Keywords
Computer Science and Digital Science
- A5.1.8. 3D User Interfaces
- A5.4. Computer vision
- A5.4.4. 3D and spatio-temporal reconstruction
- A5.4.5. Object tracking and motion analysis
- A5.5.1. Geometrical modeling
- A5.5.4. Animation
- A5.6. Virtual reality, augmented reality
- A6.2.8. Computational geometry and meshes
Other Research Topics and Application Domains
- B2.6.3. Biological Imaging
- B2.8. Sports, performance, motor skills
- B9.2.2. Cinema, Television
- B9.2.3. Video games
- B9.4. Sports
1 Team members, visitors, external collaborators
Research Scientists
- Edmond Boyer [INRIA, Senior Researcher, HDR]
- Stefanie Wuhrer [INRIA, Researcher, HDR]
Faculty Members
- Jean Franco [Team leader, GRENOBLE INP, Associate Professor, HDR]
- Sergi PUJADES [UGA, Associate Professor]
PhD Students
- Jean Basset [University of Bordeaux, ATER, until Jun 2022]
- Nicolas Comte [Anatoscope, CIFRE]
- Anilkumar Erappanakoppal Swamy [NAVER LABS, CIFRE]
- Mathieu Marsot [INRIA]
- Di Meng [INRIA, until Nov 2022]
- Aymen Merrouche [INRIA]
- Rim Rekik Dit Nekhili [INRIA]
- Briac Toussaint [UGA]
- Boyao Zhou [UGA, until Nov 2022]
- Pierre Zins [INRIA]
Technical Staff
- Laurence Boissieux [INRIA]
- Shivam Chandhok [UGA, Engineer, from Apr 2022]
- Abdelmouttaleb Dakri [Inria, Engineer, from Oct 2022]
- Maxime Genisson [INRIA, Engineer, from Sep 2022]
- Julien Pansiot [INRIA]
Administrative Assistant
- Nathalie Gillot [INRIA]
Visiting Scientists
- Kate Duquesne [UNIV GAND, from Sep 2022 until Sep 2022]
- Takumi Kitamura [university of kyushu , from Jun 2022 until Jul 2022]
- Diego Thomas [University of Kyushu, from Apr 2022 until Jul 2022]
External Collaborators
- Marilyn Keller [Max Planck Institute for Intelligent Systems]
- Joao Pedro Cova Regateiro [Inria Rennes]
2 Overall objectives
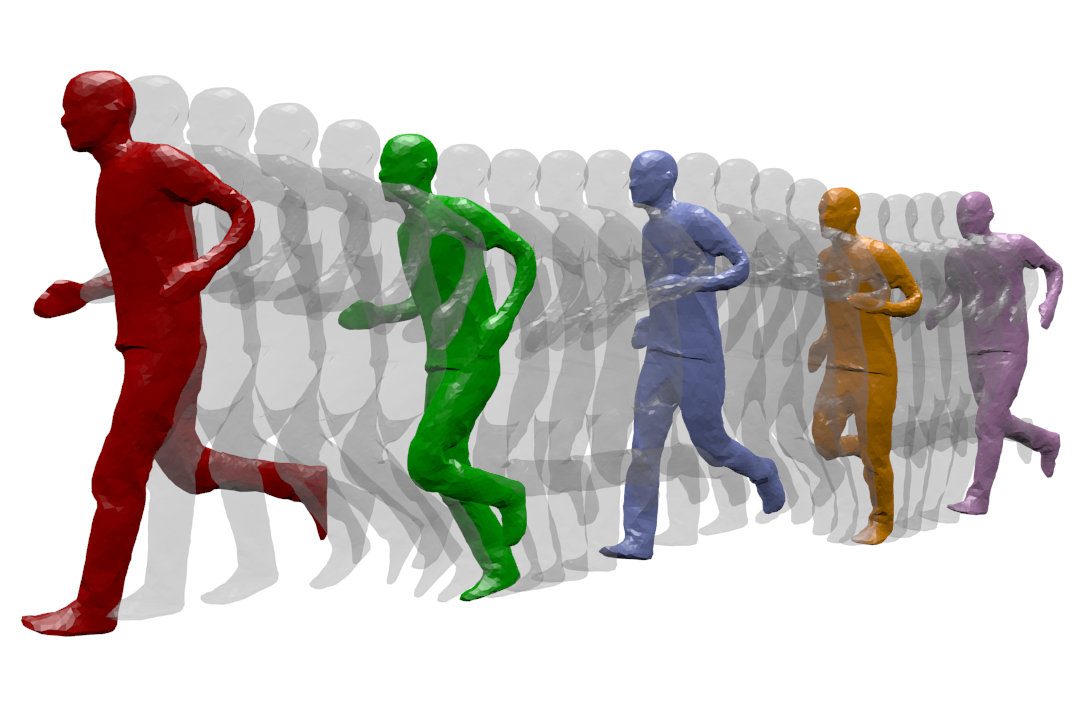
Dynamic Geometry Modeling: a human is captured while running. The different temporal acquisition instances are shown on the same figure.
MORPHEO's ambition is to perceive and interpret shapes that move using multiple camera systems. Departing from standard motion capture systems, based on markers, that provide only sparse information on moving shapes, multiple camera systems allow dense information on both shapes and their motion to be recovered from visual cues. Such ability to perceive shapes in motion brings a rich domain for research investigations on how to model, understand and animate real dynamic shapes, and finds applications, for instance, in gait analysis, bio-metric and bio-mechanical analysis, animation, games and, more insistently in recent years, in the virtual and augmented reality domain. The MORPHEO team particularly focuses on three different axes within the overall theme of 3D dynamic scene vision or 4D vision:
- Shape and appearance models: how to build precise geometric and photometric models of shapes, including human bodies but not limited to, given temporal sequences.
- Dynamic shape vision: how to register and track moving shapes, build pose spaces and animate captured shapes.
- Inside shape vision: how to capture and model inside parts of moving shapes using combined color and X-ray imaging.
- Shape animation: Morpheo is actively investigating animation acquisition and parameterization methodologies for efficient representation and manipulability of acquired 4D data.
The strategy developed by Morpheo to address the mentioned challenges is based on methodological tools that include in particular learning-based approaches, geometry, Bayesian inference and numerical optimization. In recent years, and following many successes in the team and the computer vision community as a whole, a particular effort is ongoing in the team to investigate the use of machine learning and neural learning tools in 4D vision.
3 Research program
3.1 Shape and Appearance Modeling
Standard acquisition platforms, including commercial solutions proposed by companies such as Microsoft, 3dMD or 4DViews, now give access to precise 3D models with geometry, e.g. meshes, and appearance information, e.g. textures. Still, state-of-the-art solutions are limited in many respects: They generally consider limited contexts and close setups with typically at most a few meter side lengths. As a result, many dynamic scenes, even a body running sequence, are still challenging situations; They also seldom exploit time redundancy; Additionally, data driven strategies are yet to be fully investigated in the field. The MORPHEO team builds on the Kinovis platform for data acquisition and has addressed these issues with, in particular, contributions on time integration, in order to increase the resolution for both shapes and appearances, on representations, as well as on exploiting machine learning tools when modeling dynamic scenes. Our originality lies, for a large part, in the larger scale of the dynamic scenes we consider as well as in the time super resolution strategy we investigate. Another particularity of our research is a strong experimental foundation with the multiple camera Kinovis platforms.
3.2 Dynamic Shape Vision
Dynamic Shape Vision refers to research themes that consider the motion of dynamic shapes, with e.g. shapes in different poses, or the deformation between different shapes, with e.g. different human bodies. This includes for instance shape tracking and shape registration, which are themes covered by MORPHEO. While progress has been made over the last decade in this domain, challenges remain, in particular due to the required essential task of shape correspondence that is still difficult to perform robustly. Strategies in this domain can be roughly classified into two categories: (i) data driven approaches that learn shape spaces and estimate shapes and their variations through space parameterizations; (ii) model based approaches that use more or less constrained prior models on shape evolutions, e.g. locally rigid structures, to recover correspondences. The MORPHEO team is substantially involved in both categories. The second one leaves more flexibility for shapes that can be modeled, an important feature with the Kinovis platform, while the first one is interesting for modeling classes of shapes that are more likely to evolve in spaces with reasonable dimensions, such as faces and bodies under clothing. The originality of MORPHEO in this axis is to go beyond static shape poses and to consider also the dynamics of shape over several frames when modeling moving shapes, and in particular with shape tracking, animation and, more recently, face registration.
