2023Activity reportProject-TeamAVIZ
RNSR: 200818367J- Research center Inria Saclay Centre at Université Paris-Saclay
- In partnership with:CNRS, Université Paris-Saclay
- Team name: Analysis and VIsualiZation
- In collaboration with:Laboratoire Interdisciplinaire des Sciences du Numérique
- Domain:Perception, Cognition and Interaction
- Theme:Interaction and visualization
Keywords
Computer Science and Digital Science
- A2.1.10. Domain-specific languages
- A3.1.4. Uncertain data
- A3.1.7. Open data
- A3.1.8. Big data (production, storage, transfer)
- A3.3. Data and knowledge analysis
- A3.3.1. On-line analytical processing
- A3.3.3. Big data analysis
- A3.5.1. Analysis of large graphs
- A5.1. Human-Computer Interaction
- A5.1.1. Engineering of interactive systems
- A5.1.2. Evaluation of interactive systems
- A5.1.6. Tangible interfaces
- A5.1.8. 3D User Interfaces
- A5.1.9. User and perceptual studies
- A5.2. Data visualization
- A5.6.1. Virtual reality
- A5.6.2. Augmented reality
- A6.3.3. Data processing
- A9.6. Decision support
Other Research Topics and Application Domains
- B1. Life sciences
- B1.1. Biology
- B1.2. Neuroscience and cognitive science
- B9.5.6. Data science
- B9.6. Humanities
- B9.6.1. Psychology
- B9.6.3. Economy, Finance
- B9.6.6. Archeology, History
- B9.6.10. Digital humanities
1 Team members, visitors, external collaborators
Research Scientists
- Jean Daniel Fekete [Team leader, INRIA, Senior Researcher, HDR]
- Tobias Isenberg [INRIA, Senior Researcher, HDR]
- Petra Isenberg [INRIA, Senior Researcher, HDR]
Faculty Member
- Frederic Vernier [UNIV PARIS SACLAY, Associate Professor]
Post-Doctoral Fellows
- Florent Cabric [INRIA, Post-Doctoral Fellow]
- Alexis Pister [University of Edinburgh, from Mar 2023 until Sep 2023]
- Mickael Sereno [INRIA, Post-Doctoral Fellow]
PhD Students
- Katerina Batziakoudi [BERGER-LEVRAULT, CIFRE, from Feb 2023]
- Tingying He [UNIV PARIS SACLAY]
- Jiayi Hong [INRIA, until Mar 2023]
- Mohammad Alaul Islam [INRIA, until Mar 2023]
- Yucheng Lu [UNIV PARIS SACLAY, from Nov 2023]
- Lijie Yao [INRIA]
Technical Staff
- Ludovic David [INRIA, Engineer]
- Olivier Gladin [INRIA, Engineer]
- Hande Gozukan [INRIA, Engineer]
- Christian Poli [INRIA, Engineer]
Interns and Apprentices
- Anne-Flore Cabouat [UNIV PARIS SACLAY, Intern, from Oct 2023]
- Anne-Flore Cabouat [INRIA, Intern, from Mar 2023 until Aug 2023]
- Yucheng Lu [UNIV PARIS SACLAY, Intern, from Mar 2023 until Aug 2023]
- Lisa Taldir [Université Paris-Saclay, Intern, from May 2023 until Jul 2023]
Administrative Assistant
- Katia Evrat [INRIA]
Visiting Scientists
- Fairouz Grioui [Univ Stuttgart, until Mar 2023]
- Johannes Knittel [University of Stuttgart, until Jan 2023]
- Gabriela Carolina Molina Leon [Univ Bremen, from May 2023 until Jul 2023]
- Hendrik Strobelt [IBM Research, until Jan 2023]
- Junxiu Tang [Univ Zhejiang, from Nov 2023]
- Karelia Alexandra Vilca Salinas [UNIV SAO PAULO, from Apr 2023]
External Collaborator
- Gabriela Carolina Molina Leon [Univ Bremen, from Aug 2023]
2 Overall objectives
2.1 Objectives
Aviz (Analysis and VIsualiZation) is a multidisciplinary project that seeks to improve data exploration methods, techniques, and tools based on Interactive Visualization. Visualization, in general, refers to the graphical representation of data or concepts to aid access, distribution or explanations of data. Card et al. give a general definition for visualization as
“the use of computer-supported, interactive, visual representations of data to amplify cognition.” 42
Taking this definition, visualization is a means of creating visual aids that lead to insight in the underlying data sets. It is not about producing nice pictures but about making data understandable and explorable so that visualizations help viewers gain knowledge about the data. It is about aiding the process of forming a mental model for the acquired data and so helping the viewer to understand underlying concepts, patterns, and connections within the data 67. In partiular, visualization has the goal to improve humans' sensemaking of complex data by taking advantage of the capabilities of their vision system: visual information can be processed in parallel and with a high bandwidth into the human cognitive centers 75. Ware defines five advantages of visualization 75:
- Comprehension: Supports the comprehension of large amounts of data.
- Pattern Perception: Previously unnoticed properties of data may emerge.
- Problem Analysis: Problems within the data may become immediately apparent.
- Adaptability: facilitates understanding of large- and small-scale features of data.
- Interpretation: Hypothesis formulation is facilitated.
Three main areas of visualization have evolved in the computer science community: Scientific Visualization, Information Visualization, and Visual Analytics. Scientific visualization is primarily concerned with displaying real or simulated scientific data. Basic visualization techniques for this area include surface rendering, volume rendering, and animation. Typical examples include processing of satellite photographs, fluid flows, or medical data. Datasets in information visualization typically come from large information spaces or information systems and are both structured or unstructured. Examples include network data, multi-dimensional tables of abstract measurements, or unstructured data such as text. Visual analytics, finally, is concerned with augmenting human-led data exploration with automatic techniques such as machine learning. The Aviz team has expertise in all three areas of visualization.
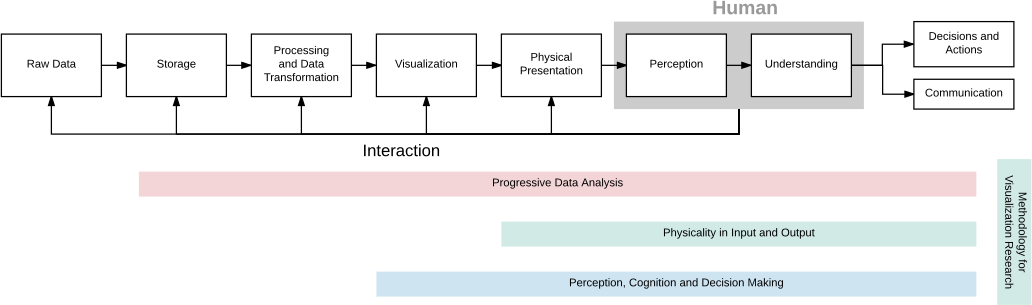
The conceptual Data Analysis Pipeline related with the four themes of AVIZ.
As shown in Figure 1, visualization deals with the data analysis pipeline and research in visualization has been addressing all the stages with less emphasis on the two initial ones and the last one. In its initial incarnation, Aviz has been focusing on interaction in combination with visualization, physical presentation, and perception. We now want to expand our research to wider questions, both in the human-side and in the system side. For the human side, we want to better understand human perception and cognition to improve the visualization techniques, so as to better convey information to the human brain. We also want to better understand the human biases to overcome them when possible, or provide methods to avoid them otherwise.
On the system side, we want to expand the scope of visualization that is currently limited to relatively small datasets and relatively simple analytical methods. To achieve scalability in visualization, we will focus on a paradigm shift: progressive data analysis. Long-running computations currently hamper the exploration and visualization process because human's attention is limited by latency constraints. We want to design exploratory systems that provide continuous feedback and allow interactions at any time during computation. The new progressive data analysis paradigm offers these capabilities, but to be usable, it requires the whole analytical pipeline to be re-implemented, and visualization and interaction techniques to be adapted.
2.2 Research Themes
Aviz's research on Visualization and Visual Analytics is organized around four research themes, described in more detail in the next section. Instead of addressing point problems, each research theme will address several stages of the visualization pipeline in a holistic manner, as summarized in fig:diagram.
1. Progressive Data Analysis and Scalability will address visualization scalability problems. Existing data analysis systems (such as Tableau 68, R 69, or Python with its data analysis ecosystem 56) are not scalable for exploratory analysis because their latency is not controllable. This theme will lay out the foundations of progressive data analysis systems, which generate estimates of the results and updates the analyst continuously at a bounded pace. It will focus on all the stages of the data analysis pipeline: data management mechanisms, data analysis modules, as well as visualizations, perception, understanding, and decision making.
2. Physicality in Input and Output will seek to better understand the benefits of physicality for information. Although beyond-desktop environments for visualization are generating more and more interest, theories and empirical data are lacking. This theme will consolidate the nascent areas of data physicalization, situated visualization, and immersive visualization.
