Keywords
Computer Science and Digital Science
- A5. Interaction, multimedia and robotics
- A5.3. Image processing and analysis
- A5.3.3. Pattern recognition
- A5.4. Computer vision
- A5.4.2. Activity recognition
- A5.4.4. 3D and spatio-temporal reconstruction
- A5.4.5. Object tracking and motion analysis
- A9. Artificial intelligence
- A9.1. Knowledge
- A9.2. Machine learning
- A9.3. Signal analysis
- A9.8. Reasoning
Other Research Topics and Application Domains
- B1. Life sciences
- B1.2. Neuroscience and cognitive science
- B1.2.2. Cognitive science
- B2. Health
- B2.1. Well being
- B7. Transport and logistics
- B7.1.1. Pedestrian traffic and crowds
- B8. Smart Cities and Territories
- B8.4. Security and personal assistance
1 Team members, visitors, external collaborators
Research Scientists
- François Brémond [Team leader, INRIA, Senior Researcher, HDR]
- Antitza Dantcheva [INRIA, Researcher, HDR]
- Laura Ferrari [UNIV COTE D'AZUR, Starting Research Position, from Mar 2022]
- Alexandra Konig [INRIA, Starting Research Position]
- Sabine Moisan [INRIA, Researcher, HDR]
- Jean-Paul Rigault [Professor Emeritus]
- Monique Thonnat [INRIA, Senior Researcher, HDR]
Post-Doctoral Fellows
- Michal Balazia [UNIV COTE D'AZUR]
- Indu Joshi [INRIA]
- Farhood Negin [INRIA]
- Sayan Rakshit [INRIA, from Jul 2022]
- Mohsen Tabejamaat [INRIA]
- Yaohui Wang [INRIA, until Mar 2022]
PhD Students
- Abid Ali [UNIV COTE D'AZUR]
- David Anghelone [Thales]
- Rui Dai [UNIV COTE D'AZUR]
- Mohammed Guermal [INRIA]
- Thibaud L'Yvonnet [INRIA]
- Valeriya Strizhkova [INRIA]
- Di Yang [INRIA]
Technical Staff
- Tanay Agrawal [INRIA, Engineer]
- Ezem Sura Ekmekci [INRIA, Engineer, from Oct 2022]
- Snehashis Majhi [INRIA, Engineer]
- Abdoul Djalil Ousseini Hamza [INRIA, Engineer, from Jun 2022]
- Jose Francisco Saray Villamizar [INRIA, Engineer]
- Yoann Torrado [INRIA, Engineer, from Sep 2022]
- Duc Minh Tran [INRIA, Engineer]
Interns and Apprentices
- Guillaume Astruc [INRIA, from Jul 2022]
- Agniv Chatterjee [INRIA, from May 2022]
- Ashish Marisetty [INRIA, from May 2022]
- Tomasz Stanczyk [INRIA, until Apr 2022]
- Akos Tanczos [UNIV COTE D'AZUR]
- Po-Han Wu [INRIA, from Aug 2022]
Administrative Assistant
- Sandrine Boute [INRIA]
Visiting Scientist
- Philippe Robert [Inria]
2 Overall objectives
2.1 Presentation
The STARS (Spatio-Temporal Activity Recognition Systems) team focuses on the design of cognitive vision systems for Activity Recognition. More precisely, we are interested in the real-time semantic interpretation of dynamic scenes observed by video cameras and other sensors. We study long-term spatio-temporal activities performed by agents such as human beings, animals or vehicles in the physical world. The major issue in semantic interpretation of dynamic scenes is to bridge the gap between the subjective interpretation of data and the objective measures provided by sensors. To address this problem Stars develops new techniques in the field of computer vision, machine learning and cognitive systems for physical object detection, activity understanding, activity learning, vision system design and evaluation. We focus on two principal application domains: visual surveillance and healthcare monitoring.
2.2 Research Themes
Stars is focused on the design of cognitive systems for Activity Recognition. We aim at endowing cognitive systems with perceptual capabilities to reason about an observed environment, to provide a variety of services to people living in this environment while preserving their privacy. In today's world, a huge amount of new sensors and new hardware devices are currently available, addressing potentially new needs of the modern society. However, the lack of automated processes (with no human interaction) able to extract a meaningful and accurate information (i.e. a correct understanding of the situation) has often generated frustrations among the society and especially among older people. Therefore, Stars objective is to propose novel autonomous systems for the real-time semantic interpretation of dynamic scenes observed by sensors. We study long-term spatio-temporal activities performed by several interacting agents such as human beings, animals and vehicles in the physical world. Such systems also raise fundamental software engineering problems to specify them as well as to adapt them at run time.
We propose new techniques at the frontier between computer vision, knowledge engineering, machine learning and software engineering. The major challenge in semantic interpretation of dynamic scenes is to bridge the gap between the task dependent interpretation of data and the flood of measures provided by sensors. The problems we address range from physical object detection, activity understanding, activity learning to vision system design and evaluation. The two principal classes of human activities we focus on, are assistance to older adults and video analytics.
Typical examples of complex activity are shown in Figure 1 and Figure 2 for a homecare application (See Toyota Smarthome Dataset at ). In this example, the duration of the monitoring of an older person apartment could last several months. The activities involve interactions between the observed person and several pieces of equipment. The application goal is to recognize the everyday activities at home through formal activity models (as shown in Figure 3) and data captured by a network of sensors embedded in the apartment. Here typical services include an objective assessment of the frailty level of the observed person to be able to provide a more personalized care and to monitor the effectiveness of a prescribed therapy. The assessment of the frailty level is performed by an Activity Recognition System which transmits a textual report (containing only meta-data) to the general practitioner who follows the older person. Thanks to the recognized activities, the quality of life of the observed people can thus be improved and their personal information can be preserved.
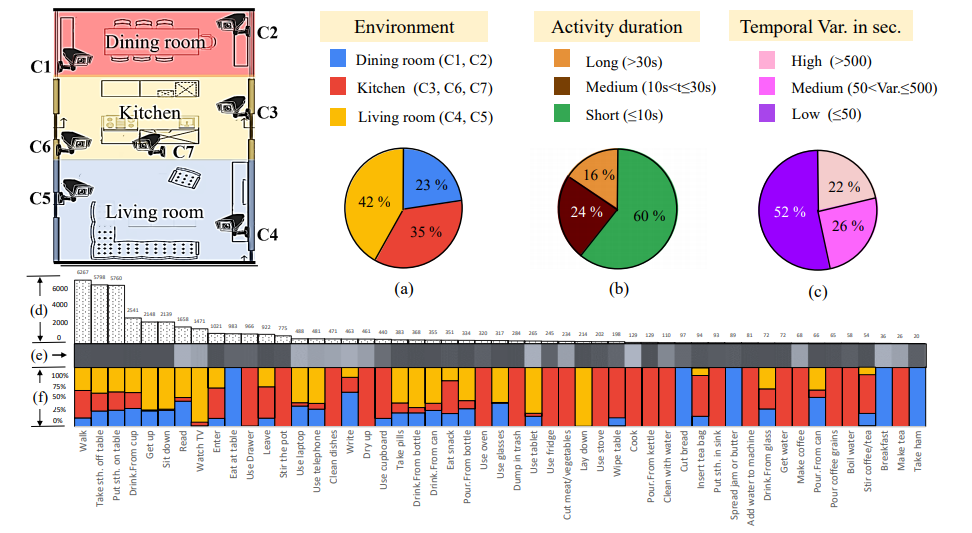
Figure 1
.png)
Figure 2
Activity (PrepareMeal, | |
PhysicalObjects( | (p : Person), (z : Zone), (eq : Equipment)) |
Components( | (s_inside : InsideKitchen(p, z)) |
(s_close : CloseToCountertop(p, eq)) | |
(s_stand : PersonStandingInKitchen(p, z))) | |
Constraints( | (z->Name = Kitchen) |
(eq->Name = Countertop) | |
(s_close->Duration >= 100) | |
(s_stand->Duration >= 100)) | |
Annotation( | AText("prepare meal"))) |
The ultimate goal is for cognitive systems to perceive and understand their environment to be able to provide appropriate services to a potential user. An important step is to propose a computational representation of people activities to adapt these services to them. Up to now, the most effective sensors have been video cameras due to the rich information they can provide on the observed environment. These sensors are currently perceived as intrusive ones. A key issue is to capture the pertinent raw data for adapting the services to the people while preserving their privacy. We plan to study different solutions including of course the local processing of the data without transmission of images and the utilization of new compact sensors developed for interaction (also called RGB-Depth sensors, an example being the Kinect) or networks of small non-visual sensors.
2.3 International and Industrial Cooperation
Our work has been applied in the context of more than 10 European projects such as COFRIEND, ADVISOR, SERKET, CARETAKER, VANAHEIM, SUPPORT, DEM@CARE, VICOMO, EIT Health.
We had or have industrial collaborations in several domains: transportation (CCI Airport Toulouse Blagnac, SNCF, Inrets, Alstom, Ratp, Toyota, GTT (Italy), Turin GTT (Italy)), banking (Crédit Agricole Bank Corporation, Eurotelis and Ciel), security (Thales R&T FR, Thales Security Syst, EADS, Sagem, Bertin, Alcatel, Keeneo), multimedia (Thales Communications), civil engineering (Centre Scientifique et Technique du Bâtiment (CSTB)), computer industry (BULL), software industry (AKKA), hardware industry (ST-Microelectronics) and health industry (Philips, Link Care Services, Vistek).
We have international cooperations with research centers such as Reading University (UK), ENSI Tunis (Tunisia), Idiap (Switzerland), Multitel (Belgium), National Cheng Kung University, National Taiwan University (Taiwan), MICA (Vietnam), IPAL, I2R (Singapore), University of Southern California, University of South Florida (USA), Michigan State University (USA), Chinese Academy of Sciences (China), IIIT Delhi (India), Hochschule Darmstadt (Germany), Fraunhofer Institute for Computer Graphics Research IGD (Germany).
2.3.1 Industrial Contracts
-
Toyota: (Action Recognition System):
This project runs from the 1st of August 2013 up to 2023. It aims at detecting critical situations in the daily life of older adults living home alone. The system is intended to work with a Partner Robot (to send real-time information to the robot for assisted living) to better interact with older adults. The funding was 106 Keuros for the 1st period and more for the following years.
- Thales: This contract is a CIFRE PhD grant and runs from September 2018 until September 2021 within the French national initiative SafeCity. The main goal is to analyze faces and events in the invisible spectrum (i.e., low energy infrared waves, as well as ultraviolet waves). In this context models will be developed to efficiently extract identity, as well as event - information. This models will be employed in a school environment, with a goal of pseudo-anonymized identification, as well as event-detection. Expected challenges have to do with limited colorimetry and lower contrasts.
- Kontron: This contract is a CIFRE PhD grant and runs from April 2018 until April 2021 to embed CNN based people tracker within a video-camera.
- ESI: This contract is a CIFRE PhD grant and runs from September 2018 until March 2022 to develop a novel Re-Identification algorithm which can be easily set-up with low interaction.
3 Research program
3.1 Introduction
Stars follows three main research directions: perception for activity recognition, action recognition and semantic activity recognition. These three research directions are organized following the workflow of activity recognition systems: First, the perception and the action recognition directions provide new techniques to extract powerful features, whereas the semantic activity recognition research direction provides new paradigms to match these features with concrete video analytics and healthcare applications.
Transversely, we consider a new research axis in machine learning, combining a priori knowledge and learning techniques, to set up the various models of an activity recognition system. A major objective is to automate model building or model enrichment at the perception level and at the understanding level.
3.2 Perception for Activity Recognition
Participants: François Brémond, Antitza Dantcheva, Sabine Moisan, Monique Thonnat.
Keywords: Activity Recognition, Scene Understanding, Machine Learning, Computer Vision, Cognitive Vision Systems, Software Engineering.
3.2.1 Introduction
Our main goal in perception is to develop vision algorithms able to address the large variety of conditions characterizing real world scenes in terms of sensor conditions, hardware requirements, lighting conditions, physical objects, and application objectives. We have also several issues related to perception which combine machine learning and perception techniques: learning people appearance, parameters for system control and shape statistics.
3.2.2 Appearance Models and People Tracking
An important issue is to detect in real-time physical objects from perceptual features and predefined 3D models. It requires finding a good balance between efficient methods and precise spatio-temporal models. Many improvements and analysis need to be performed in order to tackle the large range of people detection scenarios.
Appearance models. In particular, we study the temporal variation of the features characterizing the appearance of a human. This task could be achieved by clustering potential candidates depending on their position and their reliability. This task can provide any people tracking algorithms with reliable features allowing for instance to (1) better track people or their body parts during occlusion, or to (2) model people appearance for re-identification purposes in mono and multi-camera networks, which is still an open issue. The underlying challenge of the person re-identification problem arises from significant differences in illumination, pose and camera parameters. The re-identification approaches have two aspects: (1) establishing correspondences between body parts and (2) generating signatures that are invariant to different color responses. As we have already several descriptors which are color invariant, we now focus more on aligning two people detection and on finding their corresponding body parts. Having detected body parts, the approach can handle pose variations. Further, different body parts might have different influence on finding the correct match among a whole gallery dataset. Thus, the re-identification approaches have to search for matching strategies. As the results of the re-identification are always given as the ranking list, re-identification focuses on learning to rank. "Learning to rank" is a type of machine learning problem, in which the goal is to automatically construct a ranking model from a training data.
Therefore, we work on information fusion to handle perceptual features coming from various sensors (several cameras covering a large-scale area or heterogeneous sensors capturing more or less precise and rich information). New 3D RGB-D sensors are also investigated, to help in getting an accurate segmentation for specific scene conditions.
Long-term tracking. For activity recognition we need robust and coherent object tracking over long periods of time (often several hours in video surveillance and several days in healthcare). To guarantee the long-term coherence of tracked objects, spatio-temporal reasoning is required. Modeling and managing the uncertainty of these processes is also an open issue. In Stars we propose to add a reasoning layer to a classical Bayesian framework modeling the uncertainty of the tracked objects. This reasoning layer can take into account the a priori knowledge of the scene for outlier elimination and long-term coherency checking.
Controlling system parameters. Another research direction is to manage a library of video processing programs. We are building a perception library by selecting robust algorithms for feature extraction, by insuring they work efficiently with real time constraints and by formalizing their conditions of use within a program supervision model. In the case of video cameras, at least two problems are still open: robust image segmentation and meaningful feature extraction. For these issues, we are developing new learning techniques.
3.3 Action Recognition
Participants: François Brémond, Antitza Dantcheva, Monique Thonnat.
Keywords: Machine Learning, Computer Vision, Cognitive Vision Systems.
3.3.1 Introduction
Due to the recent development of high processing units, such as GPU,it is now possible to extract meaningful features directly from videos (e.g. video volume) to recognize reliably short actions. Action Recognition benefits also greatly from the huge progress made recently in Machine Learning (e.g. Deep Learning), especially for the study of human behavior. For instance, Action Recognition enables to measure objectively the behavior of humans by extracting powerful features characterizing their everyday activities, their emotion, eating habits and lifestyle, by learning models from a large number of data from a variety of sensors, to improve and optimize for example, the quality of life of people suffering from behavior disorders. However, Smart Homes and Partner Robots have been well advertised but remain laboratory prototypes, due to the poor capability of automated systems to perceive and reason about their environment. A hard problem is for an automated system to cope 24/7 with the variety and complexity of the real world. Another challenge is to extract people fine gestures and subtle facial expressions to better analyze behavior disorders, such as anxiety or apathy. Taking advantage of what is currently studied for self-driving cars or smart retails, there is a large avenue to design ambitious approaches for the healthcare domain. In particular, the advance made with Deep Learning algorithms has already enabled to recognize complex activities, such as cooking interactions with instruments, and from this analysis to differentiate healthy people from the ones suffering from dementia.
To address these issues, we propose to tackle several challenges:
3.3.2 Action recognition in the wild
The current Deep Learning techniques are mostly developed to work on few clipped videos, which have been recorded with students performing a limited set of predefined actions in front of a camera with high resolution. However, real life scenarios include actions performed in a spontaneous manner by older people (including people interactions with their environment or with other people), from different viewpoints, with varying framerate, partially occluded by furniture at different locations within an apartment depicted through long untrimmed videos. Therefore, a new dedicated dataset should be collected in a real-world setting to become a public benchmark video dataset and to design novel algorithms for ADL activity recognition. A special attention should be taken to anonymize the videos.
3.3.3 Attention mechanisms for action recognition
Activities of Daily Living (ADL) and video-surveillance activities are different from internet activities (e.g. Sports, Movies, YouTube), as they may have very similar context (e.g. same background kitchen) with high intra-variation (different people performing the same action in different manners), but in the same time low inter-variation, similar ways to perform two different actions (e.g. eating and drinking a glass of water). Consequently, fine-grained actions are badly recognized. So, we will design novel attention mechanisms for action recognition, for the algorithm being able to focus on a discriminative part of the person conducting the action. For instance, we will study attention algorithms, which could focus on the most appropriate body parts (e.g. full body, right hand). In particular, we plan to design a soft mechanism, learning the attention weights directly on the feature map of a 3DconvNet, a powerful convolutional network, which takes as input a batch of videos.
3.3.4 Action detection for untrimmed videos
Many approaches have been proposed to solve the problem of action recognition in short clipped 2D videos, which achieved impressive results with hand-crafted and deep features. However, these approaches cannot address real life situations, where cameras provide online and continuous video streams in applications such as robotics, video surveillance, and smart-homes. Here comes the importance of action detection to help recognizing and localizing each action happening in long videos. Action detection can be defined as the ability to localize starting and ending of each human action happening in the video, in addition to recognizing each action label. There have been few action detection algorithms designed for untrimmed videos, which are based on either sliding window, temporal pooling or frame-based labeling. However, their performance is too low to address real-word datasets. A first task consists in benchmarking the already published approaches to study their limitations on novel untrimmed video datasets, recorded following real-world settings. A second task could be to propose a new mechanism to improve either 1) the temporal pooling directly from the 3DconvNet architecture using for instance Temporal Convolution Networks (TCNs) or 2) frame-based labeling with a clustering technique (e.g. using Fisher Vectors) to discover the sub-activities of interest.
3.3.5 View invariant action recognition
The performance of current approaches strongly relies on the used camera angle: enforcing that the camera angle used in testing is the same (or extremely close to) as the camera angle used in training, is necessary for the approach performs well. On the contrary, the performance drops when a different camera view-point is used. Therefore, we aim at improving the performance of action recognition algorithms by relying on 3D human pose information. For the extraction of the 3D pose information, several open-source algorithms can be used, such as openpose or videopose3D (from CMU or Facebook research, . Also, other algorithms extracting 3d meshes can be used. To generate extra views, Generative Adversial Network (GAN) can be used together with the 3D human pose information to complete the training dataset from the missing view.
3.3.6 Uncertainty and action recognition
Another challenge is to combine the short-term actions recognized by powerful Deep Learning techniques with long-term activities defined by constraint-based descriptions and linked to user interest. To realize this objective, we have to compute the uncertainty (i.e. likelihood or confidence), with which the short-term actions are inferred. This research direction is linked to the next one, to Semantic Activity Recognition.
3.4 Semantic Activity Recognition
Participants: François Brémond, Sabine Moisan, Monique Thonnat.
Keywords: Activity Recognition, Scene Understanding, Computer Vision.
3.4.1 Introduction
Semantic activity recognition is a complex process where information is abstracted through four levels: signal (e.g. pixel, sound), perceptual features, physical objects and activities. The signal and the feature levels are characterized by strong noise, ambiguous, corrupted and missing data. The whole process of scene understanding consists in analyzing this information to bring forth pertinent insight of the scene and its dynamics while handling the low-level noise. Moreover, to obtain a semantic abstraction, building activity models is a crucial point. A still open issue consists in determining whether these models should be given a priori or learned. Another challenge consists in organizing this knowledge in order to capitalize experience, share it with others and update it along with experimentation. To face this challenge, tools in knowledge engineering such as machine learning or ontology are needed.
Thus, we work along the following research axes: high level understanding (to recognize the activities of physical objects based on high level activity models), learning (how to learn the models needed for activity recognition) and activity recognition and discrete event systems.
3.4.2 High Level Understanding
A challenging research axis is to recognize subjective activities of physical objects (i.e. human beings, animals, vehicles) based on a priori models and objective perceptual measures (e.g. robust and coherent object tracks).
To reach this goal, we have defined original activity recognition algorithms and activity models. Activity recognition algorithms include the computation of spatio-temporal relationships between physical objects. All the possible relationships may correspond to activities of interest and all have to be explored in an efficient way. The variety of these activities, generally called video events, is huge and depends on their spatial and temporal granularity, on the number of physical objects involved in the events, and on the event complexity (number of components constituting the event).
Concerning the modeling of activities, we are working towards two directions: the uncertainty management for representing probability distributions and knowledge acquisition facilities based on ontological engineering techniques. For the first direction, we are investigating classical statistical techniques and logical approaches. For the second direction, we built a language for video event modeling and a visual concept ontology (including color, texture and spatial concepts) to be extended with temporal concepts (motion, trajectories, events ...) and other perceptual concepts (physiological sensor concepts ...).
3.4.3 Learning for Activity Recognition
Given the difficulty of building an activity recognition system with a priori knowledge for a new application, we study how machine learning techniques can automate building or completing models at the perception level and at the understanding level.
At the understanding level, we are learning primitive event detectors. This can be done for example by learning visual concept detectors using SVMs (Support Vector Machines) with perceptual feature samples. An open question is how far can we go in weakly supervised learning for each type of perceptual concept (i.e. leveraging the human annotation task). A second direction is to learn typical composite event models for frequent activities using trajectory clustering or data mining techniques. We name composite event a particular combination of several primitive events.
