2023Activity reportProject-TeamSTARS
RNSR: 201221015V- Research center Inria Centre at Université Côte d'Azur
- Team name: Spatio-Temporal Activity Recognition Systems
- Domain:Perception, Cognition and Interaction
- Theme:Vision, perception and multimedia interpretation
Keywords
Computer Science and Digital Science
- A5.3. Image processing and analysis
- A5.3.3. Pattern recognition
- A5.4. Computer vision
- A5.4.2. Activity recognition
- A5.4.4. 3D and spatio-temporal reconstruction
- A5.4.5. Object tracking and motion analysis
- A9. Artificial intelligence
- A9.1. Knowledge
- A9.2. Machine learning
- A9.3. Signal analysis
- A9.8. Reasoning
Other Research Topics and Application Domains
- B1. Life sciences
- B1.2. Neuroscience and cognitive science
- B1.2.2. Cognitive science
- B2. Health
- B2.1. Well being
- B7. Transport and logistics
- B7.1.1. Pedestrian traffic and crowds
- B8. Smart Cities and Territories
- B8.4. Security and personal assistance
1 Team members, visitors, external collaborators
Research Scientists
- François Brémond [Team leader, INRIA, Senior Researcher, HDR]
- Michal Balazia [INRIA, ISFP, from Oct 2023]
- Michal Balazia [INRIA, Starting Research Position, from Jul 2023 until Sep 2023]
- Antitza Dantcheva [INRIA, Researcher, HDR]
- Laura Ferrari [INRIA, Starting Research Position, until Apr 2023]
- Alexandra Konig [INRIA, Starting Research Position, until Feb 2023]
- Monique Thonnat [INRIA, Senior Researcher, HDR]
Post-Doctoral Fellows
- Baptiste Chopin [INRIA, Post-Doctoral Fellow, from Jul 2023]
- Olivier Huynh [INRIA, Post-Doctoral Fellow]
PhD Students
- Abid Ali [UNIV COTE D'AZUR]
- David Anghelone [THALES, until Jun 2023]
- Mohammed Guermal [INRIA]
- Snehashis Majhi [INRIA]
- Tomasz Stanczyk [INRIA, from Feb 2023]
- Valeriya Strizhkova [INRIA]
- Di Yang [INRIA]
Technical Staff
- Tanay Agrawal [INRIA, Engineer]
- Mahmoud Ali [INRIA, Engineer, from May 2023]
- Ezem Sura Ekmekci [INRIA, Engineer]
- Abdoul Djalil Ousseini Hamza [INRIA, Engineer, until May 2023]
- Abhay Samudrasok [INRIA, from Feb 2023 until Mar 2023]
- Yoann Torrado [INRIA, Engineer]
Interns and Apprentices
- Nibras Abo Alzahab [UNIV COTE D'AZUR, Intern, from Feb 2023 until Aug 2023]
- Pranav Balaji [INRIA, Intern, from Feb 2023 until May 2023]
- Agniv Chatterjee [INRIA, Intern, until Feb 2023]
- Tashvik Dhamija [INRIA, Intern, from Feb 2023 until Jun 2023]
- Eshan Jain [INRIA, Intern, from May 2023 until Jul 2023]
- Rui-Han Lee [INRIA, Intern, from Aug 2023]
- Cyprien Michel-Deletie [ENS DE LYON, Intern, from Oct 2023]
- Mansi Mittal [INRIA, Intern, until Mar 2023]
- Aglind Reka [UNIV COTE D'AZUR, Intern, from Apr 2023 until Aug 2023]
- Po-Han Wu [INRIA, Intern, until Jan 2023]
- Nishant Yella [INRIA, Intern, from Mar 2023 until Sep 2023]
Administrative Assistant
- Sandrine Boute [INRIA]
Visiting Scientists
- Abhijit Das [BITS PILANI HYDERABAD CAMPUS, from Nov 2023]
- Anshul Gupta [EPFL LAUSANNE, from Feb 2023 until Feb 2023]
External Collaborators
- Hao Chen [School of Computer Science, Peking University, China]
- Rui Dai [Amazon, USA]
- Srijan Das [University of North Carolina at Charlotte - USA]
- Laura Ferrari [Biorobotic institute, Sant'Anna School of Advanced Studies, Italy, from May 2023]
- Rachid Guerchouche [CHU Nice]
- Alexandra Konig [KI Elements, from Mar 2023]
- Susanne Thummler [CHU NICE]
- Yaohui Wang [AI Lab, Shanghai, China]
- Radia Zeghari [UNIV COTE D'AZUR, until Aug 2023]
2 Overall objectives
2.1 Presentation
The STARS (Spatio-Temporal Activity Recognition Systems) team focuses on the design of cognitive vision systems for Activity Recognition. More precisely, we are interested in the real-time semantic interpretation of dynamic scenes observed by video cameras and other sensors. We study long-term spatio-temporal activities performed by agents such as human beings, animals or vehicles in the physical world. The major issue in semantic interpretation of dynamic scenes is to bridge the gap between the subjective interpretation of data and the objective measures provided by sensors. To address this problem Stars develops new techniques in the field of computer vision, machine learning and cognitive systems for physical object detection, activity understanding, activity learning, vision system design and evaluation. We focus on two principal application domains: visual surveillance and healthcare monitoring.
2.2 Research Themes
Stars is focused on the design of cognitive systems for Activity Recognition. We aim at endowing cognitive systems with perceptual capabilities to reason about an observed environment, to provide a variety of services to people living in this environment while preserving their privacy. In today's world, a huge amount of new sensors and new hardware devices are currently available, addressing potentially new needs of the modern society. However, the lack of automated processes (with no human interaction) able to extract a meaningful and accurate information (i.e. a correct understanding of the situation) has often generated frustrations among the society and especially among older people. Therefore, Stars objective is to propose novel autonomous systems for the real-time semantic interpretation of dynamic scenes observed by sensors. We study long-term spatio-temporal activities performed by several interacting agents such as human beings, animals and vehicles in the physical world. Such systems also raise fundamental software engineering problems to specify them as well as to adapt them at run time.
We propose new techniques at the frontier between computer vision, knowledge engineering, machine learning and software engineering. The major challenge in semantic interpretation of dynamic scenes is to bridge the gap between the task dependent interpretation of data and the flood of measures provided by sensors. The problems we address range from physical object detection, activity understanding, activity learning to vision system design and evaluation. The two principal classes of human activities we focus on, are assistance to older adults and video analytics.
Typical examples of complex activity are shown in Figure 1 and Figure 2 for a homecare application (See Toyota Smarthome Dataset here). In this example, the duration of the monitoring of an older person apartment could last several months. The activities involve interactions between the observed person and several pieces of equipment. The application goal is to recognize the everyday activities at home through formal activity models (as shown in Figure 3) and data captured by a network of sensors embedded in the apartment. Here typical services include an objective assessment of the frailty level of the observed person to be able to provide a more personalized care and to monitor the effectiveness of a prescribed therapy. The assessment of the frailty level is performed by an Activity Recognition System which transmits a textual report (containing only meta-data) to the general practitioner who follows the older person. Thanks to the recognized activities, the quality of life of the observed people can thus be improved and their personal information can be preserved.
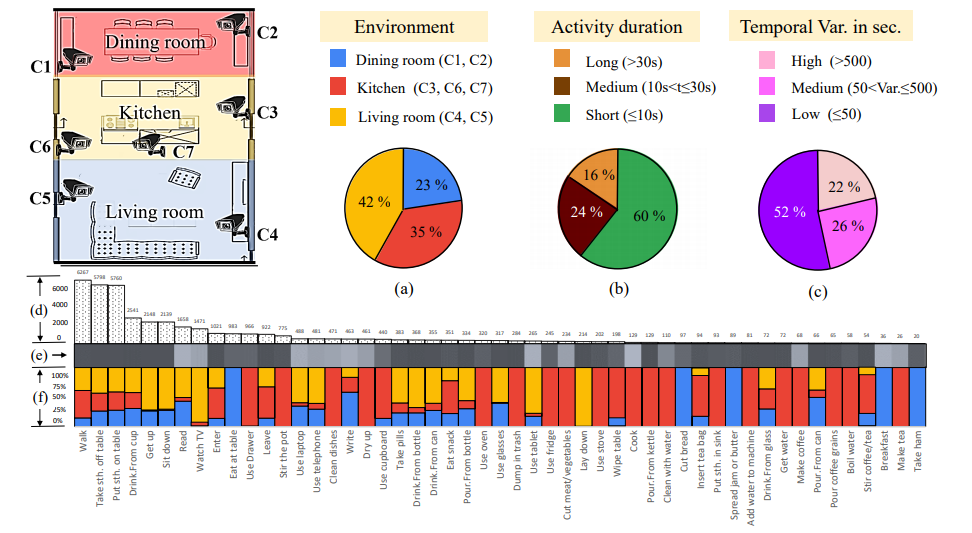
.png)
Activity (PrepareMeal, | |
PhysicalObjects( | (p : Person), (z : Zone), (eq : Equipment)) |
Components( | (s_inside : InsideKitchen(p, z)) |
(s_close : CloseToCountertop(p, eq)) | |
(s_stand : PersonStandingInKitchen(p, z))) | |
Constraints( | (z->Name = Kitchen) |
(eq->Name = Countertop) | |
(s_close->Duration >= 100) | |
(s_stand->Duration >= 100)) | |
Annotation( | AText("prepare meal"))) |
The ultimate goal is for cognitive systems to perceive and understand their environment to be able to provide appropriate services to a potential user. An important step is to propose a computational representation of people activities to adapt these services to them. Up to now, the most effective sensors have been video cameras due to the rich information they can provide on the observed environment. These sensors are currently perceived as intrusive ones. A key issue is to capture the pertinent raw data for adapting the services to the people while preserving their privacy. We plan to study different solutions including of course the local processing of the data without transmission of images and the utilization of new compact sensors developed for interaction (also called RGB-Depth sensors, an example being the Kinect) or networks of small non-visual sensors.
2.3 International and Industrial Cooperation
Our work has been applied in the context of more than 10 European projects such as COFRIEND, ADVISOR, SERKET, CARETAKER, VANAHEIM, SUPPORT, DEM@CARE, VICOMO, EIT Health.
We had or have industrial collaborations in several domains: transportation (CCI Airport Toulouse Blagnac, SNCF, Inrets, Alstom, Ratp, Toyota, GTT (Italy), Turin GTT (Italy)), banking (Crédit Agricole Bank Corporation, Eurotelis and Ciel), security (Thales R&T FR, Thales Security Syst, EADS, Sagem, Bertin, Alcatel, Keeneo), multimedia (Thales Communications), civil engineering (Centre Scientifique et Technique du Bâtiment (CSTB)), computer industry (BULL), software industry (AKKA), hardware industry (ST-Microelectronics) and health industry (Philips, Link Care Services, Vistek).
We have international cooperations with research centers such as Reading University (UK), ENSI Tunis (Tunisia), Idiap (Switzerland), Multitel (Belgium), National Cheng Kung University, National Taiwan University (Taiwan), MICA (Vietnam), IPAL, I2R (Singapore), University of Southern California, University of South Florida (USA), Michigan State University (USA), Chinese Academy of Sciences (China), IIIT Delhi (India), Hochschule Darmstadt (Germany), Fraunhofer Institute for Computer Graphics Research IGD (Germany).
3 Research program
3.1 Introduction
Stars follows three main research directions: perception for activity recognition, action recognition and semantic activity recognition. These three research directions are organized following the workflow of activity recognition systems: First, the perception and the action recognition directions provide new techniques to extract powerful features, whereas the semantic activity recognition research direction provides new paradigms to match these features with concrete video analytics and healthcare applications.
Transversely, we consider a new research axis in machine learning, combining a priori knowledge and learning techniques, to set up the various models of an activity recognition system. A major objective is to automate model building or model enrichment at the perception level and at the understanding level.
3.2 Perception for Activity Recognition
Participants: François Brémond, Antitza Dantcheva, Monique Thonnat.
Keywords: Activity Recognition, Scene Understanding, Machine Learning, Computer Vision, Cognitive Vision Systems, Software Engineering.
3.2.1 Introduction
Our main goal in perception is to develop vision algorithms able to address the large variety of conditions characterizing real world scenes in terms of sensor conditions, hardware requirements, lighting conditions, physical objects, and application objectives. We have also several issues related to perception which combine machine learning and perception techniques: learning people appearance, parameters for system control and shape statistics.
3.2.2 Appearance Models and People Tracking
An important issue is to detect in real-time physical objects from perceptual features and predefined 3D models. It requires finding a good balance between efficient methods and precise spatio-temporal models. Many improvements and analysis need to be performed in order to tackle the large range of people detection scenarios.
Appearance models. In particular, we study the temporal variation of the features characterizing the appearance of a human. This task could be achieved by clustering potential candidates depending on their position and their reliability. This task can provide any people tracking algorithms with reliable features allowing for instance to (1) better track people or their body parts during occlusion, or to (2) model people appearance for re-identification purposes in mono and multi-camera networks, which is still an open issue. The underlying challenge of the person re-identification problem arises from significant differences in illumination, pose and camera parameters. The re-identification approaches have two aspects: (1) establishing correspondences between body parts and (2) generating signatures that are invariant to different color responses. As we have already several descriptors which are color invariant, we now focus more on aligning two people detection and on finding their corresponding body parts. Having detected body parts, the approach can handle pose variations. Further, different body parts might have different influence on finding the correct match among a whole gallery dataset. Thus, the re-identification approaches have to search for matching strategies. As the results of the re-identification are always given as the ranking list, re-identification focuses on learning to rank. "Learning to rank" is a type of machine learning problem, in which the goal is to automatically construct a ranking model from a training data.
Therefore, we work on information fusion to handle perceptual features coming from various sensors (several cameras covering a large-scale area or heterogeneous sensors capturing more or less precise and rich information). New 3D RGB-D sensors are also investigated, to help in getting an accurate segmentation for specific scene conditions.
Long-term tracking. For activity recognition we need robust and coherent object tracking over long periods of time (often several hours in video surveillance and several days in healthcare). To guarantee the long-term coherence of tracked objects, spatio-temporal reasoning is required. Modeling and managing the uncertainty of these processes is also an open issue. In Stars we propose to add a reasoning layer to a classical Bayesian framework modeling the uncertainty of the tracked objects. This reasoning layer can take into account the a priori knowledge of the scene for outlier elimination and long-term coherency checking.
Controlling system parameters. Another research direction is to manage a library of video processing programs. We are building a perception library by selecting robust algorithms for feature extraction, by ensuring they work efficiently with real time constraints and by formalizing their conditions of use within a program supervision model. In the case of video cameras, at least two problems are still open: robust image segmentation and meaningful feature extraction. For these issues, we are developing new learning techniques.
3.3 Action Recognition
Participants: François Brémond, Antitza Dantcheva, Monique Thonnat.
Keywords: Machine Learning, Computer Vision, Cognitive Vision Systems.
3.3.1 Introduction
Due to the recent development of high processing units, such as GPU,it is now possible to extract meaningful features directly from videos (e.g. video volume) to recognize reliably short actions. Action Recognition benefits also greatly from the huge progress made recently in Machine Learning (e.g. Deep Learning), especially for the study of human behavior. For instance, Action Recognition enables to measure objectively the behavior of humans by extracting powerful features characterizing their everyday activities, their emotion, eating habits and lifestyle, by learning models from a large amount of data from a variety of sensors, to improve and optimize for example, the quality of life of people suffering from behavior disorders. However, Smart Homes and Partner Robots have been well advertized but remain laboratory prototypes, due to the poor capability of automated systems to perceive and reason about their environment. A hard problem is for an automated system to cope 24/7 with the variety and complexity of the real world. Another challenge is to extract people fine gestures and subtle facial expressions to better analyze behavior disorders, such as anxiety or apathy. Taking advantage of what is currently studied for self-driving cars or smart retails, there is a large avenue to design ambitious approaches for the healthcare domain. In particular, the advance made with Deep Learning algorithms has already enabled to recognize complex activities, such as cooking interactions with instruments, and from this analysis to differentiate healthy people from the ones suffering from dementia.
To address these issues, we propose to tackle several challenges which are detailed in the following subsections:
3.3.2 Action recognition in the wild
The current Deep Learning techniques are mostly developed to work on few clipped videos, which have been recorded with students performing a limited set of predefined actions in front of a camera with high resolution. However, real life scenarios include actions performed in a spontaneous manner by older people (including people interactions with their environment or with other people), from different viewpoints, with varying framerate, partially occluded by furniture at different locations within an apartment depicted through long untrimmed videos. Therefore, a new dedicated dataset should be collected in a real-world setting to become a public benchmark video dataset and to design novel algorithms for Activities of Daily Living (ADL) activity recognition. A special attention should be taken to anonymize the videos.
3.3.3 Attention mechanisms for action recognition
ADL and video-surveillance activities are different from internet activities (e.g. Sports, Movies, YouTube), as they may have very similar context (e.g. same background kitchen) with high intra-variation (different people performing the same action in different manners), but in the same time low inter-variation, similar ways to perform two different actions (e.g. eating and drinking a glass of water). Consequently, fine-grained actions are badly recognized. So, we will design novel attention mechanisms for action recognition, for the algorithm being able to focus on a discriminative part of the person conducting the action. For instance, we will study attention algorithms, which could focus on the most appropriate body parts (e.g. full body, right hand). In particular, we plan to design a soft mechanism, learning the attention weights directly on the feature map of a 3DconvNet, a powerful convolutional network, which takes as input a batch of videos.
