Keywords
Computer Science and Digital Science
- A5.1.1. Engineering of interactive systems
- A5.1.2. Evaluation of interactive systems
- A5.1.4. Brain-computer interfaces, physiological computing
- A5.1.5. Body-based interfaces
- A5.1.6. Tangible interfaces
- A5.1.7. Multimodal interfaces
- A5.3.3. Pattern recognition
- A5.4.1. Object recognition
- A5.4.2. Activity recognition
- A5.7.3. Speech
- A5.8. Natural language processing
- A5.10.5. Robot interaction (with the environment, humans, other robots)
- A5.10.7. Learning
- A5.10.8. Cognitive robotics and systems
- A5.11.1. Human activity analysis and recognition
- A6.3.1. Inverse problems
- A9. Artificial intelligence
- A9.2. Machine learning
- A9.5. Robotics
- A9.7. AI algorithmics
Other Research Topics and Application Domains
- B1.2.1. Understanding and simulation of the brain and the nervous system
- B1.2.2. Cognitive science
- B5.6. Robotic systems
- B5.7. 3D printing
- B5.8. Learning and training
- B9. Society and Knowledge
- B9.1. Education
- B9.1.1. E-learning, MOOC
- B9.2. Art
- B9.2.1. Music, sound
- B9.2.4. Theater
- B9.6. Humanities
- B9.6.1. Psychology
- B9.6.8. Linguistics
- B9.7. Knowledge dissemination
1 Team members, visitors, external collaborators
Research Scientists
- Pierre-Yves Oudeyer [Team leader, Inria, Senior Researcher, HDR]
- Clément Moulin-Frier [Inria, Researcher]
Faculty Members
- Natalia Diaz Rodriguez [École Nationale Supérieure de Techniques Avancées]
- David Filliat [École Nationale Supérieure de Techniques Avancées, Professor, HDR]
- Cécile Mazon [Univ de Bordeaux, Associate Professor]
- Mai Nguyen [École Nationale Supérieure de Techniques Avancées, until Nov 2020]
- Helene Sauzeon [Univ de Bordeaux, Professor, HDR]
Post-Doctoral Fellows
- Eleni Nisioti [Inria, from Dec 2020]
- Chris Reinke [Inria, until Feb 2020]
PhD Students
- Rania Abdelghani [Evidenceb, CIFRE, from Dec 2020]
- Maxime Adolphe [Onepoint, CIFRE, from Sep 2020]
- Mehdi Alaimi [Inria, from Feb 2020 until Aug 2020]
- Florence Carton [CEA]
- Hugo Caselles-Dupre [Softbank Robotics]
- Cedric Colas [Inria]
- Thibault Desprez [Inria, from Apr 2020 until Jun 2020]
- Mayalen Etcheverry [Poietis, CIFRE]
- Tristan Karch [Inria]
- Timothee Lesort [École Nationale Supérieure de Techniques Avancées, until May 2020]
- Eleni Nisioti [Inria, Nov 2020]
- Vyshakh Palli Thaza [Renault, CIFRE]
- Remy Portelas [Inria]
- Thomas Rojat [Renault, CIFRE, from Mar 2020]
- Julius Taylor [Inria, from Nov 2020]
- Alexandr Ten [Inria]
- Maria Teodorescu [Inria, from Mar 2020]
Technical Staff
- Benjamin Clément [Inria, Engineer]
- Mayalen Etcheverry [Inria, Engineer, from Feb 2020 until Aug 2020]
- Grgur Kovac [Inria, Engineer]
- Alexandre Pere [Inria, Engineer, until Feb 2020]
- Clement Romac [Inria, Engineer, from Oct 2020]
- Didier Roy [Inria]
Interns and Apprentices
- Maxime Adolphe [Inria, from Feb 2020 until Jul 2020]
- Thibault Audouit [Inria, from May 2020 until Jul 2020]
- Camille Chastagnol [Association pour le développement de l'enseignement et des recherches d'Aquitaine, from Feb 2020 until Jun 2020]
- Younes Rabii [Inria, from Feb 2020 until Jul 2020]
- Clement Romac [Inria, from Mar 2020 until Aug 2020]
- Djiby Soumare [Inria, from May 2020 until Jul 2020]
- Maria Teodorescu [Inria, until Feb 2020]
- Valentin Villecroze [Inria, from Apr 2020 until Aug 2020]
Administrative Assistant
- Nathalie Robin [Inria]
Visiting Scientist
- Kevyn Collins-Thompson [Université du Michigan, until Jul 2020]
External Collaborator
- Wang Chak Chan [Automated Systems Limited-Hong Kong, from Nov 2020]
2 Overall objectives
The Flowers project-team, at Inria, University of Bordeaux and Ensta ParisTech, studies models of open-ended development and learning. These models are used as tools to help us understand better how children learn, as well as to build machines that learn like children, i.e. developmental artificial intelligence, with applications in educational technologies, automated discovery, robotics and human-computer interaction.
A major scientific challenge in artificial intelligence and cognitive sciences is to understand how humans and machines can efficiently acquire world models, as well as open and cumulative repertoires of skills over an extended time span. Processes of sensorimotor, cognitive and social development are organized along ordered phases of increasing complexity, and result from the complex interaction between the brain/body with its physical and social environment.
To advance the fundamental understanding of mechanisms of development, the FLOWERS team develops computational models that leverage advanced machine learning techniques such as intrinsically motivated deep reinforcement learning, in strong collaboration with developmental psychology and neuroscience. In particular, the team focuses on models of intrinsically motivated learning and exploration (also called curiosity-driven learning), with mechanisms enabling agents to learn to represent and generate their own goals, self-organizing a learning curriculum for efficient learning of world models and skill repertoire under limited resources of time, energy and compute. The team also studies how autonomous learning mechanisms can enable humans and machines to acquire grounded language skills, using neuro-symbolic architectures for learning structured representations and handling systematic compositionality and generalization.
Beyond leading to new theories and new experimental paradigms to understand human development in cognitive science, as well as new fundamental approaches to developmental machine learning, the team explores how such models can find applications in robotics, human-computer interaction, multi-agent systems, automated discovery and educational technologies. In robotics, the team studies how artificial curiosity combined with imitation learning can provide essential building blocks allowing robots to acquire multiple tasks through natural interaction with naïve human users, for example in the context of assistive robotics. The team also studies how models of curiosity-driven learning can be transposed in algorithms for intelligent tutoring systems, allowing educational software to incrementally and dynamically adapt to the particularities of each human learner, and proposing personalized sequences of teaching activities.
Research axes
The work of FLOWERS is organized around the following axis:
- Curiosity-driven exploration and sensorimotor learning: intrinsic motivation are mechanisms that have been identified by developmental psychologists to explain important forms of spontaneous exploration and curiosity. In FLOWERS, we try to develop computational intrinsic motivation systems, and test them on embodied machines, allowing to regulate the growth of complexity in exploratory behaviours. These mechanisms are studied as active learning mechanisms, allowing to learn efficiently in large inhomogeneous sensorimotor spaces and environments;
- Cumulative learning of sensorimotor skills: FLOWERS develops machine learning algorithms that can allow embodied machines to acquire cumulatively sensorimotor skills. In particular, we develop optimization and reinforcement learning systems which allow robots to discover and learn dictionaries of motor primitives, and then combine them to form higher-level sensorimotor skills.
- Natural and intuitive social learning: FLOWERS develops interaction frameworks and learning mechanisms allowing non-engineer humans to teach a robot naturally. This involves two sub-themes: 1) techniques allowing for natural and intuitive human-robot interaction, including simple ergonomic interfaces for establishing joint attention; 2) learning mechanisms that allow the robot to use the guidance hints provided by the human to teach new skills;
- Discovering and abstracting the structure of sets of uninterpreted sensors and motors: FLOWERS studies mechanisms that allow a robot to infer structural information out of sets of sensorimotor channels whose semantics is unknown, for example the topology of the body and the sensorimotor contingencies (propriocetive, visual and acoustic). This process is meant to be open-ended, progressing in continuous operation from initially simple representations to abstract concepts and categories similar to those used by humans.
- Body design and role of the body in sensorimotor and social development: We study how the physical properties of the body (geometry, materials, distribution of mass, growth, ...) can impact the acquisition of sensorimotor and interaction skills. This requires to consider the body as an experimental variable, and for this we develop special methodologies for designing and evaluating rapidly new morphologies, especially using rapid prototyping techniques like 3D printing.
- Emergence of social behavior in multi-agent populations: We study how populations of interacting learning agents can collectively acquire cooperative or competitive strategies in challenging simulated environments. We specifically focus on the role of two factors: (i) Cognitive architectures, including the role of curiosity-driven exploration in the emergence of complex social behavior ; (2) Environmental dynamics, including how task structure and environmental variability influence emergent social behavior. Our work is grounded in principles and theories from behavioral ecology and language evolution; and uses recent advances in multi-agent reinforcement learning as a modeling framework.
- Curiosity and intrinsic motivations in cognitive learning: We are extending our research on the role of curiosity and intrinsic motivations on learning through human experimentations, and along two lines of research. The first aims to develop a lifelong approach for the role of intrinsic motivation and curiosity in cognitive learning (e.g., spatial learning, attentional learning, etc.) at all ages of life (children, young adults and older adults) in order to demonstrate the lifespan developmental nature of these mechanisms in knowledge acquisition and cognitive development. Basically, the aim is to examine whether curiosity/ intrinsic motivation is an essential ingredient for tackling inter-individual variability in learning performance. The second axis aims to study the links between states of curiosity, learning progress and metacognition. The aim is to address the question of the role of metacognitive strategies of self-regulation in the generation of states of curiosity and learning progress.
- Educational technologies and Intelligent Tutoring Systems: FLOWERS develops new educational technologies or Intelligent Tutorial Systems, using both curiosity-related models and artificial intelligence techniques (online optimization methods) in order to personalize learning sequences for each individual and to maximize curiosity and learning in real world context (at school or on MOOC platform). Two areas of research are being investigated : 1) the design of curiosity-driven interactive education systems by introducing mechanisms for self-questioning and self-exploration of knowledge during the learning process; 2) the design of Intelligent Tutoring systems promoting individual learning progress while personalizing the learning path in the task space to be covered by the learner. These two areas are enriched by applied studies in neuropsychological clinics (aging, cognitive disorders related to neurodevelopmental syndromes such as autistic spectrum or attentional disorders) where inter-individual variability is a critical challenge for designing educational programs or cognitive training or remediation programs.
3 Research program
Research in artificial intelligence, machine learning and pattern recognition has produced a tremendous amount of results and concepts in the last decades. A blooming number of learning paradigms - supervised, unsupervised, reinforcement, active, associative, symbolic, connectionist, situated, hybrid, distributed learning... - nourished the elaboration of highly sophisticated algorithms for tasks such as visual object recognition, speech recognition, robot walking, grasping or navigation, the prediction of stock prices, the evaluation of risk for insurances, adaptive data routing on the internet, etc... Yet, we are still very far from being able to build machines capable of adapting to the physical and social environment with the flexibility, robustness, and versatility of a one-year-old human child.
Indeed, one striking characteristic of human children is the nearly open-ended diversity of the skills they learn. They not only can improve existing skills, but also continuously learn new ones. If evolution certainly provided them with specific pre-wiring for certain activities such as feeding or visual object tracking, evidence shows that there are also numerous skills that they learn smoothly but could not be “anticipated” by biological evolution, for example learning to drive a tricycle, using an electronic piano toy or using a video game joystick. On the contrary, existing learning machines, and robots in particular, are typically only able to learn a single pre-specified task or a single kind of skill. Once this task is learnt, for example walking with two legs, learning is over. If one wants the robot to learn a second task, for example grasping objects in its visual field, then an engineer needs to re-program manually its learning structures: traditional approaches to task-specific machine/robot learning typically include engineer choices of the relevant sensorimotor channels, specific design of the reward function, choices about when learning begins and ends, and what learning algorithms and associated parameters shall be optimized.
As can be seen, this requires a lot of important choices from the engineer, and one could hardly use the term “autonomous” learning. On the contrary, human children do not learn following anything looking like that process, at least during their very first years. Babies develop and explore the world by themselves, focusing their interest on various activities driven both by internal motives and social guidance from adults who only have a folk understanding of their brains. Adults provide learning opportunities and scaffolding, but eventually young babies always decide for themselves what activity to practice or not. Specific tasks are rarely imposed to them. Yet, they steadily discover and learn how to use their body as well as its relationships with the physical and social environment. Also, the spectrum of skills that they learn continuously expands in an organized manner: they undergo a developmental trajectory in which simple skills are learnt first, and skills of progressively increasing complexity are subsequently learnt.
A link can be made to educational systems where research in several domains have tried to study how to provide a good learning or training experience to learners. This includes the experiences that allow better learning, and in which sequence they must be experienced. This problem is complementary to that of the learner who tries to progress efficiently, and the teacher here has to use as efficiently the limited time and motivational resources of the learner. Several results from psychology 75 and neuroscience 94 have argued that the human brain feels intrinsic pleasure in practicing activities of optimal difficulty or challenge. A teacher must exploit such activities to create positive psychological states of flow 86 for fostering the indivual engagement in learning activities. A such view is also relevant for reeducation issues where inter-individual variability, and thus intervention personalization are challenges of the same magnitude as those for education of children.
A grand challenge is thus to be able to build machines that possess this capability to discover, adapt and develop continuously new know-how and new knowledge in unknown and changing environments, like human children. In 1950, Turing wrote that the child's brain would show us the way to intelligence: “Instead of trying to produce a program to simulate the adult mind, why not rather try to produce one which simulates the child's” 152. Maybe, in opposition to work in the field of Artificial Intelligence who has focused on mechanisms trying to match the capabilities of “intelligent” human adults such as chess playing or natural language dialogue 100, it is time to take the advice of Turing seriously. This is what a new field, called developmental (or epigenetic) robotics, is trying to achieve 116156. The approach of developmental robotics consists in importing and implementing concepts and mechanisms from developmental psychology 121, cognitive linguistics 85, and developmental cognitive neuroscience 104 where there has been a considerable amount of research and theories to understand and explain how children learn and develop. A number of general principles are underlying this research agenda: embodiment 78134, grounding 98, situatedness 148, self-organization 150129, enaction 154, and incremental learning 81.
Among the many issues and challenges of developmental robotics, two of them are of paramount importance: exploration mechanisms and mechanisms for abstracting and making sense of initially unknown sensorimotor channels. Indeed, the typical space of sensorimotor skills that can be encountered and learnt by a developmental robot, as those encountered by human infants, is immensely vast and inhomogeneous. With a sufficiently rich environment and multimodal set of sensors and effectors, the space of possible sensorimotor activities is simply too large to be explored exhaustively in any robot's life time: it is impossible to learn all possible skills and represent all conceivable sensory percepts. Moreover, some skills are very basic to learn, some other very complicated, and many of them require the mastery of others in order to be learnt. For example, learning to manipulate a piano toy requires first to know how to move one's hand to reach the piano and how to touch specific parts of the toy with the fingers. And knowing how to move the hand might require to know how to track it visually.
Exploring such a space of skills randomly is bound to fail or result at best on very inefficient learning 130. Thus, exploration needs to be organized and guided. The approach of epigenetic robotics is to take inspiration from the mechanisms that allow human infants to be progressively guided, i.e. to develop. There are two broad classes of guiding mechanisms which control exploration:
- internal guiding mechanisms, and in particular intrinsic motivation, responsible of spontaneous exploration and curiosity in humans, which is one of the central mechanisms investigated in FLOWERS, and technically amounts to achieve online active self-regulation of the growth of complexity in learning situations;
- social learning and guidance, a learning mechanisms that exploits the knowledge of other agents in the environment and/or that is guided by those same agents. These mechanisms exist in many different forms like emotional reinforcement, stimulus enhancement, social motivation, guidance, feedback or imitation, some of which being also investigated in FLOWERS;
Internal guiding mechanisms
In infant development, one observes a progressive increase of the complexity of activities with an associated progressive increase of capabilities 121, children do not learn everything at one time: for example, they first learn to roll over, then to crawl and sit, and only when these skills are operational, they begin to learn how to stand. The perceptual system also gradually develops, increasing children perceptual capabilities other time while they engage in activities like throwing or manipulating objects. This make it possible to learn to identify objects in more and more complex situations and to learn more and more of their physical characteristics.
Development is therefore progressive and incremental, and this might be a crucial feature explaining the efficiency with which children explore and learn so fast. Taking inspiration from these observations, some roboticists and researchers in machine learning have argued that learning a given task could be made much easier for a robot if it followed a developmental sequence and “started simple” 6889. However, in these experiments, the developmental sequence was crafted by hand: roboticists manually build simpler versions of a complex task and put the robot successively in versions of the task of increasing complexity. And when they wanted the robot to learn a new task, they had to design a novel reward function.
Thus, there is a need for mechanisms that allow the autonomous control and generation of the developmental trajectory. Psychologists have proposed that intrinsic motivations play a crucial role. Intrinsic motivations are mechanisms that push humans to explore activities or situations that have intermediate/optimal levels of novelty, cognitive dissonance, or challenge 758688. Futher, the exploration of critical role of intrinsic motivation as lever of cognitive developement for all and for all ages is today expanded to several fields of research, closest to its original study, special education or cognitive aging, and farther away, neuropsychological clinical research. The role and structure of intrinsic motivation in humans have been made more precise thanks to recent discoveries in neuroscience showing the implication of dopaminergic circuits and in exploration behaviours and curiosity 87101145. Based on this, a number of researchers have began in the past few years to build computational implementation of intrinsic motivation 13013214372102118144. While initial models were developed for simple simulated worlds, a current challenge is to manage to build intrinsic motivation systems that can efficiently drive exploratory behaviour in high-dimensional unprepared real world robotic sensorimotor spaces 132, 130, 133, 142. Specific and complex problems are posed by real sensorimotor spaces, in particular due to the fact that they are both high-dimensional as well as (usually) deeply inhomogeneous. As an example for the latter issue, some regions of real sensorimotor spaces are often unlearnable due to inherent stochasticity or difficulty, in which case heuristics based on the incentive to explore zones of maximal unpredictability or uncertainty, which are often used in the field of active learning 8499 typically lead to catastrophic results. The issue of high dimensionality does not only concern motor spaces, but also sensory spaces, leading to the problem of correctly identifying, among typically thousands of quantities, those latent variables that have links to behavioral choices. In FLOWERS, we aim at developing intrinsically motivated exploration mechanisms that scale in those spaces, by studying suitable abstraction processes in conjunction with exploration strategies.
Socially Guided and Interactive Learning
Social guidance is as important as intrinsic motivation in the cognitive development of human babies 121. There is a vast literature on learning by demonstration in robots where the actions of humans in the environment are recognized and transferred to robots 67. Most such approaches are completely passive: the human executes actions and the robot learns from the acquired data. Recently, the notion of interactive learning has been introduced in 151, 77, motivated by the various mechanisms that allow humans to socially guide a robot 139. In an interactive context the steps of self-exploration and social guidance are not separated and a robot learns by self exploration and by receiving extra feedback from the social context 151, 109, 119.
Social guidance is also particularly important for learning to segment and categorize the perceptual space. Indeed, parents interact a lot with infants, for example teaching them to recognize and name objects or characteristics of these objects. Their role is particularly important in directing the infant attention towards objects of interest that will make it possible to simplify at first the perceptual space by pointing out a segment of the environment that can be isolated, named and acted upon. These interactions will then be complemented by the children own experiments on the objects chosen according to intrinsic motivation in order to improve the knowledge of the object, its physical properties and the actions that could be performed with it.
In FLOWERS, we are aiming at including intrinsic motivation system in the self-exploration part thus combining efficient self-learning with social guidance 126, 127. We also work on developing perceptual capabilities by gradually segmenting the perceptual space and identifying objects and their characteristics through interaction with the user 117 and robots experiments 103. Another challenge is to allow for more flexible interaction protocols with the user in terms of what type of feedback is provided and how it is provided 114.
Exploration mechanisms are combined with research in the following directions:
Cumulative learning, reinforcement learning and optimization of autonomous skill learning
FLOWERS develops machine learning algorithms that can allow embodied machines to acquire cumulatively sensorimotor skills. In particular, we develop optimization and reinforcement learning systems which allow robots to discover and learn dictionaries of motor primitives, and then combine them to form higher-level sensorimotor skills.
Autonomous perceptual and representation learning
In order to harness the complexity of perceptual and motor spaces, as well as to pave the way to higher-level cognitive skills, developmental learning requires abstraction mechanisms that can infer structural information out of sets of sensorimotor channels whose semantics is unknown, discovering for example the topology of the body or the sensorimotor contingencies (proprioceptive, visual and acoustic). This process is meant to be open- ended, progressing in continuous operation from initially simple representations towards abstract concepts and categories similar to those used by humans. Our work focuses on the study of various techniques for:
- autonomous multimodal dimensionality reduction and concept discovery;
- incremental discovery and learning of objects using vision and active exploration, as well as of auditory speech invariants;
- learning of dictionaries of motion primitives with combinatorial structures, in combination with linguistic description;
- active learning of visual descriptors useful for action (e.g. grasping).
Embodiment and maturational constraints
FLOWERS studies how adequate morphologies and materials (i.e. morphological computation), associated to relevant dynamical motor primitives, can importantly simplify the acquisition of apparently very complex skills such as full-body dynamic walking in biped. FLOWERS also studies maturational constraints, which are mechanisms that allow for the progressive and controlled release of new degrees of freedoms in the sensorimotor space of robots.
Discovering and abstracting the structure of sets of uninterpreted sensors and motors
FLOWERS studies mechanisms that allow a robot to infer structural information out of sets of sensorimotor channels whose semantics is unknown, for example the topology of the body and the sensorimotor contingencies (proprioceptive, visual and acoustic). This process is meant to be open-ended, progressing in continuous operation from initially simple representations to abstract concepts and categories similar to those used by humans.
Emergence of social behavior in multi-agent populations
FLOWERS studies how populations of interacting learning agents can collectively acquire cooperative or competitive strategies in challenging simulated environments. This differs from "Social learning and guidance" presented above: instead of studying how a learning agent can benefit from the interaction with a skilled agent, we rather consider here how social behavior can spontaneously emerge from a population of interacting learning agents. We focus on studying and modeling the emergence of cooperation, communication and cultural innovation based on theories in behavioral ecology and language evolution, using recent advances in multi-agent reinforcement learning.
Cognitive variability across Lifelong development and (re)educational Technologies
Over the past decade, the progress in the field of curiosity-driven learning generates a lot of hope, especially with regard to a major challenge, namely the inter-individual variability of developmental trajectories of learning, which is particularly critical during childhood and aging or in conditions of cognitive disorders. With the societal purpose of tackling of social inegalities, FLOWERS deals to move forward this new research avenue by exploring the changes of states of curiosity across lifespan and across neurodevelopemental conditions (neurotypical vs. learning disabilities) while designing new educational or rehabilitative technologies for curiosity-driven learning. The information gaps or learning progress, and their awareness are the core mechanisms of this part of research program due to high value as brain fuel by which the individual's internal intrinsic state of motivation is maintained and leads him/her to pursue his/her cognitive efforts for acquisitions /rehabilitations. Accordingly, a main challenge is to understand these mechanisms in order to draw up supports for the curiosity-driven learning, and then to embed them into (re)educational technologies. To this end, two-ways of investigations are carried out in real-life setting (school, home, work place etc): 1) the design of curiosity-driven interactive systems for learning and their effectiveness study ; and 2) the automated personnalization of learning programs through new algorithms maximizing learning progress in ITS.
4 Application domains
Neuroscience, Developmental Psychology and Cognitive Sciences The computational modelling of life-long learning and development mechanisms achieved in the team centrally targets to contribute to our understanding of the processes of sensorimotor, cognitive and social development in humans. In particular, it provides a methodological basis to analyze the dynamics of the interaction across learning and inference processes, embodiment and the social environment, allowing to formalize precise hypotheses and later on test them in experimental paradigms with animals and humans. A paradigmatic example of this activity is the Neurocuriosity project achieved in collaboration with the cognitive neuroscience lab of Jacqueline Gottlieb, where theoretical models of the mechanisms of information seeking, active learning and spontaneous exploration have been developed in coordination with experimental evidence and investigation, see https://
Personal and lifelong learning assistive agents Many indicators show that the arrival of personal assistive agents in everyday life, ranging from digital assistants to robots, will be a major fact of the 21st century. These agents will range from purely entertainment or educative applications to social companions that many argue will be of crucial help in our society. Yet, to realize this vision, important obstacles need to be overcome: these agents will have to evolve in unpredictable environments and learn new skills in a lifelong manner while interacting with non-engineer humans, which is out of reach of current technology. In this context, the refoundation of intelligent systems that developmental AI is exploring opens potentially novel horizons to solve these problems. In particular, this application domain requires advances in artificial intelligence that go beyond the current state-of-the-art in fields like deep learning. Currently these techniques require tremendous amounts of data in order to function properly, and they are severely limited in terms of incremental and transfer learning. One of our goals is to drastically reduce the amount of data required in order for this very potent field to work when humans are in-the-loop. We try to achieve this by making neural networks aware of their knowledge, i.e. we introduce the concept of uncertainty, and use it as part of intrinsically motivated multitask learning architectures, and combined with techniques of learning by imitation.
Educational technologies that foster curiosity-driven and personalized learning. Optimal teaching and efficient teaching/learning environments can be applied to aid teaching in schools aiming both at increase the achievement levels and the reduce time needed. From a practical perspective, improved models could be saving millions of hours of students' time (and effort) in learning. These models should also predict the achievement levels of students in order to influence teaching practices. The challenges of the school of the 21st century, and in particular to produce conditions for active learning that are personalized to the student's motivations, are challenges shared with other applied fields. Special education for children with special needs, such as learning disabilities, has long recognized the difficulty of personalizing contents and pedagogies due to the great variability between and within medical conditions. More remotely, but not so much, cognitive rehabilitative carers are facing the same challenges where today they propose standardized cognitive training or rehabilitation programs but for which the benefits are modest (some individuals respond to the programs, others respond little or not at all), as they are highly subject to inter- and intra-individual variability. The curiosity-driven technologies for learning and STIs could be a promising avenue to address these issues that are common to (mainstream and specialized)education and cognitive rehabilitation.
Automated discovery in science. Machine learning algorithms integrating intrinsically-motivated goal exploration processes (IMGEPs) with flexible modular representation learning are very promising directions to help human scientists discover novel structures in complex dynamical systems, in fields ranging from biology to physics. The automated discovery project lead by the FLOWERS team aims to boost the efficiency of these algorithms for enabling scientist to better understand the space of dynamics of bio-physical systems, that could include systems related to the design of new materials or new drugs with applications ranging from regenerative medicine to unraveling the chemical origins of life. As an example, Grizou et al. 96 recently showed how IMGEPs can be used to automate chemistry experiments addressing fundamental questions related to the origins of life (how oil droplets may self-organize into protocellular structures), leading to new insights about oil droplet chemistry. Such methods can be applied to a large range of complex systems in order to map the possible self-organized structures. The automated discovery project is intended to be interdisciplinary and to involve potentially non-expert end-users from a variety of domains. In this regard, we are currently collaborating with Poietis (a bio-printing company) and Bert Chan (an independant researcher in artificial life) to deploy our algorithms. To encourage the adoption of our algorithms by a wider community, we are also working on an interactive software which aims to provide tools to easily use the automated exploration algorithms (e.g. curiosity-driven) in various systems.