3.3 Inside Shape Vision
Another research axis is concerned with the ability to perceive inside moving shapes. This is a more recent research theme in the MORPHEO team that has gained importance. It was originally the research associated to the Kinovis platform installed in the Grenoble Hospitals. This platform is equipped with two X-ray cameras and ten color cameras, enabling therefore simultaneous vision of inside and outside shapes. We believe this opens a new domain of investigation at the interface between computer vision and medical imaging. Interesting issues in this domain include the links between the outside surface of a shape and its inner parts, especially with the human body. These links are likely to help understanding and modeling human motions. Until now, numerous dynamic shape models, especially in the computer graphics domain, consist of a surface, typically a mesh, rigged to a skeletal structure that is never observed in practice but allows to parameterize human motion. Learning more accurate relationships using observations can therefore significantly impact the domain.
3.4 Shape Animation
3D animation is a crucial part of digital media production with numerous applications, in particular in the game and motion picture industry. Recent evolutions in computer animation consider real videos for both the creation and the animation of characters. The advantage of this strategy is twofold: it reduces the creation cost and increases realism by considering only real data. Furthermore, it allows to create new motions, for real characters, by recombining recorded elementary movements. In addition to enable new media contents to be produced, it also allows to automatically extend moving shape datasets with fully controllable new motions. This ability appears to be of great importance with the recent advent of deep learning techniques and the associated need for large learning datasets. In this research direction, we investigate how to create new dynamic scenes using recorded events. More recently, this also includes applying machine learning to datasets of recorded human motions to learn motion spaces that allow to synthesize novel realistic animations.
4 Application domains
4.1 4D modeling
Modeling shapes that evolve over time, analyzing and interpreting their motion has been a subject of increasing interest of many research communities including the computer vision, the computer graphics and the medical imaging communities. Recent evolutions in acquisition technologies including 3D depth cameras (Time-of-Flight and Kinect), multi-camera systems, marker based motion capture systems, ultrasound and CT scanners have made those communities consider capturing the real scene and their dynamics, create 4D spatio-temporal models, analyze and interpret them. A number of applications including dense motion capture, dynamic shape modeling and animation, temporally consistent 3D reconstruction, motion analysis and interpretation have therefore emerged.
4.2 Shape Analysis
Most existing shape analysis tools are local, in the sense that they give local insight about an object's geometry or purpose. The use of both geometry and motion cues makes it possible to recover more global information, in order to get extensive knowledge about a shape. For instance, motion can help to decompose a 3D model of a character into semantically significant parts, such as legs, arms, torso and head. Possible applications of such high-level shape understanding include accurate feature computation, comparison between models to detect defects or medical pathologies, and the design of new biometric models.
4.3 Human Motion Analysis
The recovery of dense motion information enables the combined analysis of shapes and their motions. Typical examples include the estimation of mean shapes given a set of 3D models or the identification of abnormal deformations of a shape given its typical evolutions. The interest arises in several application domains where temporal surface deformations need to be captured and analyzed. It includes human body analyses for which potential applications are anyway numerous and important, from the identification of pathologies to the design of new prostheses.
4.4 Virtual and Augmented Reality
This domain has actually seen new devices emerge that enable now full 3D visualization, for instance the HTC Vive, the Microsoft Hololens and the Magic leap one. These devices create a need for adapted animated 3D contents that can either be generated or captured. We believe that captured 4D models will gain interest in this context since they provide realistic visual information on moving shapes that tend to avoid negative perception effects such as the uncanny valley effect. Furthermore, captured 4D models can be exploited to learn motion models that allow for realistic generation, for instance in the important applicative case of generating realistically moving human avatars. Besides 3D visualization devices, many recent applications also rely on everyday devices, such as mobile phones, to display augmented reality contents with free viewpoint ability. In this case, 3D and 4D contents are also expected.
5 Social and environmental responsibility
5.1 Footprint of research activities
We are concerned by the environmental impact of our research. While we have yet no tools to exactly measure the footprint of our activities, we are anyway evolving our practice on different aspects.
Dissemination strategy: Traditionally, Morpheo's dissemination strategy has been to publish in the top conferences of the field (CVPR, ECCV, ICCV, MICCAI), as well as to give invited talks at various locations all over the world, leading therefore to sometimes long disance work trips. Even if Morpheo's members have never been frequent long distance travelers their practice has changed. As a result of the Covid-19 crisis, our research community has started in 2020 to experiment virtual conferences. These digital meetings have some merits: beyond the obvious advantage of reduced travel (leading to fewer emissions and improved work-life balance), they democratize attendance as attending has a fraction of the previous cost. On the other hand, an important drawback is that digital attendance leads to low-quality exchanges between participants as on-line discussions can be often difficult, which actually tend to reduce the global attendance. Within the team we currently try to increase submissions to top journals in the field directly, hence reducing travels, while still attending some in-person conferences or seminars to allow for networking.
Data management: The data produced by the Morpheo team occupies large memory volumes. The Kinovis platform at Inria Grenoble typically produces around 1,5GB per second when capturing one actor at 50fps. The platform that also captures X-ray images at CHU produces around 1.3GB of data per second at 30fps for video and X-rays. For practical reasons, we reduce the data as much as possible with respect to the targeted application by only keeping e.g. 3D reconstructions or down-sampled spatial or temporal camera images. Yet, acquiring, processing and storing these data is costly in terms of resources. In addition, the data captured by these platforms are personal data with highly constrained regulations. Our data management therefore needs to consider multiple aspects: data encryption to protect personal information, data backup to allow for reproducibility, and the environmental impact of data storage and processing. For all these aspects, we are constantly checking for new and more satisfactory solutions.
For data processing, we rely heavily on cluster uses, in particular with neural networks which are known to have a heavy carbon footprint. Yet, in our research field, these types of processing methods have been shown to lead to significant performance gains. For this reason, we continue to use neural networks as tools, while attempting to use architectures that allow for faster and more energy efficient training, and simple test cases that can often be trained on local machines (with GPU) to allow for less costly network design. A typical example of this type of research is with the work of Briac Toussaint, whose first thesis contribution exhibits a high quality reconstruction algorithm for the Kinovis platform, with under a minute of GPU computation time per frame, an order of magnitude faster than our previous research solution, yet it achieves improved precision 10.
5.2 Impact of research results
Morpheo's main research topic is not related to sustainability. Yet, some of our research directions could be applied to solve problems relevant to sustainability such as:
- Realistic virtual avatar creation holds the potential to allow for realistic interactions through large geographic distances, leading to more realistic and satisfactory teleconferencing systems. As has been observed during the Covid-19 crisis, such systems can remove the need for traveling, thereby preventing costs and pollution and preserving resources. If avatars were to allow for more realistic interactions than existing teleconferencing systems, there is hope that the need to travel can be reduced even in times without health emergency. Morpheo captures and analyzes humans in 4D, thereby allowing to capture both their shapes and movement patterns, and actively works on human body modeling. These research directions tackle problems that are highly relevant for the creation of digital avatars that do not fall into the uncanny valley (even during movement). In this line of work, Morpheo particpated in the IPL Avatar project, in particular with the work of the Ph.D. student Jean Basset, and will follow up with the Nemo.AI joint lab with Interdigital on advancing this topic.
- Modeling and analyzing humans in clothing holds the potential to reduce the rate of returns of online sales in the clothing industry, which are currently high. In particular, highly realistic dynamic clothing models could help a consumer better evaluate the fit and dynamic aspects of the clothing. Morpheo's interest includes the analysis of clothing deformations based on 4D captures. Preliminary results have been achieved in the past (works of the former Ph.D. student Jinlong Yang), and we plan to continue investigating this line of work. To this end, our plans for future work include the capture a large-scale dataset of humans in different clothing items performing different motions in collaboration with NaverLabs Research. No such dataset exists to date, and it would allow the research community to study the dynamic effects of clothing.
Of course, as with any research direction, ours can also be used to generate technologies that are resource-hungry and whose necessity may be questionable, such as inserting animated 3D avatars in scenarios where simple voice communication would suffice, for instance.
6 Highlights of the year
6.1 Dagstuhl organisation
Stefanie Wuhrer co-organized Dagstuhl seminar 3D Morphable Models and Beyond, Dagstuhl, Germany, March 20 – 25, 2022.