3.3.4 Action detection for untrimmed videos
Many approaches have been proposed to solve the problem of action recognition in short clipped 2D videos, which achieved impressive results with hand-crafted and deep features. However, these approaches cannot address real life situations, where cameras provide online and continuous video streams in applications such as robotics, video surveillance, and smart-homes. Here comes the importance of action detection to help recognizing and localizing each action happening in long videos. Action detection can be defined as the ability to localize starting and ending of each human action happening in the video, in addition to recognizing each action label. There have been few action detection algorithms designed for untrimmed videos, which are based on either sliding window, temporal pooling or frame-based labeling. However, their performance is too low to address real-word datasets. A first task consists in benchmarking the already published approaches to study their limitations on novel untrimmed video datasets, recorded following real-world settings. A second task could be to propose a new mechanism to improve either 1) the temporal pooling directly from the 3DconvNet architecture using for instance Temporal Convolution Networks (TCNs) or 2) frame-based labeling with a clustering technique (e.g. using Fisher Vectors) to discover the sub-activities of interest.
3.3.5 View invariant action recognition
The performance of current approaches strongly relies on the used camera angle: enforcing that the camera angle used in testing is the same (or extremely close to) as the camera angle used in training, is necessary for the approach to perform well. On the contrary, the performance drops when a different camera view-point is used. Therefore, we aim at improving the performance of action recognition algorithms by relying on 3D human pose information. For the extraction of the 3D pose information, several open-source algorithms can be used, such as openpose or videopose3D (from CMU or Facebook research, click here). Also, other algorithms extracting 3d meshes can be used. To generate extra views, Generative Adversial Network (GAN) can be used together with the 3D human pose information to complete the training dataset from the missing view.
3.3.6 Uncertainty and action recognition
Another challenge is to combine the short-term actions recognized by powerful Deep Learning techniques with long-term activities defined by constraint-based descriptions and linked to user interest. To realize this objective, we have to compute the uncertainty (i.e. likelihood or confidence), with which the short-term actions are inferred. This research direction is linked to the next one, to Semantic Activity Recognition.
3.4 Semantic Activity Recognition
Participants: François Brémond, Monique Thonnat.
Keywords: Activity Recognition, Scene Understanding, Computer Vision.
3.4.1 Introduction
Semantic activity recognition is a complex process where information is abstracted through four levels: signal (e.g. pixel, sound), perceptual features, physical objects and activities. The signal and the feature levels are characterized by strong noise, ambiguous, corrupted and missing data. The whole process of scene understanding consists in analyzing this information to bring forth pertinent insight of the scene and its dynamics while handling the low-level noise. Moreover, to obtain a semantic abstraction, building activity models is a crucial point. A still open issue consists in determining whether these models should be given a priori or learned. Another challenge consists in organizing this knowledge in order to capitalize experience, share it with others and update it along with experimentation. To face this challenge, tools in knowledge engineering such as machine learning or ontology are needed.
Thus, we work along the following research axes: high level understanding (to recognize the activities of physical objects based on high level activity models), learning (how to learn the models needed for activity recognition) and activity recognition and discrete event systems.
3.4.2 High Level Understanding
A challenging research axis is to recognize subjective activities of physical objects (i.e. human beings, animals, vehicles) based on a priori models and objective perceptual measures (e.g. robust and coherent object tracks).
To reach this goal, we have defined original activity recognition algorithms and activity models. Activity recognition algorithms include the computation of spatio-temporal relationships between physical objects. All the possible relationships may correspond to activities of interest and all have to be explored in an efficient way. The variety of these activities, generally called video events, is huge and depends on their spatial and temporal granularity, on the number of physical objects involved in the events, and on the event complexity (number of components constituting the event).
Concerning the modeling of activities, we are working towards two directions: the uncertainty management for representing probability distributions and knowledge acquisition facilities based on ontological engineering techniques. For the first direction, we are investigating classical statistical techniques and logical approaches. For the second direction, we built a language for video event modeling and a visual concept ontology (including color, texture and spatial concepts) to be extended with temporal concepts (motion, trajectories, events ...) and other perceptual concepts (physiological sensor concepts ...).
3.4.3 Learning for Activity Recognition
Given the difficulty of building an activity recognition system with a priori knowledge for a new application, we study how machine learning techniques can automate building or completing models at the perception level and at the understanding level.
At the understanding level, we are learning primitive event detectors. This can be done for example by learning visual concept detectors using SVMs (Support Vector Machines) with perceptual feature samples. An open question is how far can we go in weakly supervised learning for each type of perceptual concept (i.e. leveraging the human annotation task). A second direction is to learn typical composite event models for frequent activities using trajectory clustering or data mining techniques. We name composite event a particular combination of several primitive events.
3.4.4 Activity Recognition and Discrete Event Systems
The previous research axes are unavoidable to cope with the semantic interpretations. However, they tend to let aside the pure event driven aspects of scenario recognition. These aspects have been studied for a long time at a theoretical level and led to methods and tools that may bring extra value to activity recognition, the most important being the possibility of formal analysis, verification and validation.
We have thus started to specify a formal model to define, analyze, simulate, and prove scenarios. This model deals with both absolute time (to be realistic and efficient in the analysis phase) and logical time (to benefit from well-known mathematical models providing re-usability, easy extension, and verification). Our purpose is to offer a generic tool to express and recognize activities associated with a concrete language to specify activities in the form of a set of scenarios with temporal constraints. The theoretical foundations and the tools being shared with Software Engineering aspects.
The results of the research performed in perception and semantic activity recognition (first and second research directions) produce new techniques for scene understanding and contribute to specify the needs for new software architectures (third research direction).
4 Application domains
4.1 Introduction
While in our research the focus is to develop techniques, models and platforms that are generic and reusable, we also make efforts in the development of real applications. The motivation is twofold. The first is to validate the new ideas and approaches we introduce. The second is to demonstrate how to build working systems for real applications of various domains based on the techniques and tools developed. Indeed, Stars focuses on two main domains: video analytic and healthcare monitoring.
Domain: Video Analytics
Our experience in video analytic (also referred to as visual surveillance) is a strong basis which ensures both a precise view of the research topics to develop and a network of industrial partners ranging from end-users, integrators and software editors to provide data, objectives, evaluation and funding.
For instance, the Keeneo start-up was created in July 2005 for the industrialization and exploitation of Orion and Pulsar results in video analytic (VSIP library, which was a previous version of SUP). Keeneo has been bought by Digital Barriers in August 2011 and is now independent from Inria. However, Stars continues to maintain a close cooperation with Keeneo for impact analysis of SUP and for exploitation of new results.
Moreover, new challenges are arising from the visual surveillance community. For instance, people detection and tracking in a crowded environment are still open issues despite the high competition on these topics. Also detecting abnormal activities may require to discover rare events from very large video data bases often characterized by noise or incomplete data.
Domain: Healthcare Monitoring
Since 2011, we have initiated a strategic partnership (called CobTek) with Nice hospital (CHU Nice, Prof P. Robert) to start ambitious research activities dedicated to healthcare monitoring and to assistive technologies. These new studies address the analysis of more complex spatio-temporal activities (e.g. complex interactions, long term activities).
4.1.1 Research
To achieve this objective, several topics need to be tackled. These topics can be summarized within two points: finer activity description and longitudinal experimentation. Finer activity description is needed for instance, to discriminate the activities (e.g. sitting, walking, eating) of Alzheimer patients from the ones of healthy older people. It is essential to be able to pre-diagnose dementia and to provide a better and more specialized care. Longer analysis is required when people monitoring aims at measuring the evolution of patient behavioral disorders. Setting up such long experimentation with dementia people has never been tried before but is necessary to have real-world validation. This is one of the challenges of the European FP7 project Dem@Care where several patient homes should be monitored over several months.
For this domain, a goal for Stars is to allow people with dementia to continue living in a self-sufficient manner in their own homes or residential centers, away from a hospital, as well as to allow clinicians and caregivers remotely provide effective care and management. For all this to become possible, comprehensive monitoring of the daily life of the person with dementia is deemed necessary, since caregivers and clinicians will need a comprehensive view of the person's daily activities, behavioral patterns, lifestyle, as well as changes in them, indicating the progression of their condition.
4.1.2 Ethical and Acceptability Issues
The development and ultimate use of novel assistive technologies by a vulnerable user group such as individuals with dementia, and the assessment methodologies planned by Stars are not free of ethical, or even legal concerns, even if many studies have shown how these Information and Communication Technologies (ICT) can be useful and well accepted by older people with or without impairments. Thus, one goal of Stars team is to design the right technologies that can provide the appropriate information to the medical carers while preserving people privacy. Moreover, Stars will pay particular attention to ethical, acceptability, legal and privacy concerns that may arise, addressing them in a professional way following the corresponding established EU and national laws and regulations, especially when outside France. Now, Stars can benefit from the support of the COERLE (Comité Opérationnel d'Evaluation des Risques Légaux et Ethiques) to help it to respect ethical policies in its applications.
As presented in 2, Stars aims at designing cognitive vision systems with perceptual capabilities to monitor efficiently people activities. As a matter of fact, vision sensors can be seen as intrusive ones, even if no images are acquired or transmitted (only meta-data describing activities need to be collected). Therefore, new communication paradigms and other sensors (e.g. accelerometers, RFID (Radio Frequency Identification), and new sensors to come in the future) are also envisaged to provide the most appropriate services to the observed people, while preserving their privacy. To better understand ethical issues, Stars members are already involved in several ethical organizations. For instance, F. Brémond has been a member of the ODEGAM - “Commission Ethique et Droit” (a local association in Nice area for ethical issues related to older people) from 2010 to 2011 and a member of the French scientific council for the national seminar on “La maladie d'Alzheimer et les nouvelles technologies - Enjeux éthiques et questions de société” in 2011. This council has in particular proposed a chart and guidelines for conducting researches with dementia patients.
For addressing the acceptability issues, focus groups and HMI (Human Machine Interaction) experts, are consulted on the most adequate range of mechanisms to interact and display information to older people.
5 Social and environmental responsibility
5.1 Footprint of research activities
We have limited our travels by reducing our physical participation to conferences and to international collaborations.
5.2 Impact of research results
We have been involved for many years in promoting public transportation by improving safety onboard and in station. Moreover, we have been working on pedestrian detection for self-driving cars, which will help also reducing the number of individual cars.
6 Highlights of the year
6.1 Awards
- Michal Balazia received a permanent research position (ISFP).
- David Anghelone received the "second prix de thèse de la mention Informatique de l'EDSTIC" of the Université Côte d'Azur.
- François Brémond was extended as 3IA chair.
7 New results
7.1 Introduction
This year Stars has proposed new results related to its three main research axes: (i) perception for activity recognition, (ii) action recognition and (iii) semantic activity recognition.
Perception for Activity Recognition
Participants: François Brémond, Antitza Dantcheva, Vishal Pani, Indu Joshi, David Anghelone, Laura M. Ferrari, Hao Chen, Valeriya Strizhkova.
The new results for perception for activity recognition are:
- Unsupervised Lifelong Person Re-identification via Contrastive Rehearsal (see 7.2)
- P-Age: Pexels Dataset for Robust Spatio-Temporal Apparent Age Classification (see 7.3)
- Current Challenges with Modern Multi-Object Trackers (see 7.4)
- MAURA: Video Representation Learning for Emotion Recognition Guided by Masking Action Units and Reconstructing Multiple Angles (see 7.5)
- HEROES: Facial age estimation using look-alike references (see 7.6)
- On estimating uncertainty of fingerprint enhancement models (see 7.7)
- ANYRES: Generating High-Resolution visible-face images from Low-Resolution thermal-face images (see 7.8)
- Face attribute analysis from structured light: an end-to-end approach (see 7.9)
- Attending Generalizability in Course of Deep Fake Detection by Exploring Multi-task Learning (see 7.10)
- Unsupervised domain alignment of fingerprint denoising models using pseudo annotations (see 7.11)
- Efficient Multimodal Multi-dataset Multitask Learning (see 7.12)
- Computer vision and deep learning applied to face recognition in the invisible spectrum (see 7.13)
- Dimitra: Diffusion Model talking head generation based on audio-speech (see 7.14)
Action Recognition
Participants: François Brémond, Antitza Dantcheva, Monique Thonnat, Mohammed Guermal, Tanay Agrawal, Abid Ali, Po-Han Wu, Di Yang, Rui Dai, Snehashis Majhi, Tomasz Stanczyk.
The new results for action recognition are:
- MultiMediate '23: Engagement Estimation and Body Behavior Recognition in Social Interactions (see 7.15)
- ACTIVIS: Loose Social-Interaction Recognition for Therapy Videos (see 7.16)
- JOADAA: joint online action detection and action anticipation (see 7.17)
- OE-CTST: Outlier-Embedded Cross Temporal Scale Transformer for Weakly-supervised Video Anomaly Detection (see 7.18)
- LAC - Latent Action Composition for Skeleton-based Action Segmentation (see 7.19)
- Self-supervised Video Representation Learning via Latent Time Navigation (see 7.20)
Semantic Activity Recognition
Participants: François Brémond, Monique Thonnat, Alexandra Konig, Rachid Guerchouche, Michal Balazia.
For this research axis, the contributions are:
- Large Vision Language Model for Temporal Action Detection (see 7.21)
- MEPHESTO: Multimodal Dataset of Psychiatric Patient-Clinician Interactions (see 7.22)
- Multimodal Transformers with Forced Attention for Behavior Analysis (see 7.23)
- StressID: a Multimodal Dataset for Stress Identification (see 7.24)
GitHub Repositories:
Along with these new results, algorithms have been designed. All codes are open source and are available via GitHub.
Open data:
Along with these algorithms, we have provided several benchmark datasets:
- Stress ID dataset : stress experiment with ECG and videos (see Valeriya Strizhkova work 7.24).
- Toyota Smarthome datasets : Real-World Activities of Daily Living (see Di Yang work 7.19).
7.2 Unsupervised Lifelong Person Re-identification via Contrastive Rehearsal
Participants: Hao Chen, François Brémond.
Existing unsupervised person re-identification (ReID) methods focus on adapting a model trained on a source domain to a fixed target domain. However, an adapted ReID model usually only works well on a certain target domain, but can hardly memorize the source domain knowledge and generalize to upcoming unseen data. In this paper, we propose unsupervised lifelong person ReID, which focuses on continuously conducting unsupervised domain adaptation on new domains without forgetting the knowledge learned from old domains. To tackle unsupervised lifelong ReID, we conduct a contrastive rehearsal on a small number of stored old samples while sequentially adapting to new domains. We further set an image-to-image similarity constraint between old and new models to regularize the model updates in a way that suits old knowledge. We sequentially train our model on several large-scale datasets in an unsupervised manner and test it on all seen domains as well as several unseen domains to validate the generalizability of our method. Our proposed unsupervised lifelong method achieves strong generalizability, which significantly outperforms in accuracy previous lifelong methods on both seen and unseen domains. The code is available here.
7.3 P-Age: Pexels Dataset for Robust Spatio-Temporal Apparent Age Classification
Participants: Abid Ali, Ashish Marisetty, François Brémond.
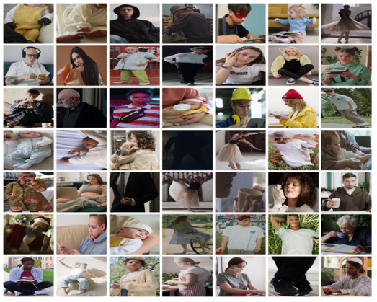
Age estimation is a challenging task that has many applications in various domains such as social robotics, video surveillance, business intelligence, social networks, and demography. Typically, the goal of age estimation involves predicting the age of a person by his/her facial appearance, which can be affected by many factors such as image resolution, lighting condition, pose, expression, occlusion, and makeup. To address these challenges, we propose AgeFormer 19 which utilizes spatio-temporal information on the dynamics of the entire body dominating face-based methods for age classification. Our novel two-stream architecture uses TimeSformer and EfficientNet as backbones, to effectively capture both facial and body dynamics information for efficient and accurate age estimation in videos. Furthermore, to fill the gap in predicting age in real-world situations from videos, we construct a video dataset called Pexels Age (P-Age) 19 (see Fig. 4 for age classification). The proposed method achieves superior results compared to existing face-based age estimation methods and is evaluated in situations where the face is highly occluded, blurred, or masked.
In conclusion, our novel video-based model achieves a precise age classification in challenging situations. The proposed architecture utilizes spatio-temporal information of the dynamics of the entire body dominating face-based methods for age classification. For more details please refer to 19. This was accepted in WACV-2024 19.
7.4 Current Challenges with Modern Multi-Object Trackers
Participants: Tomasz Stanczyk, François Brémond.
Multi-object tracking (MOT) is a task of associating the same objects in a video sequence across many frames. Current trend implies state-of-the-art algorithms following the paradigm of tracking by detection, i.e. the objects of interest (e.g. people) are detected on each frame and then associated (linked) across the frames. In this manner, so-called tracklets are created, each of which can be perceived as a collection of detections per object (person) across the consecutive video frames.
Multi-object tracking algorithms reach impressive performance on the benchmark datasets that they are trained and evaluated on, especially with their object detector parts tuned. When these algorithms are exposed to new videos though, the performance of the detection and tracking becomes poor, making them not usable. In our paper 24 published at the ACVR workshop at ICCV 2023 we shed a light on understanding this behavior and discuss how we can move forward regarding these issues.
Besides, we present the common errors made by the modern trackers, even when their trainable components are heavily tuned on the datasets. The most common errors are identity switches, fragmented tracklets and missed tracklets. An identity switch happens when one person (object) is tracked with a specific ID number and then, due to an occlusion, a crowded scene or other challenging scenario(s), another independent person gets assigned the same ID. An example is presented below in Fig. 5, where two different people are assigned to the same id number 22. Fragmented tracklet occurs when one person being tracked obtains more than one ID while present on the scene - their (ought-to-be full) track is fragmented into several separate tracklets. Missed tracklet denotes person not being tracked partially or at all during their presence at the scene. All these cases, identity switches, fragmented and missed tracklets are highly undesired, as tracking needs to be reliable in order to enable complete localization as well as further analysis of any subject of interest at any point of time. In the referenced paper, we also propose some directions and high-level ideas on how to proceed with those problems. Among the others, we mention taking into account all available association cues: appearance, motion, time, location, but also more complex manner of combining them and more constraints on the association process, e.g. based on context information.