3.4.4 Activity Recognition and Discrete Event Systems
The previous research axes are unavoidable to cope with the semantic interpretations. However, they tend to let aside the pure event driven aspects of scenario recognition. These aspects have been studied for a long time at a theoretical level and led to methods and tools that may bring extra value to activity recognition, the most important being the possibility of formal analysis, verification and validation.
We have thus started to specify a formal model to define, analyze, simulate, and prove scenarios. This model deals with both absolute time (to be realistic and efficient in the analysis phase) and logical time (to benefit from well-known mathematical models providing re-usability, easy extension, and verification). Our purpose is to offer a generic tool to express and recognize activities associated with a concrete language to specify activities in the form of a set of scenarios with temporal constraints. The theoretical foundations and the tools being shared with Software Engineering aspects.
The results of the research performed in perception and semantic activity recognition (first and second research directions) produce new techniques for scene understanding and contribute to specify the needs for new software architectures (third research direction).
4 Application domains
4.1 Introduction
While in our research the focus is to develop techniques, models and platforms that are generic and reusable, we also make efforts in the development of real applications. The motivation is twofold. The first is to validate the new ideas and approaches we introduce. The second is to demonstrate how to build working systems for real applications of various domains based on the techniques and tools developed. Indeed, Stars focuses on two main domains: video analytic and healthcare monitoring.
Domain: Video Analytics
Our experience in video analytic (also referred to as visual surveillance) is a strong basis which ensures both a precise view of the research topics to develop and a network of industrial partners ranging from end-users, integrators and software editors to provide data, objectives, evaluation and funding.
For instance, the Keeneo start-up was created in July 2005 for the industrialization and exploitation of Orion and Pulsar results in video analytic (VSIP library, which was a previous version of SUP). Keeneo has been bought by Digital Barriers in August 2011 and is now independent from Inria. However, Stars continues to maintain a close cooperation with Keeneo for impact analysis of SUP and for exploitation of new results.
Moreover, new challenges are arising from the visual surveillance community. For instance, people detection and tracking in a crowded environment are still open issues despite the high competition on these topics. Also detecting abnormal activities may require to discover rare events from very large video data bases often characterized by noise or incomplete data.
Domain: Healthcare Monitoring
Since 2011, we have initiated a strategic partnership (called CobTek) with Nice hospital (CHU Nice, Prof P. Robert) to start ambitious research activities dedicated to healthcare monitoring and to assistive technologies. These new studies address the analysis of more complex spatio-temporal activities (e.g. complex interactions, long term activities).
4.1.1 Research
To achieve this objective, several topics need to be tackled. These topics can be summarized within two points: finer activity description and longitudinal experimentation. Finer activity description is needed for instance, to discriminate the activities (e.g. sitting, walking, eating) of Alzheimer patients from the ones of healthy older people. It is essential to be able to pre-diagnose dementia and to provide a better and more specialized care. Longer analysis is required when people monitoring aims at measuring the evolution of patient behavioral disorders. Setting up such long experimentation with dementia people has never been tried before but is necessary to have real-world validation. This is one of the challenges of the European FP7 project Dem@Care where several patient homes should be monitored over several months.
For this domain, a goal for Stars is to allow people with dementia to continue living in a self-sufficient manner in their own homes or residential centers, away from a hospital, as well as to allow clinicians and caregivers remotely provide effective care and management. For all this to become possible, comprehensive monitoring of the daily life of the person with dementia is deemed necessary, since caregivers and clinicians will need a comprehensive view of the person's daily activities, behavioral patterns, lifestyle, as well as changes in them, indicating the progression of their condition.
4.1.2 Ethical and Acceptability Issues
The development and ultimate use of novel assistive technologies by a vulnerable user group such as individuals with dementia, and the assessment methodologies planned by Stars are not free of ethical, or even legal concerns, even if many studies have shown how these Information and Communication Technologies (ICT) can be useful and well accepted by older people with or without impairments. Thus one goal of Stars team is to design the right technologies that can provide the appropriate information to the medical carers while preserving people privacy. Moreover, Stars will pay particular attention to ethical, acceptability, legal and privacy concerns that may arise, addressing them in a professional way following the corresponding established EU and national laws and regulations, especially when outside France. Now, Stars can benefit from the support of the COERLE (Comité Opérationnel d'Evaluation des Risques Légaux et Ethiques) to help it to respect ethical policies in its applications.
As presented in 2, Stars aims at designing cognitive vision systems with perceptual capabilities to monitor efficiently people activities. As a matter of fact, vision sensors can be seen as intrusive ones, even if no images are acquired or transmitted (only meta-data describing activities need to be collected). Therefore, new communication paradigms and other sensors (e.g. accelerometers, RFID, and new sensors to come in the future) are also envisaged to provide the most appropriate services to the observed people, while preserving their privacy. To better understand ethical issues, Stars members are already involved in several ethical organizations. For instance, F. Brémond has been a member of the ODEGAM - “Commission Ethique et Droit” (a local association in Nice area for ethical issues related to older people) from 2010 to 2011 and a member of the French scientific council for the national seminar on “La maladie d'Alzheimer et les nouvelles technologies - Enjeux éthiques et questions de société” in 2011. This council has in particular proposed a chart and guidelines for conducting researches with dementia patients.
For addressing the acceptability issues, focus groups and HMI (Human Machine Interaction) experts, are consulted on the most adequate range of mechanisms to interact and display information to older people.
5 Social and environmental responsibility
5.1 Footprint of research activities
We have limited our travels by reducing our physical participation to conferences and to international collaborations.
5.2 Impact of research results
We have been involved for many years in promoting public transportation by improving safety onboard and in station. Moreover, we have been working on pedestrian detection for self-driving cars, which will help also reducing the number of individual cars.
6 Highlights of the year
This year, we have proposed several approaches for the action recognition and action detection tasks able to outperform the State-of-the-art algorithms 37, 39. To get these nice performances, we have developed several techniques for self-supervised pre-training on either large pre-training datasets or smaller target datasets. These self-supervised pre-training techniques 61 are able to get a generic video representation which can be effectively transfer to the downstream task on the target dataset while avoiding overfitting.
6.1 Awards
- Antitza Dantcheva won the “New Technologies Show” in conjunction with the European Conference on Computer Vision (ECCV) 2022.
7 New software and platforms
The STARS has adopted the common practice from the Computer Vision community, where software related to publications appear on Github. Example repositories include the following.
8 New results
8.1 Introduction
This year Stars has proposed new results related to its three main research axes: (i) perception for activity recognition, (ii) action recognition and (iii) semantic activity recognition.
Perception for Activity Recognition
Participants: François Brémond, Antitza Dantcheva, Juan Diego Gonzales Zuniga, Farhood Negin, Vishal Pani, Indu Joshi, David Anghelone, Laura M. Ferrari, Hao Chen, Yaohui Wang, Valeriya Strizhkova, Mohsen Tabejamaat.
The new results for perception for activity recognition are:
- Transforming Temporal Embeddings to Keypoint Heatmaps for the Detection of Tiny Vehicles in Wide Area Motion Imagery (WAMI) Sequences (see 8.2)
- DeTracker: A Joint Detection and Tracking Framework (see 8.3)
- Towards unsupervised person re-identification (see 8.4)
- Learning Invariance from Generated Variance for Unsupervised Person Re-identification (see 8.5)
- Merging Tracking Identities through Clustering (see 8.6)
- Thermal Face and Landmark Detection for Unconstrained Cross-spectral Face Recognition (see 8.7)
- Attention-Guided Generative Adversarial Network for Explainable Thermal-to-Visible Face Recognition (see 8.8)
- Pose Transfer using a Constrained Spatial Transformation (see 8.9)
- Recognition of the jersey number of the soccer players (see 8.10)
- Cross-Domain Consistent Fingerprint Denoising (see 8.11)
- Context-Aware Restoration of Noisy Fingerprints (see 8.12)
- On Restoration of Degraded Fingerprints (see 8.13)
- Latent Image Animator: Learning to Animate Images via Latent Space Navigation (see 8.14)
- 3D CNN Architectures and Attention Mechanisms for Deepfake Detection (see 8.15)
Action Recognition
Participants: François Brémond, Antitza Dantcheva, Monique Thonnat, Mohammed Guermal, Tanay Agrawal, Abid Ali, Po-Han Wu, Di Yang, Rui Dai, Snehashis Majhi, Tomasz Stanczyk.
The new results for action recognition are:
- View-invariant skeleton action representation learning via motion retargeting (see 8.17)
- Self-supervised video representation learning via latent time navigation (see 8.18)
- Fall detection in untrimmed videos (see 8.19)
- Multimodal Vision Transformers with Forced Attention for Behavior Analysis (see 8.20)
- Online Action Detection (see 8.21)
- Vision-based Seizure Classification (see 8.22)
- Action detection for untrimmed videos based on deep neural networks (see 8.23)
- Human-Scene Network: A Novel Baseline with Self-rectifying Loss for Weakly supervised Video Anomaly Detection (see 8.24)
- MVVM: Multi-View Video Masked Autoencoder for Emotion Recognition (see 8.25)
- Multimodal Personality Recognition using Cross-Attention Transformer and Behaviour Encoding (see 8.26)
Semantic Activity Recognition
Participants: Sabine Moisan, François Brémond, Monique Thonnat, Jean-Paul Rigault, Alexandra Konig, Rachid Guerchouche, Thibaud L'Yvonnet, Michal Balazia.
For this research axis, the contributions are:
- Analysis of autism spectrum disorders (see 8.27)
- Video-based Behavior Understanding of Children for Objective Diagnosis of Autism (see 8.28)
- Bodily Behaviors in Social Interaction (see 8.29)
- Phenotyping of Psychiatric Disorders from Social Interaction (see 8.30)
- Formal Probabilistic Model of the Inhibitory Control Circuit in the Brain (see 8.31)
- Datasets for multimodal emotion recognition (see 8.32)
- MePheSTO – Digital Phenotyping for Psychiatric Disorders from Social Interaction (see 8.34)
- Digital phenotyping for differential diagnosis of Major Depressive Episode: A narrative review (see 8.35)
- Detecting subtle signs of depression with automated speech analysis in a non-clinical sample (see 8.36)
- Dementia analysis (see 8.37)
8.2 Transforming Temporal Embeddings to Keypoint Heatmaps for the Detection of Tiny Vehicles in Wide Area Motion Imagery (WAMI) Sequences
Participants: Farhood Negin, Mohsen Tabejamaat, François Brémond.
Nowadays, due to its many applications, objects detection in wide area motion imagery (WAMI) sequences has received a lot of attention. Unlike natural images, object detection in WAMI faces unique challenges. Lack of appearance information due to the small size of objects makes object detection difficult for conventional methods. In addition, pixel noise, registration errors, sparse or densely populated objects, brings on pronounced artifacts which amplifies the difficulty of detection. This work aims to address object detection problem in the presence of these issues by considering objects as keypoints in the relevant background and proposes a spatio-temporal anchor-free detector for tiny vehicles in WAMI images. Instead of background subtraction, a region of interest network refines large search space of sequences to indicates object clusters. For further investigation, clusters are encoded by a codebook which is learned through an unsupervised encoder-decoder network. To accurately generate the detections, a Transformer network is trained on cluster embeddings using ground-truth heatmaps that are described by Gaussian distribution rather than hard label annotation. The network is trained with a redesigned version of Focal loss comprising a shape prior regularizer which help the generated heatmaps to conform to the shape of the keypoints. Extensive experiments on WPAFB dataset demonstrate the high capability of our method for the detection of small vehicles where it achieves competitive performance when compared to the state-of-the-art. This work has been published at the CVPR Workshop, EarthVision in June 2022 41.
8.3 DeTracker: A Joint Detection and Tracking Framework
Participants: Juan Diego Gonzales Zuniga, François Brémond.
We propose a unified network for simultaneous detection and tracking 38. Instead of basing the tracking frame- work on object detections, we focus our work directly on tracklet detection whilst obtaining object detection. We take advantage of the spatio-temporal information and features from 3D CNN networks and output a series of bounding boxes and their corresponding identifiers with the use of Graph Convolution Neural Networks. We put forward our approach in contrast to traditional tracking-by-detection methods, the major advantages of our formulation are the creation of more reliable tracklets, the enforcement of the temporal consistency, and the absence of data association mechanism for a given set of frames. We introduce DeTracker, a truly joint detection and tracking network. We enforce an intra-batch temporal consistency of features by enforcing a triplet loss over our tracklets, guiding the features of tracklets with different identities separately clustered in the feature space. Our approach is demonstrated on two different datasets, including natural images and synthetic images, and we obtain 58.7% on MOT and 56.79% on a subset of the JTA-dataset.
8.4 PhD thesis: Towards unsupervised person re-identification
Participants: Hao Chen, François Brémond.
As a core component of intelligent video surveillance systems, person reidentification (ReID) targets at retrieving a person of interest across nonoverlapping cameras. Despite significant improvements in supervised ReID, cumbersome annotation process makes it less scalable in real-world deployments. Moreover, as appearance representations can be affected by noisy factors, such as illumination level and camera properties, between different domains, person ReID models suffer a large performance drop in the presence of domain gaps. We are particularly interested in designing algorithms that can adapt a person ReID model to a target domain without human supervision. In such context, we mainly focus on designing unsupervised domain adaptation and unsupervised representation learning methods for person ReID. In this thesis 45, we first explore how to build robust representations by combining both global and local features under the supervised condition. Then, towards an unsupervised domain adaptive ReID system, we propose three unsupervised methods for person ReID, including 1) teacher-student knowledge distillation with asymmetric network structures for feature diversity encouragement, 2) joint generative and contrastive learning framework that generates augmented views with a generative adversarial network for contrastive learning, and 3) exploring inter-instance relations and designing relation-aware loss functions for better contrastive learning based person ReID. Our methods have been extensively evaluated on main-stream ReID datasets, such as Market-1501, DukeMTMC-reID and MSMT17. The proposed methods significantly outperform previous methods on the ReID datasets, significantly pushing person ReID to real-world deployments.
8.5 Learning Invariance from Generated Variance for Unsupervised Person Re-identification
Participants: Hao Chen, Yaohui Wang, Benoit Lagadec, Antitza Dantcheva, François Brémond.
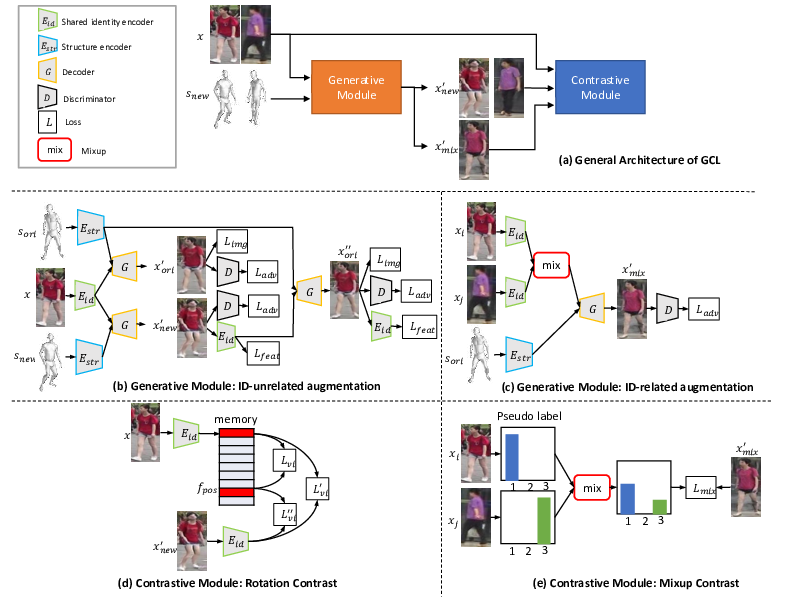
Figure 4
This work focuses on unsupervised representation learning in person re-identification (ReID). Recent self-supervised contrastive learning methods learn invariance by maximizing the representation similarity between two augmented views of a same image. However, traditional data augmentation may bring to the fore undesirable distortions on identity features, which is not always favorable in id-sensitive ReID tasks. In this work, we propose to replace traditional data augmentation with a generative adversarial network (GAN) that is targeted to generate augmented views for contrastive learning. The general architecture of our method is shown in Figure 4. A 3D mesh guided person image generator is proposed to disentangle a person image into id-related and id-unrelated features. Deviating from previous GAN-based ReID methods that only work in id-unrelated space (pose and camera style), we conduct GAN-based augmentation on both id-unrelated and id-related features. We further propose specific contrastive losses to help our network learn invariance from id-unrelated and id-related augmentations. By jointly training the generative and the contrastive modules, our method achieves new state-of-the-art unsupervised person ReID performance on mainstream large-scale benchmarks. This work 17 has been accepted to IEEE Transactions on Pattern Analysis and Machine Intelligence.
8.6 Merging Tracking Identities through Clustering
Participants: Guillaume Astruc, Tomasz Stanczyk, François Brémond.
To perform action-recognition on long videos of doctor-patient interaction, where we want to study child behavior, we need to know where the child is located. We first locate and track all persons in the video with a Yolov5 human detector and DeepSORT tracker. These two algorithms give us tracklets each containing a unique person. However, this tracking system creates far more identities than the actual people present in the video with several tracking identities representing a single person. Therefore, we must merge these tracking identities in order to recreate ground truth identities so as to improve our child detector. This work mainly focuses on the latter part.
For this, one needs to merge identities that seem to contain the same person. No additional information is provided such as an image of each person present or the number of persons in the video. The detail of the method is summarized in the following figure:
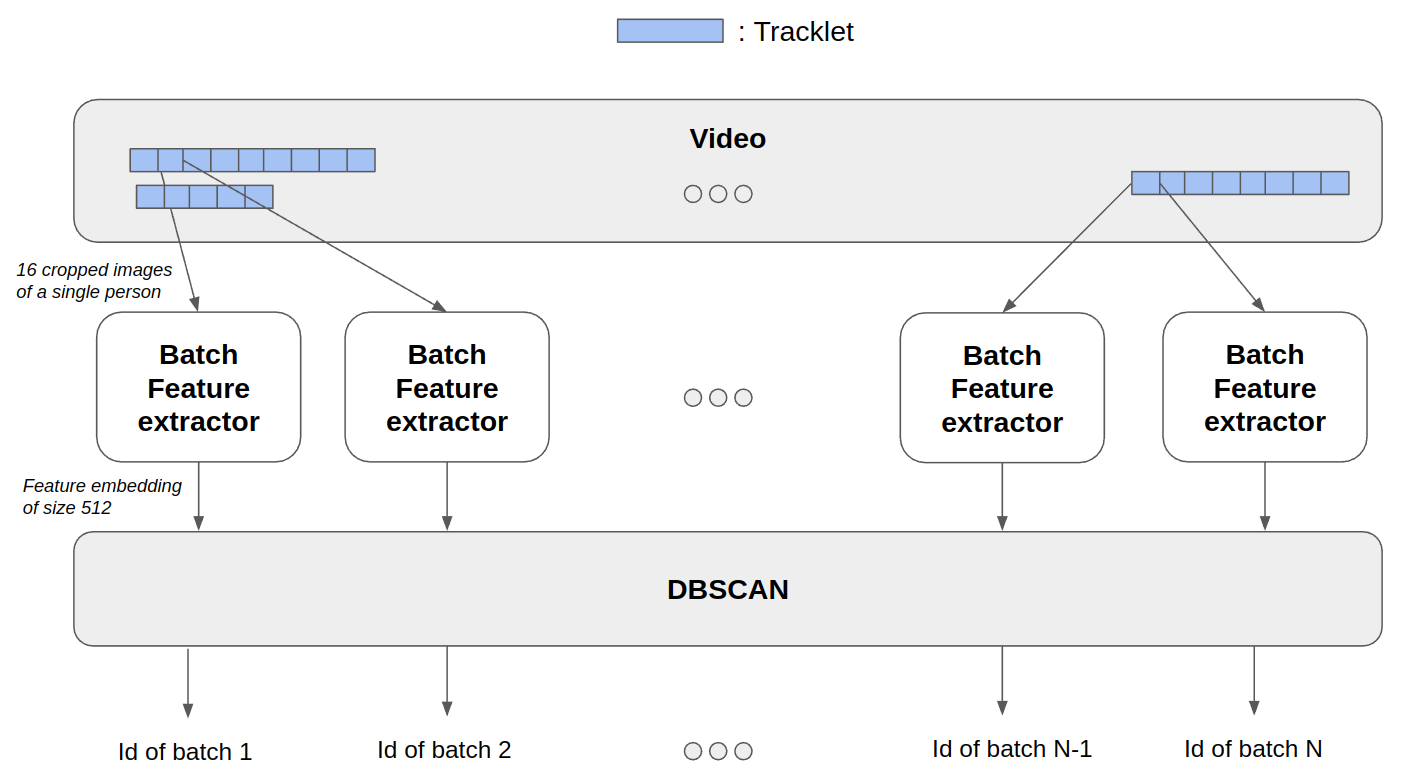
Figure 5
The tracklets are split into batches of 16 cropped images. After removing noisy frames with skeleton extraction using the HRNet-w48 model, features from all frames are extracted with a fine-tuned OSNet model for people Re-Identification. After a median pooling operation, a feature vector for the batch is obtained, which will be given together with the other feature vectors from batches of the same long video into a DBSCAN clustering method. The clustering will indicate batch ids for further merging.