7 New software and platforms
7.1 New software
7.1.1 MotionRetargeter
-
Name:
Correspondence-free online human motion retargeting
-
Keywords:
3D animation, 3D modeling
-
Functional Description:
Allows to retarget the motion of one human body to another body shape, without needing any correspondence information.
-
Contact:
Rim Rekik Dit Nekhili
7.1.2 MVPIFu
-
Name:
Multi-View Pixel-aligned Implicit Function
-
Keyword:
3D reconstruction
-
Functional Description:
Software for 3D reconstruction of dressed humans from a few sparse views.
- URL:
- Publication:
-
Contact:
Pierre Zins
7.1.3 HumanMotionSpace
-
Name:
A Structured Latent Space for Human Body Motion Generation
-
Keywords:
3D movement, Synthetic human
-
Functional Description:
Code allows to reproduce the results presented in scientific publication
M. Marsot, S. Wuhrer, J.-S. Franco, S. Durocher A Structured Latent Space for Human Body Motion Generation International Conference on 3D Vision, 2022 https://hal.archives-ouvertes.fr/hal-03250297
In particular, the code allows to learn a structured latent motion space for 3D human bodies and to use it for different tasks, including human motion completion.
- URL:
-
Contact:
Mathieu Marsot
7.1.4 FastCapDR
-
Name:
Fast Differentiable Rendering based Surface Capture
-
Keywords:
3D reconstruction, 3D, Differentiable Rendering, Synthetic human, Computer vision, Multi-View reconstruction
-
Scientific Description:
Code to the article "Fast Gradient Descent for Surface Capture with Differentiable Rendering", 3DV 2022
-
Functional Description:
This software combines differentiable rendering with sparse coarse-to-fine estimation and storage techniques on GPU to obtain a unique combination of high precision captured surfaces and maximal efficiency, yielding excellent reconstruction results in under a minute. Very high quality single-human surface reconstructions were obtained in less than 20 seconds for 68 cameras of the Inria Kinovis acquisition platform (with a single NVidia RTX A6000 GPUs).
- URL:
-
Contact:
Briac Toussaint
7.1.5 OSSO
-
Name:
Obtainig skeletal Shape from Outside
-
Keyword:
3D modeling
-
Functional Description:
Given the shape of a person as a SMPL body mesh, OSSO extracts the mesh of the corresponding skeletal structure
- URL:
-
Contact:
Sergi PUJADES
-
Partner:
Max Planck Institute for Intelligent Systems
7.1.6 VertSegId
-
Keywords:
Image segmentation, Medical imaging, 3D
-
Functional Description:
This software segments and identifies vertebrae in a volumetric CT image.
- URL:
-
Contact:
Sergi PUJADES
7.1.7 HumanCompletePST
-
Name:
Pyramidal Signed Distance Learning for Spatio-Temporal Human Shape Completion
-
Keywords:
3D reconstruction, 4D, Point cloud, Depth Perception, 3D
-
Scientific Description:
This repository contains the code of the paper Pyramidal Signed Distance Learning for Spatio-Temporal Human Shape Completion, published at ACCV2022.
-
Functional Description:
This software processes sequences of monocular, single-view obtained point clouds of humans, and provides a closed shape mesh as output for each frame. Analysis is performed with a pyramidal, semi-implicit depth inference deep network, on temporal windows of typically 4-8 frames.
- URL:
-
Contact:
Jean Franco
8 New results
8.1 Pyramidal Signed Distance Learning for Spatio-Temporal Human Shape Completion
Participants: Boyao Zhou, Jean-Sébastien Franco, Martin Delagorce, Edmond Boyer.
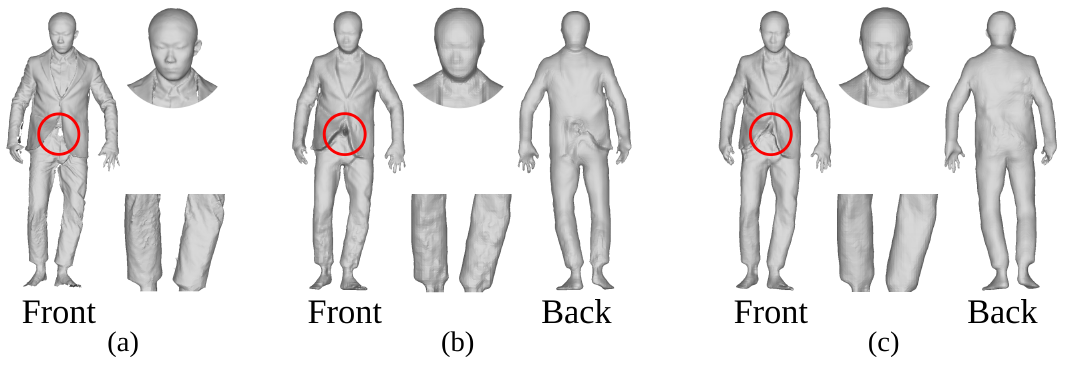
In this figure, we show the shape completion ability from partial front depth scan, on an instance of the CAPE dataset.
This work addresses the problem of completing partial human shape observations as obtained with a depth camera. Existing methods that solve this problem can provide robustness, with for instance model-based strategies that rely on parametric human models, or precision, with learning approaches that can capture local geometric patterns using implicit neural representations. We investigate how to combine both properties with a novel pyramidal spatio-temporal learning model. This model exploits neural signed distance fields in a coarse-to-fine manner, in order to benefit from the ability of implicit neural representations to preserve local geometry details while enforcing more global spatial consistency for the estimated shapes through features at coarser levels. In addition, our model also leverages temporal redundancy with spatiotemporal features that integrate information over neighboring frames. Experiments on standard datasets show that both the coarse-to-fine and temporal aggregation strategies contribute to outperform the state-of-the-art methods on human shape completion. This work was published at Asian Conference on Computer Vision 11, and the software is made available to the research community (7.1.7).
8.2 Fast Gradient Descent for Surface Capture Via Differentiable Rendering
Participants: Briac Toussaint, Maxime Genisson, Jean-Sébastien Franco.
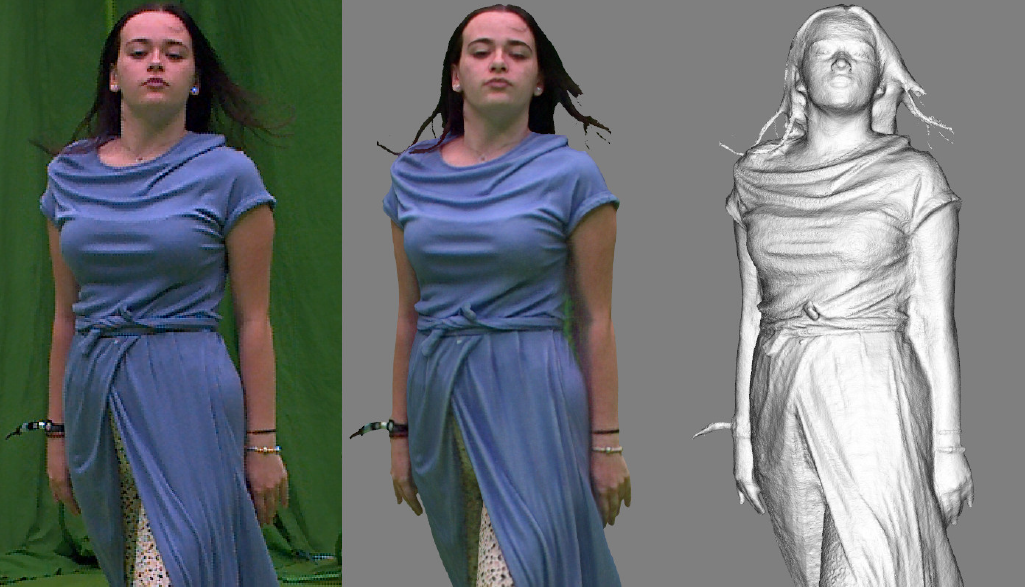
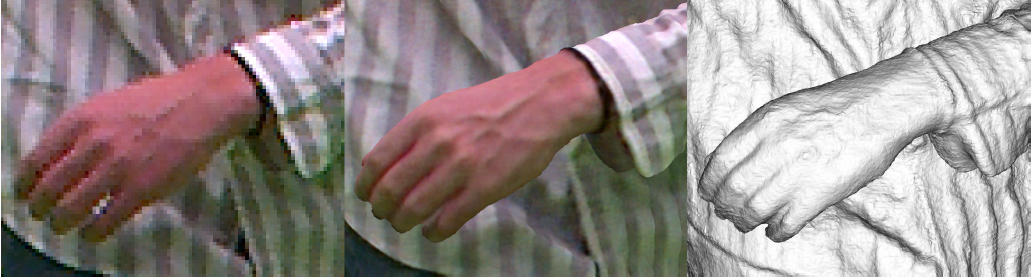
Differential rendering has recently emerged as a powerful tool for image-based rendering or geometric reconstruction from multiple views, with very high quality. Up to now, such methods have been benchmarked on generic object databases and promisingly applied to some real data, but have yet to be applied to specific applications that may benefit. In this paper, we investigate how a differential rendering system can be crafted for raw multi-camera performance capture. We address several key issues in the way of practical usability and reproducibility, such as processing speed, explainability of the model, and general output model quality. This leads us to several contributions to the differential rendering framework. In particular we show that a unified view of differential rendering and classic optimization is possible, leading to a formulation and implementation where complete non-stochastic gradient steps can be analytically computed and the full per frame data stored in video memory, yielding a straightforward and efficient implementation. We also use a sparse storage and coarse-to-fine scheme to achieve extremely high resolution with contained memory and computation time. We show experimentally that results rivaling in quality with state of the art multi-view human surface capture methods are achievable in a fraction of the time, typically around a minute per frame. This work was published at the 3D Visions conference 2022 10. The code for this work is made available to the community (7.1.4).
