2023Activity reportProject-TeamLINKMEDIA
RNSR: 201421145C- Research center Inria Centre at Rennes University
- In partnership with:Institut national des sciences appliquées de Rennes, CNRS, Université de Rennes
- Team name: Creating and exploiting explicit links between multimedia fragments
- In collaboration with:Institut de recherche en informatique et systèmes aléatoires (IRISA)
- Domain:Perception, Cognition and Interaction
- Theme:Vision, perception and multimedia interpretation
Keywords
Computer Science and Digital Science
- A3.3.2. Data mining
- A3.3.3. Big data analysis
- A3.4. Machine learning and statistics
- A3.4.1. Supervised learning
- A3.4.2. Unsupervised learning
- A3.4.8. Deep learning
- A4. Security and privacy
- A5.3.3. Pattern recognition
- A5.4.1. Object recognition
- A5.4.3. Content retrieval
- A5.7. Audio modeling and processing
- A5.7.1. Sound
- A5.7.3. Speech
- A5.8. Natural language processing
- A9.2. Machine learning
- A9.3. Signal analysis
- A9.4. Natural language processing
Other Research Topics and Application Domains
- B9. Society and Knowledge
- B9.3. Medias
- B9.6.10. Digital humanities
- B9.10. Privacy
1 Team members, visitors, external collaborators
Research Scientists
- Laurent Amsaleg [Team leader, CNRS, Senior Researcher, HDR]
- Vincent Claveau [CNRS, Researcher, until Mar 2023, HDR]
- Teddy Furon [INRIA, Senior Researcher, HDR]
- Guillaume Gravier [CNRS, Senior Researcher, HDR]
- Kassem Kallas [INRIA, Starting Research Position, until Nov 2023]
Faculty Members
- Ewa Kijak [UNIV RENNES, Associate Professor, HDR]
- Simon Malinowski [UNIV RENNES, Associate Professor]
- Pascale Sébillot [INSA RENNES, Professor, HDR]
Post-Doctoral Fellows
- Eva Giboulot [INRIA, Post-Doctoral Fellow, from Sep 2023]
- Gauthier Lyan [CNRS, Post-Doctoral Fellow, until Jun 2023]
- Ryan Webster [INRIA, Post-Doctoral Fellow, from Dec 2023]
PhD Students
- Benoit Bonnet [INRIA, until Jan 2023]
- Antoine Chaffin [IMATAG, until Oct 2023]
- Deniz Engin [INRIA]
- Gautier Evennou [IMATAG, CIFRE, from Sep 2023]
- Pierre Fernandez [FACEBOOK, CIFRE]
- Louis Hemadou [SAFRAN, CIFRE]
- Carolina Jeronimo De Almeida [GOUV BRESIL, from Sep 2023]
- Victor Klotzer [INRIA, until Jun 2023]
- Quentin Le Roux [THALES, CIFRE]
- Thibault Maho [INRIA, until Nov 2023]
- Duc Hau Nguyen [CNRS, until Nov 2023]
- Samuel Tap [ZAMA, until Nov 2023]
- Hugo Thomas [UNIV RENNES]
- Karim Tit [THALES]
- Shashanka Venkataramanan [INRIA]
Technical Staff
- Benoit Bonnet [INRIA, Engineer, from Feb 2023 until Jul 2023]
- Morgane Casanova [CNRS, Engineer, from May 2023]
- Maxence Despres [INRIA, Engineer, until Jan 2023]
- Nicolas Fouque [CNRS, Engineer, until Nov 2023]
- Guillaume Le Noé-Bienvenu [CNRS, Engineer, until Aug 2023]
Administrative Assistant
- Aurélie Patier [UNIV RENNES]
Visiting Scientist
- Carolina Jeronimo De Almeida [GOUV BRESIL, until Aug 2023]
2 Overall objectives
2.1 Context
Linkmedia is concerned with the processing of extremely large collections of multimedia material. The material we refer to are collections of documents that are created by humans and intended for humans. It is material that is typically created by media players such as TV channels, radios, newspapers, archivists (BBC, INA, ...), as well as the multimedia material that goes through social-networks. It has images, videos and pathology reports for e-health applications, or that is in relation with e-learning which typically includes a fair amount of texts, graphics, images and videos associating in new ways teachers and students. It also includes material in relation with humanities that study societies through the multimedia material that has been produced across the centuries, from early books and paintings to the latest digitally native multimedia artifacts. Some other multimedia material are out of the scope of Linkmedia, such as the ones created by cameras or sensors in the broad areas of video-surveillance or satellite images.
Multimedia collections are rich in contents and potential, that richness being in part within the documents themselves, in part within the relationships between the documents, in part within what humans can discover and understand from the collections before materializing its potential into new applications, new services, new societal discoveries, ... That richness, however, remains today hardly accessible due to the conjunction of several factors originating from the inherent nature of the collections, the complexity of bridging the semantic gap or the current practices and the (limited) technology:
- Multimodal: multimedia collections are composed of very diverse material (images, texts, videos, audio, ...), which require sophisticated approaches at analysis time. Scientific contributions from past decades mostly focused on analyzing each media in isolation one from the other, using modality-specific algorithms. However, revealing the full richness of collections calls for jointly taking into account these multiple modalities, as they are obviously semantically connected. Furthermore, involving resources that are external to collections, such as knowledge bases, can only improve gaining insight into the collections. Knowledge bases form, in a way, another type of modality with specific characteristics that also need to be part of the analysis of media collections. Note that determining what a document is about possibly mobilizes a lot of resources, and this is especially costly and time consuming for audio and video. Multimodality is a great source of richness, but causes major difficulties for the algorithms running analysis;
- Intertwined: documents do not exist in isolation one from the other. There is more knowledge in a collection than carried by the sum of its individual documents and the relationships between documents also carry a lot of meaningful information. (Hyper)Links are a good support for materializing the relationships between documents, between parts of documents, and having analytic processes creating them automatically is challenging. Creating semantically rich typed links, linking elements at very different granularities is very hard to achieve. Furthermore, in addition to being disconnected, there is often no strong structure into each document, which makes even more difficult their analysis;
- Collections are very large: the scale of collections challenges any algorithm that runs analysis tasks, increasing the duration of the analysis processes, impacting quality as more irrelevant multimedia material gets in the way of relevant ones. Overall, scale challenges the complexity of algorithms as well as the quality of the result they produce;
- Hard to visualize: It is very difficult to facilitate humans getting insight on collections of multimedia documents because we hardly know how to display them due to their multimodal nature, or due to their number. We also do not know how to well present the complex relationships linking documents together: granularity matters here, as full documents can be linked with small parts from others. Furthermore, visualizing time-varying relationships is not straightforward. Data visualization for multimedia collections remains quite unexplored.
2.2 Scientific objectives
The ambition of Linkmedia is to propose foundations, methods, techniques and tools to help humans make sense of extremely large collections of multimedia material. Getting useful insight from multimedia is only possible if tools and users interact tightly. Accountability of the analysis processes is paramount in order to allow users understanding their outcome, to understand why some multimedia material was classified this way, why two fragments of documents are now linked. It is key for the acceptance of these tools, or for correcting errors that will exist. Interactions with users, facilitating analytics processes, taking into account the trust in the information and the possible adversarial behaviors are topics Linkmedia addresses.
3 Research program
3.1 Scientific background
Linkmedia is de facto a multidisciplinary research team in order to gather the multiple skills needed to enable humans to gain insight into extremely large collections of multimedia material. It is multimedia data which is at the core of the team and which drives the design of our scientific contributions, backed-up with solid experimental validations. Multimedia data, again, is the rationale for selecting problems, applicative fields and partners.
Our activities therefore include studying the following scientific fields:
- multimedia: content-based analysis; multimodal processing and fusion; multimedia applications;
- computer vision: compact description of images; object and event detection;
- machine learning: deep architectures; structured learning; adversarial learning;
- natural language processing: topic segmentation; information extraction;
- information retrieval: high-dimensional indexing; approximate k-nn search; embeddings;
- data mining: time series mining; knowledge extraction.
3.2 Workplan
Overall, Linkmedia follows two main directions of research that are (i) extracting and representing information from the documents in collections, from the relationships between the documents and from what user build from these documents, and (ii) facilitating the access to documents and to the information that has been elaborated from their processing.
3.3 Research Direction 1: Extracting and Representing Information
Linkmedia follows several research tracks for extracting knowledge from the collections and representing that knowledge to facilitate users acquiring gradual, long term, constructive insights. Automatically processing documents makes it crucial to consider the accountability of the algorithms, as well as understanding when and why algorithms make errors, and possibly invent techniques that compensate or reduce the impact of errors. It also includes dealing with malicious adversaries carefully manipulating the data in order to compromise the whole knowledge extraction effort. In other words, Linkmedia also investigates various aspects related to the security of the algorithms analyzing multimedia material for knowledge extraction and representation.
Knowledge is not solely extracted by algorithms, but also by humans as they gradually get insight. This human knowledge can be materialized in computer-friendly formats, allowing algorithms to use this knowledge. For example, humans can create or update ontologies and knowledge bases that are in relation with a particular collection, they can manually label specific data samples to facilitate their disambiguation, they can manually correct errors, etc. In turn, knowledge provided by humans may help algorithms to then better process the data collections, which provides higher quality knowledge to humans, which in turn can provide some better feedback to the system, and so on. This virtuous cycle where algorithms and humans cooperate in order to make the most of multimedia collections requires specific support and techniques, as detailed below.
Machine Learning for Multimedia Material.