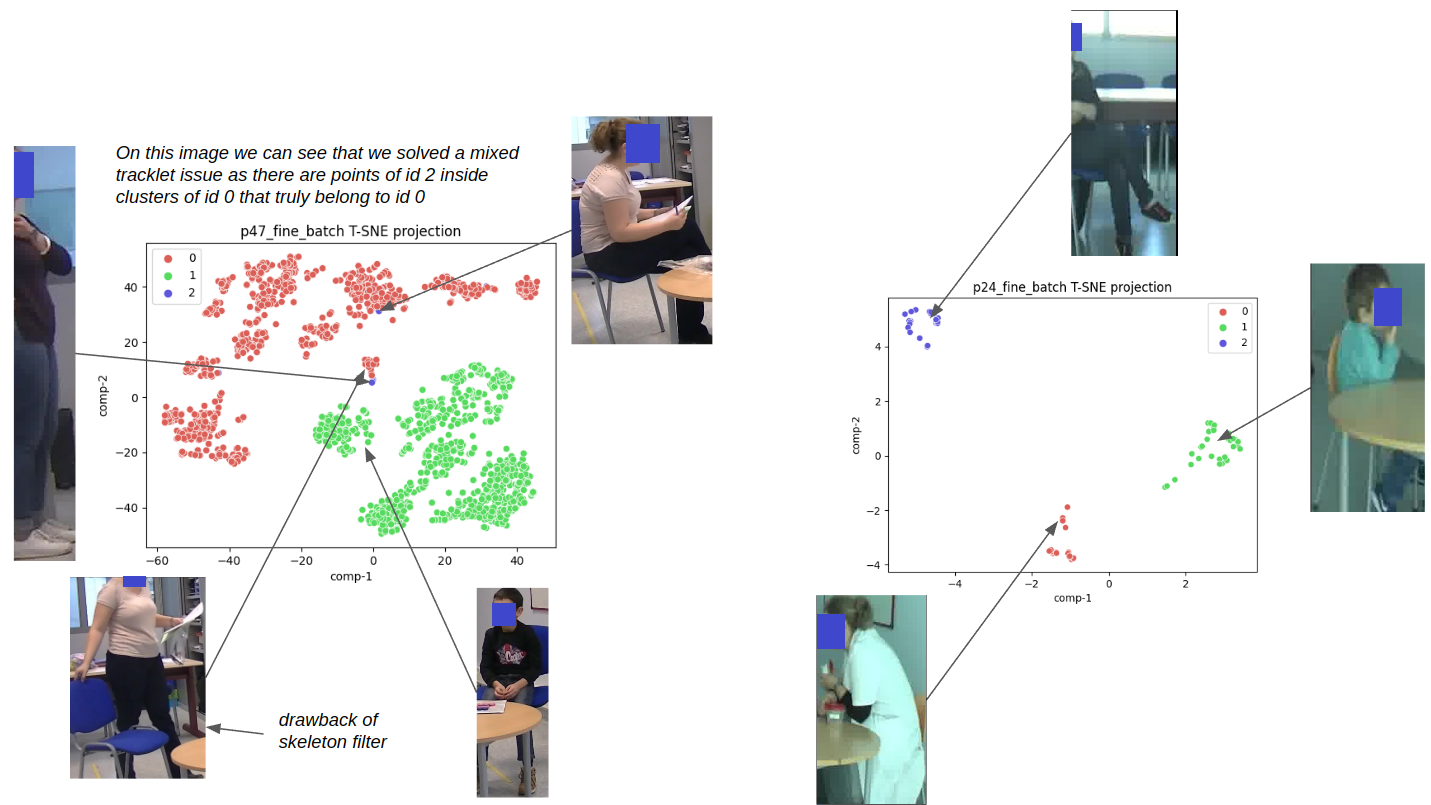
Figure 6
Satisfying results are obtained, which validate the approach described. The number of clusters is reduced almost 8 times compared to the initial quantity. On the average, approximately 1.1 cluster per identity is obtained with only 2% error.
8.7 TFLD: Thermal Face and Landmark Detection for Unconstrained Cross-spectral Face Recognition
Participants: David Anghelone, Antitza Dantcheva.
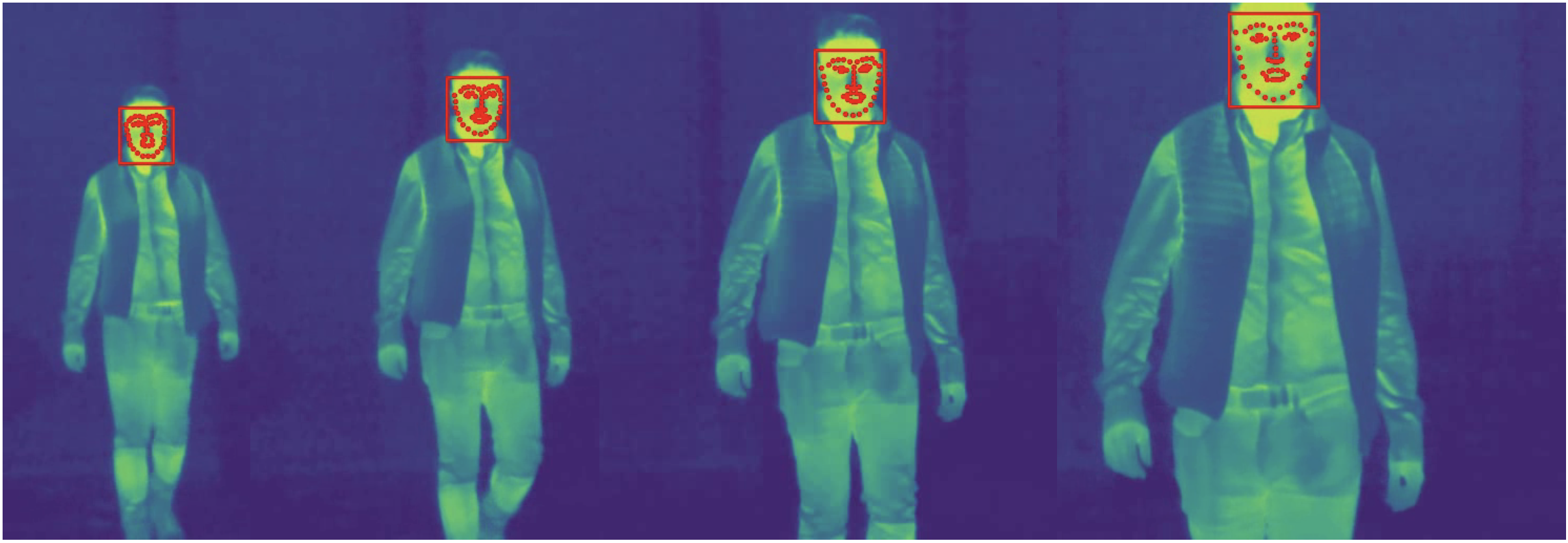
Figure 7
The field of face recognition (FR) encompasses a range of techniques and methods, among which the detection and alignment of facial landmarks could constitute the initial processing steps. While the detection of landmarks in the visible spectrum has been met with a reasonable degree of reliability, the same cannot be said of thermal images, owing to the inherent low-contrast and low-resolution of such images, as well as the poor texture information they often possess. The cross-spectral modality gap, coupled with the scarcity of annotated thermal datasets, has resulted in a dearth of research on the detection of thermal facial landmarks.
In light of these challenges, we present a novel thermal face and landmark detector (TFLD) 34 designed to be robust to a host of adversarial conditions, including variations in pose, expression, occlusion, and image quality, as well as long-range distance. Specifically, TFLD, in conjunction with a proposed data augmentation strategy, is able to (i) detect faces and landmarks in the thermal spectrum under challenging, unconstrained conditions, (ii) establish a benchmark for face and landmark detection in the thermal spectrum, and (iii) enhance the annotation of existing thermal face datasets by detecting a larger number of facial landmarks. Additionally, TFLD is instrumental in (iv) cross-spectral face recognition (CFR) and (v) thermal monitoring systems, see Figure 7.
To achieve this, we adopt YOLOv5, a state-of-the-art object detection algorithm, and design a model that incorporates a thermal face restoration (TFR) pre-processing filter followed by two YOLOv5 models, denoted as M1 and M2. TFR serves to enhance the visual details and contours of the face, improving contrast and sharpness, and ultimately leading to better detection accuracy. While M1 detects the full face in the thermal spectrum, M2 subsequently detects a set of facial landmarks in the localized face. Our method is evaluated through the assessment of landmark accuracy, as well as by determining the impact of the proposed face alignment on CFR. To the best of our knowledge, this is the first work based on YOLOv5 for large-scale thermal-based facial landmark detection, and we believe that our proposed TFLD offers a multitude of benefits when compared to prior work, particularly in terms of its ability to detect a large number of thermal facial landmarks in unconstrained environments, and its capacity to serve as an accurate automatic annotation tool for cross-spectral face recognition systems.
8.8 Attention-Guided Generative Adversarial Network for Explainable Thermal-to-Visible Face Recognition
Participants: David Anghelone, Antitza Dantcheva.
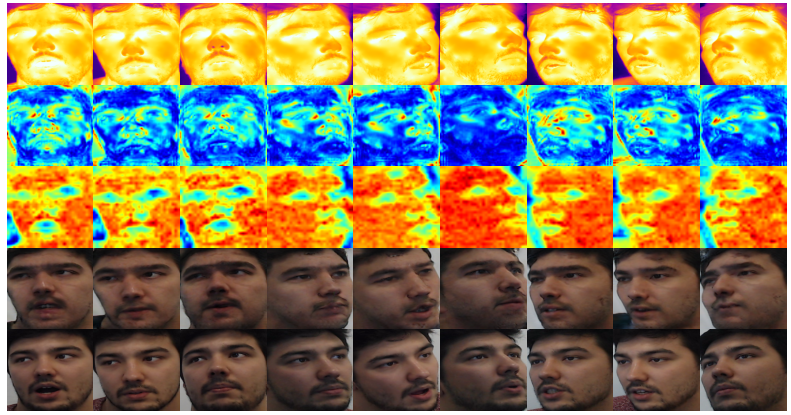
Figure 8
In recent years, the field of image-to-image translation has garnered considerable attention, owing to the rapid advancements in the realm of generative adversarial networks (GANs). In this work, we focus on the task of thermal-to-visible image synthesis via conditional adversarial networks, which entails the generation of photo-realistic visible face images conditioned on certain input thermal data. This task has a myriad of applications, including cross-spectral face recognition and face landmark detection, which are of paramount importance in defense, surveillance, and public safety.
Recent state-of-the-art models for thermal-to-visible image translation have achieved a remarkable degree of visual quality and fidelity. Rakhil et al. proposed a Transformers-based GAN that augmented the network with axial-attention layers to perform simultaneous face hallucination and translation. First, self-attention generative adversarial networks have been used to enhance attention-guided feature synthesis for synthesizing visible images from polarimetric thermal inputs. However, these works did not offer insightful explanations or visualizations of the type of axial-attention or self-attention features that were learned during the thermal-to-visible generation process. Anghelone et al. 34 utilized two separate identity and style encoders to disentangle the latent space into identity and style code representations. The associated visualization of the identity code demonstrated that the identity-related structural information was well preserved during the translation. However, their work did not incorporate attention to augment the network.
Our proposed AG-GAN model 36 is designed to overcome the limitations of previous works by encoding an input thermal image into attention feature maps, see Figure 8. The encoder is based on a ResNet style architecture, which consists of downsampling blocks that gradually reduce the spatial size and enlarge the feature channel numbers. The decoder, on the other hand, employs residual blocks with adaptive layer instance normalization (AdaLIN) to modulate the shape and texture change during the translation process. The AdaLIN parameters are computed by applying a fully connected layer to the attention feature maps. The AG-GAN is specifically engineered to learn attention modules, thereby guiding the feature synthesis to focus on regions that are of significance to the interests of the generator and the discriminator. In this regard, we consider two types of attention feature map learning: supervised and unsupervised. The supervised attention map learns to generate the attention weights based on an auxiliary classifier, whereas the unsupervised attention learning generates the attention weights via the squeeze-excitation (SE) operation. Both approaches share the commonality of learning channel-based attention weights to capture global interactions between facial contexts.
In conclusion, our proposed AG-GAN model represents a significant step forward in the field of thermal-to-visible image synthesis. Its ability to encode input thermal images into attention feature maps, and its utilization of both supervised and unsupervised attention feature map learning, make it a robust
8.9 Pose Transfer using a Constrained Spatial Transformation
Participants: Mohsen Tabejamaat, François Brémond.
In this work, we address the problem of pose transfer. It aims to generate a source image in a new target pose. The pose is already provided by a set of spatial landmarks. The transfer function is directly estimated from the difference between the landmarks given in the new target pose and the landmarks of the source image. Existing methods perform this task using two specialized networks, one to move the visible patches of the source sample to their new location and the other one to generate the new patches that are not visible in the source image but are newly introduced in the target pose. In contrast to these strategies, we develop an end-to-end trainable neural network that learns to estimate both these visible and invisible parts using a simple warping module. In other words, we propose a flow estimation method that not only displaces the patches to their new locations but also generates new pixels that are not visible in the source image, all in an unsupervised manner without the need for a ground-truth flow map. In this way, the moving of patches and the introduction of new parts are unified into a single network, ensuring that an overall minimum is achieved for these two mutual tasks. Additionally, it avoids the need for a human observer to determine a trade-off between the performance of the two separated networks, thus avoiding a cartoonish addition of new parts to the visible parts in the source sample. Extensive experiments demonstrate the superiority of our method over the state-of-the-art algorithms on the Deepfashion and Market datasets.
8.10 Recognition of the jersey number of the soccer players
Participants: Ezem Ekmekci, François Brémond.
In this project, we were collaborating with the start-up company Fair Vision, which focuses on monitoring amateur soccer matches. The topic involved mastering of player and ball tracking in soccer videos. We were given input videos from the company, which included stadium recordings of soccer matches, as well as corresponding annotation files with detections of the players and the ball per each frame. We approached ball tracking as a single object tracking (SOT) problem. For this, we tested visual trackers and prepared an adapted version of the CSRT tracker (Discriminative Correlation Filter Tracker with Channel and Spatial Reliability) to enhance tracking of the ball, especially when ball detections were missing. Player tracking was approached as multi object tracking (MOT) problem. We tried several state-of-the-art MOT algorithms, e.g., FairMOT, TransTrack, ByteTrack, through applying them on the given input videos. After studying and analyzing the algorithm limitations, we started developing new version of the ByteTrack algorithm,aiming for the reduction of identity switches and enhancement of long term tracking. This work is continued in 2022.Each soccer player has his own jersey number, th jersey number is one of the most distinguishable characteristics of the players. Detecting and recognizing these numbers may help to identify each player so automatically understanding soccer match videos becomes easier. However, there are many challenges in jersey number recognition due to motion blur, light illumination and soccer video resolution. We put into practice the jersey number recognition method of Gen Li, Shikun Xu et al. 56. We adapt their method to the Fair Vision use case by gathering data/ images from several match videos from their platform, creating an image dataset with jersey number bounding boxes and training/fine-tuning the proposed model with this new dataset. Our studies on this subject will be continued in the future.
8.11 Cross-Domain Consistent Fingerprint Denoising
Participants: Tashvik Dhamija, Antitza Dantcheva.
Performance of state-of-the-art fingerprint denoising models on poor quality fingerprints degrades due to crossdomain shift observed between training and testing domains. To address this limitation, we present a cross-domain consistent fingerprint denoising model 24, which ensures that the output of two fingerprint images with the same ridge structure, however varying contrast and ridge-valley clarity should be similar. Results indicate that the proposed CDCGAN outperforms state-of-the-art fingerprint denoising algorithms on challenging publicly available poor quality fingerprint databases.
8.12 Context-Aware Restoration of Noisy Fingerprints
Participants: Antitza Dantcheva.
Literature on fingerprint restoration algorithms firmly advocates exploiting contextual information such as ridge orientation field, ridge spacing, and ridge frequency to recover ridge details in fingerprint regions with poor quality ridge structure. However, most state-of-the-art convolutional neural network based fingerprint restoration models exploit spatial context only through convolution operations. Motivated by this observation, this work 25 introduces a novel context-aware fingerprint restoration model: context-aware GAN (CA-GAN). CA-GAN is explicitly regularized to learn spatial context by ensuring that the model not only performs fingerprint restoration but also accurately predicts the correct spatial arrangement of randomly arranged fingerprint patches. Experimental results establish better fingerprint restoration ability of CA-GAN compared to the state-of-the-art.
8.13 On Restoration of Degraded Fingerprints
Participants: Antitza Dantcheva.
The state-of-the-art fingerprint matching systems achieve high accuracy on good quality fingerprints. However, degraded fingerprints obtained due to poor skin conditions of subjects or fingerprints obtained around a crime scene often have noisy background and poor ridge structure. Such degraded fingerprints pose problem for the existing fingerprint recognition systems. This contribution 26 presents a fingerprint restoration model for a poor quality fingerprint that reconstructs a binarized fingerprint image with an improved ridge structure. In particular, we demonstrate the effectiveness of channel refinement in fingerprint restoration. The state-of-the-art channel refinement mechanisms, such as Squeeze and Excitation (SE) block, in general, create SEblock introduce redundancy among channel weights and degrade the performance of fingerprint enhancement models. We present a lightweight attention mechanism that performs channel refinement by reducing redundancy among channel weights of the convolutional kernels. Restored fingerprints generated after introducing proposed channel refinement unit obtain improved quality scores on standard fingerprint quality assessment tool. Furthermore, restored fingerprints achieve improved fingerprint matching performance. We also illustrate that the idea of introducing a channel refinement unit is generalizable to different deep architectures. Additionally, to quantify the ridge preservation ability of the model, standard metrics: Dice score, Jaccard Similarity, SSIM and PSNR are computed with the ground truth and the output of the model (CR-GAN). An ablation study is conducted to individually quantify the improvement of generator and discriminator sub-networks of CR-GAN through channel refinement. Experiments on the publicly available IIITD- MOLF, Rural Indian Fingerprint Database and a private rural fingerprint database demonstrate the efficacy of the proposed attention mechanism.
8.14 Latent Image Animator: Learning to Animate Images via Latent Space Navigation
Participants: Yaohui Wang, Di Yang, François Brémond, Antitza Dantcheva.
Due to the remarkable progress of deep generative models, animating images has become increasingly efficient, whereas associated results have become increasingly realistic. Current animation-approaches commonly exploit structure representation extracted from driving videos. Such structure representation is instrumental in transferring motion from driving videos to still images. However, such approaches fail in case the source image and driving video encompass large appearance variation. Moreover, the extraction of structure information requires additional modules that endow the animation-model with increased complexity. Deviating from such models, we here introduce the Latent Image Animator (LIA) 42, a self-supervised autoencoder that evades need for structure representation. LIA is streamlined to animate images by linear navigation in the latent space. Specifically, motion in generated video is constructed by linear displacement of codes in the latent space. Towards this, we learn a set of orthogonal motion directions simultaneously, and use their linear combination, in order to represent any displacement in the latent space. Extensive quantitative and qualitative analysis suggests that our model systematically and significantly outperforms state-of-art methods on VoxCeleb, Taichi and TED-talk datasets w.r.t. generated quality.
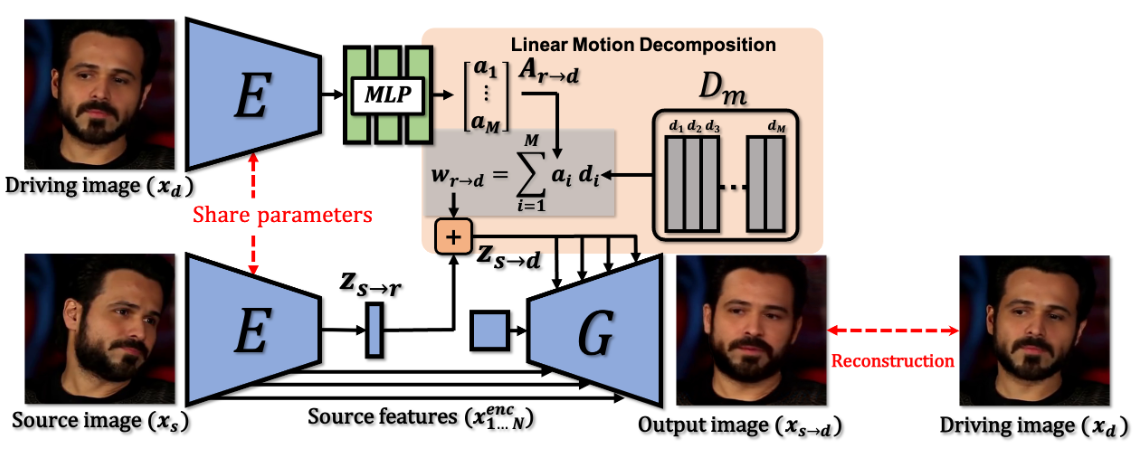
Figure 9
8.15 3D CNN Architectures and Attention Mechanisms for Deepfake Detection
Participants: Antitza Dantcheva.
Manipulated images and videos have become increasingly realistic due to the tremendous progress of deep convolutional neural networks (CNNs). While technically intriguing, such progress raises a number of social concerns related to the advent and spread of fake information and fake news. Such concerns necessitate the introduction of robust and reliable methods for fake image and video detection. Towards this in this work 44, we study the ability of state of the art video CNNs including 3D ResNet, 3D ResNeXt, and I3D in detecting manipulated videos. In addition, and towards a more robust detection, we investigate the effectiveness of attention mechanisms in this context. Such mechanisms are introduced in CNN architectures in order to ensure that robust features are being learnt. We test two attention mechanisms, namely SE-block and Non-local networks. We present related experimental results on videos tampered by four manipulation techniques, as included in the FaceForensics++ dataset. We investigate three scenarios, where the networks are trained to detect (a) all manipulated videos, (b) each manipulation technique individually, as well as (c) the veracity of videos pertaining to manipulation-techniques not included in the train set.
8.16 Detection of Tiny Vehicles from Satellite Video
Participants: Di Yang, Antitza Dantcheva, François Brémond.
In 2022, we have proposed two novel approaches for human daily living action recognition. The first work 49 (see 8.17) focuses on view-invariant action classification using the skeleton data and the second work (see 8.18) improves RGB-based action classification by proposing a time-aware self-supervised pre-training method. Our contributions are summarized in following sections.