8.3 Temporal Shape Transfer Network for 3D Human Motion
Participants: João Regateiro, Edmond Boyer.
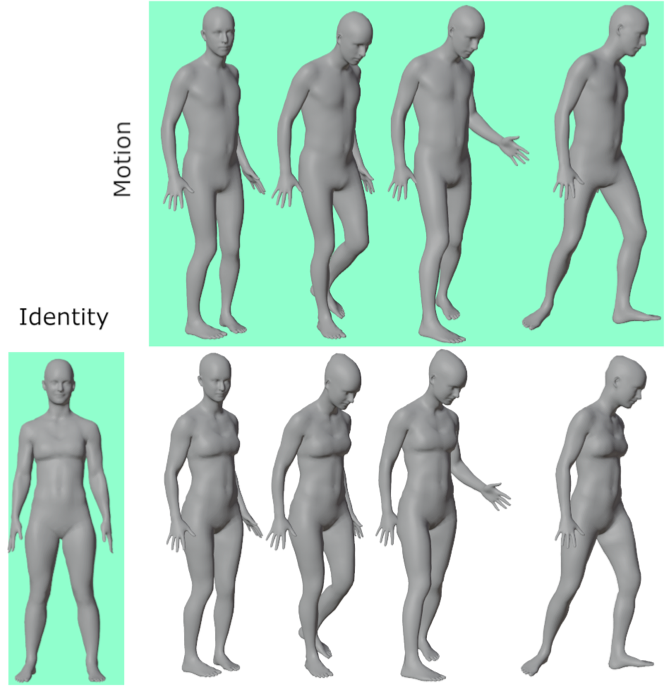
This work presents a learning-based approach to perform human shape transfer between an arbitrary 3D identity mesh and a temporal motion sequence of 3D meshes. Recent approaches tackle the human shape and pose transfer on a per-frame basis and do not yet consider the valuable information about the motion dynamics, e.g., body or clothing dynamics, inherently present in motion sequences. Recent datasets provide such sequences of 3D meshes, and this work investigates how to leverage the associated intrinsic temporal features in order to improve learning-based approaches on human shape transfer. These features are expected to help preserve temporal motion and identity consistency over motion sequences. To this aim, we introduce a new network architecture that takes as input successive 3D mesh frames in a motion sequence and which decoder is conditioned on the target shape identity. Training losses are designed to enforce temporal consistency between poses as well as shape preservation over the input frames. Experiments demonstrate substantially qualitative and quantitative improvements in using temporal features compared to optimization-based and recent learning-based methods. This work was published at the 3D Visions conference 2022 9.
8.4 A Structured Latent Space for Human Body Motion Generation
Participants: Mathieu Marsot, Stefanie Wuhrer, Jean-Sébastien Franco, Stéphane Durocher.
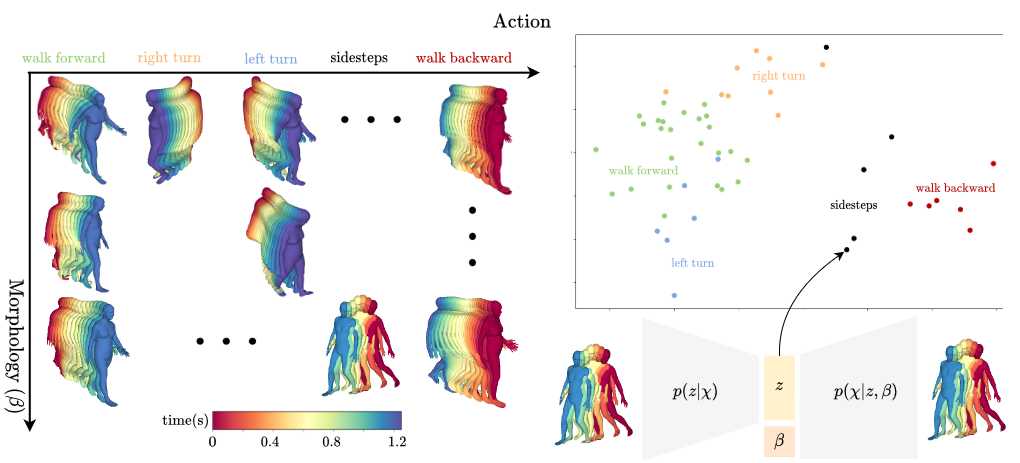
We propose a framework to learn a structured latent space to represent 4D human body motion, where each latent vector encodes a full motion of the whole 3D human shape. On one hand several data-driven skeletal animation models exist proposing motion spaces of temporally dense motion signals, but based on geometrically sparse kinematic representations. On the other hand many methods exist to build shape spaces of dense 3D geometry, but for static frames. We bring together both concepts, proposing a motion space that is dense both temporally and geometrically. Once trained, our model generates a multi-frame sequence of dense 3D meshes based on a single point in a low-dimensional latent space. This latent space is built to be structured, such that similar motions form clusters. It also embeds variations of duration in the latent vector, allowing semantically close sequences that differ only by temporal unfolding to share similar latent vectors. We demonstrate experimentally the structural properties of our latent space, and show it can be used to generate plausible interpolations between different actions. We also apply our model to 4D human motion completion, showing its promising abilities to learn spatio-temporal features of human motion. This work was published at the International Conference on 3D Vision 8. The software demonstrator for this work is made available to the community (7.1.3).
8.5 Impact of Self-Contacts on Perceived Pose Equivalences
Participants: Jean Basset, Badr Ouannas, Ludovic Hoyet, Franck Multon, Stefanie Wuhrer.