Many approaches are used to extract relevant information from multimedia material, ranging from very low-level to higher-level descriptions (classes, captions, ...). That diversity of information is produced by algorithms that have varying degrees of supervision. Lately, fully supervised approaches based on deep learning proved to outperform most older techniques. This is particularly true for the latest developments of Recurrent Neural Networkds (RNN, such as LSTMs) or convolutional neural network (CNNs) for images that reach excellent performance 44. Linkmedia contributes to advancing the state of the art in computing representations for multimedia material by investigating the topics listed below. Some of them go beyond the very processing of multimedia material as they also question the fundamentals of machine learning procedures when applied to multimedia.
- Learning from few samples/weak supervisions. CNNs and RNNs need large collections of carefully annotated data. They are not fitted for analyzing datasets where few examples per category are available or only cheap image-level labels are provided. Linkmedia investigates low-shot, semi-supervised and weakly supervised learning processes: Augmenting scarce training data by automatically propagating labels 47, or transferring what was learned on few very well annotated samples to allow the precise processing of poorly annotated data 56. Note that this context also applies to the processing of heritage collections (paintings, illuminated manuscripts, ...) that strongly differ from contemporary natural images. Not only annotations are scarce, but the learning processes must cope with material departing from what standard CNNs deal with, as classes such as "planes", "cars", etc, are irrelevant in this case.
- Ubiquitous Training. NN (CNNs, LSTMs) are mainstream for producing representations suited for high-quality classification. Their training phase is ubiquitous because the same representations can be used for tasks that go beyond classification, such as retrieval, few-shot, meta- and incremental learning, all boiling down to some form of metric learning. We demonstrated that this ubiquitous training is relatively simpler 47 yet as powerful as ad-hoc strategies fitting specific tasks 61. We study the properties and the limitations of this ubiquitous training by casting metric learning as a classification problem.
- Beyond static learning. Multimedia collections are by nature continuously growing, and ML processes must adapt. It is not conceivable to re-train a full new model at every change, but rather to support continuous training and/or allowing categories to evolve as the time goes by. New classes may be defined from only very few samples, which links this need for dynamicity to the low-shot learning problem discussed here. Furthermore, active learning strategies determining which is the next sample to use to best improve classification must be considered to alleviate the annotation cost and the re-training process 51. Eventually, the learning process may need to manage an extremely large number of classes, up to millions. In this case, there is a unique opportunity of blending the expertise of Linkmedia on large scale indexing and retrieval with deep learning. Base classes can either be "summarized" e.g. as a multi-modal distribution, or their entire training set can be made accessible as an external associative memory 67.
- Learning and lightweight architectures. Multimedia is everywhere, it can be captured and processed on the mobile devices of users. It is necessary to study the design of lightweight ML architectures for mobile and embedded vision applications. Inspired by 71, we study the savings from quantizing hyper-parameters, pruning connections or other approximations, observing the trade-off between the footprint of the learning and the quality of the inference. Once strategy of choice is progressive learning which early aborts when confident enough 52.
- Multimodal embeddings. We pursue pioneering work of Linkmedia on multimodal embedding, i.e., representing multiple modalities or information sources in a single embedded space 65, 64, 66. Two main directions are explored: exploiting adversarial architectures (GANs) for embedding via translation from one modality to another, extending initial work in 66 to highly heterogeneous content; combining and constraining word and RDF graph embeddings to facilitate entity linking and explanation of lexical co-occurrences 41.
- Accountability of ML processes. ML processes achieve excellent results but it is mandatory to verify that accuracy results from having determined an adequate problem representation, and not from being abused by artifacts in the data. Linkmedia designs procedures for at least explaining and possibly interpreting and understanding what the models have learned. We consider heat-maps materializing which input (pixels, words) have the most importance in the decisions 60, Taylor decompositions to observe the individual contributions of each relevance scores or estimating LID 28 as a surrogate for accounting for the smoothness of the space.
- Extracting information. ML is good at extracting features from multimedia material, facilitating subsequent classification, indexing, or mining procedures. Linkmedia designs extraction processes for identifying parts in the images 57, 58, relationships between the various objects that are represented in images 34, learning to localizing objects in images with only weak, image-level supervision 60 or fine-grained semantic information in texts 39. One technique of choice is to rely on generative adversarial networks (GAN) for learning low-level representations. These representations can e.g. be based on the analysis of density 70, shading, albedo, depth, etc.
- Learning representations for time evolving multimedia material. Video and audio are time evolving material, and processing them requests to take their time line into account. In 53, 37 we demonstrated how shapelets can be used to transform time series into time-free high-dimensional vectors, preserving however similarities between time series. Representing time series in a metric space improves clustering, retrieval, indexing, metric learning, semi-supervised learning and many other machine learning related tasks. Research directions include adding localization information to the shapelets, fine-tuning them to best fit the task in which they are used as well as designing hierarchical representations.
Adversarial Machine Learning.
Systems based on ML take more and more decisions on our behalf, and maliciously influencing these decisions by crafting adversarial multimedia material is a potential source of dangers: a small amount of carefully crafted noise imperceptibly added to images corrupts classification and/or recognition. This can naturally impact the insight users get on the multimedia collection they work with, leading to taking erroneous decisions for example.
This adversarial phenomenon is not particular to deep learning, and can be observed even when using other ML approaches 33. Furthermore, it has been demonstrated that adversarial samples generalize very well across classifiers, architectures, training sets. The reasons explaining why such tiny content modifications succeed in producing severe errors are still not well understood.
We are left with little choice: we must gain a better understanding of the weaknesses of ML processes, and in particular of deep learning. We must understand why attacks are possible as well as discover mechanisms protecting ML against adversarial attacks (with a special emphasis on convolutional neural networks). Some initial contributions have started exploring such research directions, mainly focusing on images and computer vision problems. Very little has been done for understanding adversarial ML from a multimedia perspective 38.
Linkmedia is in a unique position to throw at this problem new perspectives, by experimenting with other modalities, used in isolation one another, as well as experimenting with true multimodal inputs. This is very challenging, and far more complicated and interesting than just observing adversarial ML from a computer vision perspective. No one clearly knows what is at stake with adversarial audio samples, adversarial video sequences, adversarial ASR, adversarial NLP, adversarial OCR, all this being often part of a sophisticated multimedia processing pipeline.
Our ambition is to lead the way for initiating investigations where the full diversity of modalities we are used to work with in multimedia are considered from a perspective of adversarial attacks and defenses, both at learning and test time. In addition to what is described above, and in order to trust the multimedia material we analyze and/or the algorithms that are at play, Linkmedia investigates the following topics:
- Beyond classification. Most contributions in relation with adversarial ML focus on classification tasks. We started investigating the impact of adversarial techniques on more diverse tasks such as retrieval 27. This problem is related to the very nature of euclidean spaces where distances and neighborhoods can all be altered. Designing defensive mechanisms is a natural companion work.
- Detecting false information. We carry-on with earlier pioneering work of Linkmedia on false information detection in social media. Unlike traditional approaches in image forensics 42, we build on our expertise in content-based information retrieval to take advantage of the contextual information available in databases or on the web to identify out-of-context use of text or images which contributed to creating a false information 54.
- Deep fakes. Progress in deep ML and GANs allow systems to generate realistic images and are able to craft audio and video of existing people saying or doing things they never said or did 50. Gaining in sophistication, these machine learning-based "deep fakes" will eventually be almost indistinguishable from real documents, making their detection/rebutting very hard. Linkmedia develops deep learning based counter-measures to identify such modern forgeries. We also carry on with making use of external data in a provenance filtering perspective 59 in order to debunk such deep fakes.
- Distributions, frontiers, smoothness, outliers. Many factors that can possibly explain the adversarial nature of some samples are in relation with their distribution in space which strongly differs from the distribution of natural, genuine, non adversarial samples. We are investigating the use of various information theoretical tools that facilitate observing distributions, how they differ, how far adversarial samples are from benign manifolds, how smooth is the feature space, etc. In addition, we are designing original adversarial attacks and develop detection and curating mechanisms 28.
Multimedia Knowledge Extraction.
Information obtained from collections via computer ran processes is not the only thing that needs to be represented. Humans are in the loop, and they gradually improve their level of understanding of the content and nature of the multimedia collection. Discovering knowledge and getting insight is involving multiple people across a long period of time, and what each understands, concludes and discovers must be recorded and made available to others. Collaboratively inspecting collections is crucial. Ontologies are an often preferred mechanism for modeling what is inside a collection, but this is probably limitative and narrow.
Linkmedia is concerned with making use of existing strategies in relation with ontologies and knowledge bases. In addition, Linkmedia uses mechanisms allowing to materialize the knowledge gradually acquired by humans and that might be subsequently used either by other humans or by computers in order to better and more precisely analyze collections. This line of work is instantiated at the core of the iCODA project Linkmedia coordinates.
We are therefore concerned with:
- Multimedia analysis and ontologies. We develop approaches for linking multimedia content to entities in ontologies for text and images, building on results in multimodal embedding to cast entity linking into a nearest neighbor search problem in a high-dimensional joint embedding of content and entities 64. We also investigate the use of ontological knowledge to facilitate information extraction from content 41.
- Explainability and accountability in information extraction. In relation with ontologies and entity linking, we develop innovative approaches to explain statistical relations found in data, in particular lexical or entity co-occurrences in textual data, for example using embeddings constrained with translation properties of RDF knowledge or path-based explanation within RDF graphs. We also work on confidence measures in entity linking and information extraction, studying how the notions of confidence and information source can be accounted for in knowledge basis and used in human-centric collaborative exploration of collections.