3. Perception, Cognition, and Decision Making will study how we perceive and understand visualizations in order to develop generalized guidelines for optimizing effectiveness. It will generalize results obtained with simple charts to more complex visualizations of large datasets, establish theories on the use of abstraction in visualization, and contribute new empirical knowledge on decision making with visualizations.
4. Methodologies for Visualization Research will develop new methods to ground the study of the above scientific questions, and to benefit visualization more generally. This theme will develop evidence-based strategies for communicating quantitative empirical findings, and will promote methodological discussions and open research practices within the field.
3 Research program
3.1 Progressive Data Analysis and Scalability
Permanent involved: Jean-Daniel Fekete
While data analysis has made tremendous progress in scalability in the last decade, this progress has only benefited “confirmatory” analysis or model-based computation; progress in data exploration has lagged behind. Existing data analysis systems do not support data exploration at scale because, for large amounts of data or for expensive computations, their latency is not controllable: computations can take minutes, hours, even days and months. Cognitive psychologists have shown that humans' cognitive capabilities degrade when latency increases 62, 66. Miller 62 points out that the feedback of a system should remain below 10 seconds to maintain the user's attention. Therefore, to try to limit the latency, analysts currently resort to complex, inefficient, and unsatisfactory strategies, such as sampling with its issues related to representativity.
To address the scalability problem under controlled latency, instead of performing each computation in one long step that forces the analyst to wait for an unbounded amount of time, a progressive system generates estimates of the results and updates the analyst continuously at a bounded pace. The process continues until the computation is complete, or it stops early if the analyst considers that the quality of the estimates is sufficient to make a decision. During the process, a progressive system allows users to monitor the computation with visualizations and steer it with interactions.
While the topic of progressive data analysis has started to emerge in the late 90's, it has remained marginal practically because it touches three fields of computer science that are traditionally separate: data management, data analysis, and visualization. Research on progressive data analysis remains fragmented; the solutions proposed are partial and the different solutions cannot always be combined. We have organized a Dagstuhl seminar on Progressive Data Analysis and Visualization 46, 70 that acknowledged the harm of this topical separation and devised a research agenda. Aviz will participate in this agenda with specific assets.
Aviz is actively working on designing and implementing the ProgressiVis language that is natively progressive 47. The language relies on a Python interpreter but its execution semantics is different in the sense that all the operations that would take time to execute are performed progressively. The ProgressiVis system touches all the stages of the conceptual data analysis pipeline of fig:diagram; it integrates data management mechanisms, data analysis modules, as well as visualizations, perception, understanding, and decision making. Aviz will strengthen its work on the implementation of a natively progressive data science system. Such a system will lead to the following research topics:
- Progressive language kernel and data management mechanisms
- Progressive algorithms and computation strategies
- Progressive visualizations
- Management of uncertainties, computed from the algorithms and conveyed to the analysts.
Language:
ProgressiVis relies on a Python interpreter that is convenient for quick prototyping. We are using many internal mechanisms of the Python language to create a toolkit that allows programming progressive applications in a convenient way.
Algorithms:
Existing libraries implementing algorithms are either “eager”, sometimes “online”, and more rarely “streaming”, but very few are compatible with our progressive paradigm. We already designed several progressive algorithms and want to further expand the range of algorithms we can support, and better understand how algorithms could be transformed into their progressive counterpart.
Progressive computation can trade speed, memory, and quality. When analysts want to compute rough estimates of their results quickly, several progressive algorithms offer parameters to control the tradeoffs. For example, our progressive k-nearest-neighbors algorithm 54 allows choosing the number of k-d-trees that influences the memory footprint, accuracy, and speed. Progressive data analysis also uses and adapts streaming algorithms, also called “data sketches” 48, that offer various tradeoffs between speed, memory, and quality.
Visualizations:
Like algorithms need to be adapted or transformed, visualization techniques and user interfaces also need to be adapted to be used in a progressive setting.
For the visualization pipeline, including the rendering phase, there has been previous work related to progressive layout computation and progressive rendering. For example, in network visualization, there is an old tradition of using iterative force-directed solvers and showing their results progressively when computing complex graph layouts. We generalize this approach to all the visualizations. Progressive rendering is popular in the real-time computer graphics and gaming domains, but new in visualization. We are designing visualization techniques based on GPU and accelerated algorithms to better render aggregated visualizations, such as heatmaps and sampled visualizations.
We have also started to explore the problem of requirements for progressive user interfaces 38, leading the community of researchers to understand that progressive systems need to provide effective assessments of the quality of their results to allow analysts to make early decisions. This justifies the next research topic on the management of uncertainties. We have also started to propose new data models for managing aggregated visualizations 55. We now continue our research to adapt existing visualization techniques and interactive environments to deal with progressive results and parameter steering.
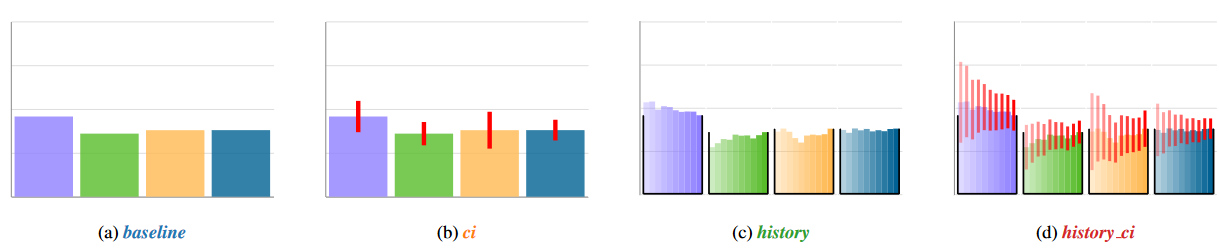
(1) simple bar char. (2) with confidence intervals. (3) with recent history, and (4) with recent history and confidence intervals.
Management of uncertainties:
When performing exploratory data analysis on large data, analysts start by trying some algorithms with different parameters until they obtain useful results. Therefore, it is of paramount importance to be able to realize that a specific algorithm or a specific set of parameters is not leading to a useful result and to abort an analysis before it ends to explore other methods. On the other side, in many circumstances when data is “well-behaved”, progressive algorithms achieve very good results quickly, and analysts can make a decision before the end of the algorithms' execution. The assessment of a quality measure related to a computed result is a complex problem, already tackled by other communities (e.g., streaming, approximate queries).
Progressive Data Analysis adds two problems: (1) controlling uncertainty during progressive computations, and (2) conveying the results of this uncertainty in a way understandable by analysts, while avoiding possible cognitive biases such as priming and anchoring 57.
To control the uncertainty during the progression of a computation, methods related to Sequential Analysis 49 should be used. They are well-known in the area of clinical trials and have evolved recently to manage large amounts of data while controlling time or quality in Approximate Query Databases such as BlinkDB 37.
We have already shown that humans are quite good at deciding when to stop a progressive process to make an early decision using progressive bar charts 5 (fig:ExamplesOfProgressiveBarChartsWeStudied); humans are cognitively equipped to make sense of progressive processes. Additionally, visualization can help humans make sense of and interact with progressive processes, such as our new-history bar chart designs that help to render visually when a progressive process starts to converge, opening a new line of research to discover the possible forms of support.