8.17 View-invariant skeleton action representation learning via motion retargeting
Participants: Di Yang, Antitza Dantcheva, François Brémond.
Current self-supervised approaches for skeleton action representation learning often focus on constrained scenarios, where videos and skeleton data are recorded in laboratory settings. When dealing with estimated skeleton data in real-world videos, such methods perform poorly due to the large variations across subjects and camera viewpoints. To address this issue, we introduce ViA, a novel View-Invariant Autoencoder for self-supervised skeleton action representation learning. ViA leverages motion retargeting between different human performers as a pretext task, in order to disentangle the latent action-specific `Motion' features on top of the visual representation of a 2D or 3D skeleton sequence. Such `Motion' features are invariant to skeleton geometry and camera view and allow ViA to facilitate both, cross-subject and cross-view action classification tasks. We conduct a study focusing on transfer-learning for skeleton-based action recognition with self-supervised pre-training on real-world data (e.g., Posetics). Our results showcase that skeleton representations learned from ViA are generic enough to improve upon state-of-the-art action classification accuracy, not only on 3D laboratory datasets such as 60 and 120, but also on real-world datasets where only 2D data are accurately estimated, e.g., Toyota Smarthome, UAV-Human and Penn Action.
8.18 Self-supervised video representation learning via latent time navigation
Participants: Di Yang, Antitza Dantcheva, François Brémond.
Self-supervised video representation learning aimed at maximizing similarity between different temporal segments of one video, in order to enforce feature persistence over time. This leads to loss of pertinent information related to temporal relationships, rendering actions such as `enter' and `leave' to be indistinguishable. To mitigate this limitation, we propose Latent Time Navigation (LTN), a time-parameterized contrastive learning strategy that is streamlined to capture fine-grained motions. Specifically, we maximize the representation similarity between different video segments from one video, while maintaining their representations time-aware along a subspace of the latent representation code including an orthogonal basis to represent temporal changes. Our extensive experimental analysis suggests that learning video representations by LTN consistently improves performance of action classification in fine-grained and human-oriented tasks (e.g., on Toyota Smarthome dataset). In addition, we demonstrate that our proposed model, when pre-trained on Kinetics-400, generalizes well onto the unseen real-world video benchmark datasets UCF101 and HMDB51, achieving state-of-the-art performance in action recognition.
8.19 Fall detection in untrimmed videos
Participants: Abdoul Djalil Ousseini Hamza, Yoann Torrado, Jose Francisco Saray, François Brémond.
Activity recognition systems which can detect falls have become popular due to the need to provide a safe, comfortable and independent living environment for elderly people. However, many of these systems require wearable sensors. Our approach, unlike these systems, does not require any sensor but uses a camera instead. We use UNIK 62, a skeleton-based activity recognition framework, and adapt it to be able to detect fall actions on untrimmed videos.
UNIK can effectively learn spatio-temporal features and generalize across datasets, we adapt it for fall detection. We use MediaPipe Pose 58 as pose extractor due to its fast and precise pose estimation. We extract 17 body landmarks and this gives us the possibility to do transfer learning with Posetics 62, which contains 142 000 clips with 2D skeletons of 17 body landmarks. Extracting new body landmarks (from 13 to 17) and doing transfer learning enhance the precision.
We use PKU-MMD dataset to fine-tune and evaluate our model. PKU-MMD is a multi-modal dataset for activity recognition with 1076 long untrimmed videos, including 400 fall events, and 50 other daily actions, filmed by 3 camera views and done by 66 unique subjects. Our contribution is to adapt UNIK for fall detection on untrimmed videos, develop an end-to-end fall detection system and post-process the results to determine the temporal localization of the fall event during an online video. The final goal is to integrate the fall detection system into an on-board device. Our demo can work on online simulated videos and online streaming videos.
In the future, we plan to refine our annotations on PKU, add new varieties of fall events from other datasets: NTU, MMact, UR-Fall, UP-Fall. We are also working on distinguishing between dangerous falls and non-dangerous falls by determining the time during which fallen persons stay on the floor.
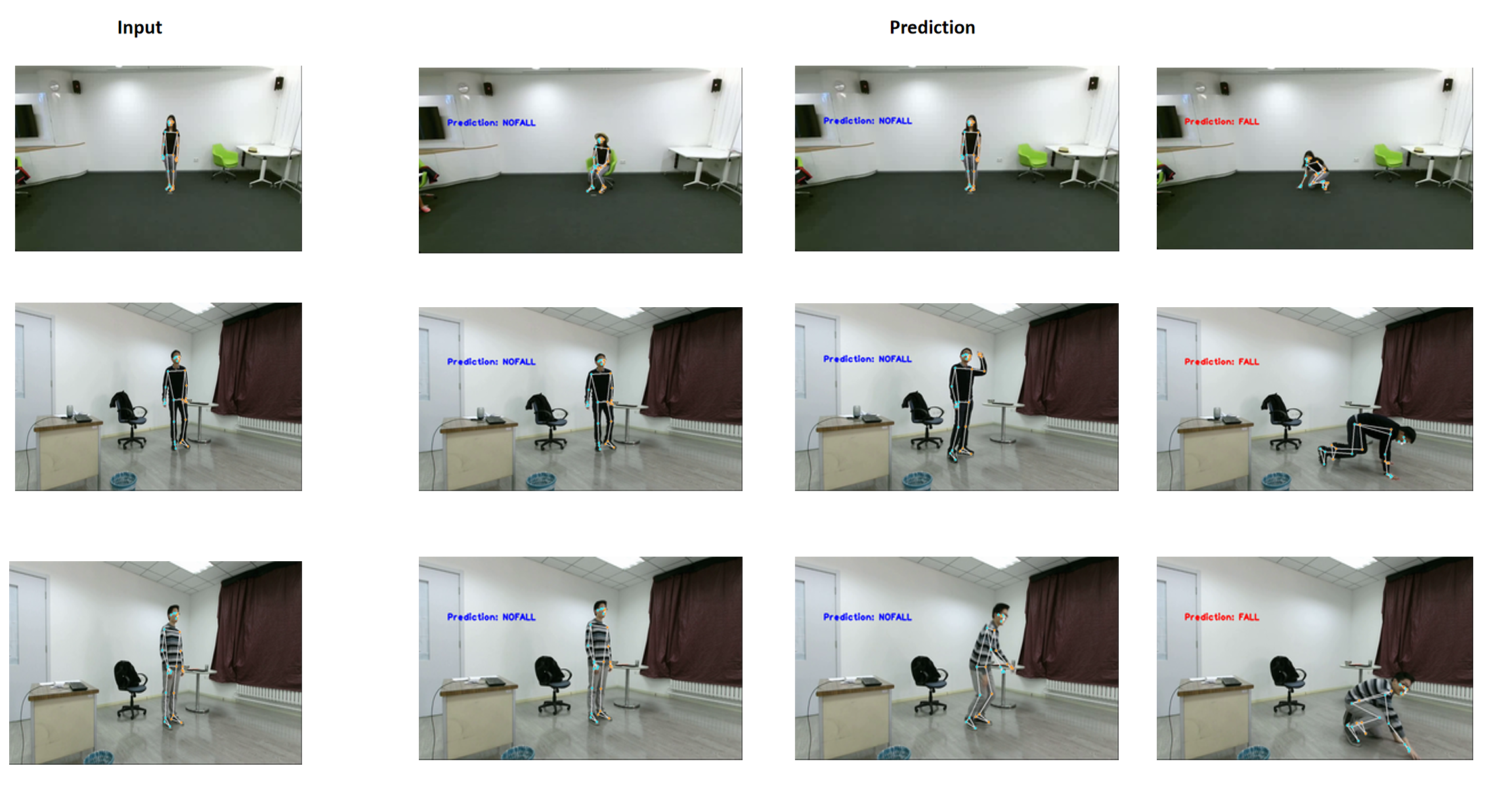
Figure 10
8.20 Multimodal Vision Transformers with Forced Attention for Behavior Analysis
Participants: Tanay Agrawal, Michal Balazia, François Brémond.
Human behavior understanding requires looking at minute details in the large context of a scene containing multiple input modalities. It is necessary as it allows the design of more human-like machines. While transformer approaches have shown great improvements, they face multiple challenges such as lack of data or background noise. To tackle these, we introduce the Forced Attention (FAt) Transformer which utilize forced attention with a modified backbone for input encoding and a use of additional inputs. In addition to improving the performance on different tasks and inputs, the modification requires less time and memory resources. We provide a model for a generalized feature extraction for tasks concerning social signals and behavior analysis 32. Our focus is on understanding behavior in videos where people are interacting with each other or talking into the camera which simulates the first person point of view in social interaction. FAt Transformers are applied to two downstream tasks: personality recognition and body language recognition. We achieve state-of-the-art results for Udiva v0.5, First Impressions v2 and MPII Group Interaction datasets. Figure 1 shows the architecture of the main branch of our network. This work has been published in the IEEE/CVF Winter Conference on Applications of Computer Vision (WACV) 2023. It was completed in collaboration with Dr. Michal Balazia and Dr. Philipp Muller (from DFKI, Saarbrucken, Germany), under the supervision of Dr. Francois Bremond.
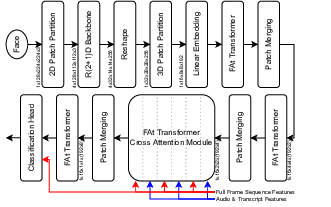
Figure 11
Udiva v0.5 Dataset
Figure 2 shows clips from Udiva v0.5 dataset. The dataset consists of two people interacting and recordings are done as shown in the figure. We only use the FC view.
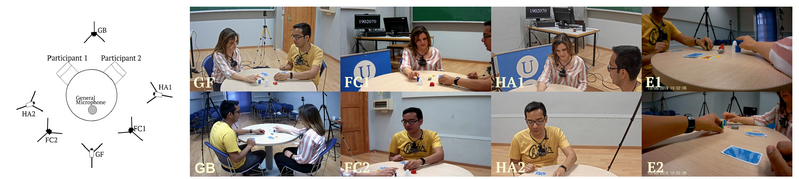
Figure 12
8.21 Online Action Detection
Participants: Mohammed Guermal, Rui Dai, François Brémond.
Action recognition is a fundamental task in computer vision with numerous applications in areas such as video surveillance, sports analysis, and human-computer interaction. With the proliferation of online video content and the increasing use of wearable cameras, there is a growing need for effective techniques for recognizing actions in real-time as they occur. Moreover, in real-life scenarios, it is often not sufficient to have detection in real-time. Hence, there is a need to anticipate some of these actions ahead of time. This could be very important in many fields, for instance, for monitoring of elders, security checking or online danger detection. In this section, we present a novel method that combines action anticipation and online action detection in a way that improves both tasks, and can tackle mainly the limitation of lack of future knowledge in online action detection. We also introduce the state-of-the-art in online action recognition, including key challenges and promising directions for future research in this important field.
In real life, human perception of actions usually predicts the up-coming actions ahead of time based on past knowledge and updates its perception based on the current information, since humans have the ability to capture complex dependencies. In this work we believe that transferring this behavior into computer vision models can greatly improve related results. Lately, transformers 59, 57, 50 have had huge impact in computer vision and video analysis, due to their capacity in capturing long range dependencies equally or better than humans. However, in order to achieve their full capacity, it is remains necessary to have ull knowledge of past, present and future to better learn action dependencies and relations. However, in OAD models we do not have the access to the full sequences, as we have to deal with real time recognition.
Earlier works, such as LSTR 60, TesTra 63 or FUTR 53, propose to tackle online action detection or action anticipation as different tasks. All the previously introduced methods use transformers backbones, achieving good results. We believe that this has not reached the full capacity of transformers. In fact, when it comes to complex and densely annotated datasets some actions can be dependent of each other but still they can occur at distant time steps, and that is why generally OAD models are only validated on simple activity datasets. Even-though they use transformers, it is generally hard to build knowledge on long-range actions dependencies, given that we do not have access to the full information. When compared to off-line action detection, online action detection is behind w.r.t. accuracy, mainly due to the fact that it does not have the access to the whole information, e.g., the future. According to this reasoning, we proposed to indirectly add future information to online action detection by introducing the action anticipation task to it. By doing so, online action models and transformers can have access to more descriptive features (past, present and pseudo-future), and so these transformer models can better capture long-range dependencies and optimize their predictions.
In this project we follow previous works by extracting features from the video clips using 3D convolutions neural networks (3D CNNs). We use I3D 51 as backbone pre-trained on kinetics dataset 55, we call these extracted features a memory bank. In JOADAA we have 3 main parts. First, past predictions: at this step we look at past information by using a transformer encoder, since transformers have proven their capacity in capturing fine-grained long-range dependencies. The output of the encoder is first passed through a classification layer which helps improve the embedding quality by making it class dependent. Secondly, we have the anticipation prediction: in this step we assume we don't have yet the current frame, and so our model using a transformer decoder and the past embedding of the first layer, learns to anticipate the up-coming action in the next frames. Finally, the online action prediction layer uses the anticipation embedding and the current frame features to predict in real time the ongoing action.
In this work we present the following contributions:
- A uniform method that performs both action anticipation and online action detection in a joint manner.
- A new method able to improve existing online action detection methods by introducing action anticipation into their models.
- We also present an ablation study on short-term and long term past information use in different datasets and propose solutions on how to improve it.
- Finally, our method achieves SOTA on both online action detection and action anticipation tasks for two challenging datasets.
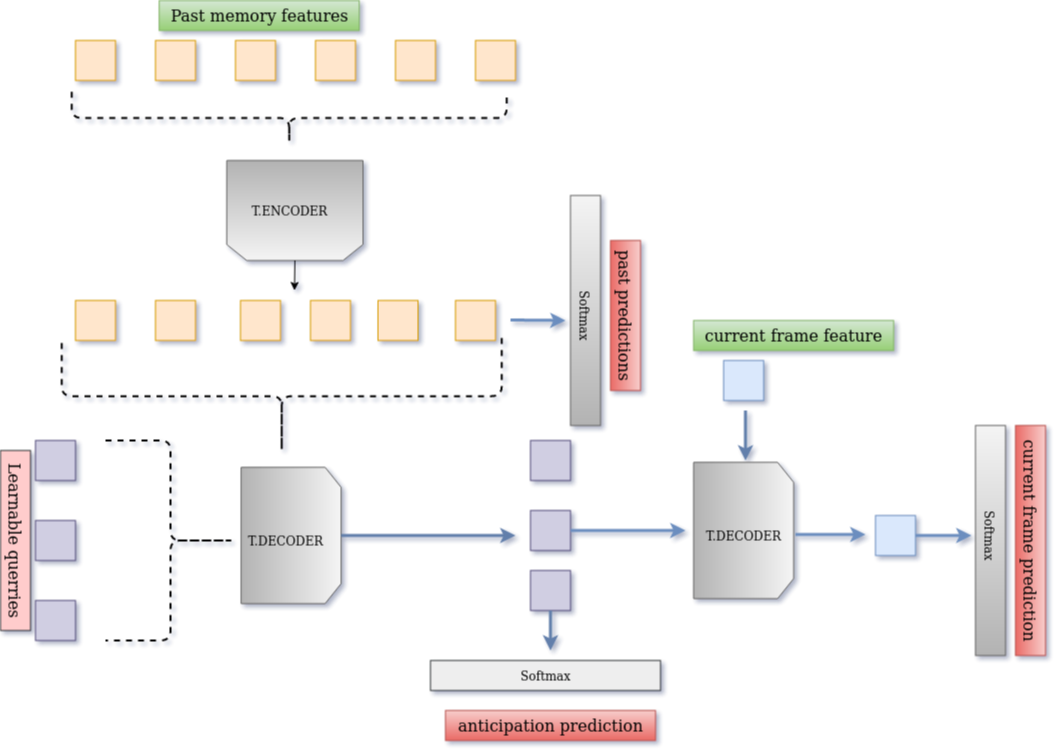
Figure 13
8.22 Vision-based Seizure Classification
Participants: Jen-Cheng Hou, Monique Thonnat.
The motivation of this research is to develop methods based on recent machine learning techniques to provide objective analysis for clinical seizure videos. The clinical signs or semiology are evaluated by neurologists, but the subjective interpretation is liable for inter-observer variability. Hence, there is an urgent need to build an automated system to analyze seizure videos with the latest computer vision progress. We have developed a framework which utilizes multi-stream information from appearance and key-points for both the bodies and faces of the patients. We applied this framework for distinguishing ES with emotion/non-emotion and dystonia/non-dystonia based on the face and body streams in the method. The LOSO validation gives satisfactory results, indicating our model can capture effective spatio-temporal features for face and body for seizure analysis. This work has been published in a clinical journal 23. An issue is the limited number of available seizure videos from real patients to learn the pertinent features for seizure classification. Thus, we have also developed a Transformer-based self-supervised pre-training framework for learning features suitable for the downstream task, i.e. classifying epileptic seizures (ES) and psychogenic non-epileptic seizures (PNES) videos. In our work, a Transformer-based model is pre-trained on a large volume of contextual videos with denoising pre-training objectives. The contextual videos cover the daily behaviors of patients in the Video-SEEG/Video-EEG monitoring unit, and they are easier to access and collect. By simply fine-tuning the pre-trained model with a minimum model modification, the experimental classification results can compete with methods from other state-of-the-art works for similar tasks. A total of 81 patients are involved, in which the ES and PNES class has 52 and 29 patients, respectively. The length of seizure videos ranges from 7 seconds to 150 seconds. We perform a leave-one-subject-out (LOSO) validation for evaluation. The F1-score and the accuracy are 0.82 and 0.75, respectively. This work has been published in a signal processing conference 40.
8.23 Action detection for untrimmed videos based on deep neural networks
Participants: Rui Dai, François Brémond.
Understanding human behaviour and its activities facilitate the advancement of numerous real-world applications and is critical for video analysis. Despite the progress of action recognition algorithms in trimmed videos, the majority of real-world videos are lengthy and untrimmed with dense regions of interest. An effective real-world action understanding system should be able to detect multiple actions in long untrimmed videos. In this thesis 46, we focus mainly on temporal action detection in untrimmed videos, which aims at finding the action occurrences along time in the video. Specifically, temporal action detection methods face three main challenges: (a) modelling in a video the temporal dependencies between actions, including composite and co-occurring actions, (b) learning the representation of fine-grained actions as well as (c) learning a representation from multiple modalities.In this thesis, we first introduce a large indoor action detection benchmark: Toyota Smarthome Untrimmed, which provides spontaneous activities with rich and dense annotations to address the detection of complex activities in real-world scenarios. After that, we propose multiple novel approaches towards action detection in untrimmed videos. These approaches are targeting the aforementioned three challenges: Firstly, we study temporal modelling for action detection. Specifically, we study how to enhance temporal representation using self-attention mechanisms. Our proposed methods allow for processing long-term video and for reasoning about temporal dependencies between video frames at multiple time scales. Secondly, we explore how to recognize and detect fine-grained actions using semantics of object and action contained in the video. In this work, we propose a general semantic reasoning framework. This framework consists of mainly two steps: (1) extracting the semantics from the video to form a structural video representation; (2) enhancing the video representation by reasoning about the extracted semantics. The proposed semantic reasoning strategy improves the detection of fine-grained actions and shows its effectiveness in action recognition and detection tasks. Thirdly, we tackle the problem on how to represent untrimmed video using multiple modalities for action detection. We propose two cross-modality baselines based either on attention mechanism or on knowledge distillation. Both methods leverage the additional modalities to enhance RGB video representation resulting in better action detection performance.Our methods have been extensively evaluated on challenging action detection benchmarks. The proposed methods outperform previous methods, significantly pushing temporal action detection to real-world deployments.
8.24 Human-Scene Network: A Novel Baseline with Self-rectifying Loss for Weakly supervised Video Anomaly Detection
Participants: Snehashis Majhi, Rui Dai, François Brémond.
Video anomaly detection in surveillance systems with only video-level labels (i.e. weakly-supervised) is challenging. This is due to, (i) the complex integration of human and scene based anomalies comprising of subtle and sharp spatio-temporal cues in real-world scenarios, (ii) non-optimal optimization between normal and anomaly instances under weak supervision. In this paper, we propose a Human-Scene Network 48 to learn discriminative representations by capturing both subtle and strong cues in a dissociative manner. In addition, a self-rectifying loss is also proposed that dynamically computes the pseudo temporal annotations from video-level labels for optimizing the Human-Scene Network effectively. The proposed Human-Scene Network optimized with self-rectifying loss is validated on three publicly available datasets i.e. UCF-Crime, ShanghaiTech and IITB-Corridor, outperforming recently reported state-of-the-art approaches on five out of the six scenarios considered.
8.25 MVVM: Multi-View Video Masked Autoencoder for Emotion Recognition
Participants: Valeriya Strizhkova, Laura Ferrari, Antitza Dantcheva, François Brémond.
Masking and reconstruction strategy is an efficient solution to self-supervised video pre-training. Video masked autoencoder (VideoMAE) has shown state-of-the-art results in action recognition both on big and small datasets. Here we expand VideoMAE, proposing a more challenging pre-task that reconstructs different views of a masked input, the Multi-View Video Masked (MVVM) strategy. As a downstream task we select emotion recognition and we use MEAD as the enabling dataset, where subjects are recorded from different angulation (e.g. front, top, down, lateral etc.). The reconstruction of different views allows the model to learn more powerful representations for each frame. Even small facial expressions, visible only from some views, are encoded in the latent space. With this approach we show for the first time we build the end-to-end video emotion classification with the big ViT-B network. We increase the recognition of low intensity/subtle emotions of around 8%, when compared with state-of-the-art methods. The capability of classifying sub-categories with fine-tuning is also tested on a very small (200 videos) in-the-wild dataset (MFA dataset) where multiple shades of anger are represented. The MVVM autoencoder is able to transfer knowledge and reach state-of-the-art emotion recognition accuracy.