- Dynamic evolution of models for information extraction. In interactive exploration and information extraction, e.g., on cultural or educational material, knowledge progressively evolves as the process goes on, requiring on-the-fly design of new models for content-based information extractors from very few examples, as well as continuous adaptation of the models. Combining in a seamless way low-shot, active and incremental learning techniques is a key issue that we investigate to enable this dynamic mechanisms on selected applications.
3.4 Research Direction 2: Accessing Information
Linkmedia centers its activities on enabling humans to make good use of vast multimedia collections. This material takes all its cultural and economic value, all its artistic wonder when it can be accessed, watched, searched, browsed, visualized, summarized, classified, shared, ... This allows users to fully enjoy the incalculable richness of the collections. It also makes it possible for companies to create business rooted in this multimedia material.
Accessing the multimedia data that is inside a collection is complicated by the various type of data, their volume, their length, etc. But it is even more complicated to access the information that is not materialized in documents, such as the relationships between parts of different documents that however share some similarity. Linkmedia in its first four years of existence established itself as one of the leading teams in the field of multimedia analytics, contributing to the establishment of a dedicated community (refer to the various special sessions we organized with MMM, the iCODA and the LIMAH projects, as well as 48, 49, 45).
Overall, facilitating the access to the multimedia material, to the relevant information and the corresponding knowledge asks for algorithms that efficiently search collections in order to identify the elements of collections or of the acquired knowledge that are matching a query, or that efficiently allow navigating the collections or the acquired knowledge. Navigation is likely facilitated if techniques are able to handle information and knowledge according to hierarchical perspectives, that is, allow to reveal data according to various levels of details. Aggregating or summarizing multimedia elements is not trivial.
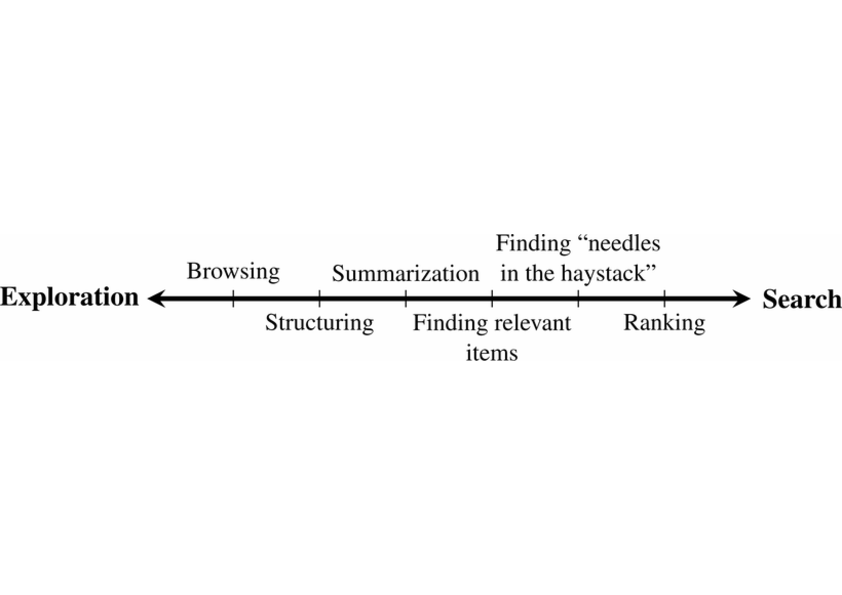
Exploration-search axis with example tasks
Three topics are therefore in relation with this second research direction. Linkmedia tackles the issues in relation to searching, to navigating and to summarizing multimedia information. Information needs when discovering the content of a multimedia collection can be conveniently mapped to the exploration-search axis, as first proposed by Zahálka and Worring in 69, and illustrated by Figure 1 where expert users typically work near the right end because their tasks involve precise queries probing search engines. In contrast, lay-users start near the exploration end of the axis. Overall, users may alternate searches and explorations by going back and forth along the axis. The underlying model and system must therefore be highly dynamic, support interactions with the users and propose means for easy refinements. Linkmedia contributes to advancing the state of the art in searching operations, in navigating operations (also referred to as browsing), and in summarizing operations.
Searching.
Search engines must run similarity searches very efficiently. High-dimensional indexing techniques therefore play a central role. Yet, recent contributions in ML suggest to revisit indexing in order to adapt to the specific properties of modern features describing contents.
- Advanced scalable indexing. High-dimensional indexing is one of the foundations of Linkmedia. Modern features extracted from the multimedia material with the most recent ML techniques shall be indexed as well. This, however, poses a series of difficulties due to the dimensionality of these features, their possible sparsity, the complex metrics in use, the task in which they are involved (instance search, -nn, class prototype identification, manifold search 47, time series retrieval, ...). Furthermore, truly large datasets require involving sketching 31, secondary storage and/or distribution 30, 29, alleviating the explosion of the number of features to consider due to their local nature or other innovative methods 46, all introducing complexities. Last, indexing multimodal embedded spaces poses a new series of challenges.
- Improving quality. Scalable indexing techniques are approximate, and what they return typically includes a fair amount of false positives. Linkmedia works on improving the quality of the results returned by indexing techniques. Approaches taking into account neighborhoods 40, manifold structures instead of pure distance based similarities 47 must be extended to cope with advanced indexing in order to enhance quality. This includes feature selection based on intrinsic dimensionality estimation 28.
- Dynamic indexing. Feature collections grow, and it is not an option to fully reindex from scratch an updated collection. This trivially applies to the features directly extracted from the media items, but also to the base class prototypes that can evolve due to the non-static nature of learning processes. Linkmedia will continue investigating what is at stake when designing dynamic indexing strategies.
Navigating.
Navigating a multimedia collection is very central to its understanding. It differs from searching as navigation is not driven by any specific query. Rather, it is mostly driven by the relationships that various documents have one another. Relationships are supported by the links between documents and/or parts of documents. Links rely on semantic similarity, depicting the fact that two documents share information on the same topic. But other aspects than semantics are also at stake, e.g., time with the dates of creation of the documents or geography with mentions or appearance in documents of some geographical landmarks or with geo-tagged data.
In multimedia collections, links can be either implicit or explicit, the latter being much easier to use for navigation. An example of an implicit link can be the name of someone existing in several different news articles; we, as humans, create a mental link between them. In some cases, the computer misses such configurations, leaving such links implicit. Implicit links are subject to human interpretation, hence they are sometimes hard to identify for any automatic analysis process. Implicit links not being materialized, they can therefore hardly be used for navigation or faceted search. Explicit links can typically be seen as hyperlinks, established either by content providers or, more aligned with Linkmedia, automatically determined from content analysis. Entity linking (linking content to an entity referenced in a knowledge base) is a good example of the creation of explicit links. Semantic similarity links, as investigated in the LIMAH project and as considered in the search and hyperlinking task at MediaEval and TRECVid, are also prototypical links that can be made explicit for navigation. Pursuing work, we investigate two main issues:
- Improving multimodal content-based linking. We exploit achievements in entity linking to go beyond lexical or lexico-visual similarity and to provide semantic links that are easy to interpret for humans; carrying on, we work on link characterization, in search of mechanisms addressing link explainability (i.e., what is the nature of the link), for instance using attention models so as to focus on the common parts of two documents or using natural language generation; a final topic that we address is that of linking textual content to external data sources in the field of journalism, e.g., leveraging topic models and cue phrases along with a short description of the external sources.
- Dynamicity and user-adaptation. One difficulty for explicit link creation is that links are often suited for one particular usage but not for another, thus requiring creating new links for each intended use; whereas link creation cannot be done online because of its computational cost, the alternative is to generate (almost) all possible links and provide users with selection mechanisms enabling personalization and user-adaptation in the exploration process; we design such strategies and investigate their impact on exploration tasks in search of a good trade-off between performance (few high-quality links) and genericity.
Summarizing.
Multimedia collections contain far too much information to allow any easy comprehension. It is mandatory to have facilities to aggregate and summarize a large body on information into a compact, concise and meaningful representation facilitating getting insight. Current technology suggests that multimedia content aggregation and story-telling are two complementary ways to provide users with such higher-level views. Yet, very few studies already investigated these issues. Recently, video or image captioning 68, 63 have been seen as a way to summarize visual content, opening the door to state-of-the-art multi-document text summarization 43 with text as a pivot modality. Automatic story-telling has been addressed for highly specific types of content, namely TV series 35 and news 55, 62, but still need a leap forward to be mostly automated, e.g., using constraint-based approaches for summarization 32, 62.
Furthermore, not only the original multimedia material has to be summarized, but the knowledge acquired from its analysis is also to summarize. It is important to be able to produce high-level views of the relationships between documents, emphasizing some structural distinguishing qualities. Graphs establishing such relationships need to be constructed at various level of granularity, providing some support for summarizing structural traits.
Summarizing multimedia information poses several scientific challenges that are:
- Choosing the most relevant multimedia aggregation type: Taking a multimedia collection into account, a same piece of information can be present in several modalities. The issue of selecting the most suitable one to express a given concept has thus to be considered together with the way to mix the various modalities into an acceptable production. Standard summarization algorithms have to be revisited so that they can handle continuous representation spaces, allowing them to benefit from the various modalities 36.
- Expressing user’s preferences: Different users may appreciate quite different forms of multimedia summaries, and convenient ways to express their preferences have to be proposed. We for example focus on the opportunities offered by the constraint-based framework.