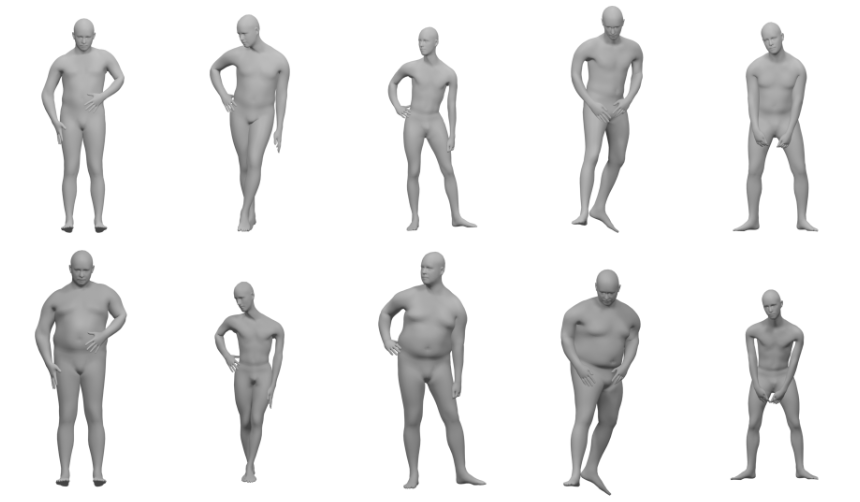
Defining equivalences between poses of different human characters is an important problem for imitation research, human pose recognition and deformation transfer. However, pose equivalence is a subjective information that depends on context and on the morphology of the characters. A common hypothesis is that interactions between body surfaces, such as self-contacts, are important attributes of human poses, and are therefore consistently included in animation approaches aiming at retargeting human motions. However, some of these self-contacts are only present because of the morphology of the character and are not important to the pose, e.g. contacts between the upper arms and the torso during a standing A-pose. In this work, we conduct a first study towards the goal of understanding the impact of self-contacts between body surfaces on perceived pose equivalences. More specifically, we focus on contacts between the arms or hands and the upper body, which are frequent in everyday human poses. We conduct a study where we present to observers two models of a character mimicking the pose of a source character, one with the same self-contacts as the source, and one with one self-contact removed, and ask observers to select which model best mimics the source pose. We show that while poses with different self-contacts are considered different by observers in most cases, this effect is stronger for self-contacts involving the hands than for those involving the arms. This work was published at the International Conference for Motion in Games 6.
8.6 A Visual Approach to Measure Cloth-Body and Cloth-Cloth Friction
Participants: Abdullah-Haroon Rasheed, Victor Romero, Florence Bertails-Descoubes, Stefanie Wuhrer, Jean-Sébastien Franco, Arnaud Lazarus.
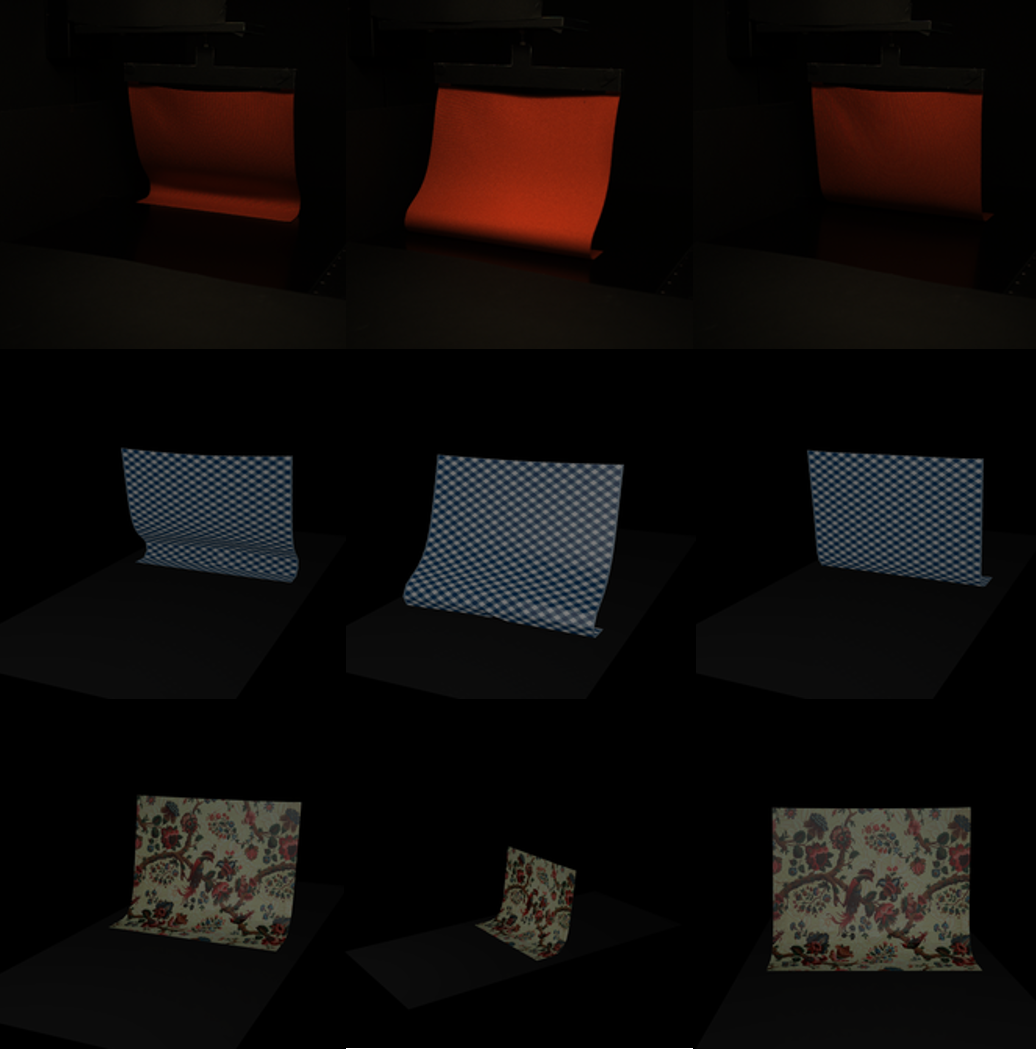
Measuring contact friction in soft-bodies usually requires a specialised physics bench and a tedious acquisition protocol. This makes the prospect of a purely non-invasive, video-based measurement technique particularly attractive. Previous works have shown that such a video-based estimation is feasible for material parameters using deep learning, but this has never been applied to the friction estimation problem which results in even more subtle visual variations. Because acquiring a large dataset for this problem is impractical, generating it from simulation is the obvious alternative. However, this requires the use of a frictional contact simulator whose results are not only visually plausible, but physically-correct enough to match observations made at the macroscopic scale. In this paper, which is an extended version of our former work [31], we propose to our knowledge the first non-invasive measurement network and adjoining synthetic training dataset for estimating cloth friction at contact, for both cloth-hard body and cloth-cloth contacts. To this end we build a protocol for validating and calibrating a state-of-the-art frictional contact simulator, in order to produce a reliable dataset. We furthermore show that without our careful calibration procedure, the training fails to provide accurate estimation results on real data. We present extensive results on a large acquired test set of several hundred real video sequences of cloth in friction, which validates the proposed protocol and its accuracy. This result was published in IEEE Transactions on Pattern Analysis and Machine Intelligenge 5.
8.7 OSSO: Obtaining Skeletal Shape from Outside
Participants: Marilyn Keller, Silvia Zuffi, Michael Black, Sergi Pujades.