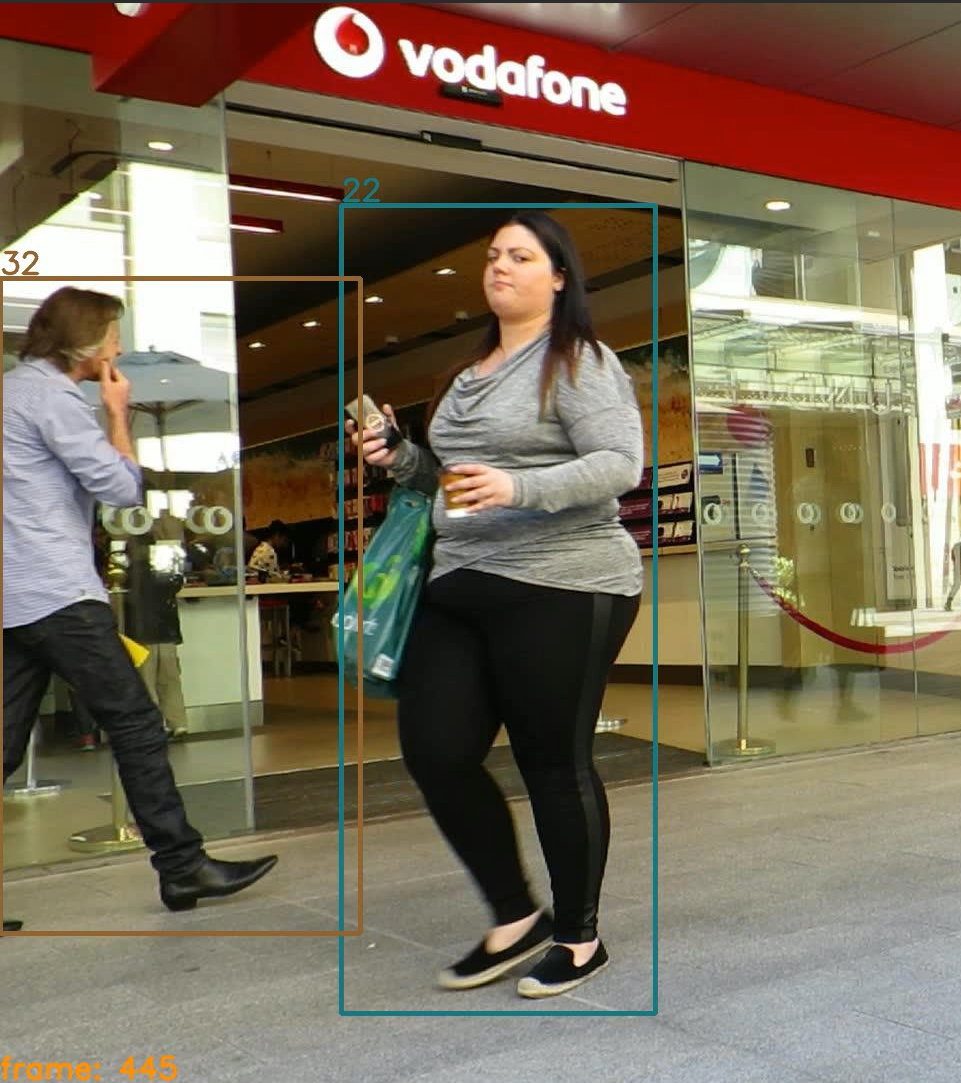
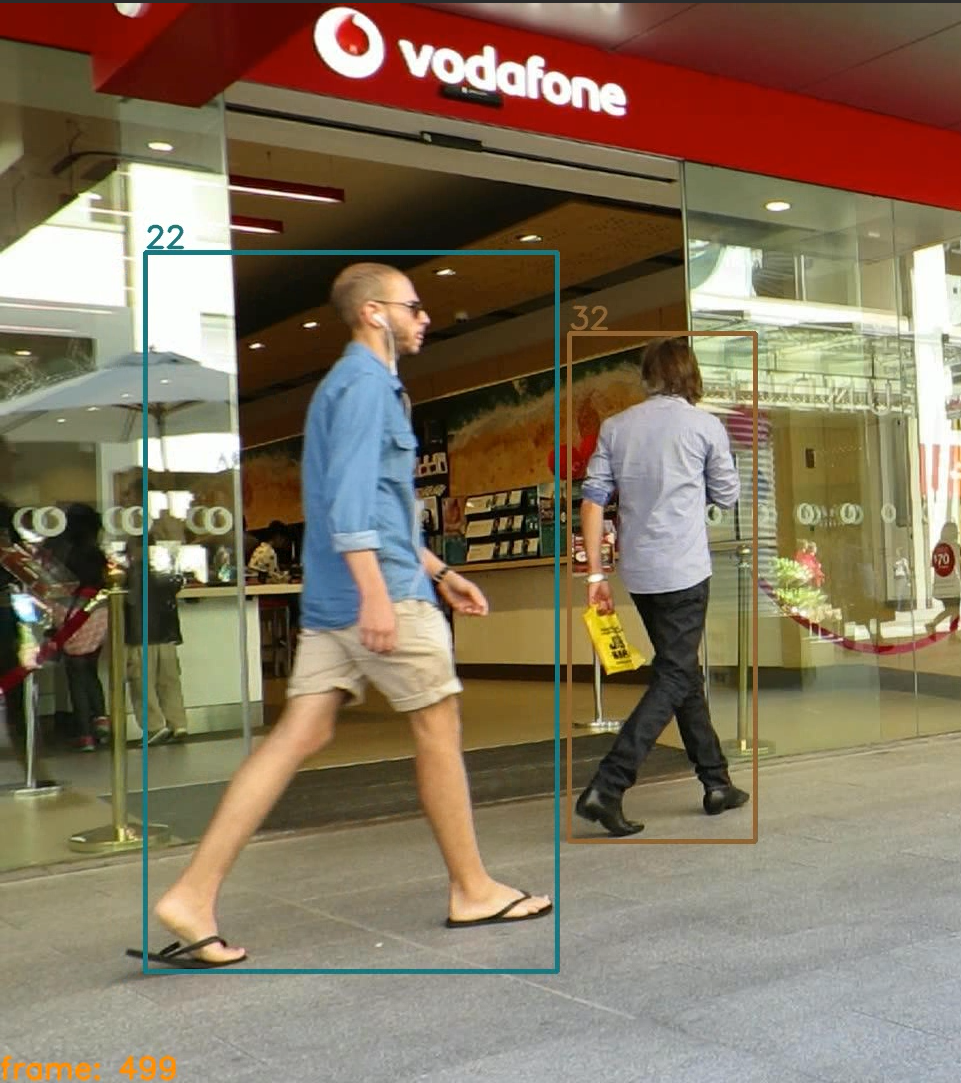
Further, we also discuss generalizability and reliability of the current multi-object trackers. Most importantly, we point that their performance is dependent on trainable components with high ranking results obtained with private detections (provided by the tracker itself) and the performance drops when not heavily tuned, public detections (provided by the dataset authors). Furthermore, we observe good performance on datasets when the trackers are tuned, yet poor performance on new videos, making the trackers not usable there.
Besides the mentioned paper, more works are currently under development, including, but not limited to incorporating the proposed directions and improvements, and aiming to resolving the mentioned challenges with the view of improving the overall performance of multi-object tracking. Notably, long-term tracking is considered, which involves tracking the subject for the longer periods of time, ideally during their whole presence at the scene.
7.5 MAURA: Video Representation Learning for Emotion Recognition Guided by Masking Action Units and Reconstructing Multiple Angles
Participants: Valeriya Strizhkova, Laura M., Antitza Dantcheva, François Brémond.
Video-based conversational emotion recognition entails challenges such as handling of facial dynamics, small available datasets, subtle and fine-grained emotions and extreme face Angle. Towards addressing these challenges, we propose the Masking Action Units and Reconstructing multiple Angles (MAURA) video autoencoder pre-training framework (see Figure 7). MAURA is an efficient self-supervised method that permits the use of raw data and small datasets, while preserving end-to-end emotion classification with Vision Transformer. Further, MAURA masks videos using the location with active Action Units and reconstructs synchronized multi-view videos, thus learning the dependencies between muscle movements and encoding information, which might only be visible in few frames and in certain poses/views. Based on one view (e.g., frontal), the encoder reconstructs additional views (e.g., top, down, laterals). Such masking and reconstructing strategy provides a powerful representation, beneficial in affective downstream tasks. Our experimental analysis shows that we consistently outperform in accuracy the state-of-the-art in the challenging settings of subtle and fine-grained emotion recognition on four video-based emotion datasets including in-the-wild DFEW, CMU-MOSEI, MFA and multi-view MEAD datasets (see Figure 6). Our results suggest that MAURA is able to learn robust and generic representations for emotion recognition.
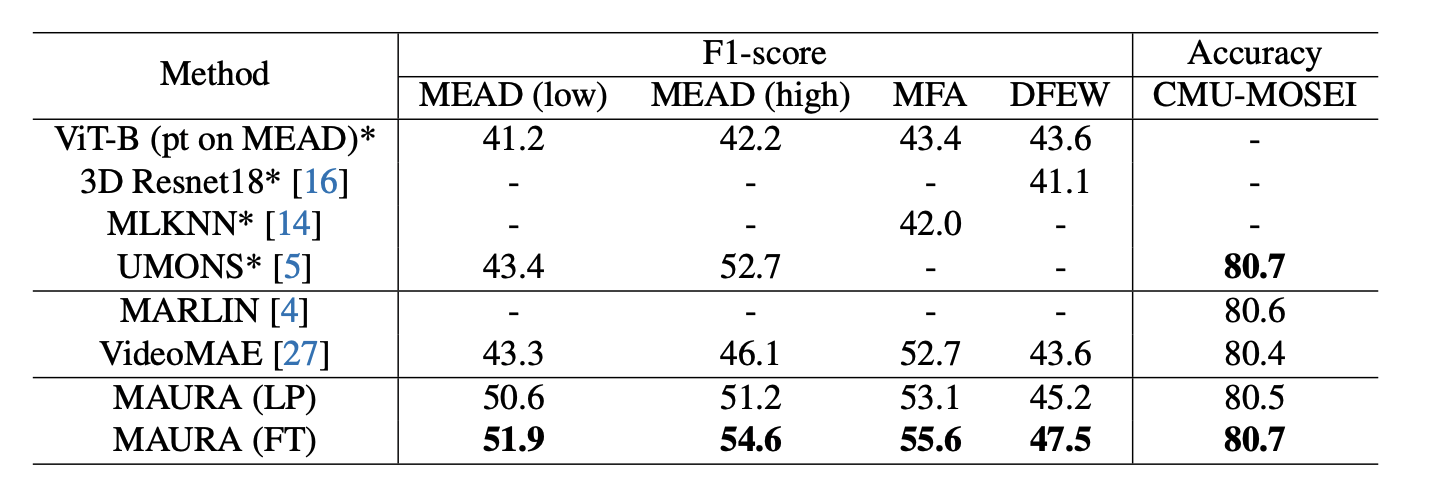
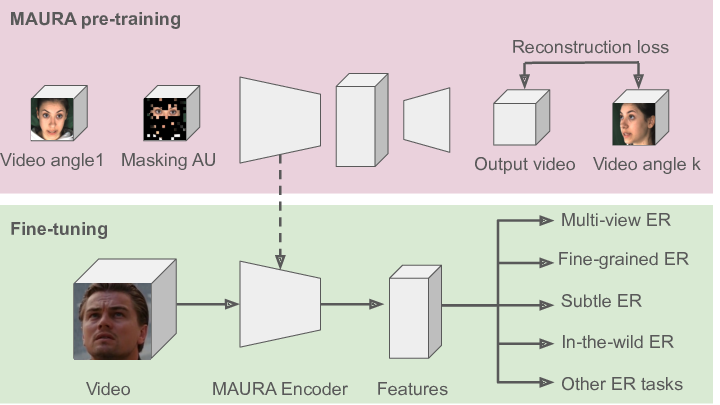
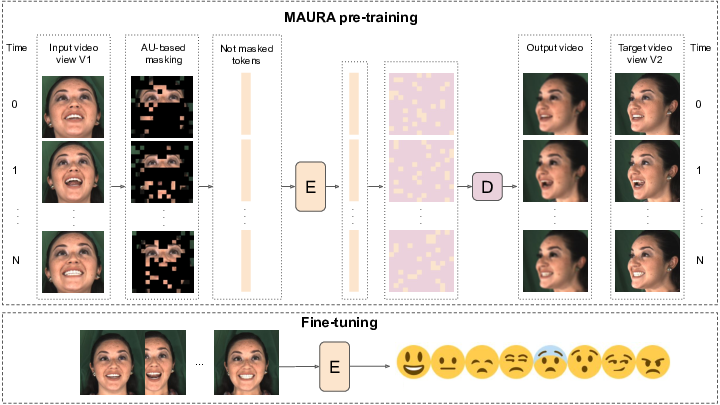
7.6 HEROES: Facial age estimation using look-alike references
Participants: Olivier Huynh, François Brémond.
By providing an accurate age estimation, forensic tools can be enhanced to reinforce the capabilities of Law Enforcement Agencies (LEAs) to combat Child Sexual Abuse and Exploitation. The research project highlights on age/gender estimation are as follows:
Age Estimation challenges identification: The main challenges related to facial age estimation have been identified and a solution has been implemented to tackle each of them: ambiguity and ordinal property (using Adaptive Label Distribution Learning method), occlusion and variation in poses (a model has been carefully designed by using attention upon multi-scale feature maps) and biases of datasets and variability between groups of people (idea of using look-alike references described below).
Performing comparisons with relevant references : This approach is inspired on how we, as humans, proceed to estimate the age of a person. This process leverages the use of look-alike labelled references to scaffold absolute markers and carry out an estimation by comparing local characteristics. It relies on the assumption : "People who share physical similarities share a close age and a close ageing trajectory" (illustrated in Figure 9). Although the assumption seems simple, its implementation in a Deep Learning model is challenging due to nature of the data used (ie. sparse crossage sequence) which can lead to overfitting.
Address the challenges of overfitting : Experiments with straightforward architectures like Vision Transformers or Convolutional Vision Transformers show that the models overfits on the combination of labels of references. To tackle this issue, we have developed a relative inference process, expressing the age labels relatively across densely (over the age trajectory) generated latent vectors. Another required ability to deal with sparse crossage sequence is to ignore non relevant references (ie. too far from the query). To this end, a specific objective function, including both ordinal reasoning and local inferring has been designed.
Include more generic data : A weakly-supervised algorithm has been developed to incorporate images from regular age datasets. It works with a trial and error logic, building batches with control sequences and select the batch whose error is minimal after one update step.
Outcomes : The results prove the viability of our approach and are superior in accuracy to state-of-the-art.
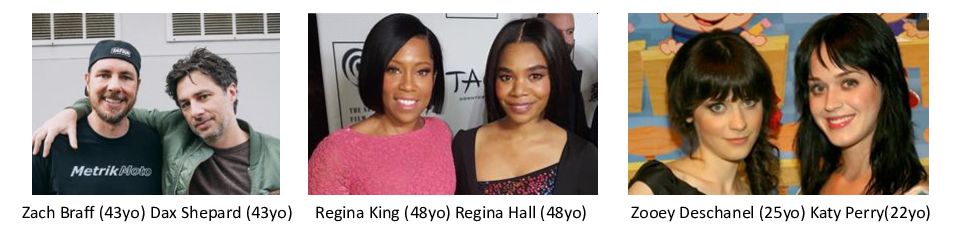
7.7 On estimating uncertainty of fingerprint enhancement models
Participants: Indu Joshi, Antitza Dantcheva.
The state-of-the-art models for fingerprint enhancement are sophisticated deep neural network architectures that eliminate noise from fingerprints by generating fingerprints image with improved ridge-valley clarity. However, these models perform fingerprint enhancement like a black box and do not specify whether a model is expected to generate an erroneously enhanced fingerprint image. Uncertainty estimation is a standard technique to interpret deep models. Generally, uncertainty in a deep model arises because of uncertainty in parameters of the model (termed as model uncertainty) or noise present in the data (termed as data uncertainty). Recent works showcase the usefulness of uncertainty estimation to interpret fingerprint preprocessing models. Motivated by these works, this book chapter 27 presents a detailed analysis of the usefulness of estimating model uncertainty and data uncertainty of fingerprint enhancement models. Furthermore, we also study the generalization ability of both these uncertainties on fingerprint ROI segmentation. A detailed analysis of predicted uncertainties presents insights into the characteristics learnt by each of these uncertainties. Extensive experiments on several challenging fingerprint databases demonstrate the significance of estimating the uncertainty of fingerprint enhancement models.
7.8 ANYRES: Generating High-Resolution visible-face images from Low-Resolution thermal-face images
Participants: David Anghelone, Antitza Dantcheva.
Cross-spectral Face Recognition (CFR) aims to compare facial images across different modalities, i.e., the visible and thermal spectra. CFR is more challenging than traditional face recognition (FR) due to the profound modality gap in-between spectra. As related applications range from night-vision FR to robust presentation attacks detection, acquisition involves capturing images at various distances, represented by different image resolutions. Prior approaches have addressed CFR by considering a fixed resolution, necessitating that a subject stands at a precise distance from a given sensor during acquisition, which constitutes an impractical scenario in real-life. Towards loosening this constraint, we propose ANYRES 20, 28, a unified model endowed with the ability to handle a wide range of input resolutions. ANYRES generates high resolution visible images from low resolution thermal images, placing emphasis on maintaining the cross-spectral identity. We demonstrate the effectiveness of the method and present extensive FR experiments on multi-spectral paired face datasets (see Figure 10).
