2023Activity reportProject-TeamCOMPO
RNSR: 202124080M- Research center Inria Centre at Université Côte d'Azur
- In partnership with:INSERM, Aix-Marseille Université, CNRS, CAC4 MARSEILLE - Institut Paoli-Calmettes
- Team name: COMPutational pharmacology and clinical Oncology
- In collaboration with:Centre de Recherche en Cancérologie de Marseille
- Domain:Digital Health, Biology and Earth
- Theme:Computational Neuroscience and Medicine
Keywords
Computer Science and Digital Science
- A3.1.1. Modeling, representation
- A3.3.2. Data mining
- A3.3.3. Big data analysis
- A3.4.1. Supervised learning
- A3.4.2. Unsupervised learning
- A3.4.5. Bayesian methods
- A6.1.1. Continuous Modeling (PDE, ODE)
- A9.2. Machine learning
Other Research Topics and Application Domains
- B1.1.8. Mathematical biology
- B2.2.3. Cancer
- B2.4.1. Pharmaco kinetics and dynamics
- B2.4.2. Drug resistance
1 Team members, visitors, external collaborators
Research Scientists
- Sebastien Benzekry [Team leader, INRIA, Researcher, HDR]
- Joseph Ciccolini [Team leader, AMU APHM, PU-PH, HDR]
Faculty Members
- Dominique Barbolosi [AMU, Professor, HDR]
- David Boulate [APHM, Professor, PUPH, Thoracic surgeon, HDR]
- Raphaelle Fanciullino [AMU APHM, Associate Professor, MCU-PH, HDR]
- Florence Gattacceca [AMU, Associate Professor, HDR]
- Laurent Greillier [AMU, Professor, PU-PH, Head of the Pneumology, allergology and oncology department, HDR]
- Athanassios Iliadis [AMU, Emeritus, HDR]
- Xavier Muracciole [APHM, Hospital Staff, Radiotherapist]
- Anne Rodallec [AMU, Associate Professor]
- Sebastien Salas [APHM, PU-PH, Medical oncologist, HDR]
Post-Doctoral Fellows
- Paul Dufosse [AMU, Post-Doctoral Fellow]
- Patricia Piris [CNRS, University Paris-Sud, from Mar 2023]
PhD Students
- Celestin Bigarre [INRIA]
- Mohamed Boussena [AMU, from Dec 2023]
- Mathilde Dacos [APHM]
- Anthéa Deschamps [AMU]
- Hafida Hamdache [INSERM, from Oct 2023]
- Mourad Hamimed [AMU]
- Clemence Marin [APHP]
- Linh Nguyen Phuong [AMU]
- Loic Osanno [APHM]
- Jessica Ou [AMU]
- Guillaume Sicard [APHM]
Technical Staff
- Anastasiia Bakhmach [Inserm, Engineer, from Oct 2023]
- Mohamed Boussena [INRIA, Engineer, from Jun 2023 until Nov 2023]
- Sarah Giacometti [AMU, Technician]
- Melanie Karlsen [INSERM, Engineer, until Apr 2023]
- Sophie Marolleau [AMU, Engineer]
- Andréa Vaglio [AMU, Engineer, from Nov 2023]
Interns and Apprentices
- Anastasiia Bakhmach [GRENOBLE INP, Intern, from Feb 2023 until Jul 2023]
- Nastasia Cellier [INRIA, Intern, from Apr 2023 until May 2023]
- Tabea Gnade [Centrale Marseille, Intern, from Apr 2023 until Sep 2023]
- Govind Kallee [APHM, Intern]
- Mariam Mahdjoub [INRIA, Intern, from Mar 2023 until Sep 2023]
- Carla Perez [ERC]
- Dorian Protzenko [APHM, Intern]
- Antonin Ronda [APHM, Intern]
- Ruben Taieb [ENS DE LYON, Intern, from Oct 2023]
Administrative Assistant
- Sandrine Boute [INRIA]
Visiting Scientists
- Mahsa Dehghani Soufi [Univ Tabriz , from Oct 2023]
- Ana Homsek [University of Belgrade, from Oct 2023 until Oct 2023]
- Zeyad Ibrahim [Perkins Institute Perth Australia , from Oct 2023 until Oct 2023]
External Collaborators
- Alice Daumas [AMU, from Mar 2023]
- Nausicaa Malissen [APHM, Dermatologist, onco-dermatology department]
- Quentin Marcou [AMU, from Oct 2023]
- Laetitia Padovani [APHM, PU-PH, Head of the radiotherapy department, HDR]
- Amelie Pouchin [CHU LA TIMONE, from Jul 2023]
2 Overall objectives
We aim to optimize therapeutic approaches (i.e., controlling toxicities while ensuring a maximal efficacy) in oncology using mechanistic and statistical modeling (see Figure 1). These therapeutic approaches include immunotherapy, radiotherapy, chemotherapy, targeted therapies and their planification: combinations, sequences, intensification – densification, dosing and scheduling. Specifically, our research will be organized along three main axes:
- Quantitative modeling for personalized clinical oncology.
- Individualizing anticancer drugs regimen.
- Optimizing combinatorial strategies with immune checkpoint inhibitors.
Of note, in the Research Priorities document released by the American Society of Clinical Oncology in February 2021, “Developing and Integrating Artificial Intelligence in Cancer Research”, “Identifying Strategies That Predict Response and Resistance to Immunotherapies” and “Optimizing Multimodality Treatment for Solid Tumors” are listed as top-priorities, which fit quite well with our 3 axes.
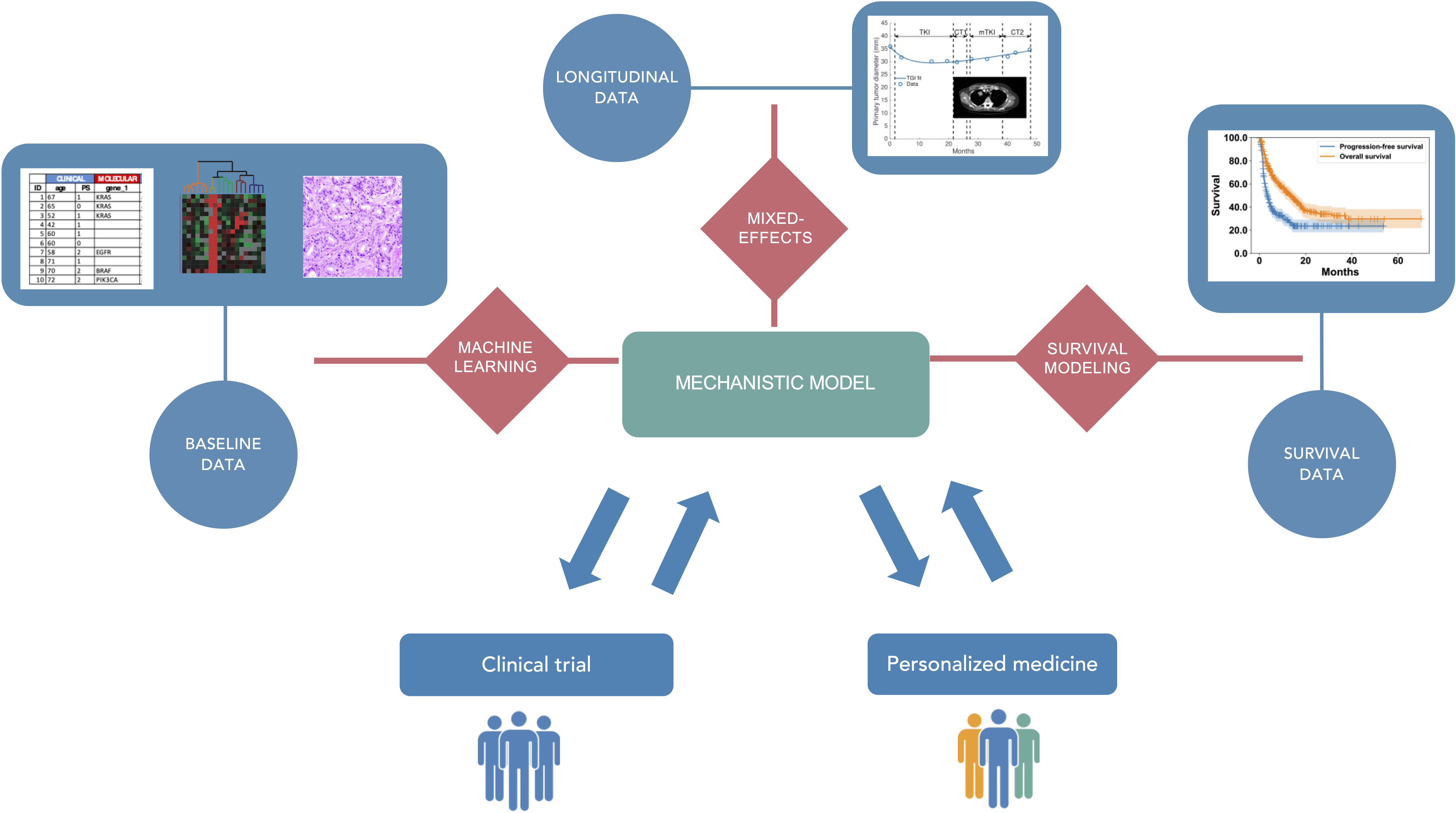
Mechanistic and statistical modeling for pharmacological and clinical oncology.
3 Research program
3.1 Scientific context and motivations
The project-team is based upon the development of model-driven clinical oncology as a means to optimize anticancer therapies. Despite continuous efforts to make available novel drugs beyond traditional cytotoxic chemotherapy (i.e., oral targeted therapies, biologics, immune checkpoint inhibitors), prognosis of outcome remains poor for many cancers. The dosing regimen of anticancer drugs given today remains largely empirical, because dose-finding studies are often performed using outdated, sub-optimal protocols (such as modified-Fibonacci dose-ranging protocols) or because concomitant administration is the rule when combining several drugs. Consequently, clinical oncologists struggle to refine the way they use the anticancer agents made at their disposal. For instance, it took several years of bedside practice to understand that paclitaxel in breast cancer patients should not be administrated using the officially approved 150 mg/m² every 3 weeks scheduling, but rather with an alternate 75 mg/m² weekly dosing 105. Similarly, multi-targets sunitinib is now given on a 50 mg two-weeks on / one-week off basis, rather than the officially approved four-weeks on / two-weeks off schedule 93. Elsewhere, several combinatorial strategies trials have failed to yield convincing results, mostly because of the lack of a strong rationale regarding the best way to sequence treatments 87. Globally, clinical oncology today is still all about finding the best way to treat patients ensuring an optimal efficacy / safety balance.
After having long been limited to cytotoxic chemotherapy (in addition to surgery and radiotherapy), the arsenal of anti-cancer agents has dramatically increased over the last two decades. Indeed, major advances in the understanding of cancer biology that occurred in 1) the discovery and quantification of (epi)genetic alterations leading to targeted therapy and 2) the realization of the importance of the non-cancer cell components of tumors, i.e., the tumor micro-environment and tumor immunity, have helped to identify novel targets. Drugs targeting the tumor vasculature (e.g., first-in-class bevacizumab, approved in the mid-2000’s 74) or tumor immunity (e.g., immune checkpoint inhibitors (ICI) such as first-in-class ipilimumab, approved in the early 2010’s 103) represent groundbreaking innovations in oncology. ICIs in particular are considered as game-changing drugs because diseases with once dismal prognosis (e.g., metastatic melanoma, non-small cell lung cancer (NSCLC), kidney cancer or head and neck cancer 125) now show 20-40% of 5-years survival. Nevertheless, these impressive results are limited to a minority of patients in a limited number of cancers. In addition, no validated biomarker predictive of response has yet been identified, thus highlighting how early prediction of response and probability of future relapse are a critical, unmet medical need. The encouraging yet still insufficient clinical results of ICIs have led current clinical oncology to consider combinations of such immunotherapies with preexisting anti-cancer modalities: radiation therapy 112, cytotoxic 99, targeted 117 or anti-angiogenic therapies 124. However, the near-infinite possibilities of combinations in terms of sequencing, dosing and scheduling challenge the ability of classical trial-and-error methods to find appropriate modes of combination 86.
In addition, day-to-day clinical decisions made by oncologists are based on a large amount of information, coming from: 1) their own knowledge integrating years of clinical practice combined to updated literature and 2) objective data coming from multiple sources (demographic data, radiology, functional imaging, molecular biology, histology, biomarkers, blood counts, etc…). The large amount of clinical and biological data generated now in clinical oncology is not properly analyzed, because of the lack of appropriate models to picture the complexity of longitudinal observations. Oncologists lack a comprehensive framework and numerical software that could support decision of therapeutic strategy (e.g., to treat or not? to what extent? with what treatment (surgery, radiotherapy, systemic therapy)? in what order? etc.), especially when their time dedicated to examination of a given patient case is limited (e.g., in multidisciplinary meetings (RCP), or individual consultations). Furthermore, modeling is the only way to retrieve similar characteristics from very different experimental conditions and clinical protocols.
To address these major issues, our project-team aims at:
- guiding anticancer therapy by developing patient-specific predictive models (individual level);
- better designing clinical trials, in particular regarding combinatorial trials (population level).
3.2 Data
We use non-clinical and clinical data related with the pharmacology of anti-cancer agents and medical monitoring of the disease status. The former includes pharmacokinetics (drug levels in plasma (patients) and full body pharmacokinetics (animal models)), pharmacodynamics (efficacy, safety), pharmacogenetics (i.e., constitutional genetic polymorphisms affecting drug transport and metabolizing enzymes), pharmacogenomics (i.e., molecular and genetic alterations affecting tumor cells). The latter include demographics, anatomical imaging (e.g., tumor sizes derived from CT scan or MRI), functional imaging (e.g., positron emission tomography), histopathology quantifications, biological variables (such as kidney and liver functions or blood counts), immuno-monitoring data (flow cytometry) or cell free DNA. We will especially rely on real-world data (also termed fragmentary data) collected from patient routine monitoring by our members with hospital activity.
Experimental data are generated by the experimental wet-lab group (involving COMPO team members: AR, RF, JC), relying on state-of-the-art experimental pharmacokinetic laboratory fully equipped to perform in vitro and in vivo explorations of drug metabolism, pharmacokinetics and experimental therapeutics in oncology, including bioanalytical support and fluorescence/bioluminescence monitoring in rodents with highly specialized staff. Clinical pharmacokinetics and pharmacogenetics data are generated by the clinical pharmacology group (JC, RF), relying on the expertise of the clinical pharmacokinetics laboratory of the La Timone University Hospital of Marseille, an FDA-labelled, ISO15189-labelled facility with state-of-the-art bioanalytical resources to assay any kind of drugs or drug metabolites in patients. Specific data regarding cancer biology and pharmacodynamics (immunomonitoring, pharmacogenomics) are generated in collaboration with other CRCM teams.
Clinical data and additional biomarker data are collected from either clinical trials or real-world studies performed by hospital pharmacists and oncologists of the joint-team (RF, JC, SS, LG, XM) and their residents, or by other medical oncologist partners. We have strong collaborations and ongoing projects with pediatrics (Pr N. Andre), hematology (Dr G. Venton), nuclear medicine (Pr D. Taïeb) and radiotherapy (Pr L. Padovani). Importantly, the project-team is located near the INCa-labeled center for early clinical trials (CLIP2), thus facilitating the data collection and later, the implementation of modeling approaches in early clinical trials.
In addition, we also rely on publicly available data from online databases such as the TCGA (genomic data), the TCIA (imaging data) or data from clinical trials.
3.3 Mathematical methodology
Our primary objective is centered on the improvement of therapeutic strategies in oncology. Nevertheless, this brings novel methodological challenges requiring developments at the formal level within the generic field of modeling biological and pharmaco-patho-physiological systems. Difficulties to take into account include: the longitudinal profile of the quantities of interest; measurement uncertainty (requiring statistical considerations); difficulties in sampling the real processes leading to scarcity of the observed data and large inter-individual variability. Many specific problems in life science systems are very different from that encountered from physical modeling in industrial applications (e.g., mechanical engineering or energy).
To summarize our methodology, we are interested in modeling the dynamics of pharmaco-oncological processes (mechanistic modeling) and their inter-individual variability (statistical modeling). Our intended methodological contributions are: 1) to invent novel mechanistic models for complex physiological processes able to describe the effect of therapeutic intervention, 2) to design appropriate statistical frameworks for parameter estimation and description of inter-individual variability, 3) to test and validate the models against experimental and clinical data, 4) to combine state-of-the art machine learning (ML) methods with mechanistic models to integrate large dimension data.
3.3.1 Mechanistic modeling
Mechanistic models are defined here as mathematical constructs that describe physiological variables (e.g., plasma drug concentration, tumor size, or biomarkers) and their dynamics based on physical and biological principles (e.g., law of mass conservation). They describe the time profiles of the variables of interest by means of ordinary or partial differential equations (ODEs and PDEs, respectively) and are thus deterministic.