3.2 Physicality in Input and Output
Permanents involved: Petra Isenberg, Tobias Isenberg, Jean-Daniel Fekete
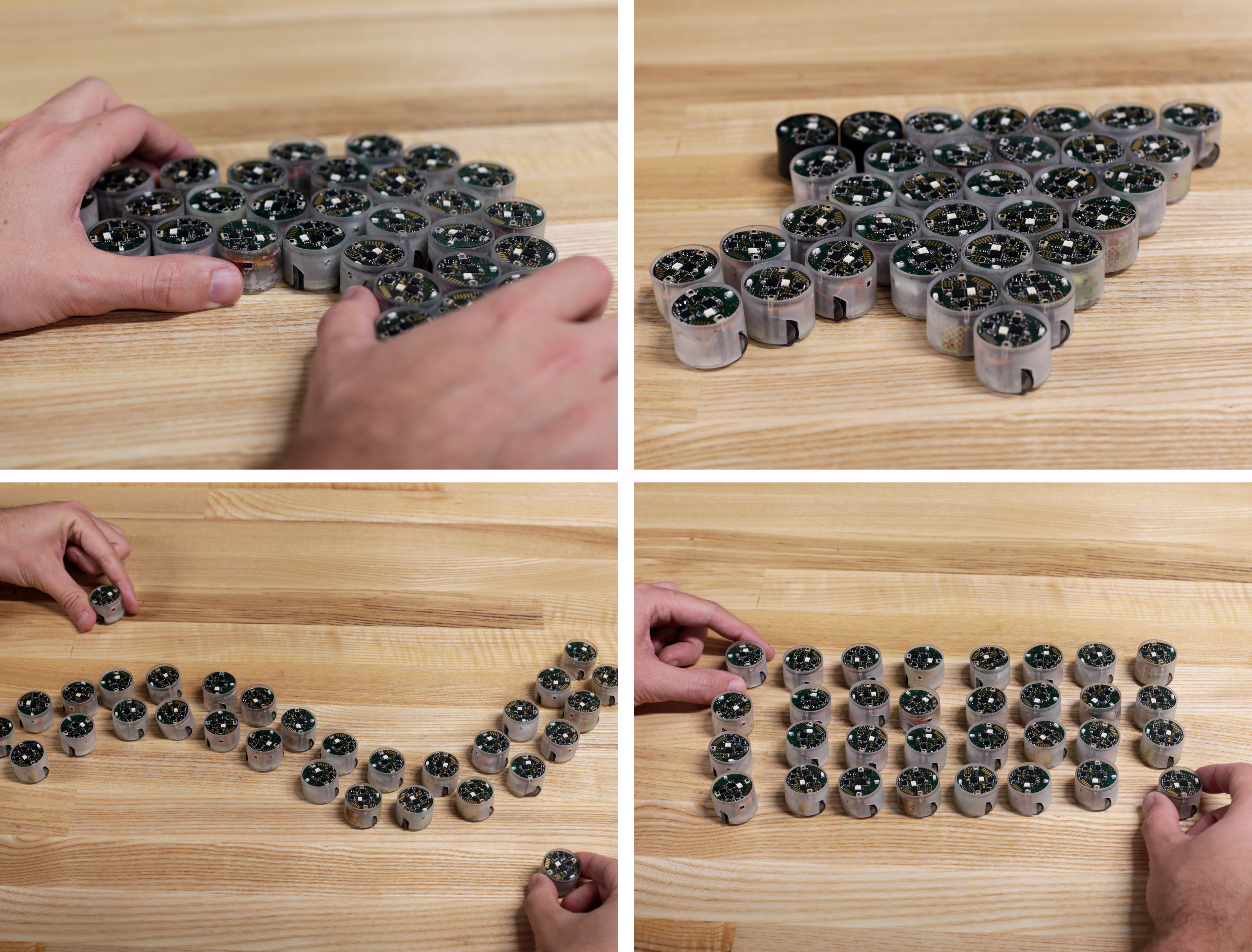
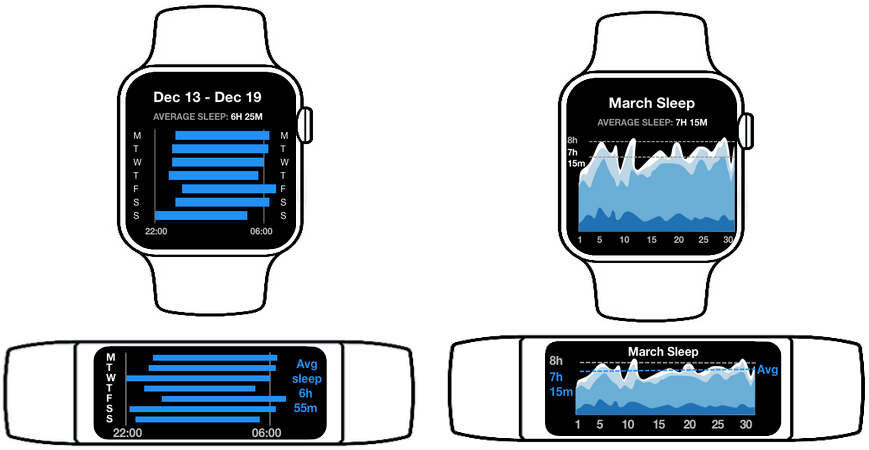
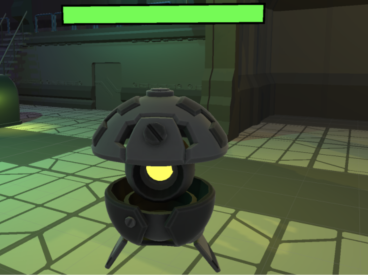
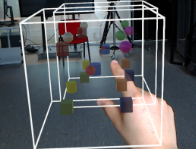
This is a compilation of images showing work the Aviz team did in the area of "physicality in input and output". The first image shows the Zooids project about miniature robots that can produce data physicalizations. The second shows a visualization for a smartwatch and fitness band about sleep data. The third shows situated visualizations in a video game. The third shows a visualization in augmented reality and a selection technique for choosing certain points.
This is a compilation of images showing work the Aviz team did in the area of "physicality in input and output". The first image shows the Zooids project about miniature robots that can produce data physicalizations. The second shows a visualization for a smartwatch and fitness band about sleep data. The third shows situated visualizations in a video game. The third shows a visualization in augmented reality and a selection technique for choosing certain points.
During the last five years, we expanded our work on beyond-desktop environments for visualization. Our team has made contributions in the areas of data physicalization, visualization for wearable devices, situated and embedded visualization, and visualization in augmented reality.
Data Physicalization: Data physicalization is a rich and vast research area that studies the use of physical artifacts to convey data. It overlaps with a number of research areas including information/scientific visualization, visual analytics, tangible user interfaces, shape-changing interfaces, fabrication, as well as graphic design, architecture, and art. Physical data visualizations tap into our lifelong experience of perceiving and manipulating the physical world, either alone or with other people. Among the earliest man-made artifacts are physical representations of semantic concepts that provide physical metaphors that allow us to reason, remember, and communicate. With the advent of computers, we have substituted physical representations with pixels on a computer screen. The resurgence of physicalization as a research area, following our early definitions 53, asks what we have lost in this transformation. Certainly, a computer-based visualization allows us to zoom an image, transform variables in real time, and to zoom through virtual computer-based world. However, these representations can sever the relationship to the natural world, depriving us of the touch, feel, and emotion that comes from interacting with real objects. We studied several aspects of data physicalization including technical challenges of constructing physicalizations, potential benefits, and how historical examples could transfer to a modern world.
Visualization for Wearable and Mobile Devices: In the area of wearable and mobile-devices we engaged in device-driven research where we considered how small device form factors may influence how we need to design visualizations and how we can use them. Portable and wearable personal devices such as fitness tracking armbands, hand-held GPS trackers, smart watches, or mobile phones are very small displays that are capable of producing data themselves (through sensors), downloading it from other sources (through Wifi or Bluetooth), and displaying it immediately 4. Often the data is shown in the form of visualizations that have to be adapted to the small display size. We consider very small visualizations that are often used on such devices under the term “micro visualization” and have been working towards a better understanding of the complexities involved in designing and using micro visualizations but also studied the influence of the unique context of use of mobile devices on visualization use and design.
Situated and Embedded Data Representations: We study how embedding data visualizations in the context of the data sources can empower people to make effective use of their data in a variety of application contexts. Our goal in this work is to go beyond the traditional platforms of data analytics by using situated data visualizations on various types of non-traditional displays. In a situated data visualization, the data is directly visualized near the physical space, object, or person it originates from 76. For example, a person may attach small e-ink displays embedded with sensors at various locations of their house or their workplace, to better understand their use of space, of equipment, or of energy resources. Or a person who wishes to exercise more may use an augmented reality device to visualize their past running performance in-place. New situated data visualizations like these can surface information in the environment—allowing viewers to interpret data in-context and take action in response to it 8.
Visualization using Augmented Reality Devices: Many datasets are 3D-spatial in nature and researchers and practitioners could benefit from seeing them in true 3D space. This is where immersive technologies shine, and the recent advances in VR and AR headset technologies have made such displays accessible to the general public—the lack of large dedicated VR installations such as a CAVE is not preventing the use of immersive rendering anymore. Nonetheless, the investigation of 3D datasets also frequently requires researchers to use tools such as scripted analysis and statistical evaluation, and such direction of investigation will continue to be a cornerstone of scientific work. In our investigations we are thus interested in looking at, in particular, hybrid setups that allow researchers to use the best of both worlds: traditional workstations combined with an AR overlay for stereoscopic rendering of 3D data 74.
3.3 Research Axis 3: Perception, Cognition and Decision-Making
Permanents involved: Petra Isenberg, Tobias Isenberg, Jean-Daniel Fekete
As we collect increasingly large amounts of data in fields such as climate science, finance, and medicine, the need to understand and communicate that data becomes more important. Data visualizations are often used to give an overview of information, however it can be challenging to predict whether these visualizations will be effective before spending resources to develop them. Consequently, researchers make use of experimental methods from visual perception and cognition to study how we perceive and understand visualizations in order to develop generalized guidelines for optimizing effectiveness. On this research axis we have three focus areas:
Perception of Visualizations in Novel Contexts. Novel technology and usage contexts have several characteristics for data visualizations that warrant a (re-)evaluation of how well people can perceive visualizations. Characteristics we were particularly interested in include:
- Physical factors: Many now common displays have characteristics that warrant (re-)evaluation of what we know about visualizations to be displayed on them. Small form factors of displays such as smartwatches and fitness bands are an obvious characteristic. Some screens deviate from the standard rectangular form we are familiar with and use a circular geometry, which is another interesting design constraint for visualization.
