2023Activity reportProject-TeamMORPHEO
RNSR: 201120981M- Research center Inria Centre at Université Grenoble Alpes
- In partnership with:CNRS, Université de Grenoble Alpes
- Team name: Capture and Analysis of Shapes in Motion
- In collaboration with:Laboratoire Jean Kuntzmann (LJK)
- Domain:Perception, Cognition and Interaction
- Theme:Vision, perception and multimedia interpretation
Keywords
Computer Science and Digital Science
- A5.1.8. 3D User Interfaces
- A5.4. Computer vision
- A5.4.4. 3D and spatio-temporal reconstruction
- A5.4.5. Object tracking and motion analysis
- A5.5.1. Geometrical modeling
- A5.5.4. Animation
- A5.6. Virtual reality, augmented reality
- A6.2.8. Computational geometry and meshes
Other Research Topics and Application Domains
- B2.6.3. Biological Imaging
- B2.7.2. Health monitoring systems
- B2.8. Sports, performance, motor skills
- B9.2.2. Cinema, Television
- B9.2.3. Video games
- B9.4. Sports
1 Team members, visitors, external collaborators
Research Scientist
- Stefanie Wuhrer [INRIA, Researcher, HDR]
Faculty Members
- Jean Franco [Team leader, Grenoble INP Ensimag, Associate Professor, HDR]
- Sergi PUJADES [UGA, Associate Professor]
PhD Students
- Nicolas Comte [Anatoscope, CIFRE, from May 2023]
- Nicolas Comte [Inria, Anatoscope, CIFRE, until Apr 2023]
- Antoine Dumoulin [INRIA, from Nov 2023]
- Anilkumar Erappanakoppal Swamy [NAVER LABS]
- Vicente Estopier Castillo [INSERM, from Sep 2023]
- Mathieu Marsot [INRIA, until Apr 2023]
- Aymen Merrouche [INRIA]
- Rim Rekik Dit Nekhili [INRIA]
- Briac Toussaint [UGA]
- Pierre Zins [INRIA, until Mar 2023]
Technical Staff
- Vaibhav Arora [INRIA, Engineer, from May 2023]
- Léo Challier [LJK, Engineer, from Oct 2023]
- Shivam Chandhok [UGA, Engineer, until Sep 2023]
- Mohammed Chekroun [INRIA, Engineer, from Dec 2023]
- Abdelmouttaleb Dakri [INRIA, Engineer]
- Maxime Genisson [INRIA, Engineer, until Sep 2023]
- Samara Ghrer [UGA, Engineer, from Aug 2023]
Interns and Apprentices
- Samara Ghrer [INRIA, Intern, from Feb 2023 until Aug 2023]
- Bhaswar Gupta [INRIA, Intern, from Mar 2023 until Sep 2023]
- Xuan Li [INRIA, Intern, until Jun 2023]
- Anne-Flore Mailland [INRIA, Intern, from Jul 2023 until Aug 2023]
- Anne-Flore Mailland [UGA, Intern, from Feb 2023 until Jul 2023]
- Gagan Mundada [INRIA, Intern, from May 2023 until Jul 2023]
- Hector Piteau [INRIA, Intern, from Feb 2023 until Aug 2023]
- Leticia Itzel Rivera Contreras [INRIA, Intern, from Feb 2023 until Aug 2023]
Administrative Assistant
- Nathalie Gillot [INRIA]
2 Overall objectives
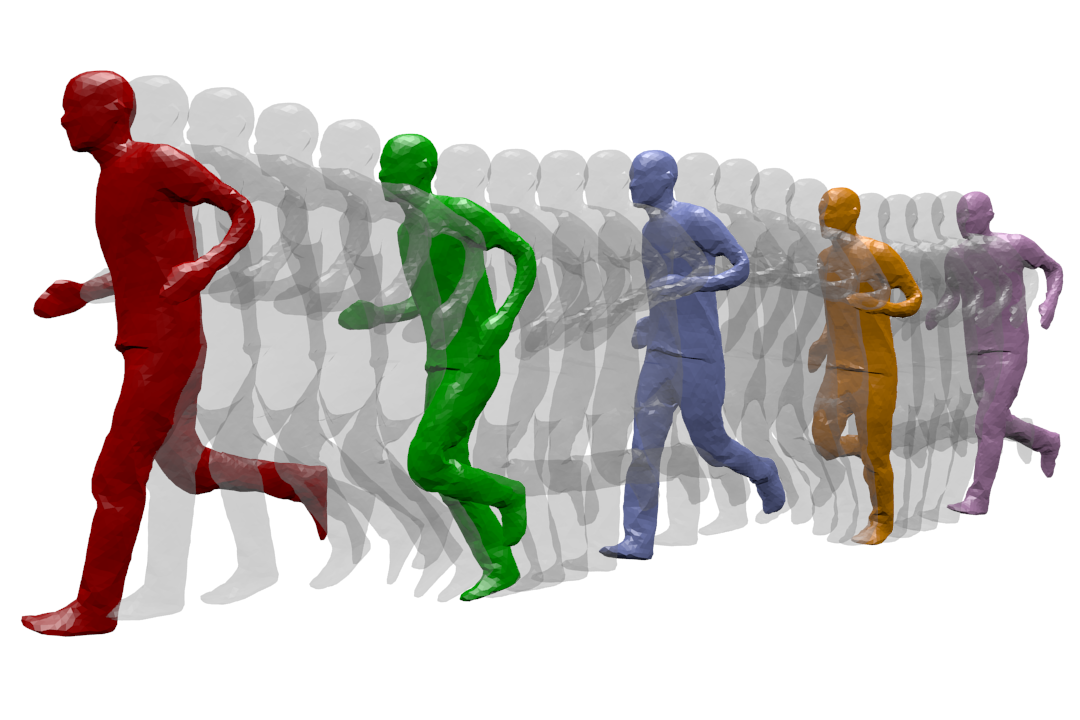
Dynamic Geometry Modeling: a human is captured while running. The different temporal acquisition instances are shown on the same figure.
MORPHEO's ambition is to perceive and interpret shapes that move using multiple camera systems. Departing from standard motion capture systems, based on markers, that provide only sparse information on moving shapes, multiple camera systems allow dense information on both shapes and their motion to be recovered from visual cues. Such ability to perceive shapes in motion brings a rich domain for research investigations on how to model, understand and animate real dynamic shapes, and finds applications, for instance, in gait analysis, bio-metric and bio-mechanical analysis, animation, games and, more insistently in recent years, in the virtual and augmented reality domain. The MORPHEO team particularly focuses on four different axes within the overall theme of 3D dynamic scene vision or 4D vision:
- Shape and appearance models: how to build precise geometric and photometric models of shapes, including human bodies but not limited to, given temporal sequences.
- Dynamic shape vision: how to register and track moving shapes, build pose spaces and animate captured shapes.
- Inside shape vision: how to capture and model inside parts of moving shapes using combined color and X-ray imaging.
- Shape animation: Morpheo is actively investigating animation acquisition and parameterization methodologies for efficient representation and manipulability of acquired 4D data.
The strategy developed by Morpheo to address the mentioned challenges is based on methodological tools that include in particular learning-based approaches, geometry, Bayesian inference and numerical optimization. In recent years, and following many successes in the team and the computer vision community as a whole, a particular effort is ongoing in the team to investigate the use of machine learning and neural learning tools in 4D vision.
3 Research program
3.1 Shape and Appearance Modeling
Standard acquisition platforms, including commercial solutions proposed by companies such as Microsoft, 3dMD or 4DViews, now give access to precise 3D models with geometry, e.g. meshes, and appearance information, e.g. textures. Still, state-of-the-art solutions are limited in many respects: They generally consider limited contexts and close setups with typically at most a few meter side lengths. As a result, many dynamic scenes, even a body running sequence, are still challenging situations; They also seldom exploit time redundancy; Additionally, data driven strategies are yet to be fully investigated in the field. The MORPHEO team builds on the Kinovis platform for data acquisition and has addressed these issues with, in particular, contributions on time integration, in order to increase the resolution for both shapes and appearances, on representations, as well as on exploiting machine learning tools when modeling dynamic scenes. Our originality lies, for a large part, in the larger scale of the dynamic scenes we consider as well as in the time super resolution strategy we investigate. Another particularity of our research is a strong experimental foundation with the multiple camera Kinovis platforms.
3.2 Dynamic Shape Vision
Dynamic Shape Vision refers to research themes that consider the motion of dynamic shapes, with e.g. shapes in different poses, or the deformation between different shapes, with e.g. different human bodies. This includes for instance shape tracking and shape registration, which are themes covered by MORPHEO. While progress has been made over the last decade in this domain, challenges remain, in particular due to the required essential task of shape correspondence that is still difficult to perform robustly. Strategies in this domain can be roughly classified into two categories: (i) data driven approaches that learn shape spaces and estimate shapes and their variations through space parameterizations; (ii) model based approaches that use more or less constrained prior models on shape evolutions, e.g. locally rigid structures, to recover correspondences. The MORPHEO team is substantially involved in both categories. The second one leaves more flexibility for shapes that can be modeled, an important feature with the Kinovis platform, while the first one is interesting for modeling classes of shapes that are more likely to evolve in spaces with reasonable dimensions, such as faces and bodies under clothing. The originality of MORPHEO in this axis is to go beyond static shape poses and to consider also the dynamics of shape over several frames when modeling moving shapes, and in particular with shape tracking and animation.
3.3 Inside Shape Vision
Another research axis is concerned with the ability to perceive inside moving shapes. This is a more recent research theme in the MORPHEO team that has gained importance. It was originally the research associated to the Kinovis platform installed in the Grenoble Hospitals. This platform is equipped with two X-ray cameras and ten color cameras, enabling therefore simultaneous vision of inside and outside shapes. We believe this opens a new domain of investigation at the interface between computer vision and medical imaging. Interesting issues in this domain include the links between the outside surface of a shape and its inner parts, especially with the human body. These links are likely to help understanding and modeling human motions. Until now, numerous dynamic shape models, especially in the computer graphics domain, consist of a surface, typically a mesh, rigged to a skeletal structure that is never observed in practice but allows to parameterize human motion. Learning more accurate relationships using observations can therefore significantly impact the domain.
3.4 Shape Animation
3D animation is a crucial part of digital media production with numerous applications, in particular in the game and motion picture industry. Recent evolutions in computer animation consider real videos for both the creation and the animation of characters. The advantage of this strategy is twofold: it reduces the creation cost and increases realism by considering only real data. Furthermore, it allows to create new motions, for real characters, by recombining recorded elementary movements. In addition to enable new media contents to be produced, it also allows to automatically extend moving shape datasets with fully controllable new motions. This ability appears to be of great importance with deep learning techniques and the associated need for large learning datasets. In this research direction, we investigate how to create new dynamic scenes using recorded events. More recently, this also includes applying machine learning to datasets of recorded human motions to learn motion spaces that allow to synthesize novel realistic animations.
4 Application domains
4.1 4D modeling
Modeling shapes that evolve over time, analyzing and interpreting their motion has been a subject of increasing interest of many research communities including the computer vision, the computer graphics and the medical imaging communities. Recent evolutions in acquisition technologies including 3D depth cameras (Time-of-Flight and Kinect), multi-camera systems, marker based motion capture systems, ultrasound and CT scanners have made those communities consider capturing the real scene and their dynamics, create 4D spatio-temporal models, analyze and interpret them. A number of applications including dense motion capture, dynamic shape modeling and animation, temporally consistent 3D reconstruction, motion analysis and interpretation have therefore emerged.