The main challenge of the modeling exercise is to find the appropriate balance between the degree of integration of biological phenomena (model complexity) and granularity of the data available (i.e., sampling time resolution, observed variables, spatio-temporal or only temporal measurements) ensuring the feasibility of parameter estimation. Indeed, cancer biology is extremely complex, involving processes at multiple temporal and spatial scales (intra- and inter-cellular, tissular, organism). It is thus tempting to build intricate models integrating as many phenomena as possible. Along these lines, the last decades have witnessed the proliferation of multiple such complex models. We see two shortcomings to this approach. First, in contrast with models of physical phenomena, the parameters of biological models are often not directly measurable and thus have to be estimated from fitting the models to experimental or clinical data. Therefore, their number has to be commensurate to the available data in order to ensure identifiability. Unfortunately, many complex models from mathematical oncology have too many parameters to be reasonably identified and have thus had a limited application in terms of biological insights or clinical applications. Second, complex, multiscale models are characterized by a reductionist point-of-view whereby general phenomena could be explained by decomposing them into elementary pieces. However, corresponding elementary experiments would not be suitable for quantification of the several homeostatic mechanisms involved in the whole real process. Thereby, we do not adhere to this reductionist vision and for modeling purposes we rather adopt a holistic approach considering the process as an indivisible whole.
To avoid the above-mentioned caveats, our methodology always starts from: 1) a clinically relevant medical problem but more importantly 2) the data available to build models.
In several instances, the mechanistic models are ordinary differential equations (ODEs). This is the case for the simplest type of experimental data that we generate, i.e., tumor growth kinetics. Departing from previous works establishing models for untreated experimental growth, we are now actively engaged into designing pharmacokinetics (PK)/ pharmacodynamics (PD) models of the effect of multiple therapies. These models have to account for the specificities of the drug delivery (e.g., nanoparticles), the biological effect of the treatments (e.g., cytotoxics, antiangiogenics or immunotherapies) and resistance to the therapy (either innate or acquired). The resulting models are novel nonlinear ODEs that need to be validated against the data and, when necessary, theoretically studied for their qualitative behavior. With the advent of immunotherapies, there has been a regain of interest to modeling tumor-immune interactions. Again, despite a wide literature on the subject, very few models have been validated against empirical data. A methodological objective is to establish and validate such models, including effect of immunotherapies.
Description of other phenomena are more adapted to partial differential equations (PDE) models. For instance, following an approach initiated by Iwata et al. 108, structured PDE models can be written for description of a population of metastases (see 4.3.2). Indeed, at the organism scale, cancer diseases are often characterized by a generalized (metastatic) state. However, few modeling efforts are currently focused on this aspect. The only validated models in large cohorts for systemic disease concern the sum of largest diameters as defined by the RECIST criteria 83. We aim to go beyond this state of the art by: 1) providing models of coupled tumor growth with interactions (and quantification of inter-lesion variability) 79, 80 and more importantly 2) developing models accounting not only for growth of the tumors, but also dissemination (birth of new lesions).
To date, most of the available and collectable clinical data about tumor growth and response to therapy consist of scalar data, often even limited to lesion diameters or sum of diameters. This is why we primarily focus our efforts on developing kinetic models of such data, the novelty coming from integrating other longitudinal biological data (e.g., from blood counts). Nevertheless, imaging data are now increasingly accessible and recent advances in image analysis allow the automatic segmentation of lesions make it possible to quantify the spatial shape and texture of tumors without a prohibitive cost for radiologists. This opens the way to develop spatially distributed PDE models of tumor dynamics. The existing models have largely remained unconfronted to data, apart from notable exceptions from the Inria MONC 90 and EPIONE 88 teams, as well as the Swanson 75 and Yankeelov 104 groups. Radiomics approaches quantifying heterogeneity in the images could bring additional information. We will rely on existing or establish collaborations with other dedicated Inria teams (EPIONE, MONC) for such purpose. COMPO would ideally bridge the gap between clinical studies and the Inria ecosystem. Finally, PDE models are also well adapted to describe intra-tumor drug penetration and we have recently developed such models for description of intra-tumor fluid flow and transport of antibody nano-conjugates 126.
3.3.2 Statistical modeling
Statistical models are defined here as mathematical constructs that describe the stochastic sources of variability in the data. They comprise both: 1) classical statistical models defining the functional and probabilistic relationship explicitly and 2) machine learning (ML) algorithms highly based on the data alone (e.g., tree-based models and associated ensemble methods or support vector machines) 101. We use such models for the following purposes: defining appropriate frameworks for parameter estimation; quantitative testing of biological hypothesis; addressing interindividual variability (using nonlinear mixed-effects (NLME) modeling); and building predictive models.
NLME – also termed the population approach in PK/PD modeling, or hierarchical modeling 110 – consists in assuming a statistical distribution of the parameters of the structural (often mechanistic) model, in order to describe longitudinal observations within a population of individuals. Instead of estimating individual parameters on a subject per subject basis – leading to identifiability issues in sparse data situations characteristic of longitudinal measurements in oncology – all data can be pooled together and a joint likelihood is obtained. Likelihood maximization becomes more complex than for classical nonlinear regression, nevertheless this problem has already been addressed by means of algorithms such as the deterministic first-order conditional expansion (FOCE) algorithm 111 or the stochastic approximation of the expectation-maximization algorithm (SAEM) 92. These algorithms are implemented in widely used software in the PMX community such as NONMEM® (Icon) or Monolix® (Lixoft), or R packages (e.g., saemix or nlme). Once the population distribution is estimated, empirical Bayes estimates (EBEs) can be derived for estimation of individual parameters. We also use the language Stan that implements state-of-the art Bayesian methods 84.
Departing from a general distribution of the parameters (often assumed log-normal) with quantified but unexplained interindividual variability, covariates are incorporated to explain this variability and build predictive models. This is traditionally done by means of linear models (possibly up to a functional transformation). However, with the increase in number of such covariates, the traditional tools and algorithms are limited. We thus develop advanced covariate models in NLME incorporating ML algorithms. Such methods require novel contributions. A possible lead is to first identify the EBEs and then use ML algorithms to predict these from the covariates 118. In other cases, ensemble models could be built from the heterogeneous sources of data, integrating one sub-model from EBEs identified from early data. Another, more challenging, avenue would be to adapt the parameter estimation algorithms like SAEM to include ML models in the covariate part.
In addition, because few data have been available longitudinally so far (i.e., small number of quantities measured at each time point), the current use of NLME relies on models with a small number of output variables. In this respect, modern clinical oncology studies bring new modeling and statistical challenges because many more quantitative data are collected at each time point (e.g., hundreds of variables from immuno-monitoring or possibly tens of thousands from circulating DNA, or radiomics features from imaging). Defining high-dimensional ODE models describing all the physiologically meaningful variables becomes intractable, therefore new methods are required. A possible avenue is to have a sequential approach, using first ML methods to reduce the dimension, then model the reduced number of variables. Another, more challenging, avenue would be to perform the two tasks (dimension reduction and temporal modeling) at the same time, and include this in an NLME framework for population estimation. The first part could be done using tools from unsupervised learning such as auto-encoders.
Following the availability of longitudinal tumor measurements, recent developments in the field of NLME have concerned joint modeling 94. This consists in modeling the longitudinal kinetics of a biomarker (e.g., tumor size) together with censored time-to-event data (e.g., overall survival) in a single step. Promising results have been obtained so far and we intend to develop methods beyond the state-of-the art in this area. This includes, in connection with above: 1) extension to models with emergence of new metastases, 2) integration of high-dimensional covariates and 3) high-dimensional longitudinal data. This Bayesian integration of data for updated survival predictions could lead to high impact results, as demonstrated by a recent publication in Cell 109.
Finally, we intend to bring the use of established ML tools to address concrete clinical problems emerging from the data collected in routine or clinical trials. Indeed, such data is so far analyzed using traditional statistical methods. ML algorithms could bring added value for predicting efficacy or toxicity from demographic, clinical and biological data.
3.4 Experimental therapeutics in oncology
The project-team is based upon generating experimental and clinical data to identify and test the models, and to provide proof-of-concept studies so as to validate the model-based dosing and scheduling prior to transposing them in patients. Historically, experimental therapeutics in oncology has relied on a wide variety of in vitro and in vivo models mimicking human cancer disease. In oncology, hundreds of in vitro models using cancer cell lines cultivated following 2D or 3D (spheroids) fashion, plus more sophisticated models with cancer cells enriched with fibroblasts or endothelial cells 119, eventually leading to complex organoids 98. Similarly, almost all kind of tumors can be tested in vivo, mostly in small rodents. In oncology, in vivo models are mostly based upon xenografting human tumors from established cell lines or from patient biopsies (patient-derived xenografts or PDX) so as to better mimic human pharmacology when testing active compounds next. To achieve this, several strains of immune-compromised mice have been successfully developed. Because immune checkpoint inhibitors do not exert direct anti-proliferative activity on cancer cells but are rather expected to harness tumor immunity, human xenografts in immuno-compromised mice is not anymore a suitable model. This has led investigators to shift towards immune-competent syngeneic mice models. Non-clinical experiments with drug candidates in immunotherapy mostly focus on deciphering the pharmacology of the targeted pathways, assessing the cytokine release potential, studying receptor occupancy, by using models the most likely to mimic tumor immunity in human. More sophisticated animal models such as human knock-in mice, immuno-avatar, hemato-lymphoid humanized mice or immune-PDX mice have been developed 122 (i.e., allowing to test immune checkpoint inhibitors in mice models combining human xenograft with relevant, humanized immunity and stroma cells) have been made available as well. Beyond generating data on efficacy such as reduction in primary tumor mass or metastatic spreading, experimental models help providing as well in depth knowledge on human and animal target cells, in vitro and in vivo concentration-effect studies, search for biomarkers, plus the most comprehensive knowledge on animal vs. human differences on dose – exposure – effects relationships and finally drug distribution throughout the body, target expression, affinity of target-binding and intrinsic efficacy, duration and reversibility of the effects. In particular, animal drug metabolism and pharmacokinetics (i.e., exploration of liver metabolism and distribution / absorption processes using in vitro or in vivo dedicated models) help understanding the disposition and distribution of the drug in the body throughout time, especially its ability to target tumor tissues (i.e., in vivo distribution in tumor-bearing mice) and help understanding sources of pharmacokinetic variability. All this information requires state-of-the-art techniques for measuring drugs and drug metabolites into biological fluids in tissues, such as fluorescence-imaging, high-performance liquid chromatography or liquid-chromatography-mass spectrometry bioanalysis. Our team has proven track records in the field of experimental therapeutics in oncology, with two PhDs on developing anticancer nanoparticles in breast and colorectal cancer 97, 121 plus experiments on model-driven way to combine anti-angiogenics with cytotoxics in breast and lung cancers 107, 114, 123, model-driven determination of alternate dosing in neuroblastoma, or methodological studies on monitoring tumor growth 115.
3.5 Axis 1: Quantitative modeling for personalized clinical oncology
The different steps of patient therapeutic management by clinicians consist mostly of: diagnosis, estimation of the extension of the disease, choice of therapy and evaluation of the therapy (efficacy, toxicity). This axis is specifically concerned with such clinical problems, apart from the pharmacological aspects addressed in the other axes.
In this axis, we aim to develop mathematical and statistical models and methods able to process this information to bring added value by inferring hidden parameters and provide simulations and predictions about the past and future behavior of the disease.
In the short-term (4 years), our research projects are: (1) modeling large-scale longitudinal data from immuno-oncology for prediction of response to immune checkpoint inhibition (QUANTIC and TGI-ML projects), (2) developing clinically relevant mathematical models of metastasis and (3) modeling the kinetics of clinical biomarkers.
3.6 Axis 2: Individualizing anticancer drugs dosing regimen
This axis fits within the “population approach” introduced in the 80’s by L. Sheiner 121 and aims at gathering exhaustive information about the multiple sources for variability in response in patients (including but not limited to drug-drug interactions, pharmacogenetics, and cormorbidities affecting renal and liver functions), build specific mechanistic models including relevant covariates and determine the PK/PD relationships of drugs used in oncology-hematology or for treating solid tumors. This covers cytotoxics, oral targeted therapies, biologics or immune checkpoint inhibitors. The overall goal is to achieve precision and personalized drug administration, i.e., the right dosing and scheduling regimen for the right patient.
In the short-term (4 years), our research projects will be focused on the following objectives: 1) predict response or toxicity variability dependent on pharmacogenetic (PGx) and pharmacogenomic data, 2) assess PMX of anticancer agents such as biologics, including ICI, and 3) develop physiologically-based PK models of nanoparticles distribution. Together, these objectives will allow to gain insights in the variability in drug response that depends on PK (1, 2 and 3) or germinal genetic alterations (1).
3.7 Axis 3: Optimizing combinatorial strategies with immune checkpoint inhibitors
Our hypothesis is that so many attempts to combine drugs fail not because the underlying pharmacological concepts are wrong (such as immunogenic cell death triggered by cytotoxics or radiation therapy, or increase in T cells infiltration with anti-angiogenics) but because these combinations probably require fine tuning in terms of dosing, scheduling and sequencing, whereas in practice all the drugs are given the same day. The goal of this second axis is therefore to shift from current empirical and suboptimal combinatorial regimen to model-informed designs to best combine drugs and therapeutic approaches so as to maximize efficacy while controlling toxicities. To do so, we will rely on our pioneering work about model-driven scheduling in early phase trials for combination of cytotoxic agents in metastatic breast cancer (MODEL1 trial) 102, 113 and metronomic vinorelbine in lung cancers (MetroVino trial) 78, 96. Leveraging the unique multidisciplinary aspect of our team, we implement a fully translational approach going from experimental therapeutics, PMX and quantitative systems pharmacology, to clinical trials either in early (phase I/II) or late (phase III) settings. Of note, our group has already an expertise in developing mathematical models determining the best sequencing between chemotherapy and anti-angiogenics.
In the short-term (4 years), our research projects will be focused on providing model-informed designs for combining ICI with: (1) cytotoxics, (2) an experimental immunoliposome and (3) radiotherapy.
3.8 Mid-term objectives
3.8.1 Modeling
We plan to achieve two main things on the modeling side: 1) the development of effective numerical tools (either as web applications or as part of simulation software) and 2) the empirical validation of the models.
For 1), this includes a tool able to predict response to ICI monotherapy in NSCLC from baseline and early response data . We will work with our industrial partners (in particular, HalioDX from the PIONeeR consortium), to transfer the tool for commercial use. Second, we plan to have a validated numerical tool able to predict metastatic relapse from clinical biomarkers at diagnosis, using our mechanistic model. This model will integrate the effect of adjuvant therapy (hormonotherapy or cytotoxic therapy) and will be able to simulate the long-term impact of alternative treatments (e.g., number of cycles to be administered to prevent distant relapse). It will have been validated from our local databases and will be implemented in a clinically-usable online tool such as PREDICT 127. The main difference with this tool will be the ability to mechanistically simulate the effect of therapy. We plan to have initiated a larger initiative at the national or European level to collect large data bases, validate further the predictive power of the model, and refine its structure if required. We will also extend this tool to other pathologies that share the same problematic (diagnosis at early-stage, important probability of future distant relapse) such as kidney cancer, following our initial work from preclinical data 76, 81, 91.
The pharmacometric models that will have been developed will also be implemented as clinically effective dose adaptation numerical tools directly usable to personalize the dose and scheduling of multiple anti-cancer agents not only by the clinicians and pharmacists from our group, but also by others, at least at a regional level.
For 2), our strategy of validation is the following. First, during the development phase, a proportion of the dataset (usually, 30%) is left aside unused for establishment of the model and initial calibration, and then used as a test set. When the sample size is too small (n 100 patients), only (nested) cross-validation is employed to assess the predictive power of the model. Evaluation metrics will be the classical ones, adapted to the task (classification, regression or survival regression) and include discrimination and calibration. In addition, specific methods will be used when in the context of mixed-effects approach (e.g., visual predictive checks for evaluation at the population level). The second step consists in evaluating the predictive power of the models in retrospective, external data sets. We have for instance initiated a collaboration with Dr C. Scherer (Clermont-Ferrand) to validate our metastatic prediction model on an external database of 3061 patients. The third step is to validate the added value of the model-based approach compared with the standard of care and is a long-term rather than mid-term objective.
3.8.2 Pharmacological and clinical oncology
In axis 2, in addition to standard drugs, developing tools for similarly better understanding the sources of therapeutic and PK variability and understanding the PK/PD relationships of cell therapy in oncology such as CAR-T cell therapies, is a challenging task. The challenge with CAR-T cells is that first, developing bioanalytical tools to monitor them in patients is not trivial, and second, little but nothing is known regarding their PK properties and possible sources impacting on PK/PD relationships such as disease status or immune status of the patient. We aim at developing both a platform to monitor CAR-T cells and future cell therapies in plasma and mechanistic models to describe the disposition of these new therapies in the body.
The nanoPBPK model will be extrapolated to humans and used to determine the specifications of an optimal nanosystem in order to penetrate solid tumors such as pancreatic tumors. The rationally designed nanosystem will be evaluated in vitro and in vivo in order to validate the approach. The nanoPBPK model will be interfaced to become a software and be shared with the scientific community. To achieve this goal, a partnership with ESQlabs, the company developing the opensource PBPK platform PKSim, has already been approved by both sides. The nanoPBPK model will be combined with pharmacodynamic modeling describing the effect of the loaded anticancer drug on tumor growth and metastases spread, on the immune system, and on dose-limiting toxicities.