- Data display mobility: Data display mobility captures the movement of the display(s) containing visual representations of data. Fixed, movable, carryable, wearable, and independently moving displays can be differentiated along this dimension. We conducted some of our research on carryable devices, including mobile devices such as smartphones and tablets but also wearable displays such as smartwatches and head-mounted displays.
- Context-of-use: Many novel displays are used in contexts that are much unlike traditional office settings. Visualizations here may be subjected to different lighting conditions and viewers may only afford very quick glances at the displays themselves. For example, when in a car the driver can only afford very quick glances at the GPS before returning to the primary task of arriving safely.
Within this focus area we worked on some of these aspects; such as the need for quick glances, the display size of visualizations, the movement of viewers or the data, and the understanding of 3D augmented reality spaces. We relied mostly on mixed-methods user studies where a quantitative analysis methodology was coupled with interviews or questionnaires.
Illustrative Visualization. This focus area takes inspiration from illustrators' decades to centuries of experience on perception and cognition to better portray scientific subject matter. Another input arises from the field of non-photorealistic rendering which has developed numerous techniques of stylizing images and other input data. Traditionally, illustrative visualization has thus been applied primarily to data with a concrete spatial mapping in 2D and, more frequently, in 3D space.
Another main direction of research in this context is what the role of abstraction is in illustrative visualization 73, 72 as well as visualization in general, and specifically how we can provide dedicated means to control the abstraction being applied to visual representations of data. This means that we need to go beyond seeing abstraction only as a side-product of stylization as it has traditionally been viewed in many approaches in non-photorealistic rendering as well as illustrative visualization to date, and investigate how we can interactively adjust it to provide practitioners with a means to find the best visual representations for a given task. For example, we have investigated this question in the context of structural biology 79 and DNA nanostructures 59, 60, 61, but also want to expand this work to other application domains in the future.
Decision-Making with Visualizations. Human decision-making and cognitive biases are important research topics in the fields of psychology, economics and marketing. Visualization systems are increasingly used to support decision-making: large companies switch to visualization solutions to improve their decisions in a range of areas, where large sums of money or human lives are at stake. More and more, the ultimate goal of visualization is not to understand patterns in the data and get insights as was traditionally assumed, but to make good decisions. In order to fully understand how information visualizations can support decision-making, it is important to go beyond traditional evaluations based on data understanding, and study how visualizations interact with human judgment, human heuristics, and cognitive biases.
We pursue this important stream of research by investigating decision-making in the presence of uncertainty and incomplete information (in connection with the topics discussed in sec:progressive and the use of visualizations to support social choice and group decisions in the presence of conflicts of interest. How cognitive biases interact with visual perception is also an important and difficult question that has remained largely unexplored.
3.4 Research Axis 4: Methodology for Visualization Research
Permanents involved: Petra Isenberg, Tobias Isenberg, Jean-Daniel Fekete
An important aspect of any scientific research is to establish and follow rigorous and effective methodologies for acquiring new knowledge. In the field of Visualization in particular, scientific discourse on the validity, use, and establishment of methodologies is important as the field is highly interdisciplinary, with diverse influences and opinions. It is important to establish, for example, what level of rigor the field should require of its methods, how to choose among established methods and methodologies, and how to best communicate the results of our empirical research. We focus our efforts on three main topics related to visualization research methodologies.
Promoting and Following Open Research Practices
For issues with open research practices to be addressed, educational materials and guidelines need to be written, so researchers have clarity about how to make their research more credible. Aviz members are working with the organizing bodies of the visualization research community to establish incentives for making research artifacts and potentially establish minimal requirements for openness in published articles. Meanwhile, it is important to continue measuring and cataloging openness in the field to monitor progress. The goal is to improve the credibility and applicability of the field’s research.
Shaping the Scientific Visualization Community Aviz researchers are heavily involved in the organization structure of IEEE visualization conferences, the most prestigious conference in our field, by proposing workshops, tutorials, serving on various organizing committees, steering committees, editorial boards. We, in particular, aid the process by providing data collection and analysis services through the vispubdata.org dataset that we are collecting, cleaning, and making available to the community. The dataset has already been used in research (e. g., 58) but also to shape the scientific community by proposing program committee members, new processes, and was used by the Visualization Restructuring Committee (ReVISe). We are also involved in the EuroVis community and participate at multiple levels to its organization and management.
4 Application domains
4.1 Natural Sciences
As part of a CORDI PhD project, we collaborated with researchers at CERN on interactive data visualization using augmented reality, with the goal to better understand this new visualization environment and to support the physicists in analysing their 3D particle collision data. As part of another CORDI PhD project, we collaborated with researchers at the German Center for Climate Computation (DKRZ) and the Helmholtz-Zentrum Hereon (formerly Helmholtz-Zentrum Geesthacht, HZG), to better understand collaborative data exploration and interaction in immersive analytics contexts. Finally, as part of the Inria IPL “Naviscope,” we collaborate with researchers at INRAE (as well as other Inria teams) on interactive visualization tools for the exploration of plant embryo development. We also work with experts from the natural sciences in general to create illustrative visualizations of scientific data, such as a continuous zooming technique from the nucleus of a cell all the way down to the atom configuration of the DNA, for example for the application in education.
4.2 Social Sciences
We collaborate with social science researchers from EHESS Paris on the visualization of dynamic networks; they use our systems (GeneaQuilts 39, Vistorian 65, PAOHVis 71, PK-Clustering 63) and teach them to students and researchers. Our tools are used daily by ethnographers and historians to study the evolution of social relations over time. In the social sciences, many datasets are gathered by individual researchers to answer a specific question, and automated analytical methods cannot be applied to these small datasets. Furthermore, the studies are often focused on specific persons or organizations and not always on the modeling or prediction of the behavior of large populations. The tools we design to visualize complex multivariate dynamic networks are unique and suited to typical research questions shared by a large number of researchers. This line of research is supported by the DataIA “HistorIA” project, and by the “IVAN” European project. We currently collaborate with PayAnalytics, an Icelandic company to visualize data to help companies close their gender pay gaps.
4.3 Medicine
We collaborate with the Health-Data-Hub on the analysis and visualization of CNAM Data “parcours de santé” to help referent doctors and epidemiologists make sense of French health data. In particular, we are working on a subset of the CNAM Data focused on urinary problems, and we have received very positive feedback from doctors who can see what happens to the patients treated in France vs. what they thought happened through the literature. This project is starting but is already getting a lot of traction from our partners in medicine, epidemiology, and economy of health.
We are also collaborating with the “Assistance Publique - Hôpitaux de Paris” AP-HP with a newly funded project called URGE, aimed at improving the emergency services for the parisian hospitals. See the press announcement.
5 Social and environmental responsibility
5.1 Impact of research results
Aviz' work on illustrative visualization has the potential to be integrated into future teaching materials for students in schools, visitors in museums, or similar.
Aviz' work on visualization of large documents corpora with Cartolabe is used to present the results of the French “Grand débat”, as well as other citizen expressions.
Aviz' work on the gender pay gap aims at improving decision making for closing the adjusted pay gap.
Open science: Aviz regularly shares full research material on the repository of the Center for Open Science to facilitate scrutiny, reuse, and replication:
- Visualization in Motion: A Research Agenda and Two Evaluations 78 — osf.io/km3s2/, osf.io/9c4bz/, osf.io/t748d/, osf.io/t748d/,gitlab.inria.fr/lyao/visinmotion, GRSI Stamp
- Reflections on Visualization in Motion for Fitness Trackers 52 — hal.inria.fr/hal-03775633
- Situated Visualization in Motion for Swimming 77 — hal.inria.fr/hal-03700406
- Situated Visualization in Motion for Video Game 40 — hal.inria.fr/hal-03694019
- Situated Visualization in Motion in Video Game for Different Types of Data 41 — hal.inria.fr/hal-03700418
- Preferences and Effectiveness of Sleep Data Visualizations for Smartwatches and Fitness Bands 50 – osf.io/yz8ar/
- Context Specific Visualizations on Smartwatches 51 – osf.io/vhn43/
- Studying Early Decision Making with Progressive Bar Charts 5 — osf.io/tn2ph/
- Scalability in Visualization 64 — osf.io/xrvu7/
- Six methods for transforming layered hypergraphs to apply layered graph layout algorithms 45 — osf.io/grvwu/
- Pyramid-based Scatterplots Sampling for Progressive and Streaming Data Visualization 44 — ProgressivePyramidBasedSampling and www.yunhaiwang.net/Vis2021/progressive-sampling/
Graphics Replicability Stamp: In 2023, Aviz members received recogniztion for the replicability of their work from the Graphics Replicability Stamp Initiative for several of their journal publications:
- Designing for Visualization in Motion: Embedding Visualizations in Swimming Videos 24
- Eliciting Multimodal and Collaborative Interactions for Data Exploration on Large Vertical Displays 19
- Design Characterization for Black-and-White Textures in Visualization 13
- Visualizing and Comparing Machine Learning Predictions to Improve Human-AI Teaming on the Example of Cell Lineage 15
- V-Mail: 3D-Enabled Correspondence about Spatial Data on (Almost) All Your Devices 20
- BeauVis: A Validated Scale for Measuring the Aesthetic Pleasure of Visual Representations 12
6 Highlights of the year
6.1 Events
- Florent Cabric , post-doctoral fellow at Aviz co-organized the French “Visualization Day” (“Journées Visu”) at Univ. Paris-Saclay in June.
