2024Activity reportProject-TeamWILLOW
RNSR: 200718311C- Research center Inria Paris Centre
- In partnership with:Ecole normale supérieure de Paris, CNRS
- Team name: Embodied computer vision
- In collaboration with:Département d'Informatique de l'Ecole Normale Supérieure
- Domain:Perception, Cognition and Interaction
- Theme:Vision, perception and multimedia interpretation
Keywords
Computer Science and Digital Science
- A3.1.1. Modeling, representation
- A3.4. Machine learning and statistics
- A5.3. Image processing and analysis
- A5.4. Computer vision
- A5.10. Robotics
- A9. Artificial intelligence
- A9.1. Knowledge
- A9.2. Machine learning
- A9.3. Signal analysis
- A9.5. Robotics
- A9.7. AI algorithmics
Other Research Topics and Application Domains
- B9.5.1. Computer science
- B9.5.6. Data science
1 Team members, visitors, external collaborators
Research Scientists
- Justin Carpentier [Team leader, INRIA, Researcher]
- Stephane Caron [INRIA, Researcher, HDR]
- Shizhe Chen [INRIA, Researcher]
- Frederike Dumbgen [INRIA, Starting Research Position, from May 2024]
- Cordelia Schmid [INRIA, Senior Researcher, HDR]
Faculty Member
- Jean Ponce [ENS Paris, Professor, HDR]
Post-Doctoral Fellows
- Oumayma Bounou [ENS, from Nov 2024]
- Ewen Dantec [ENS Paris, Post-Doctoral Fellow]
- Wilson Jallet [INRIA, Post-Doctoral Fellow, from Dec 2024]
- Etienne Menager [INRIA, Post-Doctoral Fellow]
- Etienne Moullet [INRIA, Post-Doctoral Fellow]
- Ajay Sathya [INRIA, Post-Doctoral Fellow]
PhD Students
- Roland Andrews [INRIA, from Oct 2024]
- Adrien Bardes [META, until Apr 2024]
- Theo Bodrito [INRIA]
- Oumayma Bounou [INRIA, until Sep 2024]
- Thomas Chabal [INRIA]
- Nicolas Chahine [Inria, from Aug 2024]
- Nicolas Chahine [DXO, until Aug 2024]
- Zerui Chen [INRIA]
- Ludovic De Matteis [UNIV TOULOUSE III]
- Yann Dubois De Mont-Marin [INRIA]
- Gabriel Fiastre [INRIA]
- Matthieu Futeral-Peter [INRIA]
- Ricardo Garcia Pinel [INRIA]
- Francois Garderes [LOUIS VUITTON, CIFRE]
- Umit Bora Gokbakan [INRIA, from Jun 2024]
- Wilson Jallet [UNIV TOULOUSE III, until Nov 2024]
- Zeeshan Khan [INRIA]
- Quentin Le Lidec [INRIA]
- Guillaume Le Moing [INRIA, until Jul 2024]
- Shiyao Li [ENPC]
- Imen Mahdi [University of Freiburg, from Oct 2024]
- Louis Montaut [INRIA]
- Paul Pacaud [INRIA, from Oct 2024]
- Sara Pieri [INRIA, from Oct 2024]
- Fabian Schramm [INRIA]
- Romain Seailles [ENS Paris, from Sep 2024]
- Basile Terver [FACEBOOK, CIFRE, from Nov 2024]
- Lucas Ventura [ENPC]
- Elliot Vincent [Ministère Transition, until Aug 2024]
Technical Staff
- Roland Andrews [INRIA, Engineer, until Sep 2024]
- Etienne Arlaud [INRIA, Engineer]
- Umit Bora Gokbakan [INRIA, Engineer, until May 2024]
- Lucas Haubert [INRIA, Engineer, from Dec 2024]
- Louise Manson [INRIA, Engineer, from Dec 2024]
- Megane Millan [INRIA, Engineer]
- Julie Perrin [Inria, Engineer]
- Pierre-Guillaume Raverdy [Inria, Engineer]
- Valentin Tordjman–Levavasseur [INRIA, Engineer, from Nov 2024]
- Joris Vaillant [INRIA, Engineer]
Interns and Apprentices
- Theotime Le Hellard [ENS Paris, Intern, from Feb 2024 until Jul 2024]
- Imen Mahdi [INRIA, Intern, from Apr 2024 until Sep 2024]
- Franki Nguimatsia Tiofack [INRIA, Intern, from May 2024 until Nov 2024]
- Axel Nguyen–Kerbel [INRIA, Intern, from Apr 2024 until Oct 2024]
- Marguerite Petit–Talamon [INRIA, Intern, from Apr 2024 until Sep 2024]
- Sara Pieri [INRIA, from May 2024 until Sep 2024]
- Valentin Tordjman–Levavasseur [INRIA, Intern, from May 2024 until Oct 2024]
Administrative Assistant
- Julien Guieu [INRIA]
Visiting Scientists
- Qikai Huang [UNIV GEORGIA]
- Kim Jae Myung [UNIV TUBINGEN, from Dec 2024]
- Lander Vanroye [KU Leuven, from Oct 2024 until Nov 2024]
- Lander Vanroye [KU Leuven, from Mar 2024 until Jun 2024]
External Collaborators
- Theotime Le Hellard [ENS Paris, from Jul 2024]
- Elliot Vincent [Ministère Transition, from Sep 2024]
2 Overall objectives
2.1 Statement
Building machines that can automatically understand complex visual inputs is one of the central scientific challenges in artificial intelligence. Truly successful visual understanding technology will have a broad impact in application domains as varied as defense, entertainment, healthcare, human-computer interaction, image retrieval and data mining, industrial and personal robotics, manufacturing, scientific image analysis, surveillance and security, and transportation.
The problem is, however, very difficult due to the large variability of the visual world and the high complexity of the underling physical phenomena. For example, people easily learn how to perform complex tasks such as changing a car tire or performing resuscitation by observing other people. This involves advanced visual perception and interaction capabilities including interpreting sequences of human actions, learning new visuomotor skills from only a few example demonstrations, grounding instructions in appropriate scene elements and actions, and applying the learned skills in new environments and situations. Currently, however, there is no artificial system with a similar level of cognitive visual competence. Our goal for the next 10 years is to develop models, methods and algorithms providing sufficient level of visual intelligence to enable applications such as personal visual assistants or home robots that will, for example, prepare a meal in response to a chat request.
Despite the tremendous progress in visual recognition in the last decade, current visual recognition systems still require large amounts of carefully annotated training data, often use black-box architectures that do not model the 3D physical nature of the visual world, are typically limited to simple pattern recognition tasks such as detecting and recognizing objects from a predefined vocabulary, and do not capture real-world semantics. We plan to address these limitations with an ambitious research program that aims at developing models of the entire visual understanding process from image acquisition to the high-level embodied interpretation of visual scenes. We target learnable models that require minimal to no supervision, support complex reasoning about visual data, and are grounded in interactions with the physical world. More concretely, we will address fundamental scientific challenges along three research axes: (i) visual recognition in images and videos with an emphasis on weakly supervised learning and models grounded in the physical 3D world; (ii) learning embodied visual representations for robotic manipulation and locomotion; and (iii) image restoration and enhancement. These challenges will be tackled by a team of researchers with core expertise in computer vision and robotics, who will simultaneously advance both fields towards convergence. The complementary expertise in areas such as machine learning and natural language understanding will be gained through collaboration with relevant research teams.
We believe that foundational research should be grounded in applications and we plan to pursue applications with high scientific, societal, and/or economic impact in domains such as transportation; augmented reality; education; advanced manufacturing; and quantitative visual analysis in sciences, humanities and healthcare.
3 Research program
3.1 Visual recognition and reconstruction of images and videos
It is now possible to efficiently detect individual objects and people in cluttered images and videos. Current methods, however, rely on large-scale, manually-annotated image collections, often use black-box architectures that do not model the 3D physical nature of the visual world, and are typically limited to simple pattern recognition tasks. In this part of research program, we address these fundamental limitations. In particular, we address the following three key open challenges: (i) how to leverage available but weak annotations including text, audio and speech, (ii) how to enable automatic reasoning about visual data, and (iii) how to develop models grounded in the physical 3D world including learnable models for 3D object and scene reconstruction. We also continue theoretical work aimed at understanding the geometric underpinnings of computer vision.
Our current efforts in this area are outlined in detail in Section. 8.1.
3.2 Learning embodied representations
Computer vision has come a long way toward understanding images and videos in terms of scene geometry, object labels, locations and poses of people or classes of human actions. This “understanding”, however, remains largely disconnected from reasoning about the physical world. For example, what will happen when removing a tablecloth from a set table? What actions will be needed to resume an interrupted meal? We believe that a true embodied understanding of dynamic scenes from visual observations is the next major research challenge. We address this challenge by developing new models and algorithms with an emphasis on the synergy between vision, learning, robotics and natural language understanding. To this end, we study learning methods for motion planning and optimal control for known environments in state space. At the same time, we develop models and algorithms for learning visio-motor policies that do not rely on the known structure of environments and instead integrate visual perception directly into control algorithms. We also address natural language providing additional modality for more efficient learning and communication with emodied agents.
Our current efforts in this area are outlined in detail in Section 8.2.
3.3 Image restoration and enhancement
Although image processing is a mature field, it is more important than ever with the advent of high-quality camera phones, scientific applications in microscopy and astronomy and, recently, the emergence of multi-modal sensing for autonomous cars for example. In addition, it is an excellent proving ground for learning-based techniques since (a) it is in general (relatively) easy to generate realistic corrupted images from clean ones since reasonable models of the physical image corruption problem as often available (Abdelhamed et al., 2019; Nah et al., 2017), and (b) it is possible to incorporate natural image priors such as self-similarities (Buades et al., 2005) and sparsity (Mairal et al., 2009) in the modelling and optimization processes. We have conducted work on image restoration since the time of Julien Mairal's PhD thesis, addressing problems such as demosaicking, denoising, inpainting, and inverse half-toning with a combination of sparse coding/dictionary learning methods and non-local means, then moving on to blind deblurring including motion segmentation and, more recently, deep-learning methods. In our on-going efforts we address several challenges for learning-based approaches to image restoration: (i) how to combine different modalities such as depth and RGB images to improve the quality of the joint observations; (ii) how to construct tunable, fully interpretable approaches to image restoration in a functional framework; and (iii) how to incorporate machine learning methods that go beyond the traditional fully supervised setting into the image restoration pipeline.
Our current work in this area is outlined in detail in Section 8.3.
4 Application domains
We believe that foundational modeling work should be grounded in applications. This includes (but is not restricted to) the following high-impact domains.
4.1 Automated visual assistants
The modern seamless video communication has enabled new applications in education, medicine and manufacturing, such as remote surgery or remotely-supervised product assembly. The abundance of online instructional videos further confirms the high demand of assistance including daily tasks such as cooking and gardening. Our work on embodied video understanding and on the joint modeling of vision and language will support automatic visual assistants. Similar to existing driving navigation assistants, such applications will guide people in daily living, inspection and manufacturing tasks. Some of these applications are studied within our MSR-Inria collaboration.
4.2 Robotics
In 2023, the Willow team has pursued the development of the Pinocchio library both from a scientific and software perspective. The recent versions of Pinocchio now accounts for closed-loop mechanisms (based on a proximal optimization), code source generation on GPUs, etc. All these new features make Pinocchio a unique tool to efficiently control complex robotic systems such as legged robots or industrial robots. We are now closely collaborating with Pal Robotics which plan to use Pinocchio to control its next generation of humanoid robots called Kangaroo. In the near future, the plan is to extend Pinocchio to become a generic-purposed and efficient robotic simulator simulating both rigid and compliant contact interactions between a robot and its environment, with the ambition of making Pinocchio the next golden framework for simulation in robotics, offering advanced features for optimal control, reinforcement learning, like differentiable simulation. Such features should position Pinocchio as the central simulator in Robotics.
4.3 Image restoration
We are pursuing applications of our image restoration work to personal photography, to enhance the images taken by consumer cameras and smartphones by deblurring and denoising them, and improving their spatial resolution and dynamic range. In this context, we are collaborating with DXOMark, the world leader in smartphone camera evaluation, through a CIFRE thesis. Two of the objectives are to develop a public database of portraits fully compliant with European GDRP regulations with informed consent from the models, and to automate the rating of image quality using this dataset. We also apply the mixture of physical image formation model and machine learning principles that has made our image restoration work successful to scientific fields: We collaborate with Anne-Marie Lagrange (Observatoire de Paris), Maud Langlois (SNRS/Observatoire de Lyon) and Julien Mairal (Inria) on direct exoplanet detection from ground-based telescope imagery. This work also involves a post-doc, Olivier Flasseur, and a PhD Student, Théo Bodrito. We will apply next year the same principles to molecular microscopy, in collaboration with Jean-Baptiste Masson (Institut Pasteur).
5 Social and environmental responsibility
Artificial intelligence holds great potential for improving our environment, for example, by reducing energy consumption and optimizing energy production. Computer vision, in particular, can be used to monitor emissions from coal plants and to track forest growth using satellite imagery. Autonomous drones can monitor and prevent failures of pipelines, power lines, power plants and other remote installations. However, as larger and more powerful AI models require increased compute power at training and deployment, AI itself stands for an increasingly high carbon footprint. One direction of our research aims to develop efficient and low-resource neural network models. To this end we have previously proposed Cross-Covariance Image Transformers (El-Nouby et al. NeurIPS'21) that avoid quadratic complexity in terms of image size. In 2024, we have been also working on the development of new optimization methods and associated software 16 to reduce the overall computationel burden and reduce their energetical impact when applied to industrial and practical scenarios. In the light of these devleopments, with the help of the Inria Soft infrastructure, we are considering creating a new software consortium, named Maestro, to accelerate the developement and the dissemination of efficient algorithmic solutions for the control of robotics systems. One objective of this consortium is to provide software solutions that reduce the computational burden and energetic consumption of modern robots currently deployed in industry or in societal sectors.
6 Highlights of the year
6.1 Awards
- J. Carpentier obtained an ERC StG in 2024 named ARTIFACT, standing for the Artificial Motion Factory.
- J. Carpentier was keynote speacker at Humanoids 2024.
- C. Schmid was keynote speacker at CoRL 2024.
- C. Schmid was awarded the Heinrich-Hertz-Gastprofessur award , Karlsruher Institut für Technologie (KIT), 2024.
- C. Schmid obtained the European Inventor Award 2024, category research, awarded by European Patent Office.
- C. Schmid obained Prix Monte-Carlo Femme de l'Année in 2024.
7 New software, platforms, open data
7.1 New software
7.1.1 alignsdf
-
Keywords:
Computer vision, 3D reconstruction
-
Functional Description:
This is the PyTorch implementation of the AlignSDF research paper:
AlignSDF: Pose-Aligned Signed Distance Fields for Hand-Object Reconstruction Zerui Chen, Yana Hasson, Ivan Laptev, Cordelia Schmid ECCV 2022
- Publication:
-
Contact:
Zerui Chen
-
Participants:
Zerui Chen, Yana Hasson, Ivan Laptev, Cordelia Schmid
7.1.2 BLERC
-
Name:
Benchmarking Learning Efficiency in Deep Reservoir Computing
-
Keywords:
Machine learning, Continual Learning
-
Functional Description:
Measuring learning efficiency of machine learning models.
- URL:
- Publication:
-
Contact:
Hugo Cisneros
7.1.3 BurstSR
-
Name:
Super-resolution from image bursts
-
Keyword:
Image processing
-
Functional Description:
This is a research prototpye allowing to take as input a sequence of raw or rgb images produced by a smartphone or digital camera. This code produces a high quality color images with higher resolution.
-
Release Contributions:
This new version, v0.2, introduces various improvements, as well as C++ code that accelerates the original Python code.
- Publication:
-
Contact:
Julien Mairal
-
Participants:
Bruno Lecouat, Julien Mairal, Jean Ponce
7.1.4 FrozenBiLM
-
Name:
Zero-Shot Video Question Answering via Frozen Bidirectional Language Models
-
Keywords:
Computer vision, Natural language processing, Deep learning
-
Functional Description:
Code, datasets and models associated with the paper "Zero-Shot Video Question Answering via Frozen Bidirectional Language Models"
- URL:
-
Contact:
Antoine Yang
7.1.5 hiveformer
-
Keywords:
Robotics, NLP, Transformer
-
Functional Description:
This is the PyTorch implementation of the Hiveformer research paper:
Instruction-driven history-aware policies for robotic manipulations Pierre-Louis Guhur, Shizhe Chen, Ricardo Garcia, Makarand Tapaswi, Ivan Laptev, Cordelia Schmid CoRL 2022 (oral)
- Publication:
-
Contact:
Pierre-Louis Guhur
-
Participants:
Pierre-Louis Guhur, Shizhe Chen, Ricardo Garcia Pinel, Makarand Tapaswi, Ivan Laptev, Cordelia Schmid
7.1.6 HM3DAutoVLN
-
Name:
Learning from Unlabeled 3D Environments for Vision-and-Language Navigation
-
Keyword:
Computer vision
-
Functional Description:
Open source release of the software package for the ECCV'22 paper by Chen et al. "Learning from Unlabeled 3D Environments for Vision-and-Language Navigation". This release provides a full implementation of the method, including code for training models, and testing on standard datasets, generated datasets as well as trained models.
- URL:
- Publication:
-
Contact:
Shizhe Chen
-
Participants:
Shizhe Chen, Pierre-Louis Guhur, Makarand Tapaswi, Cordelia Schmid, Ivan Laptev
7.1.7 Just Ask: Learning to Answer Questions from Millions of Narrated Videos
-
Keywords:
Computer vision, Natural language processing, Deep learning
-
Functional Description:
Code, datasets and models associated with the paper "Just Ask: Learning to Answer Questions from Millions of Narrated Videos"
- URL:
-
Contact:
Antoine Yang
7.1.8 Pinocchio
-
Name:
Pinocchio
-
Keywords:
Robotics, Biomechanics, Mechanical multi-body systems
-
Functional Description:
Pinocchio instantiates state-of-the-art Rigid Body Algorithms for poly-articulated systems based on revisited Roy Featherstone's algorithms. In addition, Pinocchio instantiates analytical derivatives of the main Rigid-Body Algorithms like the Recursive Newton-Euler Algorithms or the Articulated-Body Algorithm. Pinocchio is first tailored for legged robotics applications, but it can be used in extra contexts. It is built upon Eigen for linear algebra and FCL for collision detection. Pinocchio comes with a Python interface for fast code prototyping.
- URL:
-
Contact:
Justin Carpentier
-
Partner:
CNRS
7.1.9 ProxSuite
-
Name:
ProxSuite
-
Keywords:
Conic optimization, Linear optimization, Robotics
-
Functional Description:
ProxSuite is a collection of open-source, numerically robust, precise and efficient numerical solvers (e.g., LPs, QPs, etc.) rooted in revisited primal-dual proximal algorithms. Through ProxSuite, we aim to offer the community scalable optimizers that can deal with dense, sparse or matrix-free problems. While the first targeted application is Robotics, ProxSuite can be used in other contexts without limits.
ProxSuite is actively developed and supported by the Willow and Sierra research groups, joint research teams between Inria, École Normale Supérieure de Paris and Centre National de la Recherche Scientifique localized in France.
-
Contact:
Justin Carpentier
7.1.10 SPE
-
Name:
Semantics Preserving Encoder
-
Keywords:
NLP, Adversarial attack, Word embeddings
-
Functional Description:
Semantics Preserving Encoder is a simple, fully supervised sentence embedding technique for textual adversarial attacks.