4.2 Shape Analysis
Most existing shape analysis tools are local, in the sense that they give local insight about an object's geometry or purpose. The use of both geometry and motion cues makes it possible to recover more global information, in order to get extensive knowledge about a shape. For instance, motion can help to decompose a 3D model of a character into semantically significant parts, such as legs, arms, torso and head. Possible applications of such high-level shape understanding include accurate feature computation, comparison between models to detect defects or medical pathologies, and the design of new biometric models.
4.3 Human Motion Analysis
The recovery of dense motion information enables the combined analysis of shapes and their motions. This allows to classify based on motion cues, which can help in the identification of pathologies or the design of new prostheses. Analyzing human motion can also be used to learn generative models of realistic human motion, which is a recent research topic within Morpheo.
5 Social and environmental responsibility
5.1 Footprint of research activities
The footprint of our research activity is dominated by dissemination and data costs.
Dissemination strategy: Traditionally, Morpheo's dissemination strategy has been to publish in the top conferences of the field (CVPR, ECCV, ICCV, MICCAI), as well as to give invited talks, leading to some long disance work trips. We currently try to increase submissions to top journals in the field directly, hence reducing travels, while still attending some in-person conferences or seminars to allow for networking.
Data management: The data produced by the Morpheo team occupies large memory volumes. The Kinovis platform typically produces around 1,5GB per second when capturing one actor at 50fps. The platform that also captures X-ray images at CHU produces around 1.3GB of data per second at 30fps for video and X-rays. For practical reasons, we reduce the data as much as possible with respect to the targeted application by only keeping e.g. 3D reconstructions or down-sampled spatial or temporal camera images. Yet, acquiring, processing and storing these data is costly in terms of resources. In addition, the data captured by these platforms are personal data with highly constrained regulations. Our data management therefore needs to consider multiple aspects: data encryption to protect personal information, data backup to allow for reproducibility, and the environmental impact of data storage and processing. For all these aspects, we are constantly checking for new and more satisfactory solutions.
For data processing, we rely heavily on cluster uses, in particular with neural networks which are known to have a heavy carbon footprint. Yet, in our research field, these types of processing methods have been shown to lead to significant performance gains. For this reason, we continue to use neural networks as tools, while attempting to use architectures that allow for faster and more energy efficient training, and simple test cases that can often be trained on local machines (with GPU) to allow for less costly network design. A typical example of this type of research is the work of Briac Toussaint, whose first thesis contribution exhibits a high quality reconstruction algorithm for the Kinovis platform, with under a minute of GPU computation time per frame, an order of magnitude faster than our previous research solution, yet it achieves improved precision.
5.2 Impact of research results
Morpheo's main research topic is not related to sustainability. Yet, some of our research directions could be applied to solve problems relevant to sustainability.
Realistic digital human modeling holds the potential to allow for realistic interactions through large geographic distances, leading to more realistic and satisfactory teleconferencing systems. Morpheo captures and analyzes humans in 4D, thereby allowing to capture both their shapes and movement patterns, and actively works on human body modeling. In this line of work, Morpheo works on the project 3DMOVE to model realistic human motion, e.g. 20, and participates in the Nemo.AI joint lab with Interdigital on advancing this topic.
Modeling and analyzing humans in clothing holds the potential to reduce the rate of returns of online sales in the clothing industry, which are currently high. Morpheo's interest includes the analysis of clothing deformations based on 4D captures. This year, we have published a large-scale dataset of humans in different clothing items performing different motions in collaboration with NaverLabs Research 6. This dataset allows the research community to study the dynamic effects of clothing.
Of course, as with any research direction, ours can also be used to generate technologies that are resource-hungry and whose necessity may be questionable, such as inserting animated 3D avatars in scenarios where simple voice communication would suffice, for instance.
6 Highlights of the year
6.1 Awards
- Sergi Pujades and co-authors received a best paper runner-up award at Siggraph Asia 2023, for their paper 8. (9 awardees out of >650 submissions)
- Nicolas Comte, Sergi Pujades, Jean-Sébastien Franco, Edmond Boyer and co-authors received a best poster presentation award at IABM 2023 for their communication on 18 (2 awardees out of 100 submissions)
- Sergi Pujades and co-authors received a "Best in Physics" award by the American Association of Physics in Medicine (AAPM) for their communication 21 - (top 15 abstracts out of >2200 submissions)
7 New software, platforms, open data
7.1 New software
7.1.1 DGUShapeMatching
-
Name:
Deformation-Guided Unsupervised Non-Rigid Shape Matching
-
Keywords:
Geometry Processing, 3D, Shape recognition
-
Functional Description:
Code allows to reproduce the results presented in scientific publication:
Aymen Merrouche, João Regateiro, Stefanie Wuhrer, Edmond Boyer. Deformation-Guided Unsupervised Non-Rigid Shape Matching. BMVC 2023 - 34th British Machine Vision Conference, Nov 2023, Aberdeen, United Kingdom.
- URL:
-
Contact:
Aymen Merrouche
7.1.2 Multi-View SRDF
-
Name:
Multi-View Reconstruction using Signed Ray Distance Functions (SRDF)
-
Keywords:
3D, 3D reconstruction
-
Functional Description:
Software for 3D reconstruction of shapes from multiple images. Code allows to reconstruct the results in: Pierre Zins, Yuanlu Xu, Edmond Boyer, Stefanie Wuhrer, Tony Tung. Multi-View Reconstruction using Signed Ray Distance Functions (SRDF). CVPR 2023.
- URL:
- Publication:
-
Contact:
Pierre Zins
7.1.3 OSSO
-
Name:
Obtainig skeletal Shape from Outside
-
Keyword:
3D modeling
-
Functional Description:
Given the shape of a person as a SMPL body mesh, OSSO extracts the mesh of the corresponding skeletal structure
- URL:
-
Contact:
Sergi PUJADES
-
Partner:
Max Planck Institute for Intelligent Systems
7.1.4 VertSegId
-
Keywords:
Image segmentation, Medical imaging, 3D
-
Functional Description:
This software segments and identifies vertebrae in a volumetric CT image.
- URL:
-
Contact:
Sergi PUJADES
7.1.5 FastCapDR
-
Name:
Fast Differentiable Rendering based Surface Capture
-
Keywords:
3D reconstruction, 3D, Differentiable Rendering, Synthetic human, Computer vision, Multi-View reconstruction
-
Scientific Description:
Code to the article "Fast Gradient Descent for Surface Capture with Differentiable Rendering", 3DV 2022
-
Functional Description:
This software combines differentiable rendering with sparse coarse-to-fine estimation and storage techniques on GPU to obtain a unique combination of high precision captured surfaces and maximal efficiency, yielding excellent reconstruction results in under a minute. Very high quality single-human surface reconstructions were obtained in less than 20 seconds for 68 cameras of the Inria Kinovis acquisition platform (with a single NVidia RTX A6000 GPUs).
- URL:
-
Contact:
Briac Toussaint
7.1.6 HumanCompletePST
-
Name:
Pyramidal Signed Distance Learning for Spatio-Temporal Human Shape Completion
-
Keywords:
3D reconstruction, 4D, Point cloud, Depth Perception, 3D
-
Scientific Description:
This repository contains the code of the paper Pyramidal Signed Distance Learning for Spatio-Temporal Human Shape Completion, published at ACCV2022.
-
Functional Description:
This software processes sequences of monocular, single-view obtained point clouds of humans, and provides a closed shape mesh as output for each frame. Analysis is performed with a pyramidal, semi-implicit depth inference deep network, on temporal windows of typically 4-8 frames.
- URL:
-
Contact:
Jean Franco
7.1.7 KinovisCalibration
-
Name:
Multi-view calibration software for the Kinovis platform
-
Keywords:
3D, Computer vision, Calibration
-
Functional Description:
Multi-camera calibration software specialized in the management of large multi-camera platforms, based on a T-shaped calibration stick.
- URL:
-
Contact:
Julien Pansiot
7.1.8 KinovisReconstruction
-
Name:
Differential rendering based surface reconstruction for the Kinovis platform
-
Keywords:
3D, 4D, Computer vision, Differentiable Rendering, 3D reconstruction, Multi-View reconstruction
-
Functional Description:
Very fast reconstruction and rendering software based on neural inverse differential rendering.
- URL:
-
Contact:
Briac Toussaint
7.1.9 Representing 3D human motion as a sequence of latent primitives
-
Name:
Representing 3D human motion as a sequence of latent primitives
-
Keyword:
3D
-
Functional Description:
Code that allows to represent a 4D human motion sequence in a latent space as a sequence of vectors. Code used to produce the results described in https://arxiv.org/abs/2206.13142.
- URL:
-
Contact:
Mathieu Marsot
7.1.10 SKEL
-
Name:
From Skin to Skeleton: Towards Biomechanically Accurate 3D Digital Humans
-
Keywords:
Digital twin, Biomechanics, 3D movement
-
Functional Description:
The software allows to generate 3D biomechanical skeletons from 3D skin surface observations. This software is associate with the publication "From Skin to Skeleton: Towards Biomechanically Accurate 3D Digital Humans" Sigg Asia 2023 (https://skel.is.tue.mpg.de)
- URL:
-
Contact:
Sergi PUJADES
-
Partners:
Max Planck Institute for Intelligent Systems, Stanford University
7.1.11 KinovisEnvironment
-
Name:
Video and 3D data processing environment
-
Keywords:
3D, Videos
-
Functional Description:
Complete environment to automatically process data from the Kinovis platform: copy, demosaicing, camera calibration, reconstruction, post-processing, verification, archiving, etc.
-
Contact:
Julien Pansiot
7.2 Open data
7.2.1 4DHumanOutfit
In collaboration with NaverLabs Europe and Inria SED, Morpheo team captured and published a large dataset of 4D human motion sequences of varying actors performing varying motions while wearing different outfits. The data is described in a journal article 6 and available at https://kinovis.inria.fr/4dhumanoutfit/.
Contact: Laurence Boissieux
8 New results
8.1 4DHumanOutfit: a multi-subject 4D dataset of human motion sequences in varying outfits exhibiting large displacements
Participants: Matthieu Armando, Laurence Boissieux, Edmond Boyer, Jean-Sébastien Franco, Martin Humenberger, Christophe Legras, Vincent Leroy, Mathieu Marsot, Julien Pansiot, Sergi Pujades, Rim Rekik, Grégory Rogez, Anilkumar Swamy, Stefanie Wuhrer.