Human-Robot Collaboration. Robots play a vital role for industry and ensure the efficient and competitive production of a wide range of goods. They replace humans in many tasks which otherwise would be too difficult, too dangerous, or too expensive to perform. However, the new needs and desires of the society call for manufacturing system centered around personalized products and small series productions. Human-robot collaboration could widen the use of robot in this new situations if robots become cheaper, easier to program and safe to interact with. The most relevant systems for such applications would follow an expert worker and works with (some) autonomy, but being always under supervision of the human and acts based on its task models.
Environment perception in intelligent vehicles. When working in simulated traffic environments, elements of FLOWERS research can be applied to the autonomous acquisition of increasingly abstract representations of both traffic objects and traffic scenes. In particular, the object classes of vehicles and pedestrians are if interest when considering detection tasks in safety systems, as well as scene categories (”scene context”) that have a strong impact on the occurrence of these object classes. As already indicated by several investigations in the field, results from present-day simulation technology can be transferred to the real world with little impact on performance. Therefore, applications of FLOWERS research that is suitably verified by real-world benchmarks has direct applicability in safety-system products for intelligent vehicles.
5 Social and environmental responsibility
5.1 Footprint of research activities
AI is a field of research that currently requires a lot of computational resources, which is a challenge as these resources have an environmental cost. In the team we try to address this challenge in two ways:
- by working on developmental machine learning approaches that model how humans manage to learn open-ended and diverse repertoires of skills under severe limits of time, energy and compute: for example, curiosity-driven learning algorithms can be used to guide agent's exploration of their environment so that they learn a world model in a sample efficient manner, i.e. by minimizing the number of runs and computations they need to perform in the environment;
- by monitoring the number of CPU and GPU hours required to carry out our experiments. For instance, our work 43 used a total of 2.5 cpu years. More globally, our work uses large scale computational resources, such as the Jean Zay supercomputer platform, for which we obtained a credit of 2 millions hours of GPU and CPU for year 2021.
5.2 Impact of research results
Our research activities are organized along two fundamental research axis (models of human learning and algorithms for developmental machine learning) and one application research axis (involving multiple domains of application, see the Application Domains section). This entails different dimensions of potential societal impact:
- Towards autonomous agents that can be shaped to human preferences and be explainable We work on reinforcement learning architectures where autonomous agents interact with a social partner to explore a large set of possible interactions and learn to master them, using language as a key communication medium. As a result, our work contributes to facilitating human intervention in the learning process of agents (e.g. digital assistants, video games characters, robots), which we believe is a key step towards more explainable and safer autonomous agents.
- Reproducibility of research: By releasing the codes of our research papers, we believe that we help efforts in reproducible science and allow the wider community to build upon and extend our work in the future. In that spirit, we also provide clear explanations on the statistical testing methods when reporting the results.
- AI and personalized educational technologies that support inclusivity and diversity and reduce inequalities The Flowers team develops AI technologies aiming to personalize sequences of educationa activities in digital educational apps: this entails the central challenge of designing systems which can have equitable impact over a diversity of students and reduce inequalitie. Using models of curiosity-driven learning to design AI algorithms for such personalization, we have been working to enable them to be positively and equitably impactful across several dimensions of diversity: for young learners or for aging populations; for learners with low initial levels as well as for learners with high initial levels; for "normally" developping children and for children with developmental disorders; and for learners of different socio-cultural backgrounds (e.g. we could show in the KidLearn project that the system is equally impactful along these various kinds of diversities).
- Health: Bio-printing The Flowers team is studying the use of curiosity-driven exploraiton algorithm in the domain of automated discovery, enabling scientists in physics/chemistry/biology to efficiently explore and build maps of the possible structures of various complex systems. One particular domain of application we are studying is bio-printing, where a challenge consists in exploring and understanding the space of morphogenetic structures self-organized by bio-printed cell populations. This could facilitate the design and bio-printing of personalized skins or organoids for people that need transplants, and thus could have major impact on the health of people needing such transplants.
- Tools for human creativity and the arts Curiosity-driven exploration algorithms could also in principle be used as tools to help human users in creative activities ranging from writing stories to painting or musical creation, which are domains we aim to consider in the future, and thus this constitutes another societal and cultural domain where our research could have impact.
-
Education to AI As artificial intelligence takes a greater role in human society, it is of foremost importance to empower individuals with understanding of these technologies. For this purpose, the Flowers lab has been actively involved in educational and popularization activities, in particular by designing educational robotics kits that form a motivating and tangible context to understand basic concepts in AI: these include the Inirobot kit (used by >30k primary school students in France, see https://
pixees. fr/ dm1r. fr/ and the Poppy Education kit (https:// www. poppy-education. org) now supported by the Poppy Station educational consortium (see https:// www. poppy-station. org)
6 Highlights of the year
Automated discovery in the sciences
The team made major progress in developping the new application domain of automated discovery in the sciences. We formalized this new research area, and introduced proof-of-concept results showing how intrinsically motivated goal exploration algorithms can be used as a tool to explore, map and learn to represent a diversity of self-organized patterns in complex dynamical systems. This opens stimulating perspectives in domains ranging from biology to chemistry and physiscs.
This work was presented in two papers accepted for oral presentation (< 1.5 % acceptance rate) at Neurips and ICLR 2020 conference. The work was achieved by Mayalen Etcheverry
(CIFRE PhD with the Poïetis company, for ICLR and Neurips) and Chris Reinke (Postdoc, for ICLR), and co-supervised
by C. Moulin-Frier (Neurips) and PY Oudeyer (ICLR and Neurips). See 38 (ICLR paper)
and 35, as well as the blog post https://
Language-guided curiosity-driven deep reinforcement learning with systematic generalization
The team made major advances in developmental machine learning, introducing techniques enabling autonomous agents to use language as a cognitive tool to imagine goals in intrinsically motivated exploration. This enables new forms of creative exploration, where agents can imagine goals that are outside the distribution of goals known so far. This approach, conceptually rooted in developmental psychology ideas from Vygotsky also leverages modular deep learning techniques enabling agents to generalize its understanding of new sentences. This work was published at Neurips 43. This work was published at Neurips 2020 43 First authors were Cécric Colas, Tristan Karch, Nicolas Lair, with co-supervision from PY Oudeyer, PF. Dominey, C. Moulin-Frier.
Educational technologies that foster curiosity-driven learning in humans
Together with the edTech industrial consortium Adaptiv'Maths (https://
Awards
PY Oudeyer was awarded an individual ANR Chair in Artificial Intelligence, and elected as Distinguised speaker of the IEEE Computational Ingelligence Society. C Moulin-Frier obtained an ANR JCJC grant, an Inria Exploratory Action and an Inria Cordi PhD grant in 2020 (see 10 for detail).
7 New software and platforms
7.1 New software
7.1.1 Explauto
- Name: an autonomous exploration library
- Keyword: Exploration
-
Scientific Description:
An important challenge in developmental robotics is how robots can be intrinsically motivated to learn efficiently parametrized policies to solve parametrized multi-task reinforcement learning problems, i.e. learn the mappings between the actions and the problem they solve, or sensory effects they produce. This can be a robot learning how arm movements make physical objects move, or how movements of a virtual vocal tract modulates vocalization sounds. The way the robot will collects its own sensorimotor experience have a strong impact on learning efficiency because for most robotic systems the involved spaces are high dimensional, the mapping between them is non-linear and redundant, and there is limited time allowed for learning. If robots explore the world in an unorganized manner, e.g. randomly, learning algorithms will be often ineffective because very sparse data points will be collected. Data are precious due to the high dimensionality and the limited time, whereas data are not equally useful due to non-linearity and redundancy. This is why learning has to be guided using efficient exploration strategies, allowing the robot to actively drive its own interaction with the environment in order to gather maximally informative data to optimize the parametrized policies. In the recent year, work in developmental learning has explored various families of algorithmic principles which allow the efficient guiding of learning and exploration.
Explauto is a framework developed to study, model and simulate curiosity-driven learning and exploration in real and simulated robotic agents. Explauto’s scientific roots trace back from Intelligent Adaptive Curiosity algorithmic architecture 131, which has been extended to a more general family of autonomous exploration architectures by 70 and recently expressed as a compact and unified formalism 123. The library is detailed in 125. In Explauto, interest models are implementing the strategies of active selection of particular problems / goals in a parametrized multi-task reinforcement learning setup to efficiently learn parametrized policies. The agent can have different available strategies, parametrized problems, models, sources of information, or learning mechanisms (for instance imitate by mimicking vs by emulation, or asking help to one teacher or to another), and chooses between them in order to optimize learning (a processus called strategic learning 128). Given a set of parametrized problems, a particular exploration strategy is to randomly draw goals/ RL problems to solve in the motor or problem space. More efficient strategies are based on the active choice of learning experiments that maximize learning progress using bandit algorithms, e.g. maximizing improvement of predictions or of competences to solve RL problems 131. This automatically drives the system to explore and learn first easy skills, and then explore skills of progressively increasing complexity. Both random and learning progress strategies can act either on the motor or on the problem space, resulting in motor babbling or goal babbling strategies.
- Motor babbling consists in sampling commands in the motor space according to a given strategy (random or learning progress), predicting the expected effect, executing the command through the environment and observing the actual effect. Both the parametrized policies and interest models are finally updated according to this experience.
- Goal babbling consists in sampling goals in the problem space and to use the current policies to infer a motor action supposed to solve the problem (inverse prediction). The robot/agent then executes the command through the environment and observes the actual effect. Both the parametrized policies and interest models are finally updated according to this experience.It has been shown that this second strategy allows a progressive solving of problems much more uniformly in the problem space than with a motor babbling strategy, where the agent samples directly in the motor space 70.
Figure 1: Complex parametrized policies involve high dimensional action and effect spaces. For the sake of visualization, the motor M and sensory S spaces are only 2D each in this example. The relationship between M and S is non-linear, dividing the sensorimotor space into regions of unequal stability: small regions of S can be reached very precisely by large regions of M, or large regions in S can be very sensitive to variations in M.: s as well as a non-linear and redundant relationship. This non-linearity can imply redundancy, where the same sensory effect can be attained using distinct regions in M. -
Functional Description:
This library provides high-level API for an easy definition of:
- Real and simulated robotic setups (Environment level),
- Incremental learning of parametrized policies (Sensorimotor level),
- Active selection of parametrized RL problems (Interest level).
The library comes with several built-in environments. Two of them corresponds to simulated environments: a multi-DoF arm acting on a 2D plan, and an under-actuated torque-controlled pendulum. The third one allows to control real robots based on Dynamixel actuators using the Pypot library. Learning parametrized policies involves machine learning algorithms, which are typically regression algorithms to learn forward models, from motor controllers to sensory effects, and optimization algorithms to learn inverse models, from sensory effects, or problems, to the motor programs allowing to reach them. We call these sensorimotor learning algorithms sensorimotor models. The library comes with several built-in sensorimotor models: simple nearest-neighbor look-up, non-parametric models combining classical regressions and optimization algorithms, online mixtures of Gaussians, and discrete Lidstone distributions. Explauto sensorimotor models are online learning algorithms, i.e. they are trained iteratively during the interaction of the robot in theenvironment in which it evolves. Explauto provides also a unified interface to define exploration strategies using the InterestModel class. The library comes with two built-in interest models: random sampling as well as sampling maximizing the learning progress in forward or inverse predictions.
Explauto environments now handle actions depending on a current context, as for instance in an environment where a robotic arm is trying to catch a ball: the arm trajectories will depend on the current position of the ball (context). Also, if the dynamic of the environment is changing over time, a new sensorimotor model (Non-Stationary Nearest Neighbor) is able to cope with those changes by taking more into account recent experiences. Those new features are explained in Jupyter notebooks.
This library has been used in many experiments including:
- the control of a 2D simulated arm,
- the exploration of the inverse kinematics of a poppy humanoid (both on the real robot and on the simulated version),
- acoustic model of a vocal tract.
Explauto is crossed-platform and has been tested on Linux, Windows and Mac OS. It has been released under the GPLv3 license.
-
URL:
https://
github. com/ flowersteam/ explauto - Contacts: Clément Moulin-Frier, Pierre Rouanet, Sebastien Forestier
7.1.2 KidBreath
- Keyword: Machine learning
- Functional Description: KidBreath is a web responsive application composed by several interactive contents linked to asthma and displayed to different forms: learning activities with quiz, short games and videos. There are profil creation and personalization, and a part which describes historic and scoring of learning activities, to see evolution of Kidreath use. To test Kidlearn algorithm, it is iadapted and integrated on this platform. Development in PHP, HTML-5, CSS, MySQL, JQuery, Javascript. Hosting in APACHE, LINUX, PHP 5.5, MySQL, OVH.
- Contacts: Pierre-Yves Oudeyer, Manuel Lopes, Alexandra Delmas
- Partner: ItWell SAS
7.1.3 Kidlearn: money game application
-
Functional Description:
The games is instantiated in a browser environment where students are proposed exercises in the form of money/token games (see Figure 2). For an exercise type, one object is presented with a given tagged price and the learner has to choose which combination of bank notes, coins or abstract tokens need to be taken from the wallet to buy the object, with various constraints depending on exercises parameters. The games have been developed using web technologies, HTML5, javascript and Django.
Figure 2: Four principal regions are defined in the graphical interface. The first is the wallet location where users can pick and drag the money items and drop them on the repository location to compose the correct price. The object and the price are present in the object location. Four different types of exercises exist: M : customer/one object, R : merchant/one object, MM : customer/two objects, RM : merchant/two objects. -
URL:
https://
flowers. inria. fr/ research/ kidlearn/ - Contact: Benjamin Clement
7.1.4 Kidlearn: script for Kidbreath use
- Keyword: PHP
- Functional Description: A new way to test Kidlearn algorithms is to use them on Kidbreath Plateform. The Kidbreath Plateform use apache/PHP server, so to facilitate the integration of our algorithm, a python script have been made to allow PHP code to use easily the python library already made which include our algorithms.
-
URL:
https://
flowers. inria. fr/ research/ kidlearn/ - Contact: Benjamin Clement
7.1.5 KidLearn
- Keyword: Automatic Learning
- Functional Description: KidLearn is a software which adaptively personalize sequences of learning activities to the particularities of each individual student. It aims at proposing to the student the right activity at the right time, maximizing concurrently his learning progress and its motivation.
-
URL:
https://
flowers. inria. fr/ research/ kidlearn/ - Authors: Benjamin Clement, Didier Roy, Pierre-Yves Oudeyer, Manuel Lopes
- Contacts: Benjamin Clement, Pierre-Yves Oudeyer
- Participants: Benjamin Clement, Didier Roy, Manuel Lopes, Pierre Yves Oudeyer
7.1.6 Poppy
- Keywords: Robotics, Education
-
Functional Description:
The Poppy Project team develops open-source 3D printed robots platforms based on robust, flexible, easy-to-use and reproduce hardware and software. In particular, the use of 3D printing and rapid prototyping technologies is a central aspect of this project, and makes it easy and fast not only to reproduce the platform, but also to explore morphological variants. Poppy targets three domains of use: science, education and art.
In the Poppy project we are working on the Poppy System which is a new modular and open-source robotic architecture. It is designed to help people create and build custom robots. It permits, in a similar approach as Lego, building robots or smart objects using standardized elements.
Poppy System is a unified system in which essential robotic components (actuators, sensors...) are independent modules connected with other modules through standardized interfaces:
- Unified mechanical interfaces, simplifying the assembly process and the design of 3D printable parts.
- Unified communication between elements using the same connector and bus for each module.
- Unified software, making it easy to program each module independently.
Our ambition is to create an ecosystem around this system so communities can develop custom modules, following the Poppy System standards, which can be compatible with all other Poppy robots.
-
URL:
https://
www. poppy-project. org/ - Contacts: Matthieu Lapeyre, Pierre Rouanet, Pierre-Yves Oudeyer, Didier Roy, Stephanie Noirpoudre, Theo Segonds, Damien Caselli, Nicolas Rabault
- Participants: Jonathan Grizou, Matthieu Lapeyre, Pierre Rouanet, Pierre-Yves Oudeyer
7.1.7 Poppy Ergo Jr
- Name: Poppy Ergo Jr
- Keywords: Robotics, Education
-
Functional Description:
Poppy Ergo Jr is an open hardware robot developed by the Poppy Project to explore the use of robots in classrooms for learning robotic and computer science.
It is available as a 6 or 4 degrees of freedom arm designed to be both expressive and low-cost. This is achieved by the use of FDM 3D printing and low cost Robotis XL-320 actuators. A Raspberry Pi camera is attached to the robot so it can detect object, faces or QR codes.
The Ergo Jr is controlled by the Pypot library and runs on a Raspberry pi 2 or 3 board. Communication between the Raspberry Pi and the actuators is made possible by the Pixl board we have designed.
Figure 3: Poppy Ergo Jr, 6-DoFs arm robot for education The Poppy Ergo Jr robot has several 3D printed tools extending its capabilities. There are currently the lampshade, the gripper and a pen holder.
Figure 4: The available Ergo Jr tools: a pen holder, a lampshade and a gripper With the release of a new Raspberry Pi board early 2016, the Poppy Ergo Jr disk image was updated to support Raspberry Pi 2 and 3 boards. The disk image can be used seamlessly with a board or the other.
-
URL:
https://
github. com/ poppy-project/ poppy-ergo-jr - Contacts: Theo Segonds, Damien Caselli
7.1.8 S-RL Toolbox
- Name: Reinforcement Learning (RL) and State Representation Learning (SRL) for Robotics
- Keywords: Machine learning, Robotics
- Functional Description: This repository was made to evaluate State Representation Learning methods using Reinforcement Learning. It integrates (automatic logging, plotting, saving, loading of trained agent) various RL algorithms (PPO, A2C, ARS, ACKTR, DDPG, DQN, ACER, CMA-ES, SAC, TRPO) along with different SRL methods (see SRL Repo) in an efficient way (1 Million steps in 1 Hour with 8-core cpu and 1 Titan X GPU).
-
URL:
https://
github. com/ araffin/ robotics-rl-srl - Contact: David Filliat
- Partner: ENSTA
7.1.9 Deep-Explauto
- Name: Deep-Explauto
- Keywords: Deep learning, Unsupervised learning, Learning, Experimentation
-
Functional Description:
Until recently, curiosity driven exploration algorithms were based on classic learning algorithms, unable to handle large dimensional problems (see explauto). Recent advances in the field of deep learning offer new algorithms able to handle such situations.
Deep explauto is an experimental library, containing reference implementations of curiosity driven exploration algorithms. Given the experimental aspect of exploration algorithms, and the low maturity of the libraries and algorithms using deep learning, proposing black-box implementations of those algorithms, enabling a blind use of those, seem unrealistic.
Nevertheless, in order to quickly launch new experiments, this library offers an set of objects, functions and examples, allowing to kickstart new experiments.
- Contact: Alexandre Pere
7.1.10 Orchestra
- Name: Orchestra
- Keyword: Experimental mechanics
-
Functional Description:
Ochestra is a set of tools meant to help in performing experimental campaigns in computer science. It provides you with simple tools to:
+ Organize a manual experimental workflow, leveraging git and lfs through a simple interface. + Collaborate with other peoples on a single experimental campaign. + Execute pieces of code on remote hosts such as clusters or clouds, in one line. + Automate the execution of batches of experiments and the presentation of the results through a clean web ui.
A lot of advanced tools exists on the net to handle similar situations. Most of them target very complicated workflows, e.g. DAGs of tasks. Those tools are very powerful but lack the simplicity needed by newcomers. Here, we propose a limited but very simple tool to handle one of the most common situation of experimental campaigns: the repeated execution of an experiment on variations of parameters.
In particular, we include three tools: + expegit: a tool to organize your experimental campaign results in a git repository using git-lfs (large file storage). + runaway: a tool to execute code on distant hosts parameterized with easy to use file templates. + orchestra: a tool to automate the use of the two previous tools on large campaigns.
- Contact: Alexandre Pere
7.1.11 Curious
- Name: Curious: Intrinsically Motivated Modular Multi-Goal Reinforcement Learning
- Keywords: Exploration, Reinforcement learning, Artificial intelligence
- Functional Description: This is an algorithm enabling to learn a controller for an agent in a modular multi-goal environment. In these types of environments, the agent faces multiple goals classified in different types (e.g. reaching goals, grasping goals for a manipulation robot).
- Contact: Cedric Colas
7.1.12 teachDeepRL
- Name: Teacher algorithms for curriculum learning of Deep RL in continuously parameterized environments
- Keywords: Machine learning, Git
-
Functional Description:
Codebase from our CoRL2019 paper https://arxiv.org/abs/1910.07224
This github repository provides implementations for the following teacher algorithms: - Absolute Learning Progress-Gaussian Mixture Model (ALP-GMM), our proposed teacher algorithm - Robust Intelligent Adaptive Curiosity (RIAC), from Baranes and Oudeyer, R-IAC: robust intrinsically motivated exploration and active learning. - Covar-GMM, from Moulin-Frier et al., Self-organization of early vocal development in infants and machines: The role of intrinsic motivation.
-
URL:
https://
github. com/ flowersteam/ teachDeepRL - Author: Remy Portelas
- Contact: Remy Portelas
7.1.13 Automated Discovery of Lenia Patterns
- Keywords: Exploration, Cellular automaton, Deep learning, Unsupervised learning
- Scientific Description: In many complex dynamical systems, artificial or natural, one can observe selforganization of patterns emerging from local rules. Cellular automata, like the Game of Life (GOL), have been widely used as abstract models enabling the study of various aspects of self-organization and morphogenesis, such as the emergence of spatially localized patterns. However, findings of self-organized patterns in such models have so far relied on manual tuning of parameters and initial states, and on the human eye to identify “interesting” patterns. In this paper, we formulate the problem of automated discovery of diverse self-organized patterns in such high-dimensional complex dynamical systems, as well as a framework for experimentation and evaluation. Using a continuous GOL as a testbed, we show that recent intrinsically-motivated machine learning algorithms (POP-IMGEPs), initially developed for learning of inverse models in robotics, can be transposed and used in this novel application area. These algorithms combine intrinsically motivated goal exploration and unsupervised learning of goal space representations. Goal space representations describe the “interesting” features of patterns for which diverse variations should be discovered. In particular, we compare various approaches to define and learn goal space representations from the perspective of discovering diverse spatially localized patterns. Moreover, we introduce an extension of a state-of-the-art POP-IMGEP algorithm which incrementally learns a goal representation using a deep auto-encoder, and the use of CPPN primitives for generating initialization parameters. We show that it is more efficient than several baselines and equally efficient as a system pre-trained on a hand-made database of patterns identified by human experts.
- Functional Description: Python source code of experiments and data analysis for the paper " Intrinsically Motivated Discovery of Diverse Patterns in Self-Organizing Systems" (Chris Reinke, Mayalen Echeverry, Pierre-Yves Oudeyer in Submitted to ICLR 2020). The software includes: Lenia environment, exploration algorithms (IMGEPs, random search), deep learning algorithms for unsupervised learning of goal spaces, tools and configurations to run experiments, and data analysis tools.
-
URL:
https://
github. com/ flowersteam/ automated_discovery_of_lenia_patterns - Contacts: Chris Reinke, Mayalen Etcheverry
7.1.14 ZPDES_ts
- Name: ZPDES in typescript
- Keywords: Machine learning, Education
- Functional Description: ZPDES is a machine learning-based algorithm that allows you to customize the content of training courses for each learner's level. It has already been implemented in the Kidlern software in python with other algorithms. Here, ZPDES is implemented in typescript.
-
URL:
https://
flowers. inria. fr/ research/ kidlearn/ - Authors: Benjamin Clement, Pierre-Yves Oudeyer, Didier Roy, Manuel Lopes
- Contact: Benjamin Clement
7.1.15 GEP-PG
- Name: Goal Exploration Process - Policy Gradient
- Keywords: Machine learning, Deep learning
- Functional Description: Reinforcement Learning algorithm working with OpenAI Gym environments. A first phase implements exploration using a Goal Exploration Process (GEP). Samples collected during exploration are then transferred to the memory of a deep reinforcement learning algorithm (deep deterministic policy gradient or DDPG). DDPG then starts learning from a pre-initialized memory so as to maximize the sum of discounted rewards given by the environment.
- Contact: Cedric Colas
7.1.16 EpidemiOptim
- Name: EpidemiOptim: a toolbox for the optimization of control policies in epidemiological models
- Keywords: Epidemiology, Optimization, Dynamical system, Reinforcement learning, Multi-objective optimisation
- Functional Description: This toolbox proposes a modular set of tools to optimize intervention strategies in epidemiological models. The user can define or use a pre-coded epidemiological model to represent an epidemic. He/she can define a set of cost functions to define a particular optimization problem. Finally, given an optimization problem (epidemiological model and cost functions and action modalities), the user can define/reuse optimization algorithms to optimize intervention strategies that minimize the costs. Finally, the toolbox contains visualization and comparison tools. This allows to investigate various hypotheses easily.
-
URL:
https://
github. com/ flowersteam/ EpidemiOptim - Contacts: Cedric Colas, Clément Moulin-Frier, Melanie Prague
7.1.17 IMAGINE
- Keywords: Exploration, Reinforcement learning, Modeling language, Artificial intelligence
- Functional Description: This software provides: 1. An environment modelling the social interaction between an autonomous agent and a social partner. The social partner gives natural language descriptions when the agent performs something interesting in the environment. 2. A modular architecture allowing the autonomous agent to manipulate and to target goals expressed in natural language. This architecture is divided into three modules: 2.a. A goal achievement function mapping language descriptions and the agent's observations to a reward signal 2.b. A goal conditioned-policy that uses the reward signal in order to learn the behaviour required to reach the goal (expressed in natural language). This module is trained via Reinforcement Learning 2.c. A goal imagination module allowing the agent to compose known goals into new sentences in order to creatively explore new outcomes in its environment.
-
URL:
https://
github. com/ flowersteam/ Imagine - Contacts: Tristan Karch, Cedric Colas, Clément Moulin-Frier, Pierre-Yves Oudeyer
7.1.18 DECSTR
- Name: Grouding Language to Autonomously-Acquired Skills via Goal Generation
- Keywords: Reinforcement learning, Curiosity, Intrinsic motivations
- Functional Description: DECSTR is a learning algorithm that trains an agent to reach semantic goals made of predicates characterizing spatial relations between pairs of blocks. After this first skill learning phase, the agent trains a language generation module that converts linguistic inputs into semantic goals. This module enables efficient language grounding.
- Contact: Cedric Colas
7.1.19 holmes
- Name: IMGEP-HOLMES, an algorithm for meta-diversity search applied to the automated discovery of novel structures in complex dynamical systems
- Keywords: Exploration, Incremental learning, Unsupervised learning, Hierarchical architecture, Intrinsic motivations, Cellular automaton, Complexity
- Functional Description: Python source code to reproduce the experiments and data analysis for the paper "Hierarchically Organized Latent Modules for Exploratory Search in Morphogenetic Systems" (Mayalen Echeverry, Clément Moulin-Frier and Pierre-Yves Oudeyer, published at NeurIPS 2020). The user can define a complex system he would like to explore, or use the Lenia environment which is already provided. He/she can select an explorer to explore this system (Random or IMGEP explorer). For the IMGEP explorer, many variants of goal space representations are provided in the source code: hand-defined descriptors of the Lenia system, unsupervisedly learned descriptors that can be trained online during the course of exploration (VAE variants and Contrastive Learning variants) and the hierarchical progressively-learned architecture presented in the paper (HOLMES). To this purpose, the software includes tools and configurations to run experiments and for data analysis and comparison of the results, as well as for running the scripts on super-computers (SLURM job manager).