Our mid-term objective in axis 3 is to assist the design of scheduling regimen for combinatorial treatments in early phase clinical trials, which represent an important clinical challenge of the next 10 years. To do so, we will benefit from our close connection to the INCa-labeled AP-HM's center for early phase clinical trials (CLIP2). Our aim is to design model-based, individualized and adaptive scheduling regimen that depend on the monitoring of the disease evolution. We plan to run phase I/II trials based on the model recommendations. Depending on our achievements and success in phase I/II trials our mid-term goal would be to lead a prospective, randomized, phase III trial comparing a model-based adaptive regimen to the standard of care for combination of immune checkpoint inhibition with chemotherapy and/or anti-angiogenic and targeted therapy. According to our team expertise, the target malignancies would be primarily lung cancer (LG) and head and neck cancer (SS).
3.9 Long-term objectives
At long-term, we globally wish to have established a worldwide leader position in the fields of quantitative mathematical oncology and PMX, as well as the pharmacokinetics of nanoparticles. We hope that this would translate into the achievement of three goals: (1) the development of software effectively used for clinical decision-making and dosing adjustment (estimated achievable), (2) the initiation of prospective, phase III clinical trials comparing model-guided therapy versus standard of care (highly challenging), (3) clinical trials of nanosystems designed by our group (estimated achievable). In addition, we foresee several avenues both in terms of modeling opportunities and applications.
3.9.1 Modeling
Our short-term program is devoted to the development of new models and their confrontation to empirical data. The mid-term program will focus on the validation and refinement of these models. In the long-term, we foresee that this will bring novel questions in terms of mathematical analysis of the models. For instance, metastatic modeling will establish validated models for tumor-tumor interactions, including immune-mediated interactions. In turn, this leads to nonlinear, size-structured, renewal PDEs. Study of the asymptotic behavior of such equations is non-trivial.
More generally, we expect that physiologically structured PDEs (psPDE) can become relevant to practical modeling in oncology, from two types of data: flow cytometry and single-cell sequencing. Flow cytometry is currently becoming of increasing relevance to characterize multiple populations of cells, for instance in the context of immuno-oncology. In the QUANTIC project (10.2), we are starting to interact with such data, only by means of scalar quantities so far. However, the structure of this data is to have, for each cell, a quantitative measure (e.g., a surface marker). Measuring these in a population of millions or billions of cells makes it adapted to modeling by such psPDE. Similarly, single-cell sequencing is a technique by which every cell of a population (e.g., in a tumor) is sequenced, thus having mutation information. In turn, this allows to quantify subclones in the population. Such data has already generated fascinating results, for instance in the study of metastatic development theories 100, 106. Although evolutionary modeling is a wide field with established groups (M. Nowak or F. Michor in Harvard, C. Curtis in Stanford, T. Graham at the CRUK), few groups are modeling dynamical data at single-cell resolution. To this regard, the theoretical work initiated by J. Clairambault and B. Perthame (Inria MAMBA) suggesting to use psPDEs to model evolution in cancer cell populations could be appropriate 85. Parameter estimation in such models is a challenging task 95 and data assimilation from flow cytometry or single-cell sequencing data sets would represent an important avenue. Dynamical data can be provided by circulating tumor DNA and we have already initiated contacts with an important clinical and biological study in Marseille on this topic (the SCHISM study, PIs: SS and F. Fina). The recent developments of technology enabling spatial resolution of single-cell sequencing also paves the way to exciting avenues in terms of modeling 116.
We will also build models that can optimize the effectiveness of treatments incorporating new criteria (other than the evolution of tumor mass) of diagnostic and therapeutic evaluation, especially those we have forged around the information provided by functional imaging (T80) computational algorithm time at which 80% of FDG is metabolized 77, 89, 120.
3.9.2 Pharmacological and clinical oncology
A general, challenging, long-term objective, would be to run prospective clinical trials in which a model-informed arm would be compared to the standard of care. In the model-informed arm, therapeutic decision would be based on the recommendation of the model. This applies to the models developed in all axes. For instance, in breast cancer, the number of cycles of chemotherapy would be adapted based on the model indication (axis 1), decision of the maximum tolerated dose in the treatment of leukemia patients would be based on the PGx/PK/PD model (axis 2) or the combination scheduling regimen would be given by model calculations (axis 3).
Regarding nanosystems initially designed by our group based on the nanoPBPK modeling, they will also be tested in early clinical trials. Our group will drive the design of these based on simulations performed with the nanoPBPK and pharmacodynamic model, in order to guarantee the highest chances of success while ensuring patients safety. In particular, nanoparticles specifically transporting cytoxics could replace standard systemic myelo-ablation in hematopoietic stem cell transplantation, a risky strategy with frequent life-threatening, when not lethal, toxicities. Because of the fully controlled distribution phase in the body, nanoparticles encapsulating several drugs could thus be implemented in the preparative regimens for allogeneic stem cell transplantation in leukemia or myeloid malignancies.
In addition, several groups predict that in addition to standard drugs or biologics, or rising gene therapy and cell therapy strategies, new devices such as nanobots will be developed to treat cancers. Nanobots are entities which are not designed to interact with standard pharmacological targets or genes like current anticancer treatments, but could fix the cancer cell, either by providing a missing protein, or ultimately trigger a mechanic cell-death using radiation, thermal wave, or by disrupting cell membrane. These new entities should exhibit totally new pharmacokinetics, because they are unlikely to be metabolized in the liver or to be cleared by the kidney or the biliary tract. Therefore, new models for PK/PD should be developed, because neither behavior in the body nor intrinsic mechanisms of action are known yet. In addition, the issue of nanosafety with such devices will be particularly critical and will require extensive PMX resources, to predict long-term effects or to keep under control the mechanism of action. Should such nanobots be developed, the COMPO joint-team should develop specific resources to monitor their fate in the body, specific resources to write equations describing nanobots/body, nanobots/immune system, and nanobots/cancer cells interactions, plus new global models encapsulating all the interactions, and pharmacodynamics impact of such devices.
Depending on the expertise we gain in the PK/PD knowledge of those cell therapies as part of the mid-term objectives, optimizing combinatorial strategies to such cell therapies will be another long-term objective.
Last, pharmaco-economic studies including impact of quality of life, increase in both tolerability and efficacy will be performed to determine whether PMX-based dosing is a cost-saving strategy. For instance, by refining the scheduling of immune checkpoint inhibitors, such as determining, using modeling and simulation strategies, when the plasma concentration of the drug reaches the threshold in trough levels necessary to ensure maximal target engagement, it will be possible to customize the frequency of the administrations, with possible strong impact on treatment costs. By using real-life data, we propose to use dedicated models to quickly define alternate and more appropriate dosing and/or scheduling with newly marketed anticancer agents, either chemotherapy, targeted therapies or biologics including immune checkpoint inhibitors.
4 Application domains
The COMPO research team's projects all focus on a serial of complementary and inter-related domains described in an itemized fashion below:
- Health: all the models to be developed within the framework of the COMPO team are related to improving healthcare;
- Cancer: in particular, the models will be developed to address specific issues related to cancerous diseases;
- Precision Medicine: in particular, in cancer the developed models will be part of the implementation of precision medicine in oncology focusing on the following items;
- Combinatorial regimen: developing model-informed strategies to determine the optimal modalities when combining several treatments altogether. With the increasingly diversified arsenal of therapeutic approaches to treat cancers (surgery, radiotherapy, chemotherapy, targeted and anti-angiogenic therapy and immunotherapy), defining optimal combination protocols is highly challenging 86. This spans the issues of sequencing, scheduling and dosing of those therapies, which are to date largely addressed using a trial-and-error approaches. Consequently, too many combinatorial trials fail, and attrition rate with combinatorial immunotherapy is now a rising issue in clinical oncology and we hypothesize that extensive modeling and pharmacometrics could help refining the way anticancer drugs are combined;
- Tools for decision-making: developing model-informed strategies to forecast clinical outcome, i.e., during clinical trials. Assessing the predictive power of markers not only at baseline but also in their change over time is a current challenge. The information available, on the basis of which decision is made, includes clinico-demographic variables, classical biomarkers (e.g., blood counts, thyroglobulin, lactate dehydrogenase levels, etc...) but also an increasing amount of data from other sources (e.g., immuno-monitoring, anatomical functional imaging or genomics) that require state-of-the-art modeling to analyze extremely dense and longitudinal data;
- Adaptive dosing strategies: developing model-informed strategies to customize dosing so as to ensure an optimal toxicity-efficacy ratio. All anticancer agents are approved upon registration trials performed in highly selected patients (i.e., with controlled lifestyle, little comorbidities, controlled polymedication and restricted range of age), thus smoothing the interindividual variability. In real-world practice however, patients are all heavily co-treated with a wide variety of other drugs plus herbal medications, likely to interact with drug metabolism and transport, and are frequently older than in clinical trials. In addition, genetic polymorphisms affecting genes coding for drug transport proteins or drug-metabolizing enzymes in the liver, or transcriptional factors can impact as well on dose-exposure relationships. Consequently, standard dosing may not be suitable in non-standard patients to reach the adequate drug exposure levels associated with optimal toxicity/efficacy balance;
- Nanomedicines: developing model-informed strategies to conceptualize drug-loaded nanoparticles likely to improve the toxicity-efficacy ratio over conventional treatments. As of today, the biodistribution phase of anticancer agents is totally aspecific, making "on-taget off-site" actions an issue because it is associated with drug-related side effects affecting healthy tissues. Nanoparticles present unique features likely to deliver specifically a high amount of payload directly on a tumor site, thus improving the antiproliferative action while sparing healthy tissues. In addition, nanoparticles are expected to reshape the tumor micro-environment, making them good candidates to be further associated with immunotherapy (see Combinatorial Regimen above).
5 Social and environmental responsibility
5.1 Impact of research results
Due to its unique composition including medical oncologists, clinical pharmacologists and mathematical modelers, COMPO is at stake with important social challenges: oncology healthcare and innovation in drug development. The software and results developed by COMPO are devoted to these challenges and aim to be directly used by medical and pharmaceutical oncologists or by the biotech and pharmaceutical industry to help drug development and biomarker discovery.
To give a few examples:
- the KineticsPro software historically developed by Pr Iliadis is used daily by pharmacists to individually adapt the dose of anti-cancer drugs (e.g., for cisplatin or busulfan)
- the compoEDA package is used by physicians to produce automated statistical reports, helping to analyze the data collected for specific medical questions.
- the nlml_onco software is developed in collaboration with the industry (Roche / Genentech) to help anticipate the results of phase 3 clinical trials using machine learning models trained on the results of earlier (phase 2) clinical trials 58-
- COMPO is in charge of the biostatistical, machine learning and mechanistic modeling analysis of the large-scale PIONeeR RHU project to identify biomarker signatures predictive of the resistance to immunotherapy in lung cancer
- the LUCA-pi RHU, led by COMPO member Pr Boulate, will conduct research to implement lung cancer screening in France (currently not performed)
6 Highlights of the year
- Organisation of the first Mathematics and Medicine workshop in presence of high-impact clinical oncologists, including Prs F. Barlesi, F. Andre and E. Deutsch, respectively CEO, head of research and chair of the radiation therapy department, Gustave Roussy Institute.
- The LUCA-pi project (Lung cancer prevention and interception), led by COMPO member Pr D. Boulate was laureate of the sixth RHU call (large national initiatives for clinical research, 10M€ budget, 5 years duration)
- COMPO is part of the South-ROCK consortium, a Lyon-Marseille integrated research center for pediatric oncology, that has been laureate of the PEDIACRIEIX national cancer institute call (total funding 6M€, 5 years duration)
- Pr L. Greillier has been promoted to Head of the Department of Pneumology, Allergology and Oncology at APHM (Assistance Publique - Hopitaux de Marseille), elected President of the French group of thoracic oncology (GFPC), and elected General Secretary of the Oncology Group of the French Respiratory Society (GOLF).
- Dr R. Fanciullino has been elected Chief-Coordinator for the Clinical Pharmacy Group of the Société Française des Pharmaciens Oncologues (SFPO).
- COMPO has joined the PETRA consortium (réseau PrEclinique et TRAnslationnel de recherche en neuro-oncologie ) supported by the Canceropôle PACA.
- Two Ph.D. students at COMPO have succesfully defended their PhD's in 2023: Guillaume Sicard (May 2023, supervisors Joseph Ciccolini and Raphaelle Fanciullino) and Mourad Hamimed (October 2023, supervisors Joseph Ciccolini and Nicolas André).
6.1 Awards
- Mathilde Dacos : Best Poster Presentation Award, XIVth SFPO Meeting Nancy France (supervisor: R. Fanciullino)
7 New software, platforms, open data
7.1 New software
7.1.1 compo.EDA
-
Name:
Exploratory Data Analysis for Clinical Oncology Data
-
Keywords:
Clinical oncology, Data visualization, Biostatistics, Statistical analysis
-
Scientific Description:
This library implements as an R package:
- Exploratory analysis:
- Clinical characteristics table
- Kaplan-Meier estimation of the progression-free and overall survival
- Clinical and biological features distribution
- Classification analysis:
- Univariate and multivariate logistic regression
- Odds ratio
- Area under ROC curve
- t test / chi2 test
- Survival analysis:
- Univariate and multivariate Cox regression
- Hazard ratio
- Area under ROC curve
- log-rank test
- Data visualization:
- Correlation plots (Pearson correlation)
- Volcano plots (p-value and adjusted p-value)
- Boxplots (quantitative features) and barplots (qualitative feaures)
- Kaplan-Meier curves
- Automatic comprehensive and customizable statistical reports
- Exploratory analysis:
-
Functional Description:
The package compoEDA aims to provide a comprehensive exploratory analysis of data from clinical studies in oncology. These studies commonly investigate biological markers able to reveal and distinguish different tumor profiles, in order to early adapt the therapeutic strategy for patients.
The objective of this software is to provide a simplified tool for both computational scientists and clinical researchers to easily generate agraphical results and automatic reports containing the following analyses:
- Overview and visualization of clinical data and biological markers
- Overview and visualization of clinical data and biological markers
- Univariate and multivariate classification analysis (logistic regression)
- Univariate and multivariate survival analysis (Cox regression, Kaplan-Meier analysis)
- Correlation analysis
- Statistical tests
- Visualization of markers (boxplots, barplots, volcano plots, forest plots ...).
-
Release Contributions:
First release
-
News of the Year:
- Creation of the package
- Improvement and prettification of the generation of data plots (boxplots, barplots, ...)
- Automation of the results generation: classification and survival analysis
- Automation, refactor and improvements of automatic statistical reports
- URL:
-
Contact:
Sebastien Benzekry
-
Participants:
Sebastien Benzekry, Linh Nguyen Phuong, Celestin Bigarre, Paul Dufosse, Melanie Karlsen
7.1.2 compo.NLME
-
Keywords:
Nonlinear mixed effects models, Biostatistics, Statistics, Cache, Cancer
-
Scientific Description:
Available features:
- Structural models
- constant
- linear
- double exponential
- double exponential with dropout
- hyperbolic
- preprocess blood marker datasets
- preprocess tumor kinetics datasets
- fit NLME models using monolix API
- post-process of results
Available data:
- Tumor Kinetics with dropout data. A simulated dataset of tumor kinetics following the double-exponential model, with parameters obtained from (Benzekry et al., PAGE 20, 2022), which deals with the RECIST-based sum of largest diameters (SLD, in mm) of lung cancer treated with immune-checkpoint blockade (anti-PDL1 drug atezolizumab). Dropout was also simulated using a Weibull survival model.
- Tumor and Blood marker Kinetics with dropout data. A simulated dataset of joint tumor and blood markers (albumin C-reactive protein, lactate dehydrogenase, neutrophils) kinetics following the models and parameters established in (Benzekry et al., PAGE 20, 2022). These are monitoring data during immune-checkpoint blockade (anti-PDL1drug atezolizumab) in lung cancer. Dropout was also simulated using a Weibull survival model.
- Structural models
-
Functional Description:
This R package implements a framework to work with Non-linear Mixed effects models in the context of clinical oncology to predict relapse and survival using longitudinal data.
-
News of the Year:
Development of the initial version of the package.
- Publications:
-
Contact:
Sebastien Benzekry
-
Participants:
Sebastien Benzekry, Celestin Bigarre, Ruben Taieb
7.1.3 ml.tidy
-
Name:
Machine learning with tidymodels
-
Keywords:
Survival analysis, Machine learning, Data analysis, Oncology
-
Scientific Description:
This software maximizes the use of the R package tidymodels.
-
Functional Description:
This package provides multiple functions to perform machine learning analysis using the `tidymodels` framework. Tasks include: feature selection, plot feature importances, train, corss-validate or apply supervised machine learning algorithms (classification or survival analyses), evaluate metrics of predictive performances, compute learning curves.
Initial development was part of the `stats_pioneer` package (also called `pioneerPackage`) and `ml.tidy` evolved as a standalone package only in February 2023.
-
News of the Year:
- Added "LASSO" as an option to compute incremential model by varying the penalization value lambda in a logistic LASSO regression
- Fixing one hot encoding for non-dichotomic variables that are qualitative
- Creating vignettes (long form HTML guide) for ML classification and survival algorithms
- Cleaning documentation and description of function in package and formatting for better readability
- URL:
- Publications:
-
Authors:
Sebastien Benzekry, Melanie Karlsen, Mohamed Boussena
-
Contact:
Sebastien Benzekry
7.1.4 stats_pioneer
-
Name:
Statistical analysis and reporting of the PIONeeR study
-
Keywords:
PIONeeR, Statistical analysis, Biostatistics
-
Functional Description:
This software was built to analyse the PIONeeR (Precision Immuno-Oncology for advanced Non-small cell lung cancer patients with PD-(L) 1 ICI Resistance) data. PIONeeR is a prospective, multicenter study with primary objective being to validate the existence of a hypothetical immune profile explaining resistance to immunotherapy in non-small cell lung cancer patients.