8.26 Multimodal Personality Recognition using Cross-Attention Transformer and Behaviour Encoding
Participants: Tanay Agrawal, Michal Balazia, François Brémond.
Personality computing and affective computing have gained recent interest in many research areas. The datasets for the task generally have multiple modalities like video, audio, language and bio-signals. In this work 31, we propose a flexible model for the task which exploits all available data. The task involves complex relations and to avoid using a large model for video processing specifically, we propose the use of behaviour encoding which boosts performance with minimal change to the model. Cross-attention using transformers has become popular in recent times and is utilised for fusion of different modalities. Since long term relations may exist, breaking the input into chunks is not desirable, thus the proposed model processes the entire input together. Our experiments show the importance of each of the above contributions.
8.27 Analysis of autism spectrum disorders
Participants: Po-Han Wu, Abid Ali, François Brémond.
One of the main diagnostic criteria for autism spectrum disorders (ASD) is the identification of stereotyped behaviors. However, it is based mainly on parental interviews and clinical observations, resulting in a prolonged diagnostic cycle that does not allow children with ASD to receive timely treatment. In addition to understanding behavioral patterns, gaze has played an integral role in aiding clinicians in identifying ASD in young children. Modern computer vision technology has been shown to help analyze gaze and capture important features that can help identify the presence of ASD. First, we predict the child gaze position in the extreme situations (with or without face appearance). Secondly, we tried to study the child gaze-attention by classifying the child gaze into three different categories (looking at face, looking at objects, and others). Finally, considering privacy concerns, we performed experiments using three different modalities (RGB, Pose, and Depth). These results show that in addition to RGB, other modalities could also be used to predict the gaze position of children with ASD, hence preserving privacy.
Informations
The Activis gaze dataset consists of more than a thousand video clips of 60 children recording during diagnosis. Around 30 video clips were selected to further conduct gaze prediction experiments. These videos were annotated frame-by-frame for gaze information.
Based on the gaze-attention behavior of both the child and the clinician, the data were divided into three different classes. “Face”, which means that a person (child or clinician) is looking at another person's face; “Object”, means that a person is looking at an object which is related or interactive during the assessment sessions; and “Other” means that a person is neither focusing or gazing on “Face” or “Object”. The Depth and Skeleton information were extracted using SOTA methods, including MiDaS and HRFormer, respectively.
We adapt the SOTA gaze prediction model proposed by our collaborator at Idiap 54. The model inputs three different modalities (RGB/Depth/Pose) separately or combined to predict the gaze position. We further fine-tuned their model on our own dataset to achieve the best results. We achieved an L2 distance of 0.1/0.19 after/before fine-tuning.1 A visualization of gaze prediction on all three modalities is given in Fig. 1. Our next step is to use our classification strategy to further classify the gaze-attention behavior of the child with that of the clinician.
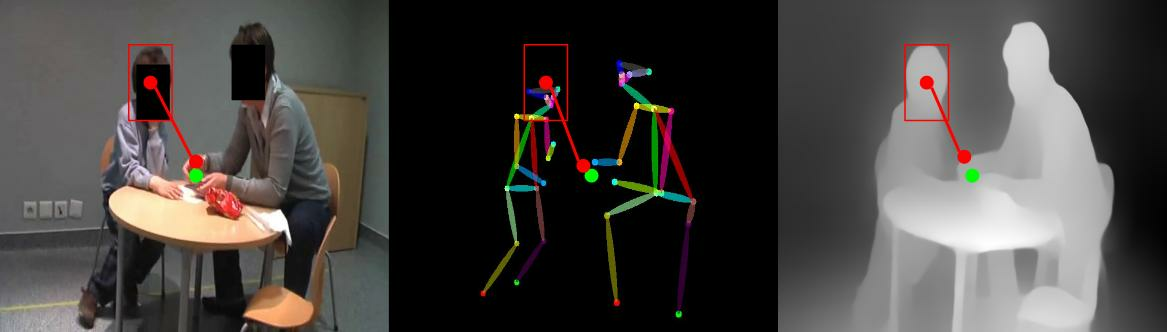
Figure 14
8.28 Video-based Behavior Understanding of Children for Objective Diagnosis of Autism
Participants: Abid Ali, Susanne Thümmler, François Brémond.
This work has been carried out in collaboration with Lenval Hospital on the project "Activis".
One of the major diagnostic criteria for Autism Spectrum Disorder (ASD) is the recognition of stereotyped behaviors. However, it is based primarily on parental interviews and clinical observations, resulting in a prolonged diagnosis cycle that prevents children with ASD from receiving timely treatment. To help clinicians speed up the diagnosis process, we propose a computer vision-based solution 33. First, we collected and annotated a novel dataset for action recognition tasks in videos of children with ASD in an uncontrolled environment. Based on the nature of the data type, we split the actions into two parts, Short actions and Task-based actions. Second, we propose a multimodality fusion network based on 3D CNNs for short-actions. Lastly, we dealt with the task-based actions proposing a multi-stream X3D with different level fusions for child-clinician interaction understanding. The results of our architectures demonstrate the potential of an action-recognition-based system to assist clinicians with a reliable, accurate, and timely diagnosis of ASD disorder.
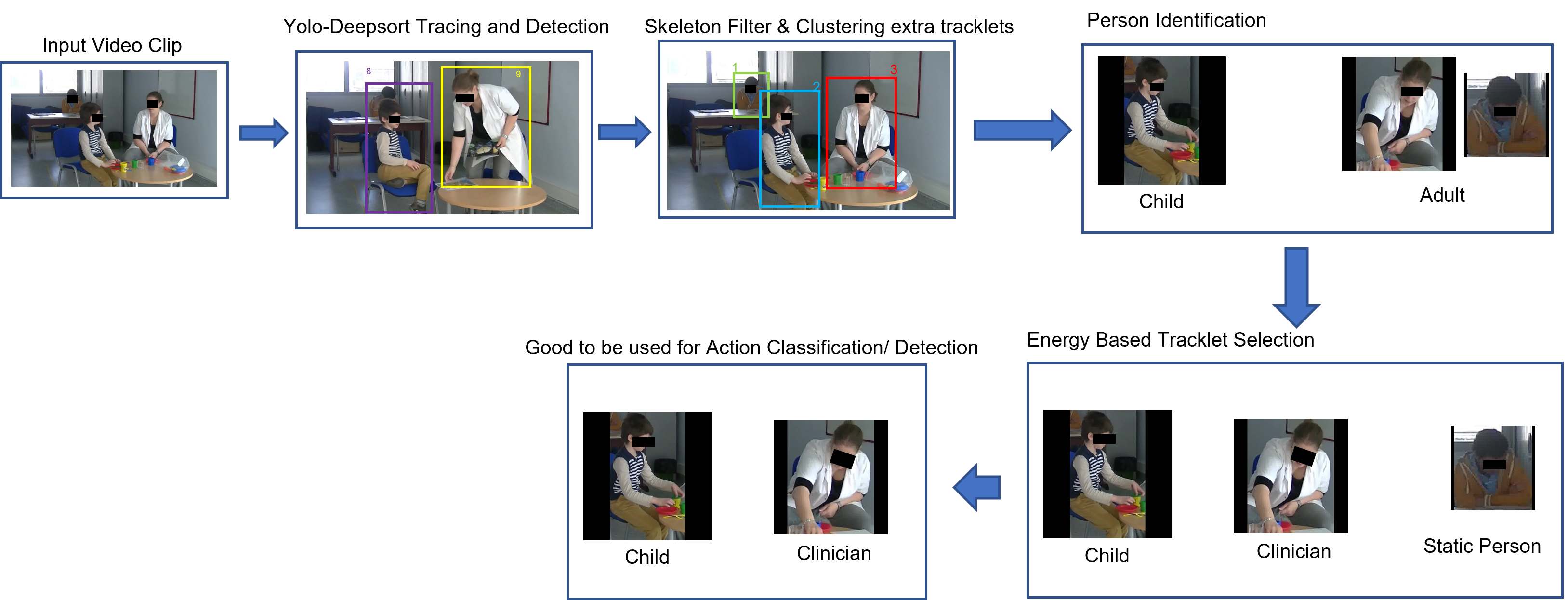
Based on the nature of our dataset, we pre-process our data; the steps involved are Person Detection, Tracking, Clustering, Energy-based tracklet selection, and Person-Identification. An overview of the entire process is illustrated in Figure 15. A novel two-stream architecture is proposed for the recognition of the child-clinician interaction. A modified X3D model (in Fig. 17) is utilized with fusion at different levels. We also explore different attention mechanisms to further improve the model prediction.
Task-based actions are different tasks (long action occurs within a time span of 2 - 10 min) carried out with the child to analyze his ASD behavior based on the ADOS diagnosis tool. These activities infect a composition of several small activities that occur within a task. For example, task Anniversary is a composition of certain actions like pick cup, take toy knife, cut fake cake, and give cup etc.. Therapists use these tasks to diagnose the child for his or her behavior with ASD.
The dataset includes 845 videos from 10 task-based action classes. We achieved an accuracy of 60% with a late fusion between the child-clinician branch, a confusion matrix, and the results are given in Fig. 16. A paper on this work is in progress for MICCAI 2023.
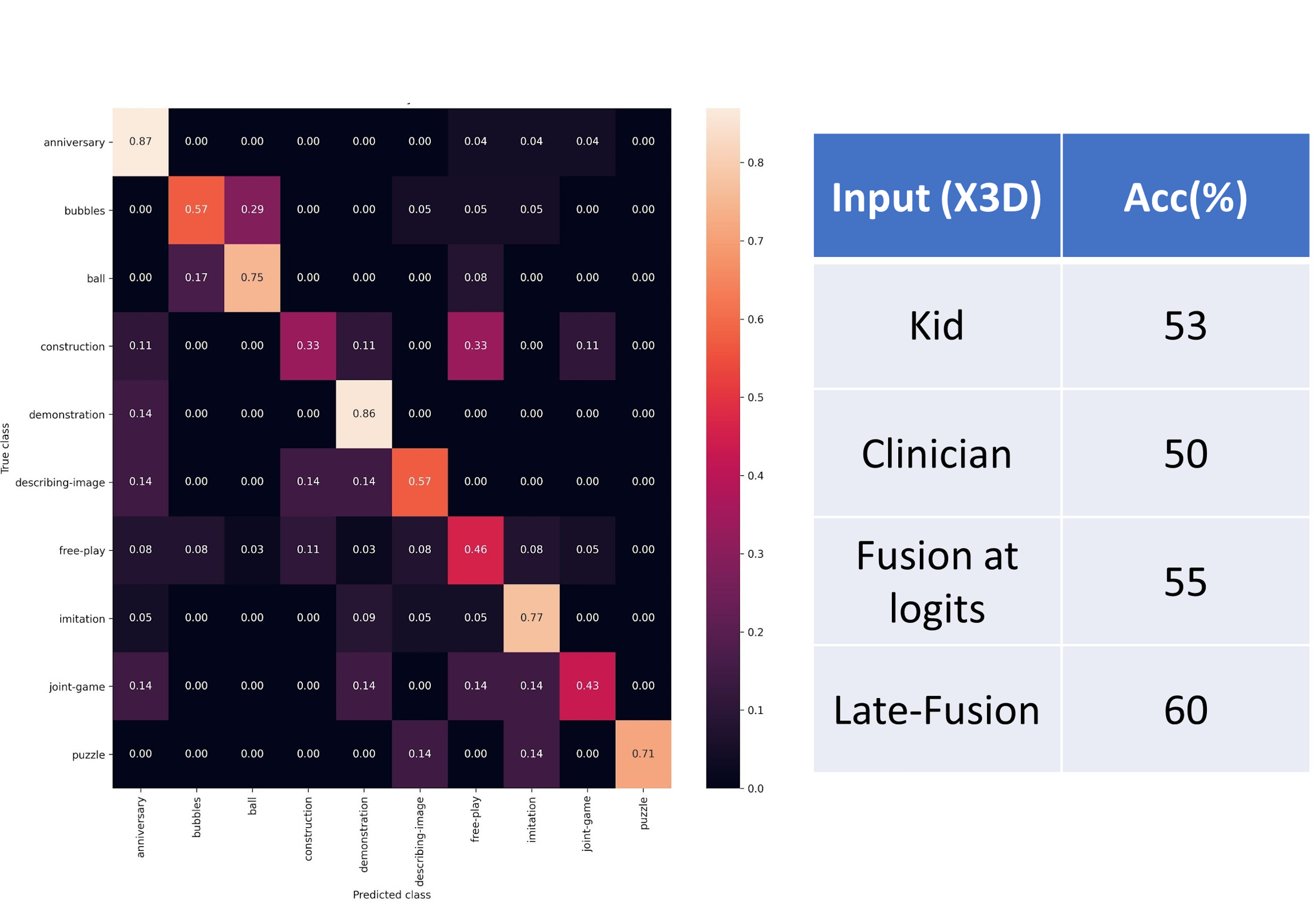
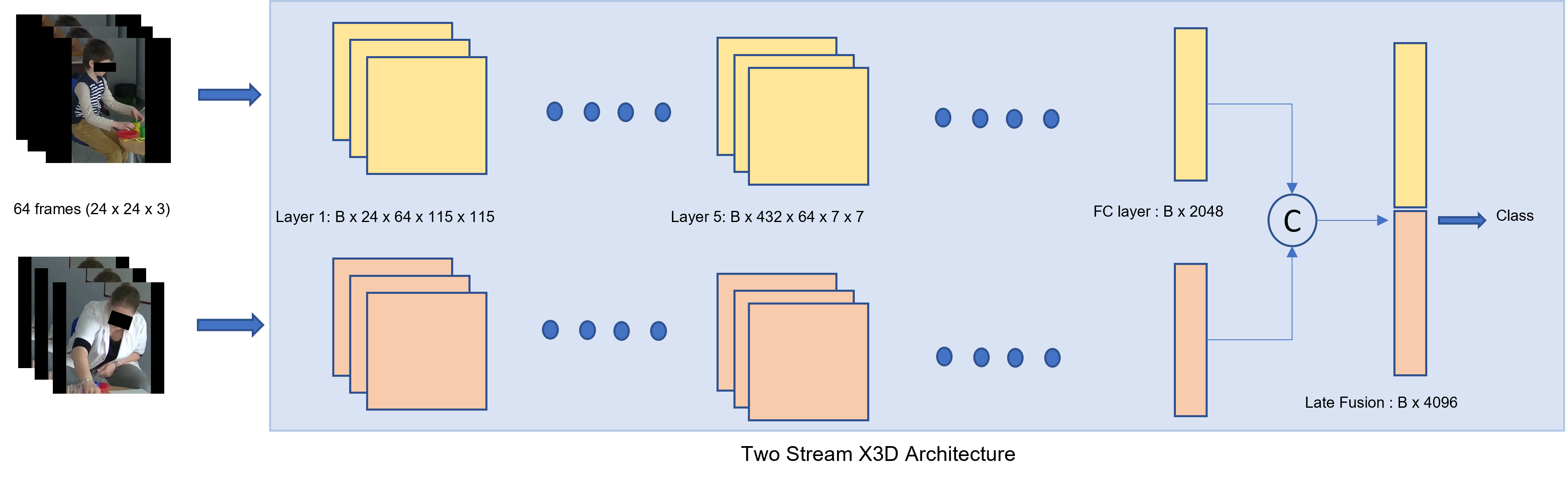
Figure 17
8.29 Bodily Behaviors in Social Interaction
Participants: Michal Balazia, Akos Levente Tanczos, François Brémond.
Body language is an eye-catching social signal and its automatic analysis can significantly advance artificial intelligence systems to understand and actively participate in social interactions. While computer vision has made impressive progress in low-level tasks like head and body pose estimation, the detection of more subtle behaviors such as gesturing, grooming, or fumbling is not well explored.
In 35 we present BBSI, the first set of annotations of complex Bodily Behaviors embedded in continuous Social Interactions in a group setting. Based on previous work in psychology, we manually annotated 26 hours of spontaneous human behavior in the MPIIGroupInteraction dataset with 15 distinct body language classes based on the the Ethological Coding System for Interviews (ECSI). This coding system includes many bodily behaviors that were shown to be connected to different social phenomena. We selected all ECSI behaviors involving the limbs and torso and excluded behavior classes based on facial behavior, gaze, and head pose as these are not the focus of this work and highly accurate methods to analyze such behaviors already exist. We also excluded the two classes Crouch and Relax, as they were only very rarely annotated. In addition to the bodily behaviors included in ECSI, we scanned the MPIIGroupInteraction dataset for additional behaviors that occur frequently and carry potential meaning in a social situation. As a result, we included the five additional classes: Adjusting Clothing, Leg Movement, Legs Crossed, Smearing Hands, Stretching. Screenshots of some of these classes are presented in Figure 18. We present comprehensive descriptive statistics on the resulting dataset as well as results of annotation quality evaluations.
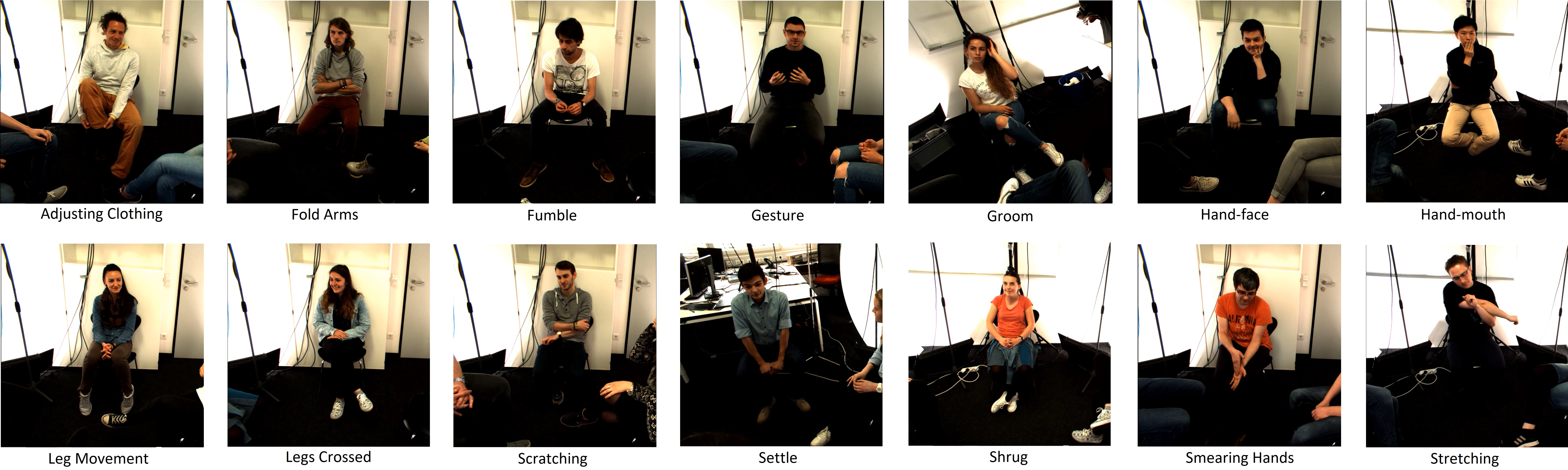
Figure 18
For automatic detection of the 15 behaviors, we adapt the Pyramid Dilated Attention Network (PDAN), a state-of-the-art approach for human action detection. We perform experiments using four variants of spatial-temporal features as input to PDAN: Two-Stream Inflated 3D CNN, Temporal Segment Networks, Temporal Shift Module and Swin Transformer. Results are promising and indicate a great room for improvement in this difficult task. Representing a key piece in the puzzle towards automatic understanding of social behavior, BBSI is fully available to the research community.
8.30 Phenotyping of Psychiatric Disorders from Social Interaction
Participants: Alexandra Koenig, François Brémond, Michal Balazia.
Identifying objective and reliable markers to tailor diagnosis and treatment of psychiatric patients remains a challenge, as conditions like major depression, bipolar disorder, or schizophrenia are qualified by complex behavior observations or subjective self-reports instead of easily measurable somatic features. Recent progress in computer vision, speech processing and machine learning has enabled detailed and objective characterization of human behavior in social interactions. However, the application of these technologies to personalized psychiatry is limited due to the lack of sufficiently large corpora that combine multi-modal measurements with longitudinal assessments of patients covering more than a single disorder. To close this gap, we introduce Mephesto, a multi-centre, multi-disorder longitudinal corpus creation effort designed to develop and validate novel multi-modal markers for psychiatric conditions. Mephesto will consist of multi-modal audio-, video-, and physiological recordings as well as clinical assessments of psychiatric patients covering a six-week main study period as well as several follow-up recordings spread across twelve months.
In 28, we outline the rationale and study protocol and introduce four cardinal use cases that will build the foundation of a new state of the art in personalized treatment strategies for psychiatric disorders. The overall study design is presented in Figure 19 and consists of two phases. During the main study phase, interactions between the patients and clinician will be recorded multimodally, i.e. with video, audio, and physiological sensors. In the succeeding follow-up phase videoconference-based recordings and ecological momentary assessments will be audio and video recorded with a videoconferencing system.