- Evaluating multimedia summaries: Finding criteria to characterize what a good summary is remains challenging, e.g., how to measure the global relevance of a multimodal summary and how to compare information between and across two modalities. We tackle this issue particularly via a collaboration with A. Smeaton at DCU, comparing the automatic measures we will develop to human judgments obtained by crowd-sourcing.
- Taking into account structuring and dynamicity: Typed links between multimedia fragments, and hierarchical topical structures of documents obtained via work previously developed within the team are two types of knowledge which have seldom been considered as long as summarization is concerned. Knowing that the event present in a document is causally related to another event described in another document can however modify the ways summarization algorithms have to consider information. Moreover the question of producing coarse-to-fine grain summaries exploiting the topical structure of documents is still an open issue. Summarizing dynamic collections is also challenging and it is one of the questions we consider.
4 Application domains
4.1 Asset management in the entertainment business
Media asset management—archiving, describing and retrieving multimedia content—has turned into a key factor and a huge business for content and service providers. Most content providers, with television channels at the forefront, rely on multimedia asset management systems to annotate, describe, archive and search for content. So do archivists such as the Institut National de l'Audiovisuel, the bibliothèque Nationale de France, the Nederlands Instituut voor Beeld en Geluid or the British Broadcast Corporation, as well as media monitoring companies, such as Yacast in France. Protecting copyrighted content is another aspect of media asset management.
4.2 Multimedia Internet
One of the most visible application domains of linked multimedia content is that of multimedia portals on the Internet. Search engines now offer many features for image and video search. Video sharing sites also feature search engines as well as recommendation capabilities. All news sites provide multimedia content with links between related items. News sites also implement content aggregation, enriching proprietary content with user-generated content and reactions from social networks. Most public search engines and Internet service providers offer news aggregation portals. This also concerns TV on-demand and replay services as well as social TV services and multi-screen applications. Enriching multimedia content, with explicit links targeting either multimedia material or knowledge databases is central here.
4.3 Data journalism
Data journalism forms an application domain where most of the technology developed by Linkmedia can be used. On the one hand, data journalists often need to inspect multiple heterogeneous information sources, some being well structured, some other being fully unstructured. They need to access (possibly their own) archives with either searching or navigational means. To gradually construct insight, they need collaborative multimedia analytics processes as well as elements of trust in the information they use as foundations for their investigations. Trust in the information, watching for adversarial and/or (deep) fake material, accountability are all crucial here.
5 Social and environmental responsibility
5.1 Impact of research results
Social biases in text generation.
Recent advances in the domain of text generation allow realistic text-based interaction with a computer. These systems rely on complex neural architectures that leverage very large amount of training texts collected the Web. The problem is that these texts contains unwanted biases (sexism, racism, harmful language...) that are sometimes even amplified by the training procedure. Curating the training texts once for all is not feasible due to the complexity of defining a priori what is relevant or not at the training time. Our work on controlled generation 24 takes another point of view and tries to impose constraints at the inference time. This work aims at making the text generation respect application-specific conditions with the help of a simple classifier. The proposed approach can be used to correct biases in generated texts as well as, for exemple, to de-hate existing texts.
6 Highlights of the year
6.1 Awards
- Best Student Paper Award, IEEE Workshop on Information Forensics and Security, Pierre Fernandez, Antoine Chaffin, Karim Tit, Vivien Chappelier, Teddy Furon. December 2023.
- Top 3% of all papers accepted at IEEE International Conference on Acoustics Speech and Signal Processing - IEEE ICASSP, Kassem Kallas, Teddy Furon. June 2023.
- Best Paper Award, 30th conference on Traitement automatique des langues naturelles, Loïc Fosse, Duc Hau Nguyen, Pascale Sébillot, Guillaume Gravier. June 2023.
7 New results
7.1 Extracting and Representing Information
7.1.1 How to choose your best allies for a transferable attack?
Participants: Thibault Maho, Seyed-Mohsen Moosavi-Dezfooli [Imperial College London ], Teddy Furon.
The transferability of adversarial examples is a key issue in the security of deep neural networks. The possibility of an adversarial example crafted for a source model fooling another targeted model makes the threat of adversarial attacks more realistic. Measuring transferability is a crucial problem, but the Attack Success Rate alone does not provide a sound evaluation. This paper proposes a new methodology for evaluating transferability by putting distortion in a central position 15. This new tool shows that transferable attacks may perform far worse than a black box attack if the attacker randomly picks the source model. To address this issue, we propose a new selection mechanism, called FiT, which aims at choosing the best source model with only a few preliminary queries to the target. Our experimental results show that FiT is highly effective at selecting the best source model for multiple scenarios such as single-model attacks, ensemble-model attacks and multiple attacks.
7.1.2 Embedding Space Interpolation Beyond Mini-Batch, Beyond Pairs and Beyond Examples
Participants: Shashanka Venkataramanan, Ewa Kijak, Laurent Amsaleg, Yannis Avrithis [IARAI].
Mixup refers to interpolation-based data augmentation, originally motivated as a way to go beyond empirical risk minimization (ERM). Its extensions mostly focus on the definition of interpolation and the space (input or embedding) where it takes place, while the augmentation process itself is less studied. In most methods, the number of generated examples is limited to the mini-batch size and the number of examples being interpolated is limited to two (pairs), in the input space. We make progress in this direction by introducing MultiMix, which generates an arbitrarily large number of interpolated examples beyond the mini-batch size, and interpolates the entire mini-batch in the embedding space 17. Effectively, we sample on the entire convex hull of the mini-batch rather than along linear segments between pairs of examples. On sequence data we further extend to Dense MultiMix. We densely interpolate features and target labels at each spatial location and also apply the loss densely. To mitigate the lack of dense labels, we inherit labels from examples and weight interpolation factors by attention as a measure of confidence. Overall, we increase the number of loss terms per mini-batch by orders of magnitude at little additional cost. This is only possible because of interpolating in the embedding space. We empirically show that our solutions yield significant improvement over state-of-the-art mixup methods on four different benchmarks, despite interpolation being only linear. By analyzing the embedding space, we show that the classes are more tightly clustered and uniformly spread over the embedding space, thereby explaining the improved behavior.
7.1.3 The Stable Signature: Rooting Watermarks in Latent Diffusion Models
Participants: Pierre Fernandez [Meta IA], Guillaume Couairon [Meta IA], Hervé Jégou [Meta IA], Teddy Furon, Matthijs Douze [Meta IA].
Generative image modeling enables a wide range of applications but raises ethical concerns about responsible deployment. We introduce an active content tracing method combining image watermarking and Latent Diffusion Models. The goal is for all generated images to conceal an invisible watermark allowing for future detection and/or identification. The method quickly fine-tunes the latent decoder of the image generator, conditioned on a binary signature10. A pre-trained watermark extractor recovers the hidden signature from any generated image and a statistical test then determines whether it comes from the generative model. We evaluate the invisibility and robustness of the watermarks on a variety of generation tasks, showing that the Stable Signature is robust to image modifications. For instance, it detects the origin of an image generated from a text prompt, then cropped to keep 10% of the content, with 90+% accuracy at a false positive rate below .
7.1.4 FBI: Fingerprinting models with Benign Inputs
Participants: Thibault Maho, Teddy Furon, Erwan Le Merrer [WIDE].
Recent advances in the fingerprinting of deep neural networks are able to detect specific instances of models, placed in a black-box interaction scheme. Inputs used by the fingerprinting protocols are specifically crafted for each precise model to be checked for. While efficient in such a scenario, this nevertheless results in a lack of guarantee after a mere modification of a model (e.g. finetuning, quantization of the parameters). This work generalizes fingerprinting to the notion of model families and their variants and extends the task-encompassing scenarios where one wants to fingerprint not only a precise model (previously referred to as a detection task) but also to identify which model or family is in the black-box (identification task) 4 14. The main contribution is the proposal of fingerprinting schemes that are resilient to significant modifications of the models. We achieve these goals by demonstrating that benign inputs, that are unmodified images, are sufficient material for both tasks. We leverage an information-theoretic scheme for the identification task. We devise a greedy discrimination algorithm for the detection task. Both approaches are experimentally validated over an unprecedented set of more than 1,000 networks.
7.1.5 Three bricks to consolidate watermarks for large language models
Participants: Pierre Fernandez [Meta IA], Antoine Chaffin [Imatag], Karim Tit [Thalès], Vivien Chappelier [Imatag], Teddy Furon.
Discerning between generated and natural texts is increasingly challenging. In this context, watermarking emerges as a promising technique for ascribing text to a specific generative model. It alters the sampling generation process to leave an invisible trace in the output, facilitating later detection. This research consolidates watermarks for large language models based on three theoretical and empirical considerations 8. First, we introduce new statistical tests that offer robust theoretical guarantees which remain valid even at low false-positive rates (less than ). Second, we compare the effectiveness of watermarks using classical benchmarks in the field of natural language processing, gaining insights into their real-world applicability. Third, we develop advanced detection schemes for scenarios where access to the LLM is available, as well as multi-bit watermarking.
7.1.6 "Honey, tell me what's wrong", global explainability and diagnosing of NLP models through cooperative generation
Participants: Antoine Chaffin [IMATAG], Julien Delaunay [Lacodam].