It initially integrated preprocessing, exploratory data analysis, visualization, statistical analysis, feature selection, machine learning and results generation and reporting. Since, exploratory data analysis, visualization and statistical analysis have been promoted to the COMPO-level `compoEDA` package and feature selection and machine learning to the COMPO-level `ml.tidy` package.
This software corresponds to the very first step of the data analysis, which is the preprocessing, and the very last: generation of results. Some of its functions aim at:
- preprocessing the data (creation of clinical variables, dictionary, outcome variables, data monitoring and correcctions, treatment of the variables types)
- generating the tools to load the data and metadata
- computing statistical tests, logistic or Cox regression, or performing a correlation analysis
- visualising the data (boxplots, barplots, survival curves, ROC curves, volcano plots)
- providing detailed and interactive statistical reports on the data
- automating the production of these reports using Gitlab CI/CD
-
News of the Year:
Two releases of the analyses of the data locks 3 and 4. Analysis of data lock 5 ongoing (60%). Analyses performed included:
- Preprocess:
- monitoring and corrections
- multiple data and metadata wrangling and organization
- Improved statistical analysis reports:
- refactor and improvement of multiple visualizations
- patient history app displays longitudinal markers
- factorization of automatic reports, then promoted to the `compoEDA` COMPO-wise package
- extensive univariate statistical analysis of all biomarkers at screening (436 variables)
- shiny apps for patient history and variable summary
- Continuous integration / continuous development:
- refactor
- extensions
- centralized website for sharing the results with the partners
- Feature selection:
- clean and reproducible versions of the feature selection stability report: `reports/technical_reports/supervised/feature_selection/signature_volatility`
- benchmark of FS methods
- unsupervised analysis of BOLASSO-filtered variables (clustering)
- multiple signature defined and investigated
-
Machine learning (ML) for prediction of early progression in 2+ line patients:
- clean Rmd ML reports
- clean low-NA dataset
- classical ML algorithms benchmark
- Addition and development of a python package python-based analyses:
- Reporting:
- scripts for reproducible Figures of three ongoing papers
- slide decks for the two biannual general assembly meetings
- Preprocess:
- URL:
- Publications:
-
Authors:
Sebastien Benzekry, Melanie Karlsen, Kokou Atsou, Paul Dufosse, Celestin Bigarre, Mohamed Boussena, Andréa Vaglio
-
Contact:
Sebastien Benzekry
-
Partners:
Veracyte, Innate pharma, APHM
7.1.5 nlml_onco
-
Name:
Nonlinear mixed-effects modeling and machine learning for oncology
-
Keywords:
Nonlinear mixed effects models, Machine learning, Oncology, Survival analysis
-
Scientific Description:
- Exploratory data analysis
- Automated mixed-effects modeling analysis
- Preprocess
- Analysis of high dimensional transcriptomic data
- Dimension reduction
- Feature selection
- Survival machine learning algorithms
- Applications (+ 3,000 patients)
- individual predictions of survival
- predictions of hazard ratios for applications in drug development, for both monotherapy and combinatorial treatments
-
Functional Description:
This software analyses multiple data arising from clinical oncology (routine care and clinical trials). This data can be of two types:
- Static (e.g., baseline features), from clinical, biological, molecular (e.g., transcriptomic or mutation data)
- Longitudinal (multiple time points per individual): tumor kinetics, biomarkers. The second type is modeled using the framework of nonlinear mixed-effects modeling. All features are then analyzed using data science techniques (preprocess, feature selection, machine learning algorithms), in order to predict survival outcome.
-
Release Contributions:
- Established the first clean version of the software with multiple use cases
- Checked that all runs and produces the expected outputs
- Separated code from input (data) and output
-
News of the Year:
- New visualization feature
- Code refactor
- Fixed minor bugs
- Extensive use for generation of results
- URL:
- Publications:
-
Contact:
Sebastien Benzekry
-
Participant:
Sebastien Benzekry
-
Partner:
Roche
7.1.6 metamats
-
Keywords:
Statistical modeling, Tumor growth
-
Functional Description:
This R package is the implementation of a general framework to build and use models of the metastatic process based on the initial model of Iwata et al. (2000). The family of model that can be built describe the metastatic disease with a partial differential equation (pde) on the size structured distribution of the tumors. These models have three components, a function that characterize the growth of the primary tumor, a function that characterize the growth of the metastases, and a dissemination function that decribes how new metastases are produced.
-
Release Contributions:
Features:
- Model structure
- Direct computation of (C++)
- Individual fit of cumulative size distribution (direct only)
- Many diagnostic plots
-
News of the Year:
Development of the first version of the sofware and several updates.
This sofware was used and will be described in a work to be published in 2024. The application was the use of this model to describe the dynamics of the brain metastasis disease in small cell lung cancer patient with or without prophylactic cranial irradiation (PCI) and study the impact of PCI on the overall survival of the patients as well as the progression of the brain disease.
- URL:
-
Contact:
Celestin Bigarre
-
Participants:
Sebastien Benzekry, Celestin Bigarre
7.1.7 metamatsModels
-
Keyword:
Tumor growth
-
Functional Description:
A collection of models implementation for the metamats R package
-
Release Contributions:
Available models:
- Gompertz growth model (alpha, mu parametrization)
- Gompertz growth model (alpha, K parametrization)
- Gompertz with dormancy growth model (alpha, mu, tau parametrization)
- Proportional dissemination model
- Power dissemination model (mu, gamma parametrization)
- Iwata model object (identical gompertz growth, proportional dissemination)
- Benzekry model object (identical gompertz growth with dormance, power diss)
-
News of the Year:
Development of the first version of the sofware and several updates.
This sofware is works in combinaison with the metamats R package and was used and will be described in a work to be published in 2024 (see the BIL entry of metamats R package for more details).
-
Contact:
Celestin Bigarre
-
Participants:
Celestin Bigarre, Sebastien Benzekry
7.1.8 SChISModeling
-
Name:
SChISM modeling
-
Keywords:
SChISM, Statistical analysis, Biostatistics
-
Scientific Description:
- Preprocess
- Exploratory data analysis
- Classification analysis (logistic regression)
- Survival analysis (Cox regression)
- Mixed-effects modeling analysis
-
Functional Description:
SChISModeling aims to analyze SChISM data (Size CfDNA Immunotherapies Signature Monitoring). SChISM is a clinical study that introduces an innovative approach to quantify circulating free DNA in cancer patients treated with immunotherapy. The study's objective is to early predict response to immunotherapy in patients at an advanced/metastatic stage according to these quantitative cfDNA data.
This software corresponds to the very first step of the data analysis, which is the statistical analysis. Some of its functions aim at:
- preprocessing the data (creation of clinical variables, dictionary, outcome variables, clinical biomarkers, treatment of the variables types)
- computing statistical tests, logistic or Cox regression, performing a correlation analysis
- visualizing the data (boxplots, barplots, survival curves, ROC curves, volcano plots)
- providing detailed and interactive statistical reports on the data
-
News of the Year:
- Preprocess
- Exploratory data analysis
- Classification analysis (logistic regression)
- Survival analysis (Cox regression)
- Mixed-effects modeling analysis
-
Contact:
Sebastien Benzekry
-
Participants:
Sebastien Benzekry, Sebastien Salas, Linh Nguyen Phuong
8 New results
8.1 Predicting survival and trial outcome in non-small cell lung cancer integrating tumor and blood markers kinetics with machine learning
Participants: Sébastien Benzekry, Mélanie Karlsen, Célestin Célestin, Abdessamad El Kaoutari, Rene Bruno [Roche Genentech], Ales Neubert [Roche pRED], François Mercier [Roche Genentech], Martin Stern [Roche pRED], Bruno Gomes [Roche pRED], Suresh Vatakuti [Roche pRED], Peter Curle [Roche pRED], Candice Jamois [Roche pRED].
Funding and data: Roche pRED
Publication: Submitted to Clinical Cancer Research (preprint in medrxiv 58), Oral communications 34, 35, 33, 36, 37
Existing survival prediction models rely only on baseline or tumor kinetics data and lack machine learning integration. We introduce a novel kinetics-machine learning (kML) model that integrates baseline markers, tumor kinetics and four on-treatment simple blood markers (albumin, CRP, lactate dehydrogenase and neutrophils).
Developed for immune-checkpoint inhibition (ICI) in non-small cell lung cancer on three phase 2 trials (533 patients), kML was validated on the two arms of a phase 3 trial (ICI and chemotherapy, 377 and 354 patients). It outperformed the current state-of-the-art for individual predictions with a test set c-index of , a 12-months survival accuracy of and a hazard ratio of ( CI: – , ) to identify long-term survivors.
Critically, kML predicted the success of the phase 3 trial using only 25 weeks of on-study data (predicted HR ( – ) versus final study HR ( – )). Our model constitutes a valuable approach to support personalized medicine and drug development.
8.2 Mechanistic modeling of metastatic relapse in early breast cancer to investigate the biological impact of prognostic biomarkers
Participants: Célestin Bigarré, François Bertucci [Paoli Calmettes Institute], Pascal Finetti [Paoli Calmettes Institute], Gaëtan Macgrogan [Bergonié Institute], Xavier Muracciole, Sébastien Benzekry.
Funding: Inria-Inserm Phd Grant
Publication: Published in ”Computer Methods and Programs in Biomedicine” 15 and AACR communication in 2022 82
Background and Objective
Estimating the risk of metastatic relapse is a major challenge to decide adjuvant treatment options in early-stage breast cancer (eBC). To date, distant metastasis-free survival (DMFS) analysis mainly relies on classical, agnostic, statistical models (e.g., Cox regression). Instead, we propose here to derive mechanistic models of DMFS.
Methods
The present series consisted of eBC patients who did not receive adjuvant systemic therapy from three datasets, composed respectively of 692 (Bergonié Institute), 591 (Paoli-Calmettes Institute, IPC), and 163 (Public Hospital Marseille, AP-HM) patients with routine clinical annotations. The last dataset also contained expression of three non-routine biomarkers. Our mechanistic model of DMFS relies on two mathematical parameters that represent growth () and dissemination (). We identified their population distributions using the mixed-effects modeling. Critically, we propose a novel variable selection procedure allowing to: (i) identify the association of biological parameters with either , or both and (ii) generate an optimal candidate model for DMFS prediction.
Results
We found that Ki67 and Thymidine Kinase-1 were associated with and nodal status and Plasminogen Activator Inhibitor-1 with . The predictive performances of the model were excellent in calibration but moderate in discrimination, with c-indices of ( confidence interval , AP-HM), (, Bergonié) and ( CI , IPC).
Conclusions
Overall, we demonstrate that our novel method combining mechanistic and advanced statistical modeling is able to unravel the biological roles of clinicopathological parameters from DMFS data.
8.3 Computational markers for personalized prediction of outcomes in non‑small cell lung cancer patients with brain metastases
Participants: Sébastien Benzekry, Pirmin Schlicke [TU München], Alice Mogenet [AP-HM], Laurent Greillier, Pascale Tomasini [AP-HM], Eleonore Simon [AP-HM].
Data: early-stage NSCLC patients with brain metastases, Multidisciplinary oncology and therapeutic innovation department, APHM
Publication: 14
Background
Intracranial progression after curative treatment of early-stage non-small cell lung cancer (NSCLC) occurs from 10 to 50% and is difficult to manage, given the heterogeneity of clinical presentations and the variability of treatments available.
Objective
The objective of this study was to develop a mechanistic model of intracranial progression to predict survival following a firstbrain metastasis (BM) event occurring at a time .
Methods
Data included early-stage NSCLC patients treated with a curative intent who had a BM as the first and single relapse site (N = 31). We propose a mechanistic mathematical model able to derive computational markers from primary tumor and BM data at and estimate the amount and sizes of (visible and invisible) BMs, as well as their future behavior. These two key computational markers are , the proliferation rate of a single tumor cell; and , the per day, per cell, probability to metastasize. The predictive value of these individual computational biomarkers was evaluated.
Results
The model was able to correctly describe the number and size of metastases at for 20 patients. Parameters and were significantly associated with overall survival (OS) (HR 1.65 (1.07–2.53) p = 0.0029 and HR 1.95 (1.31–2.91)p = 0.0109, respectively). Adding the computational markers to the clinical ones significantly improved the predictive valueof OS (c-index increased from 0.585 (95% CI 0.569–0.602) to 0.713 (95% CI 0.700–0.726), p < 0.0001). We demonstrated that our model was applicable to brain oligoprogressive patients in NSCLC and that the resulting computational markers had predictive potential.
Conclusion
This may help lung cancer physicians to guide and personalize the management of NSCLC patients with intracranial oligoprogression.
8.4 Machine-learning and mechanistic modeling of primary and metastatic breast cancer growth after neoadjuvant targeted therapy
Participants: Sébastien Benzekry, Michalis Mastri, Chiara Nicolò, John ML Ebos.
Data: Preclinical data of primary tumor and metastatic growth in 128 mice.
Publication: under revision at PLoS Computational Biology 59
Background
Clinical trials involving systemic neoadjuvant treatments in breast cancer aim to shrink tumors prior to surgery while simultaneously allowing for controlled evaluation of biomarkers, toxicity, and suppression of distant (occult) metastatic disease. Yet such trials are rarely preceded by preclinical testing involving surgery.
Objective
Here we used a mouse model of spontaneous metastasis after surgical removal to develop a predictive mathematical model of neoadjuvant treatment response to sunitinib, a receptor tyrosine kinase inhibitor (RTKI).
Methods
Longitudinal data consisted of measurements of presurgical primary tumor size and postsurgical metastatic burden in 128 mice (104 for model training, 24 for validation), following variable neoadjuvant treatment schedules over a 14-day period. A nonlinear mixed-effects modeling approach was used to quantify inter-animal variability. Machine learning algorithms were applied to investigate the significance of several biomarkers at resection as predictors of individual kinetics. Biomarkers included circulating tumor- and immune-based cells (circulating tumor cells and myeloid-derived suppressor cells) as well as immunohistochemical tumor proteins (CD31 and Ki67).
Results
Our simulations showed that neoadjuvant RTKI treatment inhibits primary tumor growth but has little efficacy in preventing (micro)-metastatic disease progression after surgery. Surprisingly, machine-learning algorithms demonstrated only limited predictive power of tested biomarkers on the mathematical parameters. These results suggest that presurgical modeling might be an effective tool to screen biomarkers prior to clinical trial testing.
Conclusion
Mathematical modeling combined with artificial intelligence techniques represent a novel platform for integrating preclinical surgical metastasis models in outcome prediction of neoadjuvant treatment.
8.5 A comparison of machine learning algorithms for survival with missing data
Participants: Paul Dufossé, Sébastien Benzekry.
Funding: Laënnec Institute.
Publication: 39
Survival analysis is an essential tool for the study of health data. An inherent component of such data is the presence of missing values. In recent years, researchers proposed new learning algorithms for survival tasks based on neural networks. Here, we studied the predictive performance of such algorithms coupled with different methods for handling missing values on simulated data that reflect a realistic situation, i.e., when individuals belong to unobserved clusters. We investigated different patterns of missing data. The results show that, without further feature engineering, no single imputation method is better than the others in all cases. The proposed methodology can be used to compare other missing data patterns and/or survival models. The Python code is accessible via the package survivalsim.
8.6 Pharmacokinetic Modeling for drug delivery
Participants: Anne Rodallec, Sophie Marolleau, Sébastien Benzekry.
Data: PK and PD data on breast cancer bearing mice, Paris-Sud.
Background
Commercial Paclitaxel (PTX) formulations such as Taxol are associated with adverse drug toxicities related to the added emulsionizer. Therefore, PTX formulations have been explored as they could increase efficacy and have improved pharmacokinetic (PK) properties. Recently our partners have developed a new polymer prodrug of PTX for which we proposed that metronomic dosing could result in an effective and tolerable anticancer treatment that is simultaneously convenient for patients as it allows for subcutaneous (SC) administration.
Objective
The objective of this study was to develop a PK/PD model of a novel polymer prodrug subcutaneously injectable to predict the best administration scheme.
Methods
We developed a population PK model and calibrated it with experimental data of a novel PTX-Polymer from a separate study using Monolix software.
Results
The model was able to correctly describe treatment pharmacokinetic and efficacy profiles. Pharmacokinetic was described using a two-compartment model with an additional compartment for the polymer groupe. We selected the reduced Gompertz model as the optimal model to represent unperturbed tumor growth using available pharmacodynamic (PD) data. Efficacy was best described using Simeoni model. We discovered that adding drug resistance of the treatment efficacy was necessary to correctly fit the data. Our simulations show that administrating the prodrug daily is a promising strategy for further preclinical studies.
Conclusion
We developed a PK/PD model describing preclinical data of a novel anticancer polymer prodrug and validated that metronomic dosing could result in greater efficacy.