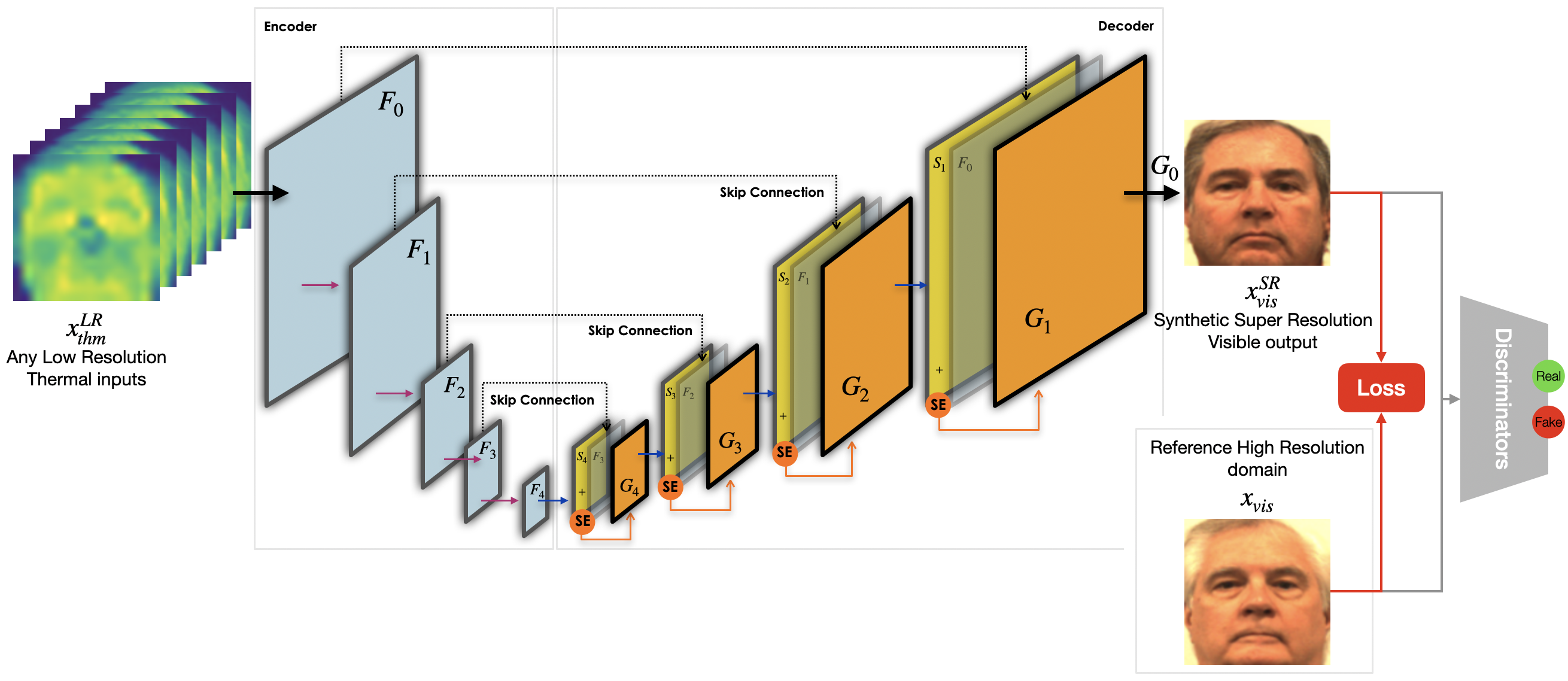
7.9 Face attribute analysis from structured light: an end-to-end approach
Participants: Antitza Dantcheva, Francois Bremond.
In this work 16 we explore structured-light imaging for face analysis. Towards this and due to lack of a publicly available structured-light face dataset, we (a) firstly generate a synthetic structured-light face dataset constructed based on the RGB-dataset London Face and the RGB-D dataset Bosphorus 3D Face. We then (b) propose a conditional adversarial network for depth map estimation from generated synthetic data. Associated quantitative and qualitative results suggest the efficiency of the proposed depth estimation technique. Further, we (c) study the estimation of gender and age directly from (i) structured-light, (ii) binarized structured-light, as well as (iii) estimated depth maps from structured-light. In this context we (d) study the impact of different subject-to-camera distances, as well as pose-variations. Finally, we (e) validate the proposed gender and age models that we train on synthetic data on a small set of real data, which we acquire. While these are early results, our findings clearly indicate the suitability of structured-light based approaches in facial analysis.
7.10 Attending Generalizability in Course of Deep Fake Detection by Exploring Multi-task Learning
Participants: Pranav Balaji, Antitza Dantcheva.
This work 21 explores various ways of exploring multi-task learning (MTL) techniques aimed at classifying videos as original or manipulated in cross-manipulation scenario to attend generalizability in deep fake scenario. The dataset used in our evaluation is FaceForensics++, which features 1000 original videos manipulated by four different techniques, with a total of 5000 videos. We conduct extensive experiments on multi-task learning and contrastive techniques, which are well studied in literature for their generalization benefits. It can be concluded that the proposed detection model is quite generalized, ie, accurately detects manipulation methods not encountered during training as compared to the state-of-the-art.
7.11 Unsupervised domain alignment of fingerprint denoising models using pseudo annotations
Participants: Indu Joshi, Antitza Dantcheva.
State-of-the-art fingerprint recognition systems perform far from satisfactory on noisy fingerprints. A fingerprint denoising algorithm is designed to eliminate noise from the input fingerprint and output a fingerprint image with improved clarity of ridges and valleys. To alleviate the unavailability of annotated data to train the fingerprint denoising model, state-of-the-art fingerprint denoising models generate synthetically distorted fingerprints and train the fingerprint denoising model on the synthetic data. However, a visible domain shift exists between synthetic training data and the real-world test data. Subsequently, state-of-the-art fingerprint denoising models suffer from poor generalization. To counter this drawback of state-of-the-art, this research proposes to align the synthetic and real fingerprint domains. Experiments conducted on publicly available rural Indian fingerprint demonstrate that after the proposed domain alignment, equal error rate improves from 7.30 to 6.10 on Bozorth matcher and 5.96 to 5.31 on minutiae cylinder code (MCC) matcher. Similar improved fingerprint recognition results are obtained for IIITD-MOLF database and private rural fingerprints database as well.
7.12 Efficient Multimodal Multi-dataset Multitask Learning
Participants: Tanay Agrawal, Mohammed Guermal, Michal Balazia, François Brémond.
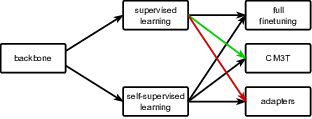
This work presents a new model agnostic architecture for cross-learning, called CM3T, applicable to transformer-based models (see Figure 11). Challenges in cross-learning involve inhomogeneous or even inadequate amount of training data, and lack of resources for retraining large pretrained models. Inspired from transfer learning techniques in NLP (adapters and prefix tuning), we introduce a plugin architecture that makes the model robust towards new or missing information. We also show that the backbone and other plugins do not have to be finetuned with these additions which makes training more efficient, requiring less resources and training data. We introduce two adapter blocks called multi-head vision adapters and cross-attention adapters for transfer learning and multimodal learning respectively. Through experiments and ablation studies on three datasets – Epic-Kitchens-100, MPIIGroupInteraction and UDIVA v0.5 – with different recording settings and tasks, we show the efficacy of this framework. With only 12.8% trainable parameters as compared to the backbone for video input and 22.3% trainable parameters for two additional modalities, we achieve comparable or even better results as compared to the state-of-the-art. Compared to similar methods, our work achieves this result without any specific requirements for pretraining/training and is a step towards bridging the gap between research and practical applications for the field of video classification. This work will be submitted to IJCAI-2024.
7.13 Computer vision and deep learning applied to face recognition in the invisible spectrum
Participants: David Anghelone, Antitza Dantcheva.
Cross-spectral face recognition (CFR) refers to recognizing individuals using face images stemming from different spectral bands, such as infrared vs. visible. While CFR is inherently more challenging than classical face recognition due to significant variation in facial appearance caused by the modality gap, it is useful in many scenarios including night-vision biometrics and detecting presentation attacks. The aim of this thesis 28 has been to develop an end-to-end thermal-to-visible face recognition system, which integrates new algorithms for (a) thermal face detection, as well as (b) thermal-to-visible spectrum translation, streamlined to bridge the modality gap. We note that any off-the-shelf facial recognition algorithm can be used for recognition, a fact ensured by the facial recognition platform (FRP) of Thales.
Towards the above goal, we present following contributions. Firstly, we collected a database comprising of multi-spectral face images captured simultaneously under four electromagnetic spectra. The database provides essential, rich and varied resources well-suited to replicate practical scenario of real-life operation for a cross-spectral biometrics system. Secondly, we proposed a pre-processing algorithm, streamlined to detect face and facial landmarks (TFLD) in the thermal spectrum. We designed the algorithm to be robust to adversarial conditions such as pose, expression, occlusion, poor image quality, long-range distance and related face alignment contributed significantly to improving face recognition scores. The third contribution had to do with spectrum translation, aimed at reducing the modality gap of visible and thermal spectrum w.r.t. face recognition. We presented a novel model, Latent-Guided Generative Adversarial Network (LG-GAN), which translates one spectrum (e.g., thermal) to another (visible), while preserving the identity across different spectral bands. The main focus of LG-GAN is related to explainability, namely providing insight in pertinent salient features. Discriminative across spectra, has additionally been pursued by an additional model, namely the Attention-Guided Generative Network (AG-GAN). Finally, tackling the challenge of multi-scale face recognition stemming from varying acquisition distance, we proposed an algorithm, ANYRES, which accepts any resolution of thermal face images, proceeding to translate such images into synthetic high-resolution visible images. We extended ANYRES to settings encountering users experiencing (extreme) facial poses, placing emphasis on thermal-to-visible face recognition in unconstrained environments.
7.14 Dimitra: Diffusion Model talking head generation based on audio-speech
Participants: Baptiste Chopin, Antitza Dantcheva, Tashvik Dhamija, Yaohui Wang.
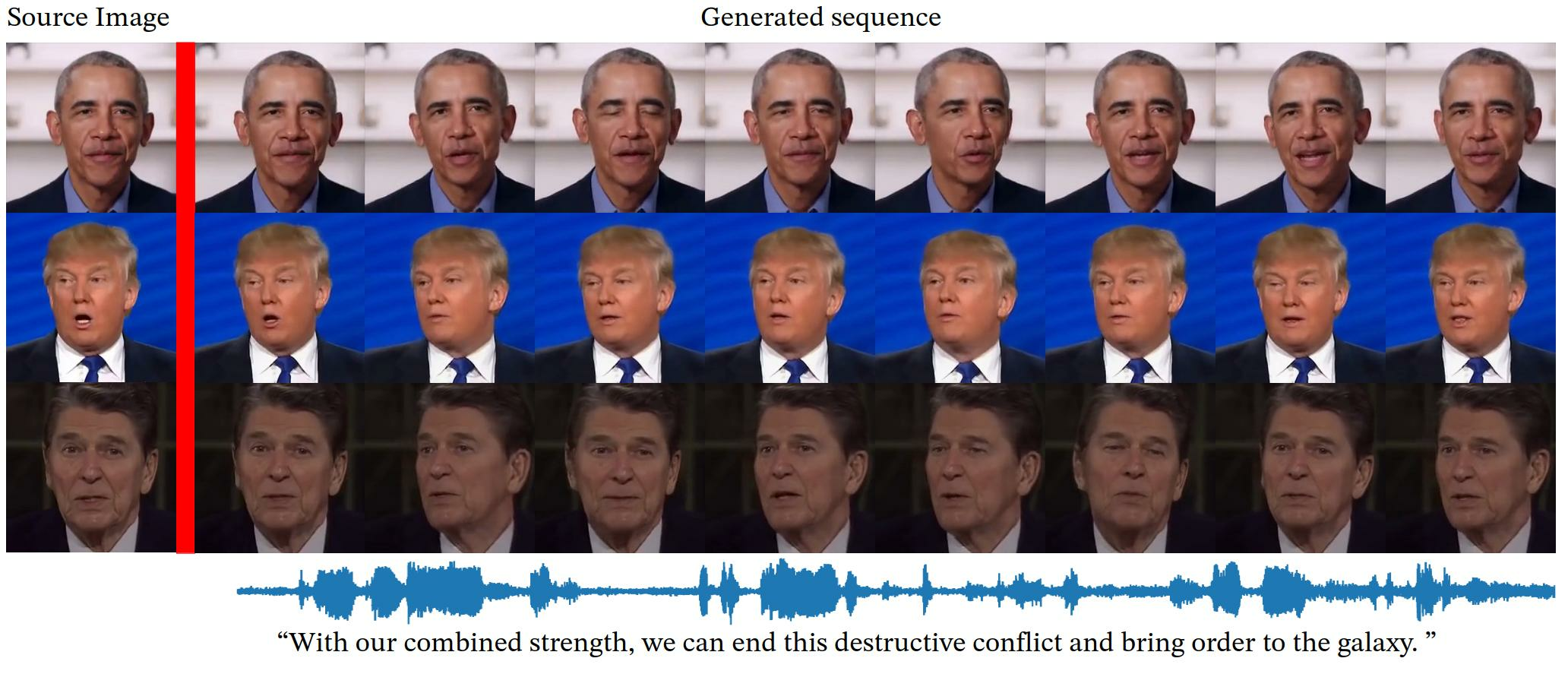
Applying deep generative models in talking head animation has sparked increasingly attention, aiming to animate real face videos, while keeping appearance and motion realistic. This progress has been fueled by a number of applications including digital humans, AR/VR, as well as filemaking, video games and chatbots. While video-driven talking head generation has become highly realistic, some applications necessitate other type of modalities as driving signal. For example, audio, as the most relevant and useful signal, has been explored by many previous works for talking head generation. Towards learning realistic talking heads from audio, previous works usually require motion and appearance information as input. An input image is used to provide appearance while a sequence of 3D Morphable Model (3DMM) is used to represent motion. In this work, we introduce Dimitra, a novel speech-driven talking head generation framework aiming at animating single human images based on speech (see Figure 12). With focus on producing photorealistic images, as well as natural and diverse motion. Deviating from previous works, our target is to learn global motion including lips, as well as head pose and expression motion directly from audio input. Towards achieving this goal, we propose a transformer-based diffusion model which takes a sequence phoneme, corresponding text as input to produce facial 3DMM parameters.
Our main contributions include the following.
- Diffusion model for talking head generation, placing emphasis on generating diverse videos, given the same audio input.
- Global generative model, which animates lips, expressions, as well as the head.
7.15 MultiMediate '23: Engagement Estimation and Body Behavior Recognition in Social Interactions
Participants: Michal Balazia, Mohammed Guermal, François Brémond.
Automatic analysis of human behavior is a fundamental prerequisite for the creation of machines that can effectively interact with and support humans in social interactions. In MultiMediate'23 17, we address two key human social behavior analysis tasks for the first time in a controlled challenge: engagement estimation and body behavior recognition in social interactions. For engagement estimation, we collected novel annotations on the NOvice eXpert Interaction database (NOXI, see Figure 13 left). For body behavior recognition, we annotated test recordings of the MPIIGroupInteraction corpus (MPIIGI, see Figure 13 right). We also present baseline results for both challenge tasks.