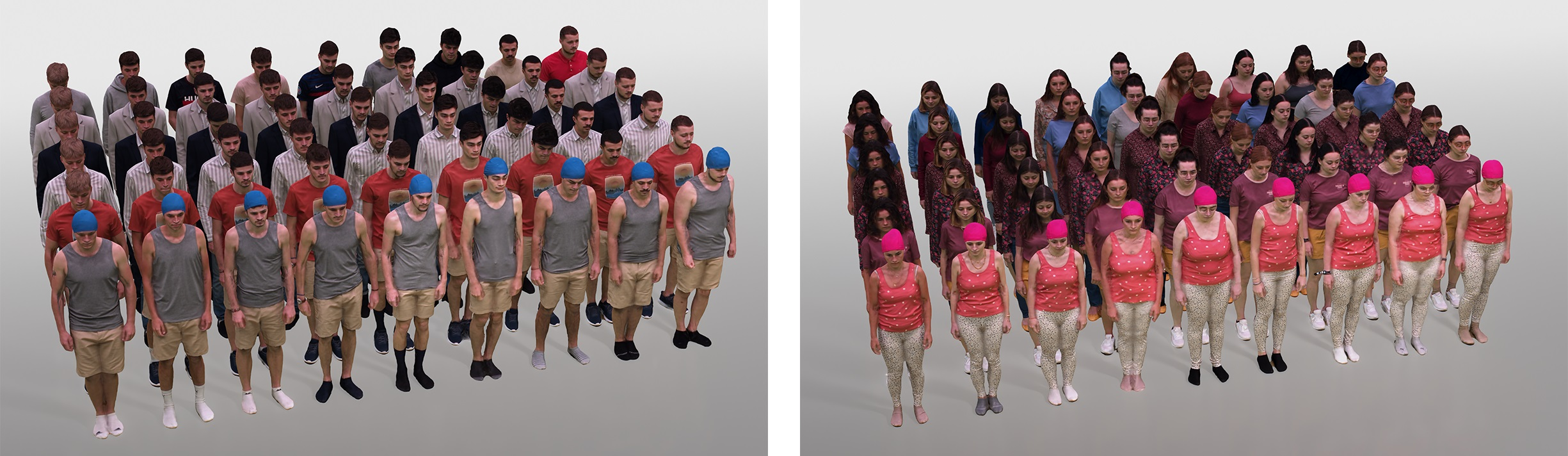
In this figure, we show the identity and outfit axes of the 4DHumanOutfit dataset.
4DHumanOutfit is a new dataset of densely sampled spatio-temporal 4D human motion data of different actors, outfits and motions. The dataset is designed to contain different actors wearing different outfits while performing different motions in each outfit. In this way, the dataset can be seen as a cube of data containing 4D motion sequences along 3 axes with identity, outfit and motion. This rich dataset has numerous potential applications for the processing and creation of digital humans, e.g. augmented reality, avatar creation and virtual try on. 4DHumanOutfit is released for research purposes at kinovis.inria.fr/4dhumanoutfit/. In addition to image data and 4D reconstructions, the dataset includes reference solutions for each axis. We present independent baselines along each axis that demonstrate the value of these reference solutions for evaluation tasks. This work was published in the journal Computer Vision and Image Understanding 6.
8.2 Multi-View Reconstruction using Signed Ray Distance Functions (SRDF)
Participants: Pierre Zins, Yuanlu Xu, Edmond Boyer, Stefanie Wuhrer, Tony Tung.
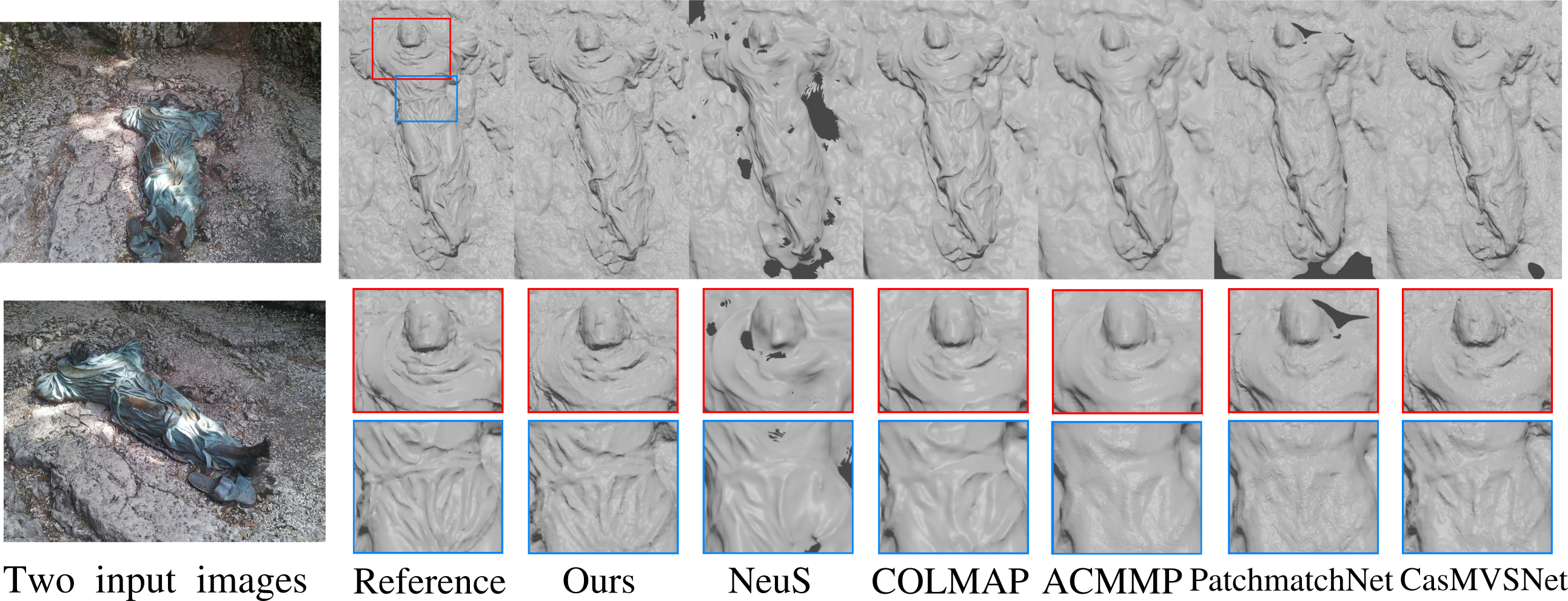
Reconstructions with various methods using 14 images of a model from BlendedMVS.
We investigate a new optimization framework for multi-view 3D shape reconstructions. Recent differentiable rendering approaches have provided breakthrough performances with implicit shape representations though they can still lack precision in the estimated geometries. On the other hand multi-view stereo methods can yield pixel wise geometric accuracy with local depth predictions along viewing rays. Our approach bridges the gap between the two strategies with a novel volumetric shape representation that is implicit but parameterized with pixel depths to better materialize the shape surface with consistent signed distances along viewing rays. The approach retains pixel-accuracy while benefiting from volumetric integration in the optimization. To this aim, depths are optimized by evaluating, at each 3D location within the volumetric discretization, the agreement between the depth prediction consistency and the photometric consistency for the corresponding pixels. The optimization is agnostic to the associated photo-consistency term which can vary from a median-based baseline to more elaborate criteria e.g. learned functions. Our experiments demonstrate the benefit of the volumetric integration with depth predictions. They also show that our approach outperforms existing approaches over standard 3D benchmarks with better geometry estimations. This work was published at the Conference on Computer Vision and Pattern Recognition 14.
8.3 Deformation-Guided Unsupervised Non-Rigid Shape Matching
Participants: Aymen Merrouche, João Regateiro, Stefanie Wuhrer, Edmond Boyer.
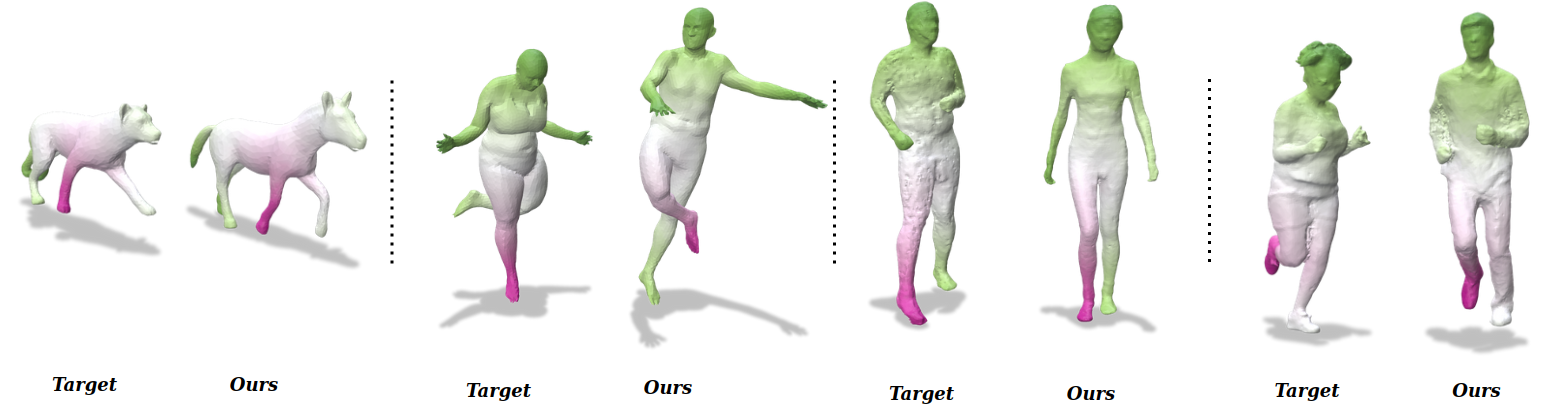
Results on challenging data.
We present an unsupervised data-driven approach for non-rigid shape matching. Shape matching identifies correspondences between two shapes and is a fundamental step in many computer vision and graphics applications. Our approach is designed to be particularly robust when matching shapes digitized using 3D scanners that contain fine geometric detail and suffer from different types of noise including topological noise caused by the coalescence of spatially close surface regions. We build on two strategies. First, using a hierarchical patch based shape representation we match shapes consistently in a coarse to fine manner, allowing for robustness to noise. This multi-scale representation drastically reduces the dimensionality of the problem when matching at the coarsest scale, rendering unsupervised learning feasible. Second, we constrain this hierarchical matching to be reflected in 3D by fitting a patch-wise near-rigid deformation model. Using this constraint, we leverage spatial continuity at different scales to capture global shape properties, resulting in matchings that generalize well to data with different deformations and noise characteristics. Experiments demonstrate that our approach obtains significantly better results on raw 3D scans than state-of-the-art methods, while performing on-par on standard test scenarios. This work was published at the British Machine Vision Conference 2023 12.
8.4 Human Body Shape Completion with Implicit Shape and Flow Learning
Participants: Boyao Zhou, Di Meng, Jean-Sébastien Franco, Edmond Boyer.