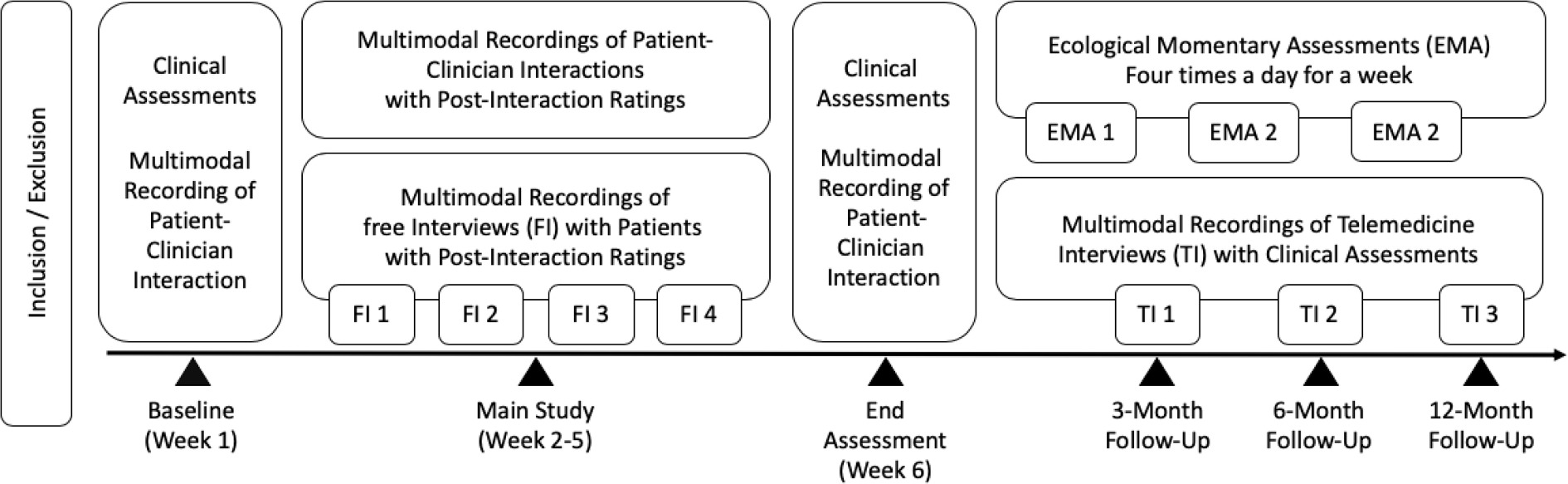
Figure 19
In addition to the protocol, we are collecting a large multi-modal dataset of patient-clinician interaction under the name Mephesto. The dataset is being recorded at multiple locations:
- Nice – Hospital Pasteur: 150 recordings of 35 patients
- Nice – Centre Therapeutique La Madeleine: 0 recordings of 0 patients
- Homburg – Universitatsklinikum des Saarlandes: 40 recordings of 10 patients
- Oldenburg – Carl von Ossietzky University: 50 recordings of 20 patients
It currently contains patients of schizophrenia, bipolar disease, depression and Alzheimer's disease. There are four clinicians involved in administering the recording process and performing the clinical interviews with the patients. Dataset does not include control subjects. Each patient is contributing with 1–6 videos, roughly 4.2 videos on average. In addition to video, the recordings include patients' and clinicians' biosignals EDA, BVP, IBI, heart rate, temperature, and accelerometer. Videos are recorded by Azure Kinect and biosignals by Empatica. People do not wear face masks while being recorded, although to minimize the transmission of COVID-19 there is a large transparent plexi-glass. Dataset is by default confidential, but many patients agreed to publish their data anonymized or even raw. Figure 20 shows the recording scene with two clinicians on the opposite sides of an office desk, wearing Empatica wristbands and separated by the plexi-glass that is out of camera receptive fields. Screenshot of an example recording with a clinician and a patient is displayed in Figure 21.
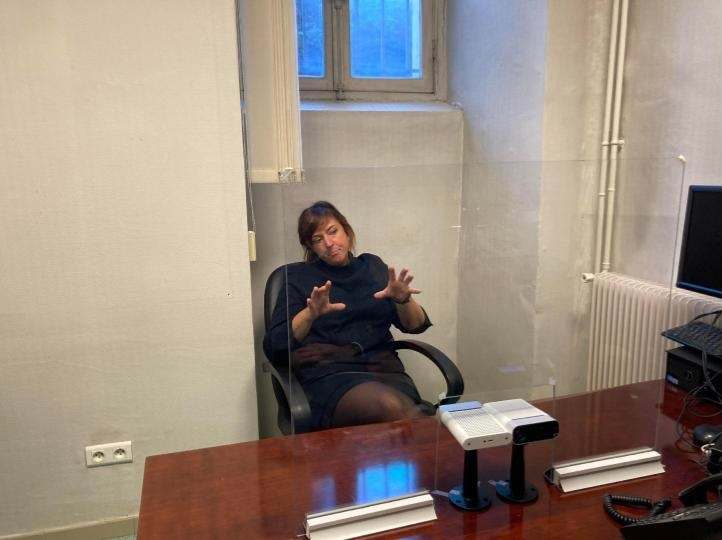
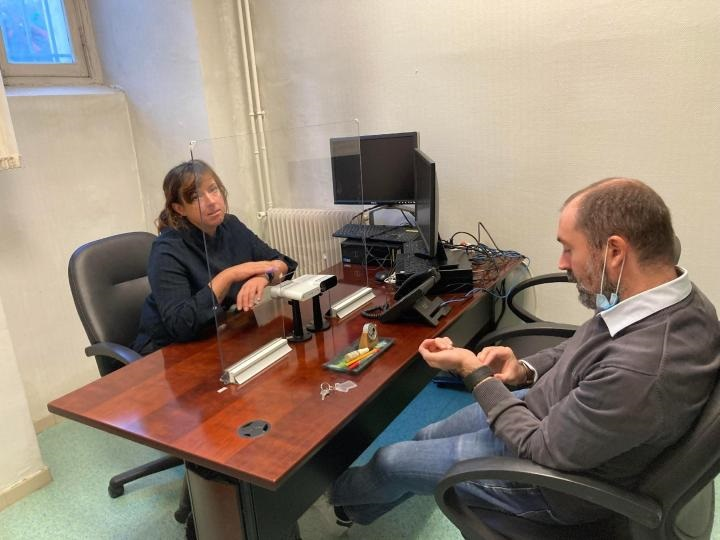
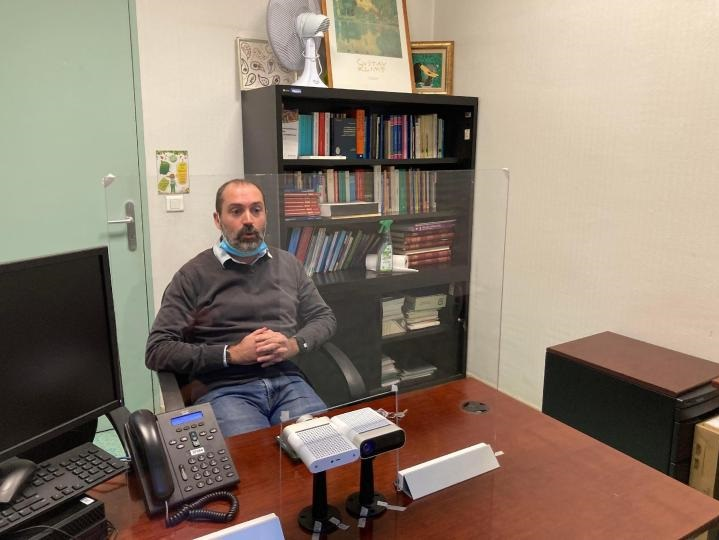
Figure
Figure
Figure
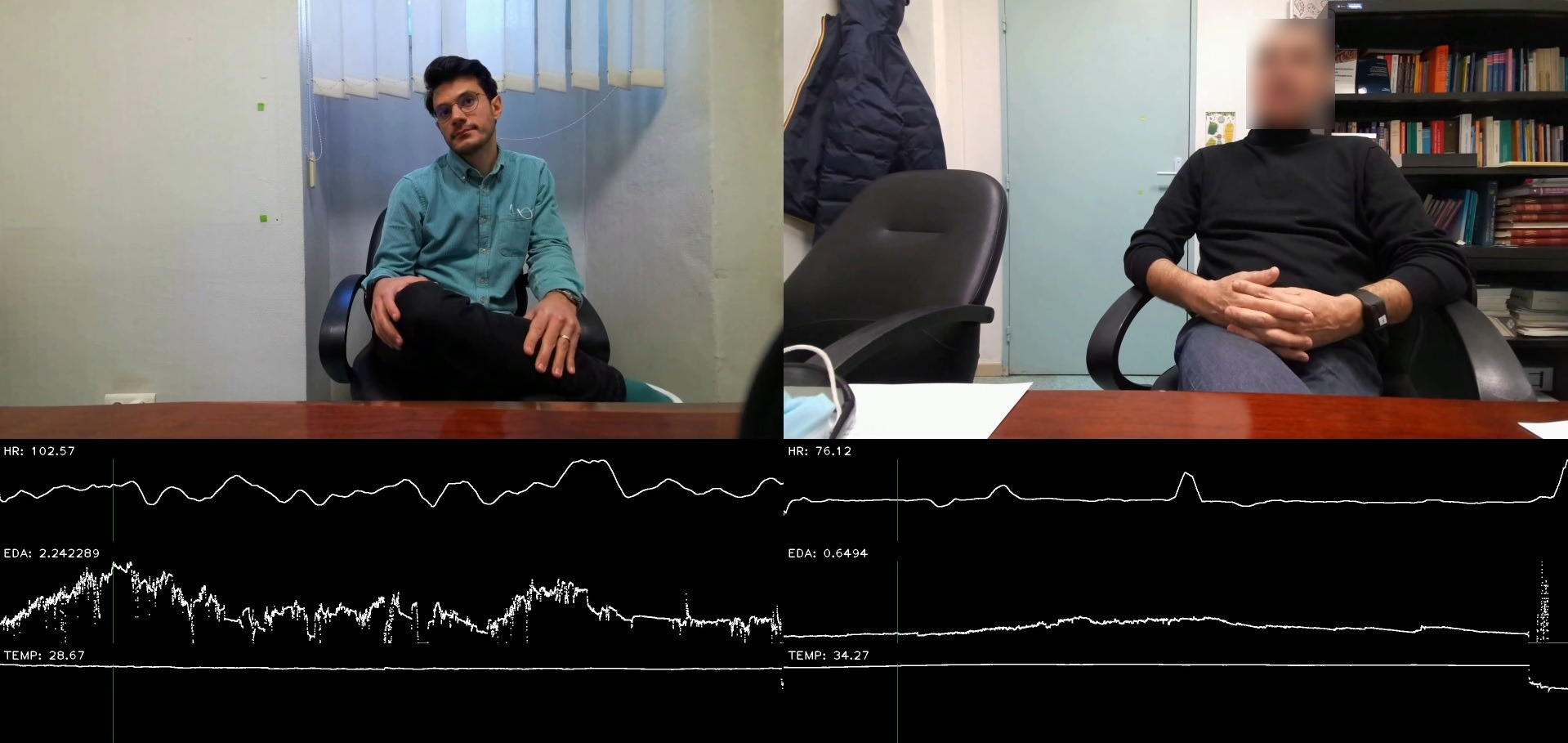
Figure 21
8.31 Formal Probabilistic Model of the Inhibitory Control Circuit in the Brain
Participants: Thibaud L’Yvonnet , Sabine Moisan , Jean-Paul Rigault.
The decline of inhibitory control efficiency in aging subjects with neurodegenerative diseases, such as Parkinson's disease or Alzheimer's disease with Parkinsonian syndrome, is due to anatomical and functional changes in prefrontal/frontal regions of the brain. This year we propose a probabilistic formal model 47 of the biological neural network governing the inhibitory control function and we study some of its relevant dynamic properties. We also explore how some important parameter variations influence the probability for the model to display some key behaviors. The final aim is to detect sources of pathological behaviors in the neural network responsible for inhibitory control. In the context of early onsets of neuropathologies, this approach is convenient as even healthy subjects are not necessarily expected to ace clinical tests.
A better understanding of the mechanisms of inhibitory control could allow targeted treatments for different classes of patients with dementia. The main advantage of probabilistic models is their ability to represent a wide variability of behavior with a single model. We chose the inhibitory control function because it has been studied for a long time and several models already exist, moreover, it is managed by a restricted amount of brain structures (basal ganglia) which makes it easier to model than other cognitive functions. Basal ganglia contain several anatomical structures e.g., the striatum (STr) and the substantia nigra pars reticulata (SNpr). We use Prism (a state-of-the-art probabilistic model checker) to implement the model and to perform model checking.
The first task was to provide a formal model of the main interactions between the different basal ganglia nuclei. Our model reproduces known biological behaviors from the literature such as the pathway race which describes the inhibitory control function as a competition between a "go" and a "stop" process in the brain. It also faithfully represents the importance of some connections in the pathways. In order to model the interactions of structures of the brain (made of thousands of neurons) while keeping model checking tractable, we introduce a generalization of the Leaky Integrate and Fire (LI&F) neuron to neuron boxes. Each anatomical structure of the inhibitory control circuit is thus represented by probabilistic discrete Markov chains implementing a box of ten neurons of LI&F type. Boxes make it possible to have a behavior relatively close to a small network of neurons, without requiring a lot of computing power. The model is presented in the graph of figure 22.
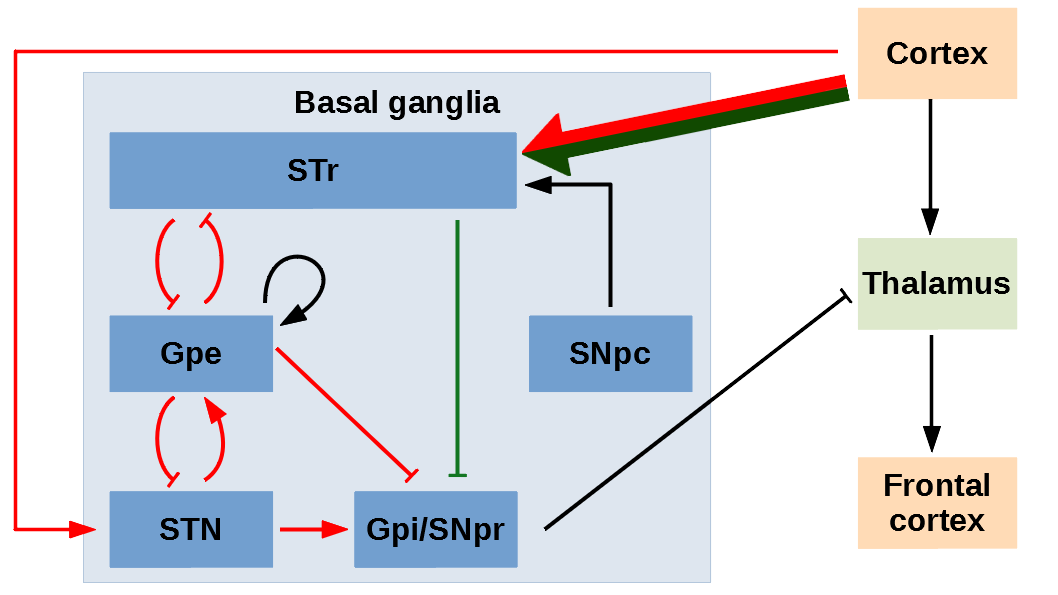
Figure 22
Second, we automatically tested probabilistic temporal properties of this model thanks to model-checking to explore potential sources of pathological behavior in the inhibitory control circuit. Interesting biological behaviors were translated in PCTL logics properties to check the adequacy of the model. We validate the boxes individually, e.g., by verifying that a box does not emit a spike until its potential is greater than a threshold. Then we checked the synchronization of the boxes and their connections, e.g., by computing the probability for STr to be inhibited and SNpr to be activated at a given instant. Prism explicit model checking engine gives the expected valid answers (P=1).
We also ran an experiment to explore the sensitivity of inhibitory control to the modulation of some connections. The modified model complies with Parkinson's disease. Further modifications to represent, e.g., Alzheimer's disease are planned as future work. In the future, the model will also be coupled with the activity model of a patient playing a serious game targeting the inhibitory control function. The goal is to explore modifications in the brain neural network that may generate a patient behavior characteristic of neurocognitive disorders.
This work opens new avenues for the formal modeling of cognitive functions. Moreover, it has proven the feasibility of such model exploration using only off the shelf laptops.
8.32 Datasets for multimodal emotion recognition
Participants: Laura M. Ferrari , François Brémond.
State-of-the-art emotion recognition in machine learning typically relies on the interpretation of dynamic scenes observed by video cameras, especially from facial expressions. The accuracy of computer vision algorithms is limited by the identification of the real emotion. A person may be happy even if she is not smiling and people differ widely in how expressive they are. Recently, multimodal sentiment analysis has been proposed, exploiting multimodal data and fusion methods through deep neural networks architectures. The idea is to combine salient information from different modalities such as RGB cameras, biosensors and audio. Lately, we have worked as editors for a special issue on multimodal emotion recognition with biosignals and video 16. Despite the proven increased accuracy over singular modality the limits of multimodal sentiment analysis are multiple. One of the main open issues is the limited data availability, especially when biosignals are considered. Thanks to STARS expertise in biosensors and biosignals 21, 52, we have designed a protocol and started the acquisition of two novel multimodal datasets with multiple biosignals (e.g. ECG, EDA, Respiration rate), video and audio (in one case) with more than 60 subjects. Having access to large and high-quality datasets will permit to process them following several downstream tasks, such as social interactions analysis and clinical evaluations.
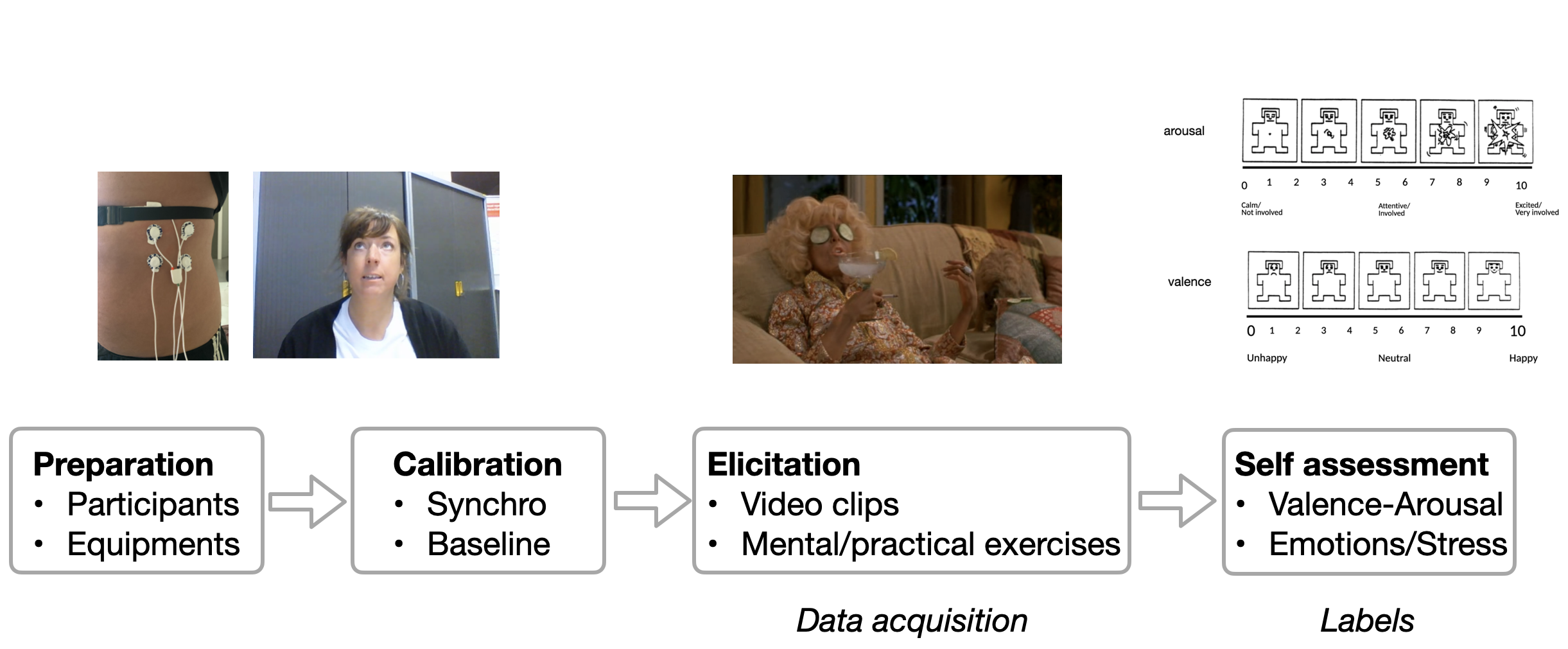
Figure 23
8.33 Activis: 3d-Convolutional-neural network for analysis of Autism Spectrum Disorder
Participants: Ashish Marisetty, Abid Ali, François Brémond.
This work has been carried out in collaboration with Lenval Hospital on the project "Activis".
Recognizing stereotyped behaviors is one of the main diagnostic criteria for Autism Spectrum Disorder (ASD). However, it is based primarily on parental interviews and clinical observations, resulting in a lengthy diagnosis cycle that prevents children with ASD from receiving timely treatment. We propose a computer vision-based solution to assist clinicians in speeding up the diagnosis process. First, we collected and annotated a new dataset for action recognition tasks in uncontrolled videos of children with ASD. Prior to the action recognition task, we needed to identify and extract the information of both the child and the clinician from the whole scene. For this purpose, we propose a novel method for age-based person identification.