-
URL:
https://
github. com/ flowersteam/ holmes - Contact: Mayalen Etcheverry
7.1.20 metaACL
- Name: Meta Automatic Curriculum Learning
- Keywords: Machine learning, Git
-
Functional Description:
Codebase from our arxiv paper https://arxiv.org/abs/2011.08463
This github repository provides implementations for AGAIN (Alp-Gmm and Inferred Progress Niches), our proposed Meta automatic curriculum learning teacher algorithm.
-
URL:
https://
github. com/ flowersteam/ meta-acl - Contact: Remy Portelas
7.1.21 EmComPartObs
- Name: Studying the joint role of partial observability and channel reliability in emergent communication
- Keywords: Multi-agent, Reinforcement learning, Emergent communication
- Functional Description: This source code contains a new grid-world environment where two agents interact to solve a task, Multi-Agent Reinforcement algorithms that solve that task, as well as plotting utilities.
-
URL:
https://
github. com/ UnrealLink/ emergent_communication - Publication: hal-03100681
- Contacts: Valentin Villecroze, Clément Moulin-Frier
7.1.22 grimgep
- Name: GRIMGEP: Learning Progress for Robust Goal Sampling in Visual Deep Reinforcement Learning
- Keywords: Machine learning, Reinforcement learning, Artificial intelligence, Exploration, Intrinsic motivations, Git, Deep learning
- Functional Description: Source code for the GRIMGEP paper (https://arxiv.org/abs/2008.04388) Contains: - Implementation of the GRIMGEP framework on top of three different underlying imgeps (Skew-fit, CountBased, OnlineRIG). - image-based 2D environment (PlaygroundRGB)
-
URL:
https://
gitlab. com/ Grg/ grimgep - Contacts: Grgur Kovac, Adrien Laversanne-Finot, Pierre-Yves Oudeyer
7.1.23 flowers-OL
- Name: flowers-open-lab
- Keyword: Experimentation
- Functional Description: This web platform designed for planning and implementing remote behavioural studies provides the following features: - Registration and login of participants - Presentation of the instructions concerning the experience and get informed consent - Behavioural task and questionnaires - Automatic management of a participant's schedule (sends emails before the user's appointments) - Quick and easy addition of new experimental conditions
-
URL:
https://
flowers-mot. bordeaux. inria. fr/ - Contacts: Alexandr Ten, Maxime Adolphe
8 New results
8.1 Computational Models of Curiosity-Driven Learning in Humans
8.1.1 Testing the Learning Progres Hypothesis in Curiosity-Driven explortion in Human Adults
Participants: Pierre-Yves Oudeyer, Alexandr Ten.
This project involves a collaboration between the Flowers team and the Cognitive Neuroscience Lab of J. Gottlieb at Columbia Univ. (NY, US), on the understanding and computational modeling of mechanisms of curiosity, attention and active intrinsically motivated exploration in humans.
It is organized around the study of the hypothesis that subjective meta-cognitive evaluation of information gain (or control gain or learning progress) could generate intrinsic reward in the brain (living or artificial), driving attention and exploration independently from material rewards, and allowing for autonomous lifelong acquisition of open repertoires of skills. The project combines expertise about attention and exploration in the brain and a strong methodological framework for conducting experimentations with monkeys, human adults and children together with computational modeling of curiosity/intrinsic motivation and learning.
Such a collaboration paves the way towards a central objective, which is now a central strategic objective of the Flowers team: designing and conducting experiments in animals and humans informed by computational/mathematical theories of information seeking, and allowing to test the predictions of these computational theories.
Context
Curiosity can be understood as a family of mechanisms that evolved to allow agents to maximize their knowledge (or their control) of the useful properties of the world - i.e., the regularities that exist in the world - using active, targeted investigations. In other words, we view curiosity as a decision process that maximizes learning/competence progress (rather than minimizing uncertainty) and assigns value ("interest") to competing tasks based on their epistemic qualities - i.e., their estimated potential allow discovery and learning about the structure of the world.
Because a curiosity-based system acts in conditions of extreme uncertainty (when the distributions of events may be entirely unknown) there is in general no optimal solution to the question of which exploratory action to take 115, 133, 141. Therefore we hypothesize that, rather than using a single optimization process as it has been the case in most previous theoretical work 95, curiosity is comprised of a family of mechanisms that include simple heuristics related to novelty/surprise and measures of learning progress over longer time scales 13071, 122. These different components are related to the subject's epistemic state (knowledge and beliefs) and may be integrated with fluctuating weights that vary according to the task context. Our aim is to quantitatively characterize this dynamic, multi-dimensional system in a computational framework based on models of intrinsically motivated exploration and learning.
Because of its reliance on epistemic currencies, curiosity is also very likely to be sensitive to individual differences in personality and cognitive functions. Humans show well-documented individual differences in curiosity and exploratory drives 113, 140, and rats show individual variation in learning styles and novelty seeking behaviors 90, but the basis of these differences is not understood. We postulate that an important component of this variation is related to differences in working memory capacity and executive control which, by affecting the encoding and retention of information, will impact the individual's assessment of learning, novelty and surprise and ultimately, the value they place on these factors 135, 149, 66, 153. To start understanding these relationships, about which nothing is known, we will search for correlations between curiosity and measures of working memory and executive control in the population of children we test in our tasks, analyzed from the point of view of a computational models of the underlying mechanisms.
A final premise guiding our research is that essential elements of curiosity are shared by humans and non-human primates. Human beings have a superior capacity for abstract reasoning and building causal models, which is a prerequisite for sophisticated forms of curiosity such as scientific research. However, if the task is adequately simplified, essential elements of curiosity are also found in monkeys 113, 107 and, with adequate characterization, this species can become a useful model system for understanding the neurophysiological mechanisms.
Objectives
Our studies have several highly innovative aspects, both with respect to curiosity and to the traditional research field of each member team.
- Linking curiosity with quantitative theories of learning and decision making: While existing investigations examined curiosity in qualitative, descriptive terms, here we propose a novel approach that integrates quantitative behavioral and neuronal measures with computationally defined theories of learning and decision making.
- Linking curiosity in children and monkeys: While existing investigations examined curiosity in humans, here we propose a novel line of research that coordinates its study in humans and non-human primates. This will address key open questions about differences in curiosity between species, and allow access to its cellular mechanisms.
- Neurophysiology of intrinsic motivation: Whereas virtually all the animal studies of learning and decision making focus on operant tasks (where behavior is shaped by experimenter-determined primary rewards) our studies are among the very first to examine behaviors that are intrinsically motivated by the animals' own learning, beliefs or expectations.
- Neurophysiology of learning and attention: While multiple experiments have explored the single-neuron basis of visual attention in monkeys, all of these studies focused on vision and eye movement control. Our studies are the first to examine the links between attention and learning, which are recognized in psychophysical studies but have been neglected in physiological investigations.
- Computer science: biological basis for artificial exploration: While computer science has proposed and tested many algorithms that can guide intrinsically motivated exploration, our studies are the first to test the biological plausibility of these algorithms.
- Developmental psychology: linking curiosity with development: While it has long been appreciated that children learn selectively from some sources but not others, there has been no systematic investigation of the factors that engender curiosity, or how they depend on cognitive traits.
Results
We provide empirical evidence that humans are sensitive to variation learniing progress (LP) by means of a novel experimental paradigm and computational modeling. We showed that while humans rely on competence information to avoid easy tasks, models that include an LP component provide the best fit to task selection data. These results provide a new bridge between research on artificial and biological curiosity, reveal strategies that are used by humans but have not been considered in computational research, and provide new tools for probing how humans become intrinsically motivated to learn and acquire interests and skills on extended time scales. The results were submitted to the journal Nature Communications and are currently under revision.
Participants () performed an online task in which they could freely engage with a set of learning activities (Fig. 5a). Each trial started with a free-choice panel prompting the participant to choose one of 4 activities depicted as families of “monsters” (Fig. 5a, (1)) and, after making a choice, received a randomly drawn member from the chosen family, made a binary guess about which food that member liked to eat (Fig. 5a, (2)), and received immediate feedback regarding their guess (Fig. 5a, (3)). To understand how participants self-organized their learning curriculum, we required them to complete 250 trials but did not impose any other constraint on their choice of activity.
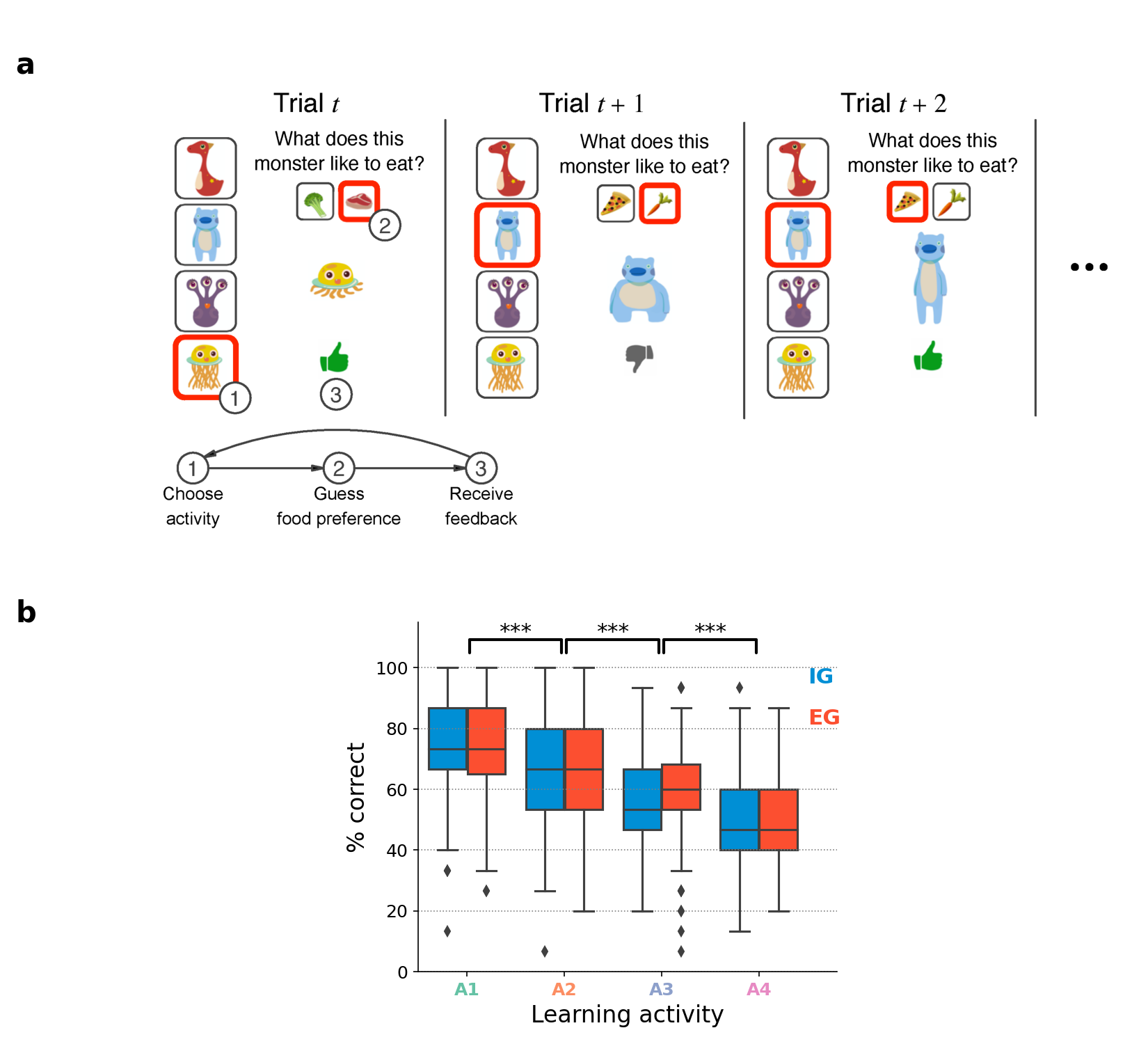
Our key questions were (1) how people self-organize their exploration over a set of activities of variable difficulty, and (2) whether they spontaneously adopt learning maximization objectives when they do not receive explicit instructions. To examine these questions, we manipulated the difficulty of the available activities as a within-participant variable, and the instructions participants received as an across-participant variable. Difficulty was controlled by the complexity of the categorization rule governing the food preferences in each activity. In the easiest activity (A1), individual monster-family members differed in only one feature and that feature governed their food preference (e.g., a short octopus liked steak and a tall octopus liked broccoli; 1-dimensional categorization). In the next easiest level (A2), family members varied along two features but only one feature determined preference (1-dimensional with an irrelevant feature). In the most difficult learnable activity (A3) food preferences were determined by a conjunction of 2 variable features (2-dimensional categorization). Finally, the 4th activity (A4) was random and unlearnable: individual monsters had two variable features, but their food preferences were assigned randomly each time a new monster was sampled.
Learning objectives were manipulated across two randomly selected participant groups. Participants in the “external goal” group (EG; ) were asked to maximize learning across all the activities and were told that they will be tested at the end of the session. In contrast, participants in the “internal goal” group (IG, ) were told to choose any activity they wished with no constraint except for completing 250 trials. Except for this difference in instructions (and the fact that the EG group received the announced test), the two groups received identical treatments. Each group started with 15 forced-choice familiarization trials on each activity, followed by a 250-trial free-play stage, and gave several subjective ratings of the activities before and after the free play stage.
As shown in Fig. 5, our difficulty manipulation worked as expected in both groups. Average accuracy on the more difficult activites was lower than on easier activities.
To investigate whether LP played a role in self-determined study curriculum we fit the participants’ activity choices with a simple softmax choice model in which the utility of an activity was a linear combination of PC and LP:
PC and LP were dynamically evaluated for each activity at each trial based on the recent feedback history. PC was defined as the number of correct guesses over the last 15 trials of activity , and LP was defined as the difference in PC between first versus second parts of the same interval. We fit each participants’ data as a probabilistic (softmax) choice over 4 discrete classes, using maximum likelihood estimation with 3 free parameters - the softmax temperature (capturing choice stochasticity) and weights , indicating the extent to which each participant was sensitive to, respectively, PC and LP (REFERENCE NOT FOUND: FLOWERS-RA-2020/label/subsubsec:methods/analysis/modeling).
The bivariate form of the model that included both LP and PC (eq. 1) provided a superior fit to the data (average AIC score of ) relative to an alternative model based on random selection (). Importantly, the bivariate model also outperformed univariate models that included only LP or only PC terms in each instruction group (Fig. 6a). Moreover, the bivariate models had the lowest AIC scores in 75.63% of participants, and at least a 2 point lead from the next-best model in 63.13% (average minimum pairwise difference in AIC scores of , Wilcoxon signed-rank test for repeated measurements). This provides direct evidence that participants are sensitive to LP – a heuristic for the temporal derivative of PC – above and beyond overall error rates.
The fitted bivariate models were successful at qualitatively reproducing time-allocation patterns across learning activities and groups of participants (Fig. 6b). As shown in Fig. 6b, the fitted models captured the behavioral tendencies of individual subgroups in our data.
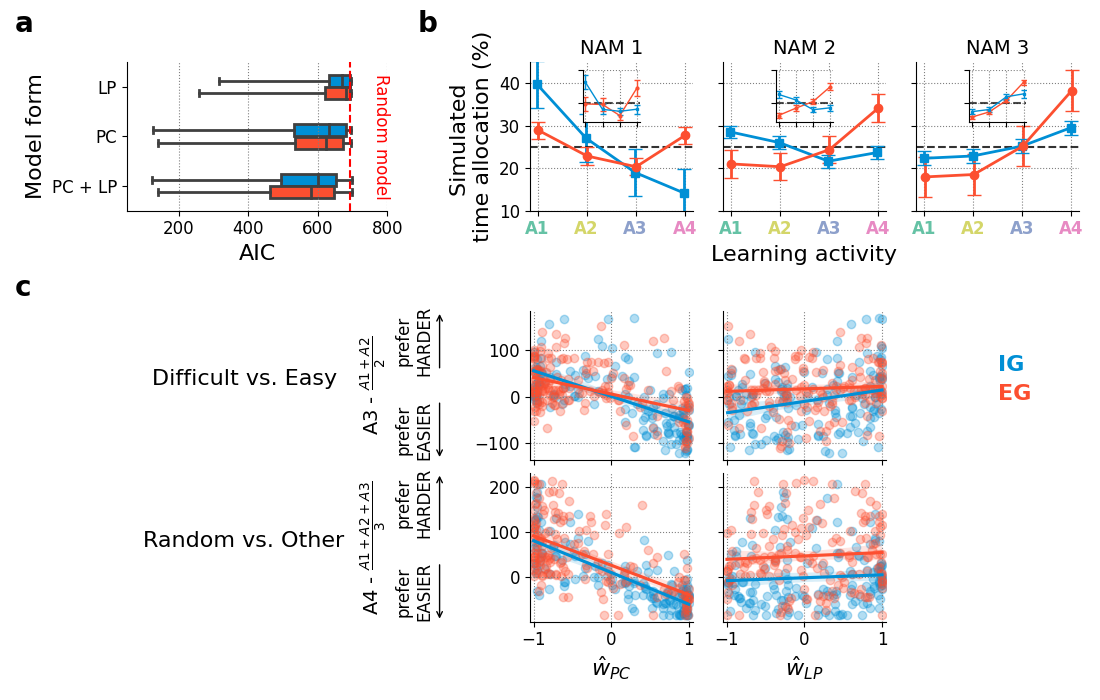
Next, we examined the fitted model coefficients from the bivariate models. The normalized coefficients coefficients were, on average, positive in the IG group and negative in the EG group (IG: ; EG: ; 1-way ANOVA; main effect of instruction, ) consistent with the fact that the EG group were relatively more attracted to challenging activities with higher error rates. In contrast, the coefficients did not differ by instruction (IG: ; 1-way ANOVA; main effect of instruction, ) suggesting that they captured a different aspect of the participants’ choices.
These differences are consistent with computational studies suggesting that sensitivities to PC and LP play distinct roles. A sensitivity to PC may motivate people to learn by steering them away from overly easy activities, while a sensitivity to LP may steer learners away from overly difficult or impossible activities. To examine this hypothesis, we analyzed how the two coefficients correlated with individual preferences for challenging over easier activities when the more challenging activity was, respectively, learnable or unlearnable. Figure 6 shows that while PC helped participant in engaging with more challening activities, LP seemed to have this effect only in the context of A3 vs A1, but not A4 vs A3, suggesting that LP does not lead people to the unlearnability trap as PC does.
8.1.2 Examining the effect of time on subjective judgments of learning dynamics
Participants: Alexandr Ten, Pierre-Yves Oudeyer, Hélène Sauzéon, Maxime Balan.
Our previous work left open the question of what is an optimal time period for which humans can accurately estimate their progress in learning a skill that requires a prolonged exposure or practice time to be mastered. Thus, this ongoing project aims to investigate veridical time scales for estimating progress in competence in humans. For this, we designed a behavioral sensorimotor task that requires some extended amount of time to be learned. The task is presented in the form of a video game, similar to the arcade game Lunar Lander, where the objective is to control a spacecraft and safely land it on the surface. As participants practice the task, we will record their performance (e.g. time to complete a single trial, success rate etc.), which will allow us to examine the accuracy of their subjective judgments about their performance. The subjective judgments of performance, along with different control measures, will be obtained via verbal questionnaires.
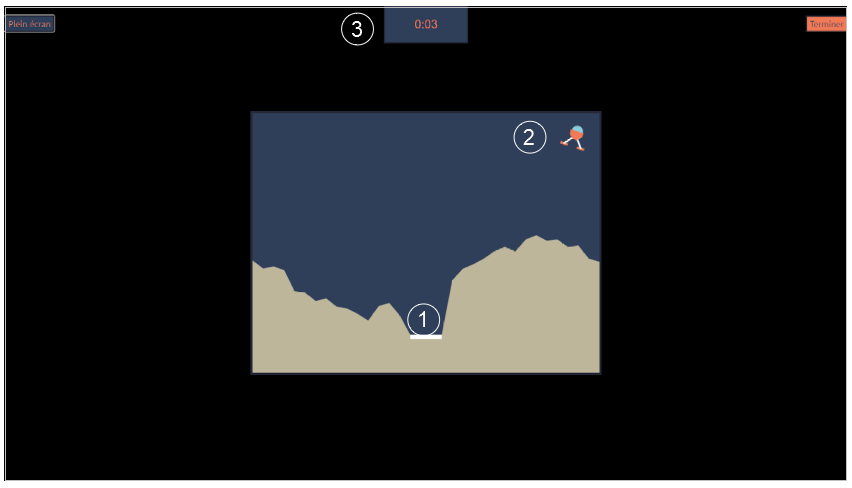
Crucially, we control the time frames in which we sollicit the participant's subjective judgments of learning in order examine the link between how much time has passed (5 minutes, 2 days, 5 days) and the accuracy of participants' beliefs about their ongoing progress. We designed a tool which can be used to administer this experiment remotely via the internet. Participants will be asked to log in and complete part of the task on 3 different days, each day consisting the same 3 phases: (1) 5 minute of practice (2) questionnaire (3) optional practice. The last, optional practice phase can be engaged by participants but is not required as part of the experiment. This will let us measure behaviorally, the level of interest / enthusiasm participants have for playing the game, which is hypothesized to be a function of the learning progress they experience.
8.2 Intrinsically Motivated Learning in Artificial Intelligence
Participants: Pierre-Yves Oudeyer, Olivier Sigaud, Cédric Colas, Adrien Laversanne-Finot, Rémy Portelas, Tristan Karch, Grgur Kovac.
8.2.1 Intrinsically Motivated Goal-Conditioned Reinforcement Learning: a Short Survey
Building autonomous machines that can explore open-ended environments, discover possible interactions and autonomously build repertoires of skills is a general objective of artificial intelligence. Developmental approaches argue that this can only be achieved by autonomous and intrinsically motivated learning agents that can generate, select and learn to solve their own problems. In recent years, we have seen a convergence of developmental approaches, and developmental robotics in particular, with deep reinforcement learning (RL) methods, forming the new domain of developmental machine learning. Within this new domain, we review here a set of methods where deep RL algorithms are trained to tackle the developmental robotics problem of the autonomous acquisition of open-ended repertoires of skills. Intrinsically motivated goal-conditioned RL algorithms train agents to learn to represent, generate and pursue their own goals. The self-generation of goals requires the learning of compact goal encodings as well as their associated goal-achievement functions, which results in new challenges compared to traditional RL algorithms designed to tackle pre-defined sets of goals using external reward signals. This project 58 proposes a typology of these methods at the intersection of deep RL and developmental approaches, surveys recent approaches and discusses future avenues.
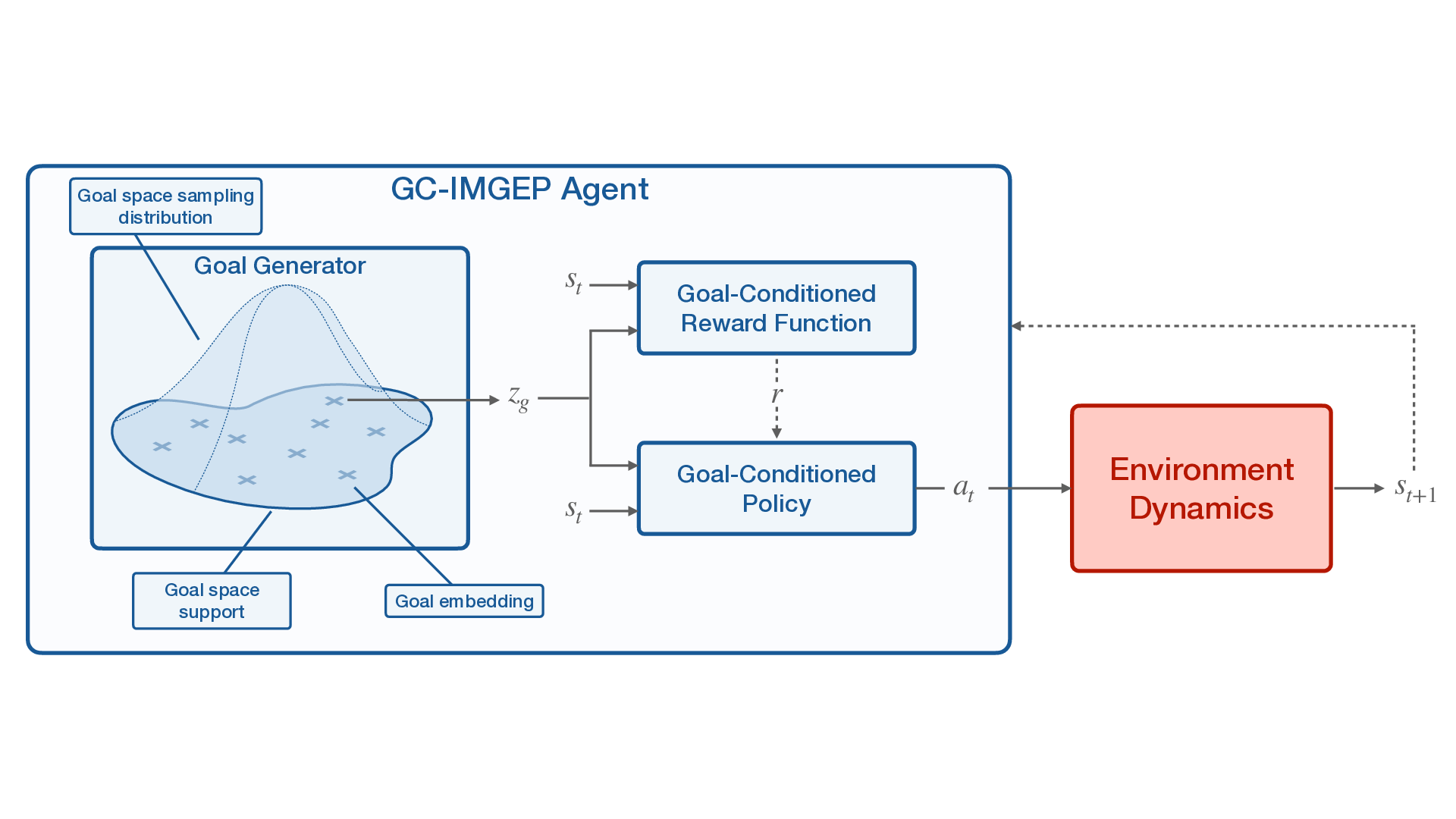
8.2.2 Intrinsically Motivated Exploration of Learned Goal Spaces
Participants: Adrien Laversanne-Finot, Pierre-Yves Oudeyer.
Finding algorithms that allow agents to discover a wide variety of skills efficiently and autonomously, remains a challenge of Artificial Intelligence. Intrinsically Motivated Goal Exploration Processes (IMGEPs) have been shown to enable real world robots to learn repertoires of policies producing a wide range of diverse effects. They work by enabling agents to autonomously sample goals that they then try to achieve. In practice, this strategy leads to an efficient exploration of complex environments with high-dimensional continuous actions. Until recently, it was necessary to provide the agents with an engineered goal space containing relevant features of the environment. In this article we show that the goal space can be learned using deep representation learning algorithms, effectively reducing the burden of designing goal spaces. Our results pave the way to autonomous learning agents that are able to autonomously build a representation of the world and use this representation to explore the world efficiently. We present experiments in two environments using population-based IMGEPs. The first experiments are performed on a simple, yet challenging, simulated environment. Then, another set of experiments tests the applicability of those principles on a real-world robotic setup, where a 6-joint robotic arm learns to manipulate a ball inside an arena, by choosing goals in a space learned from its past experience. This work was published in 28
8.2.3 GRIMGEP: Learning Progress for Robust Goal Sampling in Visual Deep Reinforcement Learning
Participants: Grgur Kovač, Adrien Laversanne-Finot, Pierre-Yves Oudeyer.