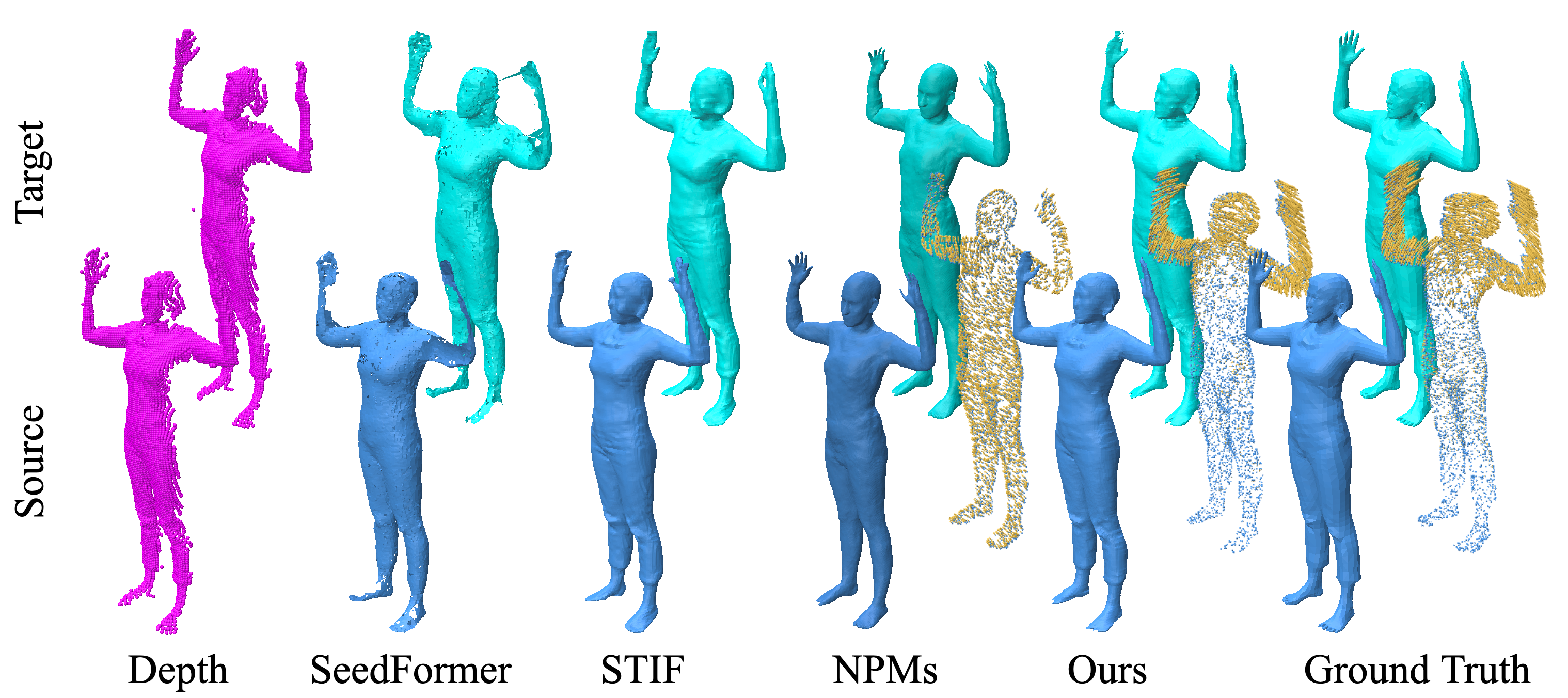
Results on challenging data.
In this work, published at the Computer Vision and Pattern Recognition 2023 conference 13, we investigate how to complete human body shape models by combining shape and flow estimation given two consecutive depth images. Shape completion is a challenging task in computer vision that is highly under-constrained when considering partial depth observations. Besides model based strategies that exploit strong priors, and consequently struggle to preserve fine geometric details, learning based approaches build on weaker assumptions and can benefit from efficient implicit representations. We adopt such a representation and explore how the motion flow between two consecutive frames can contribute to the shape completion task. In order to effectively exploit the flow information, our architecture combines both estimations and implements two features for robustness: First, an all-to-all attention module that encodes the correlation between points in the same frame and between corresponding points in different frames; Second, a coarse-dense to fine-sparse strategy that balances the representation ability and the computational cost. Our experiments demonstrate that the flow actually benefits human body model completion. They also show that our method outperforms the state-of-the-art approaches for shape completion on 2 benchmarks, considering different human shapes, poses, and clothing.
8.5 Multi-Modal Data Correspondence for the 4D Analysis of the Spine with Adolescent Idiopathic Scoliosis
Participants: Nicolas Comte, Sergi Pujades, Olivier Daniel, Aurélien Courvoisier, Jean-Sébastien Franco, François Faure, Edmond Boyer.
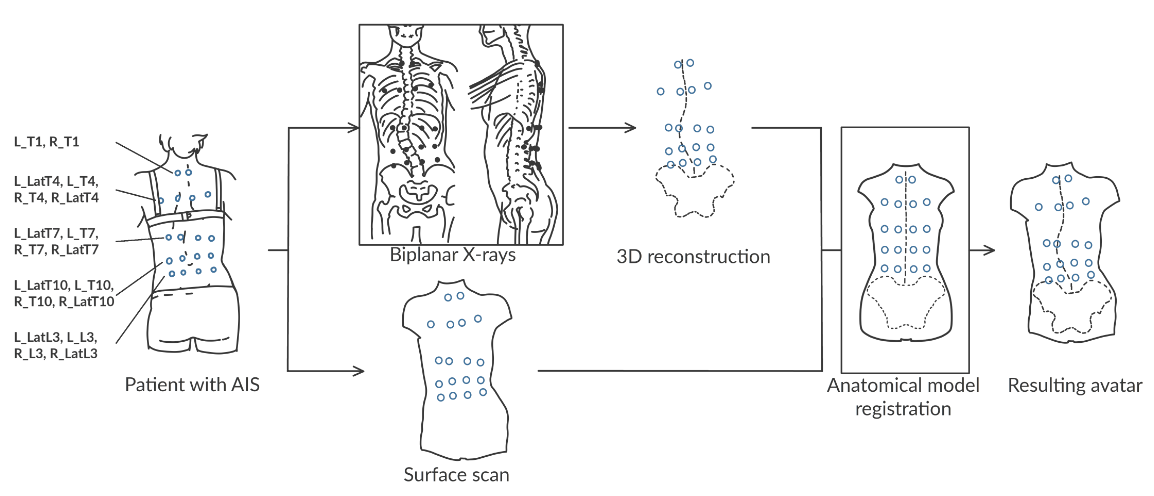
Results on challenging data.
Adolescent idiopathic scoliosis is a three-dimensional spinal deformity that evolves during adolescence. Combined with static 3D X-ray acquisitions, novel approaches using motion capture allow for the analysis of the patient dynamics. However, as of today, they cannot provide an internal analysis of the spine in motion. In this study, we investigated the use of personalized kinematic avatars, created with observations of the outer (skin) and internal shape (3D spine) to infer the actual anatomic dynamics of the spine when driven by motion capture markers. Towards that end, we propose an approach to create a subject-specific digital twin from multi-modal data, namely, a surface scan of the back of the patient and a reconstruction of the 3D spine (EOS). We use radio-opaque markers to register the inner and outer observations. With respect to the previous work, our method does not rely on a precise palpation for the placement of the markers. We present the preliminary results on two cases, for which we acquired a second biplanar X-ray in a bending position. Our model can infer the spine motion from mocap markers with an accuracy below 1 cm on each anatomical axis and near 5 degrees in orientations. This work was published in Bioengineering 7.
8.6 From Skin to Skeleton: Towards Biomechanically Accurate 3D Digital Humans
Participants: Marilyn Keller, Keenon Werling, Soyong Shin, Scott Delp, Sergi Pujades, C. Karen Liu, Michael J. Black.
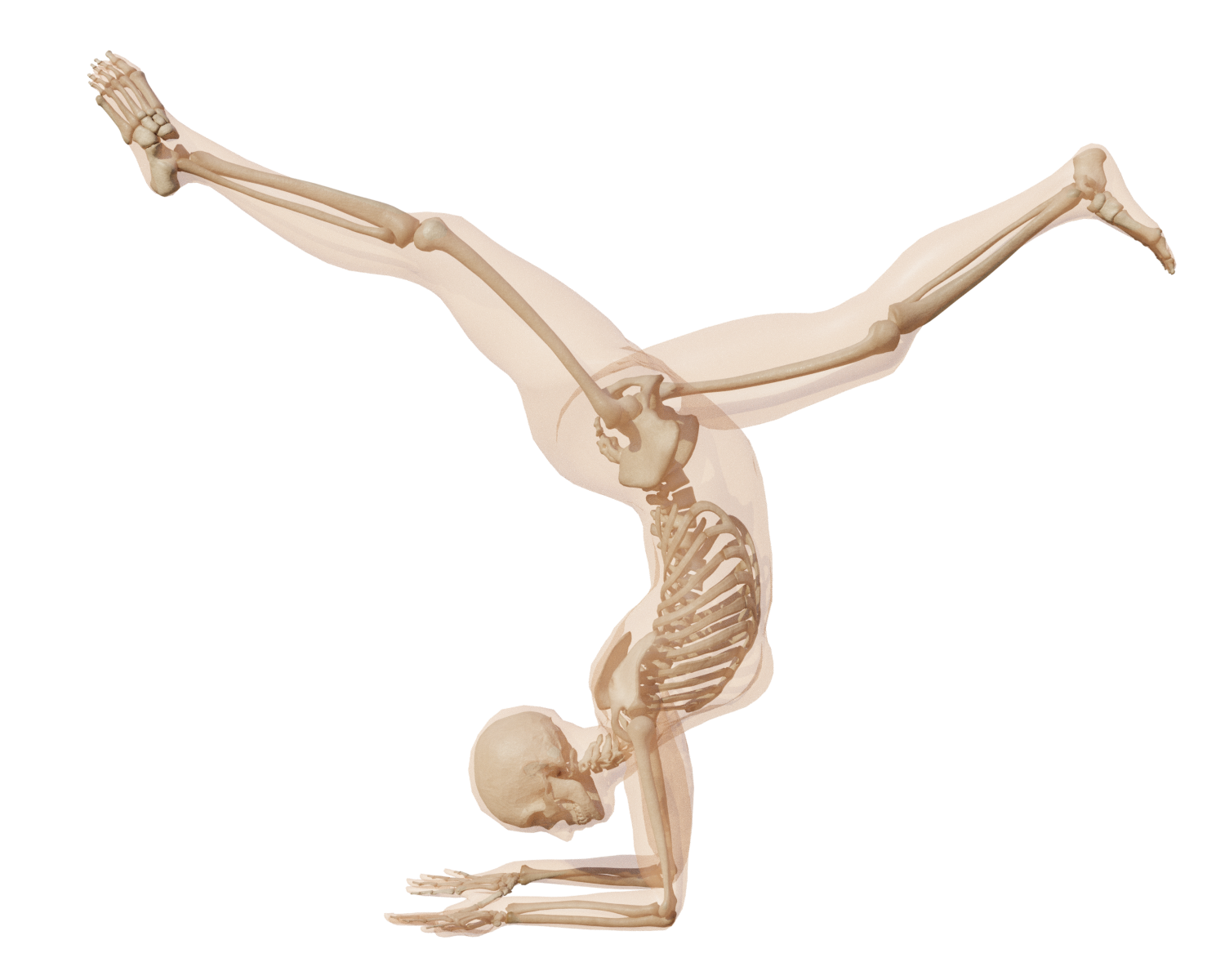
Result on a challenging Yoga pose.