- URL:
-
Contact:
Hugo Cisneros
-
Participants:
Hugo Cisneros, David Herel, Daniela Hradilová
7.1.11 TubeDETR
-
Name:
TubeDETR: Spatio-Temporal Video Grounding with Transformers
-
Keywords:
Computer vision, Natural language processing, Deep learning
-
Functional Description:
Code, datasets and models associated with the paper "TubeDETR: Spatio-Temporal Video Grounding with Transformers"
- URL:
-
Contact:
Antoine Yang
7.1.12 vil3dref
-
Name:
Language Conditioned Spatial Relation Reasoning for 3D Object Grounding
-
Keyword:
Computer vision
-
Functional Description:
Open source release of the software package for the NeurIPS'22 paper by Chen et al. "Language Conditioned Spatial Relation Reasoning for 3D Object Grounding". This release provides a full implementation of the method, including code for training models, and testing on standard datasets, as well as trained models.
- URL:
- Publication:
-
Contact:
Shizhe Chen
-
Participants:
Shizhe Chen, Pierre-Louis Guhur, Makarand Tapaswi, Cordelia Schmid, Ivan Laptev
7.1.13 VLN-DUET
-
Name:
Think Global, Act Local: Dual-scale Graph Transformer for Vision-and-Language Navigation
-
Keyword:
Computer vision
-
Functional Description:
Open source release of the software package for the CVPR'22 paper by Chen et al. "Think Global, Act Local: Dual-scale Graph Transformer for Vision-and-Language Navigation". This release provides a full implementation of the method, including codes for training models, and testing on standard datasets, as well as trained models.
- URL:
- Publication:
-
Contact:
Shizhe Chen
-
Participants:
Shizhe Chen, Pierre-Louis Guhur, Makarand Tapaswi, Cordelia Schmid, Ivan Laptev
Participants: Jean Ponce, Justin Carpentier, Cordelia Schmid, Ivan Laptev, Etienne Arlaud, Pierre-Guillaume Raverdy, Stephane Caron, Shizhe Chen.
7.2 New platforms
Together with SED we are bulding the new robotics laboratory at Inria Paris located on the 5th floor of the C building. This laboratory is currently composed of two robotic anthropomorphic arms for manipulation experiments mounted on a fixed frame basement, the Tigao++ robot equipped with a manipulator and a moving platform as well as the SOLO quadruped robot. The robotics laboratory is also equipped with a dedicated Motion Capture system for precise object localization and robot calibration. These robotic patforms will enable our future research and experiments with locomotion navigation and manipulation.
In 2023, we have made the acquisition of one ALEGRO hand for dexterous manipulation to achieve fine manipulation of everydaylife objects.
7.3 Open data
8 New results
8.1 Visual recognition and reconstruction of images and videos
8.1.1 Online 3D Scene Reconstruction Using Neural Object Priors
Participants: Thomas Chabal, Shizhe Chen, Jean Ponce, Cordelia Schmid.
This paper 19 addresses the problem of reconstructing a scene online at the level of objects given an RGB-D video sequence. While current object-aware neural implicit representations hold promise, they are limited in online reconstruction efficiency and shape completion. Our main contributions to alleviate the above limitations are twofold. First, we propose a feature grid interpolation mechanism to continuously update grid-based object-centric neural implicit representations as new object parts are revealed. Second, we construct an object library with previously mapped objects in advance and leverage the corresponding shape priors to initialize geometric object models in new videos, subsequently completing them with novel views as well as synthesized past views to avoid losing original object details. Extensive experiments on synthetic environments from the Replica dataset, real-world ScanNet sequences and videos captured in our laboratory demonstrate that our approach outperforms state-of-the-art neural implicit models for this task in terms of reconstruction accuracy and completeness.
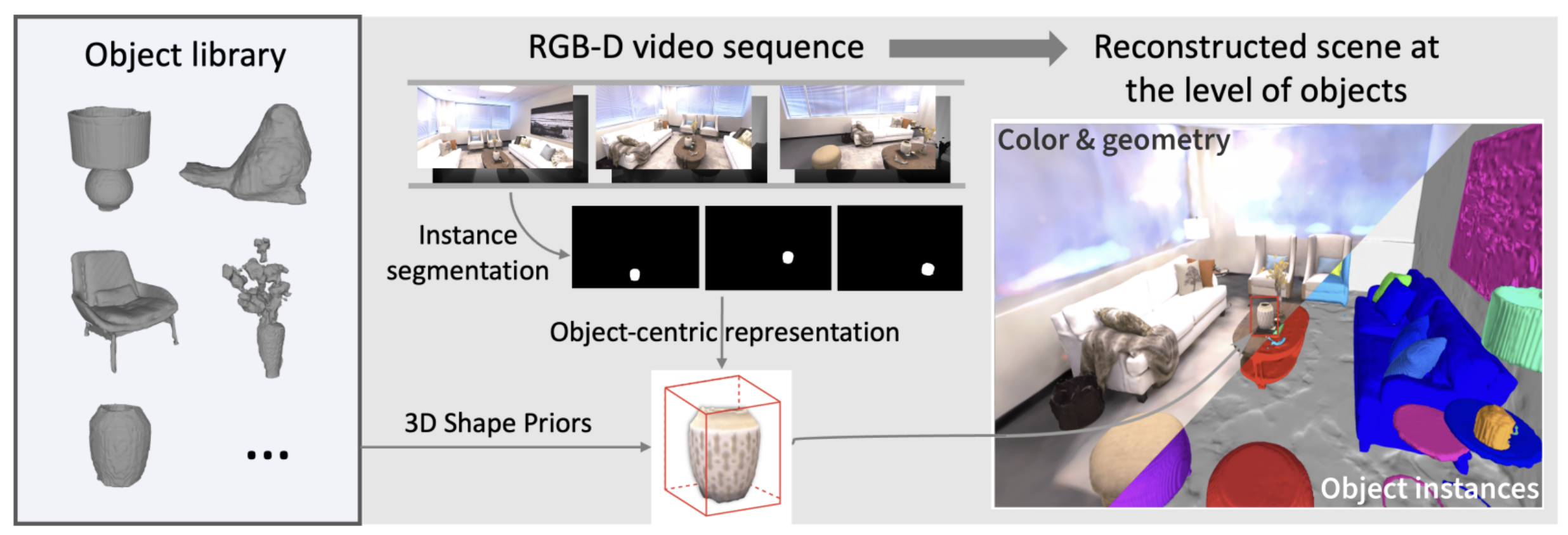
8.1.2 CoVR-2: Automatic Data Construction for Composed Video Retrieval
Participants: Lucas Ventura, Antoine Yang, Cordelia Schmid, Gül Varol.
Composed Image Retrieval (CoIR) has recently gained popularity as a task that considers both text and image queries together, to search for relevant images in a database. Most CoIR approaches require manually annotated datasets, comprising image-text-image triplets, where the text describes a modification from the query image to the target image. However, manual curation of CoIR triplets is expensive and prevents scalability. In this work 15, we instead propose a scalable automatic dataset creation methodology that generates triplets given video-caption pairs, while also expanding the scope of the task to include Composed Video Retrieval (CoVR). To this end, we mine paired videos with a similar caption from a large database, and leverage a large language model to generate the corresponding modification text. Applying this methodology to the extensive WebVid2M collection, we automatically construct our WebVid-CoVR dataset, resulting in 1.6 million triplets. Moreover, we introduce a new benchmark for CoVR with a manually annotated evaluation set, along with baseline results. We further validate that our methodology is equally applicable to image-caption pairs, by generating 3.3 million CoIR training triplets using the Conceptual Captions dataset. Our model builds on BLIP-2 pretraining, adapting it to composed video (or image) retrieval, and incorporates an additional caption retrieval loss to exploit extra supervision beyond the triplet, which is possible since captions are readily available for our training data by design. We provide extensive ablations to analyze the design choices on our new CoVR benchmark. Our experiments also demonstrate that training a CoVR model on our datasets effectively transfers to CoIR, leading to improved state-of-the-art performance in the zero-shot setup on the CIRR, FashionIQ, and CIRCO benchmarks. Our code, datasets, and models are publicly available at here.
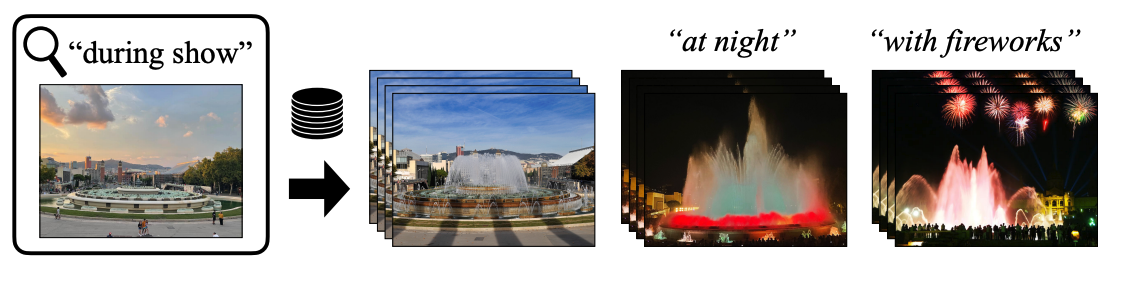
8.1.3 Historical Printed Ornaments: Dataset and Tasks
Participants: Sayan Kumar Chaki, Zeynep Sonat Baltaci, Elliot Vincent, Rémi Emonet, Fabienne Vial-Bonacci, Christelle Bahier-Porte, Mathieu Aubry, Thierry Fournel.
This paper 20 aims to develop the study of historical printed ornaments with modern unsupervised computer vision. We highlight three complex tasks that are of critical interest to book historians: clustering, element discovery, and unsupervised change localization. For each of these tasks, we introduce an evaluation benchmark, and we adapt and evaluate state-of-the-art models. Our Rey’s Ornaments dataset is designed to be a representative example of a set of ornaments historians would be interested in. It focuses on an XVIIIth century bookseller, Marc-Michel Rey, providing a consistent set of ornaments with a wide diversity and representative challenges. Our results highlight the limitations of state-of-the-art models when faced with real data and show simple baselines such as k-means or congealing can outperform more sophisticated approaches on such data. Our dataset and code can be found at here.
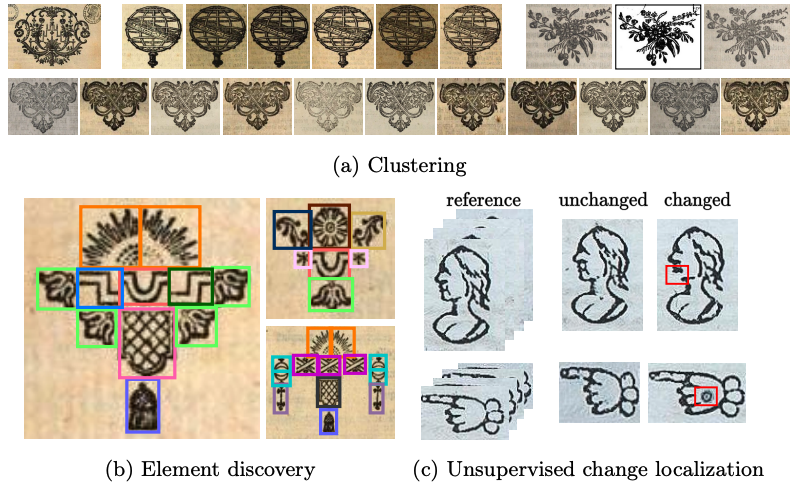
8.1.4 Satellite Image Time Series Semantic Change Detection: Novel Architecture and Analysis of Domain Shift
Participants: Elliot Vincent, Jean Ponce, Mathieu Aubry.
Satellite imagery plays a crucial role in monitoring changes happening on Earth's surface and aiding in climate analysis, ecosystem assessment, and disaster response. In this paper 53, we tackle semantic change detection with satellite image time series (SITS-SCD) which encompasses both change detection and semantic segmentation tasks. We propose a new architecture that improves over the state of the art, scales better with the number of parameters, and leverages long-term temporal information. However, for practical use cases, models need to adapt to spatial and temporal shifts, which remains a challenge. We investigate the impact of temporal and spatial shifts separately on global, multi-year SITS datasets using DynamicEarthNet and MUDS. We show that the spatial domain shift represents the most complex setting and that the impact of temporal shift on performance is more pronounced on change detection than on semantic segmentation, highlighting that it is a specific issue deserving further attention.
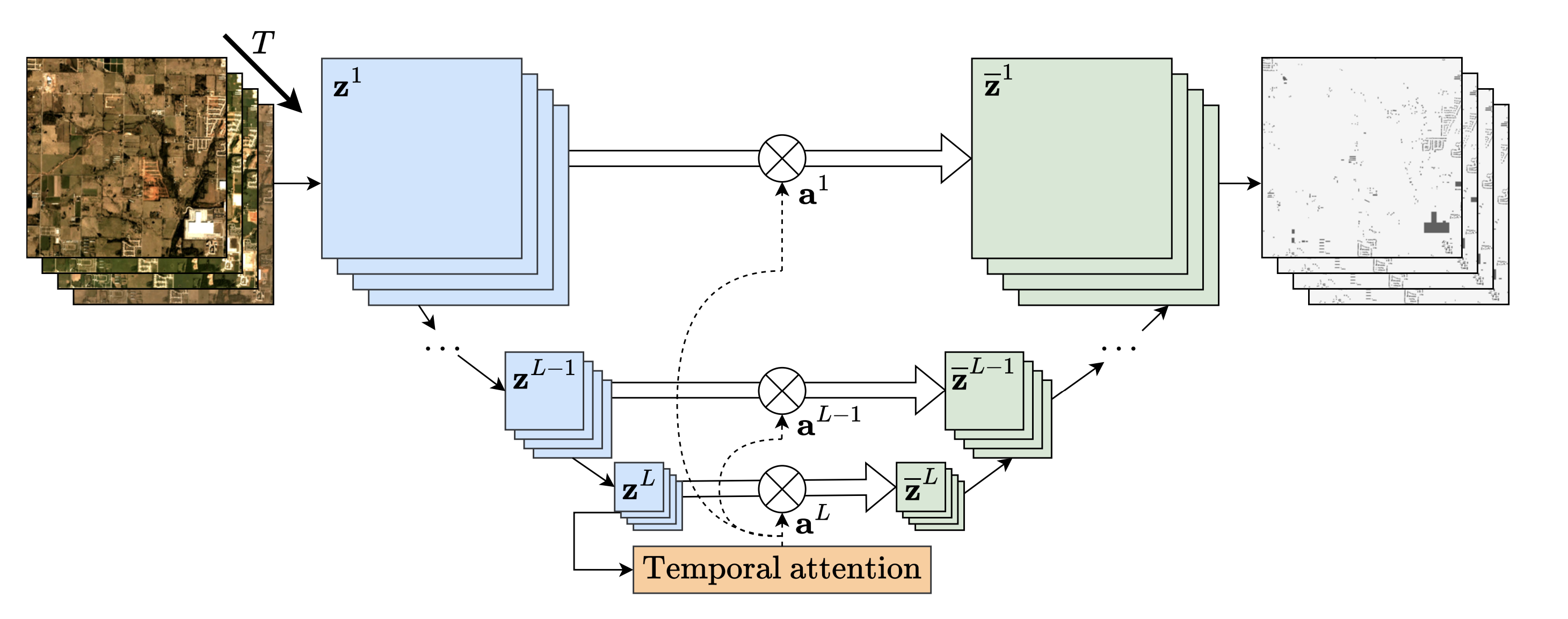
8.1.5 Detecting Looted Archaeological Sites from Satellite Image Time Series
Participants: Elliot Vincent, Mehraïl Saroufim, Jonathan Chemla, Yves Ubelmann, Philippe Marquis, Jean Ponce, Mathieu Aubry.
Archaeological sites are the physical remains of past human activity and one of the main sources of information about past societies and cultures. However, they are also the target of malevolent human actions, especially in countries having experienced inner turmoil and conflicts. Because monitoring these sites from space is a key step towards their preservation, we introduce the DAFA Looted Sites dataset 54, a labeled multi-temporal remote sensing dataset containing 55,480 images acquired monthly over 8 years across 675 Afghan archaeological sites, including 135 sites looted during the acquisition period. It is particularly challenging because of the limited number of training samples, the class imbalance, the weak binary annotations only available at the level of the time series, and the subtlety of relevant changes coupled with important irrelevant ones over a long time period. It is also an interesting playground to assess the performance of satellite image time series (SITS) classification methods on a real and important use case. We evaluate a large set of baselines, outline the substantial benefits of using foundation models and show the additional boost that can be provided by using complete time series instead of using a single image.
8.1.6 A Grasping Movement Intention Estimator for Intuitive Control of Assistive Devices
Participants: Etienne Moullet, Justin Carpentier, Christine Azevedo Coste, François Bailly.
This study 28 introduces i-GRIP, an innovative movement goal estimator designed to facilitate the control of assistive devices for grasping tasks in individuals with upperlimb impairments. The algorithm operates within a collaborative control paradigm, eliminating the need for specific user actions apart from naturally moving their hand toward a desired object. i-GRIP analyzes the hand’s movement in an object-populated scene to determine its target and select an appropriate grip. In an experimental study involving 11 healthy participants, i-GRIP showed promising goal estimation performances and responsiveness and the potential to facilitate the daily use of assistive devices for individuals with upper-limb impairments in the future.
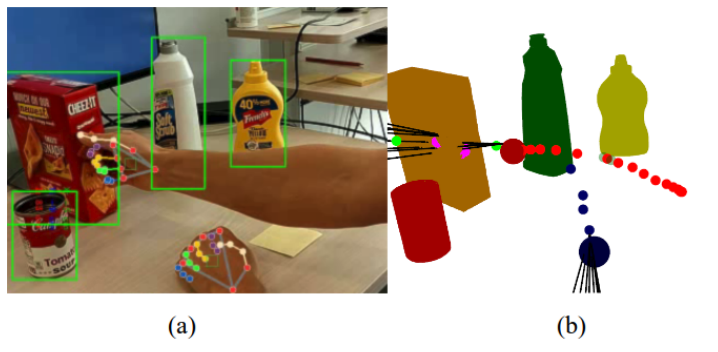
8.1.7 I-GRIP, a Grasping Movement Intention Estimator for Intuitive Control of Assistive Devices
Participants: Etienne Moullet, Justin Carpentier, Christine Azevedo Coste, François Bailly.
This study 29 describes and evaluates i-GRIP, a novel movement intention estimator designed to facilitate the control of assistive devices for grasping tasks in individuals with upper limb impairments. Operating within a collaborative grasping control paradigm, the users naturally move their hand towards an object they wish to grasp and i-GRIP identifies the target of the movement and selects an appropriate grip for the assistive device to perform. In an experimental study involving 11 healthy participants, i-GRIP exhibited promising estimation performances and responsiveness. The proposed approach paves the way towards more intuitive control of grasping assistive device for individuals with upper limb impairments.
8.1.8 PICNIQ: Pairwise Comparisons for Natural Image Quality Assessment
Participants: Nicolas Chahine, Sira Ferradans, Jean Ponce.
Blind image quality assessment (BIQA) approaches, while promising for automating image quality evaluation, often fall short in real-world scenarios due to their reliance on a generic quality standard applied uniformly across diverse images. This one-size-fits-all approach overlooks the crucial perceptual relationship between image content and quality, leading to a 'domain shift' challenge where a single quality metric inadequately represents various content types. Furthermore, BIQA techniques typically overlook the inherent differences in the human visual system among different observers. In response to these challenges, this paper 41 introduces PICNIQ, an innovative pairwise comparison framework designed to bypass the limitations of conventional BIQA by emphasizing relative, rather than absolute, quality assessment. PICNIQ is specifically designed to assess the quality differences between image pairs. The proposed framework implements a carefully crafted deep learning architecture, a specialized loss function, and a training strategy optimized for sparse comparison settings. By employing psychometric scaling algorithms like TrueSkill, PICNIQ transforms pairwise comparisons into just-objectionable-difference (JOD) quality scores, offering a granular and interpretable measure of image quality. We conduct our research using comparison matrices from the PIQ23 dataset, which are published in this paper. Our extensive experimental analysis showcases PICNIQ's broad applicability and superior performance over existing models, highlighting its potential to set new standards in the field of BIQA.