The ubiquity of complex machine learning has raised the importance of model-agnostic explanation algorithms. These methods sample artificial instances by slightly perturbing target instances and observing the variations in the model decision. However, such methods require access to initial samples and only provide explanations of the decision for these. To tackle these problems, we propose Therapy, the first model-agnostic explanation method adapted to text which requires no input dataset 19. This method generates texts following the distribution learned by a classifier through cooperative generation. Not relying on initial samples, in addition to allowing use in cases where no data is available (e.g, for confidentiality reasons), provides global explanations of the model rather than multiple local ones, offering an overview of the model behavior. Our experiments show that although no input data is used to generate samples, Therapy provides insightful information about features used by the classifier that are competitive with the ones from methods relying on input samples.
7.1.7 What hides behind relation embeddings?
Participants: Guillaume Gravier, Pascale Sébillot, Hugo Thomas.
In this line of work, rather than focusing on the performance scores usually provided (e.g., the F1 measure), we proposed an in-depth analysis, according to several criteria, of the relation embedding resulting from different model architectures for relation typing. This analysis aims at better understanding the organization and properties of the latent embedded space, an important issue for models exploiting distances in this vector space 21. We evaluate the influence on these models of the lexicon, the syntax, and the semantics of relations, the representation of the entities, as well as the geometry of their latent spaces. It appears that the relation embeddings are learned unevenly from one model to another trained in the same way; in this case, the indicators we proposed are additional knowledge about the latent space to better exploit its properties.
7.1.8 Geometry of self-attention in classification
Participants: Loïc Fosse [INSA Rennes], Duc Hau Nguyen, Pascale Sébillot, Guillaume Gravier.
Various studies have highlighted the anisotropy of BERT word embeddings within an utterance, i.e., their concentration in a given direction, especially in a classification task. We aim at better understanding this phenomenon and how this convergence is built by analyzing the geometric properties of the word embeddings within a self-attention layer. We show that the direction towards which embeddings align themselves characterizes class membership. We also study the intrinsic mechanism of the self-attention layer and the mechanisms at play between keys and values to ensure the construction of an anisotropic representation 20. This construction is progressive when several layers are stacked. It also proves to be robust to external constraints on the distribution of attention weights, which the model compensates through the values and keys.
7.1.9 Improving the plausibility of attention weights through regularization, semi-supervision, and supervision
Participants: Duc Hau Nguyen, Cyrielle Mallart [Shaman], Guillaume Gravier, Pascale Sébillot.
Attention mechanism is contributing to the majority of recent advances in machine learning for natural language processing. Additionally, it results in an attention map that shows the proportional influence of each input in its decision. Empirical studies postulate that attention maps can be provided as an explanation for model output. However, it is still questionable to ask whether this explanation helps regular people to understand and accept the model output (the plausibility of the explanation). Recent studies show that attention weights in RNN encoders are hardly plausible because they spread on input tokens. We thus propose three additional constraints to the learning objective function to improve the plausibility of the attention map: regularization to increase the attention weight sparsity, semi-supervision to supervise the map by a heuristic and supervision by human annotation 12. Results show that all techniques can improve the attention map plausibility at some level. We also observe that specific instructions for human annotation might have a negative effect on classification performance. Beyond the attention map, results on text classification tasks also show that the contextualization layer plays a crucial role in finding the right space for finding plausible tokens, no matter how constraints bring the gain.
7.1.10 Gradient-Informed Neural Network Statistical Robustness Estimation
Participants: Karim Tit [Thalès], Teddy Furon, Mathias Rousset [SimSmart].
Deep neural networks are robust against random corruptions of the inputs to some extent. This global sense of safety is not sufficient in critical applications where probabilities of failure must be assessed with accuracy. Some previous works applied known statistical methods from the field of rare event analysis to classification. Yet, they use classifiers as black-box models without taking into account gradient information, readily available for deep learning models via autodifferentiation. We propose a new and highly efficient estimator of probabilities of failure dedicated to neural networks as it leverages the fast computation of gradients of the model through back-propagation 16.
7.1.11 Functional invariants to watermark large transformers
Participants: Pierre Fernandez [Meta IA], Guillaume Couairon [Meta IA], Teddy Furon, Matthijs Douze [Meta IA].
The rapid growth of transformer-based models increases the concerns about their integrity and ownership insurance. Watermarking addresses this issue by embedding a unique identifier into the model, while preserving its performance. However, most existing approaches require to optimize the weights to imprint the watermark signal, which is not suitable at scale due to the computational cost. This paper explores watermarks with virtually no computational cost, applicable to a non-blind white-box setting (assuming access to both the original and watermarked networks) 9. They generate functionally equivalent copies by leveraging the models' invariance, via operations like dimension permutations or scaling/unscaling. This enables to watermark models without any change in their outputs and remains stealthy. Experiments demonstrate the effectiveness of the approach and its robustness against various model transformations (fine-tuning, quantization, pruning), making it a practical solution to protect the integrity of large models.
7.1.12 Histoire Récente de la Sécurité des Contenus Multimédia Un Focus sur la Dissimulation d'Information
Participants: Patrick Bas [CRIStAL - Centre de Recherche en Informatique, Signal et Automatique de Lille - UMR 9189], Gwenael Doerr [Synamedia Technologies France], Teddy Furon, William Puech [LIRMM - Laboratoire d'Informatique de Robotique et de Microélectronique de Montpellier].
Le tatouage numérique et la stéganographie sont les deux faces de la dissimulation d'information dans les contenus multimédia. Dans cet article, nous passons en revue les avancées techniques de ces deux domaines et nous indiquons comment ces technologies se sont installées dans notre vie de tous les jours 18.
7.1.13 Mixer: DNN Watermarking using Image Mixup
Participants: Kassem Kallas, Teddy Furon.
It is crucial to protect the intellectual property rights of DNN models prior to their deployment. The DNN should perform two main tasks: its primary task and watermarking task. This paper proposes a lightweight, reliable, and secure DNN watermarking that attempts to establish strong ties between these two tasks 13. The samples triggering the watermarking task are generated using image Mixup either from training or testing samples. This means that there is an infinity of triggers not limited to the samples used to embed the watermark in the model at training. The extensive experiments on image classification models for different datasets as well as exposing them to a variety of attacks, show that the proposed watermarking provides protection with an adequate level of security and robustness.
7.1.14 A novel method for temporal graph classification based on transitive reduction
Participants: Carolina Stephanie Jerônimo de Almeida, Zenilton Kleber Gonçalves Do Patrocínio Jr [PUC Minas, Brésil], Simon Malinowski, Silvio J.R. Guimarães [PUC Minas, Brésil], Guillaume Gravier.
Domains such as bio-informatics, social network analysis, and computer vision, describe relations between entities and cannot be interpreted as vectors or fixed grids, instead, they are naturally represented by graphs. Often this kind of data evolves over time in a dynamic world, respecting a temporal order being known as temporal graphs. The latter became a challenge since subgraph patterns are very difficult to find and the distance between those patterns may change irregularly over time. While state-of-the-art methods are primarily designed for static graphs and may not capture temporal information, recent works have proposed mapping temporal graphs to static graphs to allow for the use of conventional static kernels and graph neural approaches. In this study, we compare the transitive reduction impact on these mappings in terms of accuracy and computational efficiency across different classification tasks 6. Furthermore, we introduce a novel mapping method using a transitive reduction approach that outperforms existing techniques in terms of classification accuracy. Our experimental results demonstrate the effectiveness of the proposed mapping method in improving the accuracy of supervised classification for temporal graphs while maintaining reasonable computational efficiency.
7.1.15 MAAIP: Multi-Agent Adversarial Interaction Priors for imitation from fighting demonstrations for physics-based characters
Participants: Mohammed Younes, Ewa Kijak, Richard Kulpa, Simon Malinowski, Franck Multon.
Simulating realistic interaction and motions for physics-based characters is of great interest for interactive applications, and automatic secondary character animation in the movie and video game industries. Recent works in reinforcement learning have proposed impressive results for single character simulation, especially the ones that use imitation learning based techniques. However, imitating multiple characters interactions and motions requires to also model their interactions. In this work, we propose a novel Multi-Agent Generative Adversarial Imitation Learning based approach that generalizes the idea of motion imitation for one character to deal with both the interaction and the motions of the multiple physics-based characters 5. Two unstructured datasets are given as inputs: 1) a single-actor dataset containing motions of a single actor performing a set of motions linked to a specific application, and 2) an interaction dataset containing a few examples of interactions between multiple actors. Based on these datasets, our system trains control policies allowing each character to imitate the interactive skills associated with each actor, while preserving the intrinsic style. This approach has been tested on two different fighting styles, boxing and full-body martial art, to demonstrate the ability of the method to imitate different styles.
7.1.16 Minimum Recall-Based Loss Function for Imbalanced Time Series Classification
Participants: Josu Ircio [IKERLAN], Aizea Lojo [IKERLAN], Usue Mori [Univ Basque Country], Simon Malinowski, Jose Lozano [Univ Basque Country].
This paper deals with imbalanced time series classification problems. In particular, we propose to learn time series classifiers that maximize the minimum recall of the classes rather than the accuracy. Consequently, we manage to obtain classifiers which tend to give the same importance to all the classes. Unfortunately, for most of the traditional classifiers, learning to maximize the minimum recall of the classes is not trivial (if possible), since it can distort the nature of the classifiers themselves. Neural networks, in contrast, are classifiers that explicitly define a loss function, allowing it to be modified. Given that the minimum recall is not a differentiable function, and therefore does not allow the use of common gradient-based learning methods, we apply and evaluate several smooth approximations of the minimum recall function. A thorough experimental evaluation shows that our approach improves the performance of state-of-the-art methods used in imbalanced time series classification, obtaining higher recall values for the minority classes, incurring only a slight loss in accuracy.