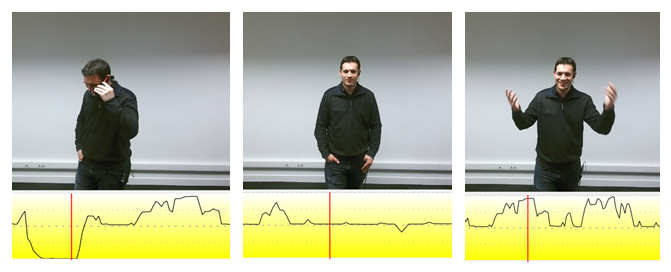
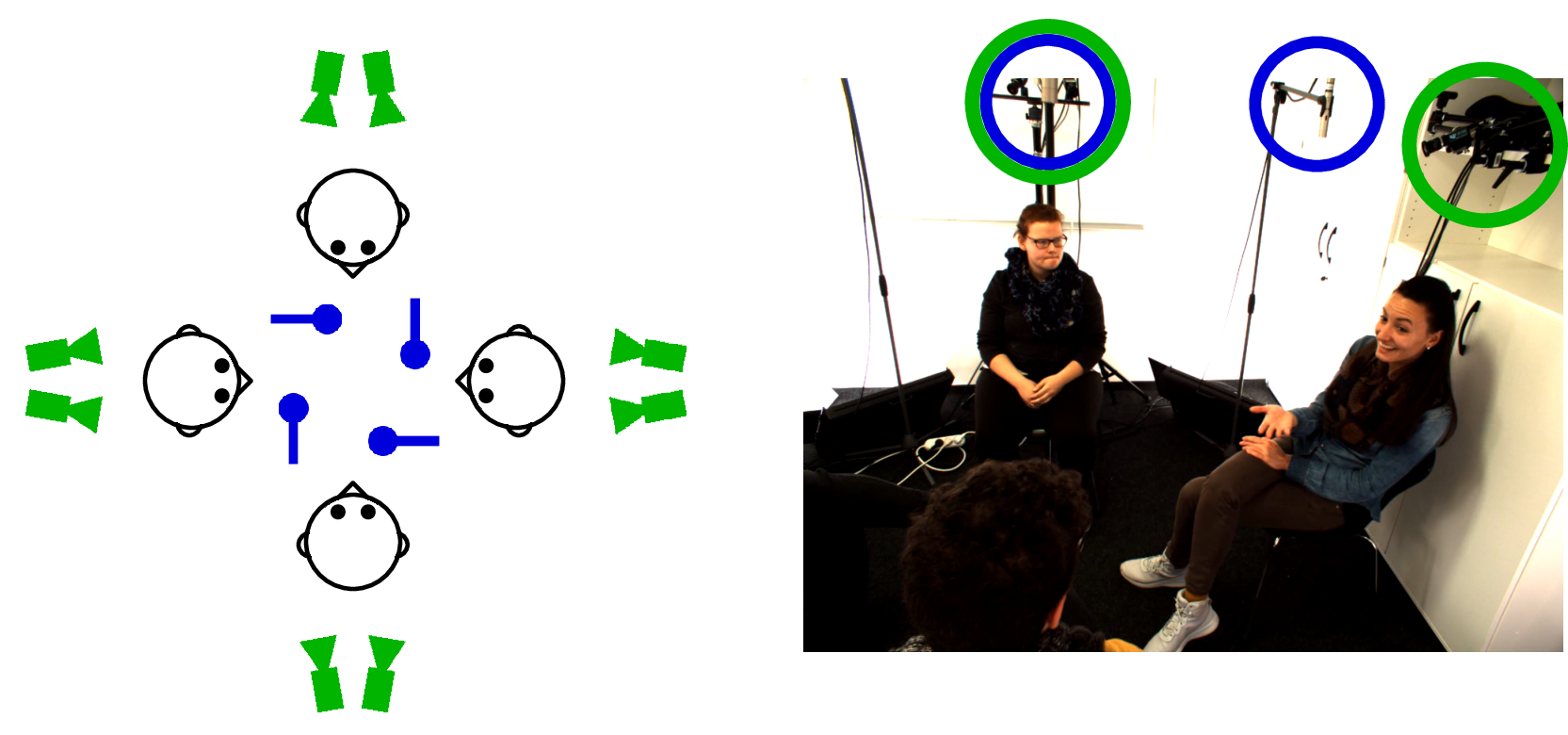
The engagement estimation task includes the continuous, frame-wise prediction of the level of conversational engagement of each participant on a continuous scale from 0 (lowest) to 1 (highest). Participants are encouraged to investigate multimodal as well as reciprocal behavior of both interlocutors. We make use of the concordance correlation coefficient to evaluate predictions on the test set.
We formulate the body behavior recognition task as multi-label classification. Challenge participants are required to predict which of 15 behavior classes are present in a 64 (2.13 sec) frame input window. For each 64-frame window, we provide a frontal view on the target participant as well as two side views (left and right). As the behavior classes on this task are highly unbalanced, we measure performance using average precision computed per class and aggregated using macro averaging, that is, giving the same weight to each class. This encourages challenge competitors to develop novel methods to improve performance on challenging low-frequency classes.
7.16 ACTIVIS: Loose Social-Interaction Recognition for Therapy Videos
Participants: Abid Ali, Rui Dai, François Brémond, Monique Thonnat, Susanne Thummler.
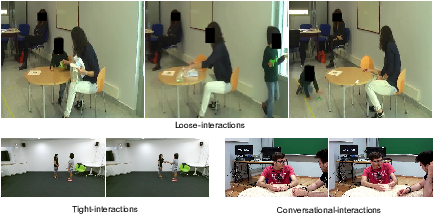
The computer vision community has explored dyadic interactions for atomic actions such as pushing, carry-object etc. But with the advancement in deep learning models, there is a need for exploring more complex dyadic situations like loose-interactions. These are interactions where two people perform certain atomic activities to complete a global action irrespective of temporal-synchronize and physical engagement, like cooking-together for example (see Fig. 14). Analyzing these types of dyadic-interactions has several useful applications in the medical domain for social-skills development and mental health diagnosis.
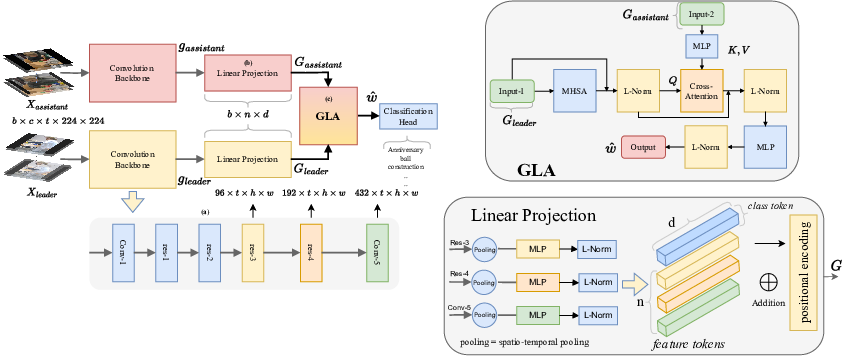
To achieve this, we propose a novel two-stream architecture to capture the loose-interaction between two individuals. Our model learns global abstract features from each of the two-streams via a CNNs backbone and fuses them using a new Global-Layer-Attention module based on a cross-attention strategy (Fig. 15). We evaluate our model on real-world autism diagnoses such as our Activis-Interactive dataset, and the publicly available Autism dataset for loose-interactions. Our network achieves baseline results on the Activis-Interactive and new SOTA results on the Autism datasets. Moreover, we study different social-interactions by experimenting on a publicly available dataset i.e. NTU-RGB+D (interactive classes from both NTU-60 and NTU-120). We have found that different interactions require different network designs. This work will be submitted to IJCAI 2024.
7.17 JOADAA: joint online action detection and action anticipation
Participants: Mohammed Guermal, Abid Ali, Rui Dai, François Brémond.
Action anticipation involves forecasting future actions by connecting past events to future ones. However, this reasoning ignores the real-life hierarchy of events which is considered to be composed of three main parts: past, present, and future. We argue that considering these three main parts and their dependencies could improve performance. On the other hand, online action detection is the task of predicting actions in a streaming manner. In this case, one has access only to the past and present information. Therefore, in online action detection (OAD) the existing approaches miss semantics or future information which limits their performance. To sum up, for both of these tasks, the complete set of knowledge (past-present-future) is missing, which makes it challenging to infer action dependencies, therefore having low performances. To address this limitation, we propose to fuse both tasks into a single uniform architecture. By combining action anticipation and online action detection, our approach can cover the missing dependencies of future information in online action detection. This method referred to as JOADAA, presents a uniform model that jointly performs action anticipation and online action detection. We validate our proposed model on three challenging datasets: THUMOS'14, which is a sparsely annotated dataset with one action per time step, CHARADES, and Multi-THUMOS, two densely annotated datasets with more complex scenarios. JOADAA achieves SOTA results on these benchmarks for both tasks.
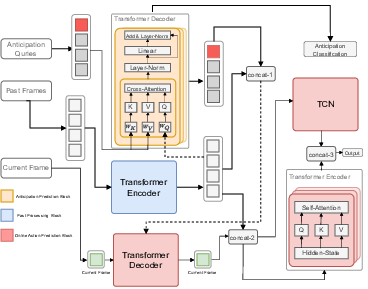
The whole architecture consists of three main parts, i) Past Processing Block, ii) Anticipation prediction Block, and iii) Online Action Prediction, as shown in Figure 16. First, a short-term past transformer-encoder enhances features. Second, an anticipation transformer-decoder anticipates the upcoming actions in the upcoming frames, using embedding output from the previous block and a set of learnable queries, which we call anticipation queries. Finally, a transformer-decoder uses the anticipation results and past information to predict the actions for the current frame (online action detection).
7.18 OE-CTST: Outlier-Embedded Cross Temporal Scale Transformer for Weakly-supervised Video Anomaly Detection
Participants: Snehashis Majhi, Rui Dai, François Brémond.
Video anomaly detection in real-world scenarios is challenging due to the complex temporal blending of long and short-length anomalies with normal ones. Further, it is more difficult to detect those than long anomalies due to: (i) Distinctive features characterizing the short and long anomalies with sharp and progressive temporal cues respectively; (ii) Lack of precise temporal information (i.e. weak-supervision) limits the temporal dynamics modeling of anomalies from normal events. In this work, we propose a novel `temporal transformer' framework for weakly-supervised anomaly detection: OE-CTST. The proposed framework 29 has two major components: (i) Outlier Embedder (OE) and (ii) Cross Temporal Scale Transformer (CTST). Unlike conventional position embedding, the proposed outlier embedder generates anomaly-aware temporal position encoding which enables the transformer to better encode global temporal relations among the normal and abnormal segments (i.e. temporal tokens). The anomaly-aware positions are generated by learning the temporal features of a uni-class distribution and treating the outlier as an anomaly. Then, the anomaly-aware position encodings are infused with the temporal tokens and processed by the CTST. The proposed CTST ensures a superior global temporal relation encoding among normal events and anomalies (i.e. both long and short) thanks to its two key components: multi-stage design choice, and Cross Temporal Field Attention block (CTFA). The multi-stage design choice allows the CTST to analyze the anomaly-aware position-infused input tokens at different scales by multi-scale tokenization. By this, the transformer encodes the fine-grained temporal relations for the short anomalies at the lower stage and coarse contextual relations for long anomalies at the higher stages. Further, each stage has a CTFA block to effectively encode the correlations between the temporal neighbor and distant tokens, where a stronger neighbor and distant correlations are encoded for short and long anomalies respectively.
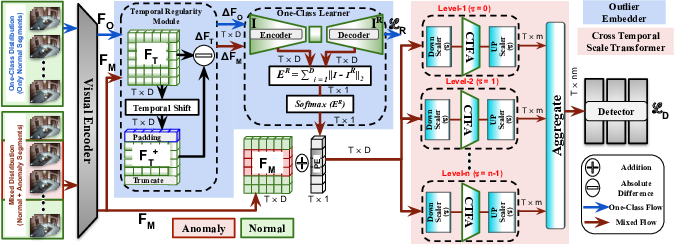
Our novel outlier embedded cross-temporal scale transformer (OE-CTST) delineated in Figure 17 aims to temporally detect normal and anomaly segments using weakly-labelled training videos. In this setting, a set of untrimmed videos with only video-level labels is given for training where a video is marked as normal (i.e. one-class) if it has no anomaly and to be anomaly (i.e. mixed) if it contains at least one abnormal clip. OE-CTST has four key building blocks: (A) Visual Encoder that extracts initial spatio-temporal representation, (B) Outlier Embedder (OE) that learns representations from normal segments and can generate anomaly-aware pseudo temporal position embeddings for long untrimmed anomaly videos, (C) Cross Temporal Scale Transformer (CTST) that ensures better global temporal relation modeling by encoding the stronger correlations between the temporally neighbor and distant tokens, (D) Detector that estimates anomaly scores for each temporal token to finally detect the anomalies.
7.19 LAC - Latent Action Composition for Skeleton-based Action Segmentation
Participants: Di Yang, Antitza Dantcheva, François Brémond.
Skeleton-based action segmentation requires recognizing composable actions in untrimmed videos. Current approaches decouple this problem by first extracting local visual features from skeleton sequences and then processing them by a temporal model to classify frame-wise actions. However, their performances remain limited as the visual features cannot sufficiently express composable actions. In this context, we propose Latent Action Composition (LAC) 25, a novel self-supervised framework aiming at learning from synthesized composable motions for skeleton-based action segmentation (see Fig. 18 for the general pipeline). LAC is composed of a novel generation module towards synthesizing new sequences. Specifically, we design a linear latent space in the generator to represent primitive motion. New composed motions can be synthesized by simply performing arithmetic operations on latent representations of multiple input skeleton sequences. LAC leverages such synthesized sequences, which have large diversity and complexity, for learning visual representations of skeletons in both sequence and frame spaces via contrastive learning. The resulting visual encoder has a high expressive power and can be effectively transferred onto action segmentation tasks by end-to-end fine-tuning without the need for additional temporal models. We conduct a study focusing on transfer-learning and we show that representations learned from pre-trained LAC outperform the state-of-the-art by a large margin on TSU, Charades, PKU-MMD datasets.
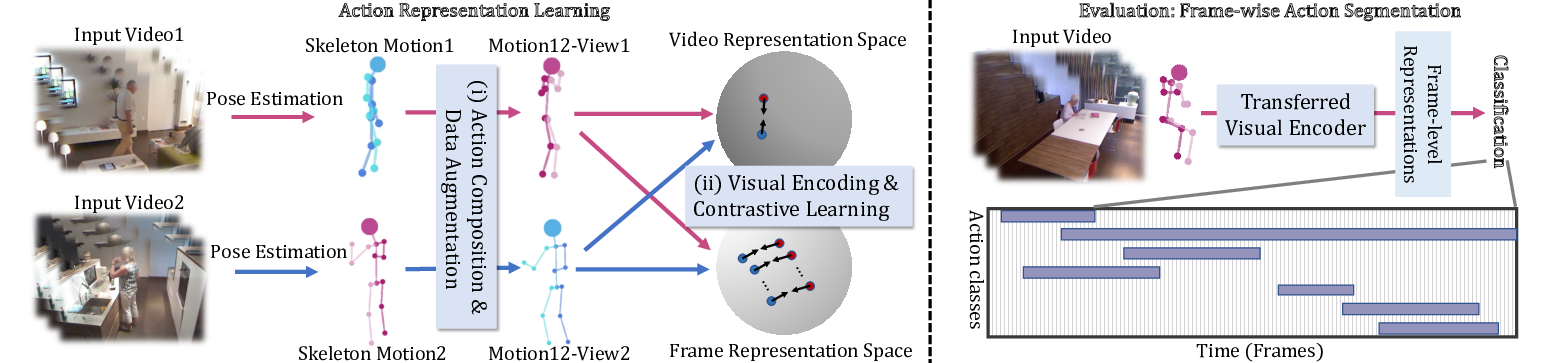
7.20 Self-supervised Video Representation Learning via Latent Time Navigation
Participants: Di Yang, Antitza Dantcheva, François Brémond.
Self-supervised video representation learning aimed at maximizing similarity between different temporal segments of one video, in order to enforce feature persistence over time. This leads to loss of pertinent information related to temporal relationships, rendering actions such as `enter' and `leave' to be indistinguishable. To mitigate this limitation, we propose Latent Time Navigation (LTN) 26, a time-parameterized contrastive learning strategy that is streamlined to capture fine-grained motions. Specifically, we maximize the representation similarity between different video segments from one video, while maintaining their representations time-aware along a subspace of the latent representation code including an orthogonal basis to represent temporal changes (see Fig. 19). Our extensive experimental analysis suggests that learning video representations by LTN consistently improves performance of action classification in fine-grained and human-oriented tasks (e.g., on Toyota Smarthome dataset). In addition, we demonstrate that our proposed model, when pre-trained on Kinetics-400, generalizes well onto the unseen real world video benchmark datasets UCF101 and HMDB51, achieving state-of-the-art performance in action recognition.
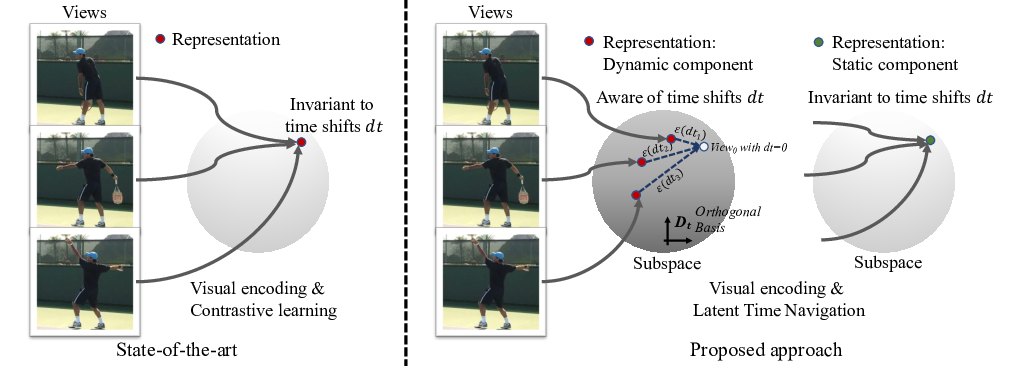
7.21 Large Vision Language Model for Temporal Action Detection
Participants: Rui Dai, Srijan Das, François Brémond.
The challenge of long-term video understanding remains constrained by the efficient extraction of object semantics and the modeling of their relationships for downstream tasks. Although OpenAI's CLIP visual features exhibit discriminative properties for various vision tasks, particularly in object encoding, they are suboptimal for long-term video understanding. To address this issue, we present the Attributes-Aware Network (AAN), which consists of two key components: the Attributes Extractor and a Graph Reasoning block. These components facilitate the extraction of object-centric attributes and the modeling of their relationships within the video. By leveraging CLIP features, AAN outperforms state-of-the-art approaches on two popular action detection datasets: Charades and Toyota Smarthome Untrimmed datasets. This work was published at the British Machine Vision Conference, BMVC 2023 in Nov 2023 23.
7.22 MEPHESTO: Multimodal Dataset of Psychiatric Patient-Clinician Interactions
Participants: Michal Balazia, François Brémond.