Autonomous agents using novelty based goal exploration are often efficient in environments that require exploration. However, they get attracted to various forms of distracting unlearnable regions. To solve this problem, Absolute Learning Progress (ALP) has been used in reinforcement learning agents with predefined goal features and access to expert knowledge. This work extends those concepts to unsupervised image-based goal exploration.
We present the GRIMGEP framework: it provides a learned robust goal sampling prior that can be used on top of current state-of-the-art novelty seeking goal exploration approaches, enabling them to ignore noisy distracting regions while searching for novelty in the learnable regions. It clusters the goal space and estimates ALP for each cluster. These ALP estimates can then be used to detect the distracting regions, and build a prior that enables further goal sampling mechanisms to ignore them.
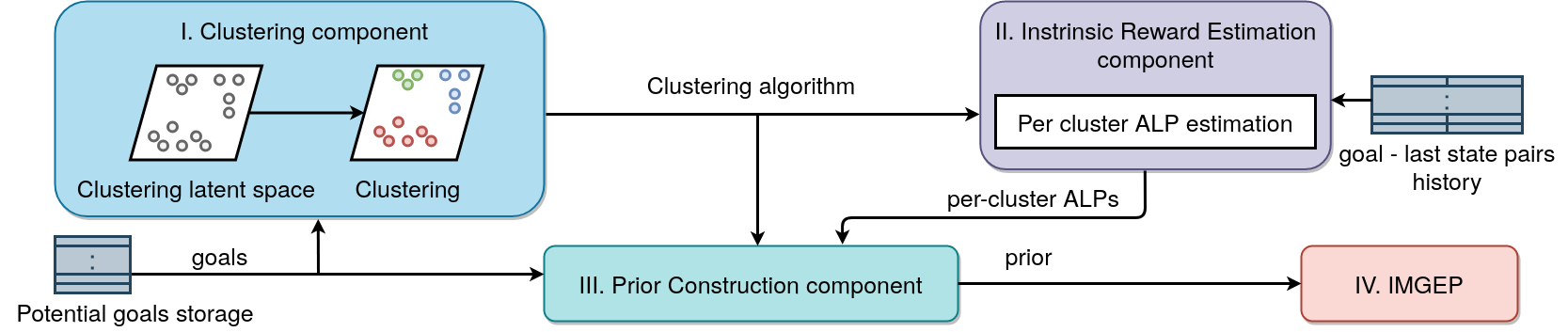
We construct an image based environment with distractors, on which we show that wrapping current state-of-the-art goal exploration algorithms with our framework allows them to concentrate on interesting regions of the environment and drastically improve performances.
This work is available as a preprint in 60 and the source code is available at https://
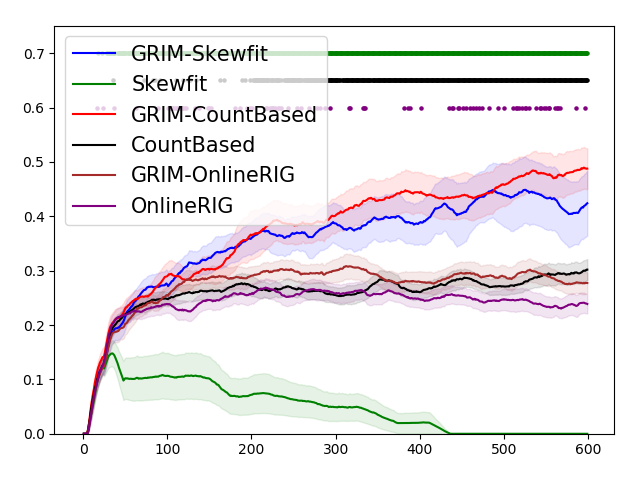
8.2.4 Language Augmented Intrinsically Motivated Agents
Participants: Cédric Colas, Tristan Karch, Pierre-Yves Oudeyer.
Language as a Cognitive Tool to Imagine Goals in Curiosity-Driven Exploration
In this project, we investigate how autonomous multi-goal reinforcement learning agents can use language as a cognitive tool in order to creatively explore their environment and grow repertoires of skills. We follow a developmental approach inspired by how children learn to manipulate language, using it as a way to represent goals and to make plans in their heads.
We develop an algorithm called IMAGINE 43 enabling an intrinsically motivated agent to build a repertoire of skills only from natural language descriptions given by a Social Partner. In our setup, the agent starts without knowing any potential goal and acts randomly. As it reaches outcomes that are meaningful for the social partner, the social partner provides descriptions of the scene in natural language. The agent then converts these natural descriptions into targetable goals and learns to reach them.
This new learning algorithm offers several benefits over previous intrinsically motivated multi-goal reinforcement learning agents that do not use language to describe goals.
First, using linguistic descriptions as sole supervision helps get rid of the need to define hand-crafted reward functions for each of the reachable goals in the environment. In curious, for instance, the agent needed to have access to the description of each of the goal types as well as their associated reward functions in order to reach them. In IMAGINE, the agent builds its own internal reward function mapping natural language descriptions to binary rewards and uses this signal to train a goal-conditioned policy.
Second, using language to represent goals enables the agent to leverage language compositionality so as to imagine new goals, assembling pieces of descriptions communicated by the social partner in order to form new targetable goals. For instance, consider an agent that received the following descriptions: “Grasp red cat”, “Grow red cat” and “Grasp red plant”. This agent can imagine the goal “Grow red plant” and use it as a target in order to discover new outcomes in its environment. We call this mechanism goal imagination. We argue that goal imagination is key to be able to make creative discoveries because the corresponding targeted behaviors are out of the distribution of the outcomes communicated by the social partner. This sort of out-of-distribution goal generation can only be achieved with goals represented as language.
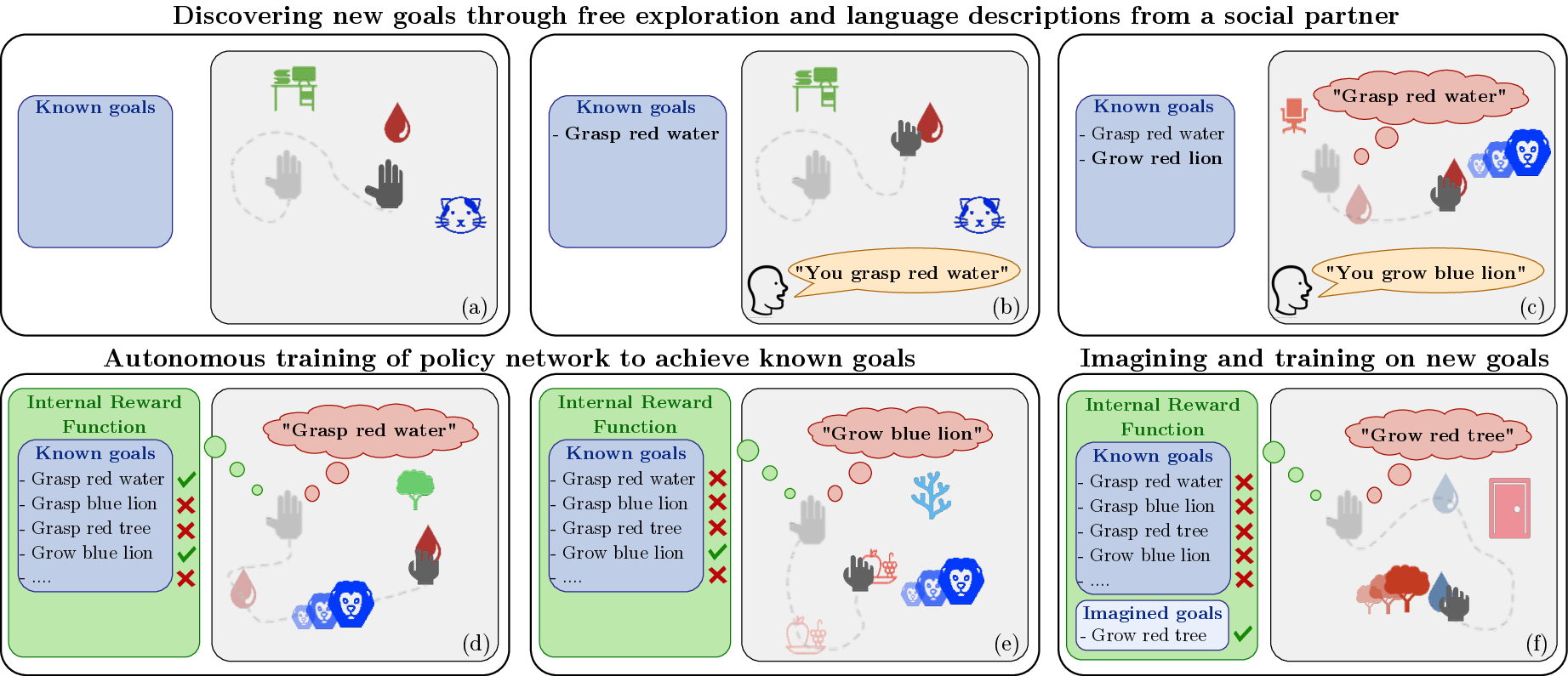
We carried out experiments in order to evaluate the benefits from goal imagination in intrinsically motivated learning. Experiments are split into two phases. In the first one, the agent interacts with the social partners, collects descriptions of goals and stores them in a set of known goal descriptions. The agent uses these descriptions paired with its observations in order to learn an internal reward function that detects when the goal represented by the descriptions are achieved in a given scene. Once this internal reward function is obtained, the agent uses its output (the reward signal) in order to train a goal-conditioned policy enabling it to reach any goal.
In the second phase, the social partner disappears and the agent starts imagining new goals by composing the descriptions stored in the set of known goals. The agent then targets these new goals and by doing so, discovers new interactions. This creative goal exploration process can only be efficient if imagined goal descriptions have a sufficient probability to be meaningful in the environment. As a result, we leveraged the construction grammar framework used to model child language acquisition with discovery of word equivalence classes in order to make sure that imagined goals follow the same construction rules as the descriptions communicated by the social partner. It is also important to note, that in order for goal imagination to work, the internal reward function trained from the social partner’s description must generalize. In other words, it should be able to detect if imagined goals are reached without receiving any new description from the social partner. To this end, we developed an object-factored learning architecture coupled with attention mechanisms 47 that facilitates generalization to new descriptions.
Finally, we measured the success rate of agents on a wide set of different skills and observed that agents that do not imagine goals (that stop at phase 1) master a smaller set of skills than agents that do imagine goals.
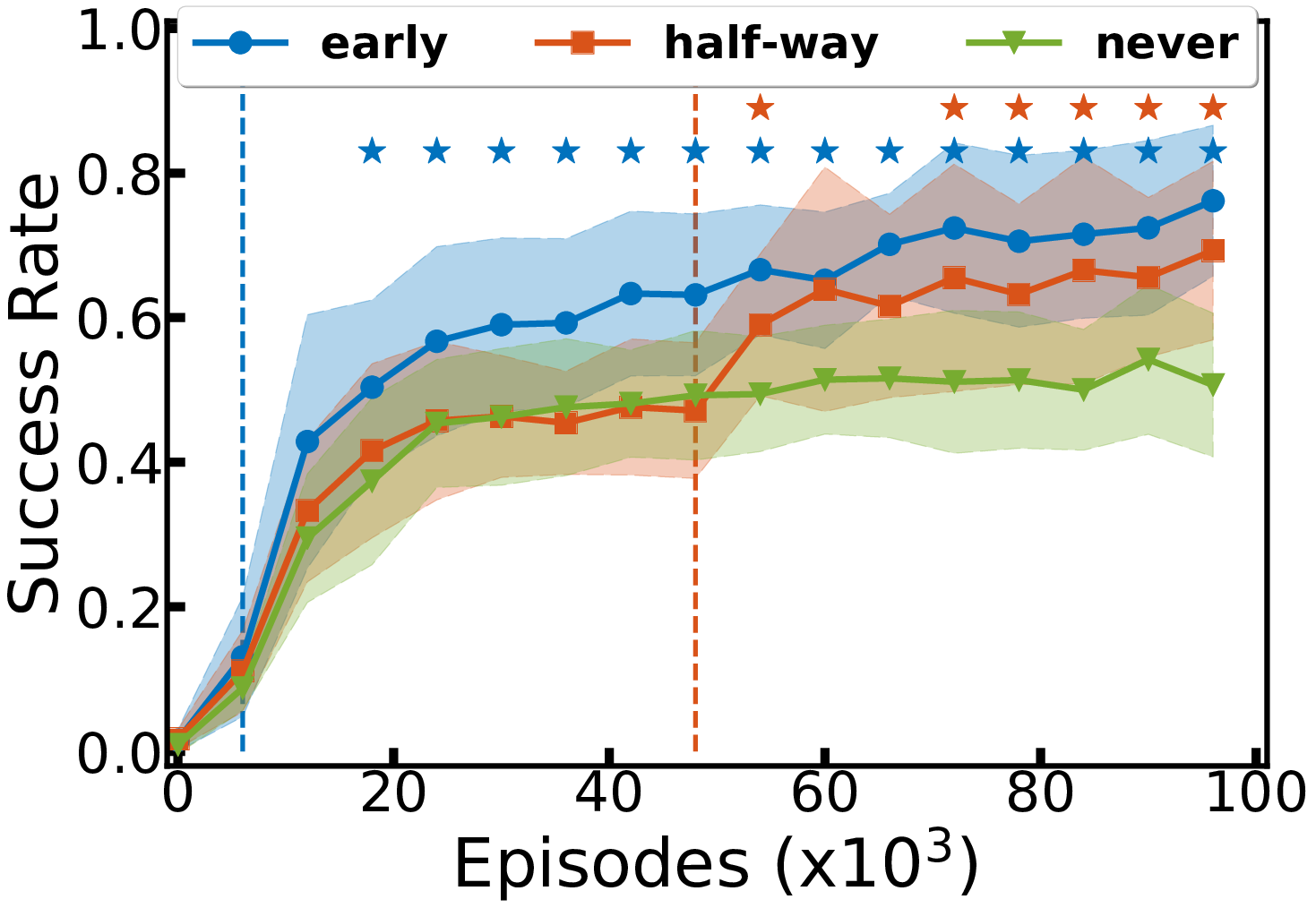
Grounding Language to Autonomously-Acquired Skills via Goal Generation
We are interested in the autonomous acquisition of repertoires of skills. Language-conditioned reinforcement learning (lc-rl) approaches are great tools in this quest, as they allow us to express abstract goals as sets of constraints on the states. However, most lc-rl agents are not autonomous and cannot learn without external instructions and feedback. Besides, their direct language condition cannot account for the goal-directed behavior of pre-verbal infants and strongly limits the expression of behavioral diversity for a given language input. To resolve these issues, we propose a new conceptual approach to language-conditioned rl: the Language-Goal-Behavior architecture (lgb). lgb decouples skill learning and language grounding via an intermediate semantic representation of the world—see Figure 13. To showcase the properties of lgb, we present a specific implementation called decstr. decstr is an intrinsically motivated learning agent endowed with an innate semantic representation describing spatial relations between physical objects–see Figure 14. In a first stage (gb), it freely explores its environment and targets self-generated semantic configurations. In a second stage (lg), it trains a language-conditioned goal generator to generate semantic goals that match the constraints expressed in language-based inputs. We showcase the additional properties of lgb w.r.t. both an end-to-end lc-rl approach and a similar approach leveraging non-semantic, continuous intermediate representations. Intermediate semantic representations help satisfy language commands in a diversity of ways, enable strategy switching after a failure and facilitate language grounding. This project led to a publication in the ICLR conference proceeding 56, 32.
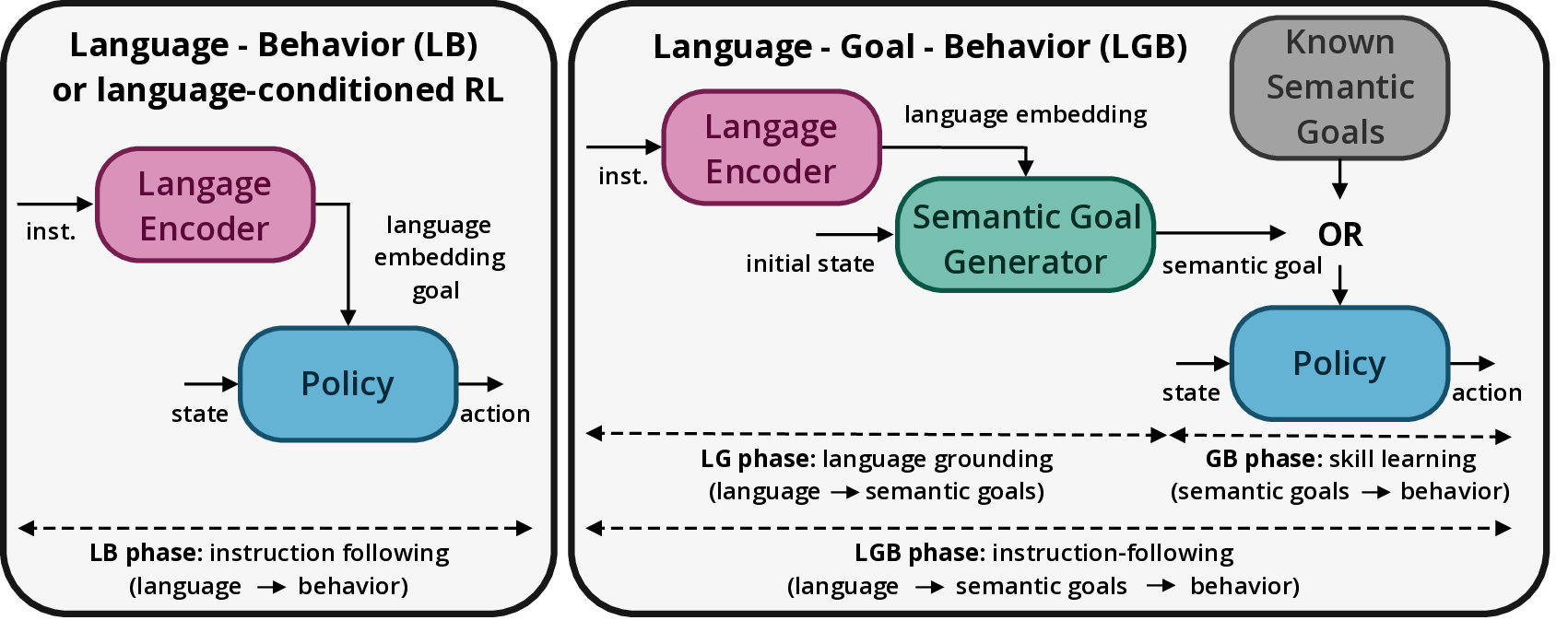
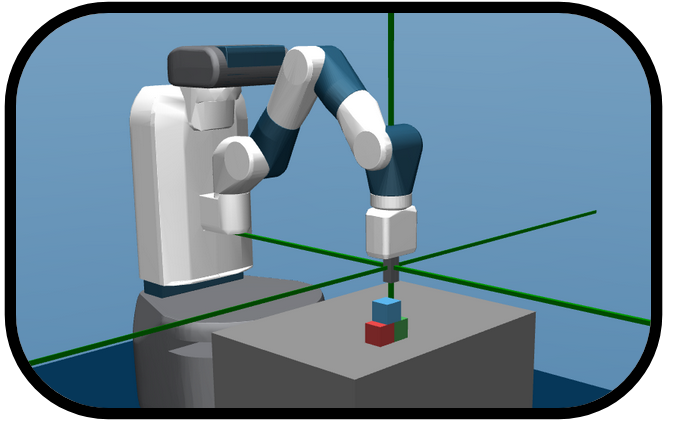
8.2.5 Intrinsically Motivated Open-Ended Multi-Task Learning Using Transfer Learning to Discover Task Hierarchy
Participants: Nicolas Duminy, Sao Mai Nguyen, Junshuai Zhu, Dominique Duhaut, Jerome Kerdreux.
In open-ended continuous environments, robots need to learn multiple parameterised control tasks in hierarchical reinforcement learning. We hypothesise that the most complex tasks can be learned more easily by transferring knowledge from simpler tasks, and faster by adapting the complexity of the actions to the task. We propose a task-oriented representation of complex actions, called procedures, to learn online task relationships and unbounded sequences of action primitives to control the different observables of the environment. Combining both goal-babbling with imitation learning, and active learning with transfer of knowledge based on intrinsic motivation, our algorithm self-organises its learning process. It chooses at any given time a task to focus on; and what, how, when and from whom to transfer knowledge. We show with a simulation and a real industrial robot arm, in cross-task and cross-learner transfer settings, that task composition is key to tackle highly complex tasks. Task decomposition is also efficiently transferred across different embodied learners and by active imitation, where the robot requests just a small amount of demonstrations and the adequate type of information. The robot learns and exploits task dependencies so as to learn tasks of every complexity.
This work lead to a publication in MDPI Applied Siences 45.
8.3 Object-Based and Relational Representations for Autonomous Agents
Participants: Laetitia Teodorescu, Tristan Karch, Cedric Colas, Katja Hoffman, Pierre-Yves Oudeyer.
In deep reinforcement learning, especially in approaches operating in symbolic observation spaces (the inputs are not images but the list of all object's x-y positions for instance), it is common to feed the agent's networks with a vector of the concatenation of all the symbolic features. However, in practice there is a lot of redundant structure in this observation space: if the first object has a feature describing it as "red" or if the second object has a feature describing it as "red", there should be a prior (or inductive bias) in the architecture reflecting the fact that these two situations should be processed in the same way. All objects share the same semantics no matter in what order they are listed. We can call this the object-centered prior. In addition to that, for acting on collections of objects, an agent often has to process information about the relations between objects. We can call this the relational prior (or inductive bias). A detailed discussion of these inductive biases can be found in 73.
8.3.1 Relational inductive biases for recognizing configurations of objects
Since the structure "objects + relations" is naturally present in the world, a good idea is to implement it into the neural networks we are training. Set structures can be used for representing collections of objects, and the Deep Set architecture is well-suited for learning on sets. Graph structures can be used for representing collections of objects and their relations; the Graph Neural Network (GNN) family is well-suited for learning on graphs. Additionnally, we should observe differences between performance and sample efficiency of architectures having only the object-centered prior versus the ones that have the object-centered and relational priors in tasks that require processing of relational information.
We have tested this hypothesis in the case of learning to recognize spatial configurations of symbolic objects. For this purpose, we have created a benchmark dataset called SpatialSim that defines two tasks. The first task, called Identification, is learning to recognize a reference configuration of objects (up to an affine transformation) from a scene with the same objects but with their positions randomly reshuffled. The second task, called Comparison, consists in comparing two different configurations of objects and deciding if they are the same (up to an affine transformation).
In this context, we have trained architectures implementing increasing levels of relational computation: Deep Sets, Recurrent Deep Sets and Message-Passing GNNs. We have observed that the models with more relational computation perform better, especially in the Comparison task where Deep Set performance is very poor. This suggests that relational models are crucial for learning to compare configurations of objects.
This work has been presented as a spotlight talk at the Bridge Between Perception and Reasoning, Graph Neural Networks and Beyond workshop at ICLR 2020 63.
8.3.2 Extracting object representations from images
The previous work was concerned with symbolic objects described by their features such as position, orientation, etc. In a realistic setting we need to be able to learn to extract these object representations directly from raw images in an unsupervised representation learning scheme, and in a disentangled manner, such that each object is represented by a unique vector, and that each of that vector's coordinates represents a unique factor of variation (such as x or y position, color, etc). In the best case, this would recover the symbolic representations such as the ones used in the approach above.
Two architectures for object-centered unsupervised representation learning have been investigated: MONet 79 (an object-based variational autoencoder) and Contrastive-Structured World Models 108 (an architecture learning to extract objects from images by learning a world model expressed as an interaction graph). Integrating these approaches (along with mechanisms for object permanence) into an intrinsically motivated deep RL setting is still ongoing work.
8.3.3 In language-conditioned Deep RL agents
The impact of object-centered architectures in a deep RL setting has also been investigated. We have benchmarked their importance in the language-imagination deep rl setting given in 8.2.4. We have observed dramatic improvements in sample efficiency in this setting when we use Deep Sets as opposed to flat, unstructured architectures (such as regular Multi-Layer Perceptrons).
In addition to that, we observe increased generalization performance in this setting (see Figures 15 and 16), suggesting that the bias that all objects should be represented and processed in the same way (and the weight-sharing that is implied by this bias in the neural networks) is helpful for transferring skills across objects.