Great progress has been made in estimating 3D human pose and shape from images and video by training neural networks to directly regress the parameters of parametric human models like SMPL. However, existing body models have simplified kinematic structures that do not correspond to the true joint locations and articulations in the human skeletal system, limiting their potential use in biomechanics. On the other hand, methods for estimating biomechanically accurate skeletal motion typically rely on complex motion capture systems and expensive optimization methods. What is needed is a parametric 3D human model with a biomechanically accurate skeletal structure that can be easily posed. To that end, we develop SKEL 8, which re-rigs the SMPL body model with a biomechanics skeleton. To enable this, we need training data of skeletons inside SMPL meshes in diverse poses. We build such a dataset by optimizing biomechanically accurate skeletons inside SMPL meshes from AMASS sequences. We then learn a regressor from SMPL mesh vertices to the optimized joint locations and bone rotations. Finally, we re-parametrize the SMPL mesh with the new kinematic parameters. The resulting SKEL model is animatable like SMPL but with fewer, and biomechanically-realistic, degrees of freedom. We show that SKEL has more biomechanically accurate joint locations than SMPL, and the bones fit inside the body surface better than previous methods. By fitting SKEL to SMPL meshes we are able to “upgrade" existing human pose and shape datasets to include biomechanical parameters. SKEL provides a new tool to enable biomechanics in the wild, while also providing vision and graphics researchers with a better constrained and more realistic model of human articulation. The model, code, and data are available for research at skel.is.tue.mpg.de. This work got an "Honorable Mention for Best Paper Award" at Siggraph Asia 2023.
8.7 Optimizing the 3D Plate Shape for Proximal Humerus Fractures
Participants: Marilyn Keller, Marcell Krall, James Smith, Hans Clement, Alexander Kerner, Andreas Gradischar, Ute Schäfer, Michael J. Black, Annelie Weinberg, Sergi Pujades.
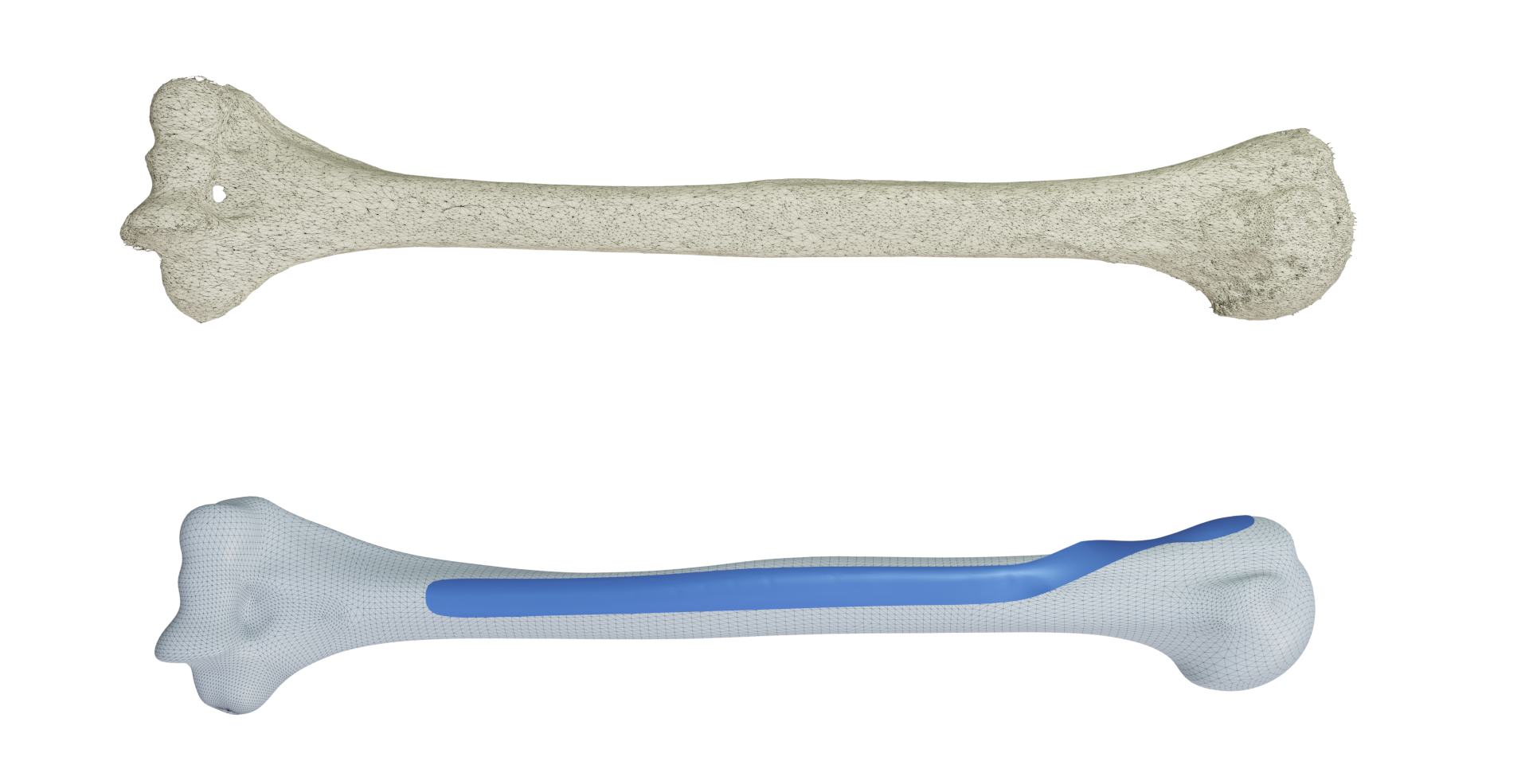
From an input 3D scan of a bone, we can generate a personalized 3D implant.
To treat bone fractures, implant manufacturers produce 2D anatomically contoured plates. Unfortunately, existing plates only fit a limited segment of the population and/or require manual bending during surgery. Patient-specific implants would provide major benefits such as reducing surgery time and improving treatment outcomes but they are still rare in clinical practice. In this work 11, we propose a patient-specific design for the long helical 2D PHILOS (Proximal Humeral Internal Locking System) plate, used to treat humerus shaft fractures. Our method automatically creates a custom plate from a CT scan of a patient's bone. We start by designing an optimal plate on a template bone and, with an anatomy-aware registration method, we transfer this optimal design to any bone. In addition, for an arbitrary bone, our method assesses if a given plate is fit for surgery by automatically positioning it on the bone. We use this process to generate a compact set of plate shapes capable of fitting the bones within a given population. This plate set can be pre-printed in advance and readily available, removing the fabrication time between the fracture occurrence and the surgery. Extensive experiments on ex-vivo arms and 3D-printed bones show that the generated plate shapes (personalized and plate-set) faithfully match the individual bone anatomy and are suitable for clinical practice.
8.8 3D inference of the scoliotic spine from depth maps of the back
Participants: Nicolas Comte, Sergi Pujades, Aurélien Courvoisier, Olivier Daniel, Jean-Sébastien Franco, François Faure, Edmond Boyer.
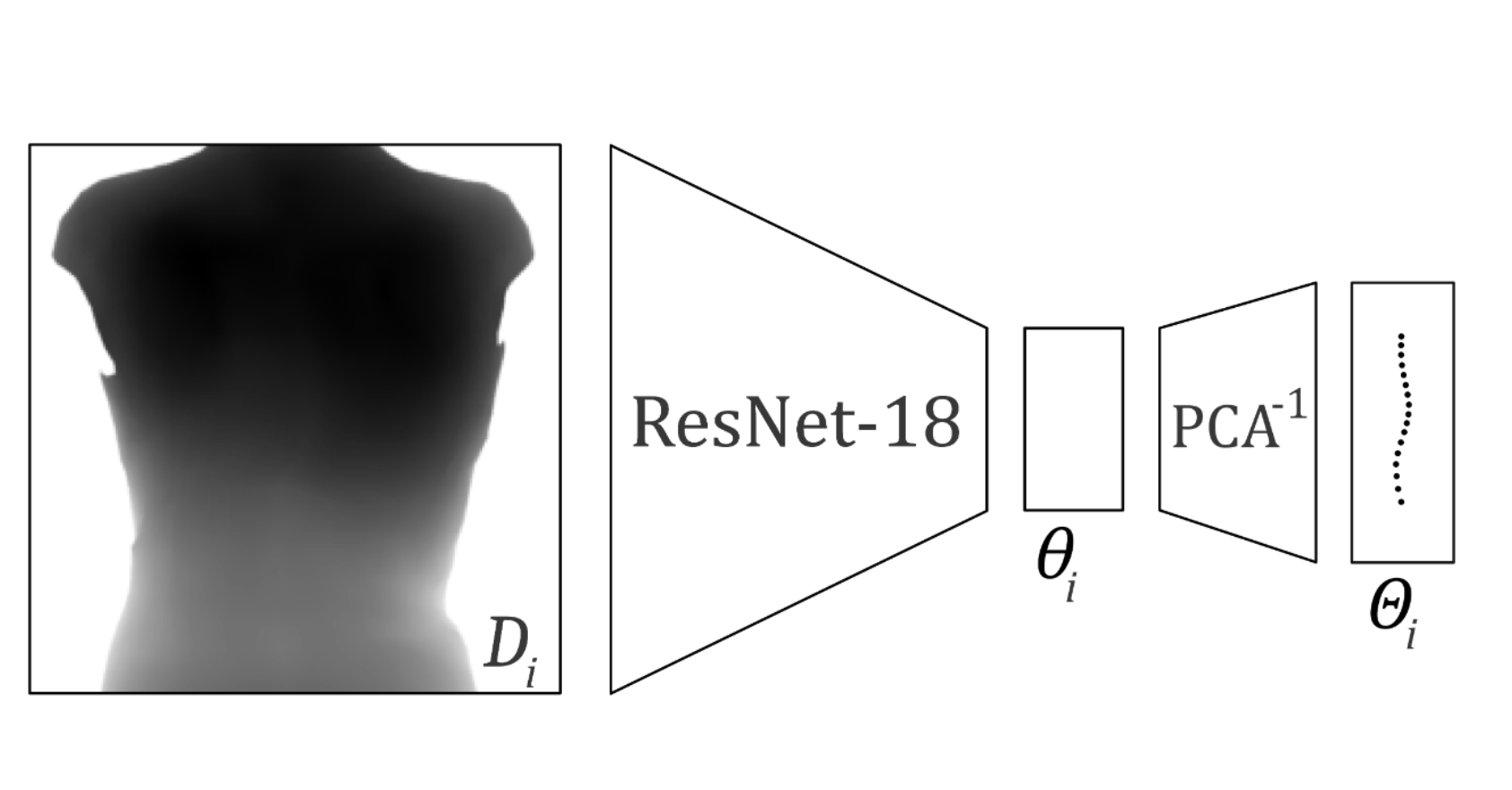
Diagram of the used architecture to predict the 3D shape of the spine.
Recent advances combining outer images and deep-learning algorithms (DLA) show promising results in the detection and the characterization of the Adolescent Idiopathic Scoliosis (AIS). However, these methods are providing a limited 2D characterization while scoliosis is defined in 3D. In this study 18, we propose an inference method that takes as input a depth map of the back of a person and outputs the 3D shape estimation of the thoracolumbar spine. Our DLA method predicts 3D vertebrae positions with an average 3D error of 7.1mm (std: 4.7mm). From the predicted 3D positions, scoliosis can be located and estimated with a mean absolute error (MAE) of 5.5◦ (std: 6.2◦) in the frontal plane. Moreover, sagittal alignments can be estimated with a MAE of 6.4◦ (std: 5.5◦) in kyphosis and 8.3◦ (std: 6.8◦) in lordosis. In addition, our non-ionizing approach can detect scoliosis with an accuracy of 89%.
