité des poids d'attention dans la tâche d'inférence en langue naturelle.Actes de la 29e Conférence sur le Traitement Automatique des Langues Naturelles. Volume 1 : conférence principaleTALN 2022 - Traitement Automatique des Langues NaturellesAvignon, FranceATALA2022, 95-103
Scientific book chapters
- 27 inbookTraitor tracing.Multimedia securityMarch 2022
- 28 inbookDeep Neural Network Attacks and Defense: The Case of Image Classification.Multimedia Security 1Multimedia Security 1: Authentication and Data Hiding1WileyMarch 2022
Reports & preprints
- 29 miscHDR-LFNet: Inverse Tone Mapping using Fusion Network.March 2022
Other scientific publications
- 30 inproceedingsAIP: Adversarial Interaction Priors for Multi-Agent Physics-based Character Control.SIGGRAPH Asia 2022 - 15th ACM SIGGRAPH Conference and Exhibition on Computer Graphics and Interactive Techniques in AsiaSIGGRAPH Asia 2022 PostersDaegu, South KoreaDecember 2022, 2
11.3 Cited publications
- 31 inproceedingsThe Vulnerability of Learning to Adversarial Perturbation Increases with Intrinsic Dimensionality.WIFS2017
- 32 inproceedingsEstimating Local Intrinsic Dimensionality.KDD2015
- 33 articlePrototyping a Web-Scale Multimedia Retrieval Service Using Spark.ACM TOMCCAP143s2018
- 34 inproceedingsScalability of the NV-tree: Three Experiments.SISAP2018
- 35 inproceedingsSketching techniques for very large matrix factorization.ECIR2016
- 36 inproceedingsConstraint Satisfaction Programming for Video Summarization.ISM2013
- 37 articleWild Patterns: Ten Years After the Rise of Adversarial Machine Learning.Pattern Recognition2018
- 38 phdthesisImage indexing and retrieval using component trees.Université de Bretagne Sud2016
- 39 phdthesisA storytelling machine? : Automatic video summarization: the case of TV series.University of Avignon, France2016
- 40 inproceedingsA Study on Multimodal Video Hyperlinking with Visual Aggregation.ICME2018
- 41 inproceedingsTime Series Retrieval using DTW-Preserving Shapelets.SISAP2018
- 42 articleAudio Adversarial Examples: Targeted Attacks on Speech-to-Text.CoRRabs/1801.019442018
- 43 inproceedingsNumerical eligibility criteria in clinical protocols: annotation, automatic detection and interpretation.AIME2017
- 44 inproceedingsImage Retrieval with Reciprocal and shared Nearest Neighbors.VISAPP2014
- 45 inproceedingsUsing Knowledge Base Semantics in Context-Aware Entity Linking.DocEng 2019 - 19th ACM Symposium on Document EngineeringBerlin, GermanyACMSeptember 2019, 1-10
- 46 bookPhoto Forensics.The MIT Press2016
- 47 articleRecent automatic text summarization techniques: a survey.Artif. Intell. Rev.4712017
- 48 bookDeep Learning.MIT Press2016
- 49 inproceedingsShaping-Up Multimedia Analytics: Needs and Expectations of Media Professionals.MMM, Special Session Perspectives on Multimedia Analytics2016
- 50 inproceedingsScaling Group Testing Similarity Search.ICMR2016
- 51 inproceedingsMining on Manifolds: Metric Learning without Labels.CVPR2018
- 52 inproceedingsA Multi-Dimensional Data Model for Personal Photo Browsing.MMM2015
- 53 inproceedingsTen Research Questions for Scalable Multimedia Analytics.MMM, Special Session Perspectives on Multimedia Analytics2016
- 54 articleDeep Video Portraits.ACM TOG2018
- 55 inproceedingsActive Learning to Assist Annotation of Aerial Images in Environmental Surveys.CBMI2018
- 56 articleIamNN: Iterative and Adaptive Mobile Neural Network for Efficient Image Classification.CoRRabs/1804.101232018
- 57 inproceedingsLearning DTW-Preserving Shapelets.IDA2017
- 58 inproceedingsContext-Aware Forgery Localization in Social-Media Images: A Feature-Based Approach Evaluation.ICIP2018
- 59 inproceedingsConnecting the dots between news articles.KDD2010
- 60 inproceedingsWeakly Supervised Object Localization Using Things and Stuff Transfer.ICCV2017
- 61 inproceedingsUnsupervised part learning for visual recognition.CVPR2017
- 62 inproceedingsMemory Vectors for Particular Object Retrieval with Multiple Queries.ICMR2015
- 63 inproceedingsProvenance filtering for multimedia phylogeny.ICIP2017
- 64 inproceedingsUnsupervised Object Discovery for Instance Recognition.WACV2018
- 65 inproceedingsDeep Metric Learning via Lifted Structured Feature Embedding.CVPR2016
- 66 inproceedingsHighly Efficient Multimedia Event Recounting from User Semantic Preferences.ICMR2014
- 67 articleShow and Tell: Lessons Learned from the 2015 MSCOCO Image Captioning Challenge.TPAMI3942017
- 68 phdthesisDeep Neural Architectures for Automatic Representation Learning from Multimedia Multimodal Data.INSA de Rennes2017
- 69 inproceedingsBidirectional Joint Representation Learning with Symmetrical Deep Neural Networks for Multimodal and Crossmodal Applications.ICMR2016
- 70 inproceedingsGenerative Adversarial Networks for Multimodal Representation Learning in Video Hyperlinking.ICMR2017
- 71 articleMemory Networks.CoRRabs/1410.39162014
- 72 inproceedingsVideo Paragraph Captioning Using Hierarchical Recurrent Neural Networks.CVPR2016
- 73 inproceedingsTowards interactive, intelligent, and integrated multimedia analytics.VAST2014
- 74 inproceedingsCrowd Counting via Scale-Adaptive Convolutional Neural Network.WACV2018
- 75 articleShuffleNet: An Extremely Efficient Convolutional Neural Network for Mobile Devices.CoRRabs/1707.010832017