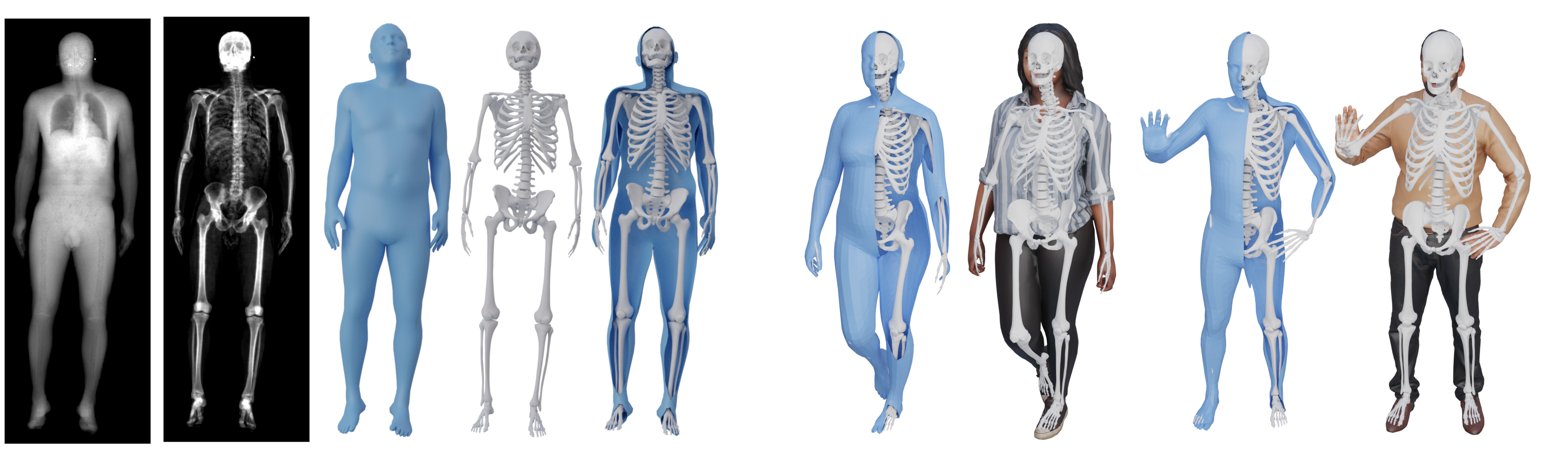
We address the problem of inferring the anatomic skeleton of a person, in an arbitrary pose, from the 3D surface of the body; i.e. we predict the inside (bones) from the outside (skin). This has many applications in medicine and biomechanics. Existing state-of-the-art biomechanical skeletons are detailed but do not easily generalize to new subjects. Additionally, computer vision and graphics methods that predict skeletons are typically heuristic, not learned from data, do not leverage the full 3D body surface, and are not validated against ground truth. To our knowledge, our system, called OSSO (Obtaining Skeletal Shape from Outside), is the first to learn the mapping from the 3D body surface to the internal skeleton from real data. We do so using 1000 male and 1000 female dual-energy X-ray absorptiometry (DXA) scans. To these, we fit a parametric 3D body shape model (STAR) to capture the body surface and a novel part-based 3D skeleton model to capture the bones. This provides inside/outside training pairs. We model the statistical variation of full skeletons using PCA in a pose-normalized space. We then train a regressor from body shape parameters to skeleton shape parameters and refine the skeleton to satisfy constraints on physical plausibility. Given an arbitrary 3D body shape and pose, OSSO predicts a realistic skeleton inside. In contrast to previous work, we evaluate the accuracy of the skeleton shape quantitatively on held out DXA scans, outperforming the state-of-the art. We also show 3D skeleton prediction from varied and challenging 3D bodies. The code to infer a skeleton from a body shape is available for research, and the dataset of paired outer surface (skin) and skeleton (bone) meshes are available as a Biobank Returned Dataset. This research has been conducted using the UK Biobank Resource. This result was published at the Computer Vision and Pattern Recognition conference 7 and the OSSO software is made available to the community (7.1.5) (Project page link).
8.8 Vertebrae localization, segmentation and identification using a graph optimization and an anatomic consistency cycle
Participants: Di Meng, Eslam Mohammed, Edmond Boyer, Sergi Pujades.
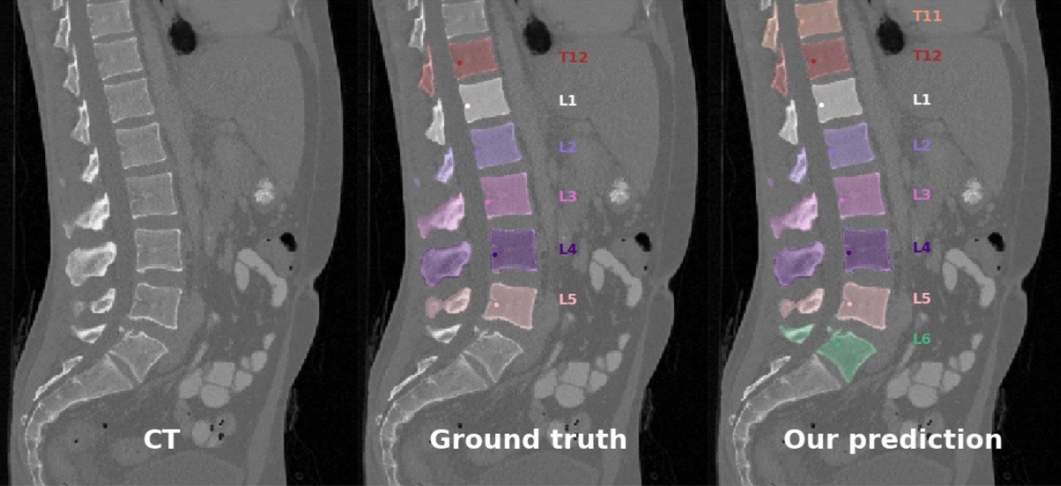
Vertebrae localization, segmentation and identification in CT images is key to numerous clinical applications. While deep learning strategies have brought to this field significant improvements over recent years, transitional and pathological vertebrae are still plaguing most existing approaches as a consequence of their poor representation in training datasets. Alternatively, proposed non-learning based methods take benefit of prior knowledge to handle such particular cases. In this work we propose to combine both strategies. To this purpose we introduce an iterative cycle in which individual vertebrae are recursively localized, segmented and identified using deep networks, while anatomic consistency is enforced using statistical priors. In this strategy, the transitional vertebrae identification is handled by encoding their configurations in a graphical model that aggregates local deep-network predictions into an anatomically consistent final result. Our approach achieves the state-of-the-art results on the VerSe20 challenge benchmark, and outperforms all methods on transitional vertebrae as well as the generalization to the VerSe19 challenge benchmark. Furthermore, our method can detect and report inconsistent spine regions that do not satisfy the anatomic consistency priors. The code and model are available for research purposes (7.1.6). This result was published at the Computer Vision and Pattern Recognition conference 14.
9 Bilateral contracts and grants with industry
9.1 Bilateral contracts with industry
Participants: Boyao Zhou, Pierre Zins, Stefanie Wuhrer, Jean-Sébastien Franco, Edmond Boyer.
- The Morpheo INRIA team and Microsoft research set up a collaboration on the capture and modelling of moving shapes using multiple videos. Two PhD works are part of this collaboration with the objective to make contributions on 4D Modeling. The PhDs take place at Inria Grenoble Rhône-Alpes and involve visits and stays at Microsoft in Cambridge (UK) and Zurich (CH). The collaboration is part of the Microsoft Research - Inria Joint Centre. One of the PhD (Matthieu Armendo) has finished in 2021 and the other PhD (Boyao Zhou) is pursuing has finished in November 2022.
- The Morpheo INRIA team has another collaboration with Meta reality lab in San Francisco. The collaboration involves one PhD who is currently at the INRIA Grenoble Rhône-Alpes working on the estimation of shape and appearance from a single image. The collaboration started in 2019 and is ongoing with the PhD of Pierre Zins.
- The Morpheo INRIA team has a collaboration with Interdigital in Rennes through the Nemo.AI joint lab. The kickoff to this collaboration has happened in November 2022. The collaboration will involve two PhD co-supervisions starting in 2023, one in INRIA Rennes and one at the INRIA de l'Université Grenoble Alpes. The subject of the collaboration revolves around digital humans, one the one hand to estimate the clothing of humans from images, and on the other to estimate hair models from one or several videos.
9.2 Bilateral grants with industry
Participants: Sergi Pujades Rocamora, Edmond Boyer, Jean-Sébastien Franco.
- The Morpheo INRIA team collaborates with the local start-up Anatoscope created by former researchers at INRIA Grenoble. The collaboration involves one CIFRE PhD (Nicolas Comte) who shares his time between Morpheo and Anatoscope offices. He is working on new classifications methods of the scoliose disease that take the motion of the patient into account in addition to their morphology. The collaboration started in 2020.
- The Morpheo team is also involved in a CIFRE PhD co-supervision (Anilkumar Swamy) with Naver Labs since July 2021, with Gregory Rogez, Mathieu Armando and Vincent Leroy. The work revolves around monocular hand-object reconstruction by showing the object to the camera.
10 Partnerships and cooperations
10.1 International initiatives
10.1.1 Participation in other International Programs
ShapeUp! Keiki
Participants: Sergi Pujades, Abdel Dakri.
- Title: ShapeUp! Keiki
-
Partner Institution(s):
- Inria Grenoble,France.
- University of Hawai'i Cancer Center, United States.
- Pennington Biomedical Research Center, Lousiana State University, United States.
- Date/Duration: 2022-2026
-
Additionnal info/keywords:
The long term goal of the Shape Up! Keiki is 1) to provide pediatric phenotype descriptors of health using body shape, and 2) to provide the tools to visualize and quantify body shape in research and clinical practice. Our overall approach is to first derive predictive models of how body shape relates to regional and total body composition (trunk fat, muscle mass, lean mass, and percent fat) with consideration to the rapidly changing hydration over the early years of life (birth to 5 years). We will then identify covariates that impact the precision and accuracy of our optical models. We will then show how our optical body composition estimates are associated with important developmental and dietary factors. Our central hypothesis is that optical estimates of body composition suitably represent a 5-compartment (5C) body composition model for studies of adiposity and health in young children and are superior to that of simple anthropometry and demographics. The rationale for this study is that early life access to accurate body composition data will enable identification of factors that increase obesity, metabolic disease, and cancer risk, and provide a means to target interventions to those that would benefit. The expected outcome is that our findings would be immediately applicable to accessible gaming (4) and imaging sensors found on modern computers.