- Petra Isenberg , Florent Cabric , and Mickaël Sereno co-organized (“PreVis”) at Univ. Paris-Saclay in June.
6.2 Awards
- Tobias Isenberg: 14 Years SciVis Test-of-Time Award at IEEE VIS 2023
- Petra Isenberg: Induction into the IEEE Visualization Academy
7 New software, platforms, open data
7.1 New software
7.1.1 Cartolabe
-
Name:
Cartolabe
-
Keyword:
Information visualization
-
Functional Description:
The goal of Cartolabe is to build a visual map representing the scientific activity of an institution/university/domain from published articles and reports. Using the HAL Database, Cartolabe provides the user with a map of the thematics, authors, and articles. ML techniques are used for dimensionality reduction, cluster, and topic identification, visualization techniques are used for a scalable 2D representation of the results.
Cartolabe has, in particular, been applied to the Grand Debat dataset (3M individual propositions from French Citizen, see https://cartolabe.fr/map/debat). The results were used to test both the scaling capabilities of Cartolabe and its flexibility to non-scientific and non-English corpora. We also added sub-map capabilities to display the result of a year/lab/word filtering as an online generated heatmap with only the filtered points to facilitate the exploration. Cartolabe has also been applied in 2020 to the COVID-19 Kaggle publication dataset (Cartolabe-COVID project) to explore these publications.
- URL:
- Publication:
-
Contact:
Philippe Caillou
-
Participants:
Philippe Caillou, Jean Daniel Fekete, Michèle Sebag, Anne-catherine Letournel, Hande Gozukan
-
Partners:
LRI - Laboratoire de Recherche en Informatique, CNRS
7.1.2 PAOHvis
-
Name:
Parallel Aggregated Ordered Hypergraph Visualization
-
Keywords:
Dynamic networks, Hypergraphs
-
Functional Description:
Parallel Aggregated Ordered Hypergraph (PAOH) is a novel technique to visualize dynamic hypergraphs 71. Hypergraphs are a generalization of graphs where edges can connect more than two vertices. Hypergraphs can be used to model co-authorship networks with multiple authors per article, or networks of business partners. A dynamic hypergraph evolves over discrete time slots. A PAOH display represents vertices as parallel horizontal bars and hyperedges as vertical lines that connect two or more vertices. We believe that PAOH is the first technique with a highly readable representation of dynamic hypergraphs without overlaps. It is easy to learn and is well suited for medium size dynamic hypergraph networks such as those commonly generated by digital humanities projects - our driving application domain.
- URL:
-
Contact:
Jean Daniel Fekete
7.1.3 ParcoursVis
-
Name:
Visualization of Patient Pathways
-
Keywords:
Visualization, Health, Progressive visualization
-
Scientific Description:
We developed ParcoursVis, our Progressive Visual Analytics (PVA) tool to explore patients' care pathways at scale. Current tools to visualize temporal event sequences are restricted to datasets as large as a few thousand sequences to remain reactive. With ParcoursVis, we aim to visualize patients' care pathways stored in country-level databases, which can contain order of magnitudes higher of event sequences, at scale using a progressive architecture. PVA tools, instead of waiting for the whole computation to finish before rendering the final results, yield partial results each time the algorithm processes small chunks of data or iterations. This paradigm makes the tool reactive and quickens processes such as checking errors of a query.
-
Functional Description:
ParcoursVis allow extracting a subset of the nationwide database from CNAMTS, transforming the raw data into meaningful medical events, and visualizing it interactively at scale via a web interface.
For the moment, ParcoursVis focuses on non-cancerous prostate adenoma. With this focus, our domain expert users extract meaningful high-level types of events (e.g., treatments and outcomes) that the patients undertake in their care pathways.
Using a progressive visualization method, ParcoursVis visualizes in an aggregated manner the care pathways of tens of millions of patients treated with thousands of events over decades, several orders of magnitude more than existing interactive systems.
- URL:
-
Authors:
Jean Daniel Fekete, Mickael Sereno
-
Contact:
Jean Daniel Fekete
7.1.4 LineageD+
-
Name:
LineageD+
-
Keywords:
Data visualization, Visual analytics, Machine learning, Human-AI teaming, Biology
-
Functional Description:
We visualize the predictions of multiple machine learning models to help biologists as they interactively make decisions about cell lineage—the development of a (plant) embryo from a single ovum cell. Based on a confocal microscopy dataset, traditionally biologists manually constructed the cell lineage, starting from this observation and reasoning backward in time to establish their inheritance. To speed up this tedious process, we make use of machine learning (ML) models trained on a database of manually established cell lineages to assist the biologist in cell assignment. Most biologists, however, are not familiar with ML, nor is it clear to them which model best predicts the embryo’s development. We thus have developed a visualization system that is designed to support biologists in exploring and comparing ML models, checking the model predictions, detecting possible ML model mistakes, and deciding on the most likely embryo development. To evaluate our proposed system, we deployed our interface with six biologists in an observational study. Our results show that the visual representations of machine learning are easily understandable, and our tool, LineageD+, could potentially increase biologists’ working efficiency and enhance the understanding of embryos.
- URL:
-
Contact:
Tobias Isenberg
-
Partner:
INRAE
7.2 New platforms
Prepare the ProgressiVis Toolkit for a Wider Distribution
Participants: Jean-Daniel Fekete [Inria, Paris-Saclay, correspondent], Christian Poli [Inria], Nivan Ferreira [Univ. Federal de Pernambuco, Brazil].
The ProgressiVis toolkit, implementing a Progressive Data Analysis and Visualization language and environment, has been under heavy development for many years. We have finished its packaging so it can be easily installed by partners who want to experiment with the toolkit and the concept it supports. A documentation is also available (in progress), as well as several graphical and interactive widgets to support its use in modern notebooks.
7.3 Open data
7.3.1 Vispubdata.org
Participants: Petra Isenberg [Inria Paris-Saclay, correspondent], Tobias Isenberg [Inria Paris-Saclay].
AVIZ members are making available for research a dataset of IEEE VIS publications at vispubdata.org. This dataset is actively being used for research and conference organization.
7.3.2 Gender in Visualization Dataset
Participants: Natkamon Tovanich [System X], Pierre Dragicevic [Inria Bordeau], Petra Isenberg [Inria Paris-Saclay, correspondent].
In the context of our paper on “Gender in 30 Years of IEEE Visualization” we make available data about the diversity of the Visualization community in terms of the gender of authors, organizing, and program committee members as well as award winners: www.aviz.fr/Research/Gender-in-Vis.
7.3.3 Visualization Images
AVIZ members are contributing to making available for research a dataset of IEEE VIS images at ieee-dataport.org/open-access/ieee-vis-figures-and-tables-image-dataset43. The set of images can be browsed online at vispubfigures.github.io/VisPubFigures/. The work is primarily led by Jian Chen at Ohio State University.
7.3.4 Multidimensional Projections of Large Corpora on Zenodo
For the Cartolabe project, we compute multidimensional projections of large publication corpora (HAL, arXiv, Wikipedia, etc.) as well as thematic clusters with meaningful labels. All there computed projections are distributed as open data files on the Zenodo repository to be reused by other projects: https://zenodo.org/communities/cartolabe?q=&l=list&p=1&s=10&sort=newest
7.3.5 Atlas of Minoiry Languages
Frederic Vernier is part of the Speaking atlas of minority languages, a repository of audios and transcriptions of the same text recorded from 920 persons over the world. https://atlas.limsi.fr/liste.html
8 New results
8.1 Visualization of Socio-Demographic Data
Participants: Florent Cabric [LISN, Université Paris-Saclay, CNRS, Inria, Saclay, France, correspondent], Margrét Vilborg Bjarnadóttir[Univ. of Maryland], Anne-Flore Cabouat [LISN, Université Paris-Saclay, CNRS, Inria, Saclay, France], Petra Isenberg [LISN, Université Paris-Saclay, CNRS, Inria, Saclay, France].