Identifying objective and reliable markers to tailor diagnosis and treatment of psychiatric patients remains a challenge, as conditions like major depression, bipolar disorder, or schizophrenia are qualified by complex behavior observations or subjective self-reports instead of easily measurable somatic features. Recent progress in computer vision, speech processing and machine learning has enabled detailed and objective characterization of human behavior in social interactions. However, the application of these technologies to personalized psychiatry is limited due to the lack of sufficiently large corpora that combine multimodal measurements with longitudinal assessments of patients covering more than a single disorder. To close this gap, we introduce Mephesto, a multi-centre, multi-disorder longitudinal corpus creation effort designed to develop and validate novel multimodal markers for psychiatric conditions. Mephesto consists of multimodal audio, video, and physiological recordings as well as clinical assessments of psychiatric patients covering a six-week main study period as well as several follow-up recordings spread across twelve months.
In this work we outline the rationale and study protocol and introduced four cardinal use cases that build the foundation of a new state-of-the-art in personalized treatment strategies for psychiatric disorders. The overall study design is presented in Figure 20 and consists of two phases. During the main study phase, interactions between the patients and clinician are recorded with video, audio, and physiological sensors. In the succeeding follow-up phase, videoconference-based recordings and ecological momentary assessments are recorded using a videoconferencing system.
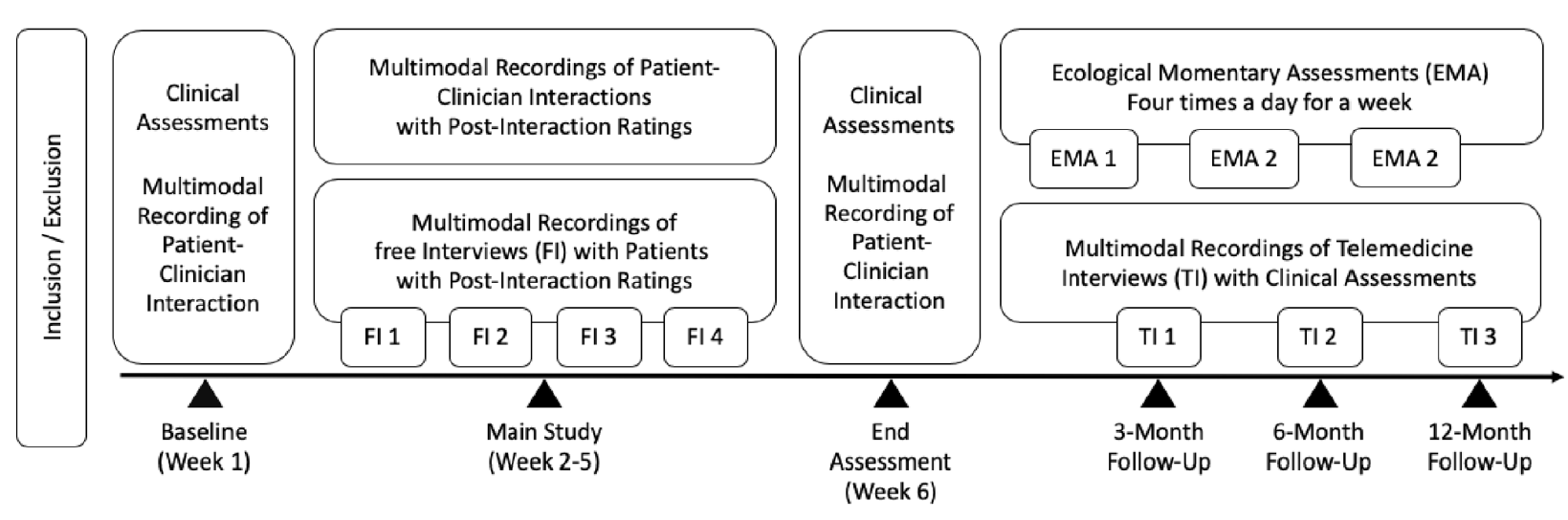
The dataset is being collected at four locations in France and Germany and a new recording site is being prepared in Georgia. Current state of recording:
- Hospital Pasteur, Nice: 27 patients, 198 recordings
- Centre Therapeutique La Madeleine, Nice: 8 patients, 31 recordings
- Universitatsklinikum des Saarlandes, Homburg: 12 patients, 44 recordings
- Carl von Ossietzky University, Oldenburg: 24 patients, 117 recordings
- Central Psychiatric Clinic, Tbilisi: 0 patients, 0 recordings
Diagnoses include schizophrenia, depression and bipolar disorder. Dataset does not include control subjects. Each patient is contributing with 1–8 videos, roughly 5.5 videos on average. In addition to video, the recordings include patients' and clinicians' biosignals: electrodermal activity (EDA), respiration signals (BVP, IBI), heart rate, temperature, and accelerometer. Videos are recorded by Azure Kinect and biosignals by Empatica. People do not wear face masks while being recorded, although to minimize the transmission of COVID-19 there is a large transparent plexi-glass. Dataset is confidential, but many patients agreed to publish their raw or anonymized data for research purposes. Figure 21 shows the recording scene with two clinicians on the opposite sides of an office desk, wearing Empatica wristbands and separated by the plexi-glass that is out of camera receptive fields. Screenshot of an example recording with a clinician and a patient is displayed in Figure 22.
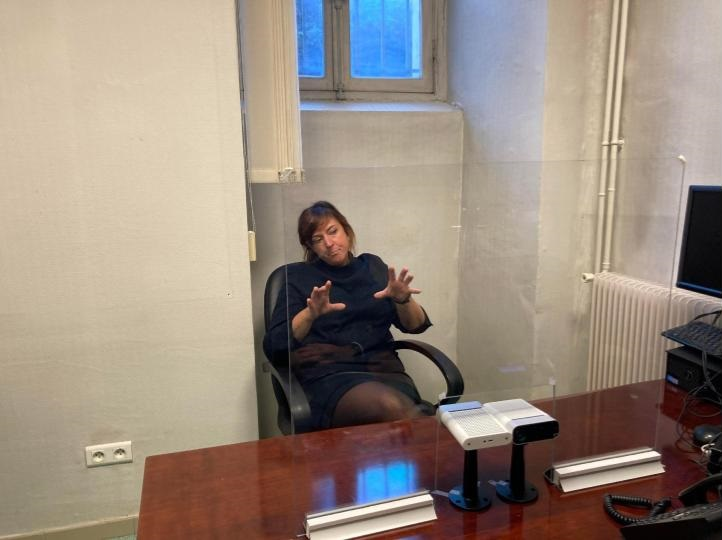
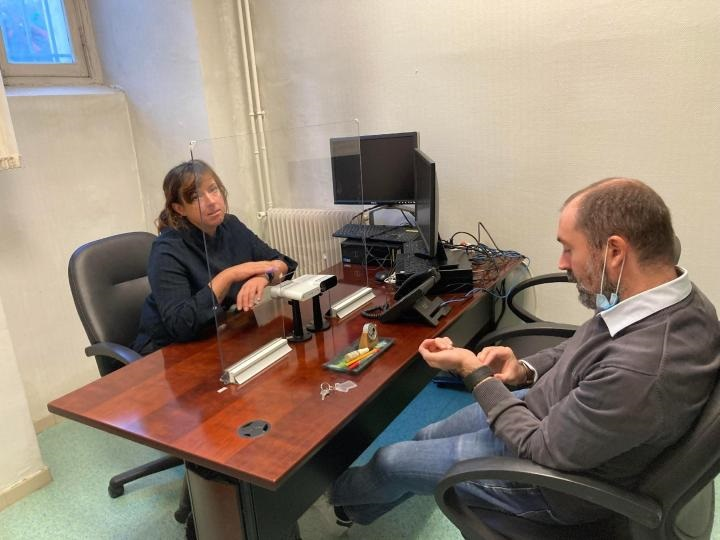
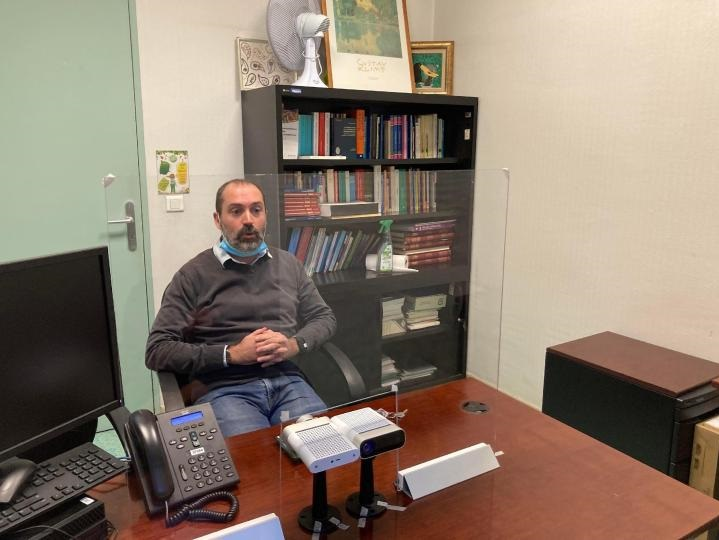
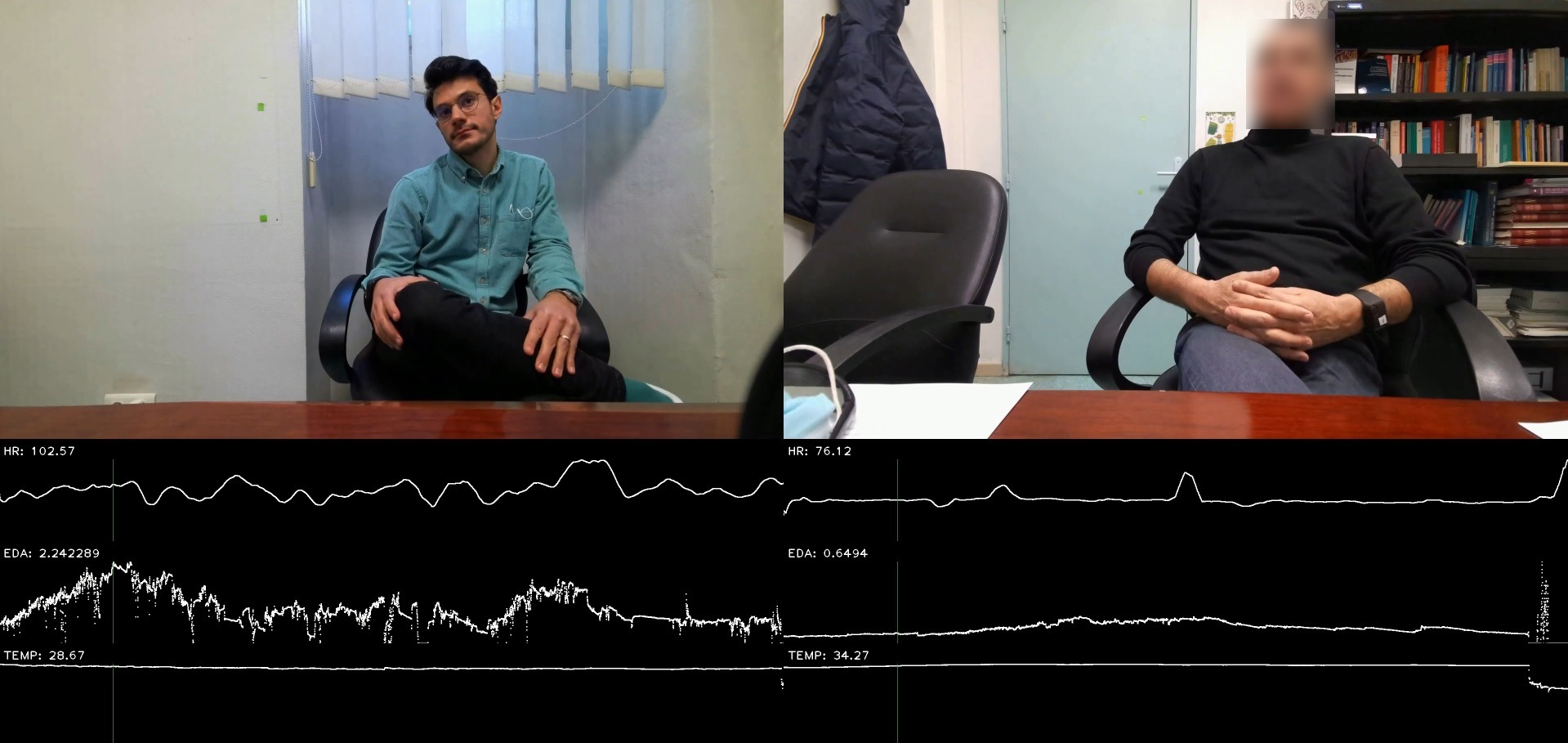
7.22.1 Demo Tool for the Analysis of MEPHESTO Dataset
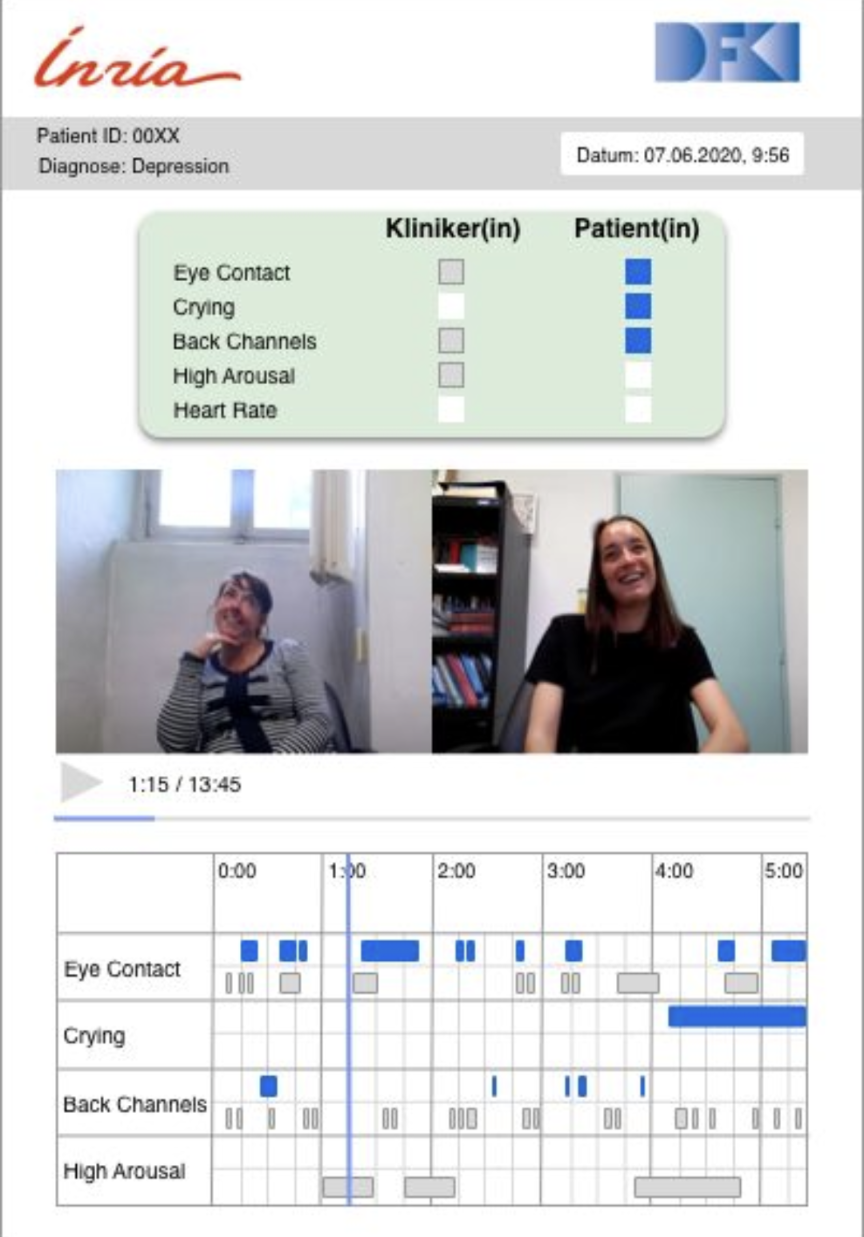
Multiple researchers are working on the MEPHESTO dataset, raising the need for a cumulative tool to visualize the output of the research. Thus, we developed a tool which visualizes various detected features along with synchronized videos for both the patients and the clinician. There is an exhaustive list of features from which the user can choose to visualize as many as they want for the patient and the clinician separately. These are visualized on a timeline which can be manipulated as desired. Synchronized biosignals can also be visualized along with the videos too. This tool will help in making the output of our research more accessible to clinicians, allowing them to utilize it for better diagnosis and formulation of treatment plans. Figure 23 shows a screenshot of the demo tool.
7.23 Multimodal Transformers with Forced Attention for Behavior Analysis
Participants: Tanay Agrawal, Michal Balazia, François Brémond.
Human behavior understanding requires looking at minute details in the large context of a scene containing multiple input modalities. It is necessary as it allows the design of more human-like machines. While transformer approaches have shown great improvements, they face multiple challenges such as lack of data or background noise. To tackle these, we introduce the Forced Attention (FAt) Transformer 18 which utilizes forced attention with a modified backbone for input encoding and a use of additional inputs.
We provide the spatial localization of the target person via a segmentation map to the network, thereby forcing the network to not attend to the background. Since the background might have important information, we observe that the network learns to assign attention to parts in the background that are also relevant to the provided background. In Figure 24 we provide five studied ways of providing the model with segmentation maps. In addition to improving the performance on different tasks and inputs, the modification requires less time and memory resources.