7.1.17 DINOv2: Learning Robust Visual Features without Supervision
Participants: Maxime Oquab [Meta IA], Timothée Darcet [Meta IA], Théo Moutakanni [Meta IA], Huy Vo [Meta IA], Marc Szafraniec [Meta IA], Vasil Khalidov [Meta IA], Pierre Fernandez [Linkmedia, Meta IA], Daniel Haziza [Meta IA], Francisco Massa [Meta IA], Alaaeldin El-Nouby [Meta IA], Mahmoud Assran [Meta IA], Nicolas Ballas [Meta IA], Wojciech Galuba [Meta IA], Russell Howes [Meta IA], Po-Yao Huang [Meta IA], Shang-Wen Li [Meta IA], Ishan Misra [Meta IA], Michael Rabbat [Meta IA], Vasu Sharma [Meta IA], Gabriel Synnaeve [Meta IA], Hu Xu [Meta IA], Hervé Jegou [Meta IA], Julien Mairal [Meta IA], Patrick Labatut [Meta IA], Armand Joulin [Meta IA], Piotr Bojanowski [Meta IA].
The recent breakthroughs in natural language processing for model pretraining on large quantities of data have opened the way for similar foundation models in computer vision. These models could greatly simplify the use of images in any system by producing all-purpose visual features, i.e., features that work across image distributions and tasks without finetuning. This work shows that existing pretraining methods, especially self-supervised methods, can produce such features if trained on enough curated data from diverse sources 26. We revisit existing approaches and combine different techniques to scale our pretraining in terms of data and model size. Most of the technical contributions aim at accelerating and stabilizing the training at scale. In terms of data, we propose an automatic pipeline to build a dedicated, diverse, and curated image dataset instead of uncurated data, as typically done in the self-supervised literature. In terms of models, we train a ViT model with 1B parameters and distill it into a series of smaller models that surpass the best available all-purpose features, OpenCLIP, on most of the benchmarks at image and pixel levels.
7.2 Accessing Information
7.2.1 Zero-Shot and Few-Shot Video Question Answering with Multi-Modal Prompts
Participants: Deniz Engin, Yannis Avrithis [IARAI].
Recent vision-language models are driven by large-scale pretrained models. However, adapting pretrained models on limited data presents challenges such as overfitting, catastrophic forgetting, and the cross-modal gap between vision and language. We introduce a parameter-efficient method to address these challenges, combining multimodal prompt learning and a transformer-based mapping network, while keeping the pretrained models frozen 7. Our experiments on several video question answering benchmarks demonstrate the superiority of our approach in terms of performance and parameter efficiency on both zero-shot and few-shot settings. Our code is available at https: //engindeniz.github.io/vitis.
7.2.2 Active image indexing
Participants: Pierre Fernandez [Meta IA], Matthijs Douze [Meta IA], Hervé Jégou [Meta IA], Teddy Furon.
Image copy detection and retrieval from large databases leverage two components. First, a neural network maps an image to a vector representation, that is relatively robust to various transformations of the image. Second, an efficient but approximate similarity search algorithm trades scalability (size and speed) against quality of the search, thereby introducing a source of error. This paper improves the robustness of image copy detection with active indexing, that optimizes the interplay of these two components 11. We reduce the quantization loss of a given image representation by making imperceptible changes to the image before its release. The loss is back-propagated through the deep neural network back to the image, under perceptual constraints. These modifications make the image more retrievable. Our experiments show that the retrieval and copy detection of activated images is significantly improved. For instance, activation improves by +40% the Recall1@1 on various image transformations, and for several popular indexing structures based on product quantization and locality sensitivity hashing.
8 Bilateral contracts and grants with industry
8.1 Bilateral contracts with industry
CIFRE PhD: Robustness of machine learning against uncertainties
Participants: Teddy Furon, Mathias Rousset, Karim Tit.
Duration: 3 years, started in December 2020 Partner: THALES La Ruche
This is a CIFRE PhD thesis project aiming to study the robustness of machine learning algorithm facing uncertainties in the acquisition chain of the data.CIFRE PhD: Certification of Deep Neural Networks
Participants: Teddy Furon, Kassem Kallas, Quentin Le Roux.
Duration: 3 years, started in November 2022 Partner:THALES
This is a CIFRE PhD thesis project aiming at assessing the security of already trained Deep Neural Networks, especially in the context of face recognition.CIFRE PhD: Watermarking and deep learning
Participants: Teddy Furon, Pierre Fernandez.
Duration: 3 years, started in May 2022 Partner: META AI
This is a CIFRE PhD thesis project aiming at watermarking deep learning models analyzing or generating images or at using deep learning to watermark images.CIFRE PhD: Domain generalization exploiting synthetic data
Participants: Ewa Kijak, Louis Hemadou.
Duration: 3 years, started in Nov. 2022 Partner: SAFRAN
This is a CIFRE PhD thesis project aiming at exploiting synthetic data to be able to perform transfer learning in presence of very few or inexistent real data in the context of image detection or classification tasks.CIFRE PhD: Detection and explanation of semantic manipulations in multimedia content
Participants: Ewa Kijak, Gautier Evennou.
Duration: 3 years, started in Sep. 2023 Partner: IMATAG
This is a CIFRE PhD thesis project aiming at detecting and explaining semantic manipulations in multimedia content, in the context of misinformation.CIFRE PhD: Machine learning for identification of factors impacting the quality of service of urban buses
Participants: Simon Malinowski, Guillaume Gravier, Erwan Vincent.
Duration: 3 years, started in Feb. 2022Partner: KEOLIS
This is a CIFRE PhD thesis project aiming at identifying factors that have an impact on the quality of service of urban buses, and at predicting inter-arrival times in order to better understand the urban bus network.Telegramme-CNRS bilateral contract: NLP for computational journalism
Participants: Vincent Claveau, Laurent Amsaleg, Pascale Sébillot, Christian Raymond [Insa Rennes], Nicolas Fouqué.
Duration: 2 years, started in Jan 2022
The project aims at developing a wide range of text-mining and classification tools with the French press group Le Télégramme. In particular, we aim at discovering cues of success in the already published news articles and then exploit them to propose new angles of coverage of newsworthy events to the journalists.CIFRE PhD: Introduction of rejection capabilities and externalized language models in deep learning systems for text reading under adverse conditions
Participants: Guillaume Gravier.
Duration: 3 years, started in June 2023 Partner: ANTAI
The thesis, in conjunction with the team SHADOC at IRISA, studies deep models for license plate recognition capable of balancing end-to-end training with separate language model training and adaptation.9 Partnerships and cooperations
9.1 International initiatives
9.1.1 Associate Teams in the framework of an Inria International Lab or in the framework of an Inria International Program
- Associate team LOGIC with PUC MINAS, Brazil from 2022 to 2024. Coordinator : Simon Malinowski
9.2 International research visitors
9.2.1 Visits of international scientists
- Roberto Santana from the University of Basque Country visited Linkmedia from the 6th to the 18th of November 2023
- Silvio Guimaraes from PUC MINAS visited Linkmedia from the 27th to the 31th of March 2023 and from the 17th to the 20th of October 2023. These visits have been organised thanks to the associate team LOGIC.
- Leonardo de Melo from UNICAMP visited Linkmedia from the 26th to the 30th of June 2023, and from the 4th to the 8th of December 2023
Research stays abroad
- Ewa Kijak has visited PUC MINAS, Brazil from the 29th of May to the 8th of June 2023 (thanks to associated team LOGIC)
- Simon Malinowski has visited PUC MINAS, Brazil from the 29th of May to the 6th of June 2023 (thanks to associated team LOGIC)
9.3 National initiatives
Chaire Security of AI for Defense Applications (SAIDA)
Participants: Teddy Furon, Laurent Amsaleg, Erwan Le Merrer [WIDE], Mathias Rousset [SIMSMART], Benoit Bonnet, Thibault Maho, Patrick Bas [CRIStAL - Centre de Recherche en Informatique, Signal et Automatique de Lille - UMR 9189], Samuel Tap, Karim Tit.
Duration: 4 years, started Sept 2020 ANR-20-CHIA-0011-01
SAIDA targets the AID "Fiabilité de l’intelligence artificielle, vulnérabilités et contre-mesures" chair. It aims at establishing the fundamental principles for designing reliable and secure AI systems: a reliable AI maintains its good performance even under uncertainties; a secure AI resists attacks in hostile environments. Reliability and security are challenged at training and at test time. SAIDA therefore studies core issues in relation with poisoning training data, stealing the parameters of the model or inferring sensitive training from information leaks. Additionally, SAIDA targets uncovering the fundamentals of attacks and defenses engaging AI at test time. Three converging research directions make SAIDA: 1) theoretical investigations grounded in statistics and applied mathematics to discover the underpinnings of reliability and security, 2) connects adversarial sampling and Information Forensics and Security, 3) protecting the training data and the AI system. SAIDA thus combines theoretical investigations with more applied and heuristic studies to guarantee the applicability of the findings as well as the ability to cope with real world settings.
ANR Archival: Multimodal machine comprehension of language for new intelligent interfaces of scientific and cultural mediation
Participants: Laurent Amsaleg, Guillaume Gravier, Guillaume Le Noé-Bienvenu, Duc Hau Nguyen, Pascale Sébillot.
Duration: 3.5 year, started in Dec. 2019
The multidisciplinary and multi-actor ARCHIVAL project aims at yielding collaborations between researchers from the fields of Information and Communication Sciences as well as Computer Sciences around archive value enhancing and knowledge sharing for arts, culture and heritage. The project is structured around the following questionings: What part can machine comprehension methods play towards the reinterpretation of thematic archive collections? How can content mediation interfaces exploit results generated by current AI approaches?