8.1.9 Generalized Portrait Quality Assessment
Participants: Nicolas Chahine, Sira Ferradans, Javier Vazquez-Corral.
Automated and robust portrait quality assessment (PQA) is of paramount importance in high-impact applications such as smartphone photography. This paper 42 presents FHIQA, a learning-based approach to PQA that introduces a simple but effective quality score rescaling method based on image semantics, to enhance the precision of fine-grained image quality metrics while ensuring robust generalization to various scene settings beyond the training dataset. The proposed approach is validated by extensive experiments on the PIQ23 benchmark and comparisons with the current state of the art. The source code of FHIQA will be made publicly available on the PIQ23 GitHub repository at here.
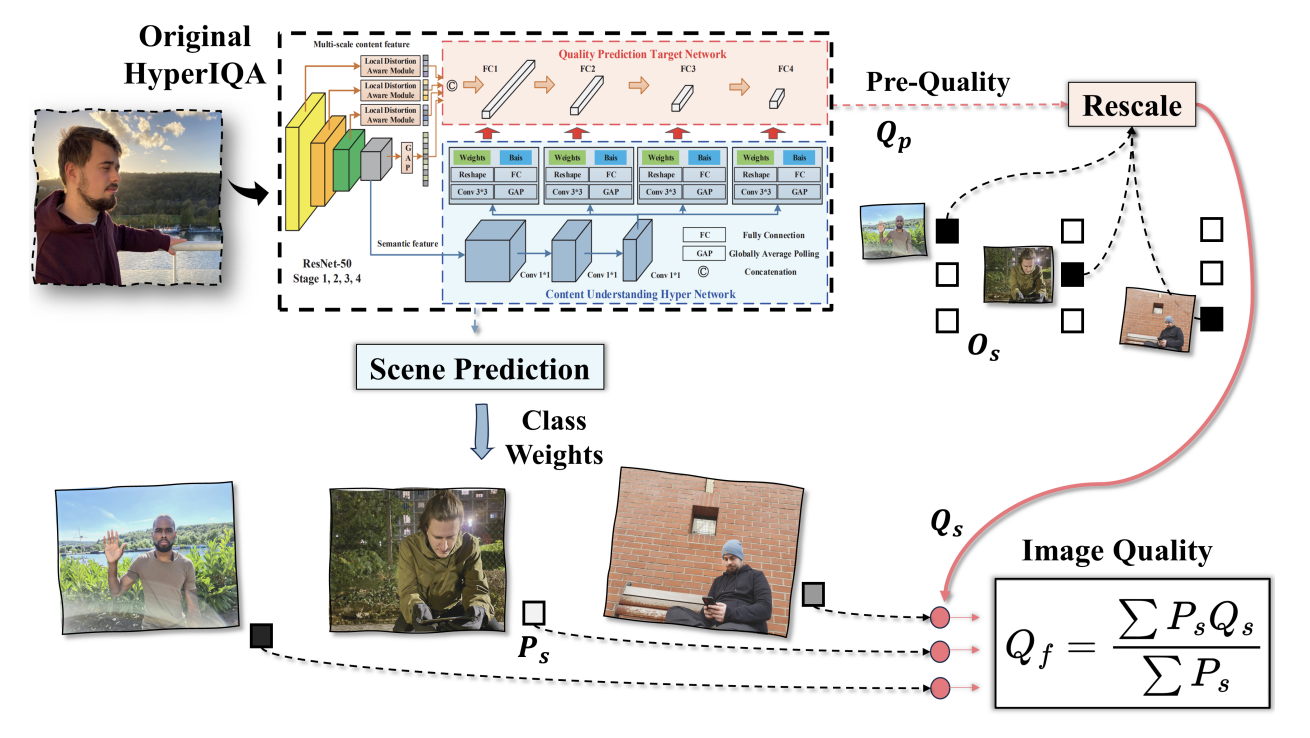
8.1.10 Towards Zero-Shot Multimodal Machine Translation
Participants: Matthieu Futeral, Cordelia Schmid, Benoît Sagot, Rachel Bawden.
Current multimodal machine translation (MMT) systems rely on fully supervised data (i.e models are trained on sentences with their translations and accompanying images). However, this type of data is costly to collect, limiting the extension of MMT to other language pairs for which such data does not exist. In this work 44, we propose a method to bypass the need for fully supervised data to train MMT systems, using multimodal English data only. Our method, called ZeroMMT, consists in adapting a strong text-only machine translation (MT) model by training it on a mixture of two objectives: visually conditioned masked language modelling and the Kullback-Leibler divergence between the original and new MMT outputs. We evaluate on standard MMT benchmarks and the recently released CoMMuTE, a contrastive benchmark aiming to evaluate how well models use images to disambiguate English sentences. We obtain disambiguation performance close to state-of-the-art MMT models trained additionally on fully supervised examples. To prove that our method generalizes to languages with no fully supervised training data available, we extend the CoMMuTE evaluation dataset to three new languages: Arabic, Russian and Chinese. We further show that we can control the trade-off between disambiguation capabilities and translation fidelity at inference time using classifier-free guidance and without any additional data. Our code, data and trained models are publicly accessible.
8.1.11 mOSCAR: A Large-scale Multilingual and Multimodal Document-level Corpus
Participants: Matthieu Futeral, Armel Zebaze, Pedro Ortiz Suarez, Julien Abadji, Rémi Lacroix, Cordelia Schmid, Rachel Bawden, Benoît Sagot.
Multimodal Large Language Models (mLLMs) are trained on a large amount of text-image data. While most mLLMs are trained on caption-like data only, Alayrac et al. [2022] showed that additionally training them on interleaved sequences of text and images can lead to the emergence of in-context learning capabilities. However, the dataset they used, M3W, is not public and is only in English. There have been attempts to reproduce their results but the released datasets are English-only. In contrast, current multilingual and multimodal datasets are either composed of caption-like only or medium-scale or fully private data. This limits mLLM research for the 7,000 other languages spoken in the world. We therefore introduce mOSCAR 45, to the best of our knowledge the first large-scale multilingual and multimodal document corpus crawled from the web. It covers 163 languages, 315M documents, 214B tokens and 1.2B images. We carefully conduct a set of filtering and evaluation steps to make sure mOSCAR is sufficiently safe, diverse and of good quality. We additionally train two types of multilingual model to prove the benefits of mOSCAR: (1) a model trained on a subset of mOSCAR and captioning data and (2) a model train on captioning data only. The model additionally trained on mOSCAR shows a strong boost in few-shot learning performance across various multilingual image-text tasks and benchmarks, confirming previous findings for English-only mLLMs.
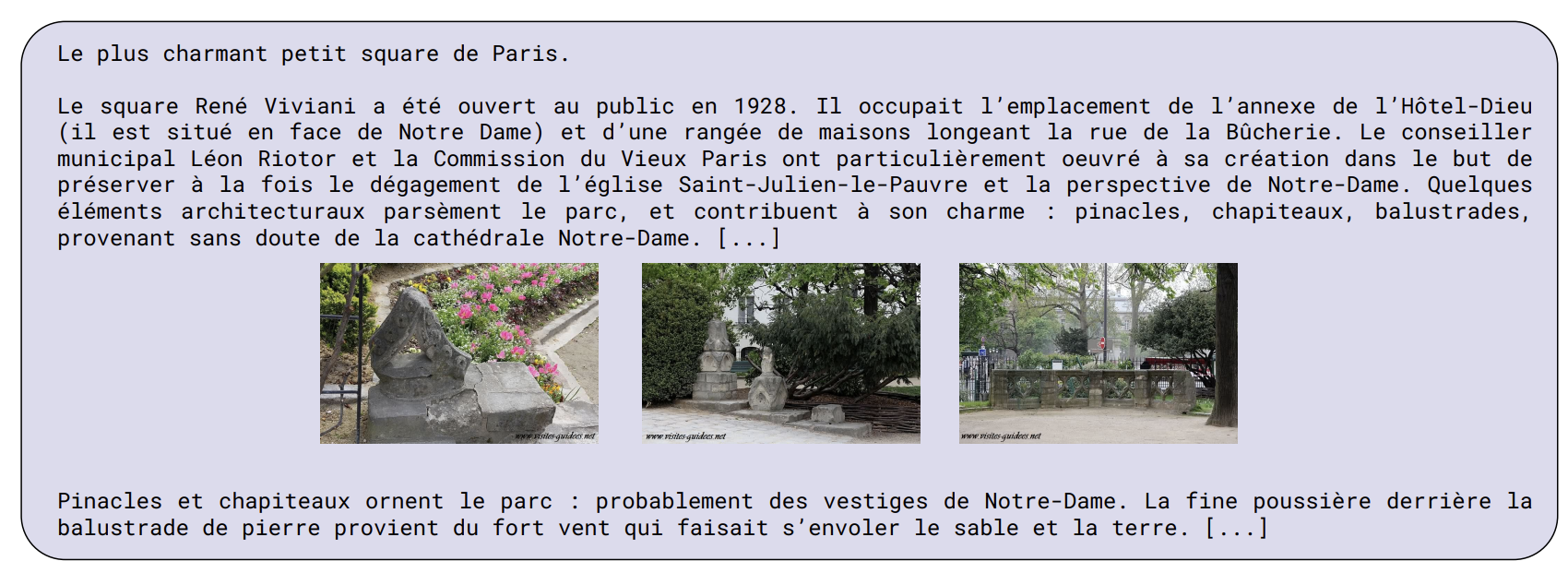
Example of a French document from mOSCAR.
8.2 Learning embodied representations
8.2.1 SUGAR: Pre-training 3D Visual Representations for Robotics
Participants: Shizhe Chen, Ricardo Garcia-Pinel, Ivan Laptev, Cordelia Schmid.
Learning generalizable visual representations from Internet data has yielded promising results for robotics. Yet, prevailing approaches focus on pre-training 2D representations, being sub-optimal to deal with occlusions and accurately localize objects in complex 3D scenes. Meanwhile, 3D representation learning has been limited to single-object understanding. To address these limitations, in this work 21, we introduce a novel 3D pre-training framework for robotics named SUGAR that captures semantic, geometric and affordance properties of objects through 3D point clouds. We underscore the importance of cluttered scenes in 3D representation learning, and automatically construct a multi-object dataset benefiting from cost-free supervision in simulation. SUGAR employs a versatile transformer-based model to jointly address five pre-training tasks, namely cross-modal knowledge distillation for semantic learning, masked point modeling to understand geometry structures, grasping pose synthesis for object affordance, 3D instance segmentation and referring expression grounding to analyze cluttered scenes. We evaluate our learned representation on three robotic-related tasks, namely, zero-shot 3D object recognition, referring expression grounding, and language-driven robotic manipulation. Experimental results show that SUGAR’s 3D representation outperforms state-of-the-art 2D and 3D representations.

8.2.2 Towards Generalizable Vision-Language Robotic Manipulation: A Benchmark and LLM-guided 3D Policy
Participants: Ricardo Garcia-Pinel, Shizhe Chen, Cordelia Schmid.
Generalizing language-conditioned robotic policies to new tasks remains a significant challenge, hampered by the lack of suitable simulation benchmarks. In this paper 46, we address this gap by introducing GemBench, a novel benchmark to assess generalization capabilities of vision-language robotic manipulation policies. GemBench incorporates seven general action primitives and four levels of generalization, spanning novel placements, rigid and articulated objects, and complex long-horizon tasks. We evaluate state-of-the-art approaches on GemBench and also introduce a new method. Our approach 3D-LOTUS leverages rich 3D information for action prediction conditioned on language. While 3D-LOTUS excels in both efficiency and performance on seen tasks, it struggles with novel tasks. To address this, we present 3D-LOTUS++, a framework that integrates 3D-LOTUS's motion planning capabilities with the task planning capabilities of LLMs and the object grounding accuracy of VLMs. 3D-LOTUS++ achieves state-of-the-art performance on novel tasks of GemBench, setting a new standard for generalization in robotic manipulation. The benchmark, codes and trained models are available at here.
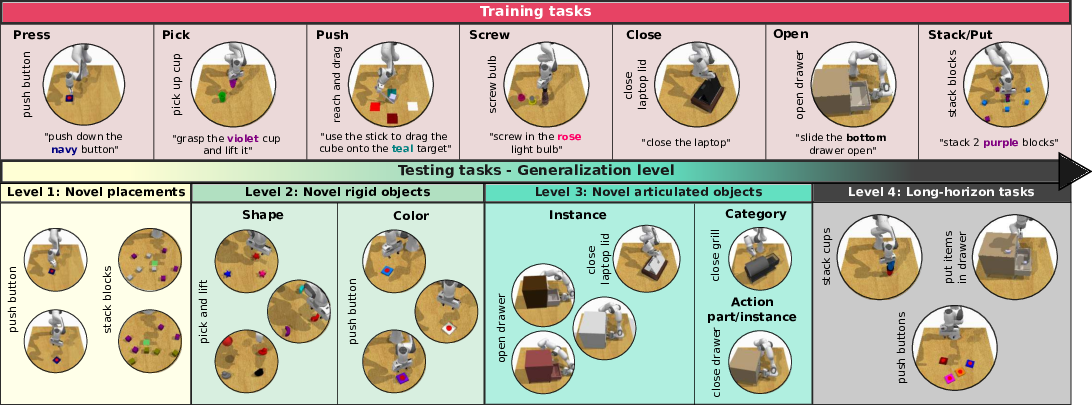
8.2.3 ViViDex: Learning Vision-based Dexterous Manipulation from Human Videos
Participants: Zerui Chen, Shizhe Chen, Etienne Arlaud, Ivan Laptev, Cordelia Schmid.
In this work 43, we aim to learn a unified vision-based policy for multi-fingered robot hands to manipulate a variety of objects in diverse poses. Though prior work has shown benefits of using human videos for policy learning, performance gains have been limited by the noise in estimated trajectories. Moreover, reliance on privileged object information such as ground-truth object states further limits the applicability in realistic scenarios. To address these limitations, we propose a new framework ViViDex to improve vision-based policy learning from human videos. It first uses reinforcement learning with trajectory guided rewards to train state-based policies for each video, obtaining both visually natural and physically plausible trajectories from the video. We then rollout successful episodes from state-based policies and train a unified visual policy without using any privileged information. We propose coordinate transformation to further enhance the visual point cloud representation, and compare behavior cloning and diffusion policy for the visual policy training. Experiments both in simulation and on the real robot demonstrate that ViViDex outperforms state-of-the-art approaches on three dexterous manipulation tasks.
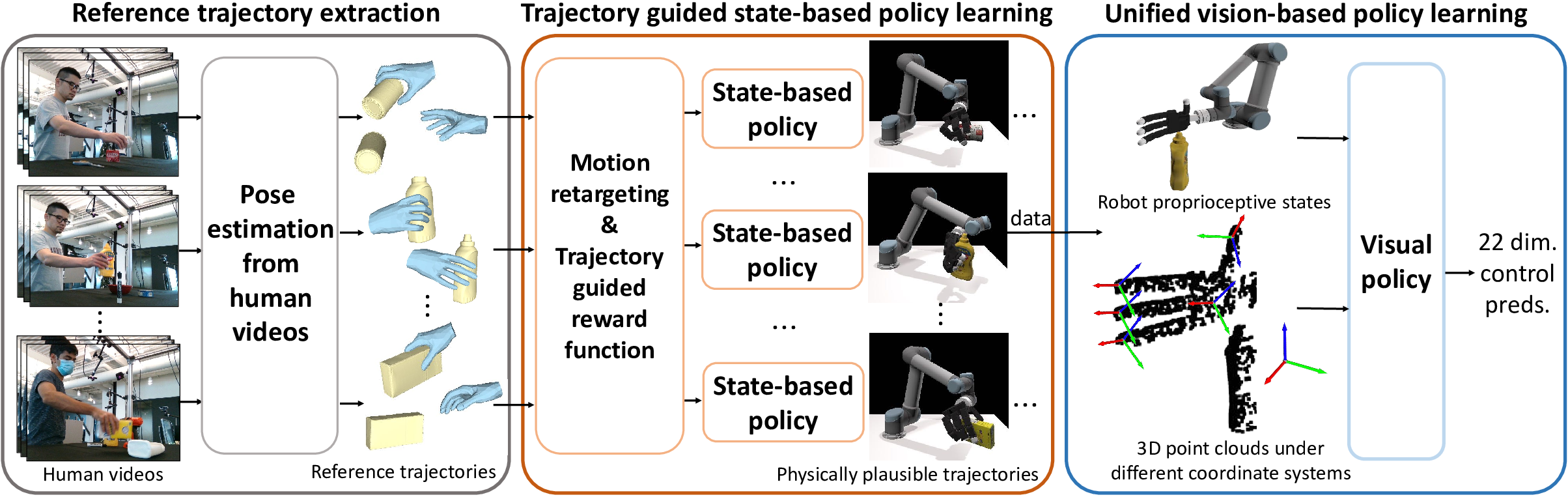
8.2.4 Leveraging augmented-Lagrangian techniques for differentiating over infeasible quadratic programs in machine learning
Participants: Antoine Bambade, Fabian Schramm, Adrien Taylor, Justin Carpentier.
Optimization layers within neural network architectures have become increasingly popular for their ability to solve a wide range of machine learning tasks and to model domain-specific knowledge. However, designing optimization layers requires careful consideration as the underlying optimization problems might be infeasible during training. Motivated by applications in learning, control and robotics, this work 16 focuses on convex quadratic programming (QP) layers. The specific structure of this type of optimization layer can be efficiently exploited for faster computations while still allowing rich modeling capabilities. We leverage primal-dual augmented Lagrangian techniques for computing derivatives of both feasible and infeasible QP solutions. More precisely, we propose a unified approach that tackles the differentiability of the closest feasible QP solutions in a classical
8.2.5 End-to-End and Highly-Efficient Differentiable Simulation for Robotics
Participants: Quentin Le Lidec, Louis Montaut, Yann de Mont-Marin, Justin Carpentier.
Over the past few years, robotics simulators have largely improved in efficiency and scalability, enabling them to generate years of simulated data in a few hours. Yet, efficiently and accurately computing the simulation derivatives remains an open challenge, with potentially high gains on the convergence speed of reinforcement learning and trajectory optimization algorithms, especially for problems involving physical contact interactions. This paper 48 contributes to this objective by introducing a unified and efficient algorithmic solution for computing the analytical derivatives of robotic simulators. The approach considers both the collision and frictional stages, accounting for their intrinsic nonsmoothness and also exploiting the sparsity induced by the underlying multibody systems. These derivatives have been implemented in C++, and the code will be open-sourced in the Simple simulator. They depict state-of-the-art timings ranging from 5 us for a 7-dof manipulator up to 95 us for 36-dof humanoid, outperforming alternative solutions by a factor of at least 100.