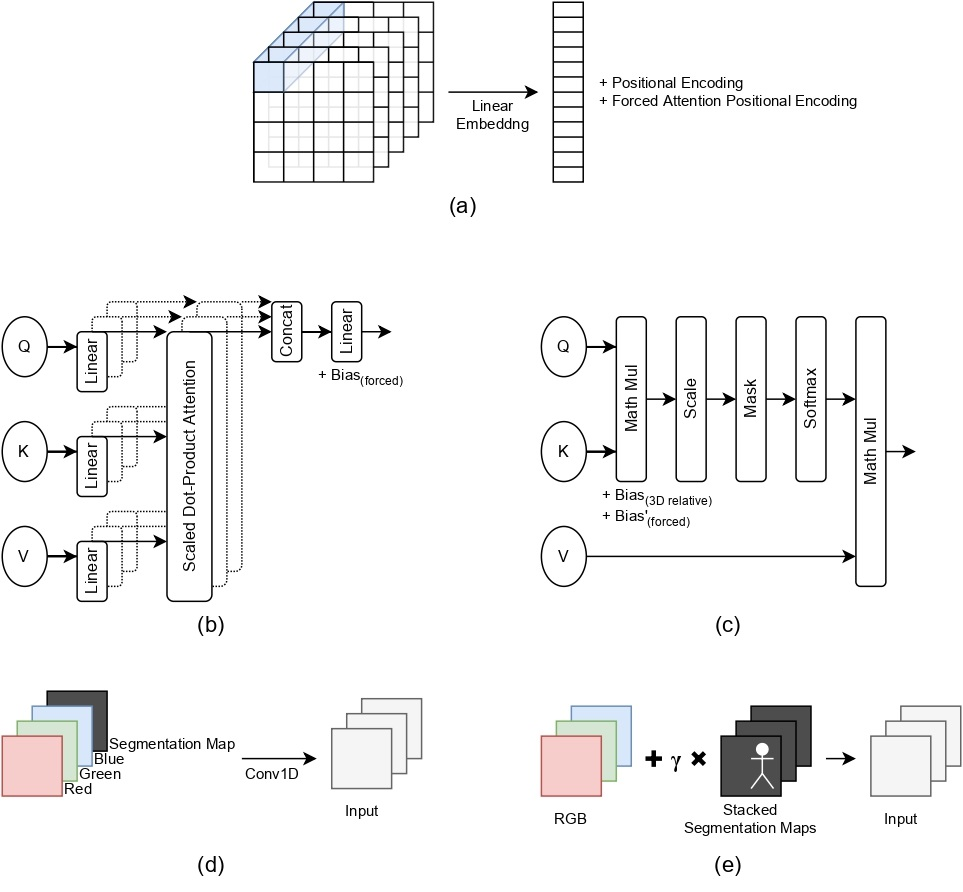
Multiple input modalities have their own branches for processing before they are combined together using cross-attention. As shown in Figure 25 and in Figure 26, there are sequential cross-attention layers with full frame sequence and audio and transcript.
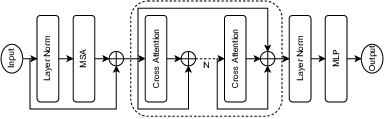
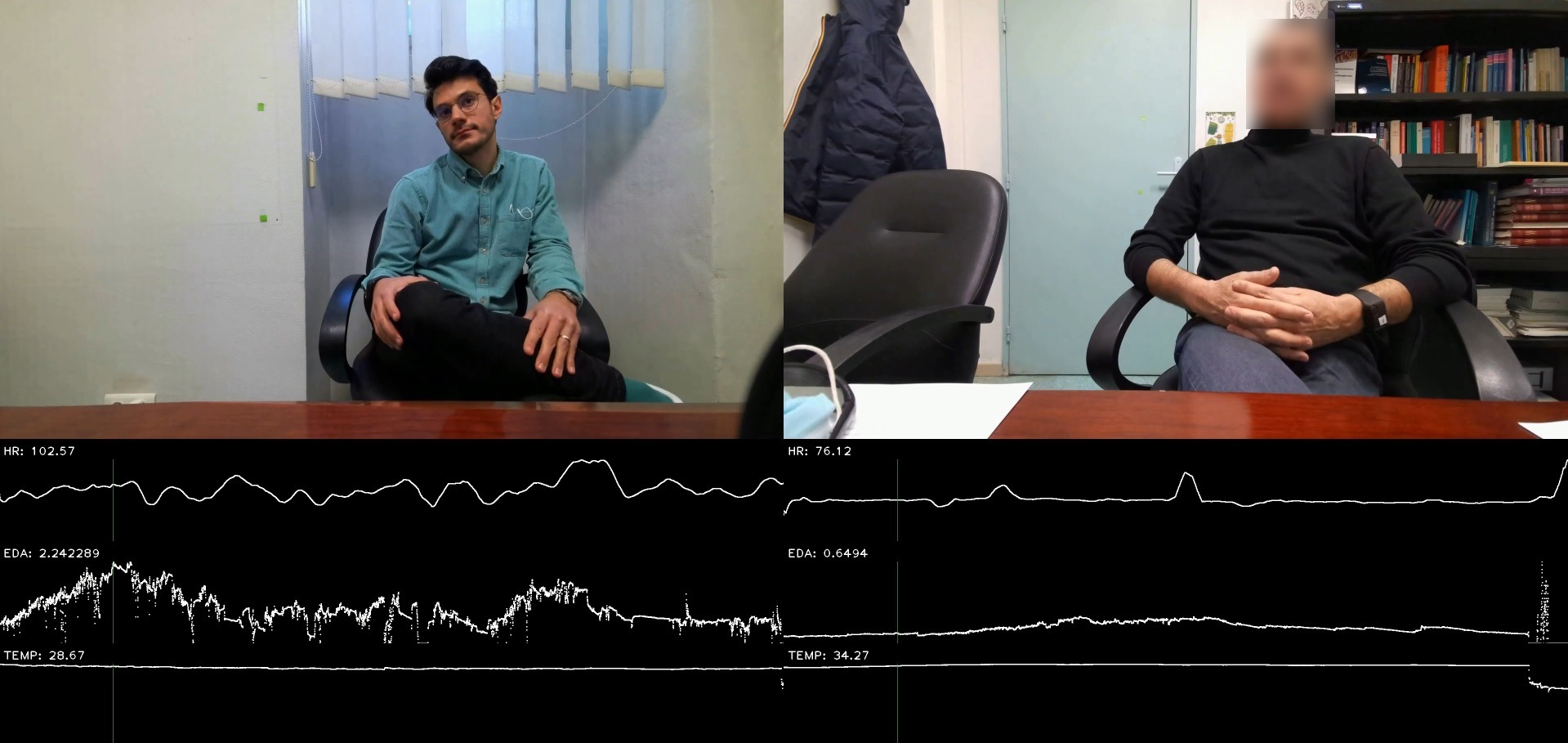
FAt Transformer is practically a model for a generalized feature extraction for tasks concerning social signals and behavior analysis. Our focus is on understanding behavior in videos where people are interacting with each other or talking into the camera which simulates the first person point of view in social interaction. FAt Transformers are applied to two downstream tasks: personality recognition and body language recognition. We achieve state-of-the-art results for Udiva v0.5, First Impressions v2 and MPIIGroupInteraction datasets. We further provide an extensive ablation study of the proposed architecture.
7.24 StressID: a Multimodal Dataset for Stress Identification
Participants: Valeriya Strizhkova, Aglind Reka, François Brémond, Laura M. Ferrari.
StressID 22 is a new dataset, which is specifically designed for stress identification based on multimodal data collection. It contains facial expression video, audio and physiological signal recordings. As shown in Figure 27, the video and audio recordings are acquired using an RGB camera with an integrated microphone. The physiological data are composed of electrocardiogram (ECG), electrodermal activity (EDA) and respiration signals that are monitored using a wearable recording device. This experimental set-up ensures synchronized, high-quality and low noise multimodal data collection. Different stress-inducing stimuli such as emotional video-clips, cognitive tasks including mathematical or comprehension exercises, and public speaking scenarios are designed to trigger a diverse range of emotional responses. The total dataset consists of recordings from 65 participants that performed 11 tasks as well as their ratings of perceived relaxation, stress, arousal and valence levels. This is the largest dataset for stress identification with three different sources of data and classes of stimuli together with more than 21 hours of annotated data. offers baseline models for stress classification for each modality. It includes a cleaning, feature extraction, feature selection and classification phase enabling a multimodal predictive model based on video, audio and physiological inputs. The data and the code for the baselines are available here.
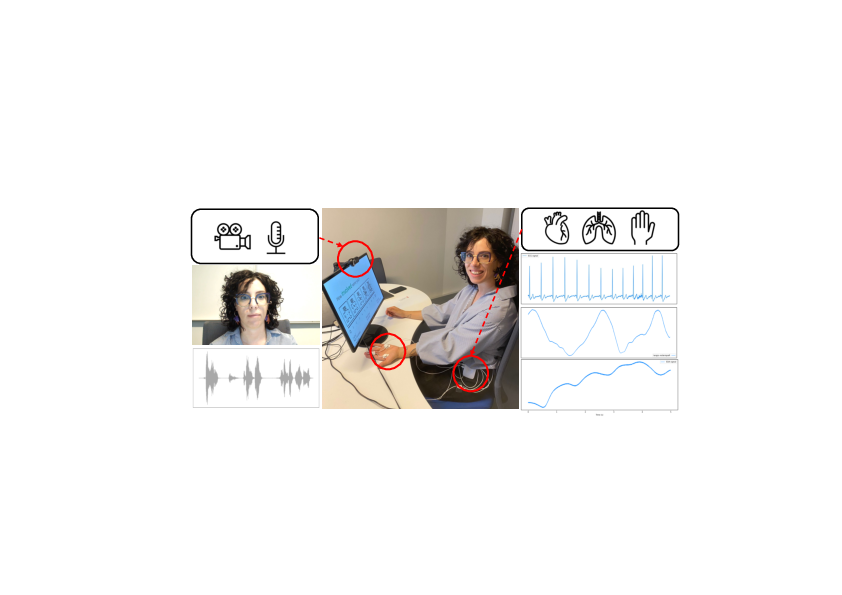
8 Bilateral contracts and grants with industry
Participants: Antitza Dantcheva, Francois Bremond.
Stars team has currently several experiences in technological transfer towards industries, which have permitted to exploit research result.
8.1 Bilateral contracts with industry
8.1.1 Toyota
Toyota is working with Stars on action recognition software to be integrated on their robot platform. This project aims at detecting critical situations in the daily life of older adults alone at home. This will require not only recognition of ADLs but also an evaluation of the way and timing in which they are being carried out. The system we want to develop is intended to help them and their relatives to feel more comfortable because they know that potentially dangerous situations will be detected and reported to caregivers if necessary. The system is intended to work with a Partner Robot - HSR - (to send real-time information to the robot) to better interact with the older adult.
8.1.2 Thales
Thales and Inria jointly explore facial analysis in the invisible spectrum. Among the different spectra low energy infrared waves, as well as ultraviolet waves will be studied. In this context following tasks will be included: 1. We are designing a model to extract biometric features from the acquired data. Analysis of the data related to contours, shape, etc. will be performed. Current methodology cannot be adopted, since colorimetry in the invisible spectrum is more restricted with less diffuse variations and is less nuanced. Then facial recognition will be performed in the invisible spectrum. Expected challenges have to do with limited colorimetry and lower contrasts. In addition to the first milestone (face recognition in the invisible spectrum), there are two other major milestones: 2. Implementation of such a face recognition system, to be tested at the passage of the access portal to a school. 3. Pseudo-anonymized identification within a school (outdoor courtyards, interior buildings). Combining biometrics in the invisible spectra and anonymization within an established group requires removing certain additional barriers that are specific to biometrics but also the use of statistical methods associated with biometrics. This pseudo-anonymized identification must also incorporate elements of information provided by the proposed electronic school IDs.
8.1.3 Fantastic Sourcing
Fantastic Sourcing is a French SME specialized in micro-electronics, it develops e-health technologies. Fantastic Sourcing is collaborating with Stars through the UniCA Solitaria project, by providing their Nodeus system. Nodeus is an IoT (Internet of Things) system for home support for the elderly, which consists of a set of small sensors (without video cameras) to collect precious data on the habits of isolated people. Solitaria project performs a multi-sensor activity analysis for monitoring and safety of older and isolated people. With the increase of the ageing population in Europe and in the rest of the world, keeping elderly people at home, in their usual environment, as long as possible, becomes a priority and a challenge of modern society. A system for monitoring activities and alerting in case of danger, in permanent connection with a device (an application on a phone, a surveillance system ...) to warn relatives (family, neighbors, friends ...) of isolated people still living in their natural environment could save lives and avoid incidents that cause or worsen the loss of autonomy. In this RD project, we propose to study a solution allowing the use of a set of innovative heterogeneous sensors in order to: 1) detect emergencies (falls, crises, etc.) and call relatives (neighbors, family, etc.); 2) detect, over short or longer predefined.
8.1.4 Nively - WITA SRL
Nively is a French SME specialized in e-health technologies, it develops position and activity monitoring of activities of daily living platforms based on video technology. Nively’s mission is to use technological tools to put people back at the center of their interests, with their emotions, identity and behavior. Nively is collaborating with Stars through the UniCA Solitaria project, by providing their MentorAge system. This software allows the monitoring of elderly people in nursing homes in order to detect all the abnormal events in the lives of residents (falls, runaways, strolls, etc.). Nively’s technology is based on RGBD video sensors (Kinects type) and a software platform for event detection and data visualization. Nively is also in charge of Software distribution for the ANR Activis project. This project is based on an objective quantification of the atypical behaviors on which the diagnosis of autism is based, with medical (diagnostic assistance and evaluation of therapeutic programs) and computer scientific (by allowing a more objective description of atypical behaviors in autism) objectives. This quantification requires video analysis of the behavior of people with autism. In particular, we propose to explore the issues related to the analysis of ocular movement, gestures and posture to characterize the behavior of a child with autism. Thus, Nively will add autistic behavior analysis software to its product range.
8.2 Bilateral grants with industry
8.2.1 LiChIE Project
The LiChIE project (Lion Chaine Image Elargie) is conducted in collaboration with AirBus and BPI to found nine topics including six on the theme of In-flight imagery and three on the robotics theme for the assembly of satellites. The two topics involving STARS are:
- Mohammed Guermal's PhD thesis on Visual Understanding of Activities for an improved collaboration between humans and robots. He began on December 1, 2020.
- Farhood Negin post-doctoral studies on detection and tracking of vehicles from satellite videos and abnormal activity detection. He started in Oct 2020 for 2 years.
-
Toyota: (Action Recognition System):
This project runs from the 1st of August 2013 up to December 2025. It aims at detecting critical situations in the daily life of older adults living home alone. The system is intended to work with a Partner Robot (to send real-time information to the robot for assisted living) to better interact with older adults. The funding was 106 Keuros for the 1st period and more for the following years.
9 Partnerships and cooperations
9.1 International initiatives
Participants: Antitza Dantcheva, Francois Bremond.
9.1.1 Inria associate team not involved in an IIL or an international program
GDD
-
Title:
Generalizable Deepfake Detection
-
Duration:
2022 -> 2024
-
Coordinator:
Abhijit Das (abhijit.das@thapar.edu)
-
Partners:
- Thapar University, Bhadson Rd, Adharse colony, Prem nagar, Punjab- 147004 (Inde)
-
Inria contact:
Antitza Dantcheva
-
Summary:
In this project we will focus on Manipulated facial videos (deepfakes) which have become highly realistic due to the tremendous progress of deep convolutional neural networks (CNNs). While intriguing, such progress raises a number of social concerns related to fake news. We propose in GDD the design of deepfake detection algorithms, which can generalize in order to detect unknown manipulations.
9.2 International research visitors
9.2.1 Visits of international scientists
- Prof. Arun Ross from the Michigan State University, USA, April 2023.
- Prof. Abhijit Das from BITS Pilani Hyderabad, India, September 2023.
- Prof. Christoph Busch from Hochschule Darmstadt, Germany, December 2023.
- Professor Hajime NAGAHARA from Osaka University, Japan, September 2023
- Kanjar De, ERCIM Research Exchange Visit, Fraunhofer-Institut für Nachrichtentechnik, Heinrich-Hertz-Institut, Germany, October 2023.
9.2.2 Visits to international teams
Antitza Dantcheva spent one week visiting the Institute for Computer Science, Artificial Intelligence and Technology (website of INSAIT) in Sofia, Bulgaria.
9.3 European initiatives
9.3.1 Horizon Europe
GAIN
GAIN project on cordis.europa.eu
-
Title:
Georgian Artificial Intelligence Networking and Twinning Initiative
-
Duration:
From October 1, 2022 to September 30, 2025
-
Partners:
- Institut National De Recherche En Informatique Et Automatique (Inria), France
- Exolaunch Gmbh (EXO), Germany
- Deutsches Forschungszentrum Fur Kunstliche Intelligenz Gmbh (DFKI), Germany
- Georgian Technical University (GTU), Georgia
-
Inria contact:
François Bremond
-
Coordinator:
George Giorgobiani
-
Summary:
GAIN will take a strategic step towards integrating Georgia, one of the Widening countries, into the system of European efforts aimed at ensuring the Europe’s leadership in one of the most transformative technologies of today and tomorrow – Artificial Intelligence (AI). It will be achieved by research profile adjusting and linking the central Georgian ICT research institute - Muskhelishvili Institute of Computational Mathematics (MICM), to the European AI research and innovation community. Two absolutely leading European research organizations (DFKI and INRIA) supported by the high-tech company EXOLAUNCH will support MICM in this endeavour. The Strategic Research and Innovation Programme (SRIP) designed by the partnership will provide the environment for the Georgian colleagues to get involved in the research projects of the European partners addressing a clearly delineated set of AI topics. Jointly, the partners will advance in capacity building and networking within the area of AI Methods and Tools for Human Activities Recognition and Evaluation, which also will contribute to strengthening core competences in such fundamental technologies as e.g. Machine (Deep) Learning. The results of the cooperation presented through the series of scientific publications and events will inform the European AI community about the potential of MICM and trigger new partnerships building, addressing e.g. Horizon Europe. The project will contribute to career development of a cohort of young researchers at MICM through joint supervision and targeted capacity building measures. Innovation and Research Administration and Management capacities of MICM will also be strengthened to allow the Institute to be better connected to the local, regional and European innovation activities. Using their extensive research and innovation networking capacities DFKI and INRIA will introduce MICM to the European AI research community by connecting to such networks as CLAIRE, ELLIS, ADRA, AI NoEs, etc.