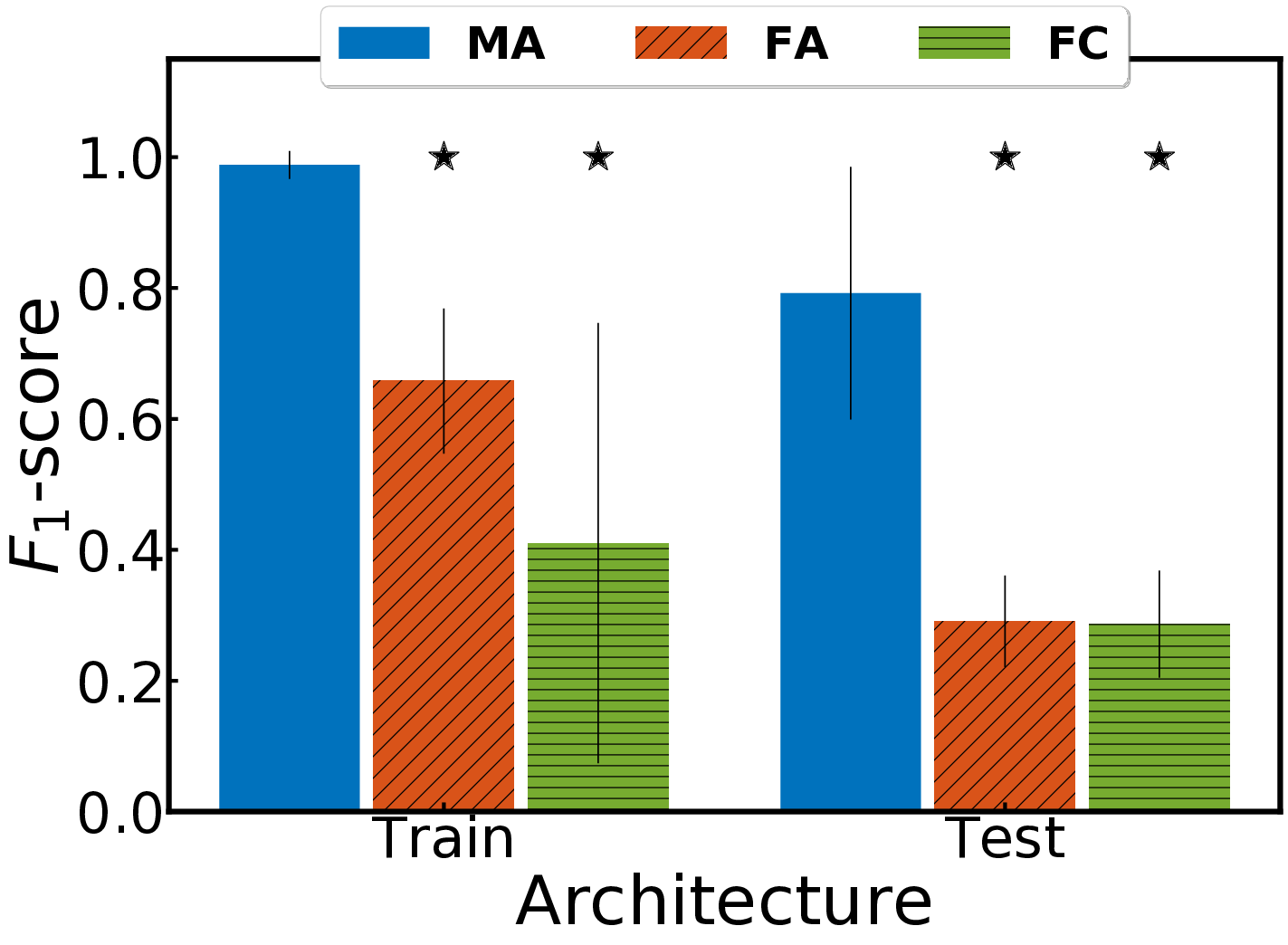
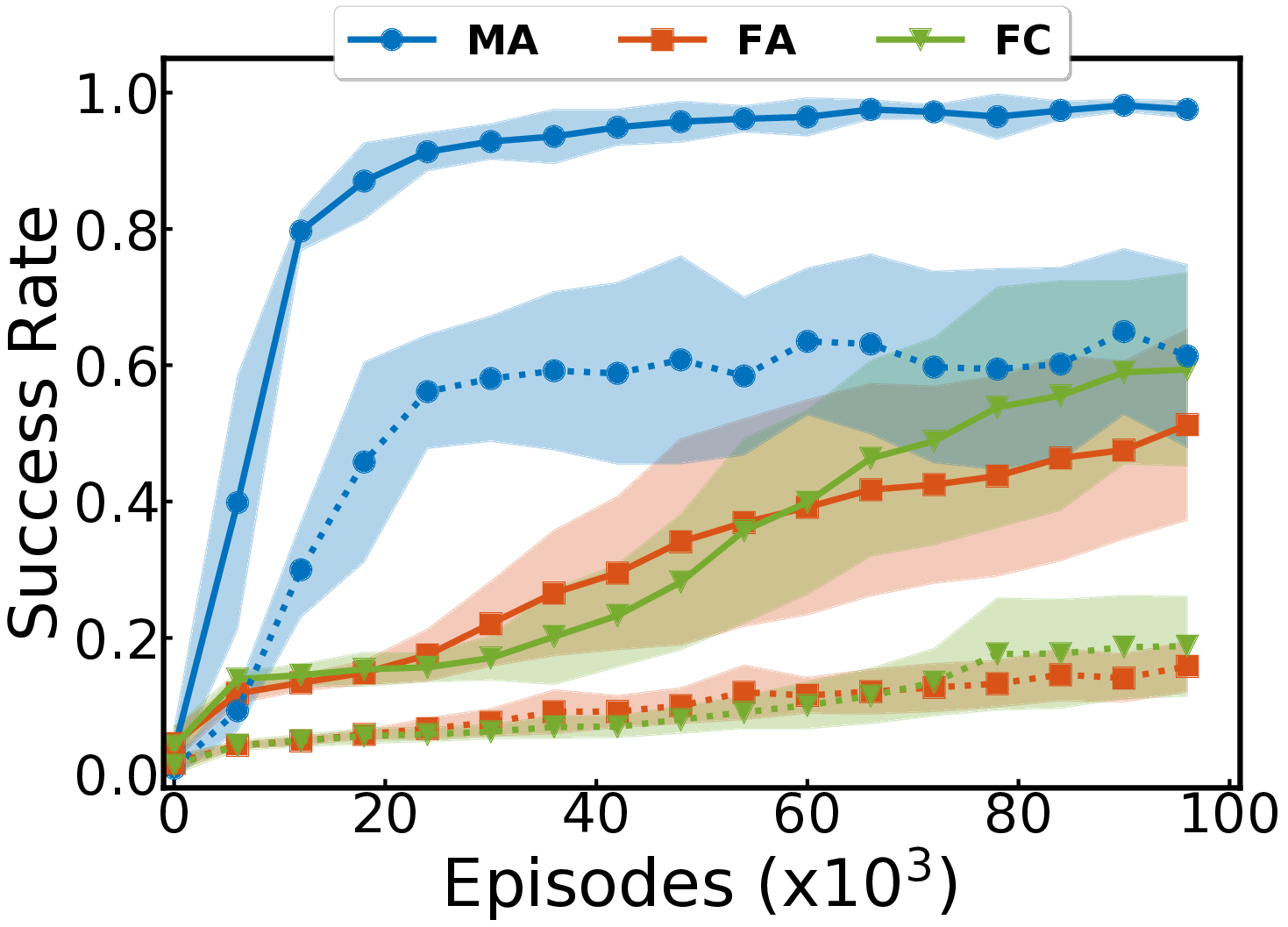
These object-based architectures are robust to the number of objects, contrary to their flat counterparts. Additionally, architectures that present biases for encoding relations between objects demonstrate increased performance in tasks that require interaction between objects, such as grasping objects that are identified by their position relative to another object.
This work was presented at the Beyond Tabula Rasa in RL ICLR 2020 workshop 47.
8.4 Automatic Curriculum Learning in Deep RL
8.4.1 Teacher algorithms for curriculum learning of Deep RL in continuously parameterized environments
Participants: Remy Portelas, Katja Hoffman, Pierre-Yves Oudeyer.
In this work we considered the problem of how a teacher algorithm can enable an unknown Deep Reinforcement Learning (DRL) student to become good at a skill over a wide range of diverse environments. To do so, we studied how a teacher algorithm can learn to generate a learning curriculum, whereby it sequentially samples parameters controlling a stochastic procedural generation of environments. Because it does not initially know the capacities of its student, a key challenge for the teacher is to discover which environments are easy, difficult or unlearnable, and in what order to propose them to maximize the efficiency of learning over the learnable ones. To achieve this, this problem is transformed into a surrogate continuous bandit problem where the teacher samples environments in order to maximize absolute learning progress of its student. We presented ALP-GMM (see figure 17), a new algorithm modeling absolute learning progress with Gaussian mixture models. We also adapted existing algorithms and provided a complete study in the context of DRL. Using parameterized variants of the BipedalWalker environment, we studied their efficiency to personalize a learning curriculum for different learners (embodiments), their robustness to the ratio of learnable/unlearnable environments, and their scalability to non-linear and high-dimensional parameter spaces. Videos and code are available at https://
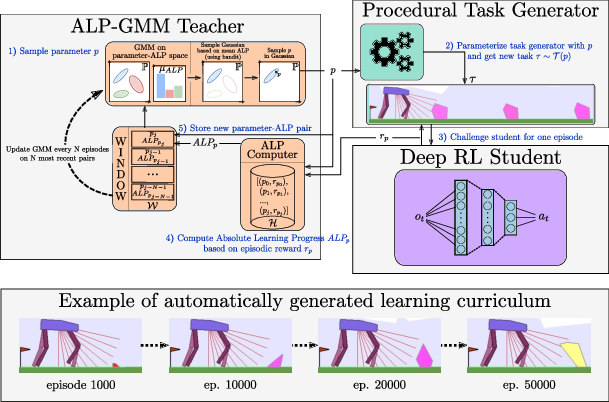
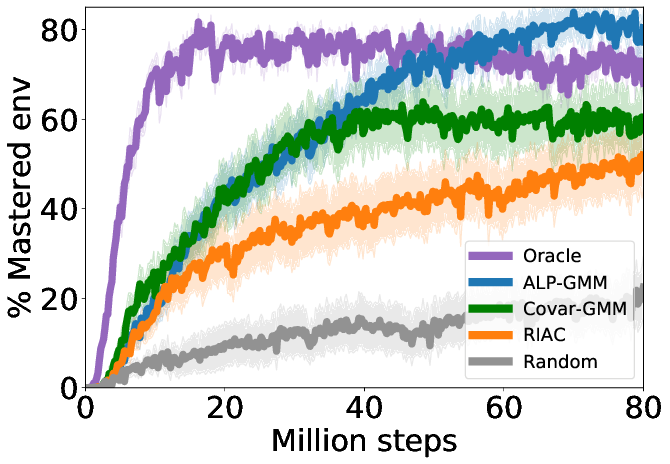
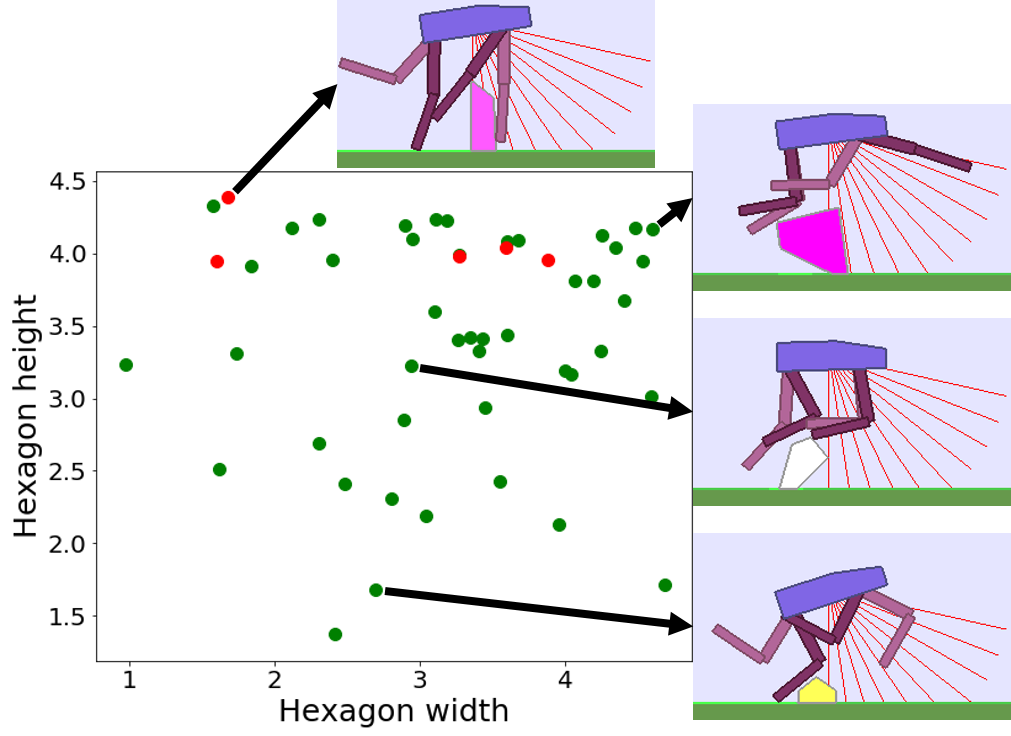
Overall, this work demonstrated that LP-based teacher algorithms could successfully guide DRL agents to learn in difficult continuously parameterized environments with irrelevant dimensions and large proportions of unfeasible tasks. With no prior knowledge of its student's abilities and only loose boundaries on the task space, ALP-GMM, our proposed teacher, consistently outperformed random heuristics and occasionally even expert-designed curricula (see figure 18). This work was presented at CoRL 2019 138.
ALP-GMM, which is conceptually simple and has very few crucial hyperparameters, opens-up exciting perspectives inside and outside DRL for curriculum learning problems. Within DRL, it could be applied to previous work on autonomous goal exploration through incremental building of goal spaces 111. In this case several ALP-GMM instances could scaffold the learning agent in each of its autonomously discovered goal spaces. Another domain of applicability is assisted education, for which current state of the art relies heavily on expert knowledge 83 and is mostly applied to discrete task sets.
8.4.2 Meta Automatic Curriculum Learning
Participants: Remy Portelas, Clement Romac, Katja Hoffman, Pierre-Yves Oudeyer.
In this work we identified that a major challenge in the Deep RL (DRL) community is to train agents able to generalize their control policy over situations never seen in training. Training on diverse tasks has been identified as a key ingredient for good generalization, which pushed researchers towards using rich procedural task generation systems controlled through complex continuous parameter spaces. In such complex task spaces, it is essential to rely on some form of Automatic Curriculum Learning (ACL) to adapt the task sampling distribution to a given learning agent, instead of randomly sampling tasks, as many could end up being either trivial or unfeasible. Since it is hard to get prior knowledge on such task spaces, many ACL algorithms explore the task space to detect progress niches over time, a costly tabula-rasa process that needs to be performed for each new learning agents, although they might have similarities in their capabilities profiles.
To address this limitation, we introduced the concept of Meta-ACL (see fig. 19, and formalized it in the context of black-box RL learners, i.e. algorithms seeking to generalize curriculum generation to an (unknown) distribution of learners. We then presented AGAIN (see fig. 10), a first instantiation of Meta-ACL, and showcased its benefits for curriculum generation over classical ACL in multiple simulated environments including procedurally generated parkour environments with learners of varying morphologies. Videos and code are available at https://
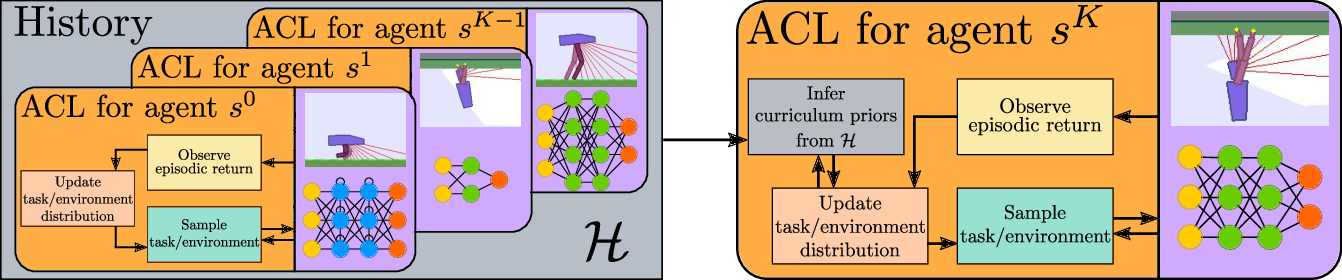
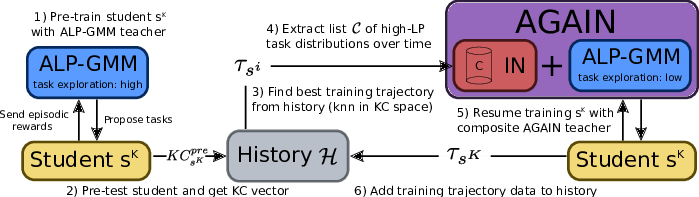
This work is available as preprint 62 and will be submitted to ICML 2021. In future work, AGAIN could be improved by using adaptive approaches to build compact pre-test sets, e.g. using decision tree based test pruning methods, or by combining curriculum priors from multiple previously trained learners. While AGAIN is built on top of an existing ACL algorithm, developing an end-to-end Meta-ACL algorithm that generates curricula using a DRL teacher policy trained across multiple students is also a promising line of work to follow. Additionally, this work opens-up exciting new perspectives in transferring Meta-ACL methods to educational data-mining, e.g. in MOOC scenarios, given a previously trained pilot classroom, one could use Meta-ACL to infer adaptive curricula for new students.
8.4.3 Automatic Curriculum Learning for Deep RL in Procedural Task Spaces: a Benchmark
Participants: Clement Romac, Remy Portelas, Katja Hoffman, Pierre-Yves Oudeyer.
Training autonomous agents able to generalize to a multiplicity of environments/tasks is a key desiderata in current Deep Reinforcement Learning (DRL) research. In parallel to searching for DRL architectures able to learn sets of tasks, many works on Automatic Curriculum Learning (ACL) studied how to emancipate from the usual random task curriculum and instead use teacher algorithms to adapt task selection to the evolving abilities of black-box DRL agents. While multiple standard benchmarks exist to compare DRL agents, there is currently no such thing for ACL algorithms, which makes comparing existing approaches difficult, as too many experimental parameters differ from paper to paper.
In this work, we identified the following key challenges faced by ACL algorithms:
- Mostly unfeasible task spaces - Given the task space is large, as in PCG-encoding task spaces, there might be a predominant amount of unfeasible tasks (or at least initially unfeasible). A good teacher algorithm must have the ability to quickly detect and exploit promising task subspaces for its learner.
- Mostly trivial task spaces - On the contrary, w.r.t. a given student, the task space might be mostly trivial. In that case the teacher has to efficiently detect and exploit the small portion of subspaces of relevant difficulty.
- Forgetting students - DRL learners are prone to catastrophic forgetting, i.e. to overwrite important skills while training new ones. This has to be detected and dealt with by the teacher for optimal curriculum generation.
- Robustness to diverse students - Being able to adapt curriculum generation to diverse students is an important desiderata to ensure a given ACL mechanism has good chances to transfer to novel scenarios.
- Rugged difficulty landscapes - Another important property for ACL algorithms is to be able to deal with task spaces for which the optimal curriculum is not a smooth task distribution sampling drift across the space but rather a series of distribution jumps, e.g. as in complex PCG-task spaces.
- Working with no or few expert knowledge - Prior knowledge over a task space w.r.t. a given student is a costly information gathering process that needs to be repeated for each new problem/student. Relying on as little expert knowledge as possible is therefore a desirable property for ACL algorithms (especially if aiming for out-of-the-lab applications).
Based on these, we presented TeachMyAgent (TA), a benchmark of current ACL algorithms including 1) skill-specific unit-tests using variants of a procedural Box2D bipedal walker environment, and 2) a new procedural Parkour environment combining most ACL challenges, making it ideal for global performance assessment. We then leveraged TeachMyAgent to conduct a comparative study of existing approaches, showcasing the competitiveness of expert-knowledge-free ACL approaches, and showing that our Parkour environment remains an open problem.
Challenge-specific comparison with Stump Tracks
In order to propose a comparison of the different ACL methods on each of the challenges introduced above, we leveraged the Stump Tracks environment introduced in 138 to create five experiments, each of them designed to highlight the ability of a teacher in one the first five ACL challenges. Additionnaly, in order to analyse separately the expert knowledge challenge, we performed each of the experiments above in three prior knowledge conditions:
- No expert knowledge
- Low expert knowledge
- High expert knowledge
Using these 15 experiments, we compared 7 ACL methods in addition of a baseline teacher sampling tasks uniformly random over the task space. In each experiment, a DRL student is trained for 20 millions steps using an ACL algorithm to set the procedural generation of the environment at every episode. We monitored the DRL student's performances on a pre-defined test set composed of 100 tasks every 500000 steps and reported the average percentage of mastered tasks (i.e. task on which the agent obtained an episodic reward greater than 230). Results are gathered in figure 21 and presented as an order of magnitude of the Random teacher (using 32 seeds per experiment).
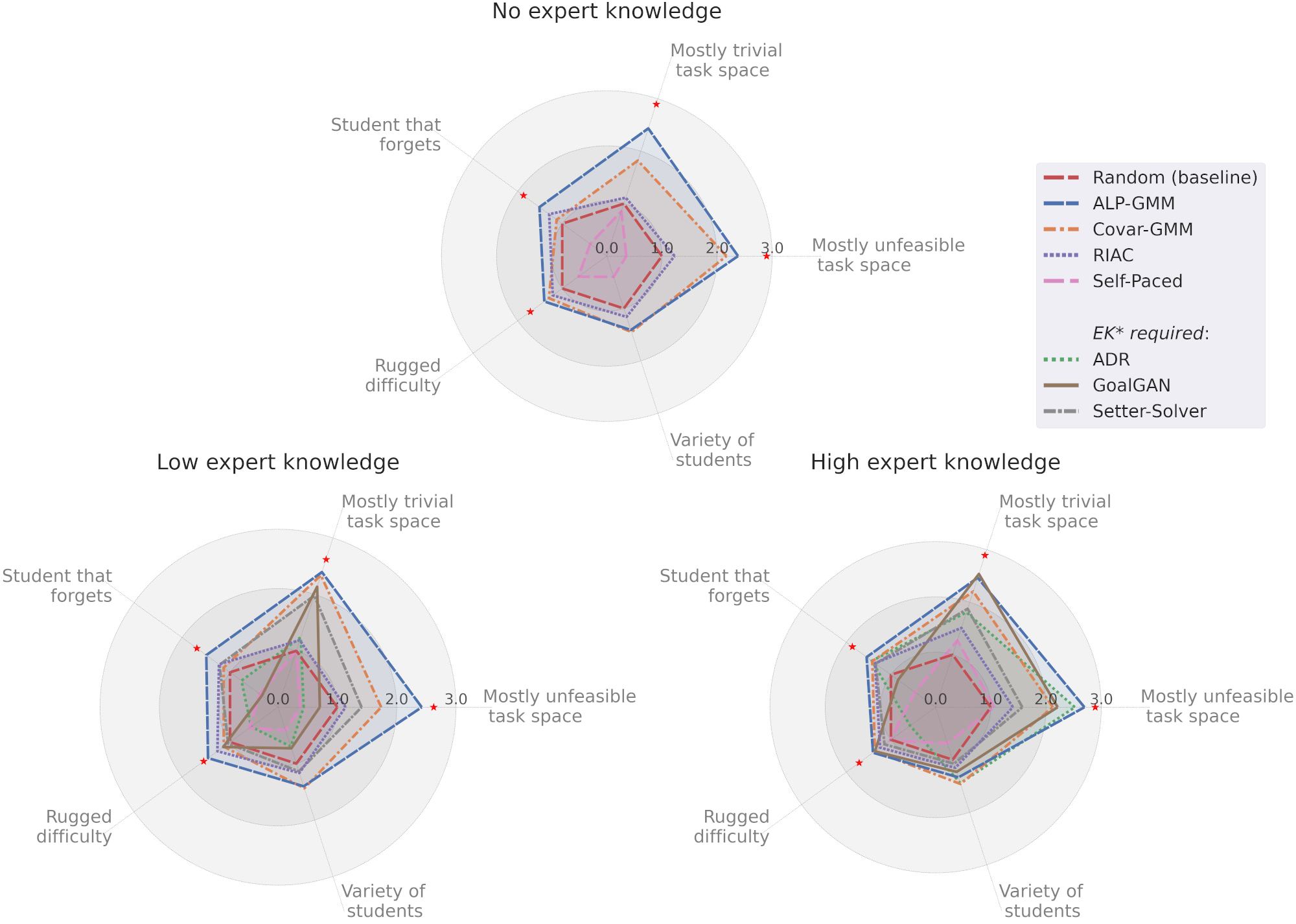
While these results highlighted the strenghs and weaknesses of each method (e.g. the inability of ADR to deal with rugged difficulty landscapes or the inertia of GoalGAN in adapting the curriculum to forgetting students), it also showed how competitive expert-knowledge-free methods like ALP-GMM are, even when compared to methods having access to a high amount of prior knowledge.
Global performances analysis using the Parkour
We then assessed the different ACL methods' performances on our Box2D Parkour track environment (see figure 22) which features most of the previously discussed ACL challenges: 1) most tasks are unfeasible, 2) Before each run, unknown to the teacher, the student's embodiment is uniformly sampled among three morphologies (bipedal walker, fish and chimpanzee), requiring the teacher to adapt curriculum generation to its current student's abilities, and 3) tasks are generated through a CCPN-based PCG, creating a rich task space with rugged difficulty landscape and hardly-definable prior knowledge.
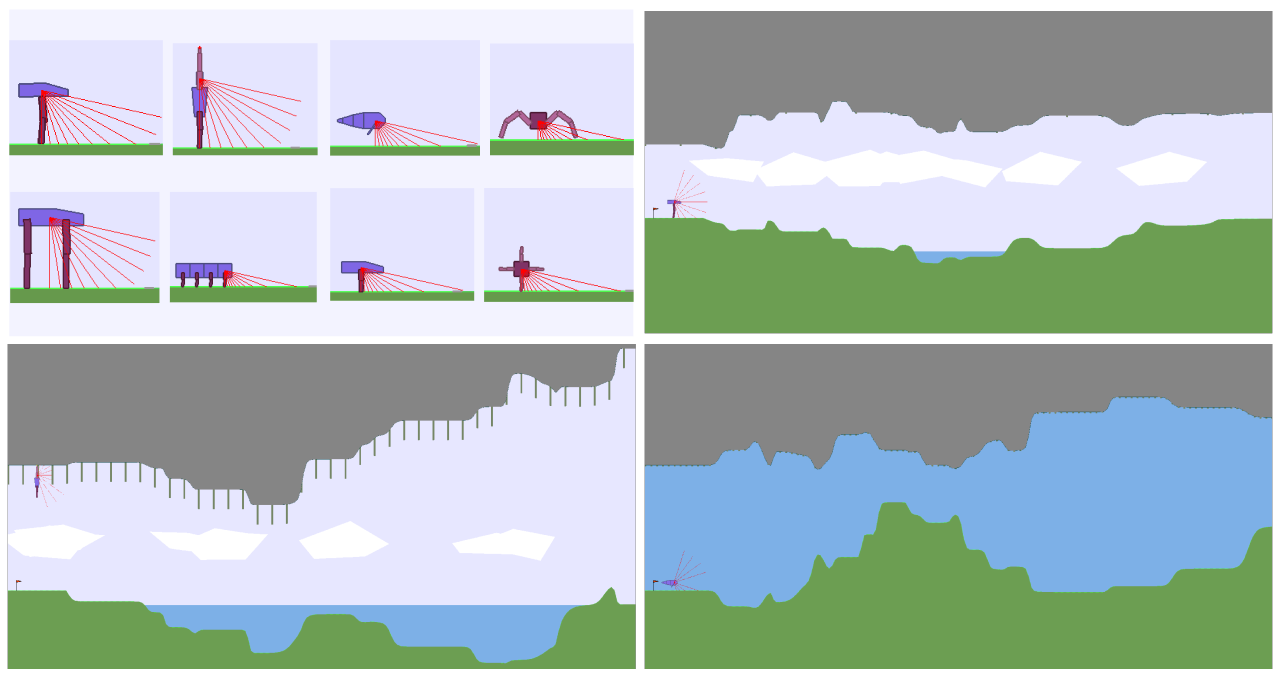
We trained a DRL student for 20 millions steps with 48 different seeds (16 per morphology) and monitored the percentage of mastered tasks as in our Stump Tracks experiments. As few expert knowledge is accessible, our results (figure 23) showed to poor performances from expert-knowledge-depend teachers (e.g. ADR or SPDL). Additionally, overall results awerere quite low, especially for the seeds using our chimpanzee embodiment, where none of the ACL methods managed to master more than of the test set. This thus leaves our Parkour track as an open challenge for future design of ACL methods.