8.7 Pancreatic ductal adenocarcinoma ubiquitination profiling reveals specific prognostic and theranostic markers
Participants: Samad El Katouary, Joseph Ciccolini.
Background and objective
Pancreatic ductal adenocarcinoma (PDAC) has been widely studied at multiomics level. However, little is known about its specific ubiquitination, a major post-translational modification (PTM). As PTMs regulate the final function of any gene, we decided to establish the ubiquitination profiles of 60 PDAC.
Methods
We used specific proteomic tools to establish the ubiquitin dependent proteome (ubiquitinome) of frozen PDXs (Patients' derived xenographs). Then, we performed bioinformatics analysis to identify the possible associations of these ubiquitination profiles with tumour phenotype, patient survival and resistance to chemotherapies. Finally, we used proximity ligation assays (PLA) to detect and quantify the ubiquitination level of one identified marker.
Results
We identified 38 ubiquitination site profiles correlating with the transcriptomic phenotype of tumours and four had notable prognostic capabilities. Seventeen ubiquitination profiles displayed potential theranostic marker for gemcitabine, seven for 5-FU, six for oxaliplatin and thirteen for irinotecan. Using PLA, we confirmed the use of one ubiquitination profile as a drug-response marker, directly on paraffin embedded tissues, supporting the possible application of these biomarkers in the clinical setting.
Conclusion
These findings bring new and important insights on the relationship between ubiquitination levels of proteins and different molecular and clinical features of PDAC patients. Markers identified in this study could have a potential application in clinical settings to help to predict response to chemotherapies thereby allowing the personalization of treatments.
8.8 Killing a fly with a sledgehammer: Atezolizumab exposure in real-world lung cancer patients
Participants: Sophie Marolleau, Mourad Hamimed, Joseph Ciccolini.
Background
Atezolizumab is an anti-PDL1 approved for treating lung cancer. A threshold of 6 μg/mL in plasma has been associated with target engagement. The extent to which patients could be overexposed with the standard 1200 mg q3w dosing remains unknown.
Patients and Methods
Here, we monitored atezolizumab peak and trough levels in 27 real-world patients with lung cancer as part of routine therapeutic drug monitoring. Individual pharmacokinetic (PK) parameters were calculated using a population approach and optimal dosing-intervals were simulated with respect to the target trough levels.
Results
No patient had plasma levels below 6 μg/mL. The results showed that the mean trough level after the first treatment was 78.3 ± 17 μg/mL, that is, 13 times above the target concentration. The overall response rate was 55.5 percent. Low-grade immune-related adverse events was observed in 37 percent of patients. No relationship was found between exposure metrics of atezolizumab (i.e., minimum plasma concentration, maximum plasma concentration, and area under the curve) and pharmacodynamic end points (i.e., efficacy and toxicity). Further simulations suggest that the dosing interval could be extended from 21 days to 49 up to 136 days (mean: 85.7 days, i.e., q12w), while ensuring plasma levels still above the 6 μg/mL target threshold. This observational, real-world study suggests that the standard 1200 mg q3w fixed-dose regimen of atezolizumab results in significant overexposure in all the patients. This was not associated with increased side effects. As plasma levels largely exceed pharmacologically active concentrations, interindividual variability in PK parameters did not impact efficacy.
Conclusion
Our data suggest that dosing intervals could be markedly extended with respect to the target threshold associated with efficacy.
8.9 Harnessing tumor immunity with cytotoxics: T cells monitoring in mice bearing lung tumors treated with anti-VEGF and pemetrexed-cisplatin doublet
Participants: Guillaume Sicard, Dorian Protzenko, Sarah Giacometti, Joseph Ciccolini, Raphaelle Fanciullino.
Background
Successful immunotherapy is restricted to some cancers only, and combinatorial strategies with other drugs could help to improve their efficacy. Here, we monitor T cells in NSCLC model after treatment with cytotoxics (CT) and anti-vascular endothelial growth factor (VEGF) drugs, to understand when immune checkpoint inhibitors should be best associated next.
Methods
In vivo study was performed on BALB/c mice grafted with KLN205 cells. Eight treatments were tested including control, cisplatin and pemetrexed as low (LD CT) and full (MTD CT) dose as single agents, flat dose anti-VEGF and the association anti-VEGF + CT. Full immunomonitoring was performed by flow cytometry on tumor, spleen and blood over 3 weeks.
Results
Immunomodulatory effect was dependent upon both treatments and time. In tumors, combination groups shown numerical lower Treg cells on Day 21. In spleen, anti-VEGF and LD CT group shown higher CD8/Treg ratio on Day 7; on Day 14, higher T CD4 were observed in both combination groups. Finally, in blood, Tregs were lower and CD8/Treg ratio higher, on Day 14 in both combination groups. On Day 21, CD4 and CD8 T cells were higher in the anti-VEGF + MTD CT group.
Conclusions
Anti-VEGF associated to CT triggers notable increase in CD8/Tregs ratio. Regarding the scheduling, a two-week delay after using anti-VEGF and CT could be the best sequence to optimize antitumor efficacy.
8.10 Cytidine deaminase status as a marker of response to azacytidine treatment in MDS and AML patients.
Participants: Mélanie Donnette, Mourad Hamimed, Guillaume Sicard, Joseph Ciccolini, Raphaelle Fanciullino.
Background
Azacitidine (Aza) is a mainstay of treatment for patients with acute myeloid leukaemia (AML) ineligible for induction chemotherapy and other high-risk myelodysplastic syndromes (MDS). Only half of patients respond, and almost all will eventually relapse. There are no predictive markers of response to Aza. Aza is detoxified in the liver by cytidine deaminase (CDA).
Patients and Methods
Here, we investigated the association between CDA phenotype, toxicity and efficacy of Aza in real-world adult patients.
Results
Median overall survival (OS) was 15 months and 13 months in AML and high-risk MDS patients respectively. In addition, our data suggest that delaying Aza treatment was not associated with lack of efficacy and should not be considered a signal to switch to an alternative treatment. Half of the patients had deficient CDA activity (i.e. <2 UA/mg), with a lower proportion of deficient patients in MDS patients (34 percent) compared to AML patients (67 percent). In MDS patients, CDA deficiency correlated with longer landmark OS (14 vs. 8 months; p = 0.03), but not in AML patients.
Conclusions
Taken together, our data suggest that CDA is an independent covariate and may therefore be a marker for predicting clinical outcome in MDS patients treated with Aza.
8.11 Determination of 5-azacitidine in human plasma by LC-MS/MS: application to pharmacokinetics pilot study in MDS/AML patients
Participants: Loic Osanno, Mélanie Donnette, Joseph Ciccolini, Raphaelle Fanciullino.
Background
Azacitidine (Vidaza®, AZA) is a mainstay for treating acute myeloid leukemia (AML) in patients unfit for standard induction and other myelodysplastic syndromes (MDS). However, only half of the patients usually respond to this drug and almost all patients will eventually relapse. Predictive markers for response to AZA are yet to be identified. AZA is metabolized in the liver by a single enzyme, cytidine deaminase (CDA). CDA is a ubiquitous enzyme coded by a highly polymorphic gene, with subsequent great variability in resulting activities in the liver. The quantitative determination of AZA in plasma is challenging due the required sensitivity and because of the instability in the biological matrix upon sampling, possibly resulting in erratic values.
Methods
We have developed and validated following EMA standards a simple, rapid, and cost-effective liquid chromatography-tandem mass spectrometry method for the determination of azacitidine in human plasma.
Results
After a simple and rapid precipitation step, analytes were successfully separated and quantitated over a 5-500 ng/mL range. The performance and reliability of this method were tested as part of an investigational study in MDS/AML patients treated with standard azacitidine (75 mg/m2 for 7 days a week every 28 days).
Conclusion
Overall, this new method meets the requirements of current bioanalytical guidelines and could be used to monitor drug levels in MDS/AML patients.
9 Bilateral contracts and grants with industry
Participants: Sebastien Benzekry, Joseph Ciccolini, Raphaelle Fanciullino, Laurent Greillier, Anne Rodallec, Sebastien Salas.
9.1 Research contracts
kML 2.0
- Partner: Genentech
- Title: Kinetics - machine learning modeling for prediction of outcome in oncology
- Funding: 25 k€
- Duration: Oct 2023 - Apr 2024
- Principal investigator: S. Benzekry
NanoImmuno
- Partner: Institut Roche France and Genentech US
- Title: NanoImmuno: a nanoparticle to harness tumor immunity in her2+ breast cancer
- Funding: 80 k€
- Duration: Jan 2020 - March 2023
- Principal investigator: Raphaelle Fanciullino (Senior), Anne Rodallec (Junior)
9.2 Clinical trials
CetuxiMAX
- Registration: NCT4218136
- Partner: Merck Serono
- Title: Maximizing Cetuximab efficacy in head and neck cancer patients through PK/PD modeling
- Funding: 40 k€
- Duration: 2020 - 2024
- Principal investigator: Sébastien Salas
IMHOTEP
- Registration: NCT04795661
- Partner: INCa
- Title: Immunotherapy in MSI/dMMR Tumors in Perioperative Setting
- Funding: 800 k€
- Duration: 2023 - 2027
- COMPO investigator: Joseph Ciccolini
MOIO
- Registration: NCT05078047
- Partner: BMS and INCa
- Title: Study Comparing the Standard Administration of IO Versus the Same IO Administered Each 3 Months in Patients With Metastatic Cancer in Response After 6 Months of Standard IO
- Funding: 500 k€
- Duration: 2020-2024
- Principal investigator: Gwenaelle Gravis (IPC)
- COMPO investigator: Joseph Ciccolini
PEMBOV
- Registration: NCT03596281
- Partner: INCa
- Title: Pembrolizumab in Combination With Bevacizumab and Pegylated Liposomal Doxorubicin in Patients With Ovarian Cancer (PEMBOV)
- Funding: 700 k€
- Duration: 2020-2023
- Principal investigator: Judith Mitchels (IGR)
- COMPO investigator: Joseph Ciccolini
REZOLVE
- Registration: ANZGOG-1101
- Partner: Sydney Medical Center Australia
- Title: Pembrolizumab in Combination With Bevacizumab and Pegylated Liposomal Doxorubicin in Patients With Ovarian Cancer (PEMBOV)
- Funding: Aus$ 800k
- Duration: 2018-2024
- Principal investigator: Sonia Yip (Sydney University)
- COMPO investigator: Joseph Ciccolini
DROP
- Registration: NCT03257969
- Partner: INCa
- Title: Drug Related problems in Oncology Practice
- Funding: 400 k€
- Duration: 2018 - 2023
- COMPO investigator: Raphaelle Fanciullino
VIDAZAML
- Registration: EudraCT 2020-A02042-37
- Partner: APHM and CAL Nice
- Title: Role of CDA and dCK metabolic enzymes in clinical response of patients treated with azacitidine for blood disorders
- Funding: 80 k€ (APHM)
- Duration: 2021-2023
- Principal investigator: Geoffroy Venton - COMPO Investigator: Raphaelle Fanciullino
VENETACIBLE
- Registration: AORC 2023-AO1546-39
- Partner: CHU Nice
- Title: Personalizing Venetoclax dosing in AML patients non-eligible for HD chemotherapy as first line treatment.
- Funding: 30 k€ (Nice University Hospitals)
- Duration: 2023-2024
- COMPO Investigator: Raphaelle Fanciullino
Morpheus-Lung
- Registration: Eudract 2017-001267-21 / NCT03337698
- Partner: Hoffmann-La Roche
- Title: A Study to Evaluate Efficacy and Safety of Multiple Immunotherapy-Based Treatment Combinations in Patients with Metastatic Non-Small Cell Lung Cancer
- Duration: 2018-2023
- COMPO investigator: Laurent Greillier
PDC-LUNG-101
- Registration: Eudract 2018-002382-19 / NCT03970746
- Partner: PDC*line Pharma SAS
- Title: Safety, immunogenicity and preliminary clinical activity study of PDC*lung01 cancer vaccine in NSCLC
- Duration: 2022-2024
- Principal investigator: Johan Vansteenkiste, Prof KU Leuven
- COMPO investigator: Laurent Greillier
NCT03708328
- Registration: Eudract 2018-000982-35 / NCT03708328
- Partner: Hoffmann-La Roche
- Title: A Dose Escalation and Expansion Study of RO7121661, a PD-1/TIM-3 Bispecific Antibody, in Participants With Advanced and/or Metastatic Solid Tumors
- Duration: 2018-2023
- COMPO investigator: Laurent Greillier
PROPEL
- Registration: Eudract 2019-003474-35 / NCT03138889
- Partner: Nektar Therapeutics
- Title: Bempegaldesleukin and Pembrolizumab With or Without Chemotherapy in Locally Advanced or Metastatic Solid Tumors
- Duration: 2017-2024
- COMPO investigator: Laurent Greillier
TRIDENT-1
- Registration: Eudract 2016-003616-13 / NCT03093116
- Partner: Turning Point Therapeutics, Inc.
- Title: A Study of Repotrectinib (TPX-0005) in Patients With Advanced Solid Tumors Harboring ALK, ROS1, or NTRK1-3 Rearrangements
- Duration: 2017-2023
- Principal investigator: Shanna Stopatschinskaja, Dan Liu
- COMPO investigator: Laurent Greillier
NCT04721015
- Registration: Eudract 2020-004953-57 / NCT04721015
- Partner: AbbVie
- Title: Study of Intravenous (IV) ABBV-637 Alone or in Combination With IV Docetaxel/Osimertinib to Assess Adverse Events and Change in Disease Activity in Adult Participants With Relapsed/Refractory (R/R) Solid Tumors
- Duration: 2021-2026
- COMPO investigator: Laurent Greillier
PIONeeR
- Registration: NCT03493581
- Partner: APHM, HalioDx, Innate Pharma, AMU
- Title: Precision Immuno-Oncology for Advanced Non-small Cell Lung Cancer Patients With PD-1 ICI Resistance
- Duration: 2018-2024
- Principal investigator: Laurent Greillier, Jean-Olivier Arnaud
ELDERLY
- Registration: NCT03977194
- Partner: Intergroupe Francophone de Cancerologie Thoracique
- Title: Atezolizumab in Elderly Patients With Advanced Non-Small-Cell Lung Cancer and Receiving Carboplatin Paclitaxel Chemotherapy
- Duration: 2019-2023
- Principal investigator: Elisabeth QUOIX, Céline MASCAUX
- COMPO investigator: Laurent Greillier
NCT01817192
- Registration: NCT01817192
- Partner: Razor Genomics
- Title: Adjuvant Chemotherapy in Patients With Intermediate or High Risk Stage I or Stage IIA Non-squamous Non-Small Cell Lung Cancer
- Duration: 2020-2025
- Principal investigator: David R Spigel, Sarah Cannon
- COMPO investigator: Laurent Greillier
SAVIMMUNE
- Registration: NCT04108026
- Partner: Intergroupe Francophone de Cancerologie Thoracique
- Title: Immunotherapy in Patient With Poor General Condition
- Duration: 2020-2023
- Principal investigator: Valérie GOUNANT, Michael DURUISSEAUX
- COMPO investigator: Laurent Greillier
NCT04042558
- Registration: NCT04042558
- Partner: Centre Francois Baclesse
- Title: A Study Evaluating Platinum-Pemetrexed-Atezolizumab (+/-Bevacizumab) for Patients With Stage IIIB/IV Non-squamous Non-small Cell Lung Cancer With EGFR Mutations, ALK Rearrangement or ROS1 Fusion Progressing After Targeted Therapies
- Duration: 2019-2024
- COMPO investigator: Laurent Greillier
NIPINEC
- Registration: NCT03591731
- Partner: Intergroupe Francophone de Cancerologie Thoracique, Federation Francophone de Cancerologie Digestive, GERCOR - Multidisciplinary Oncology Cooperative Group
- Title: Nivolumab +/- Ipilimumab in Patients With Advanced, Refractory Pulmonary or Gastroenteropancreatic Poorly Differentiated Neuroendocrine Tumors (NECs)
- Duration: 2019-2023
- Principal investigator: Nicolas GIRARD, Thomas WALTER
- COMPO investigator: Laurent Greillier
RESILIENT
- Registration: NCT03088813
- Partner: Ipsen
- Title: Study of Irinotecan Liposome Injection (ONIVYDE®) in Patients With Small Cell Lung Cancer
- Duration: 2018-2022
- COMPO investigator: Laurent Greillier
Canopy-A
- Registration: NCT03447769
- Partner: Novartis Pharmaceuticals
- Title: Study of Efficacy and Safety of Canakinumab as Adjuvant Therapy in Adult Subjects With Stages AJCC/UICC v. 8 II-IIIA and IIIB (T>5cm N2) Completely Resected Non-small Cell Lung
- Duration: 2018-2027
- COMPO investigator: Laurent Greillier
NCT04350463
- Registration: NCT04350463
- Partner: Celgene
- Title: A Safety and Efficacy Study of CC-90011 in Combination With Nivolumab in Subjects With Advanced Cancers
- Duration: 2020-2024
- COMPO investigator: Laurent Greillier
TROPION-LUNG01
- Registration: NCT04656652
- Partner: Daiichi Sankyo, Inc., AstraZeneca
- Title: Study of DS-1062a Versus Docetaxel in Previously Treated Advanced or Metastatic Non-small Cell Lung Cancer Without Actionable Genomic Alterations
- Duration: 2020-2024
- COMPO investigator: Laurent Greillier
MERMAID-1
- Registration: NCT04385368
- Partner: AstraZeneca
- Title: Phase III Study to Determine the Efficacy of Durvalumab in Combination With Chemotherapy in Completely Resected Stage II-III Non-small Cell Lung Cancer (NSCLC)
- Duration: 2020-2026
- Principal investigator: Solange Peters, Charles Swanton
- COMPO investigator: Laurent Greillier
NCT03840902
- Registration: NCT03840902
- Partner: EMD Serono Research and Development Institute, Inc., Merck KGaA
- Title: M7824 With cCRT in Unresectable Stage III Non-small Cell Lung Cancer (NSCLC)
- Duration: 2019-2023
- COMPO investigator: Laurent Greillier
CARMEN-LC03
- Registration: NCT04154956
- Partner: Sanofi
- Title: SAR408701 Versus Docetaxel in Previously Treated, Carcinoembryonic Antigen-related Cell Adhesion Molecule 5 (CEACAM5) Positive Metastatic Non-squamous Non-small Cell Lung Cancer Patients
- Duration: 2020-2024
- COMPO investigator: Laurent Greillier
SKYSCRAPER-03
- Registration: NCT04513925
- Partner: Hoffmann-La Roche
- Title: A Study of Atezolizumab and Tiragolumab Compared With Durvalumab in Participants With Locally Advanced, Unresectable Stage III Non-Small Cell Lung Cancer (NSCLC)
- Duration: 2020-2027
- COMPO investigator: Laurent Greillier
NCT03899155
- Registration: NCT03899155
- Partner: Bristol-Myers Squibb
- Title: Pan Tumor Nivolumab Rollover Study
- Duration: 2019-2025
NCT03798535
- Registration: NCT03798535
- Partner: AstraZeneca
- Title: First Real-world Data on Unresectable Stage III NSCLC Patients Treated With Durvalumab After Chemoradiotherapy
- Duration: 2018-2023
- COMPO investigator: Laurent Greillier
IMbrella A
- Registration: NCT03148418
- Partner: Hoffmann-La Roche
- Title: A Study in Participants Previously Enrolled in a Genentech- and/or F. Hoffmann-La Roche Ltd-Sponsored Atezolizumab Study
- Duration: 2017-2030
- COMPO investigator: Laurent Greillier
BEAT-meso
- Registration: NCT03762018
- Partner: European Thoracic Oncology Platform, Hoffmann-La Roche
- Title: Bevacizumab and Atezolizumab in Malignant Pleural Mesothelioma
- Duration: 2019-2024
- Principal investigator: Enriqueta Felip, Sanjay Popat
- COMPO investigator: Laurent Greillier
SPECTA
- Registration: NCT02834884
- Partner: European Organisation for Research and Treatment of Cancer - EORTC
- Title: Screening Cancer Patients for Efficient Clinical Trial Access
- Duration: 2017-2026
- Principal investigator: Vassilis Golfinopoulos
- COMPO investigator: Laurent Greillier
TROPION-Lung05
- Registration: EudraCT 2020-002774-27
- Partner: Daiichi Sankyo, Inc.