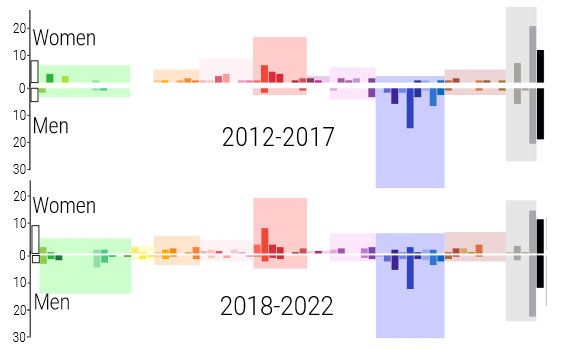
Visualization of the typical color used to represent gender in scientific publications for two different time periods.
We started investigations into the visualization of sociodemographic data. Sociodemographic data is a common part of datasets related to people, including institutional censuses, health data systems, and human-resources files. This data is sensitive, and its collection, sharing, and analysis require careful consideration. For instance, the European Union, through the General Data Protection Regulation (GDPR), protects the collection and processing of any personal data, including sexual orientation, ethnicity, and religion. Data visualization of sociodemographic data can reinforce stereotypes, marginalize groups, and lead to biased decision-making. It is, therefore, critical that these visualizations are created based on good, equitable design principles. In a first opinion paper 2, we discuss and provide a set of open research questions around the visualization of sociodemographic data. Our work contributes to an ongoing refection on representing data about people and highlights some important future research directions for the Visualization community. A version of this paper and its fgures are available online at osf.io/a2u9c.
8.2 Design Characterization for Black-and-White Textures in Visualization
Participants: Tingying He [Inria, Paris-Saclay, correspondent], Yuanyang Zhong [Inria, Paris-Saclay], Petra Isenberg [Inria, Paris-Saclay], Tobias Isenberg [Inria, Paris-Saclay].
We investigate the use of 2D black-and-white textures for the visualization of categorical data and contribute a summary of texture attributes, and the results of three experiments that elicited design strategies as well as aesthetic and effectiveness measures 3. Black-and-white textures are useful, for instance, as a visual channel for categorical data on low-color displays, in 2D/3D print, to achieve the aesthetic of historic visualizations, or to retain the color hue channel for other visual mappings. We specifically study how to use what we call geometric and iconic textures. Geometric textures use patterns of repeated abstract geometric shapes, while iconic textures use repeated icons that may stand for data categories. We parameterized both types of textures and developed a tool for designers to create textures on simple charts by adjusting texture parameters. 30 visualization experts used our tool and designed 66 textured bar charts, pie charts, and maps. We then had 150 participants rate these designs for aesthetics. Finally, with the top-rated geometric and iconic textures, our perceptual assessment experiment with 150 participants revealed that textured charts perform about equally well as non-textured charts, and that there are some differences depending on the type of chart.
8.3 Visualizing and Comparing Machine Learning Predictions to Improve Human-AI Teaming on the Example of Cell Lineage
Participants: Jiayi Hong [Inria, Paris-Saclay, correspondent], Ross Maciejewski [Arizona State University], Alain Trubuil [InraE Paris-Saclay], Tobias Isenberg [Inria, Paris-Saclay].
We visualize the predictions of multiple machine learning models to help biologists as they interactively make decisions about cell lineage—the development of a (plant) embryo from a single ovum cell. Based on a confocal microscopy dataset, traditionally biologists manually constructed the cell lineage, starting from this observation and reasoning backward in time to establish their inheritance. To speed up this tedious process, we make use of machine learning (ML) models trained on a database of manually established cell lineages to assist the biologist in cell assignment. Most biologists, however, are not familiar with ML, nor is it clear to them which model best predicts the embryo’s development. We thus have developed a visualization system that is designed to support biologists in exploring and comparing ML models, checking the model predictions, detecting possible ML model mistakes, and deciding on the most likely embryo development. To evaluate our proposed system, we deployed our interface with six biologists in an observational study. Our results show that the visual representations of machine learning are easily understandable, and our tool, LineageD+, could potentially increase biologists’ working efficiency and enhance the understanding of embryos.
9 Bilateral contracts and grants with industry
9.1 Bilateral contracts with industry
Participants: Jean-Daniel Fekete, Katerina Batziakoudi.
CIFRE PhD fellowship of Katerina Batziakoudi with the Company Berger-Levrault (2023–2026).
Participants: Petra Isenberg, Florent Cabric.
Contract with PayAnalytics for joint work on the Inria exploratory action called “Equity Analytics” (2022-2024)
10 Partnerships and cooperations
10.1 International research visitors
10.1.1 Visits of international scientists
Other international visits to the team
Nivan Ferreira
Participants: Jean-Daniel Fekete, Christian Poli, Nivan Ferreira.
-
Status
Assistant Professor
-
Institution of origin:
Universidade Federal de Pernambuco
-
Country:
Brazil
-
Dates:
5/02/2024–31/01/2025
-
Context of the visit:
Collaboration on Progressive Data Analysis and Visualization
-
Mobility program/type of mobility:
DataIA invited professor program
Hendrik Strobelt
Participants: Jean-Daniel Fekete, Petra Isenberg, Hendrik Strobelt.
-
Status
Researcher
-
Institution of origin:
IBM Research (Cambridge)
-
Country:
USA
-
Dates:
19/01/2023–21/01/2023
-
Context of the visit:
Collaboration on Visual Analytics
-
Mobility program/type of mobility:
self funded
10.1.2 Visits to international teams
Research stays abroad
Jean-Daniel Fekete
-
Visited institution:
Monash University
-
Country:
Australia
-
Dates:
16/10/2023–29/10/2023
-
Context of the visit:
Scientific talk and possible collaborations
-
Mobility program/type of mobility:
research stay
10.2 National initiatives
-
Program:
ANR PRC (ANR-19-CE33-0012)
-
Project acronym:
EMBER
-
Project title:
Situated Visualizations for Personal Analytics
-
Duration:
2020 – 2023. Total funding: 712 k€
-
Coordinator:
Pierre Dragicevic
-
Other partners:
Inria Bordeaux, Sorbonne Université
-
Participants:
Participants: Petra Isenberg, Lijie Yao.
-
Abstract:
The Ember project studies how situated data visualization systems can help people use their personal data (e.g., fitness and physiological data, energy consumption, banking transactions, online social activity,…) for their own benefit. Although personal data is generated in many areas of daily life, it remains underused by individuals. Rarely is personal data subjected to an in-depth analysis and used to inform daily decisions. This research aims to empower individuals to improve their lives by helping them become advanced consumers of their own data. This research builds on the area of personal visual analytics, which focuses on giving the general public effective and accessible tools to get insights from their own data. Personal visual analytics is a nascent area of research, but has so far focused on scenarios where the data visualization is far removed from the source of the data it refers to. The goal of this project is to address the limitations of traditional platforms of personal data analytics by exploring the potential of situated data visualizations. In a situated data visualization, the data is directly visualized near the physical space, object, or person it refers to. Situated data visualizations have many potential benefits: they can surface information in the physical environment and allow viewers to interpret data in-context; they can be tailored to highlight spatial connections between data and the physical environment, making it easier to make decisions and act on the physical world in response to the insights gained; and they can embed data into physical environments so that it remains visible over time, making it easier to monitor changes, observe patterns over time and collaborate with other people. Website: ember.inria.fr/.
Other projects:
- Health Data Hub “ParcoursVis” post-doctoral funding. Duration: 24 months. Total funding: 220k€. Partners: Inria Saclay, Health Data Hub. See www.aviz.fr/Research/ParcoursVis.
-
Participants: Jean-Daniel Fekete, Mickaël Sereno.
Participants:
- AP-HP / Inria joint project URGE. Duration 48 months. Total funding: 520k€. Partners: Inria, Hôpital Saint Antoine, Sorbonne Université. Coordinators: Xavier Leroy (Inria), Youri Yordanov (AP-HP, Inserm, Sorbonne Université).
-
Participants: Jean-Daniel Fekete, Mickaël Sereno.
Participants:
- Action Exploratoire: EquityAnalytics: Visual Analytics for Pay Equity, Action Exploratoire. Post-Doctoral Funding. Duration 24 months. Total funding: 106k€. Coordinator: Petra Isenberg . Partners: PayAnalytics.
-
Participants: Petra Isenberg, Florent Cabric.
Participants:
11 Dissemination
11.1 Promoting scientific activities
- Jean-Daniel Fekete has introduced Graphs and their visualization to high-school students during the Science Ouverte Seminar, Bobigny, Jun. 26, 2023
11.1.1 Scientific events: organisation
General chair, scientific chair
- Florent Cabric co-organized, with Vanessa Peña-Araya from Inria Ilda, the “Journée Visu” of the French Visualization Community on June 22.
- Tobias Isenberg : Co-organizer of Shonan Seminar 173—TAT: Toughening the Foundation of Abstraction in Visualization Techniques
- Petra Isenberg ,Florent Cabric , and MickaëlSereno organized “PreVis” on October 16th and 17th 2023.