Architecture | Loss used | Accuracy |
X3D + Self-Attention + 512-MLP | Finetune + BCE Loss | 93.36% |
X3D + 512-MLP | Semi hard triplet loss | 89.96% |
X3D + Self-Attention + 512-MLP | Semi hard triplet loss | 88.99% |
X3D + 512-MLP | Finetune + BCE Loss | 94.53% |
Age-based Person Identification
Most age-based person identification methods in the literature are single-image-based and limited to face features only. These models fail in real-world situations, where extracting faces can be difficult (tiny, and or blurred faces). Furthermore, in situations such as surveillance, home recordings, or at a health-care facility (for example, in the assessment of ASD), the faces are not always visible, and occlusions or camera angle make estimating age extra difficult for face/image-based architectures. To address these issues, we propose a video-based age-prediction/Person-identification network (Fig. 15). Thanks to 3D-CNNs, we can model temporal information (hence, overcoming the issue of face occlusions). Also, analyzing the full body, can help us capture important information about age (for example, shoulder width, body height etc). Our X3D-inspired architecture can classify the tracklets and filter the child from the clinician and parents in a video. To classify the tracklets, the network architecture incorporates a 512-dimensional projection head on top of the existing classifier, along with a parametric ReLu activation function. Furthermore, we tried to introduce multihead attention and triplet loss over the projection embeddings, but the improvement was not significant. Table. 1 summarizes a few of the key experimental runs. The model achieved a remarkable accuracy of 94.53% in a held-out validation set, saving a significant amount of time and effort in labeling the tracklets. The self-attention models came second, with binary cross-entropy outperforming the triplet loss as the superior loss.
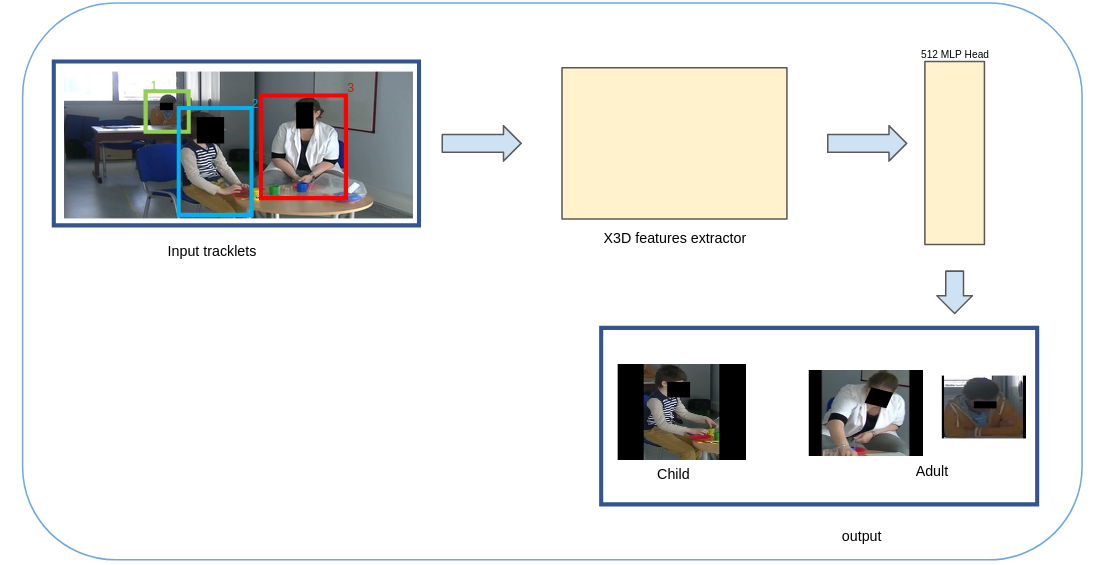
Figure 24
8.34 MePheSTO – Digital Phenotyping for Psychiatric Disorders from Social Interaction
Participants: Alexandra Koenig, François Brémond.
This contribution has to do with the clinical coordination of the INRIA-DFKI joint project “MePheSTO – Digital Phenotyping for Psychiatric Disorders from Social Interaction”. MePheSTO is an interdisciplinary research project that aims to develop a methodology based on artificial intelligence methods for the identification and classification of objective, and thus measurable, digital phenotypes of psychiatric disorders. MePheSTO builds a joint DFKI-INRIA workforce – the foundation for future and innovation projects.
MePheSTO has a solid foundation of clinically motivated scenarios and use-cases (four in total) synthesized jointly with clinical partners. Important to MePheSTO is the creation of a multimodal corpus including speech, video, and biosensors of social patient-clinician interactions in three different clinical sites, which serves as the basis for deriving methods, models and knowledge on psychiatric symptoms.
A set of novel multimodal digital biomarkers derived from the interaction data will be identified and formalized derived from the interaction data corpus allowing reliable phenotyping of the target psychiatric disorders.
The approach and developed methods are validated in at least 2 countries/languages (France and Germany). Important project outcomes include high-impact joint scientific publications as well as presentations at high-impact scientific conferences, PhD theses (supervising 2 PhD students for the project), data corpus collection, Use-case demonstrators validated – at least 2 (F2F and telemedical use-cases) and the successful submission of follow-up research innovation project proposals.
Related to Mephesto, contributions involve the clinical coordination of several other research projects on the use of technological solutions for early assessment of dementia patients.
This was published in 28.
Scientific Progress
In total, we reached approximately 40 inclusions with in average 3-4 recordings.
We also started an initiative to extend data collection in other clinical population such as patients with Autism and Anorexia (collaboration with Lenval Hospital and the Children Psychiatry department).
A follow up European research project (GAIN) was accepted and kicked off in October 2022 which will allow a certain continuation regarding data analysis of the acquired data set.
During 2022, work started on the development of the use-cases demonstrators one for psychiatric remote tele-medicine in which we are mainly active and for face to face consultation.
8.35 Digital phenotyping for differential diagnosis of Major Depressive Episode: A narrative review
Participants: Alexandra Koenig, François Brémond.
Identifying objective and reliable markers to tailor diagnosis and treatment of psychiatric patients remains a challenge, as conditions like major depression, bipolar disorder, or schizophrenia are qualified by complex behavior observations or subjective self-reports instead of easily measurable somatic features. Recent progress in computer vision, speech processing and machine learning has enabled detailed and objective characterization of human behavior in social interactions. However, the application of these technologies to personalized psychiatry is limited due to the lack of sufficiently large corpora that combine multi-modal measurements with longitudinal assessments of patients covering more than a single disorder. To close this gap, we introduce Mephesto, a multicentre, multi-disorder longitudinal corpus creation effort designed to develop and validate novel multi-modal markers for psychiatric conditions.
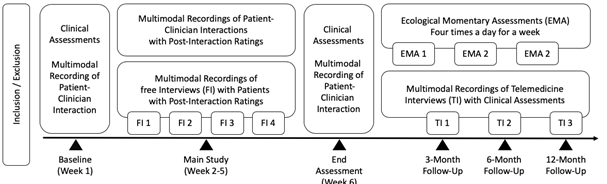
Figure 25
Mephesto will consist of multi-modal audio-, video-, and physiological recordings as well as clinical assessments of psychiatric patients covering a six-week main study period as well as several follow-up recordings spread across twelve months. We outline the rationale and study protocol and introduce four cardinal use cases that will build the foundation of a new state of the art in personalized treatment strategies for psychiatric disorders.
8.36 Detecting subtle signs of depression with automated speech analysis in a non-clinical sample
Participants: Alexandra Koenig, François Brémond.
Background:
Major depressive episode (MDE) is a common clinical syndrome. It can be found in different pathologies such as major depressive disorder (MDD), bipolar disorder (BD), post-traumatic stress disorder (PTSD) or even occur in the context of psychological trauma. However, only one syndrome is described in international classifications (DSM 5/ICD 11), which do not take into account the underlying pathology at the origin of the MDE. Clinical interviews are currently the best source of information to obtain the etiological diagnosis of MDE. Nevertheless, it does not allow an early diagnosis and there are no objective measures of extracted clinical information. To remedy this, the use of digital tools and their correlation with clinical symptomatology seems promising.
Objective:
We aimed to review the current application of digital tools for MDE diagnosis while highlighting shortcomings for further research. In addition, our work was focus on digital devices easy to use during clinical interview and mental health issues where depression is common.
Methods:
We conducted a narrative review of the use of digital tools during clinical interviews for MDE by searching papers published in PubMed/MEDLINE, Web of Science, and Google Scholar databases since February 2010. The search was conducted from June to September 2021. Potentially relevant papers were then compared against a checklist for relevance and reviewed independently for inclusion, with focus on 4 allocated topics of (1) automated voice analysis, (2) behaviour analysis by video and physiological measures, by (3) heart rate variability (HRV) and (4) electrodermal activity (EDA). For this purpose, we were interested in four frequently found clinical conditions in which MDE can occur: (1) MDD, (2) BD, (3) PTSD and (4) psychological trauma.
Results:
A total of 74 relevant papers on the subject were qualitatively analyzed and the information was synthesized. Thus, a digital phenotype of MDE seems to emerge consisting of modifications in speech features (namely temporal, prosodic, spectral, sources, formants and in speech content), modifications in nonverbal behavior (Head, hand, body and eyes movement, facial expressivity and gaze) and a decrease in physiological measurements (HRV and EDA). We found similarities but also differences when MDE occurs in MDD, BD, PTSD or psychological trauma. However, comparative studies were rare in BD or PTSD conditions which do not allow us to identify clear and distinct digital phenotypes.
Conclusion:
Our search identifies markers from several modalities that hold promise for an objective etiological diagnosis of MDE. To validate their potential, further longitudinal and prospective studies are needed.
8.37 Dementia analysis
Participants: Alexandra Koenig, François Brémond.
Background:
Automated speech analysis has gained increasing attention to help diagnosing depression. Most previous studies, however, focused on comparing speech in patients with major depressive disorder to that in healthy volunteers. An alternative may be to associate speech with depressive symptoms in a non-clinical sample as this may help to find early and sensitive markers in those at risk of depression.
Methods:
We included n = 118 healthy young adults (mean age: 23.5 3.7 years; 77% women) and asked them to talk about a positive and a negative event in their life. Then, we assessed the level of depressive symptoms with a self-report questionnaire, with scores ranging from 0–60. We transcribed speech data and extracted acoustic as well as linguistic features. Then, we tested whether individuals below or above the cut-off of clinically relevant depressive symptoms differed in speech features. Next, we predicted whether someone would be below or above that cut-off as well as the individual scores on the depression questionnaire. Since depression is associated with cognitive slowing or attentional deficits, we finally correlated depression scores with performance in the Trail Making Test.
Results:
In our sample, n = 93 individuals scored below and n = 25 scored above cut-off for clinically relevant depressive symptoms. Most speech features did not differ significantly between both groups, but individuals above cut-off spoke more than those below that cut-off in the positive and the negative story. In addition, higher depression scores in that group were associated with slower completion time of the Trail Making Test. We were able to predict with 93% accuracy who would be below or above cut-off. In addition, we were able to predict the individual depression scores with low mean absolute error (3.90), with best performance achieved by a support vector machine.
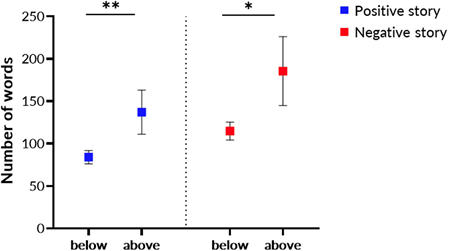
Figure 26
Conclusions:
Our results indicate that even in a sample without a clinical diagnosis of depression, changes in speech relate to higher depression scores. This should be investigated in more detail in the future. In a longitudinal study, it may be tested whether speech features found in our study represent early and sensitive markers for subsequent depression in individuals at risk.
9 Bilateral contracts and grants with industry
Stars team has currently several experiences in technological transfer towards industries, which have permitted to exploit research result.
9.1 Bilateral contracts with industry
9.1.1 Toyota
Toyota is working with Stars on action recognition software to be integrated on their robot platform. This project aims at detecting critical situations in the daily life of older adults alone at home. This will require not only recognition of ADLs but also an evaluation of the way and timing in which they are being carried out. The system we want to develop is intended to help them and their relatives to feel more comfortable because they know that potentially dangerous situations will be detected and reported to caregivers if necessary. The system is intended to work with a Partner Robot - HSR - (to send real-time information to the robot) to better interact with the older adult.
9.1.2 Thales
Thales and Inria jointly explore facial analysis in the invisible spectrum. Among the different spectra low energy infrared waves, as well as ultraviolet waves will be studied. In this context following tasks will be included: 1. We are designing a model to extract biometric features from the acquired data. Analysis of the data related to contours, shape, etc. will be performed. Current methodology cannot be adopted, since colorimetry in the invisible spectrum is more restricted with less diffuse variations and is less nuanced. Then facial recognition will be performed in the invisible spectrum. Expected challenges have to do with limited colorimetry and lower contrasts. In addition to the first milestone (face recognition in the invisible spectrum), there are two other major milestones: 2. Implementation of such a face recognition system, to be tested at the passage of the access portal to a school. 3. Pseudo-anonymized identification within a school (outdoor courtyards, interior buildings). Combining biometrics in the invisible spectra and anonymization within an established group requires removing certain additional barriers that are specific to biometrics but also the use of statistical methods associated with biometrics. This pseudo-anonymized identification must also incorporate elements of information provided by the proposed electronic school IDs.
9.1.3 European System Integration
The company ESI (European System Integration) has a collaboration with Stars, which runs from September 2018 until March 2022 to develop a novel Re-Identification algorithm which can be easily set-up with low interaction for video surveillance applications. ESI provides software solutions for remote monitoring stations, remote assistance, video surveillance, and call centers. It was created in 1999 and ESI is a leader in the French remote monitoring market. Nowadays, ensuring the safety of goods and people is a major problem. For this reason, surveillance technologies are attracting growing interest and their objectives are constantly evolving: it is now a question of automating surveillance systems and helping video surveillance operators in order to limit interventions and staff. One of the current difficulties is the human processing of video, as the multiplication of video streams makes it difficult to understand meaningful events. It is therefore necessary to give video surveillance operators suitable tools to assist them with tasks that can be automated. The integration of video analytics modules will allow surveillance technologies to gain in efficiency and precision. In recent times, deep learning techniques have been made possible by the advent of GPU processors, which offer significant processing possibilities. This leads to the development of automatic video processing.
9.1.4 Fantastic Sourcing
Fantastic Sourcing is a French SME specialized in micro-electronics, it develops e-health technologies. Fantastic Sourcing is collaborating with Stars through the UCA Solitaria project, by providing their Nodeus system. Nodeus is an IoT (Internet of Things) system for home support for the elderly, which consists of a set of small sensors (without video cameras) to collect precious data on the habits of isolated people. Solitaria project performs a multi-sensor activity analysis for monitoring and safety of older and isolated people. With the increase of the ageing population in Europe and in the rest of the world, keeping elderly people at home, in their usual environment, as long as possible, becomes a priority and a challenge of modern society. A system for monitoring activities and alerting in case of danger, in permanent connection with a device (an application on a phone, a surveillance system ...) to warn relatives (family, neighbors, friends ...) of isolated people still living in their natural environment could save lives and avoid incidents that cause or worsen the loss of autonomy. In this RD project, we propose to study a solution allowing the use of a set of innovative heterogeneous sensors in order to: 1) detect emergencies (falls, crises, etc.) and call relatives (neighbors, family, etc.); 2) detect, over short or longer predefined.
9.1.5 Nively - WITA SRL
Nively is a French SME specialized in e-health technologies, it develops position and activity monitoring of activities of daily living platforms based on video technology. Nively’s mission is to use technological tools to put people back at the center of their interests, with their emotions, identity and behavior. Nively is collaborating with Stars through the UCA Solitaria project, by providing their MentorAge system. This software allows the monitoring of elderly people in nursing homes in order to detect all the abnormal events in the lives of residents (falls, runaways, strolls, etc.). Nively’s technology is based on RGBD video sensors (Kinects type) and a software platform for event detection and data visualization. Nively is also in charge of Software distribution for the ANR Activis project. This project is based on an objective quantification of the atypical behaviors on which the diagnosis of autism is based, with medical (diagnostic assistance and evaluation of therapeutic programs) and computer scientific (by allowing a more objective description of atypical behaviors in autism) objectives. This quantification requires video analysis of the behavior of people with autism. In particular, we propose to explore the issues related to the analysis of ocular movement, gestures and posture to characterize the behavior of a child with autism. Thus, Nively will add autistic behavior analysis software to its product range.
9.2 Bilateral grants with industry
9.2.1 LiChIE Project
The LiChIE project (Lion Chaine Image Elargie) is conducted in collaboration with AirBus and BPI to found nine topics including six on the theme of In-flight imagery and three on the robotics theme for the assembly of satellites. The two topics involving STARS are:
- Mohammed Guermal's PhD thesis on Visual Understanding of Activities for an improved collaboration between humans and robots. He began on December 1, 2020.
- Farhood Negin post-doctoral studies on detection and tracking of vehicles from satellite videos and abnormal activity detection. He started in Oct 2020 for 2 years.
10 Partnerships and cooperations
10.1 International initiatives
10.1.1 Inria associate team not involved in an IIL or an international program
GDD
-
Title:
Generalizable Deepfake Detection
-
Duration:
2022 ->
-
Coordinator:
Abhijit Das (abhijit.das@thapar.edu)
-
Partners:
- Thapar University, Bhadson Rd, Adharse colony, Prem nagar, Punjab- 147004 (Inde)
-
Inria contact:
Antitza Dantcheva
-
Summary:
In this project we will focus on Manipulated facial videos (deepfakes) which have become highly realistic due to the tremendous progress of deep convolutional neural networks (CNNs). While intriguing, such progress raises a number of social concerns related to fake news. We propose in GDD the design of deepfake detection algorithms, which can generalize in order to detect unknown manipulations.
10.2 European initiatives
10.2.1 Horizon Europe
GAIN:
GAIN project on cordis.europa.eu
-
Title:
Georgian Artificial Intelligence Networking and Twinning Initiative
-
Duration:
From October 1, 2022 to September 30, 2025
-
Partners:
- INSTITUT NATIONAL DE RECHERCHE EN INFORMATIQUE ET AUTOMATIQUE (INRIA), France
- EXOLAUNCH GMBH (EXO), Germany
- DEUTSCHES FORSCHUNGSZENTRUM FUR KUNSTLICHE INTELLIGENZ GMBH (DFKI), Germany
- GEORGIAN TECHNICAL UNIVERSITY (GTU), Georgia
-
Inria contact:
François Bremond
- Coordinator:
-
Summary:
GAIN will take a strategic step towards integrating Georgia, one of the Widening countries, into the system of European efforts aimed at ensuring the Europe’s leadership in one of the most transformative technologies of today and tomorrow – Artificial Intelligence (AI). It will be achieved by research profile adjusting and linking the central Georgian ICT research institute - Muskhelishvili Institute of Computational Mathematics (MICM), to the European AI research and innovation community. Two absolutely leading European research organizations (DFKI and INRIA) supported by the high-tech company EXOLAUNCH will support MICM in this endeavor. The Strategic Research and Innovation Programme (SRIP) designed by the partnership will provide the environment for the Georgian colleagues to get involved in the research projects of the European partners addressing a clearly delineated set of AI topics. Jointly, the partners will advance in capacity building and networking within the area of AI Methods and Tools for Human Activities Recognition and Evaluation, which also will contribute to strengthening core competences in such fundamental technologies as e.g. Machine (Deep) Learning. The results of the cooperation presented through the series of scientific publications and events will inform the European AI community about the potential of MICM and trigger new partnerships building, addressing e.g. Horizon Europe. The project will contribute to career development of a cohort of young researchers at MICM through joint supervision and targeted capacity building measures. Innovation and Research Administration and Management capacities of MICM will also be strengthen to allow the Institute to be better connected to the local, regional and European innovation activities. Using their extensive research and innovation networking capacities DFKI and INRIA will introduce MICM to the European AI research community by connecting to such networks as CLAIRE, ELLIS, ADRA, AI NoEs, etc.