- Title: Phase 2 Study of DS-1062a in Advanced or Metastatic Non-small Cell Lung Cancer with Actionable Genomic Alterations
- Starting year: 2021
- COMPO investigator: Laurent Greillier
SKYSCRAPER-06
- Registration: NCT04619797
- Partner: Hoffmann-La Roche
- Title: A Study of Tiragolumab in Combination With Atezolizumab Plus Pemetrexed and Carboplatin/Cisplatin Versus Pembrolizumab Plus Pemetrexed and Carboplatin/Cisplatin in Participants With Previously Untreated Advanced Non-Squamous Non-Small Cell Lung Cancer
- Duration: 2020 - 2025
- COMPO investigator: Laurent Greillier
PERSEE
- Registration: EudraCT 2020-002626-86
- Partner: CHRU de Brest
- Title: A trial comparing the pembrolizumab platinum based chemotherapy combination with pembrolizumab monotherapy in first line treatment of non small-cell lung cancer (NSCLC) patients
- Starting year: 2020
- Principal investigator: Renaud Descourt, Chantal Decroisette, Christos Chouaid
- COMPO investigator: Laurent Greillier
SAPPHIRE
- Registration: EudraCT 2019-001043-41
- Partner: Mirati Therapeutics, Inc.
- Title: A Randomized Phase 3 Study of Sitravatinib in Combination with Nivolumab Versus Docetaxel in Patients with Advanced Non-Squamous Non-Small Cell Lung Cancer with Disease Progression On or After Platinum-Based Chemotherapy and Checkpoint Inhibitor Therapy
- Starting year: 2020
- COMPO investigator: Laurent Greillier
Nivothym
- Registration: EudraCT 2015-005504-28
- Partner: European Organisation for Research and Treatment of Cancer
- Title: Single-arm, multicenter, phase II study of immunotherapy in patients with type B3 thymoma and thymic carcinoma previously treated with chemotherapy
- Starting year: 2017
- Principal investigator: Nicolas Girard, Solange Peters
- COMPO investigator: Laurent Greillier
ELEVATE HNSCC
- Registration: NCT04854499
- Partner: Gilead Sciences
- Title: Study of Magrolimab Combination Therapy in Patients With Head and Neck Squamous Cell Carcinoma
- Duration: 2021-2-25
- COMPO investigator: Sébastien Salas
ROMANE
- Registration: ML43492
- Partner: ROCHE
- Title: Retrospective observational study of patients with locally advanced squamous cell carcinoma of the head and neck
- Starting year: 2023
- COMPO investigator: Sébastien Salas
XRAY VISION
- Registration: NCT05386550
- Partner: EMD Serono Research & Development Institute, Inc.
- Title: Phase III Xevinapant (Debio 1143) and Radiotherapy in Resected LA SCCHN, High Risk, Cisplatin-ineligible Participants
- Duration: 2022-2030
- COMPO investigator: Sébastien Salas
Iintune-1
- Registration: NCT04420884
- Partner: Takeda
- Title: A Study of Dazostinag as Single Agent and Dazostinag in Combination With Pembrolizumab in Adults With Advanced or Metastatic Solid Tumors
- Duration: 2020-2026
- COMPO investigator: Sébastien Salas
AHEAD-MERIT
- Registration: NCT04534205
- Partner: BioNTech SE
- Title: A Clinical Trial Investigating the Safety, Tolerability, and Therapeutic Effects of BNT113 in Combination With Pembrolizumab Versus Pembrolizumab Alone for Patients With a Form of Head and Neck Cancer Positive for Human Papilloma Virus 16 and Expressing the Protein PD-L1
- Duration: 2021-2028
- COMPO investigator: Sébastien Salas
CODEBREAK IGR
- Registration: NCT05631249
- Partner: Gustave Roussy, Cancer Campus, Grand Paris
- Title: Sotorasib in Previously Treated Locally Advanced or Metastatic NSCLC Subjects With Mutated KRAS p.G12C
- Duration: 2022-2026
- COMPO investigator: Laurent Greillier
ADAPTABLE
- Registration: NCT05781308
- Partner: Intergroupe Francophone de Cancerologie Thoracique
- Title: Combination of Paclitaxel-bevacizumab ± Atezolizumab in Patients With Advanced NSCLC Progressing After Immunotherapy & Chemotherapy
- Duration: 2023-2026
- COMPO investigator: Laurent Greillier
MATISSE
- Registration: NCT05742607
- Partner: Innate Pharma
- Title: IPH5201 and Durvalumab in Patients With Resectable Non-Small Cell Lung Cancer
- Duration: 2023-2026
- COMPO investigator: Laurent Greillier
DESTINY-Lung04
- Registration: NCT05048797
- Partner: AstraZeneca
- Title: A Study to Investigate the Efficacy and Safety of Trastuzumab Deruxtecan as the First Treatment Option for Unresectable, Locally Advanced/Metastatic Non-Small Cell Lung Cancer With HER2 Mutations
- Duration: 2021-2027
- COMPO investigator: Laurent Greillier
KRYSTAL-12
- Registration: NCT04685135
- Partner: Mirati Therapeutics Inc.
- Title: Phase 3 Study of MRTX849 (Adagrasib) vs Docetaxel in Patients With Advanced Non-Small Cell Lung Cancer With KRAS G12C Mutation
- Duration: 2021-2024
- COMPO investigator: Laurent Greillier
PECATI
- Registration: NCT04710628
- Partner: MedSIR
- Title: Combination of Pembrolizumab and Lenvatinib, in Pre-treated Thymic CArcinoma paTIents
- Duration: 2021-2026
- COMPO investigator: Laurent Greillier
A2A-005
- Registration: NCT05403385
- Partner: iTeos Belgium SA
- Title: Study of Inupadenant (EOS100850) With Chemotherapy as Second Line Treatment for Nonsquamous Non-small Cell Lung Cancer
- Duration: 2022-2025
- COMPO investigator: Laurent Greillier
Delivir
- Registration: NCT05788926
- Partner: Transgene
- Title: A Clinical Trial of TG6050 in Patients With Metastatic Non-Small Cell Lung Cancer
- Duration: 2023-2025
- COMPO investigator: Laurent Greillier
BEAMION-Lung 1
- Registration: NCT04886804
- Partner: Boehringer Ingelheim
- Title: A Study to Test Different Doses of BI 1810631 in People With Different Types of Advanced Cancer (Solid Tumours With Changes in the HER2 Gene)
- Duration: 2021-2027
- COMPO investigator: Laurent Greillier
ELEVATE Lung & UC
- Registration: NCT04827576
- Partner: Gilead Sciences
- Title: Study of Magrolimab in Patients With Solid Tumors
- Duration: 2021-2025
- COMPO investigator: Laurent Greillier
IDE397-001
- Registration: NCT04794699
- Partner: IDEAYA Biosciences
- Title: Study of IDE397 in Participants With Solid Tumors Harboring MTAP Deletion
- Duration: 2021-2024
- COMPO investigator: Laurent Greillier
CA099-003
- Registration: NCT05407675
- Partner: Bristol-Myers Squibb
- Title: A Study to Evaluate the Safety and Tolerability of BMS-986408 Alone and in Combination With Nivolumab or Nivolumab and Ipilimumab in Participants With Advanced Solid Tumors
- Duration: 2022-2027
- COMPO investigator: Laurent Greillier
10 Partnerships and cooperations
10.1 International research visitors
10.1.1 Visits of international scientists
Mahsa Dehgani
-
Status
PhD student
-
Institution of origin:
Tabriz University of Medical Sciences
-
Country:
Iran
-
Dates:
Oct - Dec 2023
-
Mobility program/type of mobility:
Research stay
Ana Homsek
-
Status
Assistant Professor
-
Institution of origin:
School of Pharmacy of Belgrade
-
Country:
Serbia
-
Dates:
Oct - Oct 2023
-
Mobility program/type of mobility:
PROGRAMME DE RECHERCHES PHC - Pavle Savic France-Serbie: Bioanalysis and Pharmacometrics of Pembrolizumab in NSCLC.
Zeyab Ibrahim
-
Status
Senior Scientist
-
Institution of origin:
Perkins Institute, Perth Australia West University
-
Country:
Australia
-
Dates:
Oct - Oct 2023
-
Mobility program/type of mobility:
Winston Churchill Grant - implementing PGx in Oncology
10.1.2 Visits to international teams
Research stays abroad:
Anne Rodallec
-
Visited institution:
Departement of pharmaceutics, Bonn University
-
Country:
Germany
-
Dates:
Sept 203 - Aug 2024
-
Context of the visit:
Research collaboration
-
Mobility program/type of mobility:
Research Stay
10.2 National initiatives
QUANTIC
-
Title:
QUANTitative modeling combined to statistical learning to understand and predict resistance to Immune-checkpoint inhibition in non-small cell lung Cancer
-
Partner Institutions:
- AP-HM, Marseille
- Inserm, Marseille
- Aix-Marseille University, Marseille
- Veracyte, Marseille
- InnatePharma, Marseille
-
Date/Duration:
2019 - 2023
-
Funding:
338 k€, Inserm Plan Cancer MIC
-
Principal investigator:
S. Benzekry
-
COMPO members involved:
L. Greillier, M. Karlsen, P. Dufosse.
PIONeeR - biomarkers
-
Title:
Precision Immuno-Oncology for advanced Non-small cell lung cancer patients with PD-1 ICI Resistance
-
Partner Institutions:
- AP-HM, Marseille
- 13 national cancer centers, including Centre Lyon Bérard and IUCT in Toulouse
- Marseille Immmunopôle (immuno-monitoring platform)
- Vasculo-monitoring platform of AP-HM
- Inserm
- Aix-Marseille University
- Veracyte, Marseille
- InnatePharma, Marseille
-
Date/Duration:
2018 - 2024
-
Funding:
8 M€, ANR
-
Principal investigator:
F. Barlesi (IGR)
-
COMPO members involved:
S. Benzekry, J. Ciccolini, L. Greillier, P. Dufosse, M. Boussena, M. Hamimed, M. Karlsen, S. Marolleau, C. Marin, A. Vaglio.
LUCA-pi RHU
-
Title:
Lung cancer prevention and interception
-
Partner Institutions:
- Gustave Roussy Institute (G. Kroemer, L. Zitvogel)
- Therapanacea (N. Paragios)
- CIML (P. Milpied)
-
Date/Duration:
2023 - 2028
-
Funding:
10 M€, ANR
-
Principal investigator:
D. Boulate (COMPO)
-
COMPO seniors:
D. Barbolosi, S. Benzekry
DIGPHAT PEPR Digital Health
-
Title:
Digital pharmacological twin
-
Partner Institutions:
- JB Woillard (CHU Limoges)
- C. Battail (CEA Grenoble)
- M. Ursino + S. Zohar (HEKA, Inria, Paris)
- J. Josse (PREMEDICAL, Inria, Montpellier)
- E. Chatelut + M. White-Koning (IUCT, Toulouse)
-
Date/Duration:
2023 - 2028
-
Funding:
Total 1.8 M€, COMPO 251k€
-
Principal investigator:
JB Woillard (CHU Limoges)
-
COMPO senior:
S. Benzekry.
-
COMPO junior:
A. Bakhmach
COPYCAT
-
Title:
Combining Organoid technology with Mathematics to develop innovative models mimicking tumor cellular heterogeneity and plasticity for pediatric oncology
-
Partner Institutions:
- L. Broutier (CRCL, Inserm, CNRS, UCBL, Lyon)
- E. Pasquier (CRCM)
- R. Mounier (INMG, Inserm, CNRS, UCBL, Lyon)
-
Date/Duration:
2023 - 2027
-
Funding:
Total 922k€, COMPO 115k€ (INCa)
-
Principal investigator:
L. Broutier (CRCL, Inserm, CNRS, UCBL, Lyon)
-
COMPO members involved:
S. Benzekry.
PhD H. Hamdache
-
Title:
Improving therapeutic efficacy and managing side effects and sequelae in pediatric cancer through improved and personalized nutritional programs using computer simulations
-
Partner Institutions:
- V. Pancaldi (IUCT, Inserm, Toulouse)
-
Date/Duration:
2023 - 2026
-
Funding:
120k€, Inria-Inserm PhD grant
-
Principal investigator:
V. Pancaldi (IUCT, Inserm, Toulouse)
-
COMPO members involved:
S. Benzekry (co-supervisor).
South-ROCK
-
Title:
South-research on cancer for kids
-
Partner Institutions:
28 constitutive teams
- P. Mehlen (Centre Léon Bérard + Hospices Civils de Lyon)
- E. Pasquier (CRCM, Marseille)
- M. Castets (CRCL, Lyon)
-
Date/Duration:
2023 - 2028
-
Funding:
Total 2 M€
-
Principal investigators:
P. Mehlen (CLB + HCL, Lyon), E. Pasquier (CRCM, Marseille), M. Castets (CRCL, Lyon)
-
COMPO seniors:
S. Benzekry, F. Gattacceca, J.Ciccolini
THERMONANO
-
Title:
Nanoassemblies for the subcutaneous self-administration of anticancer drugs
-
Partner Institution:
- Institut Galien Paris-Saclay (UMR CNRS 8612)
-
Date/Duration:
2019 - 2024
-
Funding:
1.8 M€, ERC
-
Principal investigator:
J. Nicolas (Institut Galien, Paris-Sud)
-
COMPO members involved:
A. Rodallec, S. Benzekry, S. Marolleau.
PEMBOV
-
Title:
Pembrolizumab in Combination With Bevacizumab and Pegylated Liposomal Doxorubicin in Patients With Ovarian Cancer
-
Partner Institution:
- Institut Gustave Roussy (IGR)
-
Date/Duration:
2021-2024
-
Funding:
300 K€, INCa
-
Principal investigator:
J. Michels (IGR)
-
COMPO members involved:
J. Ciccolini, M. Hamimed
PEMEBRAIN
-
Title:
Intrathecal administration of low dose Pemetrexed in patients with brain metastasis: pharmacokinetics exploration
-
Partner Institution:
- Assistance Publique Hôpitaux de paris(APHP)
-
Date/Duration:
2022-2023
-
Funding:
APHP
-
Principal investigator:
P. Decq (APHP)
-
COMPO members involved:
J. Ciccolini, C. Boéri
10.3 Regional initiatives
SChISM
-
Title:
Size CfDNA Immunotherapies Signature Monitoring
-
Partner Institutions:
- APHM
- Adelis (JC Garcia, F. Ginot),
- ID-Solutions Oncology (F. Fina)
- XPOP Inria (M. Lavielle)
-
Date/Duration:
2022 - 2025
-
Funding:
120k€, Institut Laënnec
-
Principal investigator:
S. Salas (clinical, COMPO), S. Benzekry (modeling, COMPO)
-
COMPO junior members:
L. Nguyen
Brain meta SCLC
-
Title:
Modeling the impact of prophylactic whole brain radiotherapy to prevent brain metastases in SCLC
-
Partner Institutions:
- APHM (L. Padovani)
-
Date/Duration:
2023 - 2024
-
Funding:
100k€, Inria-Inserm PhD grant
-
Principal investigator:
S. Benzekry (COMPO)
-
COMPO senior members:
X. Muracciole
-
COMPO junior members:
C. Bigarré, A. Daumas
PhD M. Boussena
-
Title:
Machine learning methods for clinical oncology data: application to the prediction of immunotherapy response in lung cancer
-
Partner Institutions:
- J. Josse (PREMEDICAL, Inria)
- GFPC
-
Date/Duration:
2023 - 2026
-
Funding:
120k€, Institut Laënnec
-
Principal investigator:
S. Benzekry (COMPO)
-
COMPO senior:
L. Greillier
-
COMPO junior:
M. Boussena (PhD)
TORNADO
-
Title:
TOols for Rational NAnoDrugs Optimization: development of a physiologically-based pharmacokinetic model for nanomedicines
-
Partner Institutions:
- P. Garrigue (CERIMED, Marseille)
- S. Legeay (MINT, Univ Angers)
- E. Roger (MINT, Univ Angers)
-
Date/Duration:
2021 - 2024
-
Funding:
120k€, PhD grant AMU
-
Principal investigator:
F. Gattacceca (COMPO)
-
COMPO junior:
J. Ou (PhD)
PKID
-
Title:
Population pharmacokinetic approach centered on the patient: a patient pharmacokinetic ID from one drug to predict the PK of the next one
-
Partner Institutions:
- R. Guilhaumou (APHM, Marseille)
-
Date/Duration:
2021 - 2024
-
Funding:
APHM (hospital intern)
-
Principal investigator:
F. Gattacceca (COMPO)
-
COMPO junior:
A. Deschamps (PhD)
PICOMALE
-
Title:
Phase I Clinical trials Oncology & Machine Learning
-
Partner Institutions:
- N. André (APHM, CRCM)
- M. Ouladsine (LIS, AMU)
-
Date/Duration:
2023 - 2026
-
Funding:
Total 100k€, COMPO 33k€
-
Principal investigator:
N. André (clinical, APHM)
-
COMPO senior:
S. Benzekry
-
COMPO junior:
C. Bigarré (PhD)
COMPLICITY
-
Title:
COMPutationaL tools for NanoBooster In Cancer ImmunoTherapY
-
Partner Institution:
- Pancreas Cancer Team (CRCM)
-
Date/Duration:
2022-2024
-
Funding:
25 K€ (Amidex), 30K€ (ARC), 2K€(DAAD)
-
Principal investigator:
A. Rodallec (CRCM)
-
COMPO members involved:
A. Rodallec, F. Gattacceca, C. Perez
PREVALUNG ETOILE
-
Title:
Structuration d’un programme de dépistage des cancers du poumon incluant un phénotypage clinique, radiologique et biologique utile au développement d’outils de prédiction individualisée du risque.
-
Partner Institution:
- APHM
-
Date/Duration:
2023
-
Funding:
15 K€, Amidex
-
Principal investigator:
D. Boulate (COMPO)
-
COMPO senior:
D. Barbolosi
-
COMPO junior:
A. Bakhmach
kML-m
-
Title:
kinetics-Machine Learning for advanced melanoma treated with immunotherapy
-
Partner Institutions:
- N. Malissen, J. Forestier (APHM)
-
Date/Duration:
2023 - 2024
-
Funding:
No dedicated funding
-
Principal investigators:
N. Malissen (clinical, APHM) S. Benzekry (modeling, COMPO)
-
COMPO junior:
A. Daumas (M2 intern)
11 Dissemination
Participants: Dominique Barbolosi, Sebastien Benzekry, David Boulate, Joseph Ciccolini, Raphaelle Fanciullino, Florence Gattacceca, Laurent Greillier, Xavier Muracciole, Anne Rodallec, Sebastien Salas.
11.1 Promoting scientific activities
11.1.1 Scientific events: organisation
General chair, scientific chair
- D. Barbolosi and X. Muracciole organized the first Mathematics and Medicine workshop in Ajaccio. It was a large success and was held in presence of national leaders in clinical oncology, including Prs F. Barlesi, F. Andre and E. Deutsch, respectively CEO, head of research and chair of the radiation therapy department, Gustave Roussy Institute.
- J. Ciccolini
- Secretary to the PAMM-EORTC European group.
- Board Member of the GPCO-Unicancer group.
- Board Member of the Copil H&N Taskforce group.
- Co-Head of the Translational Research and Innovative Treatment (Translate-IT) Department of the Centre de Recherche en Cancérologie de Marseille (CRCM).
- Member of the "TDM in Oncology" Committee for the organization of the International Association of Therapeutic Drug Monitoring and Clinical Toxicology (IATDMCT) which ran its 21th International Congress in Oslo, Norway, Sept 24-27 2023 (850 attendees, worldwide audience).
- F. Gattacceca
- President of the GEPK (Group of Lecturers in PK) and organized the 2023 annual meeting, Lille, France, July 3-4, 2023.
- Member of the scientific committee and chair of the workshop "First in human dose selection" organized by GMP (Group of Metabolism and Pharmacokinetics), Paris, France, Oct 17 2023.
- Founding member and the secretary of the "Treatment individualization joint GMP-SFPT (French Society of Pharmacology and Therapeutics) commission" and co-organized and chaired the commission workshop "Therapeutic Monitoring and treatment Individualisation" with GMP, Paris, France, Oct 18 2023.
11.1.2 Journal
Member of the editorial boards
- S. Benzekry, Mathematical Biosciences
- J. Ciccolini, Cancer Chemotherapy & Pharmacology (Springer)
- F. Gattacceca, Cancer Chemotherapy & Pharmacology (Springer)
- J. Ciccolini, Guest Editor Themed Issue British Journal of Cancer (Nature Publishing Group)
- L. Greillier, Associate Editor at Respiratory Medicine & Research (French Society of Respiratory Diseases)
Reviewer - reviewing activities
- S. Benzekry: Cancer Letters
- J. Ciccolini: Cancer Chemotherapy & Pharmacology, British Journal of Cancer, Clin Pharmacol Ther, Clin Pharmacol Ther PSP, British Journal of Clinical Pharmacology, Cancer Treatment Review, Frontiers in Pharmacology, Frontiers in Immunology, Cancers, Cancer Letters, Pharmaceutics, Oncotarget, Journal of Chromatography B, ESMO open, Fundamental and Clinical Pharmacology, Breast Cancer: Targets & Therapy, Journal of the National Comprehensive Cancer Network.
- R. Fanciullino: Cancer Chemotherapy & Pharmacology, Pharmaceutical Research, Leukemia
- F. Gattacceca: Cancer Chemotherapy & Pharmacology, Annales Pharmaceutiques Françaises
- L. Greillier, Respiratory Medicine & Research
11.1.3 Invited talks
- S. Benzekry
- October, 2023. Workshop for young researchers in Cancer, invited as keynote speaker, Cancéropôle PACA, Porquerolles.
- July, 2023. SMB congress (Society of Mathematical Biology). Talk within the mini-symposium "modeling approaches used to study cancer treatment." organized by K. Wilkie and G. Powathil. L. Nguyen et al., S. Benzekry, Mechanistic modeling of the longitudinal tumor and biological markers combined with quantitative cell-free DNA. Ohio State University, OH, USA.
- June, 2023. Annual meeting of the APHM center for early phase clinical trials in oncology, Marseille, France.
- June, 2023. Annual congress of the French group of clinical pneumo-oncology (GPCO), Toulon, France.
- June, 2023. Annual congress of the French society of pharmacology and therapeutics (SFPT), Limoges, France.
- May, 2023. SMAI congress (French Society of Applied and Industrial Mathematics). Talk within the mini-symposium "Modélisation Mathématique du cancer et de son environnement" organized by F. Hubert and M. Mezache. Pointe à Pitre, France.
- January, 2023. CARe graduate school workshop, Numerical and technical tools to better investigate metabolism, inflammation and cancer. Toulouse, France.
- J. Ciccolini
- November, 2023. "Anti-Body Drug Conjugates: Why « Magic Bullet » is not « Magic Pharmacology" XIeme Journées Pharmacologiques de L'Hôpital Cochin, Paris France.
- November, 2023. "Anti-Body Drug Conjugates: which clinical perspectives in digestive oncology?" Cours Saint Paul Oncologie Digestive Nice France.
- November, 2023. "ADCs: Pharmacokinetics & dosing considerations".XIXeme Journées du GPCO-Unicancer Strasbourg, France.
- November, 2023. "Anti-PARP: Aspects PK et PK/PD quelle place pour le TDM?" XIXèmes Journées du GPCO-Unicancer Strasbourg, France.
- October, 2023. "Cancer de la prostate : implications PK et PK/PD d'un antagoniste oral de la LHRH".14ème Congrès de la SFPO - société Française des Pharmaciens Oncologues Nancy, France
- September, 2023. "BRAF-i plus anti-MEK combo: targeting MAP-kinase in metastatic melanoma". 4ème Journées Horizon Mélanome Paris, France
- September, 2023."Difficulties in implementing TDM in oncology: another French paradox.IATDMCT 2023 - 21st Congress of the International Association of Therapeutic Drug Monitoring & Clinical Toxicology Oslo (Norway), Norway
- July, 2023. "Antibody-Drug Conjugates ; beyond the hype, which pharmacology in breast cancer?" Séminaire annuel du Cancéropôle PACA Frejus, France
- June, 2023. "TDM of oral targeted therapies in metastatic melanoma." Pierre Fabre Symposium Avène, France.
- May, 2023. "Unlocking the potential of ADCs for HER2 signaling pathway."" Daïchi Sankyo Breast Symposium Toulon, France
- April, 2023. "BRAF-inhibitors in mCRC: pharmacology and PK/PD perspectives". Aquitaine Gastro Annual Meeting Bordeaux, France.
- March, 2023. "From HER2+ to HER2-low: specificity of the new generation ADCs". 9ème Congrès du Monaco Age Oncology (MAO) Monaco.
- January, 2023. "PKPD with Immunotherapy".7e Journées Scientifiques Immunité & Cancer (JSIC) Paris, France
- January, 2023. "TDM in oncology: application to breast cancer patients." 22ème Cours Saint Paul Sein & Cancer Gynécologiques Cannes, France
- January, 2023. "Interaction of PGxs with Pharmacometric and modelling platform" APSA-ASCEPT 2022 JOINT conference Perth Western Australia.
- R. Fanciullino
- December, 2023. "Médicament anti-cancéreux et intégration des données vie réelle". Meeting de la Société Française de Pharmacie Clinique (SFPC); Lyon France.
- F. Gattacceca
- June-July, 2023 (2 sessions per week during 8 weeks). Invited instructor for the online "Summerschool PBPK SimulationsPlus".
- L. Greillier
- June 2023, "Real-life efficacy of nivolumab plus ipilimumab in untreated, unresectable malignant pleural mesotheliomas: results of French early access program". 16th International Conference of the International Mesothelioma Interest Group, Lille, France.
11.1.4 Scientific expertise
- S. Benzekry: COFECUB program
- J. Ciccolini: INCa Scientific Committee (PHRC-K program, Reviewer)
- J. Ciccolini: ITMO Cancer (Board Member)
- J. Ciccolini: H&N Copil Unicancer (Board Member)
- J. Ciccolini: Israeli Ministry of Innovation, Science and Technology: Grant Application (Reviewer)
- J. Ciccolini: Scientific Board for the Groupe Francophone des Myelodysplasies (GFM): trial GFM-ONUVEN-MDS (Reviewer).
- J. Ciccolini: Conseil Scientifique Régional du Languedoc Roussillon de la Ligue contre le cancer (Reviewer)
- J. Ciccolini: Selection Scientific Committee, Tenured Track Assistant-Professor Application, College of Veterinary Sciences, Faculty of Animal Husbandry and Veterinary Sciences, Peshawar, Pakistan (Reviewer).
- R. Fanciullino: Member of the Scientific Committee for Association pour la Recherche contre le Cancer (ARC) Scientific Committee CN5 (Reviewer)
- F. Gattacceca: Scientific expert at ANSM (Agence Nationale de Sécurité du Médicament), member of the permanent scientific committee "Quality and Safety of drugs".
- L. Greillier: Scientific coordinator for the national recommendations on lung cancer management at the National Institute of Cancer.
- L. Greillier: Scientific expert at the National Institute of Cancer.
11.1.5 Research administration
- A. Rodallec: Website and Social Media coordinator for the CRS benelux Local Chapter
11.2 Teaching - Supervision - Juries
11.2.1 Teaching
- S. Benzekry: M2 Biologie Santé – Parcours IA biomarqueurs (6h). M2 "Pharmacokinetics" (6h).
- S. Benzekry: CESU "Fundamentals for modeling and simulation in pharmacokinetics/pharmacodynamics" (6h).
- C. Bigarré: CESU "Fundamentals for modeling and simulation in pharmacokinetics/pharmacodynamics" (12h). MSc (2nd y) "Pharmacokinetics" (12h).
- A. Rodallec: lectures in MSc in Pharmacokinetics, MSc in Digipharm, MSc in Innovative Diagnostic and therapeutic Drug Products, DESU "Advances courses in pharmacometrics", DES in animal experiments, Pharm.D. studies (2d, 3d, 4th and 6th year), -> 190 h a year + additional lectures at University of Paris Saclay.
- J. Ciccolini Lectures at Aix Marseille univ in: MSc (2d year) in Oncology, MSc (2d year) in Oncogenetics, MSc (2d year) in Pharmacokinetics, MSc (2d year) in Digipharm, MSc (1st year) in Drugs & Health Products, D.U. in Animal Experiments, D.U. in Genetic Councelling, Master Class in Lung Cancer, Pharm.D. studies (2d, 3d, 4th and 6th year) -> 190 h a year.
- Teaching outside of AMU: additional lectures in pharmacokinetics at Université Catholique de Lyon, University of Amsterdam NL, University of Pisa Italy, and the International School of Metronomics. Lectures for Cours National Approfondissement DES Oncologie Médicale and Phase d'Approfondissement Docteur Junior, Paris Saclay.
- R. Fanciullino: Lectures in: MSc (2d year) in Pharmacokinetics, MSc (2d year) in Digipharm, CESU in Oncogeriatry, DES in PK Variability, Pharm.D. studies (3d, 4th and 5th year) -> 190 h a year .
- F. Gattacceca: lectures at Aix-Marseille University school of pharmacy (305h), teaching in other universities (50h: Nîmes, Angers, Montpellier): 90% at a post-graduate level.
- F. Gattacceca: CIVIS International Summerschool "Drug Design and Discovery", Bucharest, Romania, July 2023 (Lectures and Hands-on, 10h)
- L. Greillier: M2 Recherche clinique et Simulation en Santé.
- L. Greillier: oncology and pulmonology for 3rd – 11 year medical students.
- X. Muracciole: DIU radio-urology for resident medical student.
- X. Muracciole: DCIU radio surgery for resident medical student
- S. Salas: Medical study/initial training. Seminary: palliative care Therapeutic module: pains Oncodigestive module Cancerology (44h), for 3rd - 6th year medical students
- S. Salas: Medical, paramedical study. DU Supportive care in oncology and palliative medicine. DU Wounds and healing. DIU Supportive care in oncology and palliative medicine. Master's in Advance Practice Nursing: Cancerology module, General module, pains. CEU Service providers at home, Home-based cancer care. DU Ambulatory shift,Oncology module. (37h)
11.2.2 Teaching administration
- J. Ciccolini: Founder and Head, Digipharm MSc degree (Aix Marseille Univ).
- F. Gattacceca
- Director of the Master Program “Pharmacokinetics”.
- Director of the international University Diploma: “Modeling and simulation: population approaches in pharmacokinetics/pharmacodynamics”
- Founder and Director of the international University Diploma: “Modeling and simulation: physiologically-based pharmacokinetic modeling for pharmacology and toxicology”
- Member of the national reflection committee for the industry pharmacy studies
- Member of the training steering committee of ICI (Immunology Cancer Institute)
11.2.3 Supervision
- Postdoc
- S. Benzekry
- P. Dufossé, QUANTIC, funding Laënnec Institute
- A. Rodallec
- R. Passannante, Thermonano
- P. Piris, Thermonano
- S. Benzekry
- PhD students
- S. Benzekry
- C. Bigarré, 2020 - 2024, Mathematical modeling for prediction of metastatic relapse in breast cancer, co-supervision X. Muracciole, funding Inria – Inserm
- L. Nguyen Phuong, 2022 - 2025, SChISM, Mechanistic modeling of circulating DNA combined to machine learning for prediction of response and survival following immunotherapy, co-supervision S. Salas, funding Amidex ICI (Institute for Cancer Immunotherapy)
- H. Hamdache, 2023 - 2026, Improving therapeutic efficacy and managing side effects and sequelae in pediatric cancer through improved and personalized nutritional programs using computer simulations, co-supervision V. Pancaldi (IUCT, Inserm, Toulouse), funding Inria–Inserm.