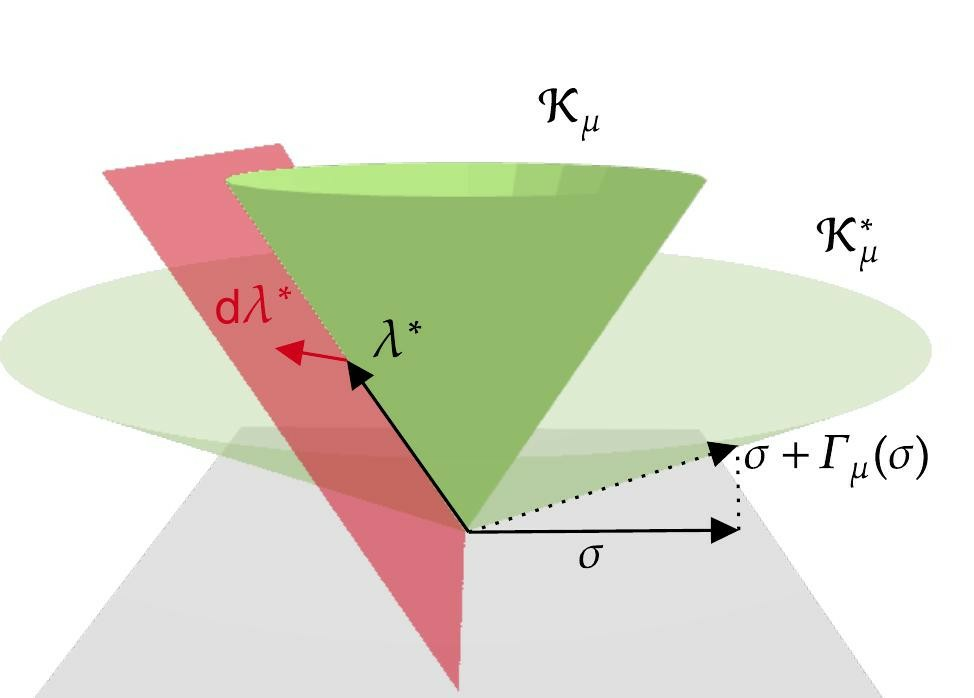
8.2.6 From centroidal to whole-body models for legged locomotion: a comparative analysis
Participants: Dantec Ewen, Jallet Wilson, Carpentier Justin.
Model predictive control is one of the most common methods for stabilizing the dynamics of a legged robot. Yet, it remains unclear which level of complexity should be considered for modeling the system dynamics. On the one hand, most embedded pipelines for legged locomotion rely on reduced models with low computational load in order to ensure real-time capabilities at the price of not exploiting the full potential of the whole-body dynamics. On the other hand, recent numerical solvers can now generate whole-body trajectories on the fly while still respecting tight time constraints. In 22 we compare the performances of common dynamic models of increasing complexity (centroidal, kino-dynamics, and whole- body models, as described in fig. 12) in simulation over locomotion problems involving challenging gaits, stairs climbing and balance recovery. We also present a 3-D kino-dynamics model that reformulates centroidal dynamics in the coordinates of the base frame by efficiently leveraging the centroidal momentum equation at the acceleration level. This comparative study uses the humanoid robot Talos and the augmented Lagrangian-based solver ALIGATOR to enforce hard constraints on the optimization problem.
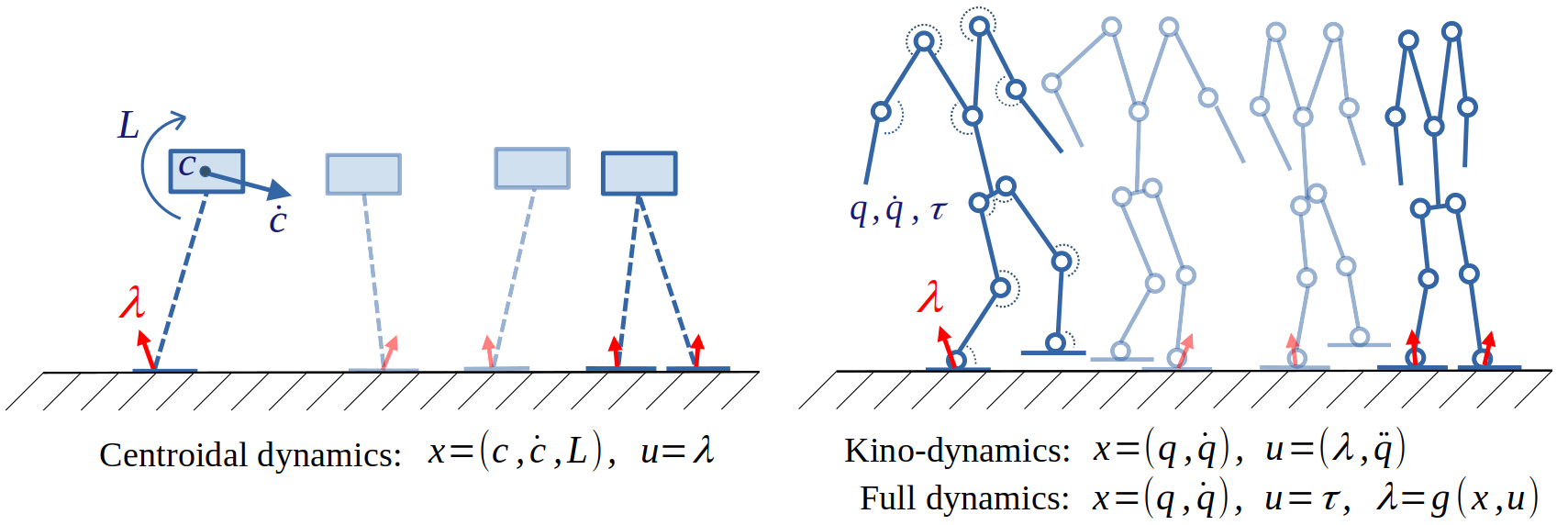
8.2.7 Condensed semi-implicit dynamics for trajectory optimizaiton in soft robotics
Participants: Etienne Ménager, Alexandre Bilger, Wilson Jallet, Justin Carpentier, Christian Duriez.
Over the past decades, trajectory optimization (TO) has become an effective solution for solving complex motion generation problems in robotics, ranging from autonomous driving to humanoids. Yet, TO methods remain limited to robots with tens of degrees of freedom (DoFs), limiting their usage in soft robotics, where kinematic models may require hundreds of DoFs in general. In 26, we introduce a generic method to perform trajectory optimization based on continuum mechanics to describe the behavior of soft robots. The core idea is to condense the dynamics of the soft robot in the constraint space in order to obtain a reduced dynamics formulation, which can then be plugged into numerical TO methods. In particular, we show that these condensed dynamics can be easily coupled with differential dynamic programming methods for solving TO problems involving soft robots. This method is evaluated on three different soft robots with different geometries and actuation, as represented in Figure 13.
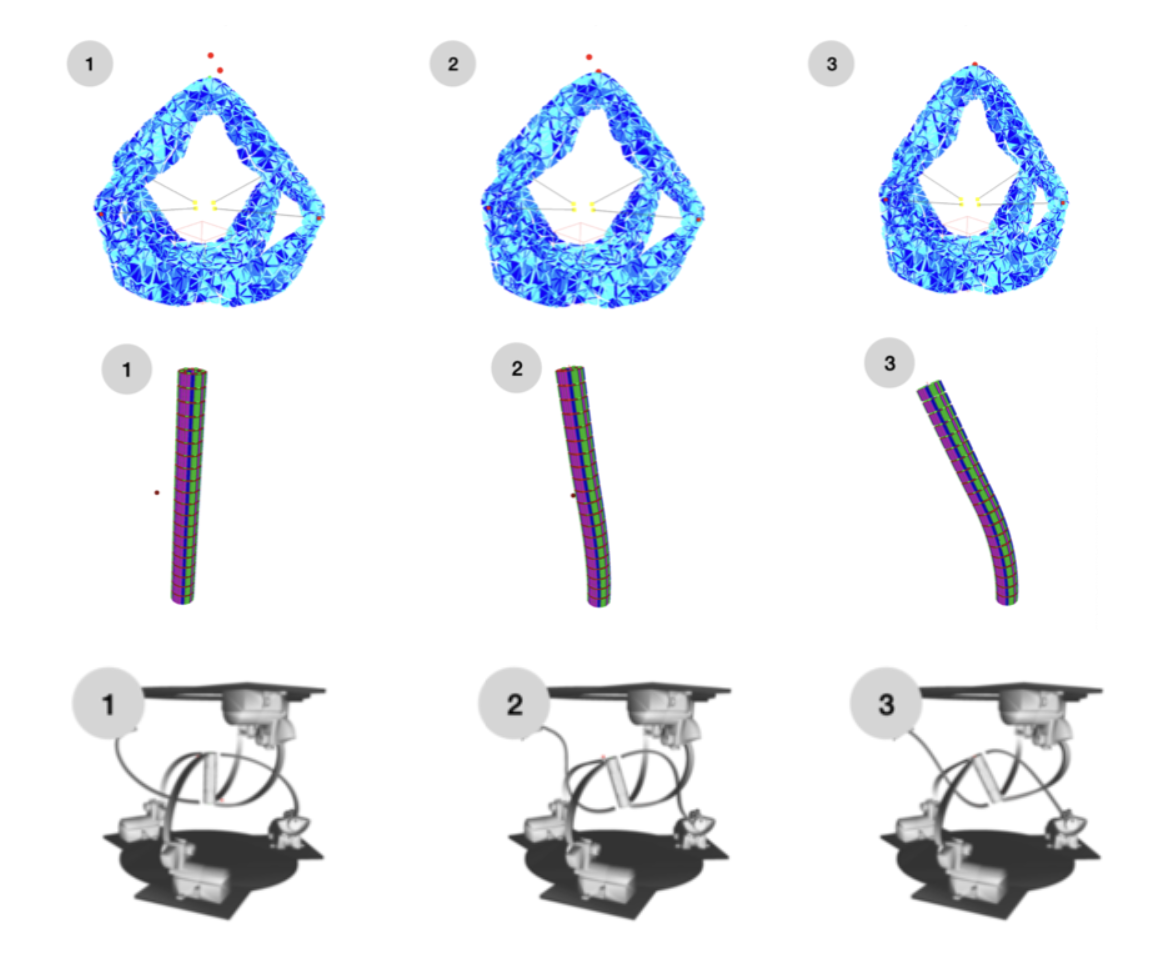
8.2.8 Constrained Articulated Body Algorithms for Closed-Loop Mechanisms
Participants: Ajay Suresha Sathya, Justin Carpentier.
Efficient rigid-body dynamics algorithms are instrumental in enabling high-frequency dynamics evaluation for resource-intensive applications (e.g., model predictive control, large-scale simulation, reinforcement learning), potentially on resource-constrained hardware. Existing recursive algorithms with low computational complexity are mostly restricted to kinematic trees with external contact constraints or are sensitive to singular cases (e.g., linearly dependent constraints and kinematic singularities), severely impacting their practical usage in existing simulators. This article 51 introduces two original lowcomplexity recursive algorithms, loop-constrained articulated body algorithm (LCABA) and proxBBO, based on proximal dynamics formulation for forward simulation of mechanisms with loops. These algorithms are derived from first principles using non-serial dynamic programming, depict linear complexity in practical scenarios, and are numerically robust to singular cases. They extend the existing constrained articulated body algorithm (constrainedABA) to handle internal loops and the pioneering BBO algorithm from the 1980s to singular cases. Both algorithms have been implemented by leveraging the open-source Pinocchio library, benchmarked in detail, and depict state-ofthe-art performance for various robot topologies, including over 6x speed-ups compared to existing non-recursive algorithms for high degree-of-freedom systems with internal loops such as recent humanoid robots.
8.2.9 A Data-driven Contact Estimation Method for Wheeled-Biped Robots
Participants: Ü. Bora Gökbakan, Frederike Dümbgen, Stéphane Caron.
Contact estimation is a key ability for limbed robots, where making and breaking contacts has a direct impact on state estimation and balance control. Existing approaches typically rely on gate-cycle priors or designated contact sensors. In this work 47, we design a contact estimator that is suitable for the emerging wheeled-biped robot types that do not have these features. To this end, we propose a Bayes filter in which update steps are learned from real-robot torque measurements while prediction steps rely on inertial measurements. We evaluate this approach in extensive real-robot and simulation experiments. Our method achieves better performance while being considerably more sample efficient than a comparable deep-learning baseline.

8.2.10 Exploiting Chordal Sparsity for Fast Global Optimality with Application to Localization
Participants: Frederike Dümbgen, Connor Holmes, Timothy D. Barfoot.
In recent years, many estimation problems in robotics have been shown to be solvable to global optimality using their semidefinite relaxations. However, the runtime complexity of off-the-shelf semidefinite programming (SDP) solvers is up to cubic in problem size, which inhibits real-time solutions of problems involving large state dimensions. In this work 23, we show that for a large class of problems, namely those with chordal sparsity, we can reduce the complexity of these solvers to linear in problem size. In particular, we show how to replace the large positive-semidefinite variable with a number of smaller interconnected ones using the well-known chordal decomposition. This formulation also allows for the straightforward application of the alternating direction method of multipliers (ADMM), which can exploit parallelism for increased scalability. We show for two example problems in simulation that the chordal solvers provide a significant speed-up over standard SDP solvers, and that global optimality is crucial in the absence of good initializations.
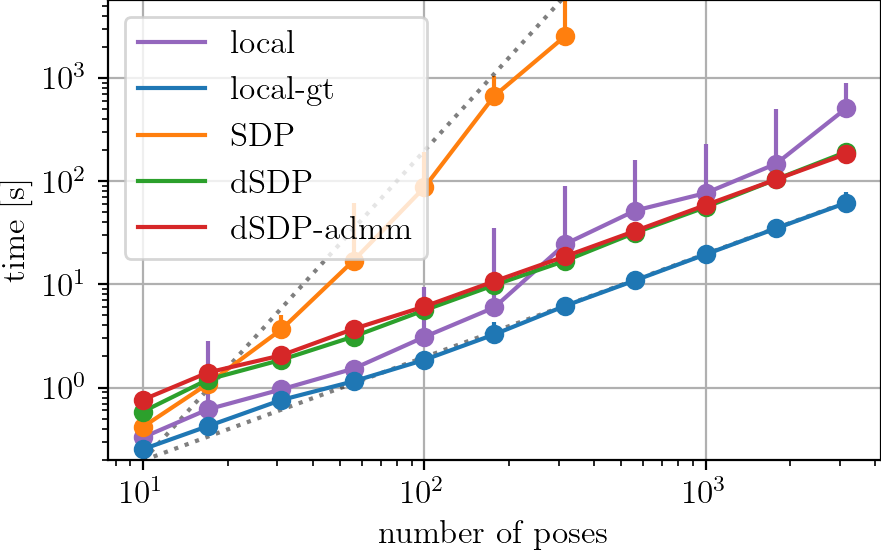
8.2.11 Parallel and Proximal Constrained Linear-Quadratic Methods for Real-Time Nonlinear MPC
Participants: Wilson Jallet, Ewen Dantec, Etienne Arlaud, Nicolas Mansard, Justin Carpentier.
Recent strides in nonlinear model predictive control (NMPC) underscore a dependence on numerical advancements to efficiently and accurately solve large-scale problems. Given the substantial number of variables characterizing typical wholebody optimal control (OC) problems —often numbering in the thousands— exploiting the sparse structure of the numerical problem becomes crucial to meet computational demands, typically in the range of a few milliseconds. Addressing the linear-quadratic regulator (LQR) problem is a fundamental building block for computing Newton or Sequential Quadratic Programming (SQP) steps in direct optimal control methods. This paper 24 concentrates on equality-constrained problems featuring implicit system dynamics and dual regularization, a characteristic of advanced interiorpoint or augmented Lagrangian solvers. Here, we introduce a parallel algorithm for solving an LQR problem with dual regularization. Leveraging a rewriting of the LQR recursion through block elimination, we first enhanced the efficiency of the serial algorithm and then subsequently generalized it to handle parametric problems. This extension enables us to split decision variables and solve multiple subproblems concurrently. Our algorithm is implemented in our nonlinear numerical optimal control library ALIGATOR1 . It showcases improved performance over previous serial formulations and we validate its efficacy by deploying it in the model predictive control of a real quadruped robot.
8.2.12 Force Feedback Model-Predictive Control via Online Estimation
Participants: Armand Jordana, Sébastien Kleff, Justin Carpentier, Nicolas Mansard, Ludovic Righetti.
Nonlinear model-predictive control has recently shown its practicability in robotics. However it remains limited in contact interaction tasks due to its inability to leverage sensed efforts. In this work 25, we propose a novel model-predictive control approach that incorporates direct feedback from force sensors while circumventing explicit modeling of the contact force evolution. Our approach is based on the online estimation of the discrepancy between the force predicted by the dynamics model and force measurements, combined with high-frequency nonlinear model-predictive control. We report an experimental validation on a torque-controlled manipulator in challenging tasks for which accurate force tracking is necessary. We show that a simple reformulation of the optimal control problem combined with standard estimation tools enables to achieve state-of-the-art performance in force control while preserving the benefits of model-predictive control, thereby outperforming traditional force control techniques. This work paves the way toward a more systematic integration of force sensors in model predictive control.
8.2.13 Toward Globally Optimal State Estimation Using Automatically Tightened Semidefinite Relaxations
Participants: Frederike Dümbgen, Connor Holmes, Ben Argo, Timothy D. Barfoot.
In recent years, semidefinite relaxations of common optimization problems in robotics have attracted growing attention due to their ability to provide globally optimal solutions. In many cases, it was shown that specific handcrafted redundant constraints are required to obtain tight relaxations and thus global optimality. Figure 16 visualizes this process. These constraints are formulation-dependent and typically identified through a lengthy manual process. Instead, in this work 7, we suggest an automatic method to find a set of sufficient redundant constraints to obtain tightness, if they exist. We first propose an efficient feasibility check to determine if a given set of variables can lead to a tight formulation. Secondly, we show how to scale the method to problems of bigger size. At no point of the process do we have to find redundant constraints manually. We showcase the effectiveness of the approach, in simulation and on real datasets, for range-based localization and stereo-based pose estimation. Finally, we reproduce semidefinite relaxations presented in recent literature and show that our automatic method always finds a smaller set of constraints sufficient for tightness than previously considered.
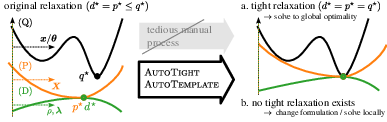
8.2.14 Co-designing versatile quadruped robots for dynamic and energy-efficient motions
Participants: Gabriele Fadini, Shivesh Kumar, Rohit Kumar, Thomas Flayols, Andrea del Prete, Justin Carpentier, Philippe Souères.
This paper 8 presents a bi-level optimization framework to concurrently optimize a quadruped hardware and control policies for achieving dynamic cyclic behaviors. The longterm vision to drive the design of dynamic and efficient robots by means of computational techniques is applied to improve the development of a new quadruped prototype. The scale of the robot and its actuators are optimized for energy efficiency considering a complete model of the motor, that includes friction, torque, and bandwidth limitations. This model is used to optimize the power consumption during bounding and backflip tasks and is validated by tracking the output trajectories on the first prototype iteration. The co-design results show an improvement of up to 87% for a single task optimization. It appears that, for jumping forward, robots with longer thighs perform better, while for backflips, longer shanks are better suited. To understand the trade-off between these different choices, a Pareto set is constructed to guide the design of the next prototype.
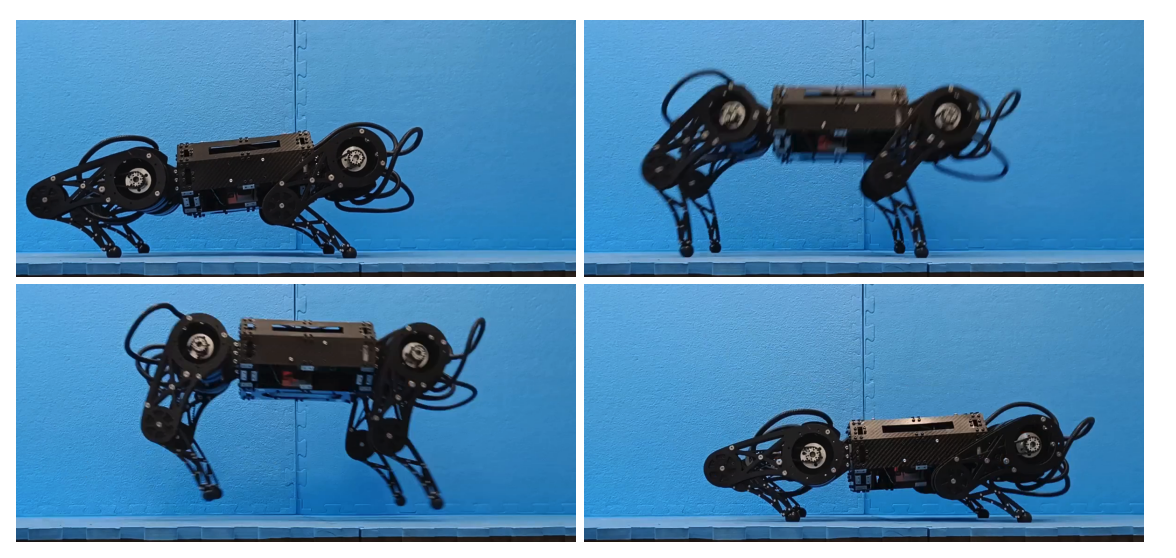
Quadruped prototype bounding tests at DFKI-RIC.