Member of the organizing committees
- Tobias Isenberg : IEEE VIS 2023
- Petra Isenberg : EuroVis, Young Researcher Award Committee
- Petra Isenberg : IEEE VIS Steering Committee Co-Chair
- Petra Isenberg : IEEE VIS Test of Time Committee Member (for IEEE InfoVis papers)
11.1.2 Scientific events: selection
Chair of conference program committees
- Tobias Isenberg : area chair for IEEE VIS 2023
Member of the conference program committees
- Jean-Daniel Fekete was PC member of the new “Undone Computer Science 2024” conference
- Jean-Daniel Fekete was PC member of the VIS 2023 conference
- Jean-Daniel Fekete was PC member of the EDBT/ICDT International Workshop on Big Data Visual Exploration and Analytics 2023
- Tobias Isenberg : EuroVis 2023 full papers, EuroVis 2023 STARs, Eurographics 2023 STARs
Reviewer
- Tobias Isenberg : alt.VIS, CHI, EuroVis full papers, EuroVis STARs, Eurographics STARs, MolVA, ACM SIGGRAPH, UIST
- Petra Isenberg : alt.VIS, CHI, CRC Press, ACM DIS, EuroVis full papers, EuroVis short papers, IEEE PacificVis, IEEE VIS
- Lijie Yao : ACM CHI , alt.CHI, ETRA, EuroVis, HRI, IEEE VIS
- Florent Cabric : ACM CHI, IEEE VIS short and full papers
11.1.3 Journal
Member of the editorial boards
- Jean-Daniel Fekete : IEEE Transactions on Visualization and Computer Graphics,
- Tobias Isenberg : Computer Graphics Forum, Elsevier Computers & Graphics,
- Petra Isenberg : Computer Graphics Forum, Associate-Editor-in-Chief CG & A
Reviewer - reviewing activities
- Jean-Daniel Fekete : IEEE Transactions on Visualization and Computer Graphics, Computer Graphics Forum
- Tobias Isenberg : Elsevier Computers & Graphics, ACM Transactions on Graphics, IEEE Transactions on Visualization and Computer Graphics, Journal of Perceptual Imaging
- Petra Isenberg : Computer Science Review, IEEE Transactions on Visualization and Computer Graphics
- Alaul Islam : IEEE Transactions on Visualization and Computer Graphics
11.1.4 Invited talks
-
Jean-Daniel Fekete : Invited talk at the “Embodied Visualization” Lab at University of Monash, Australia
Science Ouverte Seminar to high-school students (15 yo) on Graphs, Bobigny, France
- Tobias Isenberg : Peking University, China; Journées InraE-Inria, France; Keynote FAIRvis workshop, Germany; Linköping University, Sweden; CSIG-VIS International Lecture Series, China
- Petra Isenberg : Seminar Series Talk at the University of Bergen
11.1.5 Leadership within the scientific community
-
Jean-Daniel Fekete : Co-Chair of the IEEE VIS Area Curation Committee
Member of the Scientific Committee of the French journal “Humanités Numériques”
Associate Editor in Chief of IEEE Transactions on Visualization and Computer Graphics (TVCG)
Member of the Publication Board of Eurographics
- Petra Isenberg : Steering Committee BELIV workshop series, Co-chair IEEE VIS Steering Committee
- Tobias Isenberg : Steering Committee BELIV workshop series
11.1.6 Scientific expertise
- Tobias Isenberg : ANR
- Petra Isenberg : NSERC, Mitacs, the Wallenberg Foundation
11.2 Teaching - Supervision - Juries
11.2.1 Teaching
- Master: Jean-Daniel Fekete , Visual Data Analysis at the Parisian Master of Research in Computer Science with Julien Tierny. 25 hours. Joint Master with Univ. Paris-Cité, ENS Ulm, Univ. Paris-Saclay, Institut Polytechnique de Paris.
- Crash course: Jean-Daniel Fekete , on HCI and Visualization, 6h, to IRT SystemX
- Master: Petra Isenberg, Lijie Yao, “Interactive Information Visualization”, 36.5h equivalent TD (total, co-taught with Anastasia Bezerianos), M1/M2, Université Paris-Saclay.
- Master: Petra Isenberg , “Visual Analytics”, 60h equivalent TD (co-taught with Natkamon Tovanich), M2, Centrale Supelec.
- Licence: Tobias Isenberg , “Introduction to Computer Graphics”, 18h en équivalent TD, L3, Polytech Paris-Saclay, France.
- Master: Tobias Isenberg , “Photorealistic Rendering/Advanced Computer Graphics”, 28h en équivalent TD, M2, Polytech Paris-Saclay, France.
- Master: Tobias Isenberg , Jiayi Hong , “Data Visualization”, 36h en équivalent TD, M2, CentraleSupélec, France.
- Master: Frédéric Vernier , class and lab class. “Information visualization”, 24h en équivalent TD, M2 ISC, Faculté des sciences d'Orsay. Univ. Paris-Saclay, France.
- Master: Frédéric Vernier , class and lab class. “Web development with node.js”, 21h en équivalent TD, M2 HCI, Faculté des sciences d'Orsay. Univ. Paris-Saclay, France.
- Licence: Mickaël Sereno , tutorial sessions for “Introduction to Computer Graphics”, 24h en équivalent TD, ET3 (eq. L3), Polytech Paris-Saclay, France.
- Licence: Mickaël Sereno , tutorial sessions for “Informatique Graphique 3D”, 40h en équivalent TD, équivalent L3, Ecole Polytechnique Paris-Saclay, France.
- Licence: Frédéric Vernier , class and lab class. “Introduction to Computer Graphics”, 42h en équivalent TD, L1-MI, Faculté des sciences d'Orsay. Univ. Paris-Saclay, France.
- Licence: Frédéric Vernier , class and lab class. “Advanced Computer Graphics”, 36h en équivalent TD, L2-MI, Faculté des sciences d'Orsay. Univ. Paris-Saclay, France.
- Licence: Frédéric Vernier , class and lab class. “Web development”, 42h en équivalent TD, L3 computer sciences, Faculté des sciences d'Orsay. Univ. Paris-Saclay, France.
11.2.2 Supervision
- PhD in progress: Katerinal Batziakoudi , Visualization of Budget and Accounting Data,Univ. Paris-Saclay, defense planned for december 2026, Jean-Daniel Fekete .
- PhD in progress: Yucheng Lu , Hybrid Scientific Data Exploration, Univ. Paris-Saclay, defense planned for December 2026, Tobias Isenberg .
- PhD in progress: Deng Luo, Abstraction of Visual Representations for DNA Nanotechnology and Protein Data, KAUST, Saudi-Arabia, Tobias Isenberg (co-supervisor).
- PhD in progress: Tobias Rau, Interactive AR/VR Visualization of Molecular Simulation Data, Univ. Stuttgart, Germany, Tobias Isenberg (co-supervisor).
- PhD in progress: Tingying He , A Design Space for Visual Data Mapping for Low-Color Displays, Univ. Paris-Saclay, defense planned for December 2024, Tobias Isenberg .
- PhD in progress: Gabriela Carolina Molina Leon , Multimodal and Collaborative Interaction for Visual Data Exploration, defense planned for March 2024, Petra Isenberg (co-advisor).
- PhD completed: Lijie Yao , Situated Visualization in Motion, Univ. Paris-Saclay, defended on December 18, 2023, Petra Isenberg .
- PhD completed: Alaul Islam , Visualizations for Smartwatches and Fitness Trackers, Univ. Paris-Saclay, defended on March 16, 2023, Petra Isenberg .
- PhD completed: Dr. Jiayi Hong, Machine Learning Supported Interactive Visualization of Hybrid 3D and 2D Data for the Example of Plant Cell Lineage Specification, Univ. Paris-Saclay, defended successfully on Feb. 14, 2023, Tobias Isenberg .
- Masters in progress: Anne-Flore Cabouat , A Validated Scale for Measuring the Readability of Visualizations, Univ. Paris Cité, Tobias Isenberg (supervisor), Petra Isenberg (co-supervisor).