10.2 International research visitors
10.2.1 Visits of international scientists
Other international visits to the team
Diego Thomas
-
Status:
Associate Professor
-
Institution of origin:
Kyushu University, Fukuoka
-
Country:
Japan
-
Dates:
April - July 2022
-
Context of the visit:
Collaboration with Morpheo team
-
Mobility program/type of mobility:
Japanese mobility program
Takumi Kitamura
-
Status:
Ph.D. student
-
Institution of origin:
Kyushu University, Fukuoka
-
Country:
Japan
-
Dates:
June - July 2022
-
Context of the visit:
Collaboration with Morpheo team
-
Mobility program/type of mobility:
Japanese mobility program
Kristijan Bartol
-
Status:
Ph.D. student
-
Institution of origin:
University of Zagreb
-
Country:
Croatia
-
Dates:
September - October 2022
-
Context of the visit:
Collaboration with Morpheo team
-
Mobility program/type of mobility:
Croatian mobility program
David Bojanić
-
Status:
Ph.D. student
-
Institution of origin:
University of Zagreb
-
Country:
Croatia
-
Dates:
September - October 2022
-
Context of the visit:
Collaboration with Morpheo team
-
Mobility program/type of mobility:
Croatian mobility program
Kate Duquesne
-
Status:
Ph.D. student
-
Institution of origin:
University of Gent
-
Country:
Belgium
-
Dates:
September - October 2022
-
Context of the visit:
Collaboration with Morpheo team
-
Mobility program/type of mobility:
Belgium mobility program
10.3 National initiatives
10.3.1 ANR
ANR JCJC SEMBA – Shape, Motion and Body composition to Anatomy.
Existing medical imaging techniques, such as Computed Tomography (CT), Dual Energy X-Ray Absorption (DEXA) and Magnetic Resonance Imaging (MRI), allow to observe internal tissues (such as adipose, muscle, and bone tissues) of in-vivo patients. However, these imaging modalities involve heavy and expensive equipment as well as time consuming procedures. External dynamic measurements can be acquired with optical scanning equipment, e.g. cameras or depth sensors. These allow high spatial and temporal resolution acquisitions of the surface of living moving bodies. The main research question of SEMBA is: "can the internal observations be inferred from the dynamic external ones only?". SEMBA’s first hypothesis is that the quantity and distribution of adipose, muscle and bone tissues determine the shape of the surface of a person. However, two subjects with a similar shape may have different quantities and distributions of these tissues. Quantifying adipose, bone and muscle tissue from only a static observation of the surface of the human might be ambiguous. SEMBA's second hypothesis is that the shape deformations observed while the body performs highly dynamic motions will help disambiguating the amount and distribution of the different tissues. The dynamics contain key information that is not present in the static shape. SEMBA’s first objective is to learn statistical anatomic models with accurate distributions of adipose, muscle, and bone tissue. These models are going to be learned by leveraging medical dataset containing MRI and DEXA images. SEMBA's second objective will be to develop computational models to obtain a subject-specific anatomic model with an accurate distribution of adipose, muscle, and bone tissue from external dynamic measurements only.
ANR JCJC 3DMOVE - Learning to synthesize 3D dynamic human motion.
It is now possible to capture time-varying 3D point clouds at high spatial and temporal resolution. This allows for high-quality acquisitions of human bodies and faces in motion. However, tools to process and analyze these data robustly and automatically are missing. Such tools are critical to learning generative models of human motion, which can be leveraged to create plausible synthetic human motion sequences. This has the potential to influence virtual reality applications such as virtual change rooms or crowd simulations. Developing such tools is challenging due to the high variability in human shape and motion and due to significant geometric and topological acquisition noise present in state-of-the-art acquisitions. The main objective of 3DMOVE is to automatically compute high-quality generative models from a database of raw dense 3D motion sequences for human bodies and faces. To achieve this objective, 3DMOVE will leverage recently developed deep learning techniques. The project also involves developing tools to assess the quality of the generated motions using perceptual studies. This project currently involves two Ph.D. students: Mathieu Marsot hired in November 2019 and Rim Rekik-Nkhili hired November 2021.
ANR Human4D - Acquisition, Analysis and Synthesis of Human Body Shape in Motion
Human4D is concerned with the problem of 4D human shape modeling. Reconstructing, characterizing, and understanding the shape and motion of individuals or groups of people have many important applications, such as ergonomic design of products, rapid reconstruction of realistic human models for virtual worlds, and an early detection of abnormality in predictive clinical analysis. Human4D aims at contributing to this evolution with objectives that can profoundly improve the reconstruction, transmission, and reuse of digital human data, by unleashing the power of recent deep learning techniques and extending it to 4D human shape modeling. It involves 4 academic partners: The laboratory ICube at Strasbourg which is leading the project, the laboratory Cristal at Lille, the laboratory LIRIS at Lyon and the INRIA Morpheo team.
ANR Equipex+ CONTINUUM - Collaborative continuum from digital to human
The CONTINUUM project will create a collaborative research infrastructure of 30 platforms located throughout France, including Inria Grenoble's Kinovis, to advance interdisciplinary research based on interaction between computer science and the human and social sciences. Thanks to CONTINUUM, 37 research teams will develop cutting-edge research programs focusing on visualization, immersion, interaction and collaboration, as well as on human perception, cognition and behaviour in virtual/augmented reality, with potential impact on societal issues. CONTINUUM enables a paradigm shift in the way we perceive, interact, and collaborate with complex digital data and digital worlds by putting humans at the center of the data processing workflows. The project will empower scientists, engineers and industry users with a highly interconnected network of high-performance visualization and immersive platforms to observe, manipulate, understand and share digital data, real-time multi-scale simulations, and virtual or augmented experiences. All platforms will feature facilities for remote collaboration with other platforms, as well as mobile equipment that can be lent to users to facilitate onboarding.
ANR PRC Inora
The INORA project aims at understanding the mechanisms of action of shoes and orthotic insoles on Rheumatoid arthritis (RA) patients through patient-specific computational biomechanical models. These models will help in uncovering the mechanical determinants to pain relief, which will enable the long-term well-being of patients. Motivated by the numerous studies highlighting erosion and joint space narrowing in RA patients, we postulate that a significant contributor to pain is the internal joint loading when the foot is inflamed. This hypothesis dictates the need of a high-fidelity volumetric segmentation for the construction of the patient-specific geometry. It also guides the variables of interests in the exploitation of a finite element (FE) model. The INORA project aims at providing numerical tools to the scientific, medical and industrial communities to better describe the mechanical loading on diseased distal foot joints of RA patients and propose a patient-specific methodology to design pain-relief insoles. The Mines de St Etienne is leading this project.
10.3.2 Competitivity Clusters
FUI24 SPINE-PDCA
The goal of the SPINE-PDCA project is to develop a unique medical platform that will streamline the medical procedure and achieve all the steps of a minimally invasive surgery intervention with great precision through a complete integration of two complementary systems for pre-operative planning (EOS platform from EOS IMAGING) and imaging/intra-operative navigation (SGV3D system from SURGIVISIO). Innovative low-dose tracking and reconstruction algorithms will be developed by Inria, and collaboration with two hospitals (APHP Trousseau and CHU Grenoble) will ensure clinical feasibility. The medical need is particularly strong in the field of spinal deformity surgery which can, in case of incorrect positioning of the implants, result in serious musculoskeletal injury, a high repeat rate (10 to 40% of implants are poorly positioned in spine surgery) and important care costs. In paediatric surgery (e. g. idiopathic scoliosis), the rate of exposure to X-rays is an additional major consideration in choosing the surgical approach to engage. For these interventions, advanced linkage between planning, navigation and postoperative verification is essential to ensure accurate patient assessment, appropriate surgical procedure and outcome consistent with clinical objectives. The project has effectively started in October 2018 with Di Meng's recruitment as a PhD candidate.