8.9 Computer vision assisted alignment for stereotactic body radiation therapy (SBRT)
Participants: Atharva Rajesh Peshkar, Danna Gurari, Sergi Pujades, Michael J. Black, Jean-David H. Thomas.
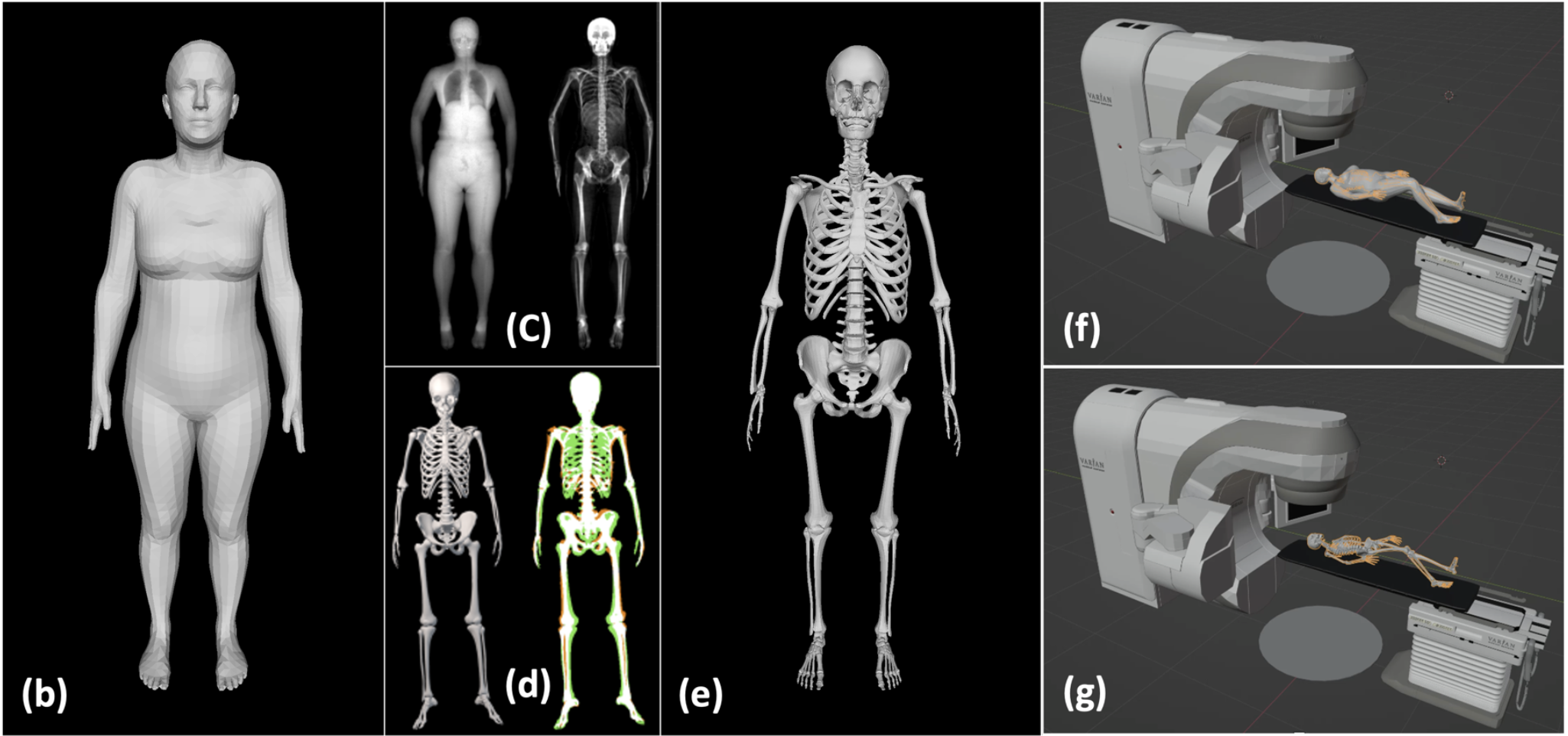
Proof of concept of the skeleton prediction for radiotherapy.
Purpose: To enhance the accuracy of surface-guided RT (SGRT) for abdominal SBRT by designing an artificial intelligence (AI) enhanced computer-vision (CV) patient setup technique that predicts skeletal anatomy from surface imaging.
Methods: We have designed a modified SGRT technique 21, 'avatar guided-RT' (AgRT), that employs patient-specific “avatars” based on the recently published Sparse Trained Articulated Human Body Regressor (STAR) model. STAR is a realistic 3D model of human surface anatomy learned from >10,000 3D body scans that considers gender and BMI for pose-dependent surface variation and can be fitted to CT-based surface contours or surface meshes acquired from 2D video/depth images. We utilize a pre-existing neural network trained on 2000 soft-tissue/skeleton training pairs obtained from dual-energy X-ray absorptiometry (DXA) scans to predict the skeletal anatomy from the body surface of a patient in treatment position.
Results: AgRT was tested using a calibrated multiple camera system. Real-time 3D pose extraction from multiple 2D images was tested in a virtual treatment room to optimize camera numbers and positions. Testing was then conducted on a healthy volunteer to track various treatment poses. The patient's 3D pose was mapped to an avatar with matching gender and BMI. The skeletal alignment technique was assessed on XCAT phantom data and retrospective patient CTs. Skeletal anatomy was predicted from surface imaging with sub-cm accuracy.
Conclusion: Real-time acquisition of 3D human pose and shape is feasible using video inputs and CT data. Inferring the skeletal anatomy can enable alignment to a patient’s X-ray imaging and improve the correspondence between surface imaging and internal anatomy. Realistic body modelling in SGRT can potentially address issues caused by insufficient surface anatomic variationthat can lead to poor correlation and large random errors in current SGRT techniques. Considering patient gender, BMI, pose, and body type can enhance SGRT's accuracy and reliability.
8.10 Accuracy and precision of 3D Optical Imaging for Body Composition by Age, BMI and Ethnicity
Participants: Michael C. Wong, Jonathan P. Bennett, Brandon Quon, Lambert T. Leong, Isaac Y. Tian, Yong E. Liu, Nisa N. Kelly, Cassidy McCarthy, Dominic Chow, Sergi Pujades, Andrea K. Garber, Gertraud Maskarinec, Steven B. Heymsfield, John A. Shepherd.
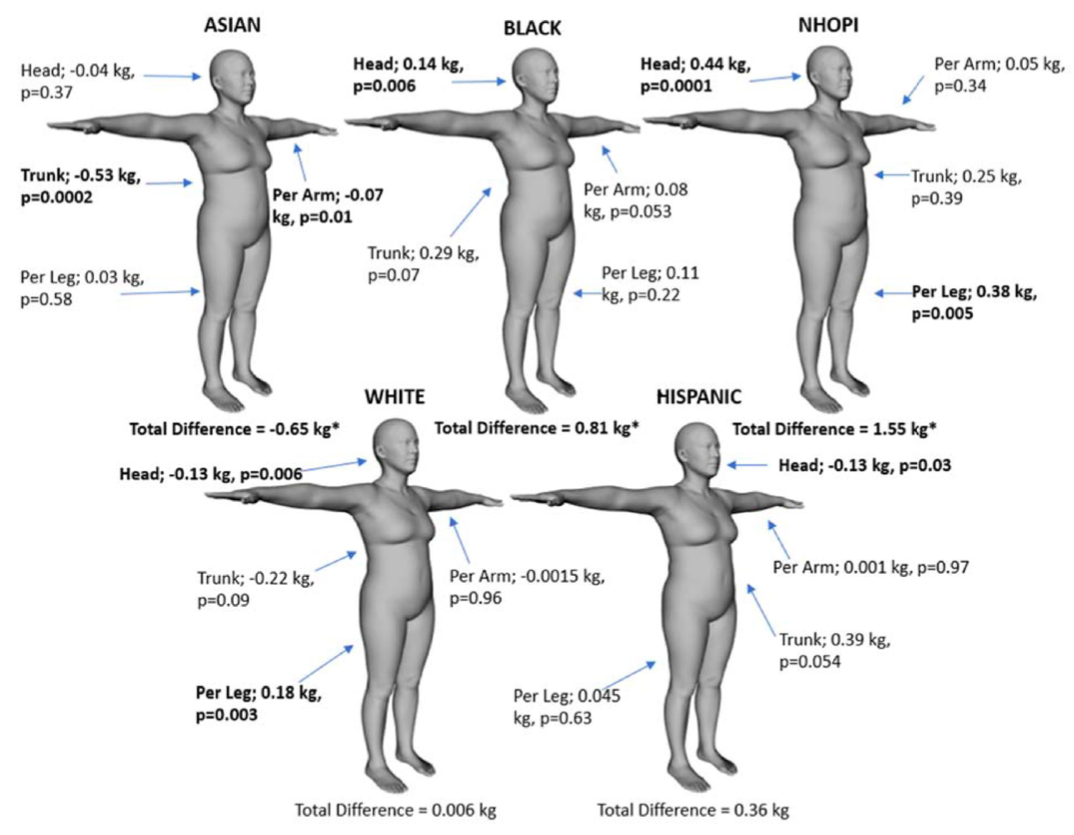
Distribution of the regional prediction results per ethnicity.
Background: The obesity epidemic brought a need for accessible methods to monitor body composition, as excess adiposity has been associated with cardiovascular disease, metabolic disorders, and some cancers. Recent 3-dimensional optical (3DO) imaging advancements have provided opportunities for assessing body composition. However, the accuracy and precision of an overall 3DO body composition model in specific subgroups are unknown.
Objectives: This study 10 aimed to evaluate 3DO’s accuracy and precision by subgroups of age, body mass index, and ethnicity.
Methods: A cross-sectional analysis was performed using data from the Shape Up! Adults study. Each participant received duplicate 3DO and dual- energy X-ray absorptiometry (DXA) scans. 3DO meshes were digitally registered and reposed using Meshcapade. Principal component analysis was performed on 3DO meshes. The resulting principal components estimated DXA whole-body and regional body composition using stepwise forward linear regression with 5-fold cross-validation. Duplicate 3DO and DXA scans were used for test–retest precision. Student’s t tests were performed between 3DO and DXA by subgroup to determine significant differences.
Results: Six hundred thirty-four participants (females 1⁄4 346) had completed the study at the time of the analysis. 3DO total fat mass in the entire sample achieved R2 of 0.94 with root mean squared error (RMSE) of 2.91 kg compared to DXA in females and similarly in males. 3DO total fat mass achieved a % coefficient of variation (RMSE) of 1.76% (0.44 kg), whereas DXA was 0.98% (0.24 kg) in females and similarly in males. There were no mean differences for total fat, fat-free, percent fat, or visceral adipose tissue by age group (P > 0.068). However, there were mean differences for underweight, asian, and black females as well as native Hawaiian or other Pacific islanders (P < 0.038).
Conclusions: A single 3DO body composition model produced accurate and precise body composition estimates that can be used on diverse populations. However, adjustments to specific subgroups may be warranted to improve the accuracy in those that had significant differences.
8.11 Vertebrae localization, segmentation and identification using a graph optimization and an anatomic consistency cycle
Participants: Di Meng, Edmond Boyer, Sergi Pujades.