- J. Ciccolini
- M. Hamimed, Provin & Ovima trials, co-supervision N.André, funding AMU.
- G. Sicard, SMART A*MIDEX project, co-supervision R. Fanciullino, funding Amidex Foundation.
- C. Marin, CetuxIMAX, funding APHM & Merck Serono
- F. Gattacceca
- A. Deschamps, 2019-2024, funding APHM, co-supervision R. Guilhaumou (APHM)
- J. Ou, 2021-2024, funding AMU doctoral school 62, co-supervision P. Garrigue (AMU)
- S. Benamara, 2023-2026, funding CIFRE, co-supervision D. Teutonico (Sanofi)
- R. Fanciullino
- M. Dacos, Nanimmuno funding APHM & Institut Roche
- L. Osanno, Venaza project funding APHM
- S. Benzekry
- Engineers
- S. Benzekry
- M. Boussena, QUANTIC
- M. Karlsen, QUANTIC
- A. Vaglio, PIONeeR
- J. Ciccolini
- S. Marolleau, PIONeeR
- C. Perez, PIONeeR
- S. Benzekry
- Interns (Master 2)
- S. Benzekry
- A. Bakhmach, Ground-truth assessment of feature selection methods from real datasets, Erasmus Mundus Master: Be In Precision Medicine
- T. Gnade, Survival analysis: Adapting Loss Functions for Cox Regression with Neural Networks, M2 IAAA, Centrale Marseille
- R. Fanciullino
- A. Ronda, Predicting MTX toxicity, APHM
- J. Ciccolini
- D. Protzenko, Machine Learning for HD Busulfan, APHM
- G. Kallee, DPD UM status and treatment failure upon 5-FU, APHM
- A. Rodallec
- C. Perez, immunomodulation of nanoparticles, AMU
- F. Gattacceca
- M. Mahdjoub, Multi-compartmental model for Lipid nanocapsules biodistribution, AMU
- S. Benzekry
11.2.4 Juries
- Sebastien Benzekry
- Reviewer of the PhD of M. Pere, Université Côte d'Azur, Sophia Antipolis
- Reviewer of the PhD of P. Chassonnery, Université Toulouse 3 Paul Sabatier
- Member of the follow-up PhD committee of 3 students
- Joseph Ciccolini: President of Pham.D. Thesis, School of Pharmacy of Marseille (approx. 30 thesis/year)
- Joseph Ciccolini: Jury of Ph.D. Defense : Ph.D. Reviewer for the Simon Verdez (Bourgogne University), Arthur Geraud-Cremieux (Université Paris Saclay) and Christophe Maritaz (Université Paris Saclay); President for Aurélien Millet (Université of Lyon).
- Joseph Ciccolini: Member of the Scienfic Committee Evaluation for the Ph.D. of Magdallena Centanni, Upssala University, Sweden (supervisor: Mats Karlsson and Lena Friberg).
- Anne Rodallec: Jury of Pharm.D. Thesis, School of Pharmacy of Marseille (approx. 5 thesis/year)
- Raphaelle Fanciullino: Jury of Pharm.D. Thesis, School of Pharmacy of Marseille (approx. 10 thesis/year) Jury of DES Hospital Pharmacist thesis, School of Pharmacy of Marseille (approx. 10/year).
- Raphaelle Fanciullino: Reviewer for the Habilitation à Diriger les recherches (HDR) of Litaty M'Batchi (Montpellier University).
- Sebastien Salas: Jury of the HDR of Matthieu Frasca
- Sebastien Salas: President of M.D. Thesis, School of Medicine of Marseille (2 thesis)
- L. Greillier: President or member of the Jury (3 theses in medicine).
- F. Gattacceca: Reviewer of 1 HDR (A. Marsot, University of Montréal, Paris Oct 2023). Reviewer of 5 PhD (C. Pouzin, Paris, Jan 2023; R. Garreau, Lyon, Sept 2023; S. Baklouti, Toulouse, Oct 2023; S. Lobet, Tours, Oct 2023; F-C. Chao, Saclay, Dec 2023). Jury of 1 HDR (L. Mbatchi, Montpellier, June 2023).
- F. Gattacceca: Scientific supervisor of a PhD in VAE (validation of experience). M. le Merdy, SimulationsPlus, Paris, Jan 2023
12 Scientific production
12.1 Major publications
- 1 articleComputational oncology — mathematical modelling of drug regimens for precision medicine.Nature Reviews Clinical Oncology134April 2016, 242-254HALDOI
- 2 inproceedings3MO - Comprehensive biomarkers (BMs) analysis to predict efficacy of PD1/L1 immune checkpoint inhibitors (ICIs) in combination with chemotherapy: a subgroup analysis of the Precision Immuno-Oncology for advanced Non-Small CEll Lung CancER (PIONeeR) trial.Annals of Oncology2022 ESMO Immuno-Oncology Congress16suppl_1Geneva, Switzerland2022HAL
- 3 articleArtificial Intelligence and Mechanistic Modeling for Clinical Decision Making in Oncology.Clinical Pharmacology and TherapeuticsJune 2020HALDOI
- 4 articleMachine Learning for Prediction of Immunotherapy Efficacy in Non-Small Cell Lung Cancer from Simple Clinical and Biological Data.Cancers1324December 2021, 6210HALDOI
- 5 miscPredicting survival and trial outcome in non-small cell lung cancer integrating tumor and blood markers kinetics with machine learning.September 2023HALDOI
- 6 articleMechanistic modeling of metastatic relapse in early breast cancer to investigate the biological impact of prognostic biomarkers.Computer Methods and Programs in Biomedicine231April 2023, 107401HALDOI
- 7 articlePharmacokinetics and Pharmacodynamics-Based Mathematical Modeling Identifies an Optimal Protocol for Metronomic Chemotherapy.Cancer Research77172017, 4723-4733HALDOI
- 8 articleWomen at a Disadvantage in Fluorouracil Treatment.JAMA oncology26June 2016, 829HALDOI
- 9 articleCDA as a predictive marker for life-threatening toxicities in patients with AML treated with cytarabine.Blood Advances25February 2018, 462 - 469HALDOI
- 10 articleTowards Rational Cancer Therapeutics: Optimizing Dosing, Delivery, Scheduling, and Combinations.Clinical Pharmacology and Therapeutics1083September 2020, 458-470HALDOI
- 11 articleRevisiting bevacizumab + cytotoxics scheduling using mathematical modeling: proof of concept study in experimental non-small cell lung carcinoma.CPT: Pharmacometrics and Systems Pharmacology2018, 1-9HALDOI
- 12 articleMachine Learning and Mechanistic Modeling for Prediction of Metastatic Relapse in Early-Stage Breast Cancer.JCO Clinical Cancer Informatics4September 2020, 259-274HALDOI
12.2 Publications of the year
International journals
National journals
Invited conferences
International peer-reviewed conferences
Conferences without proceedings
Reports & preprints
Other scientific publications
12.3 Cited publications
- 74 articleExploiting Cancer’s Tactics to Make Cancer a Manageable Chronic Disease.Cancers1266Jun 2020, 1649DOIback to text
- 75 articleFrom patient-specific mathematical neuro-oncology to precision medicine..Front Oncol3AprilJan 2013, 62–62back to text
- 76 articleComputational Modelling of Metastasis Development in Renal Cell Carcinoma.PLoS Comput Biol111100007Nov 2015, e1004626DOIback to text
- 77 articleDetermination of the unmetabolized 18F-FDG fraction by using an extension of simplified kinetic analysis method: clinical evaluation in paragangliomas.Medical & biological engineering & computing541Jan 2016, 103–111DOIback to text
- 78 articleOncotarget829Jul 2017, 47161–47166DOIback to text
- 79 articleGlobal Dormancy of Metastases Due to Systemic Inhibition of Angiogenesis.PLoS ONE9100019Jan 2014, e84249-11DOIback to text
- 80 articleMathematical Modeling of Tumor-Tumor Distant Interactions Supports a Systemic Control of Tumor Growth..Cancer Research7718Sep 2017, 5183–5193DOIback to text
- 81 articleModeling Spontaneous Metastasis following Surgery: An In Vivo-In Silico Approach.Cancer Research76300000Feb 2016, 535–547DOIback to text
- 82 inproceedingsA mechanistic model for prediction of metastatic relapse in early-stage breast cancer using routine clinical features.AACR8212New Orléans, United StatesApril 2022, 2737HALDOIback to text
- 83 articleProgress and Opportunities to Advance Clinical Cancer Therapeutics Using Tumor Dynamic Models..Clinical cancer research : an official journal of the American Association for Cancer ResearchDec 2019, 1–22DOIback to text
- 84 articleStan: A Probabilistic Programming Language.Journal of Statistical Software761Jan 2017, 1–32DOIback to text
- 85 articleEmergence of drug tolerance in cancer cell populations: an evolutionary outcome of selection, nongenetic instability, and stress-induced adaptation.Cancer Research756Mar 2015, 930–939DOIback to text
- 86 articleMechanistic Learning for Combinatorial Strategies With Immuno-oncology Drugs: Can Model-Informed Designs Help Investigators?JCO Precision Oncology4May 2020, 486–491DOIback to textback to text
- 87 articleCombinatorial immunotherapy strategies: most gods throw dice, but fate plays chess.Annals of Oncology3011Nov 2019, 1690–1691DOIback to text
- 88 articleRealistic simulation of the 3-D growth of brain tumors in MR images coupling diffusion with biomechanical deformation.IEEE Transactions on Medical Imaging2410Oct 2005, 1334–1346DOIback to text
- 89 articleA new methodology to derive 3D kinetic parametric FDG PET images based on a mathematical approach integrating an error model of measurement.EJNMMI Research8Nov 2018, URL: https://www.ncbi.nlm.nih.gov/pmc/articles/PMC6238015/DOIback to text
- 90 articlePrediction of the Evolution of Thyroidal Lung Nodules Using a Mathematical Model..82Jan 2010, 37–38back to text
- 91 articleA multi-layered systems approach for renal cell carcinoma.bioRxivJan 2020, 2020.01.13.904235DOIback to text
- 92 articleConvergence of a stochastic approximation version of the EM algorithm.The Annals of Statistics271Zbl: 0932.62094Mar 1999, 94–128DOIback to text
- 93 articleA 2/1 Sunitinib Dosing Schedule Provides Superior Antitumor Effectiveness and Less Toxicity Than a 4/2 Schedule for Metastatic Renal Cell Carcinoma: A Systematic Review and Meta-Analysis.Frontiers in Oncology102020, 313DOIback to text
- 94 articleUsing the SAEM algorithm for mechanistic joint models characterizing the relationship between nonlinear PSA kinetics and survival in prostate cancer patients.Biometrics731Mar 2017, 305–312DOIback to text
- 95 articleNumerical solution of an inverse problem in size-structured population dynamics.Inverse Problems254Apr 2009, 045008–045008back to text
- 96 articleA phase Ia/Ib clinical trial of metronomic chemotherapy based on a mathematical model of oral vinorelbine in metastatic non-small cell lung cancer and malignant pleural mesothelioma: rationale and study protocol.BMC cancer161000002016, 278DOIback to text
- 97 articleIn vitro and in vivo reversal of resistance to 5-fluorouracil in colorectal cancer cells with a novel stealth double-liposomal formulation.British Journal of Cancer977Oct 2007, 919–926DOIback to text
- 98 articleMethodological aspects and pharmacological applications of three-dimensional cancer cell cultures and organoids.Life Sciences254Aug 2020, 117784DOIback to text
- 99 articlePembrolizumab plus Chemotherapy in Metastatic Non-Small-Cell Lung.Cancer. N Engl J Med3782078–20922018back to text
- 100 articleThe evolutionary history of lethal metastatic prostate cancer.Nature5207547Apr 2015, 353–357DOIback to text
- 101 articleThe elements of statistical learning: data mining, inference, and prediction, Springer Series in Statistics.2009back to text
- 102 articleRevisiting dosing regimen using PK/PD modeling: the MODEL1 phase I/II trial of docetaxel plus epirubicin in metastatic breast cancer patients.Breast Cancer Research and Treatment1562Mar 2016, 331–341DOIback to text
- 103 articleDevelopment of ipilimumab: contribution to a new paradigm for cancer immunotherapy.Seminars in Oncology375Oct 2010, 533–546DOIback to text
- 104 articleCalibrating a Predictive Model of Tumor Growth and Angiogenesis with Quantitative MRI.Annals of Biomedical Engineering477Jul 2019, 1539–1551DOIback to text
- 105 articleSingle-agent paclitaxel for the treatment of breast cancer: an overview.Seminars in Oncology231 Suppl 1Feb 1996, 4–9back to text
- 106 articleQuantitative evidence for early metastatic seeding in colorectal cancer.Nature Genetics5177Jul 2019, 1113–1122DOIback to text
- 107 articleRevisiting Bevacizumab + Cytotoxics Scheduling Using Mathematical Modeling: Proof of Concept Study in Experimental Non-Small Cell Lung Carcinoma..CPT: Pharmacometrics & Systems Pharmacology71Jan 2018, 42–50DOIback to text
- 108 articleA Dynamical Model for the Growth and Size Distribution of Multiple Metastatic Tumors.Journal of Theoretical Biology2032Mar 2000, 177–186DOIback to text
- 109 articleDynamic Risk Profiling Using Serial Tumor Biomarkers for Personalized Outcome Prediction.Cell1783Jul 2019, 699-713.e19DOIback to text
- 110 bookMixed Effects Models for the Population Approach.CRC PressJul 2014back to text
- 111 articleNonlinear mixed effects models for repeated measures data..Biometrics463Sep 1990, 673–687back to text
- 112 articleA phase II randomized trial of nivolumab with stereotactic body radiotherapy (SBRT) versus nivolumab alone in metastatic (M1) head and neck squamous cell carcinoma (HNSCC)..Journal of Clinical Oncology3615_supplMay 2018, 6009–6009DOIback to text
- 113 articleRevisiting Dosing Regimen Using Pharmacokinetic/Pharmacodynamic Mathematical Modeling: Densification and Intensification of Combination Cancer Therapy.Clinical Pharmacokinetics55800000Aug 2016, 1015–1025DOIback to text
- 114 articleModel driven optimization of antiangiogenics + cytotoxics combination: application to breast cancer mice treated with bevacizumab + paclitaxel doublet leads to reduced tumor growth and fewer metastasis.Oncotarget814Feb 2017, 23087–23098DOIback to text
- 115 articleIn Vivo Bioluminescence Tomography for Monitoring Breast Tumor Growth and Metastatic Spreading: Comparative Study and Mathematical Modeling.Scientific Reports600000Nov 2016, 36173DOIback to text
- 116 articleIntegrating microarray-based spatial transcriptomics and single-cell RNA-seq reveals tissue architecture in pancreatic ductal adenocarcinomas.Nature Biotechnology3833Mar 2020, 333–342DOIback to text
- 117 articleAvelumab plus Axitinib versus Sunitinib for Advanced Renal-Cell Carcinoma.New England Journal of Medicine38012Mar 2019, 1103–1115DOIback to text
- 118 phdthesisMathematical modelling of neoadjuvant antiangiogenic therapy and prediction of post-surgical metastatic relapse in breast cancer patients.Université de BordeauxOct 2019, URL: https://tel.archives-ouvertes.fr/tel-02560543back to text
- 119 articleMatrix metalloproteinases at cancer tumor–host interface.Seminars in Cell & Developmental Biology191Feb 2008, 52–60DOIback to text
- 120 articleAn alternative parameter for early forecasting clinical response in NSCLC patients during radiotherapy: proof of concept study.The British Journal of Radiology891062Jun 2016, 20160061DOIback to text
- 121 articleFrom 3D spheroids to tumor bearing mice: efficacy and distribution studies of trastuzumab-docetaxel immunoliposome in breast cancer.International Journal of Nanomedicine132018, 6677–6688DOIback to text
- 122 articleDefining the optimal murine models to investigate immune checkpoint blockers and their combination with other immunotherapies.Annals of Oncology: Official Journal of the European Society for Medical Oncology2772016, 1190–1198DOIback to text
- 123 articleOptimal Scheduling of Bevacizumab and Pemetrexed/cisplatin Dosing in Non-Small Cell Lung Cancer.CPT: pharmacometrics & systems pharmacologyApr 2019DOIback to text
- 124 articleAtezolizumab for First-Line Treatment of Metastatic Nonsquamous NSCLC..New England Journal of Medicine37824Jun 2018, 2288–2301DOIback to text
- 125 articleComprehensive analysis of the clinical immuno-oncology landscape..Ann. Oncol.291Jan 2018, 84–91DOIback to text
- 126 phdthesisMathematical modeling of antibody nanoconjugates transport in tumors.Bordeaux UniversityDec 2020back to text
- 127 articlePREDICT: a new UK prognostic model that predicts survival following surgery for invasive breast cancer..Breast cancer research121Jan 2010, R1DOIback to text