- Masters completed: Yucheng Lu, Historic Sites: Combining Augmented Reality with a Mobile Device for a Hybrid Exploration Experience, Univ. Paris-Saclay, Tobias Isenberg
12 Scientific production
12.1 Major publications
- 1 inproceedingsDatabase Benchmarking for Supporting Real-Time Interactive Querying of Large Data.SIGMOD '20 - International Conference on Management of DataProceedings of the 2020 ACM SIGMOD International Conference on Management of DataPortland, OR, United StatesACMJune 2020, 1571-1587HALDOI
- 2 inproceedingsOpen Questions about the Visualization of Sociodemographic Data.IEEE VIS: Visualization & Visual Analytics Conference - Workshop on Visualization for Social Good (VIS4Good)VIS4Good 2023 - IEEE Workshop on Visualization for Social GoodMelbourne, AustraliaOctober 2023HALDOIback to text
- 3 articleBeauVis: A Validated Scale for Measuring the Aesthetic Pleasure of Visual Representations.IEEE Transactions on Visualization and Computer Graphics291January 2023, 363–373HALDOIback to text
- 4 bookMobile Data Visualization.0Chapman and Hall/CRCDecember 2021, 346HALDOIback to text
- 5 articleStudying Early Decision Making with Progressive Bar Charts.IEEE Transactions on Visualization and Computer Graphics291January 2023, 407-417HALDOIback to textback to text
- 6 articleScalability in Visualization.IEEE Transactions on Visualization and Computer GraphicsDecember 2022HALDOI
- 7 articlePerception! Immersion! Empowerment! Superpowers as Inspiration for Visualization.IEEE Transactions on Visualization and Computer Graphics281January 2022, 22-32HALDOI
- 8 articleVisualization in Motion: A Research Agenda and Two Evaluations.IEEE Transactions on Visualization and Computer Graphics2810October 2022, 3546-3562HALDOIback to text
12.2 Publications of the year
International journals
International peer-reviewed conferences
Scientific book chapters
Doctoral dissertations and habilitation theses
Reports & preprints
Other scientific publications
12.3 Cited publications
- 37 inproceedingsKnowing when You'Re Wrong: Building Fast and Reliable Approximate Query Processing Systems.Proceedings of the 2014 ACM SIGMOD International Conference on Management of DataSIGMOD '14New York, NY, USASnowbird, Utah, USAACM2014, 481--492URL: http://doi.acm.org/10.1145/2588555.2593667DOIback to text
- 38 articleSteering the Craft: UI Elements and Visualizations for Supporting Progressive Visual Analytics.Computer Graphics Forum363June 2017, 491--502HALDOIback to text
- 39 articleGeneaQuilts: A System for Exploring Large Genealogies.IEEE Transactions on Visualization and Computer Graphics166October 2010, 1073-1081HALDOIback to text
- 40 miscSituated Visualization in Motion for Video Games.PosterJune 2022HALDOIback to text
- 41 inproceedingsVisualization in Motion in Video Games for Different Types of Data.Journée Visu 2022Bordeaux, FranceJune 2022HALback to text
- 42 bookS. K.Stuart K. CardJ. D.Jock D. MackinlayB.Ben ShneidermanReadings in information visualization: using vision to think.San Francisco, CA, USAMorgan Kaufmann Publishers Inc.1999back to text
- 43 unpublishedNot As Easy As You Think-Experiences and Lessons Learnt from Creating a Visualization Image Typology.October 2022, working paper or preprintHALDOIback to text
- 44 articlePyramid-based Scatterplots Sampling for Progressive and Streaming Data Visualization.IEEE Transactions on Visualization and Computer Graphics281January 2022, 593-603HALDOIback to text
- 45 articleSix Methods for Transforming Layered Hypergraphs to Apply Layered Graph Layout Algorithms.Computer Graphics Forum413June 2022, 1467-8659HALDOIback to text
- 46 articleProgressive Data Analysis and Visualization (Dagstuhl Seminar 18411).Dagstuhl Reports8102018, 1--32URL: https://doi.org/10.4231/DagRep.8.1.1DOIback to text
- 47 unpublishedProgressive Analytics: A Computation Paradigm for Exploratory Data Analysis.July 2016, https://arxiv.org/abs/1607.05162 - working paper or preprintHALback to text
- 48 bookData stream management: processing high-speed data streams.Springer2016back to text
- 49 bookHandbook of sequential analysis.CRC Press1991back to text
- 50 inproceedingsPreferences and Effectiveness of Sleep Data Visualizations for Smartwatches and Fitness Bands.CHI 2022 - Conference on Human Factors in Computing SystemsNew Orleans, LA, United StatesApril 2022HALDOIback to text
- 51 miscContext Specific Visualizations on Smartwatches.PosterJune 2022HALDOIback to text
- 52 inproceedingsReflections on Visualization in Motion for Fitness Trackers.MobileHCI 2022 Workshop on New Trends in HCI and SportsVancouver, CanadaSeptember 2022HALback to text
- 53 phdthesisPhysical and Tangible Information Visualization.Université Paris Sud-Paris XI2014back to text
- 54 articlePANENE: A Progressive Algorithm for Indexing and Querying Approximate k-Nearest Neighbors.IEEE Transactions on Visualization and Computer Graphics262February 2020, 1347-1360HALDOIback to text
- 55 articleA Declarative Rendering Model for Multiclass Density Maps.IEEE Transactions on Visualization and Computer Graphics251January 2019, 470-480HALDOIback to text
- 56 miscSciPy: Open source scientific tools for Python.[Online; accessed <today>]2001, URL: http://www.scipy.org/back to text
- 57 bookThinking, Fast and Slow.Farrar, Straus and Giroux2011back to text
- 58 articleVIS Author Profiles: Interactive Descriptions of Publication Records Combining Text and Visualization.IEEE Transactions on Visualization and Computer Graphics251January 2019, 152-161DOIback to text
- 59 articleDimSUM: Dimension and Scale Unifying Maps for Visual Abstraction of DNA Origami Structures.Computer Graphics Forum373June 2018, 403-413HALDOIback to text
- 60 articleMultiscale Visualization and Scale-Adaptive Modification of DNA Nanostructures.IEEE Transactions on Visualization and Computer Graphics241January 2018, 1014--1024HALDOIback to text
- 61 articleMultiscale Molecular Visualization.Journal of Molecular Biology4316March 2019, 1049--1070HALDOIback to text
- 62 inproceedingsResponse Time in Man-computer Conversational Transactions.Proc. of the Fall Joint Computer Conference, Part ISan Francisco, CaliforniaACM1968, 267--277URL: http://doi.acm.org/10.1145/1476589.1476628DOIback to textback to text
- 63 articleIntegrating Prior Knowledge in Mixed Initiative Social Network Clustering.IEEE Transactions on Visualization and Computer Graphics272February 2021, 1775 - 1785HALDOIback to text
- 64 articleScalability in Visualization.IEEE Transactions on Visualization and Computer GraphicsDecember 2022HALDOIback to text
- 65 inproceedingsUnderstanding the Use of The Vistorian: Complementing Logs with Context Mini-Questionnaires.Visualization for the Digital HumanitiesPhoenix, United StatesOctober 2017HALback to text
- 66 articleResponse Time and Display Rate in Human Performance with Computers.ACM Comput. Surv.163September 1984, 265--285URL: http://doi.acm.org/10.1145/2514.2517DOIback to text
- 67 bookInformation Visualization.Boston, MA, USAAddison-Wesley Publishing Company2000back to text
- 68 miscTableau Software.Last accessed Feb 20182018, URL: http://www.tableau.comback to text
- 69 manualR: A Language and Environment for Statistical Computing.R Foundation for Statistical ComputingVienna, Austria2019, URL: https://www.R-project.orgback to text
- 70 articleProgressive Data Science: Potential and Challenges.CoRRabs/1812.080322018, URL: http://arxiv.org/abs/1812.08032back to text
- 71 articleAnalyzing Dynamic Hypergraphs with Parallel Aggregated Ordered Hypergraph Visualization.IEEE Transactions on Visualization and Computer Graphics271January 2021, 1-13HALDOIback to textback to text
- 72 incollectionVisual Abstraction.Foundations of Data VisualizationSpringer International PublishingAugust 2020, 15--37HALDOIback to text
- 73 articlePondering the Concept of Abstraction in (Illustrative) Visualization.IEEE Transactions on Visualization and Computer Graphics249September 2018, 2573--2588HALDOIback to text
- 74 inproceedingsA Vision of Bringing Immersive Visualization to Scientific Workflows.Proceedings of the Conference on Human Factors in Computing Systems (CHI) - Workshop on Interaction Design & Prototyping for Immersive AnalyticsGlasgow, United KingdomMay 2019HALback to text
- 75 bookInformation Visualization -- Perception for Design.Morgan Kaufmann Series in Interactive TechnologiesSan Francisco, CA, USAMorgan Kaufmann Publishers2000back to textback to text
- 76 articleEmbedded Data Representations.IEEE Transactions on Visualization and Computer Graphics2017, 461 - 470HALDOIback to text
- 77 inproceedingsSituated Visualization in Motion for Swimming.Journée Visu 2022Bordeaux, FranceJune 2022HALback to text
- 78 articleVisualization in Motion: A Research Agenda and Two Evaluations.IEEE Transactions on Visualization and Computer Graphics2810October 2022, 3546-3562HALDOIback to text
- 79 articleIllustrative Molecular Visualization with Continuous Abstraction.Computer Graphics Forum303May 2011, 683--690URL: https://tobias.isenberg.cc/VideosAndDemos/Zwan2011IMVDOIback to text