8.2.15 Geodesic turnpikes for robot motion planning
Participants: Yann de Mont-Marin, Martial Hebert, Jean Ponce.
Every motion planning algorithm relies on a distance and its geodesics in the configuration space. Characterizing and accelerating the computation of the geodesic is an important stake in robot motion planning. In 27, we study the geodesics in the configuration space of robots, focusing on their properties to enhance motion planning. Motivated by the observed turnpike behavior in geodesics—where transient phases lead to steady states resembling prototypical trajectories—we provide a formal framework for defining and characterizing geodesic turnpikes using vector fields on Finsler manifolds. For Lie groups with left-invariant Finsler distances, we propose a conjecture linking turnpikes to specific vector fields in the Lie algebra. As an illustrative example (see Fig. 18), this framework is applied to SE(2) equipped with minimum swept-volume distances, demonstrating how turnpikes accelerate geodesic computation and improve motion planning efficiency.
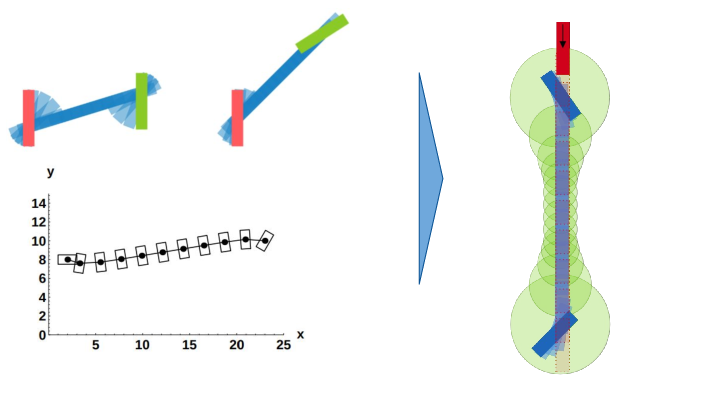
8.2.16 Reconciling RaiSim with the Maximum Dissipation Principle
Participants: Quentin Le Lidec, Justin Carpentier.
Recent progress in reinforcement learning (RL) in robotics has been obtained by training control policy directly in simulation. Particularly in the context of quadrupedal locomotion, astonishing locomotion policies depicting high robustness against environmental perturbations have been trained by leveraging RaiSim simulator. While it avoids introducing forces at distance, it has been shown recently that RaiSim does not obey the maximum dissipation principle, a fundamental principle when simulating rigid contact interactions. In this note 11, we detail these relaxations and propose an algorithmic correction of the RaiSim contact algorithm to handle the maximum dissipation principle adequately. Our experiments empirically demonstrate our approach leads to simulation following this fundamental principle.
8.2.17 Contact Models in Robotics: a Comparative Analysis
Participants: Quentin Le Lidec, Wilson Jallet, Louis Montaut, Ivan Laptev, Cordelia Schmid, Justin Carpentier.
Physics simulation is ubiquitous in robotics. Whether in model-based approaches (e.g., trajectory optimization), or model-free algorithms (e.g., reinforcement learning), physics simulators are a central component of modern control pipelines in robotics. Over the past decades, several robotic simulators have been developed, each with dedicated contact modeling assumptions and algorithmic solutions. In this article 12, we survey the main contact models and the associated numerical methods commonly used in robotics for simulating advanced robot motions involving contact interactions. In particular, we recall the physical laws underlying contacts and friction (i.e., Signorini condition, Coulomb’s law, and the maximum dissipation principle), and how they are transcribed in current simulators. For each physics engine, we expose their inherent physical relaxations along with their limitations due to the numerical techniques employed. Based on our study, we propose theoretically grounded quantitative criteria on which we build benchmarks assessing both the physical and computational aspects of simulation. We support our work with an open-source and efficient C++ implementation of the existing algorithmic variations. Our results demonstrate that some approximations or algorithms commonly used in robotics can severely widen the reality gap and impact target applications. We hope this work will help motivate the development of new contact models, contact solvers, and robotic simulators in general, at the root of recent progress in motion generation in robotics.
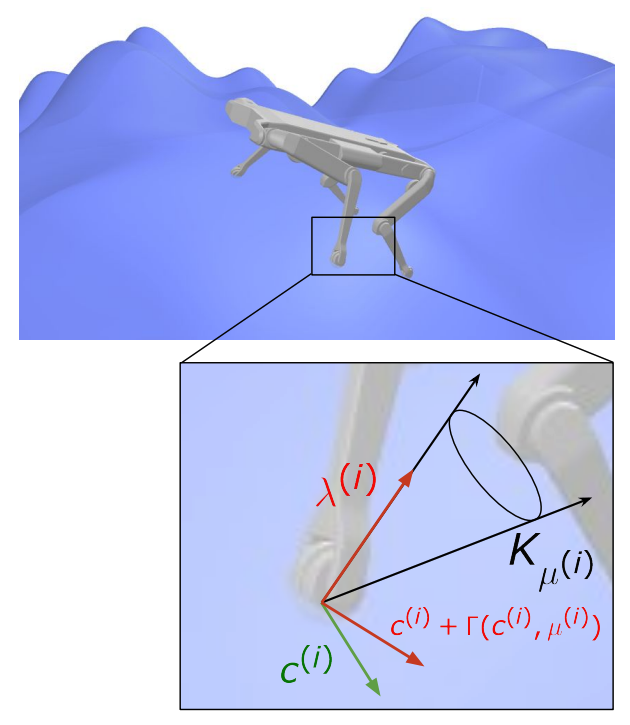
8.2.18 Certifiably optimal rotation and pose estimation based on the Cayley map
Participants: Timothy D Barfoot, Connor Holmes, Frederike Dümbgen.
We present novel, convex relaxations for rotation and pose estimation problems that can a posteriori guarantee global optimality for practical measurement noise levels. Some such relaxations exist in the literature for specific problem setups that assume the matrix von Mises-Fisher distribution (a.k.a., matrix Langevin distribution or chordal distance) for isotropic rotational uncertainty. However, another common way to represent uncertainty for rotations and poses is to define anisotropic noise in the associated Lie algebra. Starting from a noise model based on the Cayley map, we define our estimation problems, convert them to Quadratically Constrained Quadratic Programs (QCQPs), then relax them to Semidefinite Programs (SDPs), which can be solved using standard interior-point optimization methods; global optimality follows from Lagrangian strong duality. We first show how to carry out basic rotation and pose averaging. We then turn to the more complex problem of trajectory estimation, which involves many pose variables with both individual and inter-pose measurements (or motion priors). Our contribution 5 is to formulate SDP relaxations for all these problems based on the Cayley map (including the identification of redundant constraints) and to show them working in practical settings. We hope our results can add to the catalogue of useful estimation problems whose solutions can be a posteriori guaranteed to be globally optimal.
8.2.19 CLEO: Closed-Loop kinematics Evolutionary Optimization of bipedal structures
Participants: Virgile Batto, Ludovic de Matteis, Thomas Flayols, Margot Vulliez, Nicolas Mansard.
Progress in hardware design is crucial for achieving better artificial locomotion. There is a significant ongoing effort in the community to leverage dynamic capabilities of serialparallel architectures in the design of humanoid legs. However, designing such systems involves addressing high-dimensional, complex, and multi-objective challenges, where conventional optimization approaches may encounter limitations or rely on additional know-how of the human designer. In this paper 40, we propose a general approach to assist the design of serial-parallel humanoid legs using an evolutionary optimization strategy. The optimization problem incorporates design constraints and locomotion-task requirements as objective functions. It uses parallelized trajectory evaluation for efficient exploration of the design space. The effectiveness of the design methodology is shown by optimizing a new leg architecture, that fully exploits the capabilities offered by kinematic closure. The optimized leg design meets practical constraints related to foot position, joint placement, and force transmission, ensuring stability and locomotion performance. We improve our design robustness by including several criteria that increase structural stiffness. The optimized leg exhibits desirable properties and matches the required simulation design constraints. Furthermore, we compare 3D-printed prototypes and experimentally validate the impact and choice of the design criteria.
8.2.20 On Semidefinite Relaxations for Matrix-Weighted State-Estimation Problems in Robotics
Participants: Connor Holmes, Frederike Dümbgen, Timothy D Barfoot.
In recent years, there has been remarkable progress in the development of so-called certifiable perception methods, which leverage semidefinite, convex relaxations to find global optima of perception problems in robotics. However, many of these relaxations rely on simplifying assumptions that facilitate the problem formulation, such as an isotropic measurement noise distribution. In this paper 10, we explore the tightness of the semidefinite relaxations of matrix-weighted (anisotropic) stateestimation problems and reveal the limitations lurking therein: matrix-weighted factors can cause convex relaxations to lose tightness. In particular, we show that the semidefinite relaxations of localization problems with matrix weights may be tight only for low noise levels. To better understand this issue, we introduce a theoretical connection between the posterior uncertainty of the state estimate and the certificate matrix obtained via convex relaxation. With this connection in mind, we empirically explore the factors that contribute to this loss of tightness and demonstrate that redundant constraints can be used to regain it. As a second technical contribution of this paper, we show that the stateof-the-art relaxation of scalar-weighted SLAM cannot be used when matrix weights are considered. We provide an alternate formulation and show that its SDP relaxation is not tight (even for very low noise levels) unless specific redundant constraints are used. We demonstrate the tightness of our formulations on both simulated and real-world data.
8.2.21 Leveraging Randomized Smoothing for Optimal Control of Nonsmooth Dynamical Systems
Participants: Quentin Le Lidec, Fabian Schramm, Louis Montaut, Cordelia Schmid, Ivan Laptev, Justin Carpentier.
Optimal control (OC) algorithms such as differential dynamic programming (DDP) take advantage of the derivatives of the dynamics to control physical systems efficiently. Yet, these algorithms are prone to failure when dealing with non-smooth dynamical systems. This can be attributed to factors such as the existence of discontinuities in the dynamics derivatives or the presence of non-informative gradients. On the contrary, reinforcement learning (RL) algorithms have shown better empirical results in scenarios exhibiting nonsmooth effects (contacts, frictions, etc.). Our approach 13 leverages recent works on randomized smoothing (RS) to tackle non-smoothness issues commonly encountered in optimal control and provides key insights on the interplay between RL and OC through the prism of RS methods. This naturally leads us to introduce the randomized Differential Dynamic Programming (RDDP) algorithm accounting for deterministic but non-smooth dynamics in a very sample-efficient way. The experiments demonstrate that our method can solve classic robotic problems with dry friction and frictional contacts, where classical OC algorithms are likely to fail, and RL algorithms require, in practice, a prohibitive number of samples to find an optimal solution.
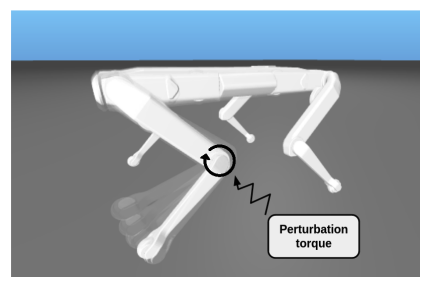
8.2.22 Optimal Control of Walkers with Parallel Actuation
Participants: Ludovic de Matteis, Virgile Batto, Justin Carpentier, Nicolas Mansard.
Legged robots with complex kinematic architectures, such as parallel linkages, offer significant advancements in mobility and efficiency. However, generating versatile movements for these robots requires accurate dynamic modeling that reflects their specific mechanical structures. Previous approaches often relied on simplified models, resulting in sub-optimal control, particularly in tasks requiring the full actuator range. Here 50, we present a method that fully models the dynamics of legged robots with parallel linkages, formulating their motion generation as an optimal control problem with specific contact dynamics. We introduce 6D kinematic closure constraints and derive their analytical derivatives, enabling the solver to exploit nonlinear transmission and the consequent variable actuator reduction. This approach reduces peak motor torques and expands the usable range of actuator motion and force. We empirically demonstrate that fully modeling the kinematics leads to superior performance, especially in demanding tasks such as fast walking and stair climbing. Beyond serialparallel designs, our method also addresses motion generation for fully-parallel walkers.
8.2.23 Constrained Articulated Body Dynamics Algorithms
Participants: Ajay Sathya, Justin Carpentier.
Rigid-body dynamics algorithms have played an essential role in robotics development. By finely exploiting the underlying robot structure, they allow the computation of the robot kinematics, dynamics, and related physical quantities with low complexity, enabling their integration into chipsets with limited resources or their evaluation at very high frequency for demanding applications (e.g., model predictive control, largescale simulation, reinforcement learning, etc.). While most of these algorithms operate on constraint-free settings, only a few have been proposed so far to adequately account for constrained dynamical systems while depicting low algorithmic complexity. In this article 52, we introduce a series of new algorithms with reduced (and lowest) complexity for the forward simulation of constrained dynamical systems. Notably, we revisit the so-called articulated body algorithm (ABA) and the Popov-Vereshchagin algorithm (PV) in the light of proximal-point optimization and introduce two new algorithms, called constrainedABA and proxPV. These two new algorithms depict linear complexities while being robust to singular cases (e.g., redundant constraints, singular constraints, etc.). We establish the connection with existing literature formulations, especially the relaxed formulation at the heart of the MuJoCo and Drake simulators. We also propose an efficient and new algorithm to compute the damped Delassus inverse matrix with the lowest known computational complexity. All these algorithms have been implemented inside the open-source framework Pinocchio and depict, on a wide range of robotic systems ranging from robot manipulators to complex humanoid robots, state-of-the-art performances compared to alternative solutions of the literature.
8.2.24 GJK++: Leveraging Acceleration Methods for Faster Collision Detection
Participants: Louis Montaut, Quentin Le Lidec, Vladimir Petrik, Josef Sivic, Justin Carpentier.
Collision detection is a fundamental problem in various domains, such as robotics, computational physics, and computer graphics. In general, collision detection is tackled as a computational geometry problem, with the so-called Gilbert, Johnson, and Keerthi (GJK) algorithm being the most adopted solution nowadays. While introduced in 1988, GJK remains the most effective solution to compute the distance or the collision between two 3D convex geometries. Over the years, it was shown to be efficient, scalable, and generic, operating on a broad class of convex shapes, ranging from simple primitives (sphere, ellipsoid, box, cone, capsule, etc.) to complex meshes involving thousands of vertices. In this article 14, we introduce several contributions to accelerate collision detection and distance computation between convex geometries by leveraging the fact that these two problems are fundamentally optimization problems. Notably, we establish that the GJK algorithm is a specific sub-case of the well-established Frank-Wolfe (FW) algorithm in convex optimization. By adapting recent works linking Polyak and Nesterov accelerations to Frank-Wolfe methods, we also propose two accelerated extensions of the classic GJK algorithm: the Polyak and Nesterov accelerated GJK algorithms. Through an extensive benchmark over millions of collision pairs involving objects of daily life, we show that these two accelerated GJK extensions significantly reduce the overall computational burden of collision detection, leading to computation times that are up to two times faster. Finally, we hope this work will significantly reduce the computational cost of modern robotic simulators, allowing the speed-up of modern robotic applications that heavily rely on simulation, such as reinforcement learning or trajectory optimization.
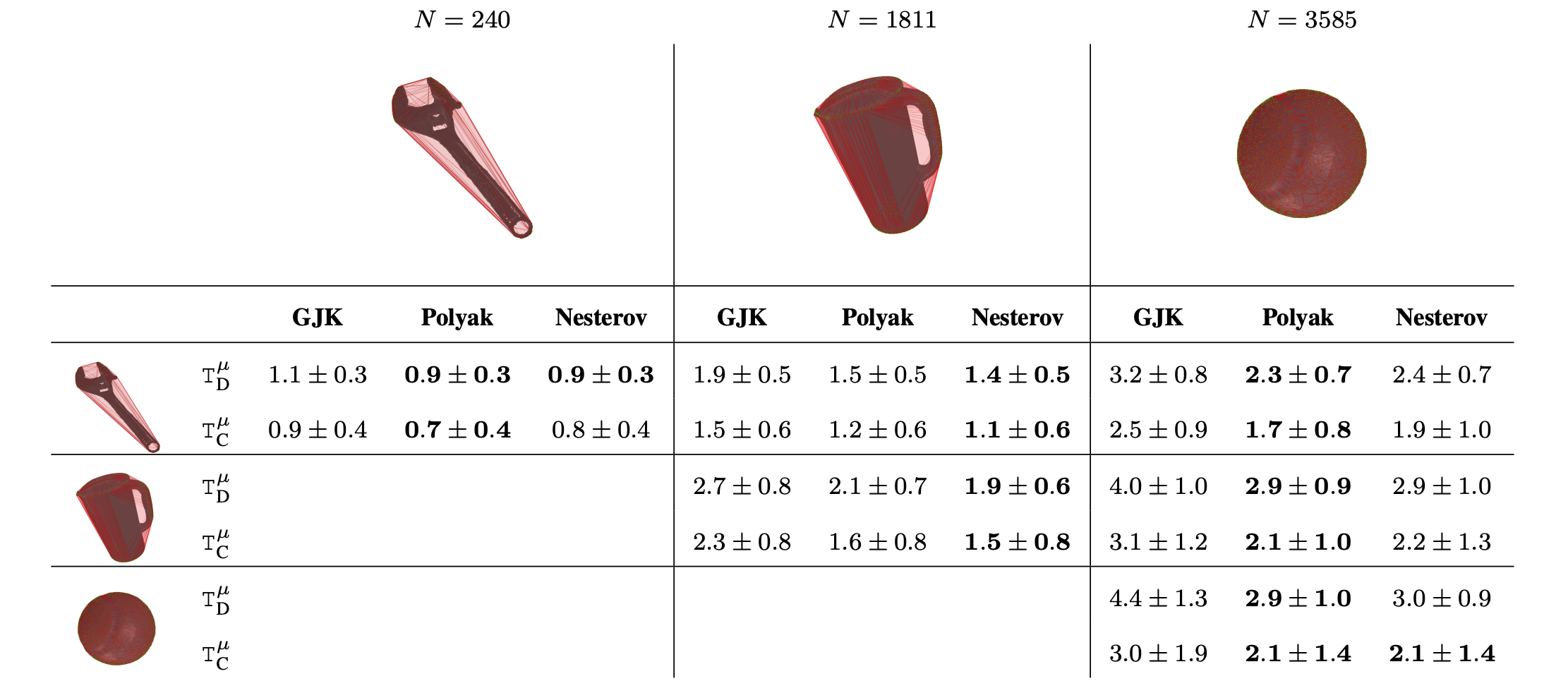
Computation times on collision detection problems of our methods, Polyak and Nesterov accelerated GJK algorithms, and the state-of-the-art GJK algorithm.
8.2.25 Learning System Dynamics from Sensory Input under Optimal Control Principles
Participants: Oumayma Bounou, Jean Ponce, Justin Carpentier.
Identifying the underlying dynamics of actuated physical systems from sensory input is of high interest in control, robotics, and engineering in general. In the context of control problems, existing approaches decouple the construction of the feature space where the dynamics identification process occurs from the target control tasks, potentially leading to a mismatch between feature and state spaces: the systems may not be controllable in feature space, and synthesized controls may not be applicable in state space. Borrowing from the Koopman formalism, we propose instead to learn an embedding of both the states and controls into a feature space where the dynamics are linear, and include the target control task in the learning objective in the form of a differentiable and robust optimal control problem. We validate the proposed approach 17 with simulation experiments using systems with non-linear dynamics, demonstrating that the controls obtained in feature space can be used to drive the corresponding physical systems and that the learned model can serve for future state prediction.