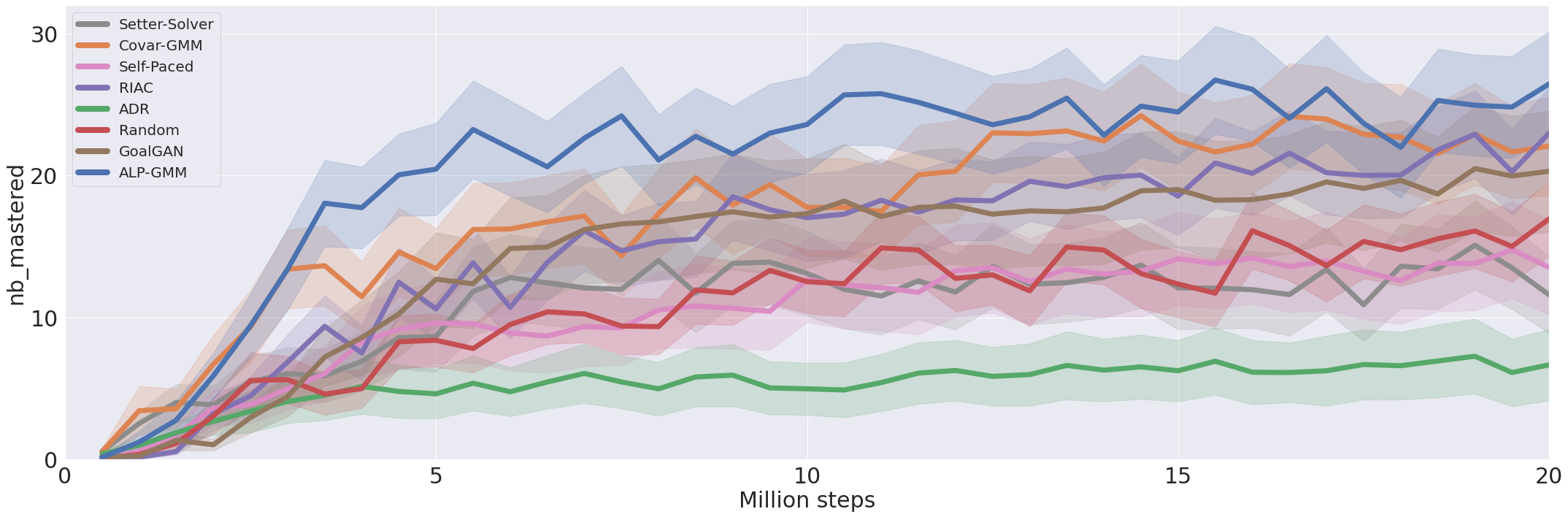
8.4.4 Other
Sensory Commutativity of Action Sequences
Participants: Hugo Caselles-Dupré, David Filliat.
We study perception in the scenario of an embodied agent equipped with first-person sensors and a continuous motor space with multiple degrees of freedom. We consider the commutative properties of action sequences with respect to sensory information perceived by such an embodied agent. We introduce the Sensory Commutativity Probability (SCP) criterion which measures how much an agent’s degree of freedom affects the environment in embodied scenarios. We show how to compute this criterion in different environments, including realistic robotic setups. We empirically illustrate how SCP and the commutative properties of action sequences can be used to learn about objects in the environment and improve sample efficiency in Reinforcement Learning.
Our research was published in the Workshop on Learning in Artificial Open Worlds at ICML20 42 and NeurIPS 2020 Workshop on BabyMind 41.
Should artificial agents ask for help in human-robot collaborative problem-solving?
Participants: Adrien Bennetot, Vicky Charisi, Natalia Díaz-Rodríguez.
Transferring as fast as possible the functioning of our brain to artificial intelligence is an ambitious goal that would help advance the state of the art in AI and robotics. It is in this perspective that we propose to start from hypotheses derived from an empirical study in a human-robot interaction and to verify if they are validated in the same way for children as for a basic reinforcement learning algorithm 40. Thus, we check whether receiving help from an expert when solving a simple close-ended task (the Towers of Hanoï) allows to accelerate or not the learning of this task, depending on whether the intervention is canonical or requested by the player. Our experiences have allowed us to conclude that, whether requested or not, a Q-learning algorithm benefits in the same way from expert help as children do.
8.5 Multi-agent Reinforcement learning for Ecologically-valid Artificial Intelligence
8.5.1 Grounding Artificial Intelligence in the Origins of Human Behavior
Participants: Clément Moulin-Frier, Eleni Nisioti, Julius Taylor.
Introduction
One of the most ambitious goal in Artificial Intelligence (AI) is the realization of a so-called Artificial General Intelligence (AGI), i.e. AI that is not limited to the realization of a predefined set of tasks but is able to generalize its capabilities to any cognitive task that can be solved by human intelligence. This is obviously a long-term objective but recent advances in AI have revived research in this field, with the vast majority of contributions focusing on
- new cognitive architectures and learning algorithms 146;
- new cost functions to be optimized 97 ;
- new databases to learn from 105
. However, although AGI is fundamentally related to the characteristics of human intelligence, research in this field rarely considers the processes that may have guided the emergence of complex cognitive capacities during the evolution of the species. Research in Human Behavioral Ecology (HBE) 76 seeks to understand how the behaviors characterizing human nature can be conceived as adaptive responses to major changes in the structure of our ecological niche. However, very little work in AI proposes to study how this long-term environmental dynamics can potentially guide and improve the acquisition of complex behaviors in artificial systems (see however recent contributions 155, including from our research group 138, 24). Moreover, to our knowledge, modern AI methods for learning behaviors in sequential environments have not yet been applied to test hypotheses in HBE (although it has been recently proposed 92).
An inter-disciplinary dialogue between AI and HBE
As a first step in our project, we conducted a targeted yet extensive literature review on HBE, in particular works studying the effect that climate complexity has had on the emergence of adaptability, cooperation and cultural repertoire in human evolution. In parallel, we have reviewed the state-of-the-art in the study of open-ended skill acquisition in, in particular, the AI sub-fields of multi-agent reinforcement and meta reinforcement learning. We have compiled our review in a position paper that summarizes the project's objectives 61. An important objective at this stage was to justify the proposed exchange of ideas between the two fields by identifying their commonalities in terms of research challenges. In Figure 24, we introduce a conceptual framework that recognizes important ecological components, as well as the feedforward and feedback links that relate them. This figure was presented in our preprint 61.
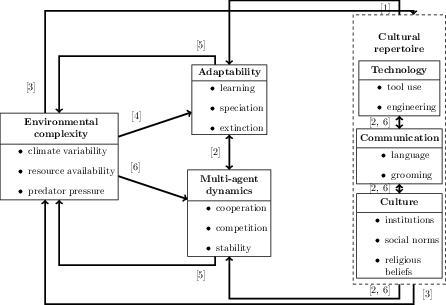
Objectives
In our next steps, we plan to work on the lines of improving the state-of-the-art in meta RL and multi-agent RL by leveraging hypotheses from HBE. Simultaneously, in a similar spirit to our group's proposal of using multi-agent RL as a computational tool for studying language development 124, we will employ RL as a computational tool for evaluating HBE hypotheses. In particular, our review has identified the following research challenges:
- identifying the effect that environmental variability has on the adaptability of meta RL agents. The rate of environmental change is an important hyper-parameter for meta RL algorithms but has only recently attracted attention 110. Our plan is to ground this investigation in HBE theories from climate variability, which state that the adaptability of species is achieved through mechanisms whose form depends on properties of the environment. If the environment is constant across time and space, natural selection may favor innate behaviors. By contrast, if the environment varies, natural selection might favor behavioral plasticity: based on environmental observations an agent may be able to switch between different behaviors following innate, and not learned instructions 92. In cases where the environment changes noticeably across generations but slowly enough within a generation, behavioral plasticity is guided by a process of developmental selection, an example of which is the learning process, where an agent’s past behavior guide its future behavior.
- studying the effect that environmental properties such as predator pressure and resource availability have on groups of RL agents. Emergent autocurricula in multi-agent RL have been observed to lead to open-ended skill acquisition in various works 69, 112. We plan to investigate how group properties, such as size and structure, are influenced by their environment and create feedback loops that lead to the emergence of autocurricula. Preliminary work was realized in this direction during the Master internship of Younès Rabii (February to August 2020), who implemented predator-preys complex systems within a multi-agent simulated environment. This work initiated a collaboration with Michael Garcia-Ortiz from City University in London (UK).
- cultural repertoire in large-scale groups of RL agents. According to the social complexity hypothesis 93, uniquely human skills such as language, social norms and institutions emerged as a need to regulate interactions in social systems of increasing size and structural complexity. We plan to study emergent communication in MARL as part of the ongoing Phd thesis of Julius Taylor (started November 2020, see also our recent position paper 48). We also recently started a collaboration with Microsoft Research New-York (USA) on a project that studies the role of fireside chats in the emergence of rich communication systems in groups of RL agent, in relation with theories of language evolution. We have recently published a paper on the emergence of social conventions in collaboration with University Popeu Fabra in Spain 25. Finally, preliminary experiments on the role of partial observability and channel reliability in emergent communication were realized during the Master internship of Valentin Villecroze (April to August 2020). This work was published as a workshop paper (53) and an extract of the results is presented in figure 25.
8.6 Applications in Educational Technologies
8.6.1 Machine Learning for Adaptive Personalization in Intelligent Tutoring Systems
Participants: Pierre-Yves Oudeyer, Benjamin Clément, Didier Roy, Hélène Sauzeon.
The Kidlearn project
Kidlearn is a research project studying how machine learning can be applied to intelligent tutoring systems. It aims at developing methodologies and software which adaptively personalize sequences of learning activities to the particularities of each individual student. Our systems aim at proposing to the student the right activity at the right time, maximizing concurrently his learning progress and his motivation. In addition to contributing to the efficiency of learning and motivation, the approach is also made to reduce the time needed to design ITS systems.
We continued to develop an approach to Intelligent Tutoring Systems which adaptively personalizes sequences of learning activities to maximize skills acquired by students, taking into account the limited time and motivational resources. At a given point in time, the system proposes to the students the activity which makes them progress faster. We introduced two algorithms that rely on the empirical estimation of the learning progress, RiARiT that uses information about the difficulty of each exercise and ZPDES that uses much less knowledge about the problem.
The system is based on the combination of three approaches. First, it leverages recent models of intrinsically motivated learning by transposing them to active teaching, relying on empirical estimation of learning progress provided by specific activities to particular students. Second, it uses state-of-the-art Multi-Arm Bandit (MAB) techniques to efficiently manage the exploration/exploitation challenge of this optimization process. Third, it leverages expert knowledge to constrain and bootstrap initial exploration of the MAB, while requiring only coarse guidance information of the expert and allowing the system to deal with didactic gaps in its knowledge. The system was evaluated in several large-scale experiments relying on a scenario where 7-8 year old schoolchildren learn how to decompose numbers while manipulating money 83. Systematic experiments were also presented with simulated students.
Kidlearn Experiments 2018-2019: Evaluating the impact of ZPDES and choice on learning efficiency and motivation
An experiment was held between mars 2018 and July 2019 in order to test the Kidlearn framework in classrooms in Bordeaux Metropole. 600 students from Bordeaux Metropole participated in the experiment. This study had several goals. The first goal was to evaluate the impact of the Kidlearn framework on motivation and learning compared to an Expert Sequence without machine learning. The second goal was to observe the impact of using learning progress to select exercise types within the ZPDES algorithm compared to a random policy. The third goal was to observe the impact of combining ZPDES with the ability to let children make different kinds of choices during the use of the ITS. The last goal was to use the psychological and contextual data measures to see if correlation can be observed between the students psychological state evolution, their profile, their motivation and their learning. The different observations showed that generally, algorithms based on ZPDES provided a better learning experience than an expert sequence. In particular, they provide a more motivating and enriching experience to self-determined students. Details of these new results, as well as the overall results of this project, are presented in Benjamin Clément PhD thesis 82 and are currently being processed to be published.
Kidlearn and Adaptiv'Math
The algorithms developed during the Kidlearn project and Benjamin Clement thesis 82 are being used in an innovation partnership for the development of a pedagogical assistant based on artificial intelligence intended for teachers and students of cycle 2. The algorithms are being written in typescript for the need of the project. The expertise of the team in creating the pedagogical graph and defining the graph parameters used for the algorithms is also a crucial part of the role of the team for the project. One of the main goal of the team here is to transfer technologies developed in the team in a project with the perspective of industrial scaling and see the impact and the feasibility of such scaling.
Kidlearn for numeracy skills with individuals with autism spectrum disorders
Few digital interventions targeting numeracy skills have been evaluated with individuals with autism spectrum disorder (ASD) 55120. Yet, some children and adolescents with ASD have learning difficulties and/or a significant academic delay in mathematics. While ITS are successfully developed for typically developed students to personnalize learning curriculum and then to foster the motivation-learning coupling, they are not or fewly proposed today to student with specific needs. The objective of this pilot study is to test the feasibility of a digital intervention using an STI with high school students with ASD and/or intellectual disability. This application (KidLearn) provides calculation training through currency exchange activities, with a dynamic exercise sequence selection algorithm (ZPDES). 24 students with ASD and/or DI enrolled in specialized classrooms were recruited and divided into two groups: 14 students used the KidLearn application, and 10 students received a control application. Pre-post evaluations show that students using KidLearn improved their calaculation performance, and had a higher level of motivation at the end of the intervention than the control group. These results encourage the use of an STI with students with specific needs to teach numeracy skills, but need to be replicated on a larger scale. Suggestions for adjusting the interface and teaching method are suggested to improve the impact of the application on students with autism. (Paper is submitted).
8.6.2 Machine learning for adaptive cognitive training
Participants: Pierre-Yves Oudeyer, Hélène Sauzéon, Masataka Sawayama, Benjamin Clément, Maxime Adolphe.
Because of its cross-cutting nature to all cognitive activities such as learning tasks, attention is a hallmark of good cognitive health throughout life and more particularly in the current context of societal crisis of attention. Recent works have shown the great potential of computerized attention training for an example of attention training, with efficient training transfers to other cognitive activities, and this, over a wide spectrum of individuals (children, elderly, individuals with cognitive pathology such as Attention Deficit and Hyperactivity Disorders). Despite this promising result, a major hurdle is challenging: the high inter-individual variability in responding to such interventions. Some individuals are good responders (significant improvement) to the intervention, others respond variably, and finally some respond poorly, not at all, or occasionally. A central limitation of computerized attention training systems is that the training sequences operate in a linear, non-personalized manner: difficulty increases in the same way and along the same dimensions for all subjects. However, different subjects require in principle a progression at a different, personalized pace according to the different dimensions that characterize attentional training exercises.
To tackle the issue of inter-individual variability, the present project proposes to apply some principles from intelligent tutorial systems (ITS) to the field of attention training. In this context, we have already developed automatic curriculum learning algorithms such as those developed in the KidLearn project, which allow to customize the learner's path according to his/her progress and thus optimize his/her learning trajectory while stimulating his/her motivation by the progress made. ITS are widely identified in intervention research as a successful way to address the challenge of personalization, but no studies to date have actually been conducted for attention training. Thus, whether ITS, and in particular personalization algorithms, can optimize the number of respondents to an attention training program remains an open question.
To investigate this question, a web platform has been designed for planning and implementing remote behavioural studies. This tool provides means for registering recruited participants remotely and executing complete experimental protocols: from presenting instructions and obtaining informed consents, to administering behavioural tasks and questionnaires, potentially throughout multiple sessions spanning days or weeks. As a result, several studies using this tool will be conducted during the following months.
8.6.3 Interactive systems that foster curiosity for education
Participants: Pierre-Yves Oudeyer, Hélène Sauzéon, Mehdi Alami, Rania Abdelghani, Didier Roy, Edith Law.
Since 2019 via the renewal of the Idex cooperation fund (between the University of Bordeaux and the University of Waterloo, Canada) led by the Flowers team and also involving F. Lotte from the Potioc team, we continue our work on the development of new curiosity-driven interaction systems. Although experimentations have been slowed down by sanitary conditions, progress has been made in this area of application of FLOWERS works. In particular, three studies have been completed.
The first study regards a new interactive educational application to foster curiosity-driven question-asking in children. This study has been performed during the Master 2 internship of Mehdi Alaimi co-supervised by H. Sauzéon, E. Law and PY Oudeyer. It addresses a key challenge for 21st-century schools, i.e., teaching diverse students with varied abilities and motivations for learning, such as curiosity within educational settings. Among variables eliciting curiosity state, one is known as « knowledge gap », which is a motor for curiosity-driven exploration and learning. It leads to question-asking which is an important factor in the curiosity process and the construction of academic knowledge. However, children questions in classroom are not really frequent and don’t really necessitate deep reasoning. Determined to improve children’s curiosity, we developed a digital application aiming to foster curiosity-related question-asking from texts and their perception of curiosity. To assess its efficiency, we conducted a study with 95 fifth grade students of Bordeaux elementary schools. Two types of interventions were designed, one trying to focus children on the construction of low-level question (i.e. convergent) and one focusing them on high-level questions (i.e. divergent) with the help of prompts or questions starters models. We observed that both interventions increased the number of divergent questions, the question fluency performance, while they did not significantly improve the curiosity perception despite high intrinsic motivation scores they have elicited in children. The curiosity-trait score positively impacted the divergent question score under divergent condition, but not under convergent condition. The overall results supported the efficiency and usefulness of digital applications for fostering children’s curiosity that we need to explore further. The overall results are published in CHI'20 33.
The second study investigates the neurophysiological underpinnings of curiosity and the opportunities of their use for Brain-computer interactions 34. Understanding the neurophysiological mechanisms underlying curiosity and therefore being able to identify the curiosity level of a person, would provide useful information for researchers and designers in numerous fields such as neuroscience, psychology, and computer science. A first step to uncovering the neural correlates of curiosity is to collect neurophysiological signals during states of curiosity, in order to develop signal processing and machine learning (ML) tools to recognize the curious states from the non-curious ones. Thus, we ran an experiment in which we used electroencephalography (EEG) to measure the brain activity of participants as they were induced into states of curiosity, using trivia question and answer chains. We used two ML algorithms, i.e. Filter Bank Common Spatial Pattern (FBCSP) coupled with a Linear Discriminant Algorithm (LDA), as well as a Filter Bank Tangent Space Classifier (FBTSC), to classify the curious EEG signals from the non-curious ones. Global results indicate that both algorithms obtained better performances in the 3-to-5s time windows, suggesting an optimal time window length of 4 seconds to go towards curiosity states estimation based on EEG signals.
Finally, the third study investigates the role of intrinsic motivation in spatial learning in children (paper in progress). In this study, the state curiosity is manipulated as a preference for a level of uncertainty during the exploration of new environments. To this end, a series of virtual environments have been created and is presented to children. During encoding, participants explore routes in environments according the three levels of uncertainty (low, medium, and high), thanks to a virtual reality headset and controllers and, are later asked to retrace their travelled routes . The exploration area and the wayfinding. ie the route overlap between encoding and retrieval phase, (an indicator of spatial memory accuracy) are measured. Neuropsychological tests are also performed. Preliminary results showed that there are better performances under the medium uncertainty condition in terms of exploration area and wayfinding score. These first results supports the idea that curiosity states are a learning booster.
At the end of 2020, we started an industrial collaboration project with EvidenceB on this topic (CIFRE contract of Rania Abdelghani currently submitted to the ANRT). The overall objective of the thesis is to propose new educational technologies driven by epistemic curiosity, and allowing children to express themselves more and learn better. To this end, a central question of the work will be to specify the impact of self-questioning aroused by states of curiosity about student performance. Another objective will be to create and study the pedagogical impact of new educational technologies in real situations (schools) promoting an active education of students based on their curiosity.
8.6.4 A computer science and robotics integration model for primary school
Participants: Didier Roy.
Integrating computer science (CS) into school curricula has become a worldwide preoccupation. Therefore, we present a CS and Robotics integration model and its validation through a large-scale pilot study in the administrative region of the Canton Vaud in Switzerland. Approximately 350 primary school teachers followed a mandatory CS continuing professional development program (CPD) of adapted format with a curriculum scaffolded by instruction modality. This included CS Unplugged activities that aim to teach CS concepts without the use of screens, and Robotics Unplugged activities that employed physical robots, without screens, to learn about robotics and CS concepts. Teachers evaluated positively the CPD and their representation of CS improved. Voluntary adoption rates reached 97 percent during the CPD and 80 percent the following year. These results combined with the underpinning literature support the generalisability of the model to other contexts. This work was published in 23 and led by our colleagues at EPFL.
8.6.5 How An Automated Gesture Imitation Game Can Improve Social Interactions With Teenagers With ASD
Participants: Linda Nanan Vallée, Sao Mai Nguyen, Christophe Lohr, Ioannis Kanellos, Olivier Asseu.
With the outlook of improving communication and social abilities of people with ASD, we propose to extend the paradigm of robot-based imitation games to ASD teenagers. In this paper 52, we present an interaction scenario adapted to ASD teenagers, propose a computational architecture using the latest machine learning algorithm Openpose for human pose detection, and present the results of our basic testing of the scenario with human caregivers. These results are preliminary due to the number of session (1) and participants (4). They include a technical assessment of the performance of Openpose, as well as a preliminary user study to confirm our game scenario could elicit the expected response from subjects.
8.7 Applications to Automated Discovery in Self-Organizing Systems
8.7.1 Curiosity-driven Learning for Automated Discovery of Physico-Chemical Structures
Participants: Chris Reinke, Mayalen Etcheverry, Pierre-Yves Oudeyer.
Introduction
Intrinsically motivated goal exploration algorithms (IMGEPs) enable machines to discover repertoires of action policies that produce a diversity of effects in complex environments. In robotics, these exploration algorithms have been shown to allow real world robots to acquire skills such as tool use 9170. In other domains such as chemistry and physics, they open the possibility to automate the discovery of novel chemical or physical structures produced by complex dynamical systems 137. However, they have so far assumed that self-generated goals are sampled in a specifically engineered feature space, limiting their autonomy. Recent work has shown how unsupervised deep learning approaches could be used to learn goal space representations 136 but they have used precollected data to learn the representations. This project studies how IMGEPs can be extended and used for automated discovery of behaviours of dynamical systems in physics or chemistry without using assumptions or knowledge about such systems.
As a first step towards this goal we choose Lenia 80, a simulated high-dimensional complex dynamical system, as a target system. Lenia is a continuous cellular automaton where diverse visual structures can self-organize (Fig.26, c). It consists of a two-dimensional grid of cells where the state of each cell is a real-valued scalar activity . The state of cells evolves over discrete time steps . The activity change is computed by integrating the activity of neighbouring cells. Lenia's behavior is controlled by its initial pattern and several settings that control the dynamics of the activity change. Lenia can produce diverse patterns with different dynamics. Most interesting, spatially localized coherent patterns that resemble in their shapes microscopic animals can emerge. Our goal was to develop methods that allow to explore a high diversity of such animal patterns.
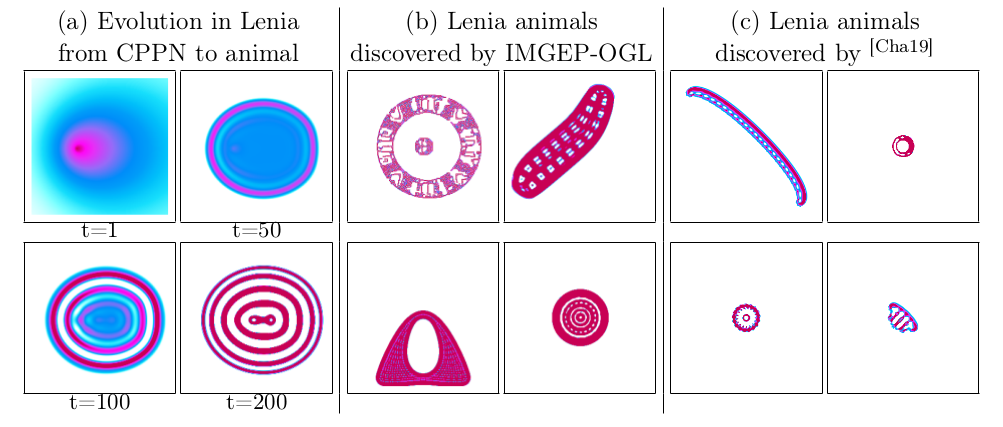
We could successfully accomplish this goal 38 based on two key contributions of our research: 1) the usage of compositional pattern producing networks (CPPNs) for the generation of initial states for Lenia, and 2) the development of a novel IMGEP algorithm that learns goal representations online during the exploration of the system.
1) CPPNs for the generation of initial states
A key role in the generation of patterns in dynamical systems is their initial state . IMGEPs sample these initial states and apply random perturbations to them during the exploration. For Lenia this state is a two-dimensional grid with cells. Performing directly a random sampling of the grid cells results in initial patterns that resemble white noise. Such random states result mainly in the emergence of global patterns that spread over the whole state space, complicating the search for spatially localized patterns. We solved the sampling problem for the initial states by using compositional pattern producing networks (CPPNs) 147. CPPNs are recurrent neural networks that allow the generation of structured initial states (Fig.26, a). The CPPNs are used as part of the system parameters which are explored by the algorithms. They are defined by their network structure (number of neurons, connections between neurons) and their connection weights. They include a mechanism for random mutation of the weights and structure.
2) IMGEP for Online Learning of Goal Space Representations
We proposed an online goal space learning IMGEP (IMGEP-OGL), which learns the goal space incrementally during the exploration process. A variational autoencoder (VAE) is used to encode Lenia patterns into a 8-dimensional latent representation used as goal space. The training procedure of the VAE is integrated in the goal sampling exploration process by first initializing the VAE with random weights. The VAE network is then trained every explorations for epochs on the previously idetnfied patterns during the exploration.
Experiments
We evaluated the performance of the novel IMGEP-OGL to other exploration algorithms by comparing the diversity of their identified patterns. Diversity is measured by the spread of the exploration in an analytic behavior space . This space is defined by a latent representation space that was build through the training of a VAE to learn the important features over a very large dataset of Lenia patterns identified during the many experiments over all evaluated algorithms. We then augmented that space by concatenating hand-defined features. Each identified Lenia pattern is represented by a specific point in this space. The space was then discretized in a fixed number of areas/bins of equal size. The final diversity measure of each algorithm is the number of areas/bins in which at least one explored pattern exists.
We compared different exploration algorithms to the novel IMGEP-OGL: 1) Random exploration of system parameters, 2) IMGEP-HGS: IMGEP with a hand-defined goal space, 3) IMGEP-PGL: IMGEP with a learned goal space via an VAE by a precollected dataset of Lenia patterns, and 4) IMGEP-RGS: IMGEP with a VAE with random weights that defines the goal space.
The system parameters consisted of a CPPN that generates the initial state for Lenia and 6 further settings defining Lenia's dynamics: . The CPPNs were initialized and mutated by a random process that defines their structure and connection weights as done. The random initialization of the other Lenia settings was done by an uniform distribution and their mutation by a Gaussian distribution around the original values.
Results
The diversity of identified patterns in the analytic behavior space show that IMGEP approaches with learned goal spaces via VAEs (PGL, OGL) could identify the highest diversity of patterns overall (Fig. 27, a). They were followed by the IMGEP with a hand-defined goal space (HGS). The lowest performance had the random exploration and the IMGEP with a random goal space (RGS). The advantage of learned goals space approaches (PGL, OGL) over all other approaches was even stronger for the diversity of animal patterns, i.e. the main goal of our exploration (Fig. 27, b).
(a) Diversity of all identified Patterns | (b) Diversity of Animal Patterns |
![]() |
![]() |
Conclusion
Our goal was to investigate new techniques based on intrinsically motivated goal exploration for the automated discovery of patterns and behaviors in complex dynamical systems. We introduced a new algorithm (IMGEP-OGL) which is capable of learning unsupervised goal space representations during the exploration of an unknown system. Our results for Lenia, a high-dimensional complex dynamical system, show its superior performance over hand-defined goal spaces or random exploration. It shows the same performance as a learned goal space based on precollected data, showing that such a precollection of data is not necessary. We furthermore introduced the usage of CPPNs for the successful initialization of the intial states of the dynamical systems. Both advances allowed us to explore an unknown and high-dimensional dynamical system which shares many similarities with different physical or chemical systems.
This work is published at ICLR 2020 38. The project website with videos and additional results can be found at https://
8.7.2 Hierarchically Organized Latent Modules for Meta-Diversity Search in Morphogenetic Systems
Participants: Mayalen Etcheverry, Clément Moulin-Frier, Pierre-Yves Oudeyer.
In the previous paper 38, the problem of automated diversity-driven discovery in morphogenetic systems was introduced, highlighting that two key ingredients are autonomous exploration and unsupervised representation learning to describe "relevant" degrees of variations in the patterns. Yet, standard diversity-driven approaches assume that the intuitive notion of diversity can be captured within a single behavioral characterization (BC) space.