10.2.2 H2020 projects
HEROES:
HEROES project on cordis.europa.eu
-
Title:
Novel Strategies to Fight Child Sexual Exploitation and Human Trafficking Crimes and Protect their Victims
-
Duration:
From December 1, 2021 to November 30, 2024
-
Partners:
- INSTITUT NATIONAL DE RECHERCHE EN INFORMATIQUE ET AUTOMATIQUE (INRIA), France
- POLICIA FEDERAL, Brazil
- ELLINIKO SYMVOULIO GAI TOUS PROSFYGES (GREEK COUNCIL FOR REFUGEES), Greece
- INTERNATIONAL CENTRE FOR MIGRATION POLICY DEVELOPMENT (ICMPD), Austria
- UNIVERSIDADE ESTADUAL DE CAMPINAS (UNICAMP), Brazil
- ASSOCIACAO BRASILEIRA DE DEFESA DA MUHLER DA INFANCIA E DA JUVENTUDE (ASBRAD), Brazil
- KOVOS SU PREKYBA ZMONEMIS IR ISNAUDOJIMU CENTRAS VSI (KOPZI), Lithuania
- FUNDACION RENACER, Colombia
- TRILATERAL RESEARCH LIMITED (TRI IE), Ireland
- VRIJE UNIVERSITEIT BRUSSEL (VUB), Belgium
- ATHINA-EREVNITIKO KENTRO KAINOTOMIAS STIS TECHNOLOGIES TIS PLIROFORIAS, TON EPIKOINONION KAI TIS GNOSIS (ATHENA - RESEARCH AND INNOVATION CENTER), Greece
- THE GLOBAL INITIATIVE VEREIN GEGEN TRANSATIONALE ORGANISIERTE KRIMINALITAT, Austria
- ESPHERA - CULTURAL,AMBIENTAL E SOCIAL, Brazil
- FUNDACAO UNIVERSIDADE DE BRASILIA (UNIVERSIDADE DE BRASÍLIA), Brazil
- IDENER RESEARCH DEVELOPMENT AGRUPACION DE INTERES ECONOMICO (IDENER RESEARCH DEVELOPMENT AIE), Spain
- UNIVERSIDAD COMPLUTENSE DE MADRID (UCM), Spain
- UNIVERSITY OF KENT (UNIKENT), United Kingdom
- KENTRO MELETON ASFALEIAS (CENTER FORSECURITY STUDIES CENTRE D'ETUDES DE SECURITE), Greece
- TRILATERAL RESEARCH LTD, United Kingdom
- POLICIA RODOVIARIA FEDERAL (FEDERA HIGHWAY POLICE), Brazil
- MINISTERIO DEL INTERIOR (ESMIR), Spain
- IEKSLIETU MINISTRIJAS VALSTS POLICIJA STATE POLICE OF THE MINISTRY OF INTERIOR (STATE POLICE OF LATVIA), Latvia
- SECRETARIA DE INTELIGENCIA ESTRATEGICA DE ESTADO - PRESIDENCIA DE LA REPUBLICA ORIENTAL DEL URUGUAY (SIEE), Uruguay
- ASSOCIACAO PORTUGUESA DE APOIO A VITIMA, Portugal
- COMANDO CONJUNTO DE LAS FUERZAS ARMADAS DEL PERU (COMANDO CONJUNTO DE LAS FUERZAS ARMADAS DEL PERU), Peru
- INTERNATIONAL CENTER FOR MISSING AND EXPLOITED CHILDREN SWITZERLAND, Switzerland
- HELLENIC POLICE (HELLENIC POLICE), Greece
- CENTRE FOR WOMEN AND CHILDREN STUDIES (CWCS), Bangladesh
- GLAVNA DIREKTSIA BORBA S ORGANIZIRANATA PRESTUPNOST (CHIEF DIRECTORATE FIGHT WITH ORGANISED CRIME), Bulgaria
-
Inria contact:
François Bremond
- Coordinator:
-
Summary:
Trafficking of human beings (THB) and child sexual abuse and exploitation (CSA/CSE) are two big problems in our society. Inadvertently, new information and communication technologies (ICTs) have provided a space for these problems to develop and take new forms, made worse by the lockdown caused by the COVID-19 pandemic. At the same time, technical and legal tools available to stakeholders that prevent, investigate, and assist victims – such as law enforcement agencies (LEAs), prosecutors, judges, and civil society organizations (CSOs) – fail to keep up with the pace at which criminals use new technologies to continue their abhorrent acts. Furthermore, assistance to victims of THB and CSA/CSE is often limited by the lack of coordination among these stakeholders. In this sense, there is a clear and vital need for joint work methodologies and the development of new strategies for approaching and assisting victims. In addition, due to the cross-border nature of these crimes, harmonization of legal frameworks from each of the affected countries is necessary for creating bridges of communication and coordination among all those stakeholders to help victims and reduce the occurrence of these horrendous crimes. To address these challenges, the HEROES project comes up with an ambitious, interdisciplinary, international, and victim-centered approach. The HEROES project is structured as a comprehensive solution that encompasses three main components: Prevention, Investigation and Victim Assistance. Through these components, our solution aims to establish a coordinated contribution with LEAs by developing an appropriate, victim-centered approach that is capable of addressing specific needs and providing protection. The HEROES project’s main objective is to use technology to improve the way in which help and support can be provided to victims of THB and CSA/CSE.
10.3 National initiatives
3IA
-
Title:
Video Analytics for Human Behavior Understanding (axis 2),
-
Duration:
From 2019
-
Chair holder:
François Brémond
-
Summary:
The goal of this chair is to design novel modern AI methods (including Computer Vision and Deep Learning algorithms) to build real-time systems for improving health and well-being as well as people safety, security and privacy. Behavior disorders affect the mental health of a growing number of people and are hard to handle, leading to a high cost in our modern society. New AI techniques can enable a more objective and earlier diagnosis, by quantifying the level of disorders and by monitoring the evolution of the disorders. AI techniques can also learn the relationships between the symptoms and their true causes, which are often hard to identify and measure.
RESPECT
-
Title:
Reliable, secure and privacy preserving multi-biometric person authentication
-
Duration:
From 2018 to 2023
-
Partners:
Inria, Hochschule Darmstadt, EURECOM.
-
Inria contact:
Antitza Dantcheva
-
Coordinator:
Hochschule Darmstadt
- Coordinator:
-
Summary:
In spite of the numerous advantages of biometric recognition systems over traditional authentication systems based on PINs or passwords, these systems are vulnerable to external attacks and can leak data. Presentations attacks (PAs) – impostors who manipulate biometric samples to masquerade as other people – pose serious threats to security. Privacy concerns involve the use of personal and sensitive biometric information, as classified by the GDPR, for purposes other than those intended. Multi-biometric systems, explored extensively as a means of improving recognition reliability, also offer potential to improve PA detection (PAD) generalization. Multi-biometric systems offer natural protection against spoofing since an impostor is less likely to succeed in fooling multiple systems simultaneously. For the same reason, previously unseen PAs are less likely to fool multi-biometric systems protected by PAD. RESPECT, a Franco-German collaborative project, explores the potential of using multi-biometrics as a means to defend against diverse PAs and improve generalization while still preserving privacy. Central to this idea is the use of (i) biometric characteristics that can be captured easily and reliably using ubiquitous smart devices and, (ii) biometric characteristics which facilitate computationally manageable privacy preserving, homomorphic encryption. The research focuses on characteristics readily captured with consumer-grade microphones and video cameras, specifically face, iris and voice. Further advances beyond the current state of the art involve the consideration of dynamic characteristics, namely utterance verification and lip dynamics. The core research objective is to determine which combination of biometrics characteristics gives the best biometric authentication reliability and PAD generalization while remaining compatible with computationally efficient privacy preserving biometric template protection schemes.
ACTIVIS
-
Title:
ACTIVIS: Video-based analysis of autism behavior
-
Duration:
From 2020 - 2023
-
Partners:
Inria, Aix-Marseille Université - LIS, Hôpitaux Pédiatriques Nice CHU-Lenval - CoBTeK, Nively
-
Inria contact:
François Brémond
-
Coordinator:
Aix-Marseille Université - LIS
-
Summary:
The ACTIVIS project is an ANR project (CES19: Technologies pour la santé) started in January 2020 and will end in December 2023 (48 months). This project is based on an objective quantification of the atypical behaviors on which the diagnosis of autism is based, with medical (diagnostic assistance and evaluation of therapeutic programs) and computer scientific (by allowing a more objective description of atypical behaviors in autism) objectives. This quantification requires video analysis of the behavior of people with autism. In particular, we propose to explore the issues related to the analysis of ocular movement, gestures and posture to characterize the behavior of a child with autism.
10.4 Regional initiatives
FairVision
-
Title:
FairVision - video monitoring for soccer games
-
Duration:
From September 2021 - January 2022
-
Coordinator:
Inria
-
Partners:
Inria (Stars): technical partner and project coordinator; FairVision.
-
Inria contact:
François Brémond (STARS)
-
Summary:
In this project, we were collaborating with the start-up company Fair Vision, which focuses on monitoring amateur soccer matches. The topic involved mastering of player and ball tracking in soccer videos. We were given input videos from the company, which included stadium recordings of soccer matches, as well as corresponding annotation files with detections of the players and the ball per each frame. We approached ball tracking as a single object tracking (SOT) problem. For this, we tested visual trackers and prepared an adapted version of the CSRT tracker (Discriminative Correlation Filter Tracker with Channel and Spatial Reliability) to enhance tracking of the ball, especially when ball detections were missing. Player tracking was approached as multi object tracking (MOT) problem. We tried several state-of-the-art MOT algorithms, e.g., FairMOT, TransTrack, ByteTrack, through applying them on the given input videos. After studying and analyzing the algorithm limitations, we started developing new version of the ByteTrack algorithm,aiming for the reduction of identity switches and enhancement of long term tracking. This work is continued in 2022.
E-Santé
-
Title:
E-Santé Silver Economy - Alcotra
-
Duration:
February 2020 - June 2022
-
Coordinator:
Nice Metropole
-
Partners:
Nice Metropole; Inria (Stars); CoBTek; Nice hospital; University of Genova; University of Torino; Liguria Region; Liguria Digitale; Provence Alpes Agglomération; University of Côte d'Azur.
-
Inria contact:
François Brémond (STARS)
-
Summary:
E-Santé Silver Economy is an Alcotra project, which performs a multi-sensor activity analysis for the monitoring and safety of older and isolated people. The E-Health (E-Santé in French and E-Sanità in Italian) / Silver Economy project is a collaborative project within the framework of the European cross-border cooperation program between France and Italy Interreg ALCOTRA. The aim is to increase innovation projects (in particular clusters, poles and companies) - and to develop innovative services at cross-border level. The E-Health / Silver Economy project tackles the problems of frailty among elderly, more particularly in rural and isolated areas; as well as access to innovations for the ALCOTRA regions, where there is an imbalance in terms of innovation and access to public services between urban and rural areas. The majority of the population, services and economic activities are concentrated in cities. The aims of the project are therefore: to experiment innovative e-health tools and to increase the accessibility of isolated people to care (screening, diagnosis and follow-up); in order to keep elderly people at their own houses as long as possible, by proposing solutions to delay the decrease in their mental, cognitive and physical capacities.
11 Dissemination
11.1 Promoting scientific activities
11.1.1 Scientific events: organisation
General chair, scientific chair
- François Brémond was general chair at IPAS 2022 (https://ipas.ieee.tn/) [100 people], IEEE International Conference on Image Processing, Applications and Systems, Genova, Italy, December 5-7, 2022.
Member of the organizing committees
- François Brémond organized IPAS 2022 (https://ipas.ieee.tn/), the IEEE International Conference on Image Processing, Applications and Systems, Genova, Italy, December 5-7, 2022.
- François Brémond and Antitza Dantcheva organized the workshop on “Artificial Intelligence for Automated Human Health-care and Monitoring” in conjunction with the 17th IEEE Conference on Automatic Face and Gesture Recognition, FG 2023.
11.1.2 Scientific events: selection
Chair of conference program committees
- François Brémond was program Chair of ICIAP’21 [400 people] in Lecce organized by the Italian Association for Research in Computer Vision, Pattern Recognition and Machine Learning.
- François Brémond was area chair of AVSS 2022 [200 people].
Reviewer
- François Brémond was reviewer in the major Computer Vision / Machine Learning conferences including ICCV, ECCV, WACV, CVPR, NeurIPS.
- Monique Thonnat was member of conference program committees IJCAI-ECAI 2022 and ICPRAM 2022.
- Di Yang served as reviewer for AAAI 2023.
- David Anghelone served as reviewer for IJCB 2022, the IEEE International Joint Conference on Biometrics, ICME 2022, the IEEE International Conference on Multimedia and Expo, FG 2023, the IEEE International Conference on Automatic Face and Gesture Recognition, as well as for the journal Image Processing.
- Michal Balazia served as reviewer for MDPI Sensors journal, MDPI Applied Sciences journal, MDPI Symmetry journal, MDPI Electronics journal, IEEE International Conference on Face and Gesture (FG), IEEE International Conference on Pattern Recognition (ICPR) and Pattern Recognition journal.
11.1.3 Journal
Member of the editorial boards
- Antitza Dantcheva serves in the editorial board of the journal Multimedia Tools and Applications (MTAP).
11.1.4 Invited talks
-
François Brémond gave lectures at thematic schools of Computer Vision, at Universities and organized specific sessions at Computer Vision Conferences:
- invited talk (1h) at Idiap, Switzerland on Activity Detection for medical applications, on the 16th-17th of August, 2022.
- lecturer (1h)- Zebra seminar: Deep Learning for Activity Detection. Online on the 6th of July, 2022.
- lecturer (1h30)- PhD seminar: Deep Learning for Activity Detection in HoChiMinh City at HCMOU (HoChiMinh Open University) on the 11th of October, 2022.
- invited talk (1h) at the 5th International Conference on Multimedia Analysis and Pattern Recognition MAPR 2022 (https://mapr.uit.edu.vn/) at Phu Quoc Island, Vietnam, October 13-14, 2022.
- invited talk (1h) at IPAS 2022 (https://ipas.ieee.tn/) IEEE International Conference on Image Processing, Applications and Systems, Genova, Italy, on December 5, 2022.
- Antitza Dantcheva gave an invited talk on “Generation and Detection of Deepfakes” at the Karlsruhe Institute of Technology, Germany in July 2022.
- Antitza Dantcheva gave an invited talk on “Generation and Detection of Deepfakes” at the GDR ISIS Workshop Deepfake Video Generation and Detection in Paris, in June 2022.
- Di Yang gave a presentation at University of Lyon 2 - Imagine Team: “Real-world skeleton-based human action recognition”.
- Di Yang gave a presentation for the industrial partner at TRACE (Toyota Workshop in Brussels): “ViA: View-invariant Skeleton Action Representation Learning via Motion Retargeting”.
11.1.5 Leadership within the scientific community
Monique Thonnat was expert for the international evaluation Ecos Nord Mexico 2021 and 2022.
11.1.6 Scientific expertise
- François Brémond was awarded as an outstanding Reviewer at CVPR22.
11.1.7 Patents
- Anghelone, D., Lannes, S. and Dantcheva, A. "System and Method of Unveiling High-Resolution visible face images from Low-Resolution face images". 2022. Patented EP22306692.9.
- Anghelone, D. Lannes, S., Strizhkova, V. , Faure, P. , Chen, C. and Dantcheva, A. "Thermal Face and Landmark detection method". 2022. Patented PCT/EP2022/085480.
- Anghelone, D. , Chen, C., Faure, P., Ross, A. and Dantcheva, A. "Cross-spectral face recognition training and cross-spectral face recognition method. 2022. Patented PCT/EP2022/085482.
11.2 Teaching - Supervision - Juries
11.2.1 Teaching
- François Brémond organized and lectured AI courses on Computer Vision & Deep Learning for the Data Science and AI - MSc program at Université Côte d'Azur: http://www-sop.inria.fr/members/Francois.Bremond/MSclass/deepLearningWinterSchool21Fall/UCA_master/, 30h class at Université Côte d'Azur in 2020, 2021 and 2022. Web-site
- Antitza Dantcheva taught 2 classes at Polytech Nice Sophia - Univ Côte d'Azur (Applied Artificial Intelligence, Master 2).
- Rui Dai taught two lectures for MSc. Data Science and Artificial Intelligence, UCA.
- Farhood Negin taught one lecture for MSc. Data Science and Artificial Intelligence, UCA.
- Hao Chen taught two lectures for MSc. Data Science and Artificial Intelligence, UCA.
- Valeriya Strizhkova taught one lecture for MSc. Data Science and Artificial Intelligence, UCA and one research project for DSAI.
- David Anghelone taught one lecture for the course Applied Artificial Intelligence, Master 2, Polytech Nice-Sophia, September 2022.
- David Anghelone one lecture for MSc. Data Science and Artificial Intelligence, UCA.
11.2.2 Supervision
- François Brémond (co)-supervised 5 PhD students and 6 Masters students in 2022.
- Antitza Dantcheva (co)-supervised 2 PhD students and 2 Masters students in 2022.
11.2.3 Juries
-
Monique Thonnat was
- in the HDR committee as Reviewer: Suzanne Thümmler HDR Université Côte d’Azur, Faculté de Médecine on 12 décembre 2022.
- PhD committee Chair: PhD of Thinhinane Yebda, University of Bordeaux, on 21 rst January 2022
- PhD committee member for PhD of Damien Bouchabou, IMT Atlantique 25 May 2022
- PhD committee member for PhD of Yasser Boutaleb, CentraleSupElec, Rennes, 6th December 2022
-
François Brémond was - in the HDR committee of Damien Vivet, from the Université Fédérale Toulouse Midi-Pyrénées, ISAE-SUPAERO, 2 June 2022.
- in the Ph.D. committee of George ADAIMI, from EPFL - Ecole Polytechnique Fédérale de Lausanne, 16 August 2022.
- in the Ph.D. committee of Jhony Heriberto Giraldo-Zuluaga, from University of La Rochelle, 31 August 2022.
- in the Ph.D. committee of Rémi DUFOUR, from Université Gustave Eiffel, 22 November 2022.
- in the mid-term committee of Georgios Kopanas, from Inria Sophia, 30 May, 2022.
- in the mid-term committee of Francesco Galati, from Eurecom, December 14, 2022.
-
Antitza Dantcheva was
- in the Ph.D. committee (examiner) of Xiangnan Yin (Laboratory LIRIS, Lyon), June 2022.
- in the Ph.D. committee (examiner) of Anis Trabelsi (Eurecom, France), November 2022.
- in the Ph.D. committee (examiner) of Deivid Botina (Laboratoire ImVia, University Bourgogne), November 2022.
- in the Ph.D. committee (reviewer) of Robin Kips (Télécom Paris), April 2022.
- in the CS of Santiago Smith Silva Rincon (Team EPIONE), October 2022.
- in the CS of Mehdi Atamna (Laboratory LIRIS, Lyon), April 2022.
- David Anghelone was a jury member for the Master defense of Xuan-Huy NGUYEN.
11.2.4 Recruitment committees
Monique Thonnat was
- Co-chair of Inria Sophia Antipolis recruitment committee CRCN/ISFP 2022
- member of Inria Sophia Antipolis admission recruitment committee ISFP 2022
12 Scientific production
12.1 Major publications
- 1 inproceedingsLearning to match appearances by correlations in a covariance metric space.European Conference on Computer VisionSpringer2012, 806--820
- 2 articleExploiting Feature Correlations by Brownian Statistics for People Detection and Recognition.IEEE transactions on systems, man, and cybernetics2016
- 3 inproceedingsVideo Covariance Matrix Logarithm for Human Action Recognition in Videos.IJCAI 2015 - 24th International Joint Conference on Artificial Intelligence (IJCAI)Buenos Aires, ArgentinaJuly 2015
- 4 articleSemantic Event Fusion of Different Visual Modality Concepts for Activity Recognition.IEEE Transactions on Pattern Analysis and Machine Intelligence382016, 1598 - 1611
- 5 articleGender estimation based on smile-dynamics.IEEE Transactions on Information Forensics and Security2016, 11
- 6 inproceedingsToyota Smarthome: Real-World Activities of Daily Living.ICCV 2019 -17th International Conference on Computer VisionSeoul, South KoreaOctober 2019
- 7 inproceedingsVPN: Learning Video-Pose Embedding for Activities of Daily Living.ECCV 2020 - 16th European Conference on Computer VisionGlasgow (Virtual), United KingdomAugust 2020
- 8 inproceedingsGesture Recognition by Learning Local Motion Signatures.CVPR 2010 : IEEE Conference on Computer Vision and Pattern RecognitionSan Franscico, CA, United StatesIEEE Computer Society PressJune 2010
- 9 articleRecognizing Gestures by Learning Local Motion Signatures of HOG Descriptors.IEEE Transactions on Pattern Analysis and Machine Intelligence2012
- 10 inproceedingsKnowledge Representation for Program Reuse.European Conference on Artificial Intelligence (ECAI)Lyon, FranceJuly 2002, 240-244
- 11 articleBlocks, a Component Framework with Checking Facilities for Knowledge-Based Systems.Informatica, Special Issue on Component Based Software Development254November 2001, 501-507
- 12 articleCompilation Modulaire d'un Langage Synchrone.Revue des sciences et technologies de l'information, série Théorie et Science Informatique430June 2011, 441-471URL: http://hal.inria.fr/inria-00524499/en
- 13 article What Can Program Supervision Do for Software Re-use? IEE Proceedings - Software Special Issue on Knowledge Modelling for Software Components Reuse 147 5 2000
- 14 inproceedingsAutomatic Video Interpretation: A Novel Algorithm based for Temporal Scenario Recognition.The Eighteenth International Joint Conference on Artificial Intelligence (IJCAI'03)Acapulco, Mexico9-15 September 2003
- 15 inproceedingsG3AN: Disentangling Appearance and Motion for Video Generation.CVPR 2020 - IEEE Conference on Computer Vision and Pattern RecognitionSeattle / Virtual, United StatesJune 2020
12.2 Publications of the year
International journals
International peer-reviewed conferences
Conferences without proceedings
Scientific book chapters
Doctoral dissertations and habilitation theses
Reports & preprints
12.3 Cited publications
- 50 inproceedingsVivit: A video vision transformer.Proceedings of the IEEE/CVF International Conference on Computer Vision2021, 6836--6846
- 51 inproceedingsQuo Vadis, Action Recognition? A New Model and the Kinetics Dataset.07 2017, 4724-4733
- 52 inproceedingsOne-class autoencoder approach for optimal electrode set identification in wearable EEG event monitoring.2021 43rd Annual International Conference of the IEEE Engineering in Medicine & Biology Society (EMBC)IEEE2021, 7128--7131
- 53 inproceedingsFuture Transformer for Long-term Action Anticipation.Proceedings of the IEEE/CVF Conference on Computer Vision and Pattern Recognition2022, 3052--3061
- 54 articleA Modular Multimodal Architecture for Gaze Target Prediction: Application to Privacy-Sensitive Settings.IEEE/CVF Conference on Computer Vision and Pattern Recognition Workshops (CVPRW)2022
- 55 articleThe kinetics human action video dataset.arXiv preprint arXiv:1705.069502017
- 56 inproceedingsJersey number recognition with semi-supervised spatial transformer network.Proceedings of the IEEE conference on computer vision and pattern recognition workshops2018, 1783--1790
- 57 inproceedingsVideo swin transformer.Proceedings of the IEEE/CVF Conference on Computer Vision and Pattern Recognition2022, 3202--3211
- 58 articleMediapipe: A framework for building perception pipelines.arXiv preprint arXiv:1906.081722019
- 59 articleAttention is all you need.Advances in neural information processing systems302017
- 60 articleLong short-term transformer for online action detection.Advances in Neural Information Processing Systems342021, 1086--1099
- 61 inproceedings Self-supervised Video Representation Learning via Latent Time Navigation.In Proceedings of the Thirty-Seventh AAAI Conference on Artificial Intelligence (AAAI)2023
- 62 articleUnik: A unified framework for real-world skeleton-based action recognition.arXiv preprint arXiv:2107.085802021
- 63 inproceedingsReal-Time Online Video Detection with Temporal Smoothing Transformers.European Conference on Computer VisionSpringer2022, 485--502