8.2.26 From Compliant to Rigid Contact Simulation: a Unified and Efficient Approach
Participants: Justin Carpentier, Quentin Le Lidec, Louis Montaut.
Whether rigid or compliant, contact interactions are inherent to robot motions, enabling them to move or manipulate things. Contact interactions result from complex physical phenomena, that can be mathematically cast as Nonlinear Complementarity Problems (NCPs) in the context of rigid or compliant point contact interactions. Such a class of complementarity problems is, in general, difficult to solve both from an optimization and numerical perspective. Over the past decades, dedicated and specialized contact solvers, implemented in modern robotics simulators (e.g., Bullet, Drake, MuJoCo, DART, Raisim) have emerged. Yet, most of these solvers tend either to solve a relaxed formulation of the original contact problems (at the price of physical inconsistencies) or to scale poorly with the problem dimension or its numerical conditioning (e.g., a robotic hand manipulating a paper sheet). In this paper 18, we introduce a unified and efficient approach to solving NCPs in the context of contact simulation. It relies on a sound combination of the Alternating Direction Method of Multipliers (ADMM) and proximal algorithms to account for both compliant and rigid contact interfaces in a unified way. To handle illconditioned problems and accelerate the convergence rate, we also propose an efficient update strategy to adapt the ADMM hyperparameters automatically. By leveraging proximal methods, we also propose new algorithmic solutions to efficiently evaluate the inverse dynamics involving rigid and compliant contact interactions, extending the approach developed in MuJoCo. We validate the efficiency and robustness of our contact solver against several alternative contact methods of the literature and benchmark them on various robotics and granular mechanics scenarios. Overall, the proposed approach is shown to be competitive against classic methods for simple contact problems and outperforms existing solutions on more complex scenarios, involving tens of contacts and poor conditioning. Our code is made open-source at https://github.com/Simple-Robotics/Simple.
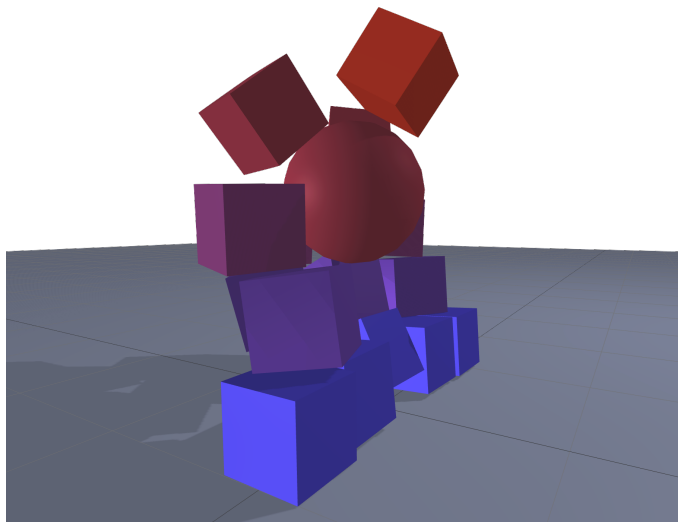
8.2.27 Linear-time Differential Inverse Kinematics: an Augmented Lagrangian Perspective
Participants: Bruce Wingo, Ajay Sathya, Stéphane Caron, Seth Hutchinson, Justin Carpentier.
Differential inverse kinematics is a core robotics problem whose state-of-the-art solutions are currently based on quadratic programming. In this paper 30, we revisit it from the perspective of augmented Lagrangian methods (AL) and the related alternating direction method of multipliers (ADMM). By embracing AL techniques in the spirit of the rigid-body dynamics algorithms proposed by Featherstone, we introduce a method that solves equality-constrained differential IK problems with lineartime complexity. Combined with the ADMM strategy popularized by OSQP, we handle the same class of problems as QP-based differential IK, but scaling linearly with problem dimensions rather than cubically. We implement our approach as C++ opensource software and evaluate it on a benchmark of robotic-arm and humanoid-locomotion tasks. We measure computation times 2–3× shorter than the QP-based state of the art.
8.3 Image restoration and enhancement
8.3.1 MODEL&CO: Exoplanet detection in angular differential imaging by learning across multiple observations
Participants: Théo Bodrito, Olivier Flasseur, Julien Mairal, Jean Ponce, Maud Langlois, Anne-Marie Lagrange.
Direct imaging of exoplanets is particularly challenging due to the high contrast between the planet and the star luminosities, and their small angular separation. In addition to tailored instrumental facilities implementing adaptive optics and coronagraphy, postprocessing methods combining several images recorded in pupil tracking mode are needed to attenuate the nuisances corrupting the signals of interest. Most of these post-processing methods build a model of the nuisances from the target observations themselves, resulting in strongly limited detection sensitivity at short angular separations due to the lack of angular diversity. To address this issue, we propose to build the nuisance model from an archive of multiple observations by leveraging supervised deep learning techniques. The proposed approach 6 casts the detection problem as a reconstruction task and captures the structure of the nuisance from two complementary representations of the data. Unlike methods inspired by reference differential imaging, the proposed model is highly non-linear and does not resort to explicit image-to-image similarity measurements and subtractions. The proposed approach also encompasses statistical modeling of learnable spatial features. The latter is beneficial to improve both the detection sensitivity and the robustness against heterogeneous data. We apply the proposed algorithm to several datasets from the VLT/SPHERE instrument, and demonstrate a superior precision-recall trade-off compared to the PACO algorithm. Interestingly, the gain is especially important when the diversity induced by ADI is the most limited, thus supporting the ability of the proposed approach to learn information across multiple observations.
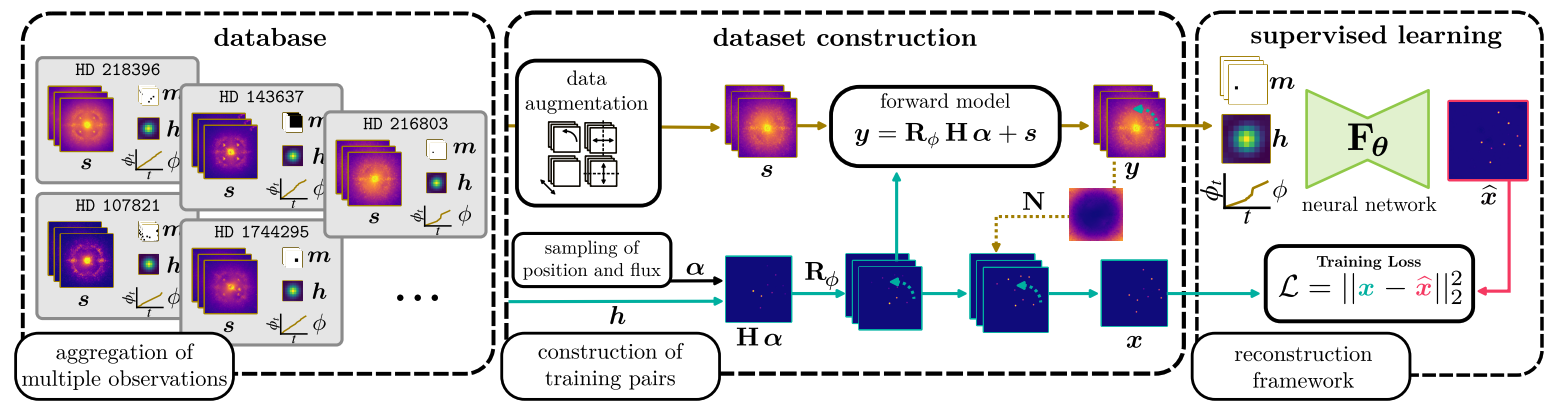
Overview of the proposed MODEL&CO algorithm.
8.3.2 deep PACO: Combining statistical models with deep learning for exoplanet detection and characterization in direct imaging at high contrast
Participants: Olivier Flasseur, Théo Bodrito, Julien Mairal, Jean Ponce, Maud Langlois, Anne-Marie Lagrange.
Direct imaging is an active research topic in astronomy for the detection and the characterization of young sub-stellar objects. The very high contrast between the host star and its companions makes the observations particularly challenging. In this context, post-processing methods combining several images recorded with the pupil tracking mode of telescope are needed. In previous works, we have presented a data-driven algorithm, PACO, capturing locally the spatial correlations of the data with a multi-variate Gaussian model. PACO delivers better detection sensitivity and confidence than the standard post-processing methods of the field. However, there is room for improvement due to the approximate fidelity of the PACO statistical model to the time evolving observations. In this paper 9, we propose to combine the statistical model of PACO with supervised deep learning. The data are first pre-processed with the PACO framework to improve the stationarity and the contrast. A convolutional neural network (CNN) is then trained in a supervised fashion to detect the residual signature of synthetic sources. Finally, the trained network delivers a detection map. The photometry of detected sources is estimated by a second CNN. We apply the proposed approach to several datasets from the VLT/SPHERE instrument. Our results show that its detection stage performs significantly better than baseline methods (cADI, PCA), and leads to a contrast improvement up to half a magnitude compared to PACO. The characterization stage of the proposed method performs on average on par with or better than the comparative algorithms (PCA, PACO) for angular separation above 0.5”.

(a) Typical observations r from the VLT/SPHERE-IRDIS instrument conducted in pupil tracking mode (i.e., with the ADI technique). (b) Illustration of the main operations performed during step 1 of the proposed approach, namely the pre-processing of the observations by statistical learning.
8.4 Doctoral dissertations and habilitation theses
8.4.1 Learning System Dynamics for Control from Sensory Input
Participants: Oumayma Bounou.
Identifying the underlying dynamics model of actuated physical systems from sensory input and using it for future state prediction and control is of high interest in fluid dynamics, robotics, and engineering in general. Borrowing from the Koopman formalism, we first introduce a method to learn a representation of the underlying state of dynamical systems directly from raw measurements. In this embedding space, we force the dynamics model to be linear, in both dynamics and control. These learned representation and linear dynamics model are then used in a control framework. To avoid having a gap between the system embedding dimension (typically higher than the original state dimension) and the control dimension, we propose to also learn an embedding of the controls to a higher dimensional space. This first approach however typically dissociates between embedding space learning and control, potentially making the systems not controllable in the embedding space. To address this, we include a control task in our embedding learning framework in the form of a differentiable optimal control problem, for which we develop a solver leveraging proximal optimization. This proximal formulation allows us to compute accurate gradients even in the case of problems which do not satisfy the standard linear independence constraint qualification (LICQ). We validate our proposed approach 33 with simulation experiments using classical dynamical systems with nonlinear dynamics and data from diverse sensory modalities. We show that the controls obtained in feature space can be used to drive the corresponding physical systems and that the learned model can serve for future state prediction. Finally, we demonstrate the effectiveness of our differentiable solver in dynamics learning and parameters identification experiments in both linear and nonlinear optimal control problems.
8.4.2 Automatic Image Quality Assessment on Portraits
Participants: Nicolas Chahine.
This thesis 34 aims to improve portrait photography by developing novel methodologies and datasets to standardize image quality assessment (IQA) on digital portraits, a subfield referred to as PQA. The work is divided into three parts. The first part addresses the limitations of traditional laboratory setups by creating a standardized approach to consistently evaluate the portrait rendering of digital cameras using custom-built lifelike mannequins and deep learning techniques for automated facial details quality evaluation. The second part expands beyond previous work to cover natural portrait scenes by introducing a comprehensive portrait dataset, PIQ23, with an emphasis on annotation precision, explainability, and diversity. This work is complemented by two deep learning architectures, SEM-HyperIQA and FULL-HyperIQA, which provide semantic-aware image quality predictions. The third part shifts from traditional regression-based IQA methods by focusing on relative quality predictions and emphasizing pairwise comparisons for training and inference. This work introduces PICNIQ, a novel pairwise comparison framework that facilitates precise image quality predictions by leveraging pairwise comparison training. PICNIQ intrinsically counters the problem of domain shift and scale variations in cross-content IQA. Additionally, the research further validates the proposed methods and opens the PQA field to the literature by introducing the NTIRE 2024 Portrait Quality Assessment Challenge, ultimately providing tools and insights for enhancing portrait photography in consumer technology.
8.4.3 Differentiable optimization for robotics: simulation, learning and control
Participants: Quentin Le Lidec.
Optimization is ubiquitous in modern robotics. Whether it is to simulate or to control a robot, numerical optimization algorithms appear to be core building blocks. Learning-based approaches rely on the ability to compute gradients and greatly benefited from the emergence of open-source automatic-differentiation libraries (e.g., PyTorch, JAX, TensorFlow). This thesis 36 aims to pursue this effort and bring some fundamental robotics tools to the differentiable programming paradigm. Starting from physical first principles, we look at robot simulation through the lens of numerical optimization techniques. We consider both the theoretical and implementation aspects to derive efficient algorithms for computing and differentiating the physics of a robotics system. Exploiting the resulting physical models, we highlight the remaining challenges to tackle locomotion and manipulation tasks and propose approaches for learning controllers.
8.4.4 Geometry and Metric Structures of Configuration Spaces for Robotic Motion Planning
Participants: Yann de Mont-Marin.
Geometry has played a central role in developing motion planning methods, from early classical mechanics to modern applications in many fields, such as robotics, computer graphics, and medicine. However, despite significant advances, current algorithms still struggle with complex, unstructured tasks. Leveraging new developments in geometry may provide the missing building blocks needed to enable more intelligent motion planning. Given this context, this doctoral thesis 38 focuses on studying and leveraging the geometry of the configuration space for robotic motion planning. In particular, we identify a relevant distance in the configuration space and develop efficient methods to compute their geodesics. A key contribution of this thesis is the formalization of a distance based on the minimum swept volume. Inspired by solid mechanics, this distance provides a natural metric structure for motion planning in environments cluttered with many obstacles. Minimizing the space occupied during motion reduces the likelihood of collisions. The swept volume distance is studied within the framework of Finsler geometry, and its application to motion planning is demonstrated. Building upon this, we address the challenge of accelerating the computation of geodesics for Finsler distances. We introduce the concept of a geodesic turnpike, where the geodesics of a system exhibit a simple behavior for most of their trajectory, except at the start and end. This phenomenon is analogous to the turnpike property in optimal control theory, where the optimal control is a steady state except for transient phases at the start and end of the time horizon. We formally define geodesic turnpikes and characterize them with vector fields on Finsler manifolds. Using simple algebraic equations, we propose a conjecture to identify geodesic turnpikes for Finsler distance on Lie groups. The identified geodesic turnpikes are leveraged to speed up geodesic computations through a new initialization of the solvers. In addition to addressing distance characterization, we leverage Lie group theory to introduce a new formulation of surface contact dynamics with friction. Specifically, we formulate the quasistatic surface contact with friction problem as a nonlinear complementarity problem on twists and wrenches. Traditional models treat surface contact as a set of discrete points; this new formulation significantly reduces the number of variables needed to model the contact.
8.4.5 Apprentissage auto-supervisé utilisant des architectures à représentations jointes
Participants: Adrien Bardes.
Animals and humans demonstrate advanced intelligence and capabilities such as planning, decision making, memorization, abstract reasoning, and common sense that far exceed those of even the most sophisticated machines. Developmental studies illustrate how human babies rapidly learn about properties of the physical world through visual observation alone, inferring concepts including objects, faces, motion, gravity, and more with minimal examples. This raises a fundamental question: What core mechanisms enable such efficient and general visual learning and how can we recreate similar processes in artificial systems ? The overarching objective of this thesis 32 is to take a step toward answering this question by exploring the problem of learning visual representation without supervision, drawing inspiration from natural intelligence. We specifically explore practical implementations of the recently proposed Joint Embedding Predictive Architecture (JEPA) for self-supervised visual learning. Across four chapters detailing novel technical contributions, we introduce new algorithms targeting self-supervised learning across both images and videos through prediction in a shared embedding space. Chapter 3 presents VICReg, a non-contrastive JEPA that explicitly prevents representation collapse, demonstrating superior performance over concurrent self-supervised methods with fewer architectural complexities. Chapter 4 extends this approach to further incorporate local feature learning, improving segmentation capabilities while maintaining strong classification accuracy. Chapter 5 explores joint motion and content learning via a multi-task framework combining optical flow estimation with representation learning, benefiting both image and video segmentation. Finally, Chapter 6 presents V-JEPA that learns spatio-temporal representations by training a masked JEPA on a large corpus of narrated web videos, setting new state-of-the-art on multiple temporal understanding benchmarks while closing the gap with image-focused models on static tasks. Together, these technical contributions represent valuable progress towards robust and general visual learning without human input, while highlighting ample opportunities for future work furthering self-supervision across modalities, model architectures, pretext tasks, mid-level inductive biases, downstream applications, and more. The techniques proposed constitute preliminary steps on the path towards artificial systems that actively acquire visual knowledge about the world much like animals and humans.
8.4.6 Real-time constrained trajectory optimisation in robotics: theory, implementation, and applications
Participants: Wilson Jallet.
Robotics has been a momentous field of research and development over the past sixty years, since the computer chip revolution in the 1960s. Realising the promise of the field entails research into motion planning and control of robotic systems in many scenarios: from fixedbase robots (e.g. industrial arms) to floating-base wheeled or legged robots. For such schemes to be able to deal with more complex robots, they must plan ahead and satisfy physical and operational constraints, and do so, hopefully, in a reactive manner. For many years, they were rather simple, limited to simple robots doing simple things. Greater complexity and capability was unlocked with more sophisticated planners and controllers, that had access to better models of their subservient systems (geometries, inertia, the existence of contact). An adequate framework to reconcile the domain's requirements is that of optimal control. It allows for predictive models of robot behaviour over given time horizons! , and constraint satisfaction, while optimising for a given performance metric. As most optimal control problems cannot be solved in closed-form, we resort to numerical methods. This numerical optimal control has a proven track record for online motion generation and control on legged robots with real-time requirements (albeit mostly while using simplified models of the robot and environment). However, it typically leads to large-scale mathematical optimisation problems with thousands of variables – a computationally expensive endeavour. Thus, its use in robotics has relied on two axes of progress: faster chips, and efficient, structure-exploiting algorithms (with carefully engineered implementations). This thesis 35 focuses on the latter axis: development of more performant, real-time capable solvers for numerical optimal control, with the objective of "on-the-fly" complex motion generation the for predictive control of sophisticated robots.
8.4.7 Algorithmic and Computational Foundations of Differentiable Collision Detection
Participants: Montaut Louis.
This thesis 39 explores advancements in collision detection, a fundamental problem in computer science that aims at determining whether and where objects in a virtual environment overlap. Collision detection lies at the heart of every physics simulators, which are used in a wide range of engineering domains, such as video games, computer animation, virtual reality, computer-assisted design, and robotics. Recent research has notably focused on developing differentiable physics simulators to allow gradient-based optimization methods to exploit and control the dynamics of simulated systems. The core objectives of this thesis are (i) to enhance the efficiency of state-of-the-art collision detection algorithms, (ii) to develop new techniques to compute the derivatives of collision features, and (iii) to integrate these advancements into differentiable physics simulators. This thesis begins by revisiting the widely used Gilbert-Johnson-Keerthi (GJK) collision detection algorithm under the prism of the Frank-Wolfe method, one of the oldest gradient-based optimization techniques. This new perspective allows for the introduction of accelerated variants of GJK, leveraging techniques like Polyak and Nesterov gradient methods. These accelerations significantly reduce the number of iterations needed for collision detection, especially in complex scenarios involving curved surfaces or highly detailed meshes, improving performance by up to two times in terms of computational speed compared to the vanilla GJK algorithm. Then, we develop efficient algorithms to compute the derivatives of collision features used in physics simulators, such as contact points and normals. This thesis presents both zero-order and first-order estimators for these derivatives, with the first-order method offering a highly efficient and accurate solution. This method is further enhanced by a randomized smoothing approach to address challenges posed by non-strictly convex shapes like meshes, often resulting in ill-defined or uninformative gradients. Finally, we integrate the proposed differentiable collision detection pipeline into a custom-built differentiable physics simulator named "Simple". The simulator achieves state-of-the-art computational performance in both forward and backward passes, providing a significant speed-up compared to alternative solutions. This thesis bridges the gap between efficient collision detection and differentiable simulation, laying the groundwork for more robust and real-time applications in robotics and other engineering fields.