In this project, we follow the proposed experimental testbed of Reinke et al.(2020) 38 on a continuous game-of-life system (Lenia, 80). We provide empirical evidence that the discoveries of an IMGEP operating in a monolithic BC space are highly-diverse in that space, yet tend to be poorly-diverse in other potentially-interesting BC spaces (see Figure 28). This draws several limitations when it comes to applying such system as a tool for assisting discovery in morphogenetic system, as the suggested discoveries are unlikely to align with the interests of a end-user.
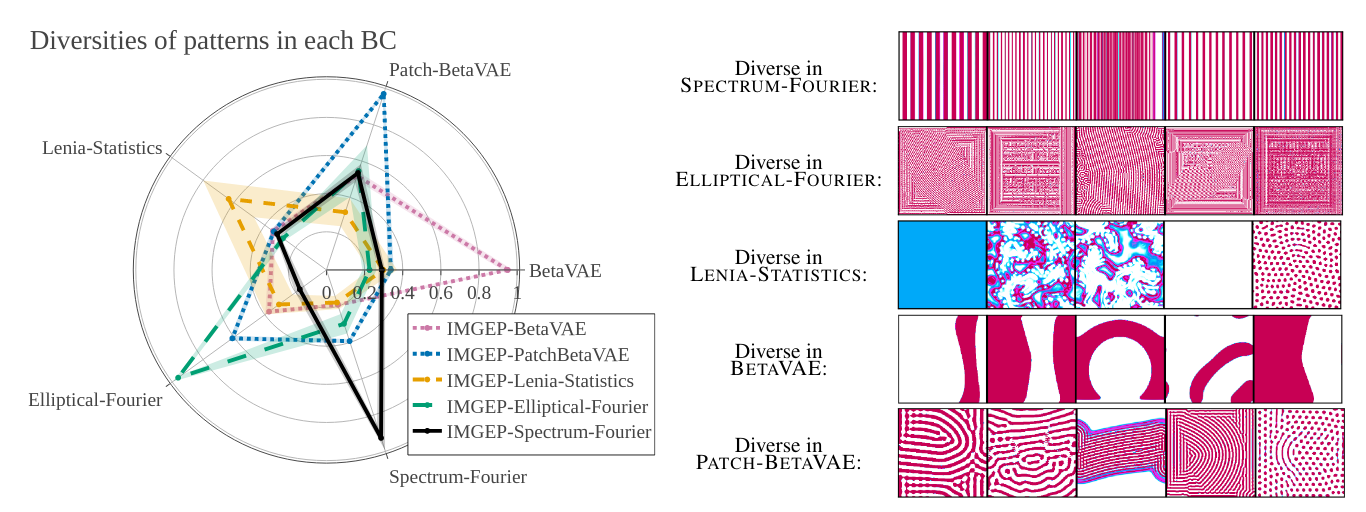
To address these limits, the contributions of this project are threefold. First, we formulate the problem of meta-diversity search as follows: an artificial “discovery assistant” incrementally learns a set of diverse BC spaces in an outer loop; and searches to discover diverse patterns within each of them in an inner loop. With minimal external feedback, a successful discovery assistant should be able to efficiently specialize the exploration strategy toward a particular type of diversity, corresponding to the initially unknown preferences of the human evaluator.
Second, we present HOLMES , a dynamic and modular model architecture for unsupervised learning of diverse representations where a hierarchy of module embedding networks is actively expanded. Additionally, we present IMGEP-HOLMES (see Figure 29) which extends the standard IMGEP framework by replacing the monolithic representation with the proposed hierarchy. We show that the hierarchical structure allows the IMGEP agent to target goals in the different nodes in order to achieve diversity in each BC space.
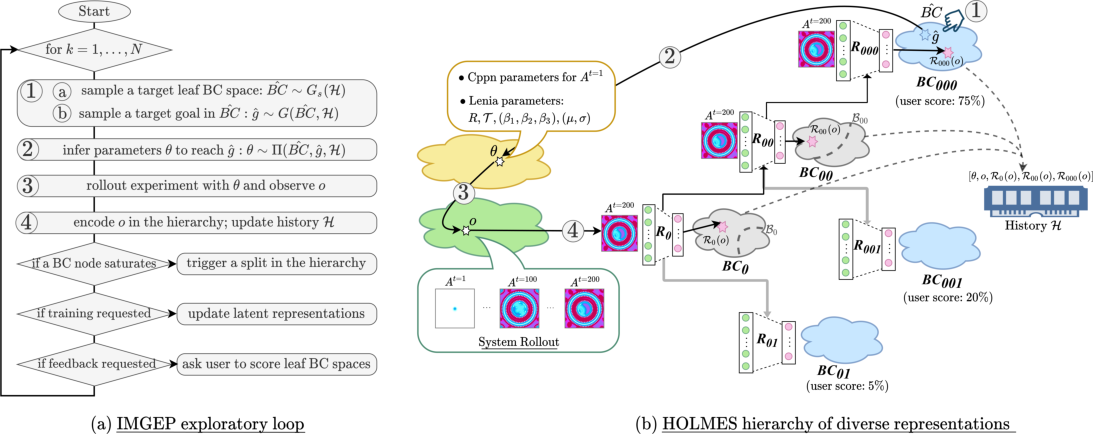
Finally, we show how this architecture can easily be leveraged to drive exploration, opening interesting perspectives for the integration of a human in the loop.
To conclude, this work shows that integrating flexible modular representation learning with intrinsically-motivated goal exploration processes for meta-diversity search are very promising directions in the context of automated discovery in morphogenetic systems. As an example, IMGEP-HOLMES was able to discover many types of solitons including unseen pattern-emitting lifeforms in less than 15000 training steps without guidance, when their existence remained an open question raised in the original Lenia paper 80.
Initial version of this work was presented at ICLR 2021 workshop "Beyond tabula rasa in Reinforcement Learning" 46. The final version of this work is published at NeurIPS 2020 35. The project website with videos and additional results can be found at http://
8.7.3 Automated exploration of neuro-mechanical models or arms using goal exploration algorithms
Participants: Pierre-Yves Oudeyer.
This work was led by Daniel Cattaert, Aymar de Rugy and their collaborators at Incia, with contributions from Pierre-Yves Oudeyer.
Objective. Neuro-mechanical models are essential to increase our understanding of the fundamental mechanisms underlying natural sensorimotor control, and to foster robotic designs using them. Yet, the complexity of those models is such that current optimization methods are unsuited to establish the range of useful behaviors they could produce, and their associated parameter settings. Our goal is to provide both using recent advances in developmental machine learning. Approach. We designed a simplified neuro-mechanical model that nevertheless has the complexity that make current optimization fail. This model consists of a single (elbow) joint actuated by two muscles and their associated spindles, alpha and gamma motoneurons, receiving simple (non-dynamic) step commands. To establish the range of movements this system is capable of doing, a goal exploration process was used that built a repertoire of valid actions through iterative sampling of target behaviors, combined with stochastic variation on the parameter settings that elicited their closest behaviors in this repertoire. Results obtained with this process were compared to those obtained with alternative optimization methods. Main results. The goal exploration was found to widely outperform optimization methods in terms of its capacity to rapidly establish a repertoire of valid actions, and to find a large range of behaviors not otherwise found. The resulting repertoire also provides diverse parameter sets for any given actions, akin to what is observed in natural control. Families of solutions originating from few initial seeds should also be exploitable to generate novel behaviors through interpolation. Significance. The proposed method provides rich perspectives to explore the structure and settings of lower-level neural circuitry, and their associated descending commands, to produce a wide range of useful behaviors. Comparison of behavioral space obtained after selective manipulation of various elements of neuro-mechanical models should also help understand natural control, and promote its emulation in robotics. We have written an article under review.
8.7.4 Design of an Interactive Software for Automated Discovery in Complex Systems
Participants: Clément Romac, Mayalen Etcheverry, Clément Moulin-Frier, Pierre-Yves Oudeyer.
Additionally, 35 also showed that adding human in the exploration loop can be a key to obtain interesting mappings. Designing interactive algorithms is thus an important step towards the adoption of automated exploration and discovery of complex systems, as users previously using hand-made heuristics would still need to add their expert knowledge in the exploration process.
Following these, we propose to design an interactive software which aims to provide tools to easily use exploration algorithms (e.g. curiosity-driven) in various systems. Several challenges are to be faced in this project among the possibility to use any complex system (numeric or physical), the need of a scalable architecture or having an user-friendly interface with efficient and modular visualisation tools.
We propose to use a microservice architecture and leverage Docker to make the software easily installable and modifiable by non-computer scientist users. We separate the front-end application on which the user will create experiments and interact with them from the automated discovery process (making the scalability issues easier to deal). We choose to use Python for Machine Learning code (as it offers a large community and efficient tools) as well as recent web tools (e.g. Angular) to provide user-friendly interfaces. See figure 30 for an overview of the functional architecture of our software.
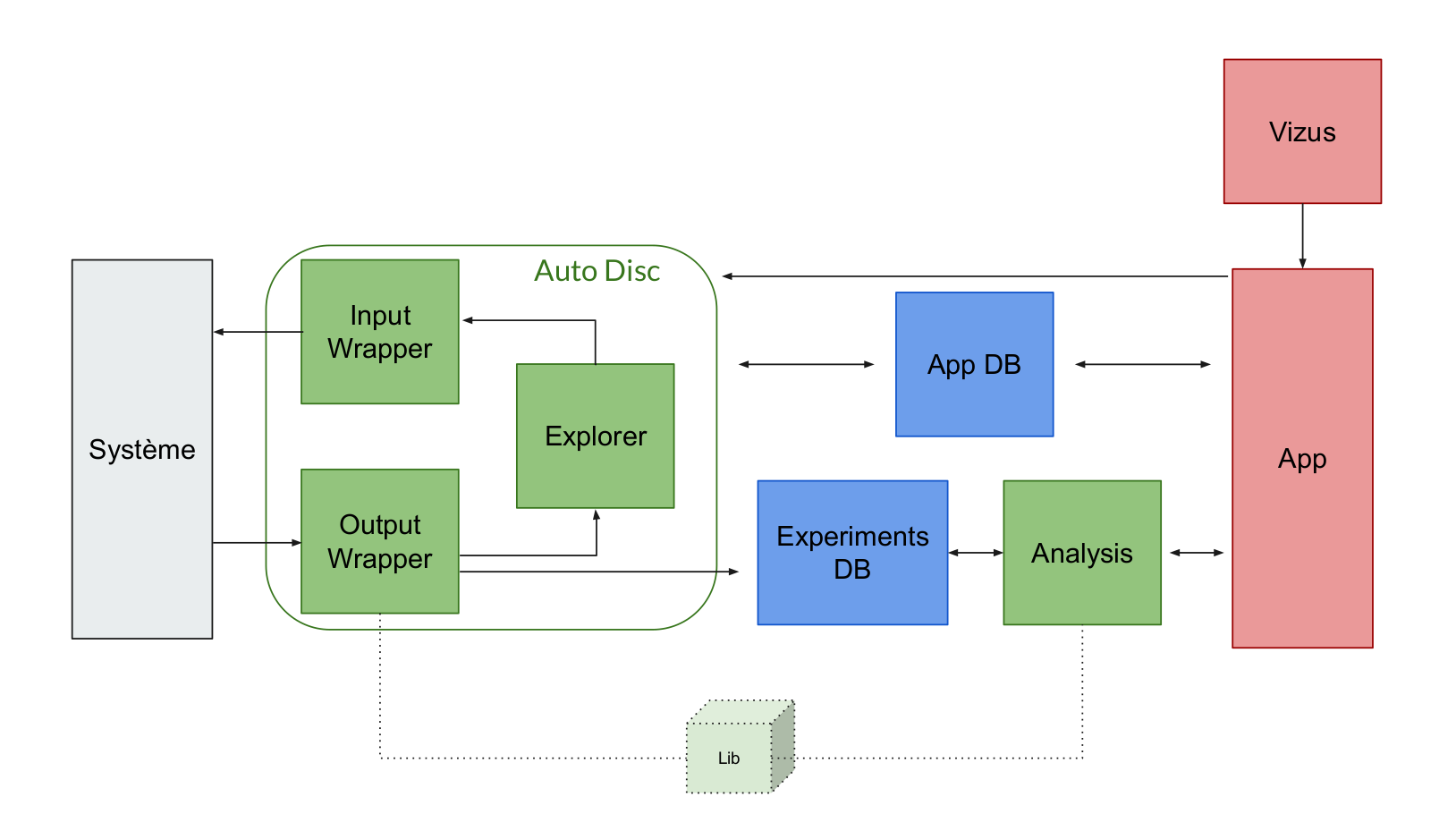
In the context of the project 8.7, we started a collaboration with Bert Chan, an independant researcher on Artificial Life and author of the Lenia system 80. During this collaboration, Bert Chan will help us design versions of IMGEP usable by scientists (non ML-experts) end-users, which is the aim of project 8.7.4. Having himself created the Lenia system, he is highly-interested to use our algorithms to automatically explore the space of possible emerging structures and will provide valuable insights into end-user habits and concerns. Additionally, we will be working with him to expand the set of discoveries of possible structures in continuous CAs, as a continuation of the project 8.7.2.
8.8 Tools for Understanding Deep Learning Systems
8.8.1 Explainable Deep Learning
Participants: Natalia Díaz Rodríguez, Adrien Bennetot.
Together with Segula Technologies and Sorbonne Université, ENSTA Paris has been working on eXplainable Artificial Intelligence (XAI) in order to make machine learning more interpretable. While opaque decision systems such as Deep Neural Networks have great generalization and prediction skills, their functioning does not allow obtaining detailed explanations of their behaviour. The objective is to fight the trade-off between performance and explainability by combining connectionist and symbolic paradigms 74.
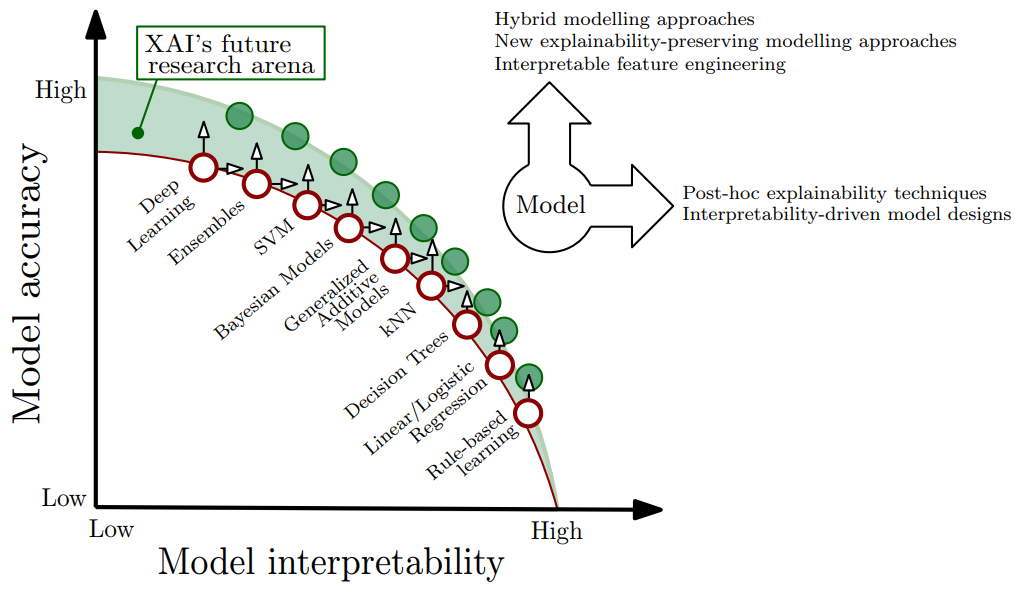
Broad consensus exists on the importance of interpretability for AI models. However, since the domain has only recently become popular, there is no collective agreement on the different definitions and challenges that constitute XAI. The first step is therefore to summarize previous efforts made in this field. We presented a taxonomy of XAI techniques in 19 and we are currently working on a prediction model that generates itself an explanation of its rationale in natural language while keeping performance as close as possible to the the state of the art 74.
8.8.2 Knowledge engineering tools for neural-symbolic learning
Participants: Natalia Díaz Rodríguez, Adrien Bennetot.
Symbolic artificial intelligence methods are experiencing a come-back in order to provide deep representation methods the explainability they lack. In this area, a survey on RDF stores to handle ontology-based triple databases has been contributed 106, as well as the use of neural-symbolic tools that aim at integrating both neural and symbolic representations 74.
8.8.3 Explainability in Deep Reinforcement Learning
Participants: Alexandre Heuillet, Fabien Couthouis, Natalia Díaz-Rodríguez.
A large set of the explainable Artificial Intelligence (XAI) literature is emerging on feature relevance techniques to explain a deep neural network (DNN) output or explaining models that ingest image source data. However, assessing how XAI techniques can help understand models beyond classification tasks, e.g. for reinforcement learning (RL), has not been extensively studied. With this project 26, we review recent works in the direction to attain Explainable Reinforcement Learning (XRL), a relatively new subfield of Explainable Artificial Intelligence, intended to be used in general public applications, with diverse audiences, requiring ethical, responsible and trustable algorithms. In critical situations where it is essential to justify and explain the agent's behaviour, better explainability and interpretability of RL models could help gain scientific insight on the inner workings of what is still considered a black box. We evaluate mainly studies directly linking explainability to RL, and split these into two categories according to the way the explanations are generated: transparent algorithms and post-hoc explainaility. We also review the most prominent XAI works from the lenses of how they could potentially enlighten the further deployment of the latest advances in RL, in the demanding present and future of everyday problems. We published this review
8.8.4 Explainable Artificial Intelligence (XAI): Concepts, Taxonomies, Opportunities and Challenges toward Responsible AI
Participants: Alejandro Barredo Arrieta, Natalia Díaz-Rodríguez, Javier del Ser, Adrien Bennetot, Siham Tabik, Alberto Barbado, Salvador García, Sergio Gil-López, Daniel Molina, Richard Benjamins, Raja Chatila, Francisco Herrera.
In the last years, Artificial Intelligence (AI) has achieved a notable momentum that may deliver the best of expectations over many application sectors across the field. For this to occur, the entire community stands in front of the barrier of explainability, an inherent problem of AI techniques brought by sub-symbolism (e.g. ensembles or Deep Neural Networks) that were not present in the last hype of AI. Paradigms underlying this problem fall within the so-called eXplainable AI (XAI) field, which is acknowledged as a crucial feature for the practical deployment of AI models. This overview 19 examines the existing literature in the field of XAI, including a prospect toward what is yet to be reached. We summarize previous efforts to define explainability in Machine Learning, establishing a novel definition that covers prior conceptual propositions with a major focus on the audience for which explainability is sought. We then propose and discuss about a taxonomy of recent contributions related to the explainability of different Machine Learning models, including those aimed at Deep Learning methods for which a second taxonomy is built. This literature analysis serves as the background for a series of challenges faced by XAI, such as the crossroads between data fusion and explainability. Our prospects lead toward the concept of Responsible Artificial Intelligence, namely, a methodology for the large-scale implementation of AI methods in real organizations with fairness, model explainability and accountability at its core. Our ultimate goal is to provide newcomers to XAI with a reference material in order to stimulate future research advances, but also to encourage experts and professionals from other disciplines to embrace the benefits of AI in their activity sectors, without any prior bias for its lack of interpretability.
8.8.5 Interdisciplinary Research in Artificial Intelligence: Challenges and Opportunities
Participants: Remy Kusters, Dusan Misevic, Hugues Berry, Antoine Cully, Yann Le Cunff, Loic Dandoy, Natalia Díaz-Rodríguez, Marion Ficher, Jonathan Grizou, Alice Othmani, Themis Palpanas, Matthieu Komorowski, Patrick Loiseau, Clément Moulin-Frier, Santino Nanini, Daniele Quercia, Michele Sebag, Françoise Soulié Fogelman, Sofiane Taleb, Liubov Tupikina, Vaibhav Sahu, Jill-Jênn Vie, Fatima Wehbi.
The use of artificial intelligence (AI) in a variety of researchfields is speeding up multiple digital revolutions, from shifting paradigms in healthcare, precision medicine and wearable sensing, to public services and education offered to the masses around the world, to futurecities made optimally efficient by autonomous driving. When a revolution happens, the consequences are not obvious straight away, and to date, there is no uniformly adaptedframework to guide AI research to ensure a sustainable societal transition. To answer this need, here we analyze three key challenges to interdisciplinary AI research, and deliver three broad conclusions 27: 1) future development of AI should not only impact other scientific domains but should also take inspiration and benefit from other fields of science, 2) AI research must be accompanied by decision explainability, dataset bias transparency aswell as development of evaluation methodologies and creation of regulatory agencies toensure responsibility, and 3) AI education should receive more attention, efforts and innovation from the educational and scientific communities. Our analysis is of interest notonly to AI practitioners but also to other researchers and the general public as it offers ways to guide the emerging collaborations and interactions toward the most fruitful outcomes.
8.8.6 Accessible Cultural Heritage through Explainable Artificial Intelligence
Participants: Natalia Díaz-Rodríguez, Galena Pisoni.
Ethics Guidelines for Trustworthy AI advocate for AI technology that is, among other things, more inclusive. Explainable AI (XAI) aims at making state of the art opaque models more transparent, and defends AI-based outcomes endorsed with a rationale explanation, i.e., an explanation that has as target the non-technical users. XAI and Responsible AI principles defend the fact that the audience expertise should be included in the evaluation of explainable AI systems. However, AI has not yet reached all public and audiences , some of which may need it the most. One example of domain where accessibility has not much been influenced by the latest AI advances is cultural heritage. In this project 44, we propose including minorities as special user and evaluator of the latest XAI techniques. In order to define catalytic scenarios for collaboration and improved user experience, we pose some challenges and research questions yet to address by the latest AI models likely to be involved in such synergy.
8.9 Other
8.9.1 Machine Learning Optimization of Intervention Strategies for Epidemics
Participants: Cédric Colas, Clément Moulin-Frier, Pierre-Yves Oudeyer.
This project is a collaboration with the SISTM team from Inria Bordeaux. Modelling the dynamics of epidemics helps proposing control strategies based on pharmaceutical and non-pharmaceutical interventions (contact limitation, lock down, vaccination, etc). Hand-designing such strategies is not trivial because of the number of possible interventions and the difficulty to predict long-term effects. This task can be cast as an optimization problem where state-of-the-art machine learning algorithms such as deep reinforcement learning, might bring significant value. However, the specificity of each domain – epidemic modelling or solving optimization problem – requires strong collaborations between researchers from different fields of expertise.
This is why we introduce EpidemiOptim, a Python toolbox that facilitates collaborations between researchers in epidemiology and optimization. EpidemiOptim turns epidemiological models and cost functions into optimization problems via a standard interface commonly used by optimization practitioners (
OpenAI Gym
)—see Figure 32. Reinforcement learning algorithms based on Q-Learning with deep neural networks (
dqn
) and evolutionary algorithms (
nsga-ii
) are already implemented. We illustrate the use of EpidemiOptim to find optimal policies for dynamical on-off lock-down control under the optimization of death toll and economic recess using a Susceptible-Exposed-Infectious-Removed (
seir
) model for COVID-19.
Using EpidemiOptim and its interactive visualization platform in Jupyter notebooks, epidemiologists, optimization practitioners and others (e.g. economists) can easily compare epidemiological models, costs functions and optimization algorithms to address important choices to be made by health decision-makers. Trained models can be explored by experts and non-experts via a web interface. This led to a submission at the journal JAIR (under review) 57. This project also led to a web interface where users can interact with trained lock-down intervention strategies, look at their effects on a models of the COVID-19 epidemics and design their own intervention strategy: https://
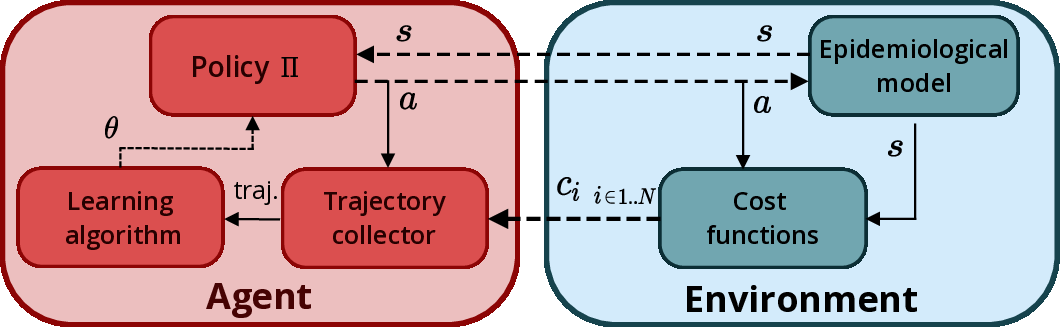
8.9.2 Applications in Robotic myoelectric prostheses
Participants: Pierre-Yves Oudeyer, Aymar de Rugy, Daniel Cattaert, Mick Sebastien.
Together with the Hybrid team at INCIA, CNRS (Sébastien Mick, Daniel Cattaert, Florent Paclet, Aymar de Rugy) and Pollen Robotics (Matthieu Lapeyre, Pierre Rouanet), the Flowers team continued to work on a project related to the design and study of myoelectric robotic prosthesis. The ultimate goal of this project is to enable an amputee to produce natural movements with a robotic prosthetic arm (open-source, cheap, easily reconfigurable, and that can learn the particularities/preferences of each user). This will be achieved by 1) using the natural mapping between neural (muscle) activity and limb movements in healthy users, 2) developing a low-cost, modular robotic prosthetic arm and 3) enabling the user and the prosthesis to co-adapt to each other, using machine learning and error signals from the brain, with incremental learning algorithms inspired from the field of developmental and human-robot interaction.
Biological Plausibility of Arm Postures Influences the Controllability of Robotic Arm Teleoperation
We investigated how participants controlling a humanoid robotic arm's 3D endpoint position by moving their own hand are influenced by the robot's postures. We hypothesized that control would be facilitated (impeded) by biologically plausible (implausible) postures of the robot. Background: Kinematic redundancy, whereby different arm postures achieve the same goal, is such that a robotic arm or prosthesis could theoretically be controlled with less signals than constitutive joints. However, congruency between a robot's motion and our own is known to interfere with movement production. Hence, we expect the human-likeness of a robotic arm's postures during endpoint teleoperation to influence controllability. Method: Twenty-two able-bodied participants performed a target-reaching task with a robotic arm whose endpoint's 3D position was controlled by moving their own hand. They completed a two-condition experiment corresponding to the robot displaying either biologically plausible or implausible postures. Results: Upon initial practice in the experiment's first part, endpoint trajectories were faster and shorter when the robot displayed human-like postures. However, these effects did not persist in the second part, where performance with implausible postures appeared to have benefited from initial practice with plausible ones. Conclusion: Humanoid robotic arm endpoint control is impaired by biologically implausible joint coordinations during initial familiarization but not afterwards, suggesting that the human-likeness of a robot's postures is more critical for control in this initial period. Application: These findings provide insight for the design of robotic arm teleoperation and prosthesis control schemes, in order to favor better familiarization and control from their users. This work was published in citemick:hal-03001362.
8.9.3 Traffic agent motion prediction
Participants: David Filliat, Vyshakh Palli Thazha.