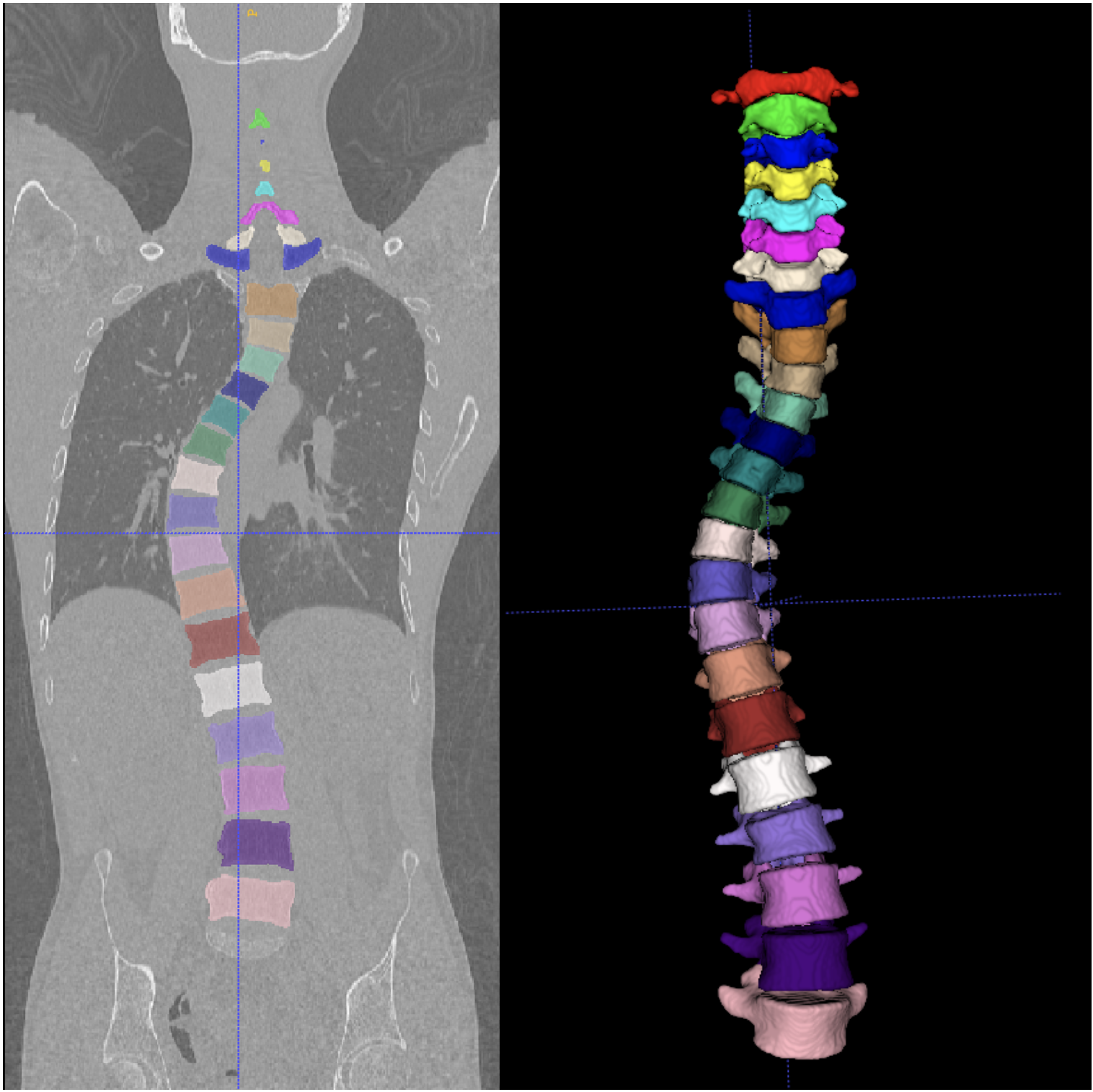
Results on challenging data: patient with scoliotic spine.
Vertebrae localization, segmentation and identification in CT images is key to numerous clinical applications. While deep learning strategies have brought to this field significant improvements over recent years, transitional and pathological vertebrae are still plaguing most existing approaches as a consequence of their poor representation in training datasets. Alternatively, proposed non-learning based methods take benefit of prior knowledge to handle such particular cases. In this work 9, we propose to combine both strategies. To this purpose we introduce an iterative cycle in which individual vertebrae are recurrently localized, segmented and identified using deep-networks, while anatomic consistency is enforced using statistical priors. In this strategy, the transitional vertebrae identification is handled by encoding their configurations in a graphical model that aggregates local deep-network predictions into an anatomically consistent final result. Our approach achieves the state-of-the-art results on the VerSe20 challenge benchmark, and outperforms all methods on transitional vertebrae as well as the generalization to the VerSe19 challenge benchmark. Furthermore, our method can detect and report inconsistent spine regions that do not satisfy the anatomic consistency priors. Our code and model are openly available for research purposes.
9 Bilateral contracts and grants with industry
9.1 Bilateral contracts with industry
Participants: Antoine Dumoulin, Stefanie Wuhrer, Jean-Sébastien Franco.
- The Morpheo INRIA team has a collaboration with Interdigital in Rennes through the Nemo.AI joint lab. The kickoff to this collaboration has happened in November 2022. The collaboration involves two PhD co-supervisions, Antoine Dumoulin and another PhD student starting in 2024, at the INRIA de l'Université Grenoble Alpes. The subject of the collaboration revolves around digital humans, one the one hand to estimate the clothing of humans from images, and on the other to estimate hair models from one or several videos.
9.2 Bilateral grants with industry
Participants: Sergi Pujades Rocamora, Edmond Boyer, Jean-Sébastien Franco.
- The Morpheo INRIA team collaborates with the local start-up Anatoscope created by former researchers at INRIA Grenoble. The collaboration involves one CIFRE PhD (Nicolas Comte) who shares his time between Morpheo and Anatoscope offices. He is working on new classifications methods of the scoliose disease that take the motion of the patient into account in addition to their morphology. The collaboration started in 2020.
- The Morpheo team is also involved in a CIFRE PhD co-supervision (Anilkumar Swamy) with Naver Labs since July 2021, with Gregory Rogez, Mathieu Armando and Vincent Leroy. The work revolves around monocular hand-object reconstruction by showing the object to the camera.
10 Partnerships and cooperations
10.1 International initiatives
10.1.1 Participation in other International Programs
Shape Up! Keiki
Participants: Sergi Pujades, Abdelmouttaleb Dakri.
- Title: Shape Up! Keiki
-
Partner Institution(s):
- University of Hawaii Cancer Center, Honolulu, HI
- Pennington Biomedical Research Center, Baton Rouge, LA
- University of Hawaii at Manoa, Honolulu, HI
- INRIA de l'Université Grenoble Alpes
- Date/Duration: 07/06/2021 - 30/12/2026
-
Additionnal info/keywords:
The rationale for this study is that early life access to accurate body composition data will enable identification of factors that increase obesity, metabolic disease, and cancer risk, and provide a mean to target interventions to those that would benefit. The expected outcome is that our findings would be immediately applicable to accessible gaming and imaging sensors found on modern computers. Funding provided by the NIH - NIDDK (www.niddk.nih.gov)
10.2 National initiatives
10.2.1 ANR
ANR JCJC SEMBA – Shape, Motion and Body composition to Anatomy.
Existing medical imaging techniques, such as Computed Tomography (CT), Dual Energy X-Ray Absorption (DEXA) and Magnetic Resonance Imaging (MRI), allow to observe internal tissues (such as adipose, muscle, and bone tissues) of in-vivo patients. However, these imaging modalities involve heavy and expensive equipment as well as time consuming procedures. External dynamic measurements can be acquired with optical scanning equipment, e.g. cameras or depth sensors. These allow high spatial and temporal resolution acquisitions of the surface of living moving bodies. The main research question of SEMBA is: “can the internal observations be inferred from the dynamic external ones only?”. SEMBA’s first hypothesis is that the quantity and distribution of adipose, muscle and bone tissues determine the shape of the surface of a person. However, two subjects with a similar shape may have different quantities and distributions of these tissues. Quantifying adipose, bone and muscle tissue from only a static observation of the surface of the human might be ambiguous. SEMBA's second hypothesis is that the shape deformations observed while the body performs highly dynamic motions will help disambiguating the amount and distribution of the different tissues. The dynamics contain key information that is not present in the static shape. SEMBA’s first objective is to learn statistical anatomic models with accurate distributions of adipose, muscle, and bone tissue. These models are going to be learned by leveraging medical dataset containing MRI and DEXA images. SEMBA's second objective will be to develop computational models to obtain a subject-specific anatomic model with an accurate distribution of adipose, muscle, and bone tissue from external dynamic measurements only.
ANR JCJC 3DMOVE - Learning to synthesize 3D dynamic human motion.
It is now possible to capture time-varying 3D point clouds at high spatial and temporal resolution. This allows for high-quality acquisitions of human bodies and faces in motion. However, tools to process and analyze these data robustly and automatically are missing. Such tools are critical to learning generative models of human motion, which can be leveraged to create plausible synthetic human motion sequences. This has the potential to influence virtual reality applications such as virtual change rooms or crowd simulations. Developing such tools is challenging due to the high variability in human shape and motion and due to significant geometric and topological acquisition noise present in state-of-the-art acquisitions. The main objective of 3DMOVE is to automatically compute high-quality generative models from a database of raw dense 3D motion sequences for human bodies and faces. To achieve this objective, 3DMOVE will leverage recently developed deep learning techniques. The project also involves developing tools to assess the quality of the generated motions using perceptual studies. This project involves two Ph.D. students: Mathieu Marsot who graduated in May 2023 and Rim Rekik hired November 2021.
ANR Human4D - Acquisition, Analysis and Synthesis of Human Body Shape in Motion
Human4D is concerned with the problem of 4D human shape modeling. Reconstructing, characterizing, and understanding the shape and motion of individuals or groups of people have many important applications, such as ergonomic design of products, rapid reconstruction of realistic human models for virtual worlds, and an early detection of abnormality in predictive clinical analysis. Human4D aims at contributing to this evolution with objectives that can profoundly improve the reconstruction, transmission, and reuse of digital human data, by unleashing the power of recent deep learning techniques and extending it to 4D human shape modeling. It involves 4 academic partners: The laboratory ICube at Strasbourg which is leading the project, the laboratory Cristal at Lille, the laboratory LIRIS at Lyon and the INRIA Morpheo team. This project involves Ph.D. student Aymen Merrouche hired in November 2021.
ANR Equipex+ CONTINUUM - Collaborative continuum from digital to human
The CONTINUUM project will create a collaborative research infrastructure of 30 platforms located throughout France, including Inria Grenoble's Kinovis, to advance interdisciplinary research based on interaction between computer science and the human and social sciences. Thanks to CONTINUUM, 37 research teams will develop cutting-edge research programs focusing on visualization, immersion, interaction and collaboration, as well as on human perception, cognition and behaviour in virtual/augmented reality, with potential impact on societal issues. CONTINUUM enables a paradigm shift in the way we perceive, interact, and collaborate with complex digital data and digital worlds by putting humans at the center of the data processing workflows. The project will empower scientists, engineers and industry users with a highly interconnected network of high-performance visualization and immersive platforms to observe, manipulate, understand and share digital data, real-time multi-scale simulations, and virtual or augmented experiences. All platforms will feature facilities for remote collaboration with other platforms, as well as mobile equipment that can be lent to users to facilitate onboarding.