ARCHIVAL teams will explore heterogeneous document collection structuration in order to explicitly reveal implicit links, to explain the nature of these links and to promote them in an intelligible way towards ergonomic mediation interfaces that will guarantee a successful appropriation of contents. A corpus has been delimited from the FMSH “self-management” collection, recently awarded as Collex, which will be completed from the large Canal-U academic audiovisual portal. The analysis and enhancement of this collection is of particular interest for Humanities and Social Sciences in a context where it becomes a necessity to structurally reconsider new models of socioeconomic development (democratic autonomy, social and solidarity-based economy, alternative development, …).
ANR MEERQAT: MultimEdia Entity Representation and Question Answering Tasks
Participants: Laurent Amsaleg, Yannis Avrithis, Ewa Kijak, Shashanka Venkataramanan.
Duration: 3.5 year, started in April 2020 Partners: Inria project-teams Linkmedia, CEA LIST, LIMSI, IRIT.
The overall goal of the project is to tackle the problem of ambiguities of visual and textual content by learning then combining their representations. As a final use case, we propose to solve a Multimedia Question Answering task, that requires to rely on three different sources of information to answer a (textual) question with regard to visual data as well as an external knowledge base containing millions of unique entities, each being represetd by textual and visual content as well as some links to other entities. An important work will deal with the representation of entities into a common tri-modal space, in which one should determine the content to associate to an entity to adequately represent it. The challenge consists in defining a representation that is compact (for performance) while still expressive enough to reflect the potential links between the entity and a variety of others.
MinArm: EVE3
Participants: Teddy Furon.
Duration: 3 year, started in April 2019 Partners: MinArm, CRIStAL Lille, LIRMM, Univ. Troyes, Univ. Paris Saclay
Teaching and technology survey on steganography and steganalysis in the real world.
AID-CNRS: FakeNews
Participants: Vincent Claveau, Ewa Kijak, Gauthier Lyan.
Duration: 2 years, started mid-2021
This AID funded project aims at building tools and concepts to help detect Fake News (incl. deepfake) in social networks. It relies on NLP and multimodal analysis to leverage textual and visual clues of manipulation.ASTRID: HybrInfox
Participants: Vincent Claveau, Guillaume Gravier, Morgane Casanova.
Duration: 20 months, started Jan. 2022
This ANR-AID funded project aims at building exploring how hybridation of symbolic and deep learning NLP tools. These hybrid tools are expected to be used to detect some types of disinformation; in particular, these NLP tools target vagueness (non precise) or subjective (opinion rather than factual) discourses.10 Dissemination
10.1 Promoting scientific activities
10.1.1 Scientific events: organisation
Member of the organizing committees
Participants: Simon Malinowski.
- Simon Malinowski was in the organization committee of the Advanced Analytic and Learning on Temporal Data 2023, co-hosted with ECML/PKDD in September 2023 in Turin, Italy.
10.1.2 Scientific events: selection
Member of the conference program committees
Participants: Laurent Amsaleg, Teddy Furon, Pascale Sébillot.
- Laurent Amsaleg was a PC member of: ACM International Conference on Multimedia, ACM International Conference on Multimedia Retrieval, Multimedia Modeling, Content-Based Multimedia Indexing, IEEE International Conference on Multimedia & Expo, International Conference on Similarity Search and Applications. Laurent Amsaleg was area chair for ACM Multimedia 2023.
- Pascale Sébillot was a PC member of Conférence nationale en intelligence artificielle CNIA 2023.
Reviewer
- Teddy Furon was a reviewer for IEEE Workshop on Information and Security, NeurIPS, AISTAT, IEEE ICASSP
10.1.3 Journal
Participants: Pascale Sébillot, Teddy Furon, Ewa Kijak, Vincent Claveau.
Member of the editorial boards
- Pascale Sébillot was editor of the Journal Traitement automatique des langues (TAL) till June 2023.
- Pascale Sébillot is a member of the editorial board of the Journal Traitement automatique des langues (TAL).
- Vincent Claveau is a member of the editorial board of the Journal Traitement automatique des langues (TAL).
Reviewer - reviewing activities
- Teddy Furon was a reviewer for IEEE Transactions on Dependable and Secure Computing, ACM Transactions on Multimedia Computing, Communications and Applications, IEEE Transactions on Pattern Analysis and Machine Intelligence, IEEE Transactions on Information Forensics and Security.
- Ewa Kijak was a reviewer for IEEE Transactions on Information Forensics and Security, International Journal of Multimedia Information Retrieval.
10.1.4 Invited talks
Participants: Teddy Furon, Ewa Kijak.
- Teddy Furon was an invited speaker to the following seminars `Souveraineté numérique, Cyber & IA' day, internal seminar of PRA Lab of Universit of Cagliari (Italy), groupe de travail `Statistics and Security', rencontre Inria FADEX, Qualcomm internal seminar, `La cyber au rendez-vous de l'IA de confiance' day.
- Ewa Kijak gave an invited talk about 'Improving data representation learning and generation' for the scientific seminar of PUC Minas (Brazil)
10.1.5 Leadership within the scientific community
Participants: Laurent Amsaleg, Teddy Furon, Guillaume Gravier, Pascale Sébillot.
- Laurent Amsaleg is a member of the Steering Committee of ACM Multimedia for the 2020-2023 term.
- Teddy Furon is a member of the Steering Committee of the Seminar SoSySec, and the seminar `Statistiques et Sécurité'.
- Guillaume Gravier is a member of the scientific board of the GDR Traitement automatique des langues.
- Pascale Sébillot is a member of the board of the GDR Traitement automatique des langues.
10.1.6 Scientific expertise
Participants: Teddy Furon.
- Teddy Furon was a reviewer for Region Normandie thesis funding,
10.1.7 Research administration
Participants: Teddy Furon, Guillaume Gravier, Pascale Sébillot.
- Guillaume Gravier is director of IRISA (UMR 6074).
- Pascale Sébillot is deputy director of IRISA.
- Teddy Furon is a member of the Commission du personnel IRISA, and head of the commission des délégations Inria.
10.2 Teaching - Supervision - Juries
10.2.1 Teaching
Participants: Teddy Furon, Ewa Kijak, Laurent Amsaleg, Guillaume Gravier, Pascale Sébillot.
- Master: Laurent Amsaleg, Bases de données avancées, 25h, M2, INSA Rennes, France
- Master: Teddy Furon, Rare Event Simulations, 40h, INSA Rennes, France
- Licence: Guillaume Gravier, Natural language processing, 12h, L3, INSA Rennes
- Licence: Guillaume Gravier, Markov models, 6h, L3, INSA Rennes
- Master: Guillaume Gravier, Natural Language Processing, 6h, M1, INSA Rennes
- Master: Guillaume Gravier, Natural Language Processing, 51h, M2, ENSAI
- Master: Pascale Sébillot, Natural Language Processing, 4h, M1, INSA Rennes, France
- Master: Pascale Sébillot, Databases, 18h, M1, DIGISPORT graduate school (EUR), France
- Licence: Pascale Sébillot, Natural Language Processing, 6h, L3, INSA Rennes, France
- Ewa Kijak is head of the Image engineering track (M1-M2) of ESIR, Univ. Rennes
- Master: Ewa Kijak, Supervised machine learning, 15h, M2R, Univ. Rennes
- Master: Ewa Kijak, Image retrieval, 12h, M2, ESIR
- Master: Ewa Kijak, Image classification, 27h, M1, ESIR
- Master: Ewa Kijak, Image processing, 45h, M1, ESIR, Univ. Rennes
10.2.2 Supervision
Participants: Teddy Furon, Ewa Kijak, Laurent Amsaleg, Guillaume Gravier, Pascale Sébillot, Simon Malinowski.