MIAI Chair Data Driven 3D Vision
Edmond Boyer obtained a chair in the new Multidisciplinary Institute in Artificial Intelligence (MIAI) of Grenoble Alpes University. The chair entitled Data Driven 3D Vision is for 4 years and aims at investigating deep learning for 3D artificial vision in order to break some of the limitations in this domain. Applications are especially related to humans and to the ability to capture and analyze their shapes, appearances and motions, for upcoming new media devices, sport and medical applications. A post doc, Joao Regateiro, was recruited in 2020 within the chair.
11 Dissemination
Participants: Sergi Pujades, Stefanie Wuhrer, Jean-Sébastien Franco, Edmond Boyer, Julien Pansiot.
11.1 Promoting scientific activities
- Edmond Boyer and Sergi Pujades co-organized the MICCAI Challenge 3DTeeth, held in Singapour during the MICCAI conference. (3DTeeth) September 18, 2022.
11.1.1 Scientific events: organisation
Member of the organizing committees
- Stefanie Wuhrer co-organized Dagstuhl seminar 3D Morphable Models and Beyond, Dagstuhl, Germany, March 20 – 25, 2022
11.1.2 Scientific events: selection
Member of the conference program committees
- Edmond Boyer was area chair for CVPR, ICCV
- Edmond Boyer is a member of the 3DV steering comitee
Reviewer
- Stefanie Wuhrer reviewed for 3DV, CVPR, ECCV
- Jean-Sébastien Franco reviewed for 3DV, CVPR, ECCV
- Sergi Pujades reviewed for CVPR, MICCAI
11.1.3 Journal
Member of the editorial boards
- Edmond Boyer was associate editor for International Journal on Computer Vision
- Jean-Sébastien Franco was associate editor for International Journal on Computer Vision
Reviewer - reviewing activities
- Stefanie Wuhrer reviewed for Computers & Graphics, Transactions on Multimedia Computing Communications and Applications
11.1.4 Invited talks
- Sergi Pujades gave an invited talk at the Arts et Métiers, Sciences et Technologies Institut de Biomécanique Humaine Georges Charpak about the 3D modeling of the human skeletal structures.
11.1.5 Scientific expertise
- Stefanie Wuhrer was part of the CRCN / ISFP hiring committee.
- Sergi Pujades was required as an expert for the United Stated - Israel Binational Science Foundation.
11.1.6 Research administration
- Starting October 2022, Stefanie Wuhrer is référente scientifique du centre Grenoble Rhône-Alpes pour les données de la recherche.
11.2 Teaching - Supervision - Juries
11.2.1 Teaching
- Master : Sergi Pujades, Numerical Geometry, 15H EqTD, M1, Université Grenoble Alpes, France
- Master: Sergi Pujades, Intelligent Systems, 27.75h EqTD, M1 MoSig, Université Grenoble Alpes.
- Master: Sergi Pujades, Introduction a l'Intelligence Artificielle, 27.75h EqTD, Ensimag 2A.
- Master: Sergi Pujades, Computer Vision, 18h, M2R Mosig GVR, Grenoble INP.
- Master: Sergi Pujades, Introduction to Computer Vision, 12h, Ensimag 3rd year, Grenoble INP.
- Master: Sergi Pujades, Internship supervision, 3h, Ensimag 3rd year, Grenoble INP.
- Master: Jean-Sébastien Franco, Introduction to Computer Graphics, 45h, Ensimag 2nd year, Grenoble INP.
- Master: Jean-Sébastien Franco, Introduction to Computer Vision, 48h, Ensimag 3rd year, Grenoble INP.
- Master: Jean-Sébastien Franco, Introduction to Artifical Intelligence practicals, 10h, Ensimag 2nd year, Grenoble INP.
- Master: Jean-Sébastien Franco, Internship supervision, 15h, Ensimag 2nd and 3rd year, Grenoble INP.
- Master: Jean-Sébastien Franco, Internship jury presidence, 12h, Ensimag 3rd year, Grenoble INP.
- Master: Edmond Boyer, 3D Modeling, 27h, M2R Mosig GVR, Grenoble INP.
- Master: Edmond Boyer, Introduction to Visual Computing, 42h, M1 MoSig, Université Grenoble Alpes.
- Master: Edmond Boyer, Introduction to Computer Vision, 4h30, Ensimag 3rd year, Grenoble INP.
- Master: Stefanie Wuhrer, 3D Graphics, 7.5h, M1 MoSig and MSIAM, Université Grenoble Alpes.
- Master: Julien Pansiot, Introduction to Visual Computing, 15h EqTD, M1 MoSig, Université Grenoble Alpes.
11.2.2 Supervision
- Master: J.S. Franco, Supervision of the 2nd year program (>300 students), 36h, Ensimag 2nd year, Grenoble INP.
- Ph.D. in progress: Mathieu Marsot, Generative modeling of 3D human motion, since 01.11.2019, supervised by Jean-Sébastien Franco, Stefanie Wuhrer
- Ph.D. in progress: Rim Rekik Dit Nkhili, Human motion generation and evaluation, since 01.11.2021, supervised by Anne-Hélène Olivier (Université Rennes), Stefanie Wuhrer
- Ph.D. in progress: Pierre Zins, Learning to infer human motion, since 01.10.2019, supervised by Edmond Boyer, Tony Tung (Facebook), Stefanie Wuhrer
- Ph.D. in progress: Aymen Merrouche, Learning non-rigid surface matching, since 01.10.2021, supervised by Edmond Boyer, Stefanie Wuhrer
- Ph.D. in progress: Anilkumar Swamy, Hand-object interaction acquisition, since 01.07.2021, supervised by Jean-Sébastien Franco, Gregory Rogez (CIFRE Naverlabs Europe)
- Ph.D. in progress: Briac Toussaint, High precision alignment of non-rigid surfaces for 3D performance capture, since 01.10.2021, supervised by Jean-Sébastien Franco, Gregory Rogez (CIFRE Naverlabs Europe)
- Ph.D. in progress: Nicolas Comte, Learning Scoliosis Patterns using Anatomical Models and Motion Capture, since 01.04.2019, supervised by Sergi Pujades, Edmond Boyer et Jean-Sébastien Franco.
- Ph.D. in progress: Marilyn Keller, 3D modeling of the human anatomy, since 01.04.2020, supervised by Sergi Pujades in collaboration with Max Planck Institute for Intelligent Systems.
- Ph.D. defended: Jean Basset, Pose transfer for 3D body models, since 01.09.2018, defended 2.6.2022, supervised by Franck Multon (Université Rennes), Edmond Boyer, Stefanie Wuhrer
- Ph.D. defended: Boyao Zhou. Inferring Dense Human Representation from Sparse or Incomplete Point Clouds, defended 22.11.2022. Supervised by J.S. Franco, E. Boyer.
- Ph.D. defended: Di Meng. Vertebrae segmentation and labelling from CT images, defended 15.11.2022. Supervised by S. Pujades, E. Boyer.
11.2.3 Juries
- S. Wuhrer was reviewer for the Ph.D. of Arianna Rampini, Sapienza University Rome
- S. Wuhrer was Ph.D. committee member of Xu Yao, Telecom Paris
- Jean-Sébastien Franco was a Ph.D. comittee member for Nolan Mestres, Université Grenoble Alpes
- Jean-Sébastien Franco was a Ph.D. comittee member for Di Meng, Université Grenoble Alpes
- Sergi Pujades was a Ph.D. comittee member for Boyao Zhuo, Université Grenoble Alpes
12 Scientific production
12.1 Major publications
- 1 inproceedingsOSSO: Obtaining Skeletal Shape from Outside.2022 IEEE/CVF Conference on Computer Vision and Pattern Recognition (CVPR)New Orleans, United StatesIEEEJune 2022, 20460-20469
- 2 inproceedingsA Structured Latent Space for Human Body Motion Generation.International Conference on 3D Vision (3DV)Prague, Czech RepublicSeptember 2022
- 3 inproceedingsFast Gradient Descent for Surface Capture Via Differentiable Rendering.3DV 2022 - International Conference on 3D VisionPrague, Czech RepublicSeptember 2022, 1-10
12.2 Publications of the year
International journals
International peer-reviewed conferences
National peer-reviewed Conferences
Conferences without proceedings
Scientific book chapters
Doctoral dissertations and habilitation theses
Reports & preprints