8.4.8 Primal-dual proximal augmented lagrangian methods for quadratic programming : theory and implementation
Participants: Antoine Bambade.
Modern applications of control problems are computationally challenging and require fast and robust numerical calculations. Quadratic programming plays for these domains a pivotal role since it is used in numerous practical applications. Recent advances of differentiable optimization have also illustrated that Quadratic Programming layers offer a rich modeling power and can be effective for solving certain machine learning tasks. In this thesis 31, we have introduced a new algorithm, PROXQP, for solving generic QPs. Among others, we notably propose to combine the bound constraint Lagrangian (BCL) globalization strategy for automatically scheduling key parameters of a primal-dual proximal augmented Lagrangian algorithm. Additionally, we show that the PROXQP algorithm features a global convergence guarantee, as well as a few other advantageous numerical properties. Furthermore, we highlight that the PROXQP algorithm actually solves the closest primal-feasible QP if the original QP appears to be primally infeasible. These convergence guarantees come together with an efficient software implementation of PROXQP in the open-source PROXSUITE library. The performance of PROXQP is evaluated on different robotic and control experiments, including a real-world closed-loop controller application. In particular, we have highlighted how solving the closest feasible QPs can be efficiently leveraged in the context of closed-loop convex MPC. Finally, through various benchmarks of the optimization literature, we have also shown that PROXQP performs at the level of state-of-the-art solvers on a large set of generic QP problems. The study of the closest feasible QPs has then motivated us to enrich the expressive capabilities of existing QP layers. In particular, we propose an extended conservative Jacobian formulation for differentiating convex QPs, covering both feasible and infeasible problems. For feasible problems, and when the solution is differentiable, this reduces to standard Jacobians. We then show how driving towards feasibility at test time a new scope of QP layers using our techniques. We illustrate that training these new types of QP layers leads to better predicting power for solving some tasks. We further provide an open-source C++ framework, referred to as QPLAYER, which efficiently implements the approach.
8.4.9 Pixel-Level Tracking and Future Prediction in Video Streams
Participants: Guillaume Le Moing.
Visual cues play a significant role for people in foreseeing (plausible) future events, a fundamental skill that aids in social interactions, object manipulation, navigation, and averting potential risks in their daily lives. Transferring this ability to machines equipped with video cameras holds immense promise, particularly in domains such as autonomous vehicles, human-robot interaction, data compression, and the comprehension of natural and social phenomena. The objective of this thesis 37 is to develop novel strategies for low-level motion estimation, with the ultimate goal to improve the efficiency of future prediction systems that synthesize plausible continuations of videos. We aim to accurately describe motion of densely sampled points between pairs of arbitrary frames in a video sequence, overcoming challenges such as low-texture regions, appearance changes due to motion blur or shadow effects, as well as occlusion events caused by object and camera movements. Leveraging the compositional nature of scenes, we aim to propose sparse motion models that may simplify video synthesis by transitioning from the high-bit-rate prediction of future frames to the low-bit-rate prediction of future motion. We ensure temporal consistency and the recovery of fine-grained details from context using warping operations derived from estimated motions. Recognizing the inherent uncertainty in predicting the future, our focus lies in modeling the distribution of possible trajectories. This allows for the sampling of different outcomes or the selection of one trajectory that aligns with a given control signal, be it a target end frame, a textual description or an audio track. Our contributions are organized into three main parts. First, we introduce a strategy using an autoencoder enhanced with a learnable optical flow module to compress video frames into compact representations, enabling efficient frame recovery. By sharing information across time steps, we bypass the autoencoder's lossy bottleneck. Through autoregressive modeling, these compact representations are used to predict future frames from past ones, guided by control signals from various modalities. Second, we propose an object layer decomposition scheme, where a small set of control points is used to estimate past deformations and predict future ones. We recover dense motion with spline interpolations and synthesize future frames using warping operations. Third, we present a novel, simple, and efficient method for dense pixel-level video tracking. This method relies on a learnable optical flow estimator to refine coarse motion estimates between pairs of time steps, derived from a small set of tracks computed with an off-the-shelf model on the entire video.
9 Bilateral contracts and grants with industry
9.1 Bilateral contracts with industry
9.1.1 Louis Vuitton/ENS chair on artificial intelligence
Participants: Jean Ponce.
The scientific chair Louis Vuitton - École normale supérieure in Artificial Intelligence has been created in 2017 and inaugurated on April 12, 2018 by the ENS Director Marc Mézard and the LV CEO Michael Burke. The goal of the chair is to establish a close collaboration between LV and ENS in the area of Artificial Intelligence. The chair enjoys the generous annual contribution of 200K Euros provided by LV in support of research activities in statistical learning and computer vision. In particular, the chair supports the costs of researchers, students, missions, computational resources as well as seminars and meetings, including the two days of meeting annually organized by LV and ENS. During 2020 ENS and LV have organized several joint meetings with the participation of researchers from SIERRA and WILLOW teams. The chair has also supported the hiring of one PhD student at the WILLOW team, missions to conferences and international research labs as well as data collection for research projects. In 2020 the chair has been extended to the next three-year period until 2023. We are planning to start a CIFRE PhD of François Gardères together with Louis Vuitton in 2023.
9.1.2 Casino/ENS chair on algorithmic and machine learning
Participants: Justin Carpentier.
The scientific chair Casino/ENS - École normale supérieure on algorithmic and machine learning has been created in 2021. J. Carpentier is in charge of the robotics axis of this chair.
10 Partnerships and cooperations
10.1 International research visitors
10.1.1 Visits of international scientists
Other international visits to the team
Bruce Wingo
-
Status
PhD Student
-
Institution of origin:
Georgia Tech
-
Country:
US
-
Dates:
full year
-
Context of the visit:
Collaboration on developping low-complexity methods and solvers for control in robotics
-
Mobility program/type of mobility:
research stay
Lander Van Roy
-
Status
PhD Student
-
Institution of origin:
KU Leuven
-
Country:
Belgium
-
Dates:
3/2024 - 7/2024 | 10/2024
-
Context of the visit:
Collaboration on developping frictional contact solvers and methods for robot simulation
-
Mobility program/type of mobility:
research stay
10.2 European initiatives
10.2.1 Horizon Europe
AGIMUS
AGIMUS project on cordis.europa.eu
-
Title:
Next generation of AI-powered robotics for agile production
-
Duration:
From October 1, 2022 to September 30, 2026
-
Partners:
- INSTITUT NATIONAL DE RECHERCHE EN INFORMATIQUE ET AUTOMATIQUE (INRIA), France
- AIRBUS, France
- KLEEMANN HELLAS SA (KLEEMANN HELLAS SA), Greece
- PAL ROBOTICS SLU (PAL ROBOTICS), Spain
- Q-PLAN INTERNATIONAL ADVISORS PC (Q-PLAN INTERNATIONAL), Greece
- PAL FRANCE, France
- THIMM OBALY, K.S., Czechia
- CESKE VYSOKE UCENI TECHNICKE V PRAZE (CVUT), Czechia
- CENTRE NATIONAL DE LA RECHERCHE SCIENTIFIQUE CNRS (CNRS), France
-
Inria contact:
Justin Carpentier
- Coordinator:
-
Summary:
AGIMUS aims to deliver an open-source breakthrough innovation in AI-powered agile production, introducing solutions that push the limits of perception, planning, and control in robotics, enabling general-purpose robots to be quick to set-up, autonomous and to easily adapt to changes in the manufacturing process. To achieve such agile production, AGIMUS leverages on cutting-edge technologies and goes beyond the state-of-the-art to equip current mobile manipulators with a combination of (i) an advanced task and motion planner that can learn from online available video demonstrations; (ii) optimal control policies obtained from advances in reinforcement learning based on efficient differentiable physics simulations of the manufacturing process; as well as (iii) advanced perception algorithms able to handle objects and situations unseen during initial training. Along the way, optimization of energy efficiency and the use of 5G technology will support further pushing the limits of autonomy. The AGIMUS solutions and their impact will be demonstrated and thoroughly stress-tested in 3 testing zones, as well as 3 industrial pilots in Europe, under numerous diverse real-world case studies and scenarios (different tools, environments, processes, etc.). In every step, and from the very beginning, AGIMUS will go beyond current norms and involve a wide range of stakeholders, starting from the production line itself, to identify the essential ethical-by-design principles and guidelines that can maximise acceptance and impact.
10.3 National initiatives
10.3.1 PRAIRIE
Participants: Justin Carpentier, Jean Ponce, Cordelia Schmid.
The Prairie Institute (PaRis AI Research InstitutE) is one of the four French Institutes for Interdisciplinary Artificial Intelligence Research (3IA), which were created as part of the national French initiative on AI announced by President Emmanuel Macron on May 29, 2018. It brings together five academic partners (CNRS, Inria, Institut Pasteur, PSL University, and University of Paris) as well as 17 industrial partners, large corporations which are major players in AI at the French, European and international levels, as well as 45 Chair holders, including three of the members of WILLOW (Carpentier, Ponce, Schmid). Ponce is the scientific director of PRAIRIE.
10.3.2 VideoPredict: Predicting future video content
Participants: Cordelia Schmid, Jean Ponce.
Predicting future video content is a challenging problem with high potential impact in downstream tasks such as self-driving cars and robotics, but also much promise for the learning process itself, from self-supervised learning to data augmentation. Existing approaches range from predicting future actions with semantic labels to creating realistic renderings of future frames. Most of them use straight predictions from convolutional features of previous frames. We propose instead to model the causality effects involved in the video formation process, and disentangle motion and appearance factors. This will result in better prediction, but also and maybe more importantly in a better, more structured understanding of the video content, leading to explicable and interpretable results, and eventually to more trustworthy learning systems. The German and French partners are, respectively, experts in machine learning and computer vision, with complementary research threads in causality and disentangled data models on the one hand, and video understanding and action recognition on the other hand, that are ideally suited for this collaborative project
10.3.3 PEPR Organic Robotics
Participants: Justin Carpentier, Stephane Caron, Megane Millan, Umit Bora Gokbakan, Etienne Menager.
The PEPR O2R "Organic Robotics" aims to initiate a change in robotics to create a new generation of robots capable of fluid and natural interactions with users, of social adaptation in their interactions, and which accompanies the technological transitions of societies by producing adapted, responsive and reliable services to citizens. In the frame of this national program, WILLOW is involved in Structuring Action 2 (Robot motion with physical interactions and social adaptation) led by Philippe Souères at LAAS-CNRS, and Structuring Action 4 (Modelling, Simulation, Multi-scale, and Biomechanics) led by Jérémie Dequidt at Inria DEFROST. J. Carpentier is also a member of the executive committee of the PEPR.
10.3.4 ARTIFACT (ANR Tremplin): La fabrique du mouvement artificiel
Participants: Justin Carpentier, Megane Millan, Franki Nguimatsia Tiofack.
Les robots modernes restent confinés dans des environnements étroitement contrôlés et même les chorégraphies complexes que les humanoïdes de Boston Dynamics exécutent sans faille, dépendent fortement de la capture des mouvements, de stratégies de contrôle élaborées à la main et de modèles détaillés de l'espace de travail, qui laissent peu de place à la perception. En clair, les robots sont loin d'atteindre le niveau d'agilité et de dextérité, et encore moins l'autonomie, la robustesse et la sécurité nécessaires à leur déploiement "dans la nature" aux côtés de l'homme. Un bond en avant dans ces capacités est nécessaire pour qu'ils tiennent leurs promesses et sortent véritablement du laboratoire. Notre principe est que la clé de cette révolution est le développement des fondements théoriques et algorithmiques d'une véritable intelligence artificielle du mouvement, une IA qui doit relever le défi supplémentaire d'interagir physiquement avec des environnements en évolution dynamique et, en fin de compte, avec des personnes. Nous romprons avec la dichotomie entre le contrôle optimal, où le rôle de la perception est traditionnellement limité à une étape précoce d'estimation de l'état, et l'apprentissage par renforcement, où les politiques de contrôle sont généralement apprises sans modèle, sans garantie de faire face à la malédiction de la dimensionnalité. Concrètement, nous utiliserons le formalisme de Koopman des systèmes dynamiques complexes pour apprendre les modèles sensorimoteurs et les stratégies de contrôle correspondantes à partir des données des capteurs. Nous développerons des méthodes puissantes pour apprendre, contrôler et partager un dictionnaire de synergies sensorimotrices à travers les tâches, faisant écho à celles utilisées par le système nerveux central humain dans les tâches quotidiennes et accélérant l'acquisition de nouvelles compétences. Nous tirerons parti de la composition des stratégies sensorimotrices et des stratégies de recherche arborescente alimentées par des réseaux neuronaux pour planifier de manière optimale les mouvements du robot sous des contraintes d'observation dynamiques. Le cadre proposé sera mis en œuvre dans de nouvelles architectures logicielles de programmation différentiable et démontré sur plusieurs tâches de locomotion et de manipulation, à la fois en simulation et sur des robots réels.
10.3.5 NIMBLE (ANR JCJC): Inexact optimization for robot control
Participants: Justin Carpentier, Stephane Caron, Etienne Arlaud, Jean Ponce, Oumayma Bounou, Joris Vaillant, Wilson Jallet, Frederike Dumbgen.
The limited agility and dexterity of modern robots prevent them from being deployed outside of laboratories, not even mentioning outside of factories. With NIMBLE, we want to point the classical sense-plan-act design pattern, widely adopted in robotics, as one of the main limiting factor. We propose to replace this three-part control paradigm by learning, from real robot experiments, a predictive model of the robot sensorimotor capabilities. This sensorimotor model will be notably exploited to take complex decisions generalizing to unforeseen situations directly from sensor measurements. While NIMBLE’s innovation takes its roots in the observation of the human motor control organization, it is grounded by advanced and principled mathematical methodologies, in particular, the Koopman operator sitting on top of (deep) learning, and exploits our recognized expertise in robot modeling, optimal control and machine learning for real robots. It will notably enable complex tasks to be defined and executed directly in the sensor space. The success of NIMBLE will be asserted by clear benchmarks in quadrupedal locomotion able to optimally adapt to unstructured terrains and in mobile manipulation for opening unknown doors using the sound combination of force and visual feedback.
10.3.6 INEXACTE (ANR PRCE): Inexact optimization for robot control
Participants: Justin Carpentier, Stephane Caron, Etienne Arlaud, Antoine Bambade, Joris Vaillant, Wilson Jallet.
Robotic systems are expected to take a large place in tomorrow’s society, far beyond current industrial robots in tightly controlled factory environments, with large impacts in terms of safety, health at work, comfort and productivity. The motion of robots is typically designed and controlled by specifying numerical objectives and constraints on what they must do, and within which limits. These specifications often conflict, and the actual control must be computed to satisfy all of them in the best possible way. This is naturally achieved by solving a numerical optimization problem. Such problems are often small enough in robotics that they can be solved exactly in theory, but they are always based on models, and by definition, models reflect reality imperfectly, even more so as we get away from tightly controlled (factory) environments.
We propose a complete change of paradigm, to acknowledge that we actually solve inaccurate optimization problems that provide inaccurate solutions by construction, and explore the following two hypotheses: (H1) We can obtain the exact same performance with imprecise numerical solutions, (H2) we can obtain these imprecise numerical solutions using less costly numerical methods, which can be computed faster, using less demanding hardware. To the best of our knowledge, these questions have barely been explored and INEXACT will provide the first comprehensive exploration of this topic.
Our short-term ambition is to significantly lower the computational requirements for solving control problems, taking advantage of the imprecisions inherent to robotics control to compute appropriate solutions faster. But ultimately, our long-term ambition is to design less fragile, less expensive and less polluting robots, since being less dependent on precise models can make us less dependent on precise and therefore complex, fragile, expensive and resource-demanding mechatronics.
10.4 Regional initiatives
10.4.1 DIM AI4IDF
Participants: Justin Carpentier, Stephane Caron, Jean Ponce, Etienne Arlaud, Julie Perrin.
AI4IDF is a structuring and federating research project devoted to artificial intelligence, a scientific and technological field that has become unavoidable.
AI4IDF aims to deepen knowledge in AI while keeping the human being at the center of its concerns. The Paris Region must play a major role in this future sector, thanks to its scientific excellence and its incomparable ecosystem.
11 Dissemination
Participants: Ajay Sathya, Axel Nguyen–Kerbel, Basile Terver, Cordelia Schmid, Elliot Vincent, Etienne Arlaud, Etienne Menager, Etienne Moullet, Ewen Dantec, Fabian Schramm, Francois Garderes, Franki Nguimatsia Tiofack, Frederike Dumbgen, Gabriel Fiastre, Guillaume Le Moing, Imen Mahdi, Jean Ponce, Joris Vaillant, Julie Perrin, Justin Carpentier, Lander Vanroye, Louis Montaut, Louise Manson, Lucas Haubert, Lucas Ventura, Ludovic De Matteis, Marguerite Petit–Talamon, Matthieu Futeral-Peter, Megane Millan, Nicolas Chahine, Oumayma Bounou, Paul Pacaud, Pierre-Guillaume Raverdy, Qikai Huang, Quentin Le Lidec, Ricardo Garcia Pinel, Roland Andrews, Romain Seailles, Sara Pieri, Shiyao Li, Shizhe Chen, Stephane Caron, Theo Bodrito, Theotime Le Hellard, Thomas Chabal, Umit Bora Gokbakan, Valentin Tordjman–Levavasseur, Wilson Jallet, Yann Dubois De Mont-Marin, Zeeshan Khan.
11.1 Promoting scientific activities
11.1.1 Scientific events: organisation
Member of the organising committees
- Ellis Workshop for Computer Vision and Machine Learning, Modena, April 2024 (C. Schmid)
- Outreach chair in the organizing committee of the 2024 IEEE-RAS International Conference on Humanoid Robots (S. Caron)
- Workshop co-organizer (Differentiable Optimization at All Scales: Simulation, Estimation, Learning, and Control) at Conference on Robot Learning (CoRL), October 2024, Munich (J. Carpentier, L. Montaut, F. Dümbgen, Q. Le Lidec)
- Workshop co-organizer (Frontiers for Optimization in Robotics) at Robotics:Science and Systems (R:SS), July 2024, Delft (J.C. Carpentier, A. Sathya, F. Dümbgen)
11.1.2 Scientific events: selection
Chair of conference program committees
- The IEEE / CVF Computer Vision and Pattern Recognition Conference (S. Chen, C. Schmid)
- The European Conference on Computer Vision (S. Chen, C. Schmid)
- The Conference and Workshop on Neural Information Processing Systems (S. Chen)
- The International Conference on Learning Representations (S. Chen, C. Schmid)
- Asian Conference on Computer Vision (S. Chen)
- Neurips (C. Schmid)
Member of the conference program committees
- Associate Editor for the IEEE-RAS International Conference on Humanoid Robotics 2024 (A. Sathya)
Reviewer
- ACM Multimedia (Z. Chen, Z. Khan)
- Asian Conference on Computer Vision (Z. Chen, S. Pieri)
- Conference on Computer Vision and Pattern Recognition (Z. Chen, Z. Khan, S. Pieri)
- European Conference on Computer Vision (Z. Chen, Z. Khan)
- IEEE/RSJ International Conference on Intelligent Robots and System (S. Caron, T. Chabal, F. Dümbgen, A. Sathya)
- IEEE-RAS International Conference on Humanoid Robots (S. Caron, F. Dümbgen)
- IEEE-RAS International Conference on Robotics and Automation (S. Caron, T. Chabal, Z. Chen, E. Dantec, F. Dümbgen, Ü. B. Gökbakan, Q. Le Lidec, A. Sathya, S. Chen)
- IEEE International Conference on Soft Robotics (E. Menager)
- International Conference on Machine Learning (Q. Le Lidec)
- Learning for Dynamics & Control Conference (Q. Le Lidec)
- Robotics: Science and Systems (F. Dümbgen, Q. Le Lidec, A. Sathya)
- International Conference on Computational Linguistics (S. Chen)
- Conference on Language Modeling (S. Chen)
11.1.3 Journal
Member of the editorial boards
- Associate Editor, IEEE Transactions on Robotics (J. Carpentier)
- Associate Editor, IEEE Robotics and Automation Letters (J. Carpentier)
- Associate Editor, IEEE Transactions on Robotics (S. Caron)
Reviewer - reviewing activities
- Transactions on Pattern Analysis and Machine Intelligence (S. Chen)
- International Journal of Computer Vision (Z. Khan, S. Chen)
- IEEE Robotics and Automation Letters (S. Caron, T. Chabal, E. Dantec, Y. de Montmarin, Q. Le Lidec, E. Menager, S. Chen)
- IEEE Transactions on Robotics (J. Carpentier, E. Dantec, Y. de Montmarin, F. Dümbgen, Q. Le Lidec, A. Sathya, L. Montaut)
- IEEE Transactions on Intelligent Transport Systems (A. Sathya)
- Book chapter review for Springer LNCIS volume: "Nonlinear and Constrained Control." ISBN.Hardcover: 978-3-031-82680-1 E-book: 978-3-031-82681-8.