ANR PRC Inora
The INORA project aims at understanding the mechanisms of action of shoes and orthotic insoles on Rheumatoid arthritis (RA) patients through patient-specific computational biomechanical models. These models will help in uncovering the mechanical determinants to pain relief, which will enable the long-term well-being of patients. Motivated by the numerous studies highlighting erosion and joint space narrowing in RA patients, we postulate that a significant contributor to pain is the internal joint loading when the foot is inflamed. This hypothesis dictates the need of a high-fidelity volumetric segmentation for the construction of the patient-specific geometry. It also guides the variables of interests in the exploitation of a finite element (FE) model. The INORA project aims at providing numerical tools to the scientific, medical and industrial communities to better describe the mechanical loading on diseased distal foot joints of RA patients and propose a patient-specific methodology to design pain-relief insoles. The Mines de St Etienne is leading this project.
11 Dissemination
11.1 Promoting scientific activities
11.1.1 Scientific events: organisation
Member of the organizing committees
- Sergi Pujades was a Session Chair at Siggraph Asia 2023
11.1.2 Scientific events: selection
Member of the conference program committees
- Stefanie Wuhrer was a PC member of 3DOR 2023
Reviewer
- Stefanie Wuhrer reviewed for CVPR, ICCV, 3DV, SIGGRAPH, and Eurographics
- Jean-Sébastien Franco reviewed for CVPR, ICCV, 3DV
- Sergi Pujades reviewed for CVPR, MICCAI, Pacific Graphics
11.1.3 Journal
Member of the editorial boards
- Jean-Sébastien Franco was associate editor for the International Journal on Computer Vision.
Reviewer - reviewing activities
- Stefanie Wuhrer reviewed for PAMI, CVIU
11.1.4 Invited talks
- Stefanie Wuhrer gave an invited speech on Learning representations of 4D human motion at ORASIS 2023
- Stefanie Wuhrer gave an invited speech on Learning representations of 4D human motion at 3DOR 2023
11.1.5 Scientific expertise
- Stefanie Wuhrer was on a hiring committee for a Maître de Conférences position at Ensimag
- Jean-Sébastien Franco co-presided a hiring committee for a Maître de Conférences position at Ensimag, after participating in the administrative effort to obtain the position opening on behalf of the LJK laboratory Image department and the Ensimag.
- Sergi Pujades was on a hiring committee for 2 ATER positions at Université Grenoble Alpes.
- Sergi Pujades was on the scientific committee for the study of the "Delegations INRIA" applications.
- Sergi Pujades was on the scientific committee for the study of the "Post-doc INRIA" applications.
- Sergi Pujades was a reviewer for the Mitacs program (Canada) to evaluate a PhD grant application.
11.1.6 Research administration
- Stefanie Wuhrer is référente données for the Inria centre of the Grenoble Alpes University
11.2 Teaching - Supervision - Juries
11.2.1 Teaching
- Master : Sergi Pujades , Numerical Geometry, 37.5h EqTD, M1, Université Grenoble Alpes, France
- Master: Sergi Pujades , Computer Vision, 54h EqTD, M2R Mosig GVR, Grenoble INP.
- Master: Sergi Pujades , Introduction to Visual Computing, 42h, M1R Mosig GVR, Université Grenoble Alpes.
- Master: Sergi Pujades , Internship supervision, 1h, Ensimag 3rd year, Grenoble INP.
- Master: Sergi Pujades , Medical Leave, 41.25h EqTD, Université Grenoble Alpes.
- Master: Jean-Sébastien Franco , Introduction to Computer Graphics, 33h, Ensimag 2nd year, Grenoble INP.
- Master: Jean-Sébastien Franco , Introduction to Computer Vision, 36, Ensimag 3rd year, Grenoble INP.
- Master: Jean-Sébastien Franco , Internship and project supervisions, 20h, Ensimag 2nd and 3rd year, Grenoble INP.
- Master: J.S. Franco, Leadership of the image pedagogic workgroup at Ensimag, 12, Ensimag 2nd and 3rd year, Grenoble INP.
- Master: Julien Pansiot , Introduction to Visual Computing, 15h EqTD, M1 MoSig, Université Grenoble Alpes.
11.2.2 Supervision
- Ph.D. defended: Mathieu Marsot . Data driven representation and synthesis of 3D human motion, defended May 2022. Supervised by Jean-Sébastien Franco , Stefanie Wuhrer .
- Ph.D. defended: Pierre Zins . 3D shape reconstruction from multiple views, defended April 2022. Supervised by Edmond Boyer , Stefanie Wuhrer .
- Ph.D. defended: Nicolas Comte . Learning Scoliosis Patterns using Anatomical Models and Motion Capture. Supervised by Jean-Sébastien Franco , Sergi Pujades , François FaureAurélien Courvoisier , Edmond Boyer
- Ph.D. in progress: Rim Rekik , Human motion generation and evaluation, since 01.11.2021, supervised by Anne-Hélène Olivier (Université Rennes), Stefanie Wuhrer .
- Ph.D. in progress: Aymen Merrouche , Learning non-rigid surface matching, since 01.10.2021, supervised by Edmond Boyer , Stefanie Wuhrer .
- Ph.D. in progress: Antoine Dumoulin , Video-based dynamic garment representation and synthesis, since 01.11.2023, supervised by Adnane Boukhayma (Inria Rennes), Pierre Héllier (Université Rennes), Stefanie Wuhrer .
- Ph.D. in progress: Vicente Estopier Castillo . NTENSIVE : Regional Lung Function Inference from Upper Body Surface Motion for Personalized Ventilation Protocols in Acute Respiratory Failure Patients, since 01.10.2023. Supervised by Jean-Sébastien Franco , Sergi Pujades , Sam Bayat .
- Ph.D. in progress: Briac Toussaint, High precision alignment of non-rigid surfaces for 3D performance capture, since 01.10.2021, supervised by Jean-Sébastien Franco .
- Ph.D. in progress: Anilkumar Swamy, Hand-object interaction acquisition, since 01.07.2021, supervised by Jean-Sébastien Franco , Gregory Rogez (CIFRE Naverlabs Europe).
- Master: Jean-Sébastien Franco , Supervision of the 2nd year program (circa 300 students), 36h, Ensimag 2nd year, Grenoble INP.
11.2.3 Juries
- Stefanie Wuhrer was reviewer for Ph.D. of Pietro Musoni, University of Verona
- Stefanie Wuhrer was reviewer for Ph.D. of Clément Lemeunier, Université Claude Bernard Lyon 1
- Stefanie Wuhrer was Ph.D comittee member of Emery Pierson, Université de Lille
- Jean-Sébastien Franco was Ph.D committee member of Florent Bartoccioni, Université Grenoble Alpes.
- Sergi Pujades was a reviewer for the Ph.D. of Enric Corona at Université Politecnica de Catalunya (UPC) in Barcelona, Spain.
11.3 Popularization
11.3.1 Interventions
- Rim Rekik presented her PhD work in the "Journée Filles, Maths et Informatique : une équation lumineuse" in front of an A-Levels audience (lycéenes).
12 Scientific production
12.1 Major publications
- 1 article4DHumanOutfit: a multi-subject 4D dataset of human motion sequences in varying outfits exhibiting large displacements.Computer Vision and Image Understanding237December 2023HALDOI
- 2 inproceedingsOptimizing the 3D Plate Shape for Proximal Humerus Fractures.International Conference on Medical Image Computing and Computer-Assisted Intervention (MICCAI)14226Lecture Notes in Computer ScienceVancouver, CanadaSpringer Nature SwitzerlandOctober 2023, 487-496HALDOI
- 3 articleFrom Skin to Skeleton: Towards Biomechanically Accurate 3D Digital Humans.ACM Transactions on Graphics426December 2023, 1-12HALDOI
- 4 inproceedingsHuman Body Shape Completion with Implicit Shape and Flow Learning.CVPR 2023 - IEEE Conference on Computer Vision and Pattern RecognitionVancouver, CanadaIEEEJune 2023HAL
- 5 inproceedingsMulti-View Reconstruction using Signed Ray Distance Functions (SRDF).CVPR 2023 - IEEE/CVF Conference on Computer Vision and Pattern RecognitionVancouver, CanadaIEEEJune 2023, 1-11HAL
12.2 Publications of the year
International journals
- 6 article4DHumanOutfit: a multi-subject 4D dataset of human motion sequences in varying outfits exhibiting large displacements.Computer Vision and Image Understanding237December 2023HALDOIback to textback to textback to text
- 7 articleMulti-Modal Data Correspondence for the 4D Analysis of the Spine with Adolescent Idiopathic Scoliosis.Bioengineering107July 2023, 874HALDOIback to text
- 8 articleFrom Skin to Skeleton: Towards Biomechanically Accurate 3D Digital Humans.ACM Transactions on Graphics426December 2023, 1-12HALDOIback to textback to text
- 9 articleVertebrae localization, segmentation and identification using a graph optimization and an anatomic consistency cycle.Computerized Medical Imaging and Graphics107July 2023, 102235HALDOIback to text
- 10 articleAccuracy and Precision of 3-dimensional Optical Imaging for Body Composition by Age, BMI, and Ethnicity.The American Journal of Clinical Nutrition1183September 2023, 657-671HALDOIback to text
International peer-reviewed conferences
- 11 inproceedingsOptimizing the 3D Plate Shape for Proximal Humerus Fractures.International Conference on Medical Image Computing and Computer-Assisted Intervention (MICCAI)14226Lecture Notes in Computer ScienceVancouver, CanadaSpringer Nature SwitzerlandOctober 2023, 487-496HALDOIback to text
- 12 inproceedingsDeformation-Guided Unsupervised Non-Rigid Shape Matching.BMVC 2023 - 34th British Machine Vision ConferenceAberdeen, United Kingdom2023, 1-22HALback to text
- 13 inproceedingsHuman Body Shape Completion with Implicit Shape and Flow Learning.CVPR 2023 - IEEE Conference on Computer Vision and Pattern RecognitionVancouver, CanadaIEEEJune 2023HALback to text
- 14 inproceedingsMulti-View Reconstruction using Signed Ray Distance Functions (SRDF).CVPR 2023 - IEEE/CVF Conference on Computer Vision and Pattern RecognitionVancouver, CanadaIEEEJune 2023, 1-11HALback to text
Doctoral dissertations and habilitation theses
Reports & preprints
- 17 misc3DTeethSeg'22: 3D Teeth Scan Segmentation and Labeling Challenge.May 2023HALDOI
- 18 misc3D inference of the scoliotic spine from depth maps of the back.December 2023HALback to textback to text
- 19 miscMedShapeNet -- A Large-Scale Dataset of 3D Medical Shapes for Computer Vision.August 2023HAL
- 20 miscCorrespondence-free online human motion retargeting.2023HALback to text
Other scientific publications
- 21 inproceedingsComputer vision assisted alignment for stereotactic body radiation therapy (SBRT).Annual Conference for the American Association of Physics in MedicineHouston, United StatesJuly 2023HALback to textback to text