9.3.2 H2020 projects
HEROES
HEROES project on cordis.europa.eu
-
Title:
Novel Strategies to Fight Child Sexual Exploitation and Human Trafficking Crimes and Protect their Victims
-
Duration:
From December 1, 2021 to November 30, 2024
-
Partners:
- institut national de recherche en informatique et automatique (inria), France
- Policia Federal, Brazil
- Elliniko symvoulio gai tous prosfyges (Greek council for refugees), Greece
- International Centre For Migration Policy Development (ICMPD), Austria
- Universidade Estadual De Campinas (UNICAMP), Brazil
- Associacao Brasileira De Defesa Da Muhler Da Infancia E Da Juventude (ASBRAD), Brazil
- Kovos Su Prekyba Zmonemis Ir Isnaudojimu Centras Vsi (KOPZI), Lithuania
- Fundacion Renacer, Colombia
- Trilateral Research Limited (TRI IE), Ireland
- Vrije Universiteit Brussel (VUB), Belgium
- Athina-Erevnitiko Kentro Kainotomias Stis Technologies Tis Pliroforias, Ton Epikoinonion Kai Tis Gnosis (Athena - Research And Innovation Center), Greece
- The Global Initiative Verein Gegen Transationale Organisierte Kriminalitat, Austria
- Esphera - Cultural, Ambiental E Social, Brazil
- Fundacao Universidade De Brasilia (Universidade De Brasília), Brazil
- Idener Research & Development Agrupacion De Interes Economico (Idener Research & Development Aie), Spain
- Universidad Complutense De Madrid (UCM), Spain
- University Of Kent (UNIKENT), United Kingdom
- Kentro Meleton Asfaleias (CENTER FORSECURITY STUDIES CENTRE D'ETUDES DE SECURITE), Greece
- Trilateral Research LTD, United Kingdom
- Policia Rodoviaria Federal (Federa Highway Police), Brazil
- Ministerio Del Interior (ESMIR), Spain
- Iekslietu Ministrijas Valsts Policija State Police Of The Ministry Of Interior (State Police Of Latvia), Latvia
- Secretaria De Inteligencia Estrategica De Estado - Presidencia De La Republica Oriental Del Uruguay (SIEE), Uruguay
- Associacao Portuguesa De Apoio A Vitima, Portugal
- Comando Conjunto De Las Fuerzas Armadas Del Peru (Comando Conjunto De Las Fuerzas Armadas Del Peru), Peru
- International Center For Missing And Exploited Children Switzerland, Switzerland
- Hellenic Police (Hellenic Police), Greece
- Centre For Women And Children Studies (CWCS), Bangladesh
- Glavna Direktsia Borba S Organiziranata Prestupnost (Chief Directorate Fight With Organised Crime), Bulgaria
-
Inria contact:
François Bremond
-
Coordinator:
Esteban Alejandro Armas Vega
-
Summary:
Trafficking of human beings (THB) and child sexual abuse and exploitation (CSA/CSE) are two big problems in our society. Inadvertently, new information and communication technologies (ICTs) have provided a space for these problems to develop and take new forms, made worse by the lockdown caused by the COVID-19 pandemic. At the same time, technical and legal tools available to stakeholders that prevent, investigate, and assist victims – such as law enforcement agencies (LEAs), prosecutors, judges, and civil society organizations (CSOs) – fail to keep up with the pace at which criminals use new technologies to continue their abhorrent acts. Furthermore, assistance to victims of THB and CSA/CSE is often limited by the lack of coordination among these stakeholders. In this sense, there is a clear and vital need for joint work methodologies and the development of new strategies for approaching and assisting victims. In addition, due to the cross-border nature of these crimes, harmonization of legal frameworks from each of the affected countries is necessary for creating bridges of communication and coordination among all those stakeholders to help victims and reduce the occurrence of these horrendous crimes. To address these challenges, the HEROES project comes up with an ambitious, interdisciplinary, international, and victim-centred approach. The HEROES project is structured as a comprehensive solution that encompasses three main components: Prevention, Investigation and Victim Assistance. Through these components, our solution aims to establish a coordinated contribution with LEAs by developing an appropriate, victim-centred approach that is capable of addressing specific needs and providing protection. The HEROES project’s main objective is to use technology to improve the way in which help and support can be provided to victims of THB and CSA/CSE.
9.4 National initiatives
3IA
-
Title:
Video Analytics for Human Behavior Understanding (axis 2),
-
Duration:
From 2019
-
Chair holder:
François Brémond
-
Summary:
The goal of this chair is to design novel modern AI methods (including Computer Vision and Deep Learning algorithms) to build real-time systems for improving health and well-being as well as people safety, security and privacy. Behavior disorders affect the mental health of a growing number of people and are hard to handle, leading to a high cost in our modern society. New AI techniques can enable a more objective and earlier diagnosis, by quantifying the level of disorders and by monitoring the evolution of the disorders. AI techniques can also learn the relationships between the symptoms and their true causes, which are often hard to identify and measure.
RESPECT
-
Title:
Reliable, secure and privacy preserving multi-biometric person authentication
-
Duration:
From 2018 to 2023
-
Partners:
Inria, Hochschule Darmstadt, EURECOM.
-
Inria contact:
Antitza Dantcheva
-
Coordinator:
Hochschule Darmstadt
-
Summary:
In spite of the numerous advantages of biometric recognition systems over traditional authentication systems based on PINs or passwords, these systems are vulnerable to external attacks and can leak data. Presentations attacks (PAs) – impostors who manipulate biometric samples to masquerade as other people – pose serious threats to security. Privacy concerns involve the use of personal and sensitive biometric information, as classified by the GDPR, for purposes other than those intended. Multi-biometric systems, explored extensively as a means of improving recognition reliability, also offer potential to improve PA detection (PAD) generalization. Multi-biometric systems offer natural protection against spoofing since an impostor is less likely to succeed in fooling multiple systems simultaneously. For the same reason, previously unseen PAs are less likely to fool multi-biometric systems protected by PAD. RESPECT, a Franco-German collaborative project, explores the potential of using multi-biometrics as a means to defend against diverse PAs and improve generalization while still preserving privacy. Central to this idea is the use of (i) biometric characteristics that can be captured easily and reliably using ubiquitous smart devices and, (ii) biometric characteristics which facilitate computationally manageable privacy preserving, homomorphic encryption. The research focuses on characteristics readily captured with consumer-grade microphones and video cameras, specifically face, iris and voice. Further advances beyond the current state of the art involve the consideration of dynamic characteristics, namely utterance verification and lip dynamics. The core research objective is to determine which combination of biometrics characteristics gives the best biometric authentication reliability and PAD generalization while remaining compatible with computationally efficient privacy preserving biometric template protection schemes.
ACTIVIS
-
Title:
ACTIVIS: Video-based analysis of autism behavior
-
Duration:
From 2020 - 2025
-
Partners:
Inria, Aix-Marseille Université - LIS, Hôpitaux Pédiatriques Nice CHU-Lenval - CoBTeK, Nively
-
Inria contact:
François Brémond
-
Coordinator:
Aix-Marseille Université - LIS
-
Summary:
The ACTIVIS project is an ANR project (CES19: Technologies pour la santé) started in January 2020 and will end in December 2023 (48 months). This project is based on an objective quantification of the atypical behaviors on which the diagnosis of autism is based, with medical (diagnostic assistance and evaluation of therapeutic programs) and computer scientific (by allowing a more objective description of atypical behaviors in autism) objectives. This quantification requires video analysis of the behavior of people with autism. In particular, we propose to explore the issues related to the analysis of ocular movement, gestures and posture to characterize the behavior of a child with autism.
10 Dissemination
Participants: Antitza Dantcheva, Francois Bremond.
10.1 Promoting scientific activities
10.1.1 Scientific events: organisation
Antitza Dantcheva gave a joint tutorial with Abhijit Das at the IEEE International Joint Conference on Biometrics (IJCB 2023), website of IJCB.
10.1.2 Scientific events: selection
Chair of conference program committees
Antitza Dantcheva was
- Publication Chair at the International Conference on Automatic Face and Gesture Recognition (FG) 2023
- Tutorial Chair at the International Joint Conference on Biometrics (IJCB) 2023.
- Session chair at the International Conference of the Biometrics Special Interest Group (BIOSIG) 2023.
Member of the conference program committees
Monique Thonnat was member of conference program committee ICPRAM 2024 (International Conference on Pattern Recognition Applications and Methods).
François Brémond was a member of the ANR Scientific Evaluation Committee - CES 23 "Artificial Intelligence and Data Science" on December 2023.
François Brémond was a member of the ANR WISG 2024 "Workshop interdisciplinaire sur la sécurité globale" on November 2023.
Reviewer
François Brémond was reviewer in the major Computer Vision / Machine Learning conferences including ICCV (International Conference on Computer Vision), ECCV (European Conference on Computer Vision), WACV (Winter Conference on Applications of Computer Vision), CVPR (Computer Vision and Pattern Recognition), NeurIPS (Neural Information Processing Systems).
10.1.3 Journal
Member of the editorial boards
Antitza Dantcheva serves in the editorial board of the journals Pattern Recognition (PR), Transactions on Multimedia (TMM), as well as Multimedia Tools and Applications (MTAP).
10.1.4 Invited talks
Antitza Dantcheva was invited to give a talk entitled "Generating and detecting deepfakes" at INSAIT, Sofia, Bulgaria in August 2023.
François Brémond was invited to give a talk entitled "recent work involving infant video data" at WACV tutorial on privacy, Hawaii in January 2024.
Monique Thonnat gave an invited talk on “Aide au diagnostic de troubles cognitifs par analyse video: le cas de crises d'épilepsie - A Self-supervised Pre-training Framework for Vision-based Seizure Classification” at JSI, Journées Scientifiques Inria in Bordeaux, 30 August -1 September 2023.
10.2 Teaching - Supervision - Juries
10.2.1 Teaching
- François Brémond organized and lectured AI courses on Computer Vision & Deep Learning for the Data Science and AI - MSc program at Université Côte d'Azur: 30h class at Université Côte d'Azur in 2023. Web-site
- François Brémond lectured an AI course on Computer Vision & Environment at the Third Inria-DFKI European Summer School on AI (IDESSAI 2023), in coordination with 3IA Côte d'Azur, Sophia, September 2023.
- François Brémond lectured an AI course on Computer Vision at the Inria Academy : "journée IA pour MINARME", CampusCyber, Paris, September 2023.
- Antitza Dantcheva taught 2 classes at Polytech Nice Sophia - Univ Côte d'Azur (Applied Artificial Intelligence, Master 2).
- Snehashis Majhi taught two lectures for MSc. Data Science and Artificial Intelligence, UniCA.
- Tomasz Stanczyk taught one lecture for MSc. Data Science and Artificial Intelligence, UniCA.
- Valeriya Strizhkova taught one lecture for MSc. Data Science and Artificial Intelligence, UniCA and one research project for DSAI.
- Baptiste Chopin taught one lecture for the course Applied Artificial Intelligence, Master 2, Polytech Nice-Sophia, September 2022.
10.2.2 Supervision
François Brémond has (co)-supervised 8 PhD students and 6 master students.
Antitza Dantcheva has (co)-supervised 3 PhD students and 2 masters students.
10.2.3 Juries
Monique Thonnat was
- HDR committee chair : Guillaume SACCO HDR Université Côte d'Azur, Faculté de Médecine on 12 of April 2023.
- PhD committee chair : PhD of Arthur FOAHOM GOUABOU University Aix Marseille, on 6 of March 2023.
Antitza Dantcheva was
- in the Ph.D. committee (reviewer) of Baptiste Chopin (CRISTAL, University of LIRIS, Lille), March 2023.
- in the Ph.D. committee (examiner) of Romain Cazorla (École nationale d'ingénieurs de Brest, France), June 2023.
- in the Ph.D. committee (examiner) of Gauthier Tallec (Sorbonne University, France), July 2023.
- in the Ph.D. committee (examiner) of Rottana Ly (Université Grenoble Alpes, France), November 2023.
- in the CS (Scientific Committee) of Mehdi Atamna (Laboratory LIRIS, Lyon, France), May 2023.
- in the CS of Sahar Husseini (Eurecom, France), December 2023.
François Brémond was
- HDR committee examiner : Bertrand Luvison, HDR, Sorbonne Université, on 4th of April 2023.
- Professor committee member : Dr Suresh Sundaram, Department of Aerospace Engineering at the Indian Institute of Science, Bangalore, Karnakata, INDIA on 24th of August 2023.
- PhD committee (examiner) : PhD of David Pagnon, University Grenoble, on 10th of March 2023.
- PhD committee (chair) : PhD of Devashish Lohani, University Lyon, on 3rd of April 2023.
- PhD committee (examiner) : PhD of Gnana Praveen Rajasekhar, École de technologie supérieure, Université du Québec, Canada, on 22th May 2023.
- PhD committee (examiner) : PhD of Rupayan Mallick, University Bordeaux/LaBRI, on 20th of October 2023.
- PhD committee (chair) : PhD of Tony Marteau University Lille, on 25th of November 2023.
- CS (Scientific Committee) of Marc Chapus (Laboratory LIRIS, Lyon), 15th of May 2023.
- CS of Fabien Lionti, Université Côte d'Azur, 5th of May 2023.
- CS of Franz Franco Gallo, Université Côte d'Azur, 1st of June 2023.
- CS of Yannick Porto, University Bourgogne Franche Comté, September 2023.
10.3 Popularization
François Brémond was invited to participate to the radio show on « vidéosurveillance algorithmique » at France Culture, "Le Temps du débat" on the 24th March 2023.
François Brémond was invited by CNIL to participate to a workshop to evaluate video-surveillance algorithms related to the 2024 Olympic and Paralympic Games bill, on the 24th of April 2023.
François Brémond was invited to participate to the radio show on "privacy and video-surveillance" at France Culture / La science, CQFD on the 29th November 2023.
François Brémond was invited to participate to a workshop on "Video Action Recognition for Human Behavior Analysis" at conseil de l'âge on the 8th of November 2023.
François Brémond was interviewed on "Automated video surveillance" by Journalist Ariane Lavrilleux from Collectif Presse-Papiers Marseille in November 2023.
François Brémond was interviewed on "Automated video surveillance" by Journalist Thomas Allard from "Magazine Science et Vie" Journal in September 2023.
11 Scientific production
11.1 Major publications
- 1 inproceedingsLearning to match appearances by correlations in a covariance metric space.European Conference on Computer VisionSpringer2012, 806--820
- 2 articleExploiting Feature Correlations by Brownian Statistics for People Detection and Recognition.IEEE transactions on systems, man, and cybernetics2016HAL
- 3 inproceedingsVideo Covariance Matrix Logarithm for Human Action Recognition in Videos.IJCAI 2015 - 24th International Joint Conference on Artificial Intelligence (IJCAI)Buenos Aires, ArgentinaJuly 2015HAL
- 4 articleSemantic Event Fusion of Different Visual Modality Concepts for Activity Recognition.IEEE Transactions on Pattern Analysis and Machine Intelligence382016, 1598 - 1611HALDOI
- 5 articleGender estimation based on smile-dynamics.IEEE Transactions on Information Forensics and Security2016, 11HALDOI
- 6 inproceedingsToyota Smarthome: Real-World Activities of Daily Living.ICCV 2019 -17th International Conference on Computer VisionSeoul, South KoreaOctober 2019HAL
- 7 inproceedingsVPN: Learning Video-Pose Embedding for Activities of Daily Living.ECCV 2020 - 16th European Conference on Computer VisionGlasgow (Virtual), United KingdomAugust 2020HAL
- 8 inproceedingsGesture Recognition by Learning Local Motion Signatures.CVPR 2010 : IEEE Conference on Computer Vision and Pattern RecognitionSan Franscico, CA, United StatesIEEE Computer Society PressJune 2010HAL
- 9 articleRecognizing Gestures by Learning Local Motion Signatures of HOG Descriptors.IEEE Transactions on Pattern Analysis and Machine Intelligence2012HAL
- 10 inproceedingsKnowledge Representation for Program Reuse.European Conference on Artificial Intelligence (ECAI)Lyon, FranceJuly 2002, 240-244
- 11 articleBlocks, a Component Framework with Checking Facilities for Knowledge-Based Systems.Informatica, Special Issue on Component Based Software Development254November 2001, 501-507
- 12 articleCompilation Modulaire d'un Langage Synchrone.Revue des sciences et technologies de l'information, série Théorie et Science Informatique430June 2011, 441-471URL: http://hal.inria.fr/inria-00524499/en
- 13 article What Can Program Supervision Do for Software Re-use? IEE Proceedings - Software Special Issue on Knowledge Modelling for Software Components Reuse 147 5 2000
- 14 inproceedingsAutomatic Video Interpretation: A Novel Algorithm based for Temporal Scenario Recognition.The Eighteenth International Joint Conference on Artificial Intelligence (IJCAI'03)Acapulco, Mexico9-15 September 2003
- 15 inproceedingsG3AN: Disentangling Appearance and Motion for Video Generation.CVPR 2020 - IEEE Conference on Computer Vision and Pattern RecognitionSeattle / Virtual, United StatesJune 2020HAL
11.2 Publications of the year
International journals
Invited conferences
International peer-reviewed conferences
Scientific book chapters
Doctoral dissertations and habilitation theses
Reports & preprints