For a vehicle to navigate autonomously, it needs to perceive its surroundings and estimate the future state of the relevant traffic-agents with which it might interact as it navigates across public road networks. Predicting the future state of the perceived entities is a challenge, as these might appear to move in a stochastic manner. However, their motion is constrained to an extent by context, in particular the road network structure. Conventional machine learning methods are mainly trained using data from the perceived entities without considering roads, as a result trajectory prediction is difficult. In this paper, the notion of maps representing the road structure are included into the machine learning process. For this purpose, 3D LiDAR points and maps in the form of binary masks are used. These are used on a recurrent artificial neural network, the LSTM encoder-decoder based architecture to predict the motion of the interacting traffic agents. A comparison between the proposed solution with one that is only sensor driven (LiDAR) is included. For this purpose, NuScenes dataset is utilised, that includes annotated 3D point clouds. The results have demonstrated the importance of context to enhance our prediction performance as well as the capability of our machine learning framework to incorporate map information.
Our results were published at the 2020 VTC conference 49
8.9.4 Egoshots, an ego-vision life-logging dataset and semantic fidelity metric to evaluate diversity in image captioning models
Participants: Pranav Agarwal, Alejandro Betancourt, Vana Panagiotou, Natalia Díaz-Rodríguez.
Image captioning models have been able to generate grammatically correct and human understandable sentences. However most of the captions convey limited information as the model used is trained on datasets that do not caption all possible objects existing in everyday life. Due to this lack of prior information most of the captions are biased to only a few objects present in the scene, hence limiting their usage in daily life. In this paper 39, we attempt to show the biased nature of the currently existing image captioning models and present a new image captioning dataset, Egoshots, consisting of 978 real life images with no captions. We further exploit the state of the art pre-trained image captioning and object recognition networks to annotate our images and show the limitations of existing works. Furthermore , in order to evaluate the quality of the generated captions, we propose a new image captioning metric, object based Semantic Fidelity (SF). Existing image captioning metrics can evaluate a caption only in the presence of their corresponding annotations; however, SF allows evaluating captions generated for images without annotations, making it highly useful for real life generated captions.
8.9.5 RobotDrlSim: A real time robot simulation platform for reinforcement learning and human interactive demonstration learning
Participants: Te Sun, Liang Gong, Xvdong Li, Shenghan Xie, Zhaorun Chen, Qizi Hu, David Filliat.
Deep reinforcement learning (DRL) techniques give robotics research an AI boost in many applications. In order to simultaneously accommodate the complex robotic behaviour simulation and DRL algorithm verification, a new simulation platform, namely the RobotDrlSim, is proposed 51. First, we design a standardized API interfacing mechanism for coordinating diverse environments on RobotDrlSim platform, where PyBullet simulator is equipped with an API to form a physical engine for robotics simulation. Second, benchmark DRL models are included in the baseline library for evaluation. Third, real-time human-robot interactions can be captured and imported to drive the RobotDrlSim tasks, which provide big data-stream for reinforcement learning. Experimentations show that cutting-edge DRL algorithms developed in python can be seamlessly deployed to the robots, and human interactions can be availed in training the robots. RobotDrlSim is valid for efficiently developing DRL algorithms for artificial intelligence models of robots, and it is especially suitable for the robot educational purposes.
9 Bilateral contracts and grants with industry
9.1 Bilateral contracts with industry
Autonomous Driving Commuter Car (Renault)
Participants: David Filliat, Emmanuel Battesti.
We developed planning algorithms for a autonomous electric car for Renault SAS in the continuation of the previous ADCC project. We improved our planning algorithm in order to go toward navigation on open roads, in particular with the ability to reach higher speed than previously possible, deal with more road intersection case (roundabouts), and with multiple lane roads (overtake, insertion...).
9.2 Bilateral grants with industry
Curiosity-driven Learning Algorithms for Exploration of Video Game Environments (Ubisoft)
Participants: Pierre-Yves Oudeyer.
Financing of a postdoc grant for a 2 year project with Ubisoft and Région Aquitaine.
Intrinsically Motivated Exploration for Lifelong Deep Reinforcement Learning in the Malmo Environment (Microsoft)
Participants: Pierre-Yves Oudeyer, Remy Portelas.
Financing of the PhD grant of Rémy Portelas by Microsoft Research.
Explainable continual learning for autonomous driving (Segula Technologies)
Participants: Natalia Díaz Rodríguez, Adrien Bennetot.
Financing of the CIFRE PhD grant of Adrien Bennetot by Segula Technologies.
Automated Discovery of Self-Organized Structures (Poïetis)
Participants: Pierre-Yves Oudeyer, Mayalen Etcheverry.
Financing of the CIFRE PhD grant of Mayalen Etcheverry by Poietis.
Machine learning for adaptive cognitive training (OnePoint)
Participants: Hélène Sauzéon, Pierre-Yves Oudeyer, Maxime Adolph.
Financing (in progress) of the CIFRE PhD grant of Maxime Adolph by Onepoint.
Curiosity-driven interaction system for learning (evidenceB)
Participants: Hélène Sauzéon, Pierre-Yves Oudeyer, Rania Abdelghani.
Financing of the CIFRE PhD grant of Rania Adolph by EvidenceB.
Perception Techniques and Sensor Fusion for Level 4 Autonomous Vehicles (Renault)
Participants: David Filliat, Vyshakh Palli-Thazha.
Financing of the CIFRE PhD grant of Vyshakh Palli-Thazha by Renault.
Exploration of reinforcement learning algorithms for drone visual perception and control (CEA)
Participants: David Filliat, Florence Carton.
Financing of the CIFRE PhD grant of Florence Carton by CEA.
Incremental learning for sensori-motor control (Softbank Robotics)
Participants: David Filliat, Hugo Caselles Dupré.
Financing of the CIFRE PhD grant of Hugo Caselles-Dupré by Softbank Robotics.
9.3 Bilateral Grants with Fundation
School+ project (FIRAH)
Participants: Hélène Sauzéon, Cécile Mazon.
Financing of one year-postdoctoral position (recruitment in progress) and the app. development by the International Foundation for Applied Research on Disability (FIRAH). The School+ project consists of a set of educational technologies to promote inclusion for children with Autism Spectrum Disorder (ASD). School+ primary aims at encouraging the acquisition of socio-adaptive behaviours at school while promoting self-determination (intrinsic motivation), and has been created according to the methods of the User-Centred Design (UCD). Requested by the stakeholders (child, parent, teachers, and clinicians) of school inclusion, Flowers team works to the adding of an interactive tool for a collaborative and shared monitoring of school inclusion of each child with ASD. This new app will be assessed in terms of user experience (usability and elicited intrinsic motivation), self-efficacy of each stakeholder and educational benefit for child. This project includes the Academie de Bordeaux –Nouvelle Aquitaine, the CRA (Health Center for ASD in Aquitania), and the ARI association.
10 Partnerships and cooperations
10.1 International initiatives
10.1.1 Inria international partners
Declared Inria international partners
Idex Bordeaux-Univ. Waterloo
- Title: Curiosity-driven learning and personalized (re-)education technologies across the lifespan
- International Partner (Institution - Laboratory - Researcher): University of Waterloo (Canada), Edith Law's HCI Lab and Dana Kulic's Robotics lab.
- Start year: 2018
- Pierre-Yves Oudeyer, Hélène Sauzéon and Fabien Lotte collaborated with Edith Law's and Myra Fernandes's research groups at University of Waterloo on the topic of "Curiosity in HCI system". They obtained a grant from Univ. Bordeaux and Univ. Waterloo. They organized several cross visits and collaborated on the design and experimentation of an educational interactive robotic system to foster curiosity-driven learning. This led to two articles accepted at CHI 2019 and CHI2020 (see new results section).
Informal international partners
Didier Roy and PY Oudeyer have created a collaboration with LSRO EPFL and Pr Francesco Mondada, about Robotics and education.
Didier Roy has created a collaboration with HEP Vaud (Teachers High School) and Bernard Baumberger and Morgane Chevalier, about Robotics and education. Scientific discussions and shared professional training.
Didier Roy has created a collaboration with Biorob - EPFL, LEARN - EPFL, and Canton de Vaud, about Robotics and Computer Science education. Scientific discussions and shared professional training.
PY Oudeyer and H Sauzéon started a collaboration with Daphne Bavelliers's research group at the University of Geneva on using machine learning for personalizing exercises in attention training educational software.
PY Oudeyer started a collaboration with Maxime Gasse (MILA, Montreal, Canada), Damien Grasset and Guillaume Gaudron (IRT Saint-Exupery, Toulouse), in the context of the project DEEL, on causal theory and reinforccement learning.
10.2 International research visitors
10.2.1 Visits of international scientists
Kevvyn Collins-Thompson, Univ. Michigan.
10.2.2 Invited talks
Germán Kruszewski (Facebook AI Research, Title: "The quest for compositional learning"), Guillermo Valle (Univ. Oxford, UK; Title: "Simplicity bias and generalization in deep neural networks"), Ferran Alet (MIT, US, Title: "Meta-learning curiosity algorithms"), Solande Denerveaud (Univ. Geneva, Switzerland; Title: "Error monitoring during learning: Neural and behavioral comparison studies of Montessori and traditionally-schooled students"), Hugo Cisneros (CIIRC, CTU in Prague, Title: "Artificial evolution and emergence in complex systems"), Remy van Trijt (Sony CSL Paris, France, Title: "Fluid Construction Grammar").
10.3 National initiatives
Myoelectric prosthesis - PEPS CNRS
PY Oudeyer collaborated with Aymar de Rugy, Daniel Cattaert, Mathilde Couraud, Sébastien Mick and Florent Paclet (INCIA, CNRS/Univ. Bordeaux) about the design of myoelectric robotic prostheses based on the Poppy platform, on the design of algorithms for co-adaptation learning between the human user and the prosthesis, and on the use of goal exploration algorithm to study the behaviour of models of neuromuscular systems. This was funded by a PEPS CNRS grant.
ANR JCJC ECOCURL
C Moulin-Frier obtained an ANR JCJC grant. The project is entitled "ECOCURL: Emergent communication through curiosity-driven multi-agent reinforcement learning". The project starts in Feb 2021 for a duration of 48 months. It will fund a PhD student (36 months) and a Research Engineer (18 months) as well as 4 Master internships (one per year).
Inria Exploratory Action ORIGINS
Clément Moulin-Frier obtained an Exploratory Action from Inria. The project is entitled "ORIGINS: Grounding artificial intelligence in the origins of human behavior". The project starts in October 2020 for a duration of 24 months. It funds a post-doc position (24 months). Eleni Nisioti has been recruited on this grant.
Inria Exploratory Action AIDE
Didier Roy is collaborator of the Inria Exploratory Action AIDE "Artificial Intelligence Devoted to Education", ported by Frédéric Alexandre (Inria Mnemosyne Project-Team), Margarida Romero (LINE Lab) and Thierry Viéville (Inria Mnemosyne Project-Team, LINE Lab). The aim of this Exploratory Action consists to explore to what extent approaches or methods from cognitive neuroscience, linked to machine learning and knowledge representation, could help to better formalize human learning as studied in educational sciences. AIDE is a four year project started middle 2020 until 2024.
https://
Poppy Station structure
-
Poppy Station Project : D. Roy, P.-Y. Oudeyer. This project aim to perpetuate the Poppy robot ecosystem by creating an external structure from outside Inria, with various partners.
After the Poppy Robot Project, the Poppy Education Project has ended and Poppy Station structure is born.
PerPoppy is the project which is building the new structure, and Poppy Station is the name of the new structure.
Poppy Station, which includes Poppy robot ecosystem (hardware, software, community) from the beginning, is a place of excellence to build future educational robots and to design pedagogical activities to teach computer science, robotics and Artificial Intelligence.
https://
www. poppy-station. org - Partners of Poppy Station : Inria, La Ligue de l’Enseignement, HESAM Université, IFÉ-ENS Lyon, MOBOTS – EPFL, Génération Robots, Pollen Robotics, KONEXInc, Mobsya, CERN Microclub, LINE Lab (Université Nice), Stripes, Canopé Martinique, Rights Tech Women, Editions Nathan.
10.3.1 Adaptiv'Math
- Adaptiv'Math
- Program: PIA
- Duration: 2019 - 2020
- Coordinator: EvidenceB
-
Partners:
- EvidenceB
- Nathan
- APMEP
- LIP6
- INRIA
- ISOGRAD
- Daesign
- Schoolab
- BlueFrog
The solution Adaptiv'Math comes from an innovation partnership for the development of a pedagogical assistant based on artificial intelligence. This partnership is realized in the context of a call for projects from the Ministry of Education to develop a pedagogical plateform to propose and manage mathematical activities intended for teachers and students of cycle 2. The role of Flowers team is to work on the AI of the proposed solution to personalize the pedagogical content to each student. This contribution is based on the work done during the Kidlearn Project and the thesis of Benjamin Clement 82, in which algorithms have been developed to manage and personalize sequence of pedagogical activities. One of the main goal of the team here is to transfer technologies developed in the team in a project with the perspective of industrial scaling.
10.4 Regional initiatives
Clément Moulin-Frier has started a project with InriaTech and the startup Gloo, located in Bordeaux.
Inria Cordi PhD grant
Julius Taylor, supervised by Clément Moulin-Frier, obtained an Inria Cordi PhD grant. He started his PhD thesis in November 2020 on model-based emergent communication in multi-agent reinforcement learning.
Inria - Region Post-doctoral grant - Call 2020 Region of New Aquitania
Hélène Sauzéon and Clément Moulin-Frier obtained an post-doctoral grant for the project entitled " Personalized Intelligent Tutorial Systems (ITS) for attention training: Modelling of personalization algorithms and effectiveness study" Masataka Sawayama started his postdoctoral position in Janvier 2021
11 Dissemination
11.1 Promoting scientific activities
11.1.1 Scientific events: organisation
Member of the organizing committees
Clément Moulin-Frier has co-organized the 1st SMILES (Sensorimotor Interaction, Language and Embodiment of Symbols) workshop at ICDL 2020, Nov 2020, Valparaiso / Virtual, Chile. https://
11.1.2 Scientific events: selection
Member of the conference program committees
PY Oudeyer was member of the program committee for ICLR, AAAI, Neurips.
Reviewer
Clément Moulin-Frier has reviewed for the ICRA conference.
Cédric Colas has reviewed for the ICML, ICLR and NeurIPS conferences.
PY Oudeyer was a reviewer for ICLR, AAAI, Neurips.
Didier Roy was a reviewer for PRUNE Conference (Poitiers) and RNRE (IFE ENS Lyon).
11.1.3 Journal
Member of the editorial boards
PY Oudeyer was member of the editorial board of: IEEE Transactions on Cognitive and Developmental Systems and Frontiers in Neurorobotics.
PY Oudeyer was co-editor of a Research Topic on "Modeling Play in Early Infant Development" in Frontiers in Neurorobotics 31, as well as of a Research Topic on "Intrinsically Motivated Open-Ended Learning in Autonomous Robots" in Frontiers in Neurorobotics 30.
Clément Moulin-Frier is co-editing a Research Topics in Frontiers:
Emergent Behavior in Animal-inspired Robotics
. https://
Reviewer - reviewing activities
Clément Moulin-Frier has reviewed for Journal of Artificial Intelligence Research (JAIR)
Mayalen Etcheverry has reviewed for the Applied Intelligence (APIN) journal.
Rémy Portelas has reviewed for the IEEE Robotics and Automation Letters (RA-L) and the KI – Künstliche Intelligenz journal.
PY Oudeyer reviewed for the journals: IEEE Transactions on Cognitive and Developmental Systems, Journal of the Royal Society Interface, Child Development, Frontiers in Psychology, Handbook of Computational Psychology, Motivation and Emotion
11.1.4 Invited talks
Cédric Colas has given an invited talk on the EpidemiOptim project at DeepMind, in the context of an internal seminar.
PY Oudeyer gave a keynote talk at the EGC conference in Brussels, on developmental machine learning, Jan. 2020, https://
PY Oudeyer gave an invited talk at the Deep RL Workshop of Neurips 2020, on intrinsically motivated goal-conditioned reinforcement learngin, Dec. 2020, https://
PY Oudeyer gave an invited seminar at the MIT embodied AI seminar, on developmental machine learning, Deep RL and artificial curiosity, April 2020, https://
PY Oudeyer gave a keynote talk at the Crossmodal Learning Center Autumn School, on Developmental Machine Learning, Curiosity and Deep RL, Dec. 2020. https://
PY Oudeyer gave an invited talk at the Brain and Cognition seminar at the University of Geneva, on Curiosity-driven learning in humans and mahcines, Oct. 2020. https://
Didier Roy has given invited talks at Adaptiv'math project webinar, Class'code AI webinar, EPFL learning sciences conference, on Flowers researches, on AI for education and education to AI.
Didier Roy has given invited talks at Réseau Canopé "Mardis du numérique" at Toulon, on computer science basics and activities to teach computer science, robotics and AI.
Didier Roy has given invited talks at IFE ENS Lyon RNRE Conference.
Didier Roy was invited to participate to the CIDREE European Expert Meeting at IFE ENS Lyon. http://
11.1.5 Leadership within the scientific community
PY Oudeyer was editor of the Cognitive and Developmental Systems newsletter of the Cognitive and Developmental Systems Technical Committee of the IEEE CIS Society
PY Oudeyer was elected as Distinguised speaker of the IEEE Computational Ingelligence Society
11.1.6 Scientific expertise
PY Oudeyer was a reviewer for the European Commission (FET program), and the ANR.
11.1.7 Research administration
PY Oudeyer has been member of piloting committees of consortium projects Adaptiv'Maths and Perseverons (eFran) on educational technologies.
11.2 Teaching - Supervision - Juries
11.2.1 Teaching
PY Oudeyer gave a course on developmental reinforcement learning at ENSEIRB master on AI and machine learning (3h), nov. 2019.
PY Oudeyer gave a course on developmental learning at CogMaster cognitive science master (8h), nov. 2019.
PY Oudeyer gave a course on developmental learning at ENSC/ENSEIRB "option robot" master (3h), dec. 2019.
During the latest academic year, Hélène Sauzéon teached 96h in the BS. and master degrees in cognitive science (Department of Mathematics & interaction, University of Bordeaux). She was (co-)responsible of 9 teaching units (3 in BS et 6 in Master).
N Díaz Rodríguez taught, at ENSTA, a total of 3.25 h in ROB313, 27h at IN104, 10.5 at IN102, 21h at IA301. She also gave 42h at IG.2410 at the engineering school ISEP, and 3h course on Continual Learning and State Representation Learning at the reinforcement learning course at ENSEIRB master on AI and machine learning (3h), nov. 2019.
Rémy Portelas and Tristan Karch gave a first year introductory course on programming at Université de Bordeaux (64h), sep. 2020 to jan. 2021.
Didier Roy gave courses on computer science basics, and on computer science, robotics and AI activities for education at Canton de Vaud teachers.
Clément Moulin-Frier gave courses on Robotics and AI at University Pompeu Fabra (Barcelona, Spain, Jan 2020, 10 hours) and Centre de Recherches Interdisciplaires (Paris, France, Apr 2020, 12 hours).
Maxime Adolphe gave courses on basics of AI (18h) at Ecole Nationale Supérieure de Cognitique (ENSC), sep. 2020 to jan.2021.
11.2.2 Supervision
- PhD defended: Cécile Mazon, "Des Technologies Numériques Pour L’inclusion Scolaire Des Collégiens Avec TSA : des approches individuelles aux approches écosystémiques pour soutenir l’individu et ses aidants ", University of Bordeaux (supervised by H. Sauzéon).
- PhD defended: Pierre-Antoine Cinquin, "Conception, intégration et validation de systèmes numériques d'enseignement accessibles aux personnes en situation de handicap cognitif ", University of Bordeaux (supervised by H. Sauzéon & P. Guitton).
- PhD in progress : Rémy Portelas, "Teacher algorithms for curriculum learning in Deep RL", beg. in sept. 2018 (supervisors: PY Oudeyer and K Hoffmann)
- PhD in progress: Cédric Colas, "Intrinsically Motivated Deep RL", beg. in sept. 2017 (supervisors: PY Oudeyer and O Sigaud)
- PhD in progress: Tristan Karch, "Language acquisition in curiosity-driven Deep RL", beg. in sept. 2019 (supervisors: PY Oudeyer and C Moulin-Frier)
- PhD in progress: Alexander Ten, "Models of human curiosity-driven learning and exploration", beg. in sept. 2018 (supervisors: PY. Oudeyer and J. Gottlieb)
- PhD in progress: Laetitia Teodorescu, "Graph Neural Networks in Curiosity-driven Exploring Agents", beg. in sept. 2020 (supervisors: PY. Oudeyer and K. Hoffman)
- PhD in progress: Maxime Adolphe, "Adaptive personalization in attention training systems", beg. in sept. 2020 (supervisors: H. Sauzéon and PY. Oudeyer)
- PhD in progress: Rania Abdelgani, "Fostering curiosity and meta-cognitive skills in educational technologies", beg. in dec. 2020 (supervisors: H. Sauzéon and PY. Oudeyer.
- PhD in progress: Julius Taylor, "Emergent communication through curiosity-driven multi-agent reinforcement learning", beg. in nov. 2020 (supervisor: C Moulin-Frier and PY Oudeyer)
- PhD in progress: Mayalen Etcheverry, "Automated Discovery of Self-Organized Structures", beg. in sept. 2020 (supervisor: PY Oudeyer)
- PhD in progress: Adrien Bennetot, "Explainable continual learning for autonomous driving", Sorbonne University and ENSTA Paris (supervised by N Díaz Rodríguez & R Chatila).
- Master thesis defended: Anouche Banikyian "Curiosity, intrinsic motivation and spatial learning in children", University of Bordeaux (supervised by H. Sauzéon).
- Master thesis defended: Mehdi Alaimi "New educational application for fostering curiosity-related question-asking in children", University of Bordeaux (supervised by H. Sauzéon & PY Oudeyer).
- Master thesis defended: Juewan Wang "Can an accessible MOOC player improve the retention of disabled students? A MOOC accessibility assessment based on analytic method ", University of Bordeaux (supervised by H. Sauzéon & P. Guitton).
- Master thesis defended: Valentin Villecroze "Emergence of communication in multi-agent systems", Ecole Polytechique (supervised by Clément Moulin-Frier).
- Master thesis defended: Younès Rabii "Conception d’un environnement de simulation écologiquement valide pour agents autonomes", ENS Cognitique Bordeaux (supervised by Clément Moulin-Frier).
- Master thesis defended: Clément Romac "Automated Curriculum Learning: a Benchmark" (co-supervised by R. Portelas and PY. Oudeyer)
- Master thesis defended: Laetitia Teodorescu, "SpatialSim: learning to recognize spatial configurations with graph-neural networks", Telecom ParisTech (supervisor: PY. Oudeyer)
- Cédric Colas supervised two ENSC students for their master 1 project on Artificial Intelligence .
11.2.3 Juries
PY Oudeyer was a member of the admissibility jury of the CR1 competition at Inria Bordeaux Sud-Ouest
PY Oudeyer was a reviewer in the PhD juries of Shoko Ota (OIST, Okinawa, Japant, Title: "Intrinsic Motivation in Creative Activity"), Japan; Benoit Choffin (Univ. Paris Saclay, Title: "Algorithmes d'espacement adaptatif de l'apprentissage pour l'optimisation de la maitrise a long terme de composante de connaissance"); Alexis Jacq (EPFL, Switzerland, Title: "Mutual understanding in educational human-robot collaborations"); Thomas Moerlan (Univ, Delft, Holland, Title: "The intersection of planning and learning").
PY Oudeyer was in the PhD "comité de suivi" of Ahmed Akazia (univ. Paris VI), Alexandre ChenuU (Univ. Paris VI), Sylvia Pagliarni (Univ. Bordeaux), Arash Rashidi (Univ. Bordeaxu), Effie Segas (Univ. Bordeaux)
Hélène Sauzéon organized a selection commitee for recruitment of Assistant professor in Rehabilitative science (University of Bordeaux).
Hélène Sauzéon was external member of a selection commitee for recruitment of Assistant professor in cognitive psychology (University of Toulouse – LeMirail).
Hélène Sauzéon performed several scientific expertises for application requests such as HDR (ED SP2, University of Bordeaux) or local careers advancement (University of Bordeaux).
N. Díaz Rodríguez was invited jury (President) of the PhD thesis "Deep Learning for Abnormal Movement Detection using Wearable Sensors: Case Studies on Stereotypical Motor Movements in Autism and Freezing of Gait in Parkinson's Disease" in the University of Trento, Italy May 2019.
C Moulin-Frier was in the PhD "comité de suivi" of Marc-Antoine Georges (Université Grenoble Alpes).
C Moulin-Frier was reviewer of the PhD of Sock Ching Low, entitled "Giving Centre Stage to Top-Down Inhibitory Mechanisms for Selective Attention", University Pompeu Fabra, Spain, Dec. 2020.
11.3 Popularization
11.3.1 Internal or external Inria responsibilities
Didier Roy was manager editor of a 370-pages computer science school textbook for kindergarten and elementary schools (collaboration Inria/EPFL/Canton de Vaud, Switzerland)
Didier Roy was manager editor of the EPFL MOOC "E-NUM", major contribution to train people, especially teachers, in computer science and digital sociology (collaboration Inria/EPFL/Canton de Vaud, Switzerland)
11.3.2 Articles and contents
Didier Roy and PY Oudeyer published an illustrated educational book on artificial intelligence and robotics for 7-8 years old children, Nathan, see https://
Didier Roy was one of the authors of the Inria White Paper "Education and Digital, Challenges and Issues" https://
Didier Roy was interviewed by Jérémy Dres, which was reported in a chapter of his comic "Les défis de l'intelligence artificielle".
PY. Oudeyer and S. Forestier were interviewed and appeared in a video documentary on Netflix, called "Babies", on models of infant development and curiosity-driven learning, https://
PY Oudeyer was interviewed by S. Paoli, which was reported in a chapter of the book "Ce qui vient", http://
H. Sauzéon was interviewed to present her research activitivies on a large-audience blog post on https://
H. Sauzéon was interviewed to present the results of the AIANA educational software to a large public on https://
C Moulin-Frier and L Chevillot wrote a large-audience web article on the new Exploratory Action ORIGINS: Grounding Artificial Intelligence in the Origins of Human Behavior. https://
Cédric Colas helped design a web interface for the EpidemiOptim project. Users can interact with lock-down intervention strategies trained with machine learning to mitigate health and economic costs in the context of simulated COVID-19 epidemics https://
Mayalen Etcheverry wrote an interactive blogpost on the paper of Reinke et al. (2020) "Intrinsically Motivated Discovery of Diverse Patterns in Self-Organizing Systems" published at ICLR 2020. https://
11.3.3 Education
Didier Roy has reviewed contents of the Class'code IAI MOOC.
11.3.4 Interventions
P.-Y. Oudeyer, B. Clément and L. Teodorescu made interventions as part of the "Le Procès du robot" animation at Cap Sciences. The goal was to present in layman's terms the research done at the lab for an audience of junior high school students and to foster discussion among them around an imagined scenario, about the legal responsibility of a domestic robot having caused a minor accient in a home. The web page of the intervention can be found there: https://
Pierre-Yves Oudeyer made several popular science interventions in Ecole Primaire AygueMarine (Ayguemorte-les-Graves), College de Cadillac (of which he is "parrain scientifique" in the context of "Maison des sciences").
12 Scientific production
Major publications
12.1 Publications of the year
International journals
National journals
Invited conferences
International peer-reviewed conferences
National peer-reviewed Conferences
Conferences without proceedings
Scientific books
Scientific book chapters
Edition (books, proceedings, special issue of a journal)
Doctoral dissertations and habilitation theses
Reports & preprints
Other scientific publications
12.2 Other
Scientific popularization
Educational activities
Patents
Softwares
Cited publications