11.1.4 Invited talks
- J. Carpentier, Keynote at Humanoids'24, Nov. 2024.
- J. Carpentier, Workshop on Biomechanics at Humanoids'24, Nov. 2024.
- J. Carpentier, Journées Nationales de la Robotique Humanoïde, Nov. 2024.
- J. Carpentier, GT Control, LJLL, May 2024.
- J. Carpentier, Rencontres INRIA-CETIM, May 2024.
- J. Carpentier, Université d'Avignon, March 2024.
- J. Carpentier, Camin Seminar, March 2024.
- S. Chen, Imaging at Paris Seminar, June 2024.
- S. Caron, School of Engineering and Design, Technical University of Munich, April 2024.
- E. Dantec, Journées nationales de la robotique humanoïde, November 2024.
- F. Dümbgen, UniBW, Munich, Germany, October 2024.
- F. Dümbgen, Workshop for Real-world Applications of Geometry and Algebra, Eindhoven, Netherlands, June 2024.
- F. Dümbgen, Karlsruhe Institute of Technology, Germany, June 2024.
- F. Dümbgen, University of Berne, Switzerland, June 2024
- A. Sathya: “Low-order algorithms for efficient robot simulation and control” at the R4 Working Group meeting, a work of robotics research groups in the Nouvelle-Aquitaine province of France on November 18, 2024.
- A. Sathya: “Efficient Constrained Robot Dynamics Simulation Algorithms” at Neuromuscular Biomechanics Lab, Stanford University, USA on September 4, 2024.
- A. Sathya: “Efficient Constrained Robot Dynamics Simulation Algorithms” at Hybrid Robotics Lab, University of California Berkeley, USA on August 30, 2024.
- A. Sathya: “Efficient algorithms and implementation for differentiable robot simulation and control”, CNRS-AIST JRL, National Institute of Advanced Industrial Science and Technology, Tsukuba, Japan on May 21, 2024.
- C. Schmid, Keynote speaker at Conference on Robot Learning (CORL), Munich, September 2024.
- C. Schmid, Invited speaker at Instance-Level Recognition Workshop, in conjunction with ECCV'24, October 2024.
- C. Schmid, Invited speaker at Tutorial on Video Content Understanding and Generation, in conjunction with ECCV'24, October 2024.
- C. Schmid, Keynote speaker at German Pattern Recognition Conference, Munich, September 2024.
- C. Schmid, Invited speaker at MMFM2: The 2nd Workshop on What is Next in Multimodal Foundation Models?, in conjunction with CVPR'24, June 2024.
- C. Schmid, Invited speaker at LPVL: Learning from Procedural Videos and Language: What is Next?, in conjunction with CVPR'24, June 2024.
- C. Schmid, Invited speaker at New Frontiers for Zero-Shot Image Captioning Evaluation (NICE) Workshop, in conjunction with CVPR'24, June 2024.
- C. Schmid, Invited speaker at Tool-Augmented VIsion (TAVI) Workshop, in conjunction with CVPR'24, June 2024.
- C. Schmid, Invited speaker at the Ellis computer vision and machine learning workshop, April 2024.
- C. Schmid, Invited speaker at the Monaco Women Forum, March 2024.
- C. Schmid, Invited speaker at the WELT-Wirtschaftsgipfel, Berlin, January 2024.
- C. Schmid, Presentation at Computer Vision Day in conjunction with Gul Varol's HdR, November 2024.
- C. Schmid, Presentation at Helmholtz Centrum München, Munich, July 2024.
- C. Schmid, Presentation at TUM, Garching, Munich, July 2024.
- C. Schmid, Presentation at Prairie scientific workshop, Paris, April 2024.
- C. Schmid, ONE MUNICH Distinguished Lecture on AI & Robotics, Munich, January 2024.
11.1.5 Leadership within the scientific community
- Co-chair, Technical Committee of Model-based Optimization (F. Dümbgen).
- Participation in “Speed Meeting”' at Rendez-vous des Jeunes Math´ematiciennes et Informaticiennes (F. Dümbgen).
11.2 Teaching - Supervision - Juries
11.2.1 Teaching
- Master: Convex Optimization, M2, École normale supérieure, and MVA, École normale supérieure Paris-Saclay (Q. Le Lidec, Teaching Assistant).
- Master: Introduction à la vision artificielle, M1, École normale supérieure Paris (J. Ponce, G. Fiastre as Teaching Assistant).
- Master: Introduction to computer vision, NYU (J. Ponce).
- Master: Object recognition and computer vision, M2, École normale supérieure, and MVA, École normale supérieure Paris-Saclay, 36h (J. Ponce and C. Schmid, with G. Le Moing as Teaching Assistant).
- Master: Robotics courses at ENS-DI, 30h (J. Carpentier, S. Caron and Y. de Montmarin as Teaching Assistant).
- Master: Robotics courses at ENS-MVA, 30h (J. Carpentier, S. Caron).
- Master: Robotics courses at Formation des Ingénieurs de l'Automobile, PSL, 15h (J. Carpentier, S. Caron).
- Master: Computer Vision courses at Formation des Ingénieurs de l'Automobile, PSL, 18h (J. Ponce).
- Introduction to computer vision course at the PSL Intensive week, 3H, (A. Yang as Teaching Assistant).
- Master: Deep learning, MVA (S. Chen as guest lecturer)
11.2.2 Supervision
PhD defenses
- Antoine Bambade, advised by J. Carpentier, A. Taylor (Sierra) and J. Ponce.
- Adrien Bardes, advised by J. Ponce.
- Nicolas Chahine, advised by J. Ponce.
- Oumayma Bounou, advised by J. Ponce and J. Carpentier.
- Yann Dubois de Mont-Marin, advised by J. Ponce.
- Wilson Jallet, started in Oct 2021, advised by J. Carpentier.
- Quentin Le Lidec, advised by J. Carpentier and C. Schmid.
- Guillaume Le Moing, advised by J. Ponce and C. Schmid.
- Louis Montaut, advised by J. Carpentier.
PhD students
- Roland Andrews, started in Oct 2024, advised by J. Carpentier and A. Taylor (Sierra).
- Imen Mahdi (University of Freiburg), started in Oct 2024, advised by C. Schmid and Abhinav Valada (University of Freiburg).
- Romain Seailles, started in Sept 2024, advised by J. Ponce and J. Mairal (Inria Grenoble).
- Theo Bodrito, started in Sep 2021, advised by J. Ponce and J. Mairal (Inria Grenoble).
- Basile Terver (Meta), started in Nov 2024, advised by J. Ponce and Y. LeCun (Meta).
- Shiyao Li (ENPC), started in Oct 2024, advided by S. Chen and V. Lepetit (ENPC).
- Thomas Chabal, started in Sept 2021, advised by J. Ponce and C. Schmid.
- Zerui Chen, started in March 2022, advised by C. Schmid and S. Chen.
- Matthieu Futeral-Peter, started in Nov 2021, advised by C. Schmid.
- Ricardo Garcia Pinel, started in Sep 2021, advised by C. Schmid and S. Chen.
- Lucas Ventura, started in Oct 2022, advised by C. Schmid and G. Varol (ENPC).
- Elliot Vincent, started in Sep 2021, advised by J. Ponce and M. Aubry (ENPC).
- Fabian Schramm, started in Feb 2023, advised by J. Carpentier and N. Perrin-Gilbert (ISIR).
- Zeeshan Khan, started in Sept 2023, advised by S. Chen and C. Schmid.
- Gabriel Fiastre, started in Sept 2023, advised by C. Schmid.
- Ludovic de Matteis, started in Oct 2023, advised by J. Carpentier and N. Mansard (CNRS).
- U. Bora Gökbakan, started in June 2024, advised by S. Caron and P. Souères (CNRS).
- S. Pieri, started in Oct 2024, advised by S. Chen and C. Schmid.
- P. Pacaud, started in Oct 2024, advised by S. Chen and C. Schmid.
- F. Gardères, started in May 2023, advised by S. Chen and J. Ponce.
11.2.3 Juries
- Examiner in the CSI committee of M. Van Waerebeke (S. Caron)
- Examiner in the CSI committee of Alexandre Schortgen (J. Carpentier)
- Examiner in the CSI committee of Said Alexander Alvarado Marin (J. Carpentier)
- Anh-Quan CAO, December 2024, PSL Université Paris (C. Schmid)
- Guillaume Jeanneret Sanmiguel, September 2024, University of Caen (C. Schmid)
- Ameya Prabhu, September 2024, Oxford University (C. Schmid)
- Taein Kwon, July 2024, ETH Zürich (C. Schmid)
- Gautier Izacard, May 2024, PSL Université Paris (C. Schmid)
- Hyung Ju Terry Suh, October 2024, MIT (J. Carpentier)
- Armand Jordana, December 2024, NYU (J. Carpentier)
11.3 Popularization
11.3.1 Specific official responsibilities in science outreach structures
Jean Ponce, together with Isabelle Ryl, have a monthly "carte blanche" within Le Monde around AI topics.
11.3.2 Participation in Live events
- Pilot participant to the Cybathlon 2024 (E. Moullet)
12 Scientific production
12.1 Major publications
- 1 inproceedingsInstruction-driven history-aware policies for robotic manipulations.CoRL 2022 - Conference on Robot LearningAukland, New ZealandDecember 2022HAL
- 2 miscA minimum swept-volume metric structure for configuration space.November 2022HALDOI
- 3 miscDifferentiable Collision Detection: a Randomized Smoothing Approach.April 2023HAL
- 4 inproceedingsVid2Seq: Large-Scale Pretraining of a Visual Language Model for Dense Video Captioning.CVPR 2023 - IEEE/CVF Conference on Computer Vision and Pattern RecognitionVancouver, Canada2023HAL
12.2 Publications of the year
International journals
- 5 articleCertifiably optimal rotation and pose estimation based on the Cayley map.The International Journal of Robotics Research2025. In press. HALDOIback to text
- 6 articleMODEL&CO: Exoplanet detection in angular differential imaging by learning across multiple observations.Monthly Notices of the Royal Astronomical Society5342September 2024, 1569-1596HALDOIback to text
- 7 articleToward Globally Optimal State Estimation Using Automatically Tightened Semidefinite Relaxations.IEEE Transactions on Robotics40September 2024, 4338-4358HALDOIback to text
- 8 articleCo-designing versatile quadruped robots for dynamic and energy-efficient motions.Robotica426May 2024, 2004-2025HALDOIback to text
- 9 articledeep PACO: Combining statistical models with deep learning for exoplanet detection and characterization in direct imaging at high contrast.Monthly Notices of the Royal Astronomical Society5271January 2024, 1534-1562HALDOIback to text
- 10 articleOn Semidefinite Relaxations for Matrix-Weighted State-Estimation Problems in Robotics.IEEE Transactions on Robotics40October 2024, 4805-4824HALDOIback to text
- 11 articleReconciling RaiSim with the Maximum Dissipation Principle.IEEE Transactions on RoboticsJuly 2024. In press. HALback to text
- 12 articleContact Models in Robotics: a Comparative Analysis.IEEE Transactions on Robotics40July 2024, 3716 - 3733HALDOIback to text
- 13 articleLeveraging Randomized Smoothing for Optimal Control of Nonsmooth Dynamical Systems.Nonlinear Analysis: Hybrid Systems52January 2024, 101468HALDOIback to text
- 14 articleGJK++: Leveraging Acceleration Methods for Faster Collision Detection.IEEE Transactions on RoboticsApril 2024. In press. HALDOIback to text
- 15 articleCoVR-2: Automatic Data Construction for Composed Video Retrieval.IEEE Transactions on Pattern Analysis and Machine IntelligenceSeptember 2024, 1-12HALDOIback to text
International peer-reviewed conferences
- 16 inproceedingsLeveraging augmented-Lagrangian techniques for differentiating over infeasible quadratic programs in machine learning.ICLR 2024 - The Twelfth International Conference on Learning RepresentationsVienne, AustriaMay 2024HALback to textback to text
- 17 inproceedingsLearning System Dynamics from Sensory Input under Optimal Control Principles.CDC 2024 Conference on Decision and ControlMilan, ItalyDecember 2024HALback to text
- 18 inproceedingsFrom Compliant to Rigid Contact Simulation: a Unified and Efficient Approach.20th edition of the “Robotics: Science and Systems” (RSS) ConferenceDelft, NetherlandsJuly 2024HALback to text
- 19 inproceedingsOnline 3D Scene Reconstruction Using Neural Object Priors.3DV 2025 - 12th International Conference on 3D Vision 2025Singapore, SingaporeMarch 2025HALback to text
- 20 inproceedingsHistorical Printed Ornaments: Dataset and Tasks.ICDAR 2024 - International Conference on Document Analysis and Recognition14806Lecture Notes in Computer ScienceAthens, GreeceSpringer Nature SwitzerlandSeptember 2024, 251-270HALDOIback to text
- 21 inproceedingsSUGAR : Pre-training 3D Visual Representations for Robotics.CVPR 2024 - IEEE/CVF Conference on Computer Vision and Pattern RecognitionSeattle WA, United StatesJune 2024, 18049-18060HALDOIback to text
- 22 inproceedingsFrom centroidal to whole-body models for legged locomotion: a comparative analysis.IEEE-RAS 23rd International Conference on Humanoid Robots (Humanoids 2024)Nancy, FranceNovember 2024HALback to text
- 23 inproceedingsExploiting Chordal Sparsity for Fast Global Optimality with Application to Localization.Proceedings of the Sixteenth Workshop on the Algorithmic Foundations of RoboticsWAFR 2024 - 16th International Workshop on the Algorithmic Foundations of RoboticsChicago, United StatesOctober 2024HALback to text
- 24 inproceedingsParallel and Proximal Constrained Linear-Quadratic Methods for Real-Time Nonlinear MPC.Robotics: Science and SystemsDelft, NetherlandsJuly 2024HALback to text
- 25 inproceedingsForce Feedback Model-Predictive Control via Online Estimation.IEEE International Conference on Robotics and Automation (ICRA 2024)Yokohama, JapanMay 2024HALDOIback to text
- 26 inproceedingsCondensed semi-implicit dynamics for trajectory optimization in soft robotics.IEEE International Conference on Soft Robotics (RoboSoft)San Diego (CA), United StatesApril 2024HALDOIback to text
- 27 inproceedingsGeodesic turnpikes for robot motion planning.WAFR 2024 - Workshop on the Algorithmic Foundations of RoboticsChicago (IL), United StatesOctober 2024HALback to text
- 28 inproceedingsA Grasping Movement Intention Estimator for Intuitive Control of Assistive Devices.ICNR 2024 - International Conference on NeuroRehabilitationLa granja, SpainNovember 2024HALback to text
- 29 inproceedingsi-GRIP, a Grasping Movement Intention Estimator for Intuitive Control of Assistive Devices.IEEE XploreHumanoids 2024 - IEEE-RAS International Conference on Humanoid RobotsNancy, FranceNovember 2024HALback to text
- 30 inproceedingsLinear-time Differential Inverse Kinematics: an Augmented Lagrangian Perspective.RSS 2024 - Robotics: Science and SystemsDelft, NetherlandsJuly 2024HALback to text
Doctoral dissertations and habilitation theses
- 31 thesisPrimal-dual proximal augmented lagrangian methods for quadratic programming : theory and implementation.Université Paris sciences et lettresJanuary 2024HALback to text
- 32 thesisSelf-Supervised Learning with Joint-Embedding Predictive Architectures.ENS Paris - Ecole Normale Supérieure de ParisApril 2024HALback to text
- 33 thesisLearning System Dynamics for Control from Sensory Input.PSL UniversitéOctober 2024HALback to text
- 34 thesisAutomatic Image Quality Assessment on Portraits.ENS-PSLNovember 2024HALback to text
- 35 thesisReal-time constrained trajectory optimisation in robotics: theory, implementation, and applications.UPS ToulouseNovember 2024HALback to text
- 36 thesisDifferentiable optimization for robotics: simulation, learning and control..Ecole normale supérieure - ENS PARISDecember 2024HALback to text
- 37 thesisPixel-Level Tracking and Future Prediction in Video Streams.Ecole normale supérieure - ENS PARISJuly 2024HALback to text
- 38 thesisGeometry and Metric Structures of Configuration Spaces for Robotic Motion Planning.Ecole normale supérieure PSLDecember 2024HALback to text
- 39 thesisAlgorithmic and Computational Foundations of Differentiable Collision Detection.PSL Research University; CVUT in Prague, Faculty of Electrical EngineeringDecember 2024HALback to text
Reports & preprints
- 40 miscCLEO: Closed-Loop kinematics Evolutionary Optimization of bipedal structures.October 2024HALback to text
- 41 miscPICNIQ: Pairwise Comparisons for Natural Image Quality Assessment.March 2024, 9968-9978HALback to text
- 42 miscGeneralized Portrait Quality Assessment.February 2024HALDOIback to text
- 43 miscViViDex: Learning Vision-based Dexterous Manipulation from Human Videos.September 2024HALback to text
- 44 miscTowards Zero-Shot Multimodal Machine Translation.July 2024HALback to text
- 45 miscmOSCAR: A Large-scale Multilingual and Multimodal Document-level Corpus.June 2024HALback to text
- 46 miscTowards Generalizable Vision-Language Robotic Manipulation: A Benchmark and LLM-guided 3D Policy.October 2024HALback to text
- 47 miscA Data-driven Contact Estimation Method for Wheeled-Biped Robots.January 2025HALback to text
- 48 miscEnd-to-End and Highly-Efficient Differentiable Simulation for Robotics.September 2024HALback to text
- 49 miscControl of Humanoid Robots with Parallel Mechanisms using Kinematic Actuation Models.March 2025HAL
- 50 miscOptimal Control of Walkers with Parallel Actuation.March 2025HALback to text
- 51 miscConstrained Articulated Body Algorithms for Closed-Loop Mechanisms.January 2025HALback to text
- 52 miscConstrained Articulated Body Dynamics Algorithms.February 2024HALback to text
- 53 miscSatellite Image Time Series Semantic Change Detection: Novel Architecture and Analysis of Domain Shift.2024HALDOIback to text
- 54 miscDetecting Looted Archaeological Sites from Satellite Image Time Series.2024HALDOIback to text