- PhD in progress: Shashanka Venkataramanan, Metric learning for instance- and category-level visual representations. Started in Dec. 2020. Yannis Avrithis, Ewa Kijak, and Laurent Amsaleg
- PhD in progress: Gautier Evennou, Detection and explanation of semantic manipulations in multimedia content. Started in Sep. 2023, Ewa Kijak
- PhD in progress: Louis Hemadou, Domain generalization exploiting synthetic data. Started Nov. 2022, Ewa Kijak
- PhD in progress: Mohamed Younes, Learning and simulating strategies in sports for VR training. Started Dec. 2020, Ewa Kijak, Simon Malinowski and Franck Multon (MIMETIC Team at IRISA)
- PhD in progress: Ahmed Abdourahman, AI-driven character simulation based on Multi-Agents Interaction Imitation Learning. Started Dec. 2023, Ewa Kijak and Franck Multon (MIMETIC Team at IRISA)
- PhD in progress: Deniz Engin, Video Query Answering. Started in Sept. 2020, Yannis Avrithis and Teddy Furon
- PhD in progress: Pierre Fernandez, Watermarking and machine learning. Started in Sept. 2021, Teddy Furon
- PhD in progress: Quentin Le Roux, Backdoors on face recognition systems. Started in Sept. 2021, Kassem Kallas and Teddy Furon
- PhD in progress: Duc Hau Nguyen, Making AI understandable for humans: the plausibility of attention-based mechanisms in natural language processing. Started in Sept. 2020, Pascale Sébillot and Guillaume Gravier
- PhD in progress: Hugo Thomas, Zero-shot and few shot relation extraction in press archives. Started in Sept. 2022, Pascale Sébillot and Guillaume Gravier
- PhD in progress: Erwan Vincent, Machine learning for the identification of factors impacting the quality of service of urban buses. Started in Feb. 2022. Simon Malinowski and Guillaume Gravier
- PhD in progress: Carolina Jeronimo, Machine learning for temporal graphs. Started in Sept. 2022. Simon Malinowski and Guillaume Gravier
- PhD in progress: Florent Meyer, Introduction of rejection capabilities and externalized language models in deep learning systems for text reading under adverse conditions. Started in June 2023, Guillaume Gravier and Bertrand Couasnon (SHADOC team at IRISA)
- PhD in progress: Paul Estano, Dynamic-Precision Training of Deep Neural Networks on the Edge. Started in Feb. 2022, Guillaume Gravier, Steven Derrien (TARAN team at IRISA), Silviu-Ioan Filip (TARAN)
- PhD in progress: Karim Tit, Robustness assessment of deep neural networks. Started Feb. 2021. Teddy Furon (with Mathias Rousset, team-project SIMSMART)
- PhD. Benoit Bonnet, Understanding, taming, and defending from adversarial examples. Defended Feb 2023. Teddy Furon (with Patrick Bas, CNRS CRIsTAL, Lille)
- PhD. Samuel Tap, Homomorphic encryption for machine learning. Defended Dec. 2023, Teddy Furon
- PhD. Thibault Maho, Machine learning vulnerabilities in real world settings. Defended Dec. 2023, Teddy Furon and Erwan Le Merrer
- PhD. Antoine Chaffin, Multimodal misinformation detection: Overcoming the training data collection challenge through data generation. Defended Nov. 2023, Ewa Kijak and Vincent Claveau
10.2.3 Juries
Participants: Teddy Furon, Ewa Kijak, Laurent Amsaleg, Pascale Sébillot.
- Laurent Amsaleg was a jury member for the PhD. of Victor Pellegrain, Univ. Paris-Saclay, July 2023.
- Teddy Furon was a jury member for the HDR of François Cayre, Univ. Grenoble, July 2023.
- Pascale Sébillot was a jury member for the HDR of Cyril Grouin, Univ. Paris-Saclay, March 2023.
- Pascale Sébillot was reviewer for the PhD. of Guillaume Le Berre, Univ. de Lorraine, and Univ. de Montréal, June 2023.
- Ewa Kijak was a jury member for the PhD. of Jianan CHEN, Univ. Rennes, October 2023.
- Ewa Kijak was a jury member for the PhD. of Paul LERNER, Université Paris-Saclay, November 2023.
- Ewa Kijak was reviewer for the PhD. of Emmanuelle SALIN, Université Aix-Marseille, November 2023.
10.3 Popularization
Participants: Laurent Amsaleg, Teddy Furon, Guillaume Gravier.
10.3.1 Education
- L. Amsaleg was involved into the "Chiche" program with 6 classes at the Lycée Saint joseph, Bruz.
10.3.2 Interventions
- L. Amsaleg conducted a few general science outreach sessions about ML, "Musée d'art et d'histoire, Cholet", Sept 2023.
- Teddy Furon was interviewed in the podcast "Thèse ? Antithèse ? Synthèse !".
- Guillaume Gravier was an invited panelist on AI opportuinities and threats at Imagine Summit, Rennes, France and at the general assembly of MEDEF 35.
11 Scientific production
11.1 Major publications
- 1 inproceedingsThree bricks to consolidate watermarks for large language models.Proceedings of IEEE WIFSWIFS 2023 - IEEE International Workshop on Information Forensics and SecurityNuremberg, GermanyIEEEDecember 2023, 1-9HAL
- 2 inproceedingsThe Stable Signature: Rooting Watermarks in Latent Diffusion Models.2023 IEEE International Conference on Computer Vision (ICCV)ICCV 2023 - International Conference on Computer Vision2023 IEEE International Conference on Computer VisionParis, FranceOctober 2023HAL
11.2 Publications of the year
International journals
International peer-reviewed conferences
National peer-reviewed Conferences
Edition (books, proceedings, special issue of a journal)
Doctoral dissertations and habilitation theses
Reports & preprints
11.3 Other
11.4 Cited publications
- 27 inproceedingsThe Vulnerability of Learning to Adversarial Perturbation Increases with Intrinsic Dimensionality.WIFS2017HALback to text
- 28 inproceedingsEstimating Local Intrinsic Dimensionality.KDD2015HALback to textback to textback to text
- 29 articlePrototyping a Web-Scale Multimedia Retrieval Service Using Spark.ACM TOMCCAP143s2018HALback to text
- 30 inproceedingsScalability of the NV-tree: Three Experiments.SISAP2018HALback to text
- 31 inproceedingsSketching techniques for very large matrix factorization.ECIR2016HALback to text
- 32 inproceedingsConstraint Satisfaction Programming for Video Summarization.ISM2013back to text
- 33 articleWild Patterns: Ten Years After the Rise of Adversarial Machine Learning.Pattern Recognition2018back to text
- 34 phdthesisImage indexing and retrieval using component trees.Université de Bretagne Sud2016HALback to text
- 35 phdthesisA storytelling machine? : Automatic video summarization: the case of TV series.University of Avignon, France2016back to text
- 36 inproceedingsA Study on Multimodal Video Hyperlinking with Visual Aggregation.ICME2018back to text
- 37 inproceedingsTime Series Retrieval using DTW-Preserving Shapelets.SISAP2018HALback to text
- 38 articleAudio Adversarial Examples: Targeted Attacks on Speech-to-Text.CoRRabs/1801.019442018back to text
- 39 inproceedingsNumerical eligibility criteria in clinical protocols: annotation, automatic detection and interpretation.AIME2017HALback to text
- 40 inproceedingsImage Retrieval with Reciprocal and shared Nearest Neighbors.VISAPP2014HALback to text
- 41 inproceedingsUsing Knowledge Base Semantics in Context-Aware Entity Linking.DocEng 2019 - 19th ACM Symposium on Document EngineeringBerlin, GermanyACMSeptember 2019, 1-10HALDOIback to textback to text
- 42 bookPhoto Forensics.The MIT Press2016back to text
- 43 articleRecent automatic text summarization techniques: a survey.Artif. Intell. Rev.4712017back to text
- 44 bookDeep Learning.MIT Press2016back to text
- 45 inproceedingsShaping-Up Multimedia Analytics: Needs and Expectations of Media Professionals.MMM, Special Session Perspectives on Multimedia Analytics2016HALback to text
- 46 inproceedingsScaling Group Testing Similarity Search.ICMR2016HALback to text
- 47 inproceedingsMining on Manifolds: Metric Learning without Labels.CVPR2018HALback to textback to textback to textback to text
- 48 inproceedingsA Multi-Dimensional Data Model for Personal Photo Browsing.MMM2015HALback to text
- 49 inproceedingsTen Research Questions for Scalable Multimedia Analytics.MMM, Special Session Perspectives on Multimedia Analytics2016HALback to text
- 50 articleDeep Video Portraits.ACM TOG2018back to text
- 51 inproceedingsActive Learning to Assist Annotation of Aerial Images in Environmental Surveys.CBMI2018back to text
- 52 articleIamNN: Iterative and Adaptive Mobile Neural Network for Efficient Image Classification.CoRRabs/1804.101232018back to text
- 53 inproceedingsLearning DTW-Preserving Shapelets.IDA2017HALback to text
- 54 inproceedingsContext-Aware Forgery Localization in Social-Media Images: A Feature-Based Approach Evaluation.ICIP2018back to text
- 55 inproceedingsConnecting the dots between news articles.KDD2010back to text
- 56 inproceedingsWeakly Supervised Object Localization Using Things and Stuff Transfer.ICCV2017back to text
- 57 inproceedingsUnsupervised part learning for visual recognition.CVPR2017HALback to text
- 58 inproceedingsMemory Vectors for Particular Object Retrieval with Multiple Queries.ICMR2015HALback to text
- 59 inproceedingsProvenance filtering for multimedia phylogeny.ICIP2017back to text
- 60 inproceedingsUnsupervised Object Discovery for Instance Recognition.WACV2018HALback to textback to text
- 61 inproceedingsDeep Metric Learning via Lifted Structured Feature Embedding.CVPR2016back to text
- 62 inproceedingsHighly Efficient Multimedia Event Recounting from User Semantic Preferences.ICMR2014back to textback to text
- 63 articleShow and Tell: Lessons Learned from the 2015 MSCOCO Image Captioning Challenge.TPAMI3942017back to text
- 64 phdthesisDeep Neural Architectures for Automatic Representation Learning from Multimedia Multimodal Data.INSA de Rennes2017HALback to textback to text
- 65 inproceedingsBidirectional Joint Representation Learning with Symmetrical Deep Neural Networks for Multimodal and Crossmodal Applications.ICMR2016HALback to text
- 66 inproceedingsGenerative Adversarial Networks for Multimodal Representation Learning in Video Hyperlinking.ICMR2017HALback to textback to text
- 67 articleMemory Networks.CoRRabs/1410.39162014back to text
- 68 inproceedingsVideo Paragraph Captioning Using Hierarchical Recurrent Neural Networks.CVPR2016back to text
- 69 inproceedingsTowards interactive, intelligent, and integrated multimedia analytics.VAST2014back to text
- 70 inproceedingsCrowd Counting via Scale-Adaptive Convolutional Neural Network.WACV2018HALback to text
- 71 articleShuffleNet: An Extremely Efficient Convolutional Neural Network for Mobile Devices.CoRRabs/